- 1Resilient Conservation, Centre for Planetary Health and Food Security, Griffith University, Nathan, QLD, Australia
- 2School of Biological Sciences, The University of Queensland, Brisbane, QLD, Australia
- 3School of Environmental Science and Engineering, Southern University of Science and Technology, Shenzhen, China
- 4School of Earth and Sustainability, Northern Arizona University, Flagstaff, AZ, United States
- 5Centre for Complex Systems in Transition, School of Public Leadership, Stellenbosch University, Stellenbosch, South Africa
- 6Environmental Foundation Limited, Colombo, Sri Lanka
- 7School of Natural Resource Management, Nelson Mandela University, George, South Africa
Large felids represent some of the most threatened large mammals on Earth, critical for both tourism economies and ecosystem function. Most populations are in a state of decline, and their monitoring and enumeration is therefore critical for conservation. This typically rests on the accurate identification of individuals within their populations. We review the most common and current survey methods used in individual identification studies of large felid ecology (body mass > 25 kg). Remote camera trap photography is the most extensively used method to identify leopards, snow leopards, jaguars, tigers, and cheetahs which feature conspicuous and easily identifiable coat patterning. Direct photographic surveys and genetic sampling are commonly used for species that do not feature easily identifiable coat patterning such as lions. We also discuss the accompanying challenges encountered in several field studies, best practices that can help increase the precision and accuracy of identification and provide generalised ratings for the common survey methods used for individual identification.
Introduction
The robust estimation of threatened wildlife numbers remains a key topic in modern-day conservation biology (Gopalaswamy et al., 2019; Elliot et al., 2020). This is because many of Earth’s most imperilled species are critical to ecotourism, may adversely impact human livelihoods, and facilitate critical ecosystem services such as top-down regulation of prey and intraguild members (Ripple et al., 2014; O’Bryan et al., 2018). Large felids, which in this review we define as apex predators belonging to the genus Panthera, and other large-sized cats (each with a mass of >25 kg) are one such emblematic group of wildlife. Large felids generate substantial revenue globally via ecotourism (e.g., jaguar viewing in Brazil’s Pantanal contributes ∼7 million USD annually, Tortato et al., 2017), but they are often killed in human-wildlife conflicts mainly in response to livestock-raiding (Tumenta et al., 2010) and are also exposed to overharvest (Packer et al., 2011), and habitat contraction (Joshi et al., 2016). Resultantly, most large felids are declining (Packer et al., 2013; Donnelly, 2020).
A key underpinning for efficient and reliable population monitoring of large felids and other mammalian species is the ability of researchers to identify individuals that make up a wild population (Kuningas et al., 2014; Balme et al., 2019). This is crucial because individual misidentifications may adversely affect management decisions, conservation assessment and even lead to erroneous quota setting for extractive activities like trophy hunting (Balme et al., 2010). Since the ground-breaking work of Karanth (1995) and Karanth and Nichols (1998), survey methods that aid in individual identification are commonly followed by the capture-recapture (CR) framework to estimate their populations. The reliability of density estimates using CR models is largely contingent on accurate identification of individuals (Choo et al., 2020). While there are population estimation models that do not rely on individual recognition of animals such as the random encounter model (REM) used to estimate the density of lions in Tanzania (Cusack et al., 2015) and tiger prey in Cambodia (Gray et al., 2020), individual identification-based analytical models are more superior and often take precedence due to their promising abilities to monitor wildlife populations while maintaining an ease of use (Johansson et al., 2020). Consequently, scientists have increasingly diverted their attention to individual-based identification as a critical first step to estimate population size, understand animal ecology and behaviour, and to inform conservation and management decisions of threatened and endangered species.
In this paper, we review the main survey techniques that have become established for large felid individual identification in recent years. We also discuss how individual misidentifications can have serious implications for management and conservation (Karanth et al., 2003; Karanth, 2011). We avoid methods of individual identification that are proven to be unreliable or inefficient when compared to others (e.g., individual identification of tigers from their pugmarks, Karanth et al., 2003). Similarly, sign surveys, oral interview surveys or records of livestock attacks may only indicate felid presence but are unable to provide individual-specific details within the animal population of interest and are often prone to erroneous and biased inferences (Janečka et al., 2008; Karmacharya et al., 2011; Suryawanshi et al., 2019).
Our literature review provides (a) a brief overview of the commonly used survey methods to identify individuals of seven large felids divided into three broad categories—species with distinct pelage markings, species with less distinct pelage markings and species that lack distinct pelage markings, (b) discussion on the challenges associated with these techniques, (c) ways to improve current individual identification survey methods that can potentially reduce and prevent misidentification and (d) generalised ratings of the survey methods that facilitate individual identification. We focus on seven large cat species namely, tiger Panthera tigris, jaguar Panthera onca, lion Panthera leo, leopard Panthera pardus, snow leopard Panthera uncia, cheetah Acinonyx jubatus and mountain lion Puma concolor (each >25 kg in body mass).
Common Methods to Individually Identify Big Cats
The most frequently used survey methods in recent field studies of large felids include camera trapping, direct photographic surveys, and genetic sampling. These survey methods use specific identification markers such as pelage patterning, vibrissae spots, genetic markers, scars, and other external features uniquely characteristic to each individual animal within a population. When appropriately used they can provide reliable data about individual identities of felids which in turn provide better understanding of their ecology, behaviour, and for population monitoring.
Previous research has already demonstrated that most large felids are cryptic, wide-ranging, and occur in low densities (Sollmann et al., 2013a; Murphy et al., 2019). While individual identification of species with distinctive markings is often straightforward, individual recognition of species that lack unambiguous markings can be more challenging (Foster and Harmsen, 2012). This is because practitioners are required to devote more time and effort to reliably identify individuals which lack distinct markings (Mattioli et al., 2018) or by choosing a survey method that can detect individuals based on other features. Failure to adopt appropriate survey methods increases the chances of individual misidentification (Soller et al., 2020), may inflate or deflate capture rates (Johansson et al., 2020; Garrote et al., 2021), bias sex/age-class structure of the population (Balme et al., 2012), and adversely impact population management and conservation decisions (Balme et al., 2010).
Species With Distinct Pelage Markings
We classified tigers, leopards, jaguars, and cheetahs as species with distinct pelage markings. Tiger stripes (Figure 1A; Shi et al., 2020), leopard (Figure 1B; Balme et al., 2019) and jaguar (Figure 1C; Soisalo and Cavalcanti, 2006) rosettes and spots, and cheetah spots (Figure 1D; Marnewick et al., 2008), and tail rings (Chelysheva, 2004) are prominent coat patterns characteristic to each individual animal found in a population. Consequently, these felid species make ideal candidates for camera trap study design (Karanth and Nichols, 1998; Balme et al., 2019). Moreover, as most large felids are sexually dimorphic, especially leopards (Balme et al., 2012), disparities in morphological size and sex-based characteristics can inform researchers about the age and sex of individuals which may assist individual recognition further.
