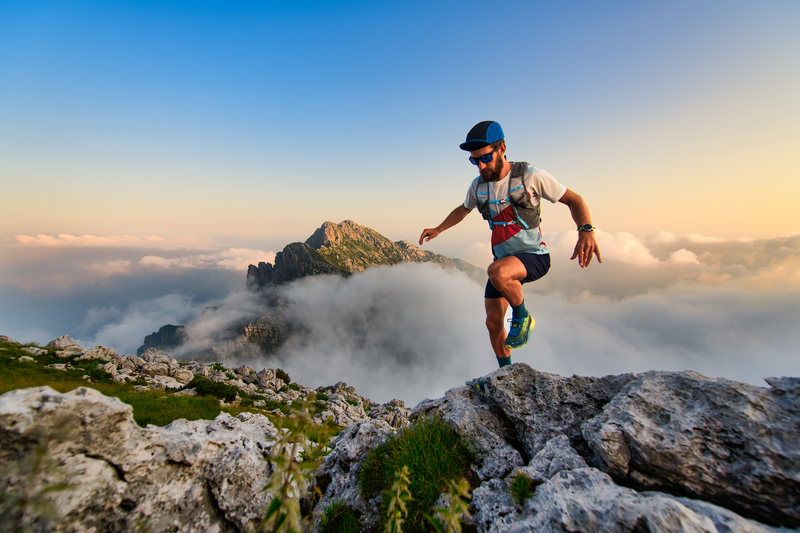
95% of researchers rate our articles as excellent or good
Learn more about the work of our research integrity team to safeguard the quality of each article we publish.
Find out more
ORIGINAL RESEARCH article
Front. Ecol. Evol. , 28 April 2022
Sec. Environmental Informatics and Remote Sensing
Volume 10 - 2022 | https://doi.org/10.3389/fevo.2022.878375
This article is part of the Research Topic Earth Observation Systems for the Urban Environment and Climate Change View all 5 articles
Rapid urban land use and land cover changes have become a major environmental issue because of their ecological effects, including loss of green space and urban heat islands. Effective monitoring and management techniques are required. The Saudi Arabian twin city of Abha-Khamis Mushyet was selected as a case study for this research. As a result, the current study aimed to statistically and spatially investigate the relationship between land surface temperature (LST) and land use land cover based urban biophysical parameters such as normalized difference built-up index (NDBI), normalized difference vegetation index (NDVI), and normalized difference water index (NDWI). This study used random forest (RF) to classify LULC in 1990, 2000, and 2018. We also validated the LULC maps in a novel way. Using mono window algorithm techniques, we extracted LST for three periods. The dynamics of LULC, LST, and biophysical parameters were investigated using standard statistical graphs such as the heat map and the Sankey diagram. The correlation coefficient and the global bivariate Moran’ I approach were used to determine the association between LST and urban biophysical parameters. The relationship was then established in greater detail by categorizing the entire pixel into percentile classes and employing parallel coordinate plots. Finally, the association was built using GeoDA software and a conditional map. The LULC maps revealed a 334.4 percent increase in urban areas between 1990 and 2018. The built-up region is the largest stable LULC, with an 83.6 percent transitional probability matrix between 1990 and 2018. While 17.9%, 21.8%, 12.4%, and 10.5% of agricultural land, scrubland, exposed rocks, and water bodies were converted to built-up areas, respectively. The LST has increased rapidly over time because of LULC changes. The link between LST and urban biophysical parameters revealed that NDBI had a positive relationship, whereas NDWI and NDVI had a negative relationship. As a result, this study could be very important because it could help decision makers figure out how to lessen the effects of urban heat islands because of changes in LULC.
One of the most obvious problems facing the world’s cities is rising urban temperatures because of land use and land cover (LULC) changes (Imran et al., 2021). During the past several decades, the fast growth of built-up surfaces at the cost of vegetation cover, croplands, and other natural pervious surfaces is to blame for the increasing temperature trend across urbanized landscapes (Pal and Ziaul, 2017; Naikoo et al., 2020). The fast growth of the urban LULC pattern alters the thermal characteristics of the land surface (Trlica et al., 2017). Built-up surfaces have a more considerable heat observing capacity and a lower emissivity than natural pervious surfaces because their thermal properties differ from natural pervious surfaces (Dimoudi et al., 2014). Impervious built-up surfaces store heat for extended periods and slowly release it because of their poor emissivity and greater heat observing capacity (Zhao et al., 2020), which results in the increasing land surface temperature (LST) as well as the formation of urban heat island (UHI) phenomenon (Abulibdeh, 2021). Besides this, the rising LST in cities is subject to various other factors such as population density (Mallick and Rahman, 2012), topographic conditions (Bindajam et al., 2020), prevailing winds (Zhou et al., 2012), surface biophysical composition (Shahfahad et al., 2020), and neighborhood environment (Zhou et al., 2018; Qiao et al., 2020).
The LST obtained from thermal satellite datasets are reliable and repeatable measurements of the Earth’s surface, allowing researchers to investigate the urban thermal environment at a variety of spatial, i.e., from local to global and as well as temporal scales, i.e., diurnal, seasonal, and inter-annual (AlQadhi et al., 2021; Bindajam et al., 2021; Shahfahad et al., 2021; Naikoo et al., 2022). Because the LST is sensitive to various land surface characteristics, it may be used to extract data on multiple LULC types (Sinha et al., 2015). The primary driver of LULC changes is urbanization, and as a result, a continuous increase of LST might disrupt the ambient environment for humans and other ecosystem members (Pal and Ziaul, 2017). Therefore, many studies have been done to analyze the LST variation because of the LU/LC pattern changes in the urban areas (Fu and Weng, 2016; Mathew et al., 2016; Gohain et al., 2021; Naikoo et al., 2022).
Previous research reported that natural land cover types such as water bodies, wetlands and vegetated surfaces have helped reduce the LST more than artificial built-up surfaces because of the cooling effect (Wentz et al., 2014; Trlica et al., 2017; Nurwanda and Honjo, 2020; Allen et al., 2021; Sussman et al., 2021). Furthermore, a few research findings showed that the size of vegetation patches, water bodies, and built-up surfaces also have varied effects on the LST. For example, Gioia et al. (2014) found that the size of the vegetation patch had a direct influence on lowering LST on the regional scale and that green areas with dense vegetation tend to lower LST on the local scale. Song et al. (2020) examined the influence of building density on LST and discovered that low- and high-density built-up regions had varied LST, although being subject to the climatic conditions and soil composition. For instance, Shahfahad et al. (2021) investigated the relationship between surface biophysical characteristics and LST in many cities from various climatic zones. They found coastal cities had a lesser link between LST and vegetation cover, but inland cities have a more significant association.
Many methodologies and strategies have been used to analyze LST and its relationships to the LULC pattern in different cities worldwide. Various approaches have been adopted to quantify LST and thermal climate variation because of LULC changes in the urban areas (Sobrino et al., 2010; Jimenez-Munoz et al., 2014; Mallick et al., 2020). For instance, Zawadzka et al. (2021) applied class-level landscape metrics to examine the thermal properties of the urban LULC pattern. Similarly, a few studies applied urban thermal field variation index (UTFVI) and urban hotspots (UHS) models for the quantification and modeling of changes in the urban thermal environment (Guha et al., 2018; Shahfahad et al., 2021). In addition, a few studies also applied local indicators of spatial autocorrelation (LISA) cluster (Das Majumdar and Biswas, 2016), temperature vegetation index (TVX) (Jiang and Tian, 2010), InVEST (Zawadzka et al., 2021), etc., for the quantification and modeling of LST change regarding LULC change. The main advantage of the LISA model is that it can quantify the spatial pattern of LST and analyze the changes in it during a period (Das Majumdar and Biswas, 2016). Thus, we used the LISA cluster technique to analyze and quantify the change in LST owing to LULC modifications in this research.
Urbanization has been changed a variety of physical and biological characteristics of the urban landscape, including vegetation cover, water bodies, soil properties, and micro (Kuang et al., 2015; Guha et al., 2018). Understanding the effect of urbanization on the urban environment is essential because sustainable urban expansion can only be realized if the link between urbanization and its environmental impact is well understood (Zhao et al., 2017). This work aims to measure and evaluate the spatio-temporal patterns of urbanization and urban biophysical components in Abha city from this viewpoint. Biophysical components are indicators that may trace the human influence on a particular environment in general (Duan et al., 2017; Tran et al., 2017; Guha et al., 2018). According to Oke and Cleugh (1987), fast urbanization may modify the urban biophysical components, substantially affecting the Earth-Atmospheric energy process at the micro-scale. Because of extraordinary changes in land-use land cover (LULC), the mean average temperature at the local level is steadily rising (Roy et al., 2020).