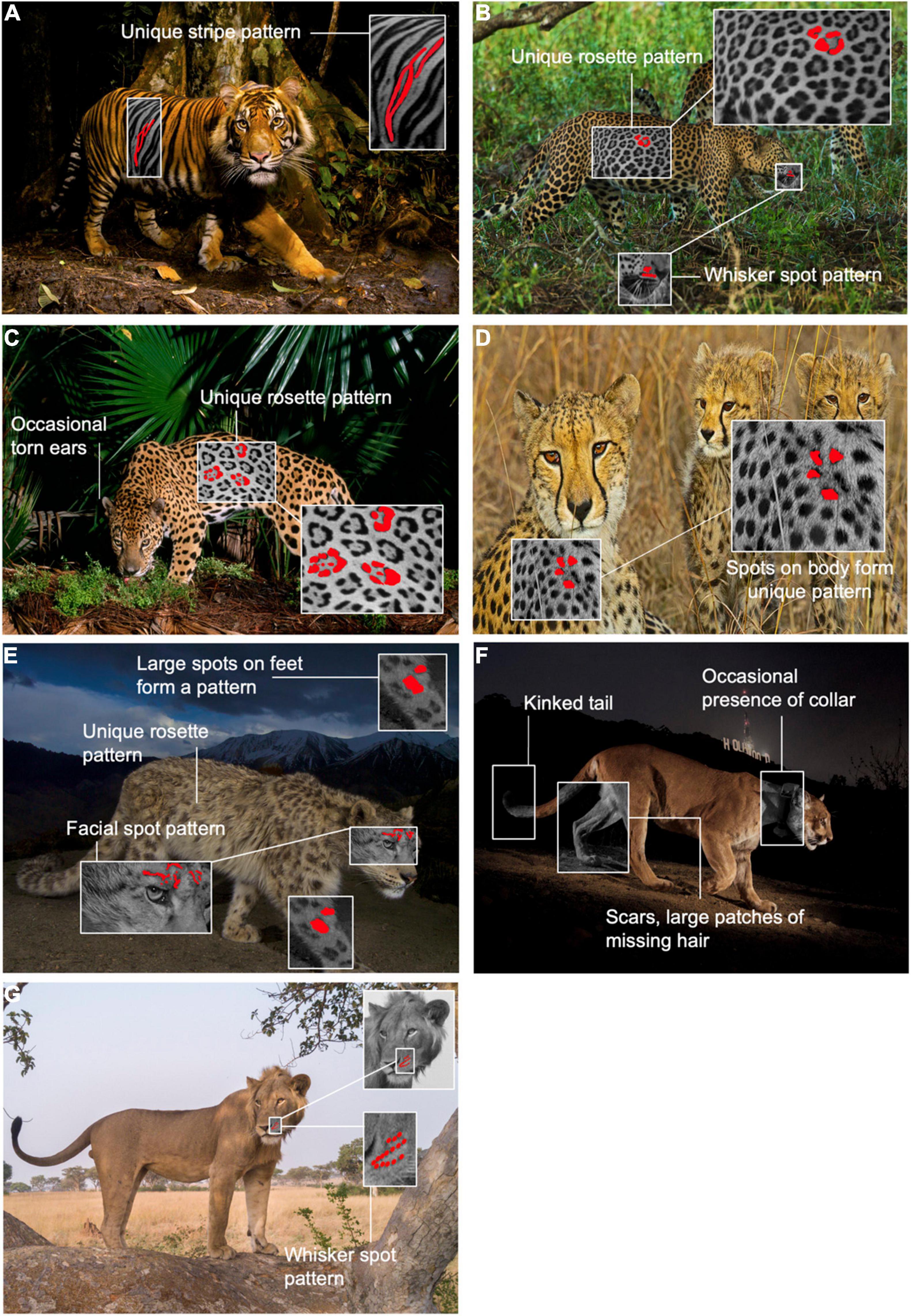
Figure 1. Most commonly used morphological locations used during surveys to identify the seven large felids described in our review. (A) Tiger, (B) leopard, (C) jaguar, (D) cheetah, (E) snow leopard, (F) mountain lion and (G) lion. Photo credits: Steve Winter and Alexander Braczkowski.
Karanth (1995) and Karanth and Nichols (1998) used the patterns of black stripes on individual tigers combined with closed CR modelling to estimate their densities in the wild. However, tigers mainly make use of trails and roads as travel and hunting routes. Therefore, to achieve a high number of individual identification records of different tigers, camera traps were set along these routes. This optimal camera trap placement along travelled routes should be considered by researchers. This can be easily determined based on the tracks, scats and other signs left behind by tigers (Karanth, 1995). Such species-specific targeting of camera traps ensures maximum likelihood of capturing individually recognisable individuals. Deployment of two camera traps and strategic deployment on either side of a trail to capture both flanks of an animal further increases the chance of recording an image where individually identifiable characteristics of an animal may be recorded (i.e., stripe patterns visible beyond just their coat but also on their face, limbs, and tail, Karanth and Nichols, 2011). To prevent the two camera flashes from interfering with each other, it is important to avoid setting them directly facing each other. Such protocol adherence is regularly observed for the monitoring of tigers (Tempa et al., 2019; Jhala et al., 2021), leopards (Hedges et al., 2015; Devens et al., 2018), cheetahs (Brassine and Parker, 2015; Broekhuis and Gopalaswamy, 2016), and jaguars (Greenspan et al., 2020; Harmsen et al., 2020). More recently, to aid practitioners in individual identification, machine learning algorithms that train Deep Convolutional Neural Networks (DCNN) to automatically identify distinctive coat markings of individuals from extensive reference image database have emerged (Pucci et al., 2020). This novel technology has been increasingly used on individually identifiable species such as tigers (Cheema and Anand, 2017; Pucci et al., 2020; Shi et al., 2020) and leopards (Pucci et al., 2020).
While individual leopards and jaguars are usually easily identifiable, some populations consist of both wild-type and melanistic phenotypes. Camera traps can be used to identify individuals from such populations using the spots, rosettes and scars recorded in photographs, but animals must walk very close to the camera for clear identification (Silveira et al., 2010). To improve the chance of identifying melanistic individuals, camera traps can be modified. For example, in Peninsular Malaysia members of a unique melanistic population of leopards were individually identified using modified infrared flash camera traps (Hedges et al., 2015). This modification forced the cameras into night mode, allowing for consistent and clear recognition of the melanistic leopard’s heavily pigmented rosettes which were invisible when cameras were operated in their daytime photo mode (Hedges et al., 2015). Such modifications highlight the prospect of camera traps being used to obtain individual identities of species even in situations when their pelage markings might be less prominent. If such camera modifications are impossible, the use of spatial mark-resight (SMR) models that only require a subset of the population to be identified may be used to provide reliable population estimates (Harihar et al., 2021).
The individual identification of cheetahs can be challenging due to their large home ranges (Brassine and Parker, 2015). Researchers may incorrectly assume individuals observed in a certain area to be the same as a resident individual they are familiar with. Furthermore, cheetahs do not regularly make use of trails and roads like other felid species (instead using open habitats like grassy plains), and regular camera trap setups may yield low capture rates (Brassine and Parker, 2015). Camera traps should therefore be placed at environmental features regularly used by cheetahs such as “play” or scent-marking trees (Kusler et al., 2019) to increase detection rates. Importantly however, these sites are mostly used to facilitate communication between adult male cheetahs and are less likely to yield individual detections of female cheetahs (see Kusler et al., 2019).
Whisker spot patterns are another prime identifiable feature beside prominent pelage markings for some felids like leopards which can be used to identify individuals during direct photographic surveys (Figure 1B; Miththapala et al., 1989). Using the vibrissae/whisker spot pattern method developed for lions by Pennycuick and Rudnai (1970), individual leopards can be identified by using an identification code based on the numbered rows of vibrissae spots from the individual’s nose to its lips (Miththapala et al., 1989). Balme et al. (2017) provides a recent example where direct photographic surveys were used to identify individual leopards based on their unique vibrissae spots in Sabi Sands Game Reserve of South Africa. The number of whisker spots and their relative placement to one another on either side of the leopard’s muzzle varies amongst individuals (Miththapala et al., 1989). When animals are individually identifiable but are difficult to monitor with camera traps, direct photographic surveys using digital cameras can be a preferred survey method (Wilson and Delahay, 2001).
Complementary to photographic survey methods, non-invasive genetic-capture sampling using faecal DNA has become commonplace for the individual identification of tigers (Mondol et al., 2009; Thapa et al., 2018), leopards (Busby et al., 2009; Bhatt et al., 2020), jaguars (Roques et al., 2014; Wultsch et al., 2014) and cheetahs (Busby et al., 2009). The faecal DNA obtained from these species are genotyped using genetic markers such as microsatellites or single nucleotide polymorphism (SNP) panels (Magliolo et al., 2021; Thuo, 2021). Genetic sampling is useful for individual identification because it can provide researchers with additional types of information that camera trapping and direct photographic surveys alone cannot provide including the diet composition and genetic diversity of a population. Such details can provide researchers with a better understanding of the behavioural and social ecology of the species of interest.
Species With Less Distinct Pelage Markings
We classify snow leopards as species with less distinct pelage markings because although they possess individually identifiable spot and rosette markings on their limbs, flanks, and dorsal tail portion (Figure 1E), their long fur often masks the pelage patterns (Jackson et al., 2006), leading to discrepancies in individual identities among human observers (Johansson et al., 2020). Additionally, because they inhabit difficult-to-access high-altitude ecosystems between 3,000 and 5,400 m above sea-level (Janečka et al., 2008; WWF Nepal, 2018) and occupy expansive home range sizes which are often highly variable, researchers may incorrectly assume one photo-captured individual as another (Alexander et al., 2016; Chetri, 2018). For these reasons, snow leopards are vulnerable to individual misidentification and are challenging to monitor in the wild (Johansson et al., 2020). Thus, multiple trained researchers are often required to match the photo-captured snow leopards independently using at least three pelage pattern patches for more accurate identification (Jackson et al., 2006; Alexander et al., 2016). This is a good practice for consistent and reliable individual identification of not only snow leopards but also other large felids and broader mammalian species.
For snow leopard individual identification, several modern-day studies have utilised camera trap surveys as an effective and efficient sampling method (Jackson et al., 2006; Sharma et al., 2014; Alexander et al., 2016). This is because like other large felids, snow leopards have well-defined travel routes generally along ridgelines and valley edges which may be identified by the presence of their signs (e.g., scats, scrapes, scent-sprays, and tracks, Jackson et al., 2006). This knowledge can be used to strategically position camera traps along frequently used trails to maximise photographic captures and to identify individuals (Janečka et al., 2011). Additionally, near- automatic machine learning algorithms have been developed to assist practitioners to recognise snow leopard from their pelage patterns (Hamilton, 2017; Snow Leopard Trust, 2019). Such algorithms have been increasingly used to identify individual snow leopards (Tariq et al., 2018; Curtin and Matthews, 2019).
Genetic sampling of snow leopard scats is another method that is time, cost and labour-efficient, and reliable (Janečka et al., 2011), and is especially well-suited for individual identification of the animal as it has a proclivity for marking sites (Jackson et al., 2006). Genetic markers such as microsatellites are extensively used to identify individual snow leopards from faecal DNA (Janečka et al., 2011; Karmacharya et al., 2011), and they usually have high mutation rates containing high information content (Magliolo et al., 2021). However, there are some major limitations concerning microsatellite loci diversity in snow leopards which could impede individual identification (see discussion in paragraphs regarding limitations). Simultaneously, in recent years, SNP markers are also being widely employed for individual identification of snow leopards (Janjua et al., 2020). Next generation sequencing (NGS) techniques using SNPs provide broad genome coverage, rich information recovery from small, degraded DNA fragments and can be standardised automatically across laboratories (Magliolo et al., 2021). Such superior benefits offered by SNP markers can help practitioners learn more about elusive felids with less effort.
Species Which Lack Distinct Pelage Markings
We grouped lions and mountain lions under species that lack distinct pelage markings category. Individual identification of these species is challenging because they lack conspicuous coat patterns and researchers often rely on smaller identifiable features (Foster and Harmsen, 2012). However, trained observers can at times identify individual mountain lions from photographs using natural time-stable characteristics such as kinked tails, undercoat spots, and colouration of the tail tip and underside of the limbs, as well as scars and botfly marks that change over time (Figure 1F; Kelly et al., 2008; Negrões et al., 2010). Such features are less conspicuous and cannot be found on all individuals within a population. Contrastingly, similar to leopards, lions can be individually identified by enumerating their whisker spots from close-up digital photographs of individuals taken during direct photographic surveys (Figure 1G; Pennycuick and Rudnai, 1970; Elliot and Gopalaswamy, 2017; Gogoi et al., 2020). This method is very accurate, as in a sample of 920 wild African lions in Tanzania, Packer and Pusey (1993) found that 96% of individuals who could be scored for the number and location for both sides of the face were unique. This method of individual identification has formed the central foundation for subsequent estimates of lion abundance and density, and long-term ecology studies in locations such as Kenya’s Maasai Mara (Blackburn et al., 2016; Elliot and Gopalaswamy, 2017), Tanzania’s Serengeti and Ngorongoro (Packer et al., 2005; Kissui et al., 2010), South Africa’s Kalahari (Beukes et al., 2017), and India’s Gir forest (Jhala et al., 2019; Gogoi et al., 2020). In addition to vibrissae spot patterns, lions may also be individually identified from ear notches and permanent facial scarring (Pennycuick and Rudnai, 1970; Gogoi et al., 2020). Simultaneously, owing to prominent sexual dimorphism, individual lions can be confidently assigned sex (Kane et al., 2015).
Camera trap surveys have often been used to identify individual mountain lions (Noss et al., 2012; Rich et al., 2014; Alexander and Gese, 2018) and in very limited circumstances African lions (Kane et al., 2015). Unlike African lions (and leopards) which observers can often drive up to using vehicles in numerous field settings, mountain lions are rarely encountered by human observers making whisker spot-based individual identification an impractical method for identifying the species. Therefore, there is reliance on camera trap-based studies that make use of multiple human observers to identify individual mountain lions from natural marks (scars, colouration, etc.) or artificial tags (radio-collars) but there is some debate whether such subtle features can be reliably used to identify individual mountain lions due to high inter-observer discrepancies (see Alexander and Gese, 2018). Similarly, Kane et al. (2015) identified African lions from scars, wounds, mane size and colour, missing tail tips, and markings on the interior of the side hind legs using camera trapping in Senegal’s Niokolo Koba National Park, but it is questionable to what degree of certainty an entire sample of camera trapped lions could be accurately identified using such features (mainly because individuals may not have such features, or they may heal/change over time). This reason, and the success of whisker spot enumeration of lions using direct photographic surveys, could possibly explain the near-complete lack of studies using camera traps to identify African lions (see Braczkowski et al., 2020 for a review).
Similar to other large felids discussed in this review, genetic analysis is a reliable and efficient method of identifying individual lions and mountain lions (Miotto et al., 2014), and DNA may be collected non-invasively from scats (Wultsch et al., 2014; Curry et al., 2019) or directly from biopsy darts which sample tissue material (Beausoleil et al., 2016).
Remaining Challenges
Camera Trap Surveys
Even though the common survey methods for individual identification of large cats discussed in this review have become increasingly reliable and efficient, several challenges remain. While camera trapping provides a high capture rate of species that are otherwise difficult to detect in the wild (Foster and Harmsen, 2012), using camera trap images to identify individuals can be challenging at times (Johansson et al., 2020). Poor lighting conditions, incorrect camera trap placement and angle, fast moving animals resulting in blurred images, and researcher inexperience may increase the probability of individual misidentification. For example, in a study conducted by Johansson et al. (2020) using a camera trap survey design, human observers misidentified 12.5% of capture occasions resulting in an average of 35% population inflation. Such misidentification can further increase with large sample sizes of images (Hiby et al., 2009; Foster and Harmsen, 2012). As larger quantities of photographs have to be identified and constantly cross-referenced, this causes observer fatigue, which in turn increases error probability (Hiby et al., 2009; Alexander et al., 2016). This issue may be particularly more problematic in species such as mountain lions and lions that lack unique coat markings (Alexander and Gese, 2018).
Direct Photographic Surveys
While direct photographic surveys using manually operated digital cameras in the field are useful for identifying individual lions that are difficult to monitor with camera trapping (Elliot and Gopalaswamy, 2017; Elliot et al., 2020), there are few challenges associated with direct photographic surveys that are similar to camera trap surveys. Observer fatigue and/or bias can cause researchers to misidentify photo-captured individuals if the photograph record database is sufficiently large, or if the image observer lacks the experience to detect subtle natural markings on the animals. Using call-ups to lure individual animals for capturing close-up images can introduce the issue of sampling bias because in some populations individuals can be observer-shy, therefore, making their encounters more difficult in the wild. Generally, call-ups have large errors, and it is not appropriate to estimate population size based on few tame animals or habituated individuals that approach field teams and hence can photographed as this will likely result in population underestimation (De Blocq, 2014). Additionally, to adequately photograph felids for individual identification via direct photographic surveys, enough time and survey effort are required. This, however, can be limited by weather conditions and terrains in the field (Corcoran et al., 2019). Such limiting factors can interfere with the efficiency of survey protocols for individual identification.
Genetic Sampling Surveys
Genetic sampling is a highly efficient method for individual identification when studying wide-ranging felids, especially across larger areas (Janečka et al., 2011), but gathering non-invasive biological samples can have substantial observer bias. Soller et al. (2020) found that when comparing new and experienced observers on their abilities to sample wolf scats, the new observers collected more scat samples and identified species correctly slightly more often than experienced observers. This is a less discussed but valid concern which requires more attention, especially as experienced observers are generally preferred for non-invasive genetic sampling. On the other hand, collecting non-target species scats can waste time and project resources, impacting the study’s efficiency (Soller et al., 2020). Laboratory assessment of genetic samples also requires relatively more time and costs compared to camera trapping (Janečka et al., 2011) and researchers have limited access to High-Throughput Sequencing techniques such as SNPs in most developing countries (Krehenwinkel et al., 2019). This unequal distribution of basic facilities and resources required for genetic sampling may cause setbacks to biodiversity monitoring and conservation in the developing world where many species such as large felids are threatened with extinction. Also, as SNPs are biallelic, their per-locus information content is lower than microsatellite markers and therefore, theoretically more SNPs are required to match the resolution microsatellites offer, but this number will vary based on a variety of factors some of which are the locus in question and the genetic variation (Fitak et al., 2016).