The main features of Indian urbanization are unpredicted and uncontrolled urban growth, increased impervious area, and haphazard development, all of which result in significant reductions in agricultural land, vegetation cover, wetland, and other natural water bodies, as well as increased pollution, slum development, and various social and economic problems (Xie et al., 2013). As a result, contemporary remote sensing methods are an excellent tool for more precise measurement and monitoring of urban land cover types (Xie et al., 2013; Sharma et al., 2015). With automated and semi-automatic procedures, detailed and improved information may be extracted from remote sensing data, and this information, i.e., images, can be supplied from the past. As a result, the urban landscape’s dynamism may be readily observed (Jain et al., 2020). Landsat satellite imageries (such as MSS, TM, ETM+, OLI) are widely used as the input database for various researches to extract the urban built-up area and estimate other various biophysical elements (Kaplan et al., 2018). Researchers use remote sensing-based indices like the Normalized Difference Built-up Index (NDBI), the Normalized Difference Vegetation Index (NDVI), the Normalized Difference Water Index (NDWI), the Normalized Difference Bareness Index (NDBal), and the Modified Normalized Difference Water Index (MNDWI) to track urban expansion and its effects (Guha et al., 2018; Mallick and Rudra, 2021).
Land surface temperature is a critical component of the semi-arid urban mountain area because it reflects changes in the environment, the terrain, and the physical processes that underpin those (Bindajam et al., 2020; AlQadhi et al., 2021). Additionally, the LULC changes in cities in countries such as Saudi Arabia are rapid, owing to the high population increase in metropolitan areas (Bindajam et al., 2021). Consequently, the cities of arid and semi-arid countries like Saudi Arabia have been witnessing the warmer climate than their surroundings (Mallick et al., 2021).
Several studies have been conducted examining the relationship between greenness (NDVI), impervious land (NDBI), and changes in land use and land cover with LST (Srikanta et al., 2018; Firozjaei et al., 2019; Ghosh et al., 2019; Roy et al., 2020). Determining the link between the different biophysical components and LULC variation is essential. However, using a hybrid way of remote sensing technology and statistical calculations, the current work attempts to evaluate and analyze the spatiotemporal patterns of LULC, dynamics of biophysical composition, and LST using machine learning and advanced geo-statistical techniques. There is much evidence of remote sensing-based research on the urban biophysical composition and urban heat island phenomena (UHI) (Zhu et al., 2018; Yao et al., 2020). As a result, the research emphasizes the spatiotemporal dynamics of urban land use and the biophysical parameters connected with them, such as plant cover, urban water bodies, soil moisture, built-up area, and changes in land surface temperature.
The explicit goals of the present study are to
(a) Map the land use land cover and quantify the LULC changes at a temporal scale using random forest and Markovian transition matrix,
(b) Determine the association between Land Surface Temperature (LST) and urban biophysical components and their spatiotemporal patterns using advanced statistical techniques.
As a result, the current research could be necessary for long-term urban planning.
Abha is a city in Saudi Arabia’s southwestern Asir province. This city’s topography is rugged and heterogeneous, with elevations ranging from 1,950 to 2,982 m above sea level (Figure 1). A cold and semi-arid climate characterizes the city. The average annual precipitation is 355 mm and occurs mainly from June to October. The average annual minimum and high temperatures were 19.3°C and 29.70°C, respectively. This city faces extreme difficulties due to land degradation caused by manufacturing activities, higher slopes, weaker geological formations, and unpredictable rainfall affecting ESs. Plant communities are thriving because of climate change and diverse topography. This city is one of the Asir Mountains’ most diverse florist regions. The urban hills are this region’s most appealing tourism destination, with the most diverse flora and fauna.
For the present study, Landsat images for 1990, 2000, and 2018 were obtained from the website of the United States Geological Survey.1 We extracted urban biophysical characteristics from Landsat Thematic Mapper (TM) images for 1990 and 2000, as well as Operational Land Imager-8 (OLI-8) images for 2018 (path/row: 167/047 and spatial resolution: 30 m). The LST was also retrieved using the Thermal Infrared Sensor (TIRS). The United States Geological Survey (see text footnote 1) provided multi-temporal images.
The Random Forest method [developed by Breiman (2001)] is generated by creating classification and regression trees-CART. The RF technique is an ensemble learning approach extensively used to classify land cover using multispectral and hyperspectral satellite sensor images. The RF generates a variety of trees based on random bootstrapped training database patterns. Above the bootstrapping strategy, this method uses random binary trees to build a training subset. A random selection of the training information is used to generate the model from the original database; out-of-the-bag (OOB) data is defined as data that is not used. Two parameters must be provided in order to implement the RF (Xu et al., 2012): the number of trees (ntree) and the number of features in each split (mtry). Many research studies obtained excellent results with the default values (Genuer et al., 2017). However, the vast number of trees will yield a steady outcome of varying relevance, according to Liaw and Wiener (2002).
Furthermore, according to Breiman (2001), utilizing more than the needed number of trees may be redundant, although it does not impact the model. Furthermore, Feng et al. (2019) claimed that RF could get accurate findings with ntree = 200. Many research employs the default value for the mtry parameter, which is mtry = p, where p is the number of predictor variables (Feng et al., 2019). However, in this work, we implemented the RF model with ntree = 200.
For 1990, 2000, and 2018, the RF model divided the multi-temporal Landsat images into eight classes. The validation was done using the Kappa coefficient after the temporal Landsat photos were classified. For the 2018 LULC map, ground truth samples were obtained from the field. Samples for validating the LULC maps for 1990 and 2000 were taken from various bands of specific Landsat images. The data was then retrieved from the LULC maps using the sample points as a guide. The accuracy rating of the LULC maps was then computed using the kappa coefficient.
We use a Markovian transition matrix to calculate the dynamics of LULC for various years after validation. It indicates the estimated number of pixels transformed from one LULC type to another during the selected time. The probabilities are shown graphically in the matrix below:
where p represents the state of probability of transition from i to j.
The transition matrix was computed for three times, such as 1990–2000, 2000–2018, and 1990–2018 using heat map visualization.
The consequences of urbanization could be seen in the variations in surface temperature. For the LST calculation, we utilized Landsat imageries that had been corrected. Following Yu et al. (2014), LST maps were created using the mono window approach with the thermal infrared band (band 10 of the OLI sensor and band 6 of the TM sensor; both of which have a spatial resolution of 30 m) of the Landsat satellite image (1990 and 2000). The same satellite images used to create the land cover were also utilized to create the LST each year. In LST calculation, band 10 of the OLI sensor was favored over band 11 due to anomalies in LST recovery utilizing band 11 (Yu et al., 2014). The digitized number of pixels in each image was transformed to top-of-atmosphere (TOA) radiance before being converted to brightness temperature. These conversions were made using calibration constants from each image’s metadata file. Finally, using the NDVI, the brightness temperature image was adjusted for land surface emissivity (Ghosh et al., 2019; Mallick et al., 2020).
The NDBI is a crucial indicator for the built-up extract area, including impervious areas (Chen et al., 2006). Using the reflectance of Middle Infrared (MIR) and Near Infrared (NIR) light, it is effective in extracting built-up area from remote sensing data (Zha et al., 2010). Researchers use it to identify impermeable surfaces, and it is a frequent and helpful approach (Zhang et al., 2009; Zha et al., 2010). The equation of NDBI is
MRI stands for medium infrared band (band 5 for TM and band 6 for OLI-8), and NIR stands for the near-infrared band (band 4 for TM and band 5 for OLI-8). The NDBI value ranges from 1 to + 1, with values closer to 0 indicating vegetation cover, negative values indicating water bodies, and positive values indicating built-up areas (Zha et al., 2010).
The loss of plant cover can readily be understood as the constructed area grows, and NDVI is a good approach for determining the greenness of any region (Sharma et al., 2015). It is an essential variable in studying urban expansion and microclimatic phenomena in cities (Chen et al., 2006). Townshend and Justice (2007) devised a technique for NDVI extraction based on NIR and red band reflectance (R). It is written as:
where NIR stands for Landsat TM band 4 and OLI-8 band 5, and R stands for TM band 3 and OLI8 band 4. The NDVI value ranges between 1 and 1. Large NDVI values imply plant cover, tiny positive values imply built-up areas or barren terrain, and negative values, i.e., values near to zero, imply water bodies.
Normalized Difference Water Index is used to estimate the amount of liquid water in vegetation since NDWI is directly related to the amount of water in the plant (Chen et al., 2006). McFeeters (2007) introduced the NDWI equation, which may be stated as utilizing the reflectance of the Green (G) and NIR bands. The equation of NDWI is
For TM, band 2 is green, and band 4 is NIR, but for OLI-8, band 3 and band 5 are green and NIR, respectively. The NDWI value ranges from −1 to + 1. In fact, NDWI is more important than NDVI since it is less affected by air scattering.
In the present study, several statistical techniques have been applied to assess the relationship between LST and urban biophysical parameters.
We converted the 2018 LST raster into a point-based vector file, yielding almost 1.6 million points in our research. We then retrieved the values of LST and biophysical parameters (NDWI, NDBI, and NDVI) from 1990 to 2018 based on the point file. We loaded the vector file into the GeoDA program. Then, using Pearson’s correlation coefficient, a correlation matrix was used to investigate the relationship between LST and urban biophysical characteristics. As a result, it can analyze the link between LST and biophysical parameters across the research region.
We employed bivariate Moran’s I to investigate spatial dispersion between LST and urban biophysical parameters. The global bivariate Moran’s I is the type of bivariate Morain’ I, applied in this study. Moran’s I bivariate global analyses if and to what degree there are spatial connections between LST and urban biophysical characteristics throughout the entire region (Anselin et al., 2014). The following are the calculating formulae we used:
Ieu is the global bivariate Moran’s I for LST and biophysical parameters. The total number of spatial units is denoted by the letter N. Wij is an N-by-N spatial weight matrix created using queen contiguity weight with the first order of neighbor in a 3 3 matrix (Anselin et al., 2014) to measure the spatial correlation between the ith and jth spatial unit. For the ith spatial unit, is the standardized value of LST derived using Eq. (1). For the jth geographical unit, is the standardized value of each urban biophysical parameter computed using Eq. (1).
Ieu has a range of values from 1 to 1. A positive Ieu value shows that the LST and biophysical parameters have a positive spatial correlation, implying that a pixel with a high LST value is likely to be surrounded by pixels with high values of urban biophysical characteristics. A negative Ieu indicates a negative spatial correlation, showing that pixels with high LST values are more likely to be surrounded by pixels with low values of urban biophysical characteristics. The better the geographical connection between LST and biophysical parameters, the higher the absolute value of Ieu. Permutation tests are performed to determine the statistical significance of bivariate Moran’s I; 999 permutations were utilized in this research (Anselin, 1995).
A Parallel Coordinates Plot (PCP) is a multivariate numerical data analysis visualization approach. It lets data analysts compare many quantitative variables to seek patterns and linkages. It is useful for comparing many numerical variables simultaneously, especially when the magnitudes (scales) and units of measurement are different. In multidimensional datasets, the goal is to uncover patterns, similarities, clusters, and positive, negative, or no particular associations. Each variable has its axis in a Parallel Coordinates Plot, and all the axes are parallel to each other. Because each variable uses a distinct unit of measurement, each axis may have a separate scale, or all the axes can be normalized to keep all the scales consistent. The data is represented as lines that link all the axes. This indicates that each line comprises a group of points on each axis that have been linked together.
Three urban biophysical measures, such as NDWI, NDVI, NDBI, and LST, were analyzed to identify a link and a data pattern utilizing PCP in this work. The data has been overlaid with the LST data pattern.
As explained in detail in Carr and Pickle (2010), this kind of map is also known by the names “conditional choropleth map” and “micro-map matrix.” As defined by the conditioning variables on the horizontal and vertical axes, a micro-map matrix is a matrix of maps that corresponds to a subset of the observations. Each map depicts the geographical distribution of the variable of interest, but only for observations that fall into the conditional variables’ categories shown on the corresponding map.
One of the primary goals of this conditioning procedure is to identify any possible interactions between the conditioning factors and the issue of interest in the concern. The reference point (also known as the null hypothesis) assumes no interaction. If this is the case, then the patterns on all the micro-maps should be roughly the same. If there is an interaction between the variables of interest and the conditioning variables, then high or low values of the variable of interest would be regularly correlated with specific categories of the conditioning variables.
Random forest was used to classify the Abha-Khamis Mushyet twin city region’s LU/LC for 1990, 2000, and 2018. Built-up areas, water bodies, dense vegetation, sparse vegetation, agricultural land, scrubland, bare soil, and exposed rock were all identified on the LULC maps (Supplementary Figure 1). The area covered by LULC maps from 1990 to 2018 was calculated. According to the 1990 LULC map, scrubland covers the most area of the study area (47,730 hectares), followed by exposed rock (45,061 hectares), bare soil (17,217 hectares), sparse vegetation (8,530 hectares), and built-up (6,246 hectares), while dense vegetation covers the least area (128 hectares), followed by water bodies (136 hectares), and agricultural land (2,821 hectares). With the LULC of 2000, the exposed rock covered the most area of the total study area (52,973 hectares), followed by scrubland (36,136 hectares), bare soil (20,302 hectares), and built-up (10,520 hectares), while the water bodies covered the least area (113 hectares), followed by dense vegetation (267 hectares), agricultural land (2,245 hectares), and sparse vegetation (5,312 hectares). Furthermore, the exposed rock covered 45,808 hectares for the 2018 LULC map, followed by scrubland (33,203 hectares), built-up area (27,145 hectares), and bare soil (11,394 hectares), while water bodies covered 52 hectares, followed by dense vegetation (944 hectares), agricultural land (1,775 hectares), and sparse vegetation (7,547 hectares). As a result, the built-up area has increased dramatically in the last 28 years.
After three classification periods, the LULC maps are subjected to accuracy assessment. As a result, during 2018, we gathered field data and Google Earth imagery. In contrast, the field survey and Google Earth image for 1990 and 2000 are not accessible. We used proxy data to validate the LULC maps for these years. We gathered ground truth using layer stacked multispectral images (seven bands of Landsat 4-5TM) for 1990 and 2000. Then, we acquired ground truth from each band for both years except for band 6 (the thermal band). We verified the LULC maps from 1990 to 2000 in this way. We also gathered samples from multispectral images and six bands for the 2018 LULC map to keep the analysis consistent. According to the accuracy evaluation results, the LULC of 2018 achieved an overall accuracy of 87.2 percent from the ground truth, including a field survey and a Google Earth image. While the overall accuracy for the layer stack multispectral band was 86.4 percent, the overall accuracy for bands 2 (blue), 3 (green), 4 (red), 5 (near-infrared), 6 (shortwave infrared-I), and 7 (shortwave infrared-II) were 85.3 percent, 84.71 percent, 86.63 percent, 85.1 percent, 84 percent, and 84.8 percent. The validation of the LULC map for the year 2000 shows that the overall accuracy for the samples of the multispectral band was 84 percent, while the accuracy for the samples of band 1, band 2, band 3, band 4, band 5, and band 7 were 82.5 percent, 82.8 percent, 83.4 percent, 83.64 percent, 81.6 percent, and 80.4 percent, respectively. The overall accuracy of the LULC map for the year 1990 was 81.6 percent for the samples of the multispectral band, while the overall accuracy of band 1, band 2, band 3, band 4, band 5, and band 7 was 80.5 percent, 81.2 percent, 79.5 percent, 80.5 percent, 79.2 percent, and 81 percent, respectively.
A Markovian transitional probability matrix was used to analyze the LULC change trend quantitatively. It reflects the probability that each cell in a land-use category will be changed into another land-use type. Using Equation 1, the current study generated the transitional probability matrices for 1990–2000, 2000–2018, and 1990–2018. A heatmap depicted the LULC transition throughout multiple periods (Figure 2). Figure 2A depicts a heat map of the transitional probability matrix between the LULCs of 1990 and 2000. The hues yellow to light orange and deep brown on the heat map depict the chance of remaining unaltered over time. For example, exposed rock (represented by the deep brown color) has 85.8 percent likelihood, bare soil (represented by the deep brown color) has 81 percent probability, and built-up (represented by the orange color) has 74.7 percent probability. Agricultural land (shown in yellow) has a 34.7 percent likelihood.
Figure 2. Transition probabilities of different LULC classes between the period of (A) 1990–2000, (B) 2000–2018, and (C) 1990–2018.
A score of 0 indicates that the land has not been moved to a different land-use type. Figure 3A shows that water bodies have the most zero values, showing that the transformation from water bodies to other land uses nil, followed by dense vegetation (one zero), bare soil (one zero), agricultural land (three close to zero values), and built-up, scrubland, and exposed rock (no zero values). The result shows that built-up areas, scrubland, and exposed rocks are highly vulnerable to conversion into other land uses throughout this period. Water (2.2 percent), scrubland (4.8 percent), sparse vegetation (4.6 percent), exposed rock (3 percent), thick vegetation (0.2 percent), bare soil (9.6 percent), and agricultural land are all possibilities for the built-up region (5 percent). The prospect of converting from water bodies to other land uses was limited throughout this time, with just a 0.3 percent chance of being converted to heavy vegetation. Dense vegetation has a meager chance of being converted to other land uses. Sparse vegetation has a 62 percent chance of converting into dense vegetation, but it has little chance of transitioning into other land uses. Agricultural land has a meager chance (less than 10%) of being converted to another land use over this period.