DNA degradation can also be problematic when conducting genetic scatology studies in the tropics and arid to semi-arid climates inhabited by jaguars and mountain lions, respectively. Faecal DNA undergoes rapid deterioration in harsh environments due to factors such as high temperatures, heavy rainfall, and ultraviolet light exposure (Wultsch et al., 2014; Fitak et al., 2016). Degraded DNA can adversely affect polymerase chain reaction (PCR) amplification success causing genotyping errors (Wultsch et al., 2014) such as false alleles and allelic dropouts. Genotyping success and error rates are largely based on the primers used for species-specific and individual identification (Wultsch et al., 2014). Additionally, microsatellite loci in snow leopards have low variability (Janecka et al., 2017) thereby limiting its applicability as a universal marker in many research areas concerning the species. Such low microsatellite diversity is typical of felids or other mammalian species whose populations have undergone periods of historic isolation or recent population bottlenecks [see Janecka et al. (2017)]. These challenges should be considered when using genetic sampling to identify individual animals.
Measures to Improve Felid Identification
Clear individual identification is fundamental for appropriate conservation and management of species (Johansson et al., 2020), therefore, we discuss ways to improve current survey methods for obtaining reliable individual identification of big cats. We support the recommendations provided by Wilson and Delahay (2001) that advise practitioners to consider a study’s aim, the target species’ ecology, environmental conditions, and an appropriate level of error associated with a particular survey method used to identify individual animals.
Photography-Based Surveys
At the data collection stage of camera trapping, appropriate placement of camera traps, favourable lighting conditions and making use of scent lures are three ways to improve the quality of photographic data collected. Similarly, to enhance capture rate during direct photographic surveys in large protected areas, call-ups (audio lures) can be used to lure individual felids such as lions toward field teams which can improve individual identification due to the better-quality of digital camera images (Midlane et al., 2015). Identifying photo-captured individuals using at least three time-stable pelage markings, using two to three observers trained in photo-identification techniques for individual identification from images, and honestly reporting unidentified captures (Bahaa-el-din et al., 2016; Alexander et al., 2020; Choo et al., 2020) are some ways to improve matching reliability. Multiple trained observers when independently identifying photo-captured felids can substantially lower the likelihood of misidentification as this prevents observer fatigue and bias (Bahaa-el-din et al., 2016; Choo et al., 2020).
To further assist practitioners in individual identification of felids, feature-based software can be beneficial as these use algorithms to recognise species and individuals from a labelled database of photographic records, such as HotSpotter (Crall et al., 2013), Wild-ID (Bolger et al., 2011) and Extract-Compare (Hiby et al., 2009). For the optimal performance of these tools, the reference image database needs to be sufficiently large without which the algorithm may underperform and incorrectly identify individuals. Additionally, as these programs are sensitive to image quality, DCNN should be trained with about 100 up to 200 images per individual animal with different image resolutions to improve their accuracy of individual recognition (Nipko et al., 2020; Pucci et al., 2020). Also, both the flanks of the animal should be simultaneously photographed and used to train the algorithm as felids have different pelage patterns on their left and right flanks. Although useful, practitioners should be mindful that these programs are not substitutes to human observers but are tools to accelerate individual felid identification by preventing observer fatigue and boredom, because human involvement is still required to confirm image matches.
Genetic Sampling Surveys
During genetic scat analysis, both microsatellite and SNP markers have low degrees of error when identifying individual felids (Janjua et al., 2020). When selecting genetic markers, researchers should consider the preferred level of accuracy, as SNPs have lower information content per locus than microsatellites (Fitak et al., 2016). Despite this limitation, SNPs can identify individuals with greater confidence than microsatellites (Janjua et al., 2020) because of their ability to amplify shorter stretches of DNA (<100 base pairs) thus, enabling efficient genotyping of individuals from degraded faecal DNA (Sollmann et al., 2013a; Fitak et al., 2016). For some species like mountain lions, tissue DNA may be preferred over faecal DNA because when the former is directly collected using biopsy darts the sample is not exposed to degrading environmental factors. Biopsy darting mountain lions may be made easier if trained hounds are used to track individual animals (Beausoleil et al., 2016). Similarly, scat detector dogs can be useful when scat sampling as they have a higher potential to recognise degraded scats than humans, and this can further improve genotyping success rates during subsequent genetic analysis (Wultsch et al., 2014). Such optimised DNA handling and sampling protocols can improve genotyping success rates for more reliable individual identification.
Combination of Survey Methods
Combining survey methods such as camera trapping and genetic sampling surveys (Gopalaswamy et al., 2012; Sollmann et al., 2013a), or with telemetry data from global positioning system (GPS) collars (Murphy et al., 2019) is another way to reduce error rates associated with individual identification of animals. Gopalaswamy et al. (2012) verified that combining camera trap and genetic sampling survey information provided the most precise individual identification of tigers and subsequent density estimates compared to density estimates provided by the two survey methods independently. Such combination methods are useful for improving inferences from sparse datasets which are usually obtained for elusive species. There is much scope for combinations of survey approaches as they significantly improve individual identification by accounting for multiple sources of information.
Rating Survey Methods That Facilitate Large Felid Identification
Our review advises researchers to practice caution when determining their survey method of choice, and we re-emphasise this by providing ratings for each survey method discussed in our review. The required level of experience, costs, reliability, and probability of bias and error are context-specific and therefore, will vary on a situational basis. We arrived at these ratings (Table 1) based on our own experience of using these methods in several published studies, and a review of the literature.
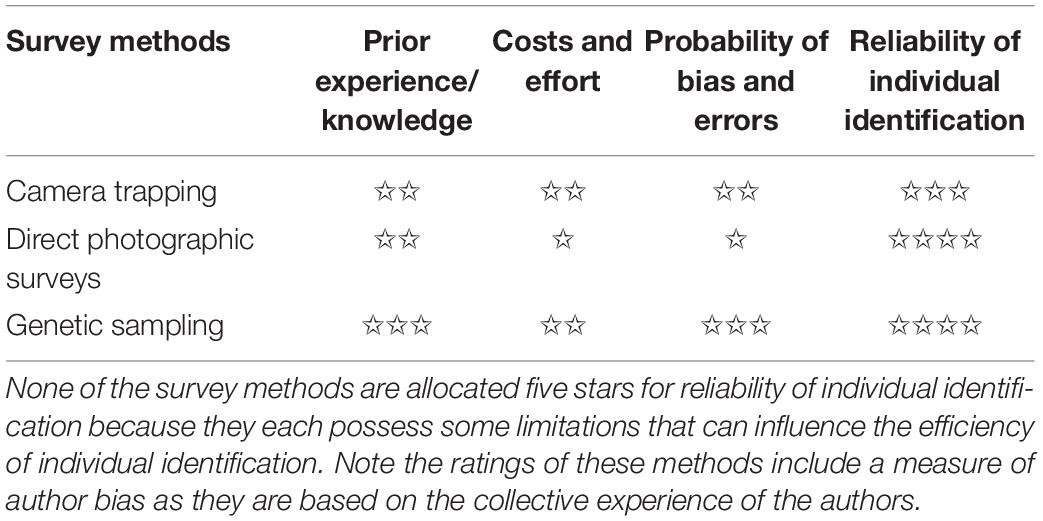
Table 1. General ratings of common survey methods used to identify individual large felids (✩ lowest/cheap–✩✩✩✩✩ highest/expensive).
Camera Trap Surveys
For reliable individual identification using camera trap surveys, persons setting up camera traps in the field and those distinguishing individual animals apart using camera trap images require sufficient training before participating in survey activities (Swann et al., 2011). Camera trap image observers should be trained using a record database of photographs to familiarise themselves with prime identifiable coat markings of target species individuals. Knowledge about the study area, the minimum sampling effort, the population of interest and ideal sites where members of the population would most likely be detected are also prudent to ensure optimal placement of camera traps (Foster and Harmsen, 2012). For instance, when deploying camera traps to study a population of cheetahs, the field team should be familiar with play-tree locations to strategically position camera trap stations nearby. Selectively placing camera traps or using scent lures may artificially introduce sampling bias towards certain individuals and this can affect the accuracy and robustness of subsequent analytic results. However, sampling bias can be reduced by standardising sampling techniques across camera stations, and if unable to completely do so it is advisable to make use of appropriate analytical approaches that adequately address bias and errors (Sollmann et al., 2013b).
While camera trap surveys are generally cost-efficient (Table 1; Green et al., 2020), the bulk of the costs originate from the purchase of camera traps, accessories such as batteries, housing cases and external hard drives (Swann et al., 2011). The costs may vary depending on whether practitioners make use of archived image databases either for portions or the entirety of their study or collect new images. Furthermore, the effort required to conduct camera trap surveys will differ based on the terrain, weather conditions and political environment of the study area (Nawaz et al., 2021). For example, camera trap surveys along ridgelines and cliffs of the snow-covered mountainous regions occupied by snow leopards can be challenging and if not considered can affect research outcomes.
Observer bias and uncertainty may be elevated when camera trapping individuals that lack conspicuous pelage markings and/or if the image sample size is very large (Hiby et al., 2009; Foster and Harmsen, 2012; Alexander et al., 2016). However, with an appropriate study design (see Alexander et al. (2020) for good practises) camera trapping can offer practitioners reliable individual identification of felids, especially those with distinct coat markings (Sollmann et al., 2013b). Contrastingly, the reliability of camera trapping to identify individuals that lack definitive pelage patterns is often debated and therefore, this method is not a recommended approach for identifying individual lions (Braczkowski et al., 2020).
Direct Photographic Surveys
We assign this method the same rating as camera trap surveys because like camera trapping persons involved in direct photographic surveys must undergo adequate training (Table 1). Field observers responsible for photographing felids using digital cameras also require prior understanding about the target species population and the sites where individuals may be found.
Direct photographic surveys are generally cheaper and require less effort as compared to camera trapping and genetic scat sampling surveys (Table 1) but the costs of fuelling vehicles should be factored into any design. Most long-term direct photographic surveys (and camera trap surveys) such as the Serengeti lion study usually incur higher costs as they require full-time staff for tracking and identifying animals daily (Packer et al., 2005) compared to short-term surveys. Additionally, less effort is required for direct photographic surveys as researchers are not required to set up and retrieve field equipment, and digital cameras produce high-resolution images that make individual identification more simplistic. Researchers can further reduce the costs and efforts of this method by making use of citizen-scientists like tourists (Rafiq et al., 2019). Photographic contributions of wildlife tourists offer a reliable and cost-effective way for individual identification. Citizen-science monitoring using direct photographic methods, while inexpensive, can provide findings comparable to traditional camera trap surveys (Rafiq et al., 2019), especially in high tourist density areas such as Ngorongoro (Packer et al., 1991) and Sabi Sand Game Reserve (Balme et al., 2019), where almost every individual in the population is amenable to close-up photography.
The probability of bias and errors are similar to camera trap surveys and hence, have been rated accordingly (Table 1). We have already highlighted the issue of sampling bias associated with direct photographic surveys when call-ups are used (De Blocq, 2014). Similarly, studies conducted using tourist-contributed images may be subjected to biased sampling as tourists may not encounter more elusive individuals of the population. Furthermore, owing to the enhanced image resolution of digital cameras, this survey method is suitable for species with and without distinct pelage markings. Hence, direct photographic surveys are often used to reliably enumerate vibrissae spots of lions for individual identification (Elliot and Gopalaswamy, 2017; Gogoi et al., 2020).
Genetic Sampling Surveys
Non-invasive genetic sampling of scats requires field teams to undertake basic training in scat sampling to ensure they can recognise signs left behind by felids along trails, differentiate between viable and heavily degraded scats, and collect the correct scat samples left behind by species of interest. Similarly, laboratory-based genetic analysis of scats requires researchers to be well-trained in analysis protocols as minor faults may cause genotyping errors (Soller et al., 2020). In general, it is good practice to re-train field and laboratory teams to keep up with advancements in scat sampling and genetic analysis techniques (Soller et al., 2020).
While scat sampling surveys in the field require less costs and effort than camera trap surveys, laboratory equipment may incur higher costs (Janečka et al., 2011). Scat sampling in the field usually can be conducted with basic field equipment for collection and storage of scats. Whereas genetic analysis of scat samples requires trained personnel and access to laboratory facilities, and if no such facility or equipment is available nearby, samples often have to be sent elsewhere for analysis. Additionally, the probability of observer bias and genotyping errors associated with non-invasive genetic sampling is worth noticing due to faecal DNA degradation in harsh environments (Wultsch et al., 2014). Survey time, efforts and costs may increase if experienced researchers are biased when collecting scat samples or if inexperienced researchers lack sufficient judgement required to detect degraded scats (Soller et al., 2020; Zemanova, 2021). However, practitioners can make use of scat detector dogs to locate viable scats only (Wultsch et al., 2014). Thus, despite the challenges of non-invasive sampling of genetic materials, this survey method can provide highly reliable individual identification, especially across larger areas (Janečka et al., 2011; Zemanova, 2021), while also providing additional individual-specific details such as age, health, and diet composition.
Conclusion
We have illustrated that non-invasive methods such as camera trapping and genetic sampling are consistently used for the individual identification of large felids. The emergence of SNP genetic markers and better camera trap equipment are gaining recognition for enabling practitioners to improve their individual identification methods. Direct photographic surveys are another preferred method for monitoring felid species, especially those with less conspicuous identification features. Recent breakthroughs in machine learning have much to offer with their near-fully automated pattern recognition capabilities of large felid images, but these should not be viewed as ultimate substitutes to rigorous data management processes that are usually employed in felid studies. Therefore, while such methodological approaches ensure efficient individual identification that is critical for the understanding of the species ecology and for conservation advancement, they are associated with few inherent challenges and limitations.
Lastly, we have discussed the importance of adopting appropriate survey (and analytical) approaches that are based on thorough consideration of target species ecology, study aims, location and required levels of accuracy and efficiency (Wilson and Delahay, 2001). To collect reliable individual identification data, rigour should be maintained when identifying individuals from camera trap images by using multiple human observers and reporting unidentified captures (Alexander et al., 2020; Choo et al., 2020), and the use of Microsoft Excel should be avoided when managing genetic sampling data to prevent errors (Ziemann et al., 2016). We therefore recommend that future studies requiring individual identification of large felids value and consider such rigorous data management approaches and take advantage of emerging methods that can together help ensure credibility of the inferences drawn to establish a reliable basis for large felid conservation and management.
Author Contributions
KP and AB conceived the idea. KP, AB, LG, DS, and DB co-wrote the manuscript. All authors contributed to the article and approved the submitted version.
Conflict of Interest
DS was employed by the company Environmental Foundation Limited.
The remaining authors declare that the research was conducted in the absence of any commercial or financial relationships that could be construed as a potential conflict of interest.
Publisher’s Note
All claims expressed in this article are solely those of the authors and do not necessarily represent those of their affiliated organizations, or those of the publisher, the editors and the reviewers. Any product that may be evaluated in this article, or claim that may be made by its manufacturer, is not guaranteed or endorsed by the publisher.
Acknowledgments
We thank Dibesh Karmacharya of the Center for Molecular Dynamics -Nepal for his advice regarding genetic scat sampling.
References
Alexander, J. S., Johansson, O., Sharma, K., Durbach, I., and Borchers, D. (2020). Snow Leopard Identification Good Practices. Available online at: https://globalsnowleopard.org/wp-content/uploads/2020/10/Best-practices-for-Individual-ID.pdf (accessed December 23, 2021).
Alexander, J. S., Zhang, C., Shi, K., and Riordan, P. (2016). A granular view of a snow leopard population using camera traps in Central China. Biol. Conservation 197, 27–31. doi: 10.1016/j.biocon.2016.02.023
Alexander, P. D., and Gese, E. M. (2018). Identifying individual cougars (Puma concolor) in remote camera images–implications for population estimates. Wildlife Res. 45, 274–281. doi: 10.1071/WR17044
Bahaa-el-din, L., Sollmann, R., Hunter, L. T., Slotow, R., Macdonald, D. W., and Henschel, P. (2016). Effects of human land-use on Africa’s only forest-dependent felid: the African golden cat Caracal aurata. Biol. Conservation 199, 1–9. doi: 10.1016/j.biocon.2016.04.013
Balme, G. A., Hunter, L. T., Goodman, P., Ferguson, H., Craigie, J., and Slotow, R. (2010). “An adaptive management approach to trophy hunting of leopards Panthera pardus: a case study from KwaZulu-Natal, South Africa,” in Biology and Conservation of Wild Felids, eds D. W. Macdonald and A. Loveridge (Oxford: Oxford University Press).