Figure 3. The transition during the period of 1990–2018 for (A) LST, and urban biophysical parameters, like (B) NDBI, (C) NDVI, and (D) NDWI.
Similarly, except for agricultural land (16.8 percent), barren land has a slim possibility of being changed into another land use (10 percent). Scrubland has a high possibility of being changed into other land uses, such as agricultural land (37.1 percent), sparse vegetation (30.5%), and water bodies (18.5%), whereas other land uses have a risk of conversion of less than 10%. Similarly, exposed rock has a 24.3 percent chance of transforming into scrubland with little flora (17.7 percent).
Figure 2B depicts a heat map of the transitional probability matrix between the LULCs of 2000 and 2018. The heatmap’s varied color sheds represent the strength of the odds of remaining unchanged throughout the period 2000–2018 (blue color band to brown color band). According to the findings, built-up regions had the highest likelihood of remaining unmodified to other land uses (83.7 percent), followed by exposed rock (75.5 percent), scant vegetation (59.2 percent), and scrubland (53.7 percent). In comparison, agricultural land had the lowest chance of remaining constant (20.7 percent), followed by thick vegetation (37.5 percent), bare soil (43.1 percent), and bodies of water (43.1 percent) (43.8 percent). Similarly, un-convertible land uses, such as water bodies, thick vegetation, and bare soil, cannot be converted to other land uses and have a very low possibility of being converted to other land uses since the probability for these land uses approaching zero. Sparse vegetation and scrubland are more likely to be converted to agricultural land (17.6%), thick vegetation (30.5%), agricultural land (33.3%), bare soil (32.1%), and sparse vegetation (32.1%) (19.1 percent).
Figure 2C depicts a heatmap of the long-term transitional probability matrix between the LULCs of 1990 and 2018. Built-up areas have the highest long-term probability of remaining unchanged to other land uses (83.7 percent), followed by exposed rock (75.1 percent), dense vegetation (53.4 percent), and sparse vegetation (47.8 percent). In contrast, agricultural land has the lowest long-term probability of remaining unchanged (15.3%), followed by water (35.1 percent), bare soil (43 percent), and scrubland (47.8 percent) (43 percent). For example, forty-five percent of water bodies, agricultural land, and bare soil are less likely to be changed to other land uses (less than 10 percent). Scrubland and sparsely vegetated areas are more likely to be converted to agricultural land or urban development.
The present study extracted the LST three times, such as 1990, 2000, and 2018 using the mono-window algorithm. Then, the LST models’ quality was cross-examined based on the field measurement. The temperature was collected from different land-use features, and then the temperature was obtained from the satellite measurement at the corresponding location. The results showed a very high correlation between satellite and ground measurement (r: 0.99 at the significance level of 0.001). The spatial and temporal distribution of LST in the Abha-Khamis Mushyet twin city area is shown in Supplementary Figure 2. When the figure was examined, it was shown that the LST had grown dramatically in the Abha-Khamis Mushyet twin city area between 1990 and 2018.
Figure 3A revealed that the LST was higher over built-up areas and also higher over bare soil and exposed rock surfaces. This is owing to the fact that heat is stored fast on exposed rocky surfaces and is released slowly from bare soil because of the high heat absorption capacity and low albedo of exposed rocky surfaces and bare soil, respectively (Trlica et al., 2017; Shahfahad et al., 2021). Abha-Khamis Mushyet twin city area observed significant levels of LST in 1990, particularly in the northern, north-central, and east-central regions of the region. LST was low to moderate in much of the western, southern, and northern sections in 1991, compared to the rest of the country. It was found that between 2000 and 2017, the LST has increased significantly, particularly in the north-eastern, eastern, and south-eastern areas of the region. The growing LST in the eastern and north-eastern sections of the Abha-Khamis Mushyet twin city area may be attributed to the large-scale conversion of natural land cover types, particularly scrublands and croplands, to built-up surfaces in the eastern and north-eastern parts of the region. During the period 1990–2017, the increase in LST in the Abha-Khamis Mushyet twin city region can be divided into two phases, namely, in 2000, the maximum increase in LST was observed in the north-eastern and northern parts of the region, and in 2017, the maximum increase in LST was observed in the eastern and southern parts of the region. In contrast to this, the region’s southwestern areas did not suffer a statistically significant rise in LST between 1990 and 2017. This is because of the dominance of the plant cover (both thick and sparse) in this study area.
In the Abha-Khamis Mushyet twin city region, three biophysical indicators were used to investigate the association between landscape pattern and LST: the NDWI, the NDBI, and the NDVI (Natural Diversity Index). According to the NDVI and NDWI analyses, the region’s plant density and water bodies had a minor but significant decline between 1990 and 2017 compared to the previous decade (Supplementary Figure 3). In accordance with the NDWI dynamics, the number of water bodies in the region has fallen dramatically between 1990 and 2017 (Figure 3D). Furthermore, the decline in the NDWI from 2000 to 2017 was larger than the decline from 1990 to 2000. High and low NDWI values were 0.476 and −0.59 in 1990, then dropping to 0.471 and −0.63 in 2000 and 0.407 and −0.69 in 2017. The high and low NDWI values in 1990 were 0.476 and −0.59. In the Abha-Khamis Mushyet twin city region, the northern and central portions have experienced the most significant decrease in NDWI, while the southern and eastern sections have experienced a minor increase in NDWI.
The NDVI, like the NDWI, shows a significant but modest change in the Abha-Khamis Mushyet twin city area between 1990 and 2017 (Supplementary Figure 4). It was found that between 1990 and 2017, the NDVI in the southwestern areas of the region was high, while the NDVI in the center and northern regions of the region was low. It is important to note that, despite the fact that the statistics of high and low NDVI show an increasing trend from 1990 to 2017, the spatial pattern of NDVI shows a decrease in the region’s vegetation density. As of 1990, the highest and lowest values for the NDVI were 0.646 and −0.34, respectively. Since then, the values have increased to 0.682 and −0.53 in 2000, 0.775 and −0.32 in 2017 (Figure 3C). When looking at the southern and southwestern portions of the area, the NDVI suggested a minor but significant decline in vegetation between 2000 and 2017.
While the NDWI and NDVI in the Abha-Khamis Mushyet twin city region suggest a substantial shift from 1990 to 2017, the NDBI in the same area reveals a considerable change from 1990 to 2017. In 1991, the NDBI was highest in the central and northern sections of the region, while it was lowest in the southern, eastern, and western parts of the region. The results of NDBI report a significant increase in built-up density in the southern and eastern sections of the Abha-Khamis Mushyet twin city region between 2000 and 2017. As reported by the results of NDBI, the peak and low NDBI in 1991 were 0.407 and −0.51, respectively. These values increased to 0.70 and −0.47 in 2000, before decreasing to 0.451 and −0.54, respectively, in 2018. However, the NDBI value decreased in 2018 does not mean that the built-up area has been decreased.
In contrast, the area coverage of the NDBI or built-up area has been increased. Nevertheless, the intensity has been decreased, which can be meant for the decreasing tendency of sky-scraper building construction and the increasing tendency of the horizontal expansion. Also, Figure 3B shows that the number of pixels of the built-up area was huge under the NDBI value of 0.01 rather than a higher value, which shows the construction of the new building in open spaces, where no built-up was there previously. This indicates the horizontal expansion of the urban area of the urbanization process.
This study examined the relationships between LST and urban biophysical variables, including NDBI, NDVI, and NDWI. As is widely known, the land cover change affects the NDBI, NDVI, and NDWI. We used biophysical parameters instead of LULC maps to get parameter intensity values that cannot be obtained from LULC maps. So we tried to highlight the connection in new and effective ways in the present work. So we applied sophisticated statistical techniques to study the LST-urban biophysical variables connection. This study examined the relationships between 1990, 2000, and 2018 using Pearson’s correlation approach (Figures 4A–C). It was discovered in 1990 that the correlation coefficient between the NDBI and LST is 0.276, meaning that urban areas have a higher effect on LST than rural areas.
Figure 4. The correlation coefficient analysis between LST and urban biophysical parameters for (A) 1990, (B) 2000, and (C) 2018.