Balme, G. A., Hunter, L., and Braczkowski, A. R. (2012). Applicability of age-based hunting regulations for African leopards. PLoS One 7:e35209. doi: 10.1371/journal.pone.0035209
Balme, G. A., Pitman, R. T., Robinson, H. S., Miller, J. R., Funston, P. J., and Hunter, L. T. (2017). Leopard distribution and abundance is unaffected by interference competition with lions. Behav. Ecol. 28, 1348–1358. doi: 10.1093/beheco/arx098
Balme, G., Rogan, M., Thomas, L., Pitman, R., Mann, G., Whittington-Jones, G., et al. (2019). Big cats at large: density, structure, and spatio-temporal patterns of a leopard population free of anthropogenic mortality. Population Ecol. 61, 256–267. doi: 10.1002/1438-390X.1023
Beausoleil, R. A., Clark, J. D., and Maletzke, B. T. (2016). A long-term evaluation of biopsy darts and DNA to estimate cougar density: an agency-citizen science collaboration. Wildlife Soc. Bull. 40, 583–592. doi: 10.1002/wsb.675
Beukes, B. O., Radloff, F. G., and Ferreira, S. M. (2017). Estimating African lion abundance in the southwestern Kgalagadi Transfrontier Park. African J. Wildlife Res. 47, 10–23. doi: 10.3957/056.047.0010
Bhatt, S., Biswas, S., Karanth, K., Pandav, B., and Mondol, S. (2020). Genetic analyses reveal population structure and recent decline in leopards (Panthera pardus fusca) across the Indian subcontinent. PeerJ 8:e8482. doi: 10.7717/peerj.8482
Blackburn, S., Hopcraft, J. G. C., Ogutu, J. O., Matthiopoulos, J., and Frank, L. (2016). Human–wildlife conflict, benefit sharing and the survival of lions in pastoralist community-based conservancies. J. Appl. Ecol. 53, 1195–1205. doi: 10.1111/1365-2664.12632
Bolger, D. T., Vance, B., Morrison, T. A., and Farid, H. (2011). Wild ID User Guide: Pattern Extraction and Matching Software for Computer-assisted Photographic Mark-recapture Analysis. Hanover, NH: Dartmouth College.
Braczkowski, A., Gopalaswamy, A. M., Nsubuga, M., Allan, J., Biggs, D., and Maron, M. (2020). Detecting early warnings of pressure on an African lion (Panthera leo) population in the Queen Elizabeth Conservation Area. Uganda. Ecol. Solutions Evidence 1:e12015. doi: 10.1002/2688-8319.12015
Brassine, E., and Parker, D. (2015). Trapping elusive cats: using intensive camera trapping to estimate the density of a rare African felid. PLoS One 10:e0142508. doi: 10.1371/journal.pone.0142508
Broekhuis, F., and Gopalaswamy, A. M. (2016). Counting cats: spatially explicit population estimates of cheetah (Acinonyx jubatus) using unstructured sampling data. PLoS One 11:e0153875. doi: 10.1371/journal.pone.0153875
Busby, G. B., Gottelli, D., Wacher, T., Marker, L., Belbachir, F., De Smet, K., et al. (2009). Genetic analysis of scat reveals leopard Panthera pardus and cheetah Acinonyx jubatus in southern Algeria. Oryx 43, 412–415. doi: 10.1017/S0030605309001197
Cheema, G. S., and Anand, S. (2017). “Automatic detection and recognition of individuals in patterned species,” in Proceedings of the Joint European Conference on Machine Learning and Knowledge Discovery in Databases, (Cham: Springer), 27–38. doi: 10.1007/978-3-319-71273-4_3
Chetri, M. (2018). Ecology of Snow Leopards in the Central Himalayas, Nepal. Available online at: http://hdl.handle.net/11250/2572228 (accessed November 25, 2021).
Choo, Y. R., Kudavidanage, E. P., Amarasinghe, T. R., Nimalrathna, T., Chua, M. A., and Webb, E. L. (2020). Best practices for reporting individual identification using camera trap photographs. Global Ecol. Conservation 24:e01294. doi: 10.1016/j.gecco.2020.e01294
Corcoran, E., Denman, S., Hanger, J., Wilson, B., and Hamilton, G. (2019). Automated detection of koalas using low-level aerial surveillance and machine learning. Sci. Rep. 9:3208. doi: 10.1038/s41598-019-39917-5
Crall, J. P., Stewart, C. V., Berger-Wolf, T. Y., Rubenstein, D. I., and Sundaresan, S. R. (2013). “Hotspotter—patterned species instance recognition,” in Proceedings of the 2013 IEEE Workshop on Applications of Computer Vision (WACV), (Piscataway, NJ: IEEE), 230–237.
Curry, C. J., White, P. A., and Derr, J. N. (2019). Genetic analysis of African lions (Panthera leo) in Zambia support movement across anthropogenic and geographical barriers. PLoS One 14:e0217179. doi: 10.1371/journal.pone.0217179
Curtin, B. H., and Matthews, S. J. (2019). “Deep learning for inexpensive image classification of wildlife on the Raspberry Pi,” in Proceedings of the 2019 IEEE 10th Annual Ubiquitous Computing, Electronics & Mobile Communication Conference (UEMCON), (Piscataway, NJ: IEEE), doi: 10.1109/UEMCON47517.2019.8993061
Cusack, J. J., Swanson, A., Coulson, T., Packer, C., Carbone, C., Dickman, A. J., et al. (2015). Applying a random encounter model to estimate lion density from camera traps in Serengeti National Park. Tanzania. J. Wildlife Manag. 79, 1014–1021. doi: 10.1002/jwmg.902
De Blocq, A. D. (2014). Estimating Spotted Hyaena (Crocuta crocuta) Population Density Using Camera Trap Data in a Spatially-explicit Capture-recapture Framework. Bachelor’s thesis, Cape Town: University of Cape Town.
Devens, C., Tshabalala, T., McManus, J., and Smuts, B. (2018). Counting the spots: the use of a spatially explicit capture–recapture technique and GPS data to estimate leopard (Panthera pardus) density in the Eastern and Western Cape. South Africa. African J. Ecol. 56, 850–859. doi: 10.1111/aje.12512
Donnelly, A. (2020). A Comparative Analysis of Felid Conservation Strategies on four Continents: Can one size fit all?. Doctoral dissertation, Salem, VA: Roanoke College.
Elliot, N. B., and Gopalaswamy, A. M. (2017). Toward accurate and precise estimates of lion density. Conservation Biol. 31, 934–943. doi: 10.1111/cobi.12878
Elliot, N. B., Bett, A., Chege, M., Sankan, K., de Souza, N., Kariuki, L., et al. (2020). The importance of reliable monitoring methods for the management of small, isolated populations. Conservation Sci. Practice 2:e217. doi: 10.1111/csp2.217
Fitak, R. R., Naidu, A., Thompson, R. W., and Culver, M. (2016). A new panel of SNP markers for the individual identification of North American pumas. J. Fish Wildlife Manag. 7, 13–27. doi: 10.3996/112014-JFWM-080
Foster, R. J., and Harmsen, B. J. (2012). A critique of density estimation from camera-trap data. J. Wildlife Manag. 76, 224–236. doi: 10.1002/jwmg.275
Garrote, G., de Ayala, R. P., Álvarez, A., Martín, J. M., Ruiz, M., de Lillo, S., et al. (2021). Improving the random encounter model method to estimate carnivore densities using data generated by conventional camera-trap design. Oryx 55, 99–104. doi: 10.1017/S0030605318001618
Gogoi, K., Kumar, U., Banerjee, K., and Jhala, Y. V. (2020). Spatially explicit density and its determinants for Asiatic lions in the Gir forests. PLoS One 15:e0228374. doi: 10.1371/journal.pone.0228374
Gopalaswamy, A. M., Karanth, K. U., Delampady, M., and Stenseth, N. C. (2019). How sampling-based overdispersion reveals India’s tiger monitoring orthodoxy. Conservation Sci. Practice 1:e128. doi: 10.1111/csp2.128
Gopalaswamy, A. M., Royle, J. A., Delampady, M., Nichols, J. D., Karanth, K. U., and Macdonald, D. W. (2012). Density estimation in tiger populations: combining information for strong inference. Ecology 93, 1741–1751. doi: 10.1890/11-2110.1
Gray, T. N., Grainger, M. J., and Grosu, R. (2020). Conservation decision-making under uncertainty: identifying when to reintroduce tiger Panthera tigris to Cambodia. Conservation Sci. Practice 2:e187. doi: 10.1111/csp2.187
Green, A. M., Chynoweth, M. W., and Şekercioğlu, ÇH. (2020). Spatially explicit capture-recapture through camera trapping: a review of benchmark analyses for wildlife density estimation. Front. Ecol. Evol. 8:563477. doi: 10.3389/fevo.2020.563477
Greenspan, E., Anile, S., and Nielsen, C. K. (2020). Density of wild felids in Sonora, Mexico: a comparison of spatially explicit capture-recapture methods. Eur. J. Wildlife Res. 66, 1–12. doi: 10.1007/s10344-020-01401-1
Hamilton, M. (2017). Saving Snow Leopards with Deep Learning and Computer Vision on Spark. Available online at: https://customers.microsoft.com/en-us/story/snow-leopard-trust-nonprofit-azure (accessed on 7 November, 2021).
Harihar, A., Lahkar, D., Singh, A., Das, S. K., Ahmed, M. F., and Begum, R. H. (2021). Population density modelling of mixed polymorphic phenotypes: an application of spatial mark-resight models. Animal Conservation 24, 709–716. doi: 10.1111/acv.12677
Harmsen, B. J., Foster, R. J., and Quigley, H. (2020). Spatially explicit capture recapture density estimates: robustness, accuracy and precision in a long-term study of jaguars (Panthera onca). PLoS One 15:e0227468. doi: 10.1371/journal.pone.0227468
Hedges, L., Lam, W. Y., Campos-Arceiz, A., Rayan, D. M., Laurance, W. F., Latham, C. J., et al. (2015). Melanistic leopards reveal their spots: infrared camera traps provide a population density estimate of leopards in Malaysia. J. Wildlife Manag. 79, 846–853. doi: 10.1002/jwmg.901
Hiby, L., Lovell, P., Patil, N., Kumar, N. S., Gopalaswamy, A. M., and Karanth, K. U. (2009). A tiger cannot change its stripes: using a three-dimensional model to match images of living tigers and tiger skins. Biol. Lett. 5, 383–386. doi: 10.1098/rsbl.2009.0028
Jackson, R. M., Roe, J. D., Wangchuk, R., and Hunter, D. O. (2006). Estimating snow leopard population abundance using photography and capture-recapture techniques. Wildlife Soc. Bull. 34, 772–781. doi: 10.1890/0012-9658(2006)87[2925:atpdup]2.0.co;2
Janečka, J. E., Jackson, R., Yuquang, Z., Diqiang, L., Munkhtsog, B., Buckley-Beason, V., et al. (2008). Population monitoring of snow leopards using noninvasive collection of scat samples: a pilot study. Animal Conservation 11, 401–411. doi: 10.1111/j.1469-1795.2008.00195.x
Janečka, J. E., Munkhtsog, B., Jackson, R. M., Naranbaatar, G., Mallon, D. P., and Murphy, W. J. (2011). Comparison of noninvasive genetic and camera-trapping techniques for surveying snow leopards. J. Mammal. 92, 771–783. doi: 10.1644/10-MAMM-A-036.1
Janecka, J. E., Zhang, Y., Li, D., Munkhtsog, B., Bayaraa, M., Galsandorj, N., et al. (2017). Range-wide snow leopard phylogeography supports three subspecies. J. Heredity 108, 597–607. doi: 10.1093/jhered/esx044
Janjua, S., Peters, J. L., Weckworth, B., Abbas, F. I., Bahn, V., Johansson, O., et al. (2020). Improving our conservation genetic toolkit: ddRAD-seq for SNPs in snow leopards. Conservation Genetics Resources 12, 257–261. doi: 10.1007/s12686-019-01082-2
Jhala, Y. V., Banerjee, K., Chakrabarti, S., Basu, P., Singh, K., Dave, C., et al. (2019). Asiatic lion: ecology, economics, and politics of conservation. Front. Ecol. Evol. 7:312. doi: 10.3389/fevo.2019.00312
Jhala, Y., Gopal, R., Mathur, V., Ghosh, P., Negi, H. S., Narain, S., et al. (2021). Recovery of tigers in India: critical introspection and potential lessons. People Nature 3, 281–293. doi: 10.1002/pan3.10177
Johansson, Ö, Samelius, G., Wikberg, E., Chapron, G., Mishra, C., and Low, M. (2020). Identification errors in camera-trap studies result in systematic population overestimation. Sci. Rep. 10:6393. doi: 10.1038/s41598-020-63367-z
Joshi, A. R., Dinerstein, E., Wikramanayake, E., Anderson, M. L., Olson, D., Jones, B. S., et al. (2016). Tracking changes and preventing loss in critical tiger habitat. Sci. Adv. 2:e1501675. doi: 10.1126/sciadv.1501675
Kane, M. D., Morin, D. J., and Kelly, M. J. (2015). Potential for camera-traps and spatial mark-resight models to improve monitoring of the critically endangered West African lion (Panthera leo). Biodiversity Conservation 24, 3527–3541. doi: 10.1007/s10531-015-1012-7
Karanth, K. U. (1995). Estimating tiger Panthera tigris populations from camera-trap data using capture—recapture models. Biol. Conservation 71, 333–338. doi: 10.1016/0006-3207(94)00057-W
Karanth, K. U. (2011). India’s Tiger Counts: the Long March to Reliable Science. Mumbai: Economic and Political Weekly.
Karanth, K. U., and Nichols, J. D. (1998). Estimation of tiger densities in India using photographic captures and recaptures. Ecology 79, 2852–2862. doi: 10.1890/0012-9658(1998)079[2852:eotdii]2.0.co;2
Karanth, K. U., and Nichols, J. D. (2011). “Estimating tiger abundance from camera trap data: field surveys and analytical issues,” in Camera Traps in Animal Ecology, ed. A. F. O’Connell (Tokyo: Springer), 97–117. doi: 10.1007/978-4-431-99495-4_7
Karanth, K. U., Nichols, J. D., Seidenstricker, J., Dinerstein, E., Smith, J. L. D., McDougal, C., et al. (2003). Science deficiency in conservation practice: the monitoring of tiger populations in India. Anim. Conserv. Forum 6, 141–146. doi: 10.1017/S1367943003003184
Karmacharya, D. B., Thapa, K., Shrestha, R., Dhakal, M., and Janecka, J. E. (2011). Noninvasive genetic population survey of snow leopards (Panthera uncia) in Kangchenjunga conservation area, Shey Phoksundo National Park and surrounding buffer zones of Nepal. BMC Res. Notes 4:516. doi: 10.1186/1756-0500-4-516
Kelly, M. J., Noss, A. J., Di Bitetti, M. S., Maffei, L., Arispe, R. L., Paviolo, A., et al. (2008). Estimating puma densities from camera trapping across three study sites: bolivia, Argentina, and Belize. J. Mammal. 89, 408–418. doi: 10.1644/06-MAMM-A-424R.1
Kissui, B. M., Mosser, A., and Packer, C. (2010). Persistence and local extinction of lion prides in the Ngorongoro Crater, Tanzania. Population Ecol. 52, 103–111. doi: 10.1007/s10144-009-0176-y
Krehenwinkel, H., Pomerantz, A., and Prost, S. (2019). Genetic biomonitoring and biodiversity assessment using portable sequencing technologies: current uses and future directions. Genes 10:858. doi: 10.3390/genes10110858
Kuningas, S., Similä, T., and Hammond, P. S. (2014). Population size, survival and reproductive rates of northern Norwegian killer whales (Orcinus orca) in 1986–2003. J. Mar. Biol. Assoc. U K. 94, 1277–1291. doi: 10.1017/S0025315413000933
Kusler, A., Jordan, N. R., McNutt, J. W., and Broekhuis, F. (2019). Cheetah marking trees: distribution, visitation, and behavior. African J. Ecol. 57, 419–422. doi: 10.1111/aje.12602
Magliolo, M., Prost, S., Orozco-terWengel, P., Burger, P., Kropff, A. S., Kotze, A., et al. (2021). Unlocking the potential of a validated single nucleotide polymorphism array for genomic monitoring of trade in cheetahs (Acinonyx jubatus). Mol. Biol. Rep. 48, 171–181. doi: 10.1007/s11033-020-06030-0
Marnewick, K., Funston, P. J., and Karanth, K. U. (2008). Evaluating camera trapping as a method for estimating cheetah abundance in ranching areas. South African J. Wildlife 38, 59–65. doi: 10.3957/0379-4369-38.1.59
Mattioli, L., Canu, A., Passilongo, D., Scandura, M., and Apollonio, M. (2018). Estimation of pack density in grey wolf (Canis lupus) by applying spatially explicit capture-recapture models to camera trap data supported by genetic monitoring. Front. Zool. 15:38. doi: 10.1186/s12983-018-0281-x
Midlane, N., O’riain, M. J., Balme, G. A., and Hunter, L. T. (2015). To track or to call: comparing methods for estimating population abundance of African lions Panthera leo in Kafue National Park. Biodiversity Conservation 24, 1311–1327. doi: 10.1007/s10531-015-0858-z
Miotto, R. A., Cervini, M., Kajin, M., Begotti, R. A., and Galetti, P. M. (2014). Estimating puma Puma concolor population size in a human-disturbed landscape in Brazil, using DNA mark–recapture data. Oryx 48, 250–257. doi: 10.1017/S0030605312000841
Miththapala, S., Seidensticker, J., Phillips, L. G., Fernando, S. B. U., and Smallwood, J. A. (1989). Identification of individual leopards (Panthera pardus kotiya) using spot pattern variation. J. Zool. 218, 527–536. doi: 10.1111/j.1469-7998.1989.tb04996.x