On the other hand, LST exhibits a statistically significant negative association with NDVI and NDWI, with a 0.001 correlation coefficient. The correlation research of LST and urban biophysical parameters provides the same results as previous studies, consistent with previous findings. NDBI has a statistically negligible but statistically significant positive relationship with LST, which shows that urbanization affects LST. In urban regions, land use (e.g., forest clearing) appears to have a more substantial impact on LST, as shown by the statistically significant negative relationship between LST and NDVI and NDWI at the significance level of 0.001. This shows that the urban heat island in the research region has been increasing since 2000, but that it has increased significantly since last year. The global bivariate Moran’s I results showed significantly positive spatial correlations between LST and NDBI, i.e., urban areas (Moran’s I: 0.415) at a significance level of 0.01 for the year 1990 (Figure 5A). This means that, overall; urbanization increases the land surface temperature. In this case, the temperature rises not just because of the higher number of people and poor housing conditions but also because of heat generating infrastructure and transport networks that release heat for hours. Therefore, the urban heat island effects have been created. On the other hand, the LST has a strongly negative spatial correlation with NDVI, i.e., vegetation cover for 1990 (Moran’ I: −0.592) at a significance level of 0.01 (Figure 5B). In fact, the higher the vegetation cover, the less the surface temperature increases. This is because the dominant heat absorption of vegetation effectively reduces the temperature increase. Therefore, it is believed that the LST values were largely affected by the larger vegetation cover in 1990. In addition, we found a positive spatial correlation between LST and water bodies (NDWI) (Moran’s I: 0.542) at a significance level of 0.01 (Figure 5C). But this result is inverse to the hypothetical assumption that there should be a negative correlation between LST and NDWI as water bodies absorb the heat, which reduces the surface temperature. The present study area covers a negligible number of water bodies, while the study area comprises over 1.6 million pixels and is used for correlation. This could be the reason. However, this situation needs to be explained further as to why it happened.
Figure 5. Global bivariate Moran’ I spatial correlation between (A) LST-NDBI, (B) LST-NDVI, (C) LST-NDWI for 1990, (D) LST-NDBI, (E) LST-NDVI, (F) LST-NDWI for 2000, (G) LST-NDBI, (H) LST-NDVI, (I) LST-NDWI for the year 2018.
It has also been reported that identical findings have been obtained for different periods, such as 2000 and 2018. For 2000 and 2018, a positive spatial association was discovered between LST and urban areas (Moran’ I: 0.367 and 0.352) (Figures 5D,G). On the other hand, a negative correlation has been discovered between LST and vegetation cover (Moran’ I: −0.614 and −0.573), indicating that the two variables are negatively related (Figures 5E,H). The positive association between NDWI and LST, on the other hand, was discovered (Figures 5F,I). We need to go into further detail about what happened in order to understand. Therefore, rather than using an overall analysis, we employed a parallel coordinate map to describe how all data patterns relate to one another.
Because we discovered that the LST and NDWI showed a positive spatial correlation, we used the parallel coordinate plot to compare all data structures to one another (Figure 6). The parallel coordinate plot combines the study of the LST data pattern and urban biophysical factors into a single graph. The LST and three biophysical characteristics were displayed on four axes, with the LST or research of interest superimposed on top. The whole LST dataset (about 1.6 million pixels) has been categorized into percentile order, such as 1%, 1–10%, 10–50%, 50–90%, 90–99 percent, and >99 percent. For the year 1990, the percentile data has a distinct range of LST values, such as 1 percent data has ranged the LST between 20.35–29.64, followed by 1–10 percent (29.65–36.48), 10–50 percent (36.48–43.27), 50–90 percent (43.27–48.86), 90–99 percent (48.86–52.68), and >99 percent (52.68–57.65) (Figure 6A). This categorization has evolved throughout time. The link was then established using several sets of LST data and relevant urban biophysical data. The 1990 results suggest that up to the 50th percentile (LST ranges 20–43) has a favorable association with built-up regions. A modest negative link was discovered between higher LST (over 43) and urban areas. This scenario must also be discussed in more detail. Overall, there was a favorable link between the urban area and the LST. In the case of NDVI, all data patterns displayed a substantially negative connection with LST, with the exception of the LST range 36–43. In the case of NDWI, the LST range of 20.35–29.65 (<1 percentile) demonstrated a strong favorable connection with LST. Other data patterns have a negative correlation. The identical pattern of relationship has been found for the years 2000 and 2018 (Figures 6B,C). This unfavorable condition must be explained spatially as to why it occurred.
Figure 6. Parallel coordinate plot for establishing relationship between LST and urban biophysical parameters for the year (A) 1990, (B) 2000, and (C) 2018.
The preceding analysis discussed the relationship between LST and biophysical parameters using both overall and stratified datasets. We discovered that the overall dataset can correctly explore the relationship between NDBI and NDVI, whereas we obtained the opposite relationship between LST and NDWI. As a result, we employed stratified datasets to dig further into the link between LST and urban biophysical characteristics. We discovered that the link between LST, NDBI, and NDVI is true, except for a few datasets that produced inverted findings. While the relationship between LST and NDWI was correct, except for the data with a value range of 0–0.2, which showed a positive relationship. Then, we cross-checked with the field and discovered that the locations with NDWI values ranging from 0 to 0.2 are not really water bodies, but mud, sand, and bare soil around the water bodies that absorb heat and raise the temperature nearby. As a result, the association between LST and NDWI is illustrated. Furthermore, several issues with NDBI and NDVI exist, which may be explained using spatial analysis. As a result, we utilized a conditional map to describe the link between LST, NDBI, and NDVI for 1990, 2000, and 2018 (Figures 7–9), and we skipped NDWI for further study since it is already well defined.
There are nine maps in total in the conditional map, with the intersection of two biophysical components as independent variables (NDVI on the vertical axis and NDBI on the horizontal axis) and LST (which is superimposed on the map based on the hinge 1.5) being emphasized on the first two maps. GeoDa software is used to determine the contiguity and clustering patterns of components across space in this map-based decision support tool. Using a fuzzy membership function, conditional mapping displays how many variables of each component were geographically divided into distinct spatial zones, which aids in the standardization of elements. Figures 7–9 depict a statistically significant clustering of LST in locations with high NDBI and low NDVI, respectively. Thus, our data lend credence to the hypothesis that greater NDBI sites have higher LST, as well as exposed rock. Higher NDVI areas have lower LST because of the cooling process and heat absorption. Co-analysis of NDBI and NDVI findings can aid in the identification of the primary elements that contribute to the formation of LST hot spots in each geographic zone.
In this paper, we have explored the spatio-temporal dynamics of LULC and its relationship with the LST through the urban biophysical parameters using sophisticated statistical techniques. Most previous research has used all the pixels in the study area and applied regression to investigate the relationship between LST and urban biophysical parameters. This paper investigated the relationship between LST and biophysical parameters straightforwardly. For example, we used all the pixels for all the parameters for three periods to show the relationship using Pearson’s correlation coefficient technique and highly standard global bivariate Moran’ I techniques. However, the ties using all the data as a whole did not provide satisfactory results. Therefore, we stratified all the pixels using the percentile technique and a parallel coordinate plot to show the relationship between LST and each pixel containing urban biophysical parameters. Also, minor errors were observed for stratified-based analysis.
Consequently, we used a decision-making process-based conditional map based on urban biophysical parameters at a spatial scale. To the best of the author’s knowledge, this type of study has not been conducted so far. Therefore, our proposed research will be helpful for urban planning, the sustainable development of cities, and decision support systems.
Because of the physical importance of vegetation to the LST, the LST-NDVI relationship has been a contentious research issue (Guo et al., 2015; Wu et al., 2019). However, NDVI and LST have a significant negative association with a “temperature edge” (Figures 5–9). Li et al. (2011), who investigated the whole city of Shanghai, obtained a similar outcome. Surprisingly, we discovered that the LST-NDBI link had a “temperature edge,” with a correlation almost as strong as between LST and NDVI (Figures 7–9). Given the yearly fluctuation of NDVI, NDBI can be used as a supplement to LST investigations. Non-linearity and seasonal changes in the link between urban LST characteristics (i.e., LST NDVI, LST–NDBI) have been recorded. This makes quantifying LST fluctuations difficult, particularly in geographically varied environments like our research areas. Various models have been used in recent research to study the influence of biophysical factors on LST (Mathew et al., 2017; Liu et al., 2018; Weng et al., 2019). Recent research has often employed regression models with regional optimization, which is a significant restriction (Guo et al., 2015; Xiang et al., 2021). This work used global bivariate Moran’s I to analyze LST and biophysical parameter fluctuations in the temporal dimension at the pixel scale. In addition, we classified all the pixels in the research region and determined their relationships. Finally, a conditional map was utilized to illustrate the relationship based on urban biophysical factors spatially. The research provided new knowledge on the overall LST variations and LGI, i.e., urban parameters influencing LST variation, during 28 years periods. Also, the study intends to promote the application of remote sensing data in environmental governance, especially for urban hydro-meteorological adaptation.
In general, a heterogeneous spatiotemporal distribution of surface biophysical factors such as brightness, greenness, and wetness of the area could lead to a heterogeneous LST distribution (Yang et al., 2017; Sattari et al., 2018). This would be due to the fact that the incoming shortwave radiation, which is nearly constant at a single point in space, can be absorbed differently by different types of surface biophysical features (Fu and Weng, 2016; Guha et al., 2018; Peng et al., 2018). For example, an individual site’s heterogeneity of surface biophysical characteristics (e.g., areas with different albedos, vegetation, and soils) may cause a spatio-temporal heterogeneous LST at the corresponding locations (Tran et al., 2017; Guha et al., 2018). The impacts of climatic conditions and topographic factors such as lapse rate and downward radiation to the surface should be investigated further in regions with diverse topography. He et al. (2018) discovered a complicated relationship between topography factors and LST in mountainous areas. As a result, the downward radiation from the surface should be approximated on a pixel scale (Malbéteau et al., 2017; He et al., 2018). The amount of overcast sky, the weather, the time of day and year, the latitude and longitude of the surface, the albedo of the surface, and the topographical characteristics of the surface and the surrounding area impact the downward radiation to the surface. Research is necessary to accurately replicate the impact of various topographic characteristics and identities outlined above on the surface temperatures at the surface level.
The LST significantly affects the interchange and interaction of energy fluxes between the ground surface and the atmosphere. Understanding the relationship between LST and urban surface features is critical for developing efficient UHI mitigation methods. The new strategy of combining geostatistical and machine learning algorithms was employed to extract LULC, LST, and urban biophysical characteristics. Then, using multiple complex methodologies not previously investigated, we measured the non-linear associations between LST and NDVI, NDBI, and NDWI in the research region. We discovered that NDVI and NDBI are negatively linked with LST; the former mitigate UHI while the latter increase it.
As a consequence, NDVI and NDBI are both powerful predictors of UHI. Furthermore, we discovered that the NDWI, like the NDVI, has a “temperature edge” connection with the LST. Because there are so few bodies of water in the research region, the findings were inverted. We employed a parallel coordinate plot via categorized datasets to properly investigate it. We discovered that most data is negatively associated with LST, which is conceptually correct, but specific datasets have a positive relationship. Around the water bodies, such places were characterized by sand, exposed rock, and bare soil, which resulted in greater temperatures. Compared to typical multiple linear regressions, conditional maps produced enhanced results for investigating non-linear correlations between LST and landscape features. The comprehensive study of LST’s interaction with all of the other bio-physical components reveals that they have a very intricate connection. The outcome of this research would provide helpful information for mitigating the consequences of urban heat islands, which have been expanding because of climate change.
Although the present study uses sophisticated techniques to provide comprehensive insights into the relationship between LST and land use biophysical parameters, the research has some limitations, such as the use of course resolution images for LULC mapping and a weak representation of the relationship between LST and biophysical parameters. These challenges may be avoided by combining spatial clustering mapping with a bivariate LISA model, high-resolution satellite images, and the addition of machine learning.
The raw data supporting the conclusions of this article will be made available by the authors, without undue reservation.
JM: conceptualization, data curation, funding acquisition, software, validation, and writing—original draft. JM, MAh, and MKA: formal analysis. MAl, NK, and MAh: investigation. JM and MAl: methodology. JM, MAl, and MKA: project administration. MAh and NK: resources. MAl and MKA: supervision. MAh: visualization. MAl, NK, MAh, and MKA: writing—review and editing. All authors contributed to the article and approved the submitted version.
Funding for this research was given under award numbers IFP-KKU-2020/13 by the deputyship for research and innovation, Ministry of Education in Saudi Arabia.
The authors declare that the research was conducted in the absence of any commercial or financial relationships that could be construed as a potential conflict of interest.
All claims expressed in this article are solely those of the authors and do not necessarily represent those of their affiliated organizations, or those of the publisher, the editors and the reviewers. Any product that may be evaluated in this article, or claim that may be made by its manufacturer, is not guaranteed or endorsed by the publisher.
The authors extend their appreciation to the deputyship for research & in-novation, Ministry of education in Saudi Arabia for funding this research work through the project number IFP-KKU-2020/13.
The Supplementary Material for this article can be found online at: https://www.frontiersin.org/articles/10.3389/fevo.2022.878375/full#supplementary-material
Abulibdeh, A. (2021). Analysis of urban heat island characteristics and mitigation strategies for eight arid and semi-arid gulf region cities. Environ. Earth Sci. 80, 1–26. doi: 10.1007/s12665-021-09540-7
Allen, M. A., Roberts, D. A., and McFadden, J. P. (2021). Reduced urban green cover and daytime cooling capacity during the 2012–2016 California drought. Urban Clim. 36:100768. doi: 10.1016/j.uclim.2020.100768
AlQadhi, S., Mallick, J., Talukdar, S., Bindajam, A. A., Shohan, A. A. A., and Shahfahad (2021). Quantification of urban sprawl for past-to-future in Abha City, Saudi Arabia. Comput. Model. Eng. Sci. 129, 755–786. doi: 10.32604/cmes.2021.016640
Anselin, L. (1995). Local indicators of spatial association—LISA. Geogr. Anal. 27, 93–115. doi: 10.1111/j.1538-4632.1995.tb00338.x
Anselin, L., Rey, S. J., and Li, W. (2014). Metadata and provenance for spatial analysis: the case of spatial weights. Int. J. Geogr. Inform. Sci. 28, 2261–2280. doi: 10.1080/13658816.2014.917313
Bindajam, A. A., Mallick, J., AlQadhi, S., Singh, C. K., and Hang, H. T. (2020). Impacts of vegetation and topography on land surface temperature variability over the semi-arid mountain cities of Saudi Arabia. Atmos 11:762. doi: 10.3390/atmos11070762
Bindajam, A. A., Mallick, J., Talukdar, S., Islam, A. R. M., and Alqadhi, S. (2021). Integration of artificial intelligence–based LULC mapping and prediction for estimating ecosystem services for urban sustainability: past to future perspective. Arab. J. Geosci. 14, 1–23.
Carr, D. B., and Pickle, L. W. (2010). Visualizing Data Patterns with Micromaps, 1st Edn. London: Chapman & Hall/CRC.
Chen, X. L., Zhao, H. M., Li, P. X., and Yin, Z. Y. (2006). Remote sensing image-based analysis of the relationship between urban heat island and land use/cover changes. Remote Sens. Environ. 104, 133–146. doi: 10.1016/j.rse.2005.11.016
Das Majumdar, D., and Biswas, A. (2016). Quantifying land surface temperature change from LISA clusters: an alternative approach to identifying urban land use transformation. Landsc. Urban Plan. 153, 51–65. doi: 10.1016/j.landurbplan.2016.05.001
Dimoudi, A., Zoras, S., Kantzioura, A., Stogiannou, X., Kosmopoulos, P., and Pallas, C. (2014). Use of cool materials and other bioclimatic interventions in outdoor places in order to mitigate the urban heat island in a medium size city in Greece. Sustain. Cities Soc. 13, 89–96. doi: 10.1016/j.scs.2014.04.003
Duan, S. B., Li, Z. L., and Leng, P. (2017). A framework for the retrieval of all-weather land surface temperature at a high spatial resolution from polar-orbiting thermal infrared and passive microwave data. Remote Sens. Environ. 195, 107–117. doi: 10.1016/j.rse.2017.04.008
Feng, W., Boukir, S., and Huang, W. (2019). “Margin-based random forest for imbalanced land cover classification,” in Proceedings of the IGARSS 2019 - 2019 IEEE International Geoscience and Remote Sensing Symposium, (Yokohama: IEEE), 3085–3088.