Mondol, S., Karanth, K. U., Kumar, N. S., Gopalaswamy, A. M., Andheria, A., and Ramakrishnan, U. (2009). Evaluation of non-invasive genetic sampling methods for estimating tiger population size. Biol. Conservation 142, 2350–2360. doi: 10.1016/j.biocon.2009.05.014
Murphy, S. M., Wilckens, D. T., Augustine, B. C., Peyton, M. A., and Harper, G. C. (2019). Improving estimation of puma (Puma concolor) population density: clustered camera-trapping, telemetry data, and generalized spatial mark-resight models. Sci. Rep. 9:4590. doi: 10.1038/s41598-019-40926-7
Nawaz, M. A., Khan, B. U., Mahmood, A., Younas, M., and Sutherland, C. (2021). An empirical demonstration of the effect of study design on density estimations. Sci. Rep. 11:13104. doi: 10.1038/s41598-021-92361-2
Negrões, N., Sarmento, P., Cruz, J., Eira, C., Revilla, E., Fonseca, C., et al. (2010). Use of camera-trapping to estimate puma density and influencing factors in central Brazil. J. Wildlife Manag. 74, 1195–1203. doi: 10.1111/j.1937-2817.2010.tb01240.x
Nipko, R. B., Holcombe, B. E., and Kelly, M. J. (2020). Identifying individual jaguars and ocelots via pattern-recognition software: comparing hotspotter and Wild-ID. Wildlife Soc. Bull. 44, 424–433. doi: 10.1002/wsb.1086
Noss, A. J., Gardner, B., Maffei, L., Cuéllar, E., Montaño, R., Romero-Muñoz, A., et al. (2012). Comparison of density estimation methods for mammal populations with camera traps in the K aa-I ya del G ran C haco landscape. Animal Conservation 15, 527–535. doi: 10.1111/j.1469-1795.2012.00545.x
O’Bryan, C. J., Braczkowski, A. R., Beyer, H. L., Carter, N. H., Watson, J. E., and McDonald-Madden, E. (2018). The contribution of predators and scavengers to human well-being. Nat. Ecol. Evol. 2, 229–236. doi: 10.1038/s41559-017-0421-2
Packer, C., and Pusey, A. E. (1993). Should a lion change its spots? Nature 362, 595–595. doi: 10.1038/362595a0
Packer, C., Brink, H., Kissui, B. M., Maliti, H., Kushnir, H., and Caro, T. (2011). Effects of trophy hunting on lion and leopard populations in Tanzania. Conservation Biol. 25, 142–153. doi: 10.1111/j.1523-1739.2010.01576.x
Packer, C., Hilborn, R., Mosser, A., Kissui, B., Borner, M., Hopcraft, G., et al. (2005). Ecological change, group territoriality, and population dynamics in Serengeti lions. Science 307, 390–393. doi: 10.1126/science.1105122
Packer, C., Loveridge, A., Canney, S., Caro, T., Garnett, S. T., Pfeifer, M., et al. (2013). Conserving large carnivores: dollars and fence. Ecol. Lett. 16, 635–641. doi: 10.1111/ele.12091
Packer, C., Pusey, A. E., Rowley, H., Gilbert, D. A., Martenson, J., and O’BRIEN, S. J. (1991). Case study of a population bottleneck: lions of the Ngorongoro Crater. Conservation Biol. 5, 219–230. doi: 10.1111/j.1523-1739.1991.tb00127.x
Pennycuick, C. J., and Rudnai, J. (1970). A method of identifying individual lions Panthera leo with an analysis of the reliability of identification. J. Zool. 160, 497–508. doi: 10.1111/j.1469-7998.1970.tb03093.x
Pucci, R., Shankaraiah, J., Jathanna, D., Karanth, U., and Subr, K. (2020). WhoAmI: an automatic tool for visual recognition of tiger and leopard individuals in the wild. arXiv [preprint] doi: 10.48550/arXiv.2006.09962
Rafiq, K., Bryce, C. M., Rich, L. N., Coco, C., Miller, D. A., Meloro, C., et al. (2019). Tourist photographs as a scalable framework for wildlife monitoring in protected areas. Curr. Biol. 29, R681–R682. doi: 10.1016/j.cub.2019.05.056
Rich, L. N., Kelly, M. J., Sollmann, R., Noss, A. J., Maffei, L., Arispe, R. L., et al. (2014). Comparing capture-recapture, mark-resight, and spatial mark-resight models for estimating puma densities via camera traps. J. Mammal. 95, 382–391. doi: 10.1644/13-MAMM-A-126
Ripple, W. J., Estes, J. A., Beschta, R. L., Wilmers, C. C., Ritchie, E. G., Hebblewhite, M., et al. (2014). Status and ecological effects of the world’s largest carnivores. Science 343:1241484. doi: 10.1126/science.1241484
Roques, S., Furtado, M., Jácomo, A. T., Silveira, L., Sollmann, R., Torres, N. M., et al. (2014). Monitoring jaguar populations Panthera onca with non-invasive genetics: a pilot study in Brazilian ecosystems. Oryx 48, 361–369. doi: 10.1017/S0030605312001640
Sharma, K., Bayrakcismith, R., Tumursukh, L., Johansson, O., Sevger, P., McCarthy, T., et al. (2014). Vigorous dynamics underlie a stable population of the endangered snow leopard Panthera uncia in Tost Mountains, South Gobi, Mongolia. PLoS One 9:e101319. doi: 10.1371/journal.pone.0101319
Shi, C., Liu, D., Cui, Y., Xie, J., Roberts, N. J., and Jiang, G. (2020). Amur tiger stripes: individual identification based on deep convolutional neural network. Int. Zool. 15, 461–470. doi: 10.1111/1749-4877.1245
Silveira, L., Jácomo, A. T., Astete, S., Sollmann, R., Tôrres, N. M., Furtado, M. M., et al. (2010). Density of the Near Threatened jaguar Panthera onca in the caatinga of north-eastern Brazil. Oryx 44, 104–109. doi: 10.1017/S0030605309990433
Snow Leopard Trust (2019). How AI Helps us Understand & Protect Snow Leopards. Available online at: https://snowleopard.org/how-ai-helps-us-understand-protect-snow-leopards/ (Accessed 7 November, 2021).
Soisalo, M. K., and Cavalcanti, S. M. (2006). Estimating the density of a jaguar population in the Brazilian Pantanal using camera-traps and capture–recapture sampling in combination with GPS radio-telemetry. Biol. Conservation 129, 487–496. doi: 10.1016/j.biocon.2005.11.023
Soller, J. M., Ausband, D. E., and Szykman Gunther, M. (2020). The curse of observer experience: error in noninvasive genetic sampling. PLoS One 15:e0229762. doi: 10.1371/journal.pone.0229762
Sollmann, R., Tôrres, N. M., Furtado, M. M., de Almeida Jácomo, A. T., Palomares, F., Roques, S., et al. (2013a). Combining camera-trapping and noninvasive genetic data in a spatial capture–recapture framework improves density estimates for the jaguar. Biol. Conservation 167, 242–247. doi: 10.1016/j.biocon.2013.08.003
Sollmann, R., Mohamed, A., and Kelly, M. J. (2013b). Camera trapping for the study and conservation of tropical carnivores. Raffles Bull. Zool. 28, 21–42.
Suryawanshi, K. R., Khanyari, M., Sharma, K., Lkhagvajav, P., and Mishra, C. (2019). Sampling bias in snow leopard population estimation studies. Population Ecol. 61, 268–276. doi: 10.1002/1438-390X.1027
Swann, D. E., Kawanishi, K., and Palmer, J. (2011). “Evaluating types and features of camera traps in ecological studies: a guide for researchers,” in Camera Traps in Animal Ecology, eds A. F. O’Connell, J. D. Nichols, and K. U. Karanth (Tokyo: Springer). doi: 10.1007/978-4-431-99495-4_3
Tariq, N., Saleem, K., Mushtaq, M., and Nawaz, M. A. (2018). “Snow leopard recognition using deep convolution neural network,” in Proceedings of the 2nd International Conference on Information System and Data Mining, (Lakeland, FL), doi: 10.1145/3206098.3206114
Tempa, T., Hebblewhite, M., Goldberg, J. F., Norbu, N., Wangchuk, T. R., Xiao, W., et al. (2019). The spatial distribution and population density of tigers in mountainous terrain of Bhutan. Biol. Conservation 238:108192. doi: 10.1016/j.biocon.2019.07.037
Thapa, K., Manandhar, S., Bista, M., Shakya, J., Sah, G., Dhakal, M., et al. (2018). Assessment of genetic diversity, population structure, and gene flow of tigers (Panthera tigris tigris) across Nepal’s Terai Arc Landscape. PLoS One 13:e0193495. doi: 10.1371/journal.pone.0193495
Thuo, D. N. (2021). Application of Recent Developments of Molecular Tools in the Conservation of Cheetahs. Bruce ACT: University of Canberra.
Tortato, F. R., Izzo, T. J., Hoogesteijn, R., and Peres