Firozjaei, M. K., Alavipanah, S. K., Liu, H., Sedighi, A., Mijani, N., Kiavarz, M., et al. (2019). –OLS model for assessing the impact of surface biophysical parameters on land surface temperature variations. Remote Sens. 11:2094. doi: 10.3390/rs11182094
Fu, P., and Weng, Q. (2016). A time series analysis of urbanization induced land use and land cover change and its impact on land surface temperature with Landsat imagery. Remote Sens. Environ. 175, 205–214. doi: 10.1016/j.rse.2015.12.040
Genuer, R., Poggi, J. M., Tuleau-Malot, C., and Villa-Vialaneix, N. (2017). Random forests for big data. Big Data Res. 9, 28–46. doi: 10.1016/j.bdr.2017.07.003
Ghosh, S., Chatterjee, N. D., and Dinda, S. (2019). Relation between urban biophysical composition and dynamics of land surface temperature in the Kolkata metropolitan area: a GIS and statistical based analysis for sustainable planning. Model. Earth Syst. Environ. 5, 307–329. doi: 10.1007/s40808-018-0535-9
Gioia, A., Paolini, L., Malizia, A., Oltra-Carrió, R., and Sobrino, J. A. (2014). Size matters: vegetation patch size and surface temperature relationship in foothills cities of northwestern Argentina. Urban Ecosyst. 17, 1161–1174. doi: 10.1007/s11252-014-0372-1
Gohain, K. J., Mohammad, P., and Goswami, A. (2021). Assessing the impact of land use land cover changes on land surface temperature over Pune city, India. Quat. Int. 575–576, 259–269. doi: 10.1016/j.quaint.2020.04.052
Guha, S., Govil, H., Dey, A., and Gill, N. (2018). Analytical study of land surface temperature with NDVI and NDBI using Landsat 8 OLI and TIRS data in Florence and Naples city, Italy. Eur. J. Remote Sens. 51, 667–678. doi: 10.1080/22797254.2018.1474494
Guo, G., Wu, Z., Xiao, R., Chen, Y., Liu, X., and Zhang, X. (2015). Impacts of urban biophysical composition on land surface temperature in urban heat island clusters. Landsc. Urban Plan. 135, 1–10. doi: 10.1016/j.landurbplan.2014.11.007
He, J., Zhao, W., Li, A., Wen, F., and Yu, D. (2018). The impact of the terrain effect on land surface temperature variation based on Landsat-8 observations in mountainous areas. Int. J. Remote Sens. 40, 1808–1827. doi: 10.1080/01431161.2018.1466082
Imran, H. M., Hossain, A., Islam, A. K. M. S., Rahman, A., Bhuiyan, M. A. E., Paul, S., et al. (2021). Impact of land cover changes on land surface temperature and human thermal comfort in dhaka city of Bangladesh. Earth Syst. Environ. 5, 667–693. doi: 10.1007/s41748-021-00243-4
Jain, S., Sannigrahi, S., Sen, S., Bhatt, S., Chakraborti, S., and Rahmat, S. (2020). Urban heat island intensity and its mitigation strategies in the fast-growing urban area. J. Urban Manag. 9, 54–66. doi: 10.1016/j.jum.2019.09.004
Jiang, J., and Tian, G. (2010). Analysis of the impact of land use/land cover change on land surface temperature with remote sensing. Proc. Environ. Sci. 2, 571–575. doi: 10.1016/j.proenv.2010.10.062
Jimenez-Munoz, J. C., Sobrino, J. A., Skokovic, D., Mattar, C., and Cristobal, J. (2014). Land surface temperature retrieval methods from landsat-8 thermal infrared sensor data. IEEE Geosci. Remote Sens. Lett. 11, 1840–1843. doi: 10.3390/s19225049
Kaplan, G., Avdan, U., and Avdan, Z. Y. (2018). Urban heat island analysis using the landsat 8 satellite data: a case Study in Skopje, Macedonia. Proceedings 2:358.
Kuang, W., Liu, Y., Dou, Y., Chi, W., Chen, G., Gao, C., et al. (2015). What are hot and what are not in an urban landscape: quantifying and explaining the land surface temperature pattern in Beijing, China. Landsc. Ecol. 30, 357–373. doi: 10.1007/s10980-014-0128-6
Li, J., Song, C., Cao, L., Zhu, F., Meng, X., and Wu, J. (2011). Impacts of landscape structure on surface urban heat islands: a case study of Shanghai, China. Remote Sens. Environ. 115, 3249–3263. doi: 10.1016/j.scitotenv.2020.138229
Liu, Y., Peng, J., and Wang, Y. (2018). Efficiency of landscape metrics characterizing urban land surface temperature. Landsc. Urban Plan. 180, 36–53. doi: 10.1016/j.landurbplan.2018.08.006
Malbéteau, Y., Merlin, O., Gascoin, S., Gastellu, J. P., Mattar, C., Olivera-Guerra, L., et al. (2017). Normalizing land surface temperature data for elevation and illumination effects in mountainous areas: a case study using ASTER data over a steep-sided valley in Morocco. Remote Sens. Environ. 189, 25–39. doi: 10.1016/j.rse.2016.11.010
Mallick, J., Bindajam, A. A., AlQadhi, S., Ahmed, M., Hang, H. T., and Thanh, N. V. (2020). A comparison of four land surface temperature retrieval method using TERRA-ASTER satellite images in the semi-arid region of Saudi Arabia. Geocarto Int. [Epub ahead of print].
Mallick, J., and Rahman, A. (2012). Impact of population density on the surface temperature and micro-climate of Delhi on JSTOR. Curr. Sci. 102, 1708–1713.
Mallick, J., Singh, V. P., Almesfer, M. K., Talukdar, S., Alsubhi, M., Ahmed, M., et al. (2021). Spatio-temporal analysis and simulation of land cover changes and their impacts on land surface temperature in urban agglomeration of Bisha Watershed, Saudi Arabia. Geocarto Int. [Epub ahead of print].
Mallick, S. K., and Rudra, S. (2021). Land use changes and its impact on biophysical environment: study on a river bank. Egypt. J. Remote Sens. Sp. Sci. 24, 1037–1049. doi: 10.1016/j.ejrs.2021.11.002
Mathew, A., Khandelwal, S., and Kaul, N. (2016). Spatial and temporal variations of urban heat island effect and the effect of percentage impervious surface area and elevation on land surface temperature: study of Chandigarh city, India. Sustain. Cities Soc. 26, 264–277. doi: 10.1016/j.scs.2016.06.018
Mathew, A., Khandelwal, S., and Kaul, N. (2017). Investigating spatial and seasonal variations of urban heat island effect over Jaipur city and its relationship with vegetation, urbanization and elevation parameters. Sustain. Cities Soc. 35, 157–177. doi: 10.1016/j.scs.2017.07.013
McFeeters, S. K. (2007). The use of the Normalized Difference Water Index (NDWI) in the delineation of open water features. Int. J. Remote Sens. 17, 1425–1432. doi: 10.1080/01431169608948714
Naikoo, M. W., Rihan, M., and Ishtiaque, M. (2020). Shahfahad Analyses of land use land cover (LULC) change and built-up expansion in the suburb of a metropolitan city: spatio-temporal analysis of Delhi NCR using landsat datasets. J. Urban Manag. 9, 347–359. doi: 10.1016/j.jum.2020.05.004
Naikoo, M. W., Rihan, M., Peer, A. H., Talukdar, S., Mallick, J., Ishtiaq, M., et al. (2022). Analysis of peri-urban land use/land cover change and its drivers using geospatial techniques and geographically weighted regression. Environ. Sci. Pollut. Res. [Epub ahead of print]. doi: 10.1007/s11356-022-18853-4
Nurwanda, A., and Honjo, T. (2020). The prediction of city expansion and land surface temperature in Bogor City, Indonesia. Sustain. Cities Soc. 52:101772. doi: 10.1016/j.scs.2019.101772
Oke, T. R., and Cleugh, H. A. (1987). Urban heat storage derived as energy balance residuals. Bound. Layer Meteorol. 39, 233–245. doi: 10.1007/bf00116120
Pal, S., and Ziaul, S. (2017). Detection of land use and land cover change and land surface temperature in English Bazar urban centre. Egypt. J. Remote Sens. Sp. Sci. 20, 125–145. doi: 10.1016/j.ejrs.2016.11.003
Peng, J., Jia, J., Liu, Y., Li, H., and Wu, J. (2018). Seasonal contrast of the dominant factors for spatial distribution of land surface temperature in urban areas. Remote Sens. Environ. 215, 255–267. doi: 10.1016/j.rse.2018.06.010
Qiao, Z., Liu, L., Qin, Y., Xu, X., Wang, B., and Liu, Z. (2020). The impact of urban renewal on land surface temperature changes: a case study in the main city of Guangzhou, China. Remote Sens. 12:794. doi: 10.3390/rs12050794
Roy, S., Pandit, S., Eva, E. A., Bagmar, M. S. H., Papia, M., Banik, L., et al. (2020). Examining the nexus between land surface temperature and urban growth in Chattogram Metropolitan Area of Bangladesh using long term Landsat series data. Urban Clim. 32:100593. doi: 10.1016/j.uclim.2020.100593
Sattari, F., Hashim, M., and Pour, A. B. (2018). Thermal sharpening of land surface temperature maps based on the impervious surface index with the TsHARP method to ASTER satellite data: a case study from the metropolitan Kuala Lumpur, Malaysia. Measurement 125, 262–278. doi: 10.1016/j.measurement.2018.04.092
Shahfahad, Kumari, B., Tayyab, M., Ahmed, I. A., Baig, M. R. I, Khan, M. F., et al. (2020). Longitudinal study of land surface temperature (LST) using mono- and split-window algorithms and its relationship with NDVI and NDBI over selected metro cities of India. Arab. J. Geosci. 13, 1–19.
Shahfahad, Talukdar, S., Rihan, M., Hang, H. T., Bhaskaran, S., and Rahman, A. (2021). Modelling urban heat island (UHI) and thermal field variation and their relationship with land use indices over Delhi and Mumbai metro cities. Environ. Dev. Sustain. 24, 3762–3790. doi: 10.1007/s10668-021-01587-7
Sharma, R., Chakraborty, A., and Joshi, P. K. (2015). Geospatial quantification and analysis of environmental changes in urbanizing city of Kolkata (India). Environ. Monit. Assess. 187, 1–12. doi: 10.1007/s10661-014-4206-7
Sinha, S., Sharma, L. K., and Nathawat, M. S. (2015). Improved Land-use/Land-cover classification of semi-arid deciduous forest landscape using thermal remote sensing. Egypt. J. Remote Sens. Sp. Sci. 18, 217–233. doi: 10.1016/j.ejrs.2015.09.005
Sobrino, J. A., Jiménez-Munoz, J. C., El-Kharraz, J., Gómez, M., Romaguera, M., and Sòria, G. (2010). Single-channel and two-channel methods for land surface temperature retrieval from DAIS data and its application to the Barrax site. Int. J. Remote Sens. 25, 215–230. doi: 10.1080/0143116031000115210
Song, J., Chen, W., Zhang, J., Huang, K., Hou, B., and Prishchepov, A. V. (2020). Effects of building density on land surface temperature in China: spatial patterns and determinants. Landsc. Urban Plan. 198:103794. doi: 10.3390/ijerph182413088
Srikanta, S., Sandeep, B., Shahid, R., Bhumika, U., Sayandeep, B., Suman, C., et al. (2018). Analyzing the role of biophysical compositions in minimizing urban land surface temperature and urban heating. Urban Clim. 24, 803–819. doi: 10.1016/j.uclim.2017.10.002
Sussman, H. S., Dai, A., and Roundy, P. E. (2021). The controlling factors of urban heat in Bengaluru, India. Urban Clim. 38:100881. doi: 10.1039/d0em00358a
Townshend, J. R. G., and Justice, C. O. (2007). Analysis of the dynamics of African vegetation using the normalized difference vegetation index. Int. J. Remote Sens. 7, 1435–1445. doi: 10.1080/01431168608948946
Tran, D. X., Pla, F., Latorre-Carmona, P., Myint, S. W., Caetano, M., and Kieu, H. V. (2017). Characterizing the relationship between land use land cover change and land surface temperature. ISPRS J. Photogramm. Remote Sens. 124, 119–132. doi: 10.1016/j.isprsjprs.2017.01.001
Trlica, A., Hutyra, L. R., Schaaf, C. L., Erb, A., and Wang, J. A. (2017). Albedo, land cover, and daytime surface temperature variation across an urbanized landscape. Earth’s Futur. 5, 1084–1101. doi: 10.1002/2017ef000569
Weng, Q., Firozjaei, M. K., Kiavarz, M., Alavipanah, S. K., and Hamzeh, S. (2019). Normalizing land surface temperature for environmental parameters in mountainous and urban areas of a cold semi-arid climate. Sci. Total Environ. 650, 515–529. doi: 10.1016/j.scitotenv.2018.09.027
Wentz, E. A., Anderson, S., Fragkias, M., Netzband, M., Mesev, V., Myint, S. W., et al. (2014). Supporting global environmental change research: a review of trends and knowledge gaps in urban remote sensing. Remote Sens. 6, 3879–3905. doi: 10.3390/rs6053879
Wu, C., Li, J., Wang, C., Song, C., Chen, Y., Finka, M., et al. (2019). Understanding the relationship between urban blue infrastructure and land surface temperature. Sci. Total Environ. 694:133742. doi: 10.1016/j.scitotenv.2019.133742
Xiang, Y., Huang, C., Huang, X., Zhou, Z., and Wang, X. (2021). Seasonal variations of the dominant factors for spatial heterogeneity and time inconsistency of land surface temperature in an urban agglomeration of central China. Sustain. Cities Soc. 75:103285. doi: 10.1016/j.scs.2021.103285
Xie, M., Wang, Y., Chang, Q., Fu, M., and Ye, M. (2013). Assessment of landscape patterns affecting land surface temperature in different biophysical gradients in Shenzhen, China. Urban Ecosyst. 16, 871–886. doi: 10.1007/s11252-013-0325-0
Xu, B., Huang, J. Z., Williams, G., Wang, Q., Ye, Y., Xu, B., et al. (2012). Classifying Very High-Dimensional Data with Random Forests Built from Small Subspaces. Int. J. Data Warehous. Min. 8, 44–63. doi: 10.4018/jdwm.2012040103
Yang, Y., Cao, C., Pan, X., Li, X., and Zhu, X. (2017). Downscaling land surface temperature in an arid area by using multiple remote sensing indices with random forest regression. Remote Sens. 9:789. doi: 10.3390/rs9080789
Yao, L., Li, T., Xu, M., and Xu, Y. (2020). How the landscape features of urban green space impact seasonal land surface temperatures at a city-block-scale: an urban heat island study in Beijing, China. Urban For. Urban Green. 52:126704. doi: 10.1016/j.ufug.2020.126704
Yu, X., Guo, X., and Wu, Z. (2014). Land surface temperature retrieval from landsat 8 TIRS—comparison between radiative transfer equation-based method, split window algorithm and single channel method. Remote Sens. 6, 9829–9852. doi: 10.3390/rs6109829
Zawadzka, J. E., Harris, J. A., and Corstanje, R. (2021). A simple method for determination of fine resolution urban form patterns with distinct thermal properties using class-level landscape metrics. Landsc. Ecol. 36, 1863–1876. doi: 10.1007/s10980-020-01156-9
Zha, Y., Gao, J., and Ni, S. (2010). Use of normalized difference built-up index in automatically mapping urban areas from TM imagery. Int. J. Remote Sens. 24, 583–594.
Zhang, Y., Odeh, I. O. A., and Han, C. (2009). Bi-temporal characterization of land surface temperature in relation to impervious surface area, NDVI and NDBI, using a sub-pixel image analysis. Int. J. Appl. Earth Obs. Geoinf. 11, 256–264.
Zhao, J., Zhao, X., Liang, S., Zhou, T., Du, X., Xu, P., et al. (2020). Assessing the thermal contributions of urban land cover types. Landsc. Urban Plan. 204:103927. doi: 10.1016/j.landurbplan.2020.103927
Zhao, Z. Q., He, B. J., Li, L. G., Wang, H. B., and Darko, A. (2017). Profile and concentric zonal analysis of relationships between land use/land cover and land surface temperature: case study of Shenyang, China. Energy Build. 155, 282–295. doi: 10.1016/j.enbuild.2017.09.046
Zhou, D., Xiao, J., Bonafoni, S., Berger, C., Deilami, K., Zhou, Y., et al. (2018). Satellite remote sensing of surface urban heat islands: progress, challenges, and perspectives. Remote Sens. 11:48. doi: 10.3390/rs11010048
Zhou, L., Tian, Y., Baidya Roy, S., Thorncroft, C., Bosart, L. F., Hu, Y., et al. (2012). Impacts of wind farms on land surface temperature. NatCC 2, 539–543. doi: 10.1038/nclimate1505
Keywords: urbanization, land surface temperature, urban hydro-biophysical parameters, machine learning, conditional map, remote sensing
Citation: Mallick J, Alsubih M, Ahmed M, Almesfer MK and Kahla NB (2022) Assessing the Spatiotemporal Heterogeneity of Terrestrial Temperature as a Proxy to Microclimate and Its Relationship With Urban Hydro-Biophysical Parameters. Front. Ecol. Evol. 10:878375. doi: 10.3389/fevo.2022.878375
Received: 17 February 2022; Accepted: 18 March 2022;
Published: 28 April 2022.
Edited by:
Md. Rejaur Rahman, University of Rajshahi, BangladeshReviewed by:
Nazrul Islam, Jahangirnagar University, BangladeshCopyright © 2022 Mallick, Alsubih, Ahmed, Almesfer and Kahla. This is an open-access article distributed under the terms of the Creative Commons Attribution License (CC BY). The use, distribution or reproduction in other forums is permitted, provided the original author(s) and the copyright owner(s) are credited and that the original publication in this journal is cited, in accordance with accepted academic practice. No use, distribution or reproduction is permitted which does not comply with these terms.
*Correspondence: Javed Mallick, am1hbGxpY2tAa2t1LmVkdS5zYQ==
Disclaimer: All claims expressed in this article are solely those of the authors and do not necessarily represent those of their affiliated organizations, or those of the publisher, the editors and the reviewers. Any product that may be evaluated in this article or claim that may be made by its manufacturer is not guaranteed or endorsed by the publisher.
Research integrity at Frontiers
Learn more about the work of our research integrity team to safeguard the quality of each article we publish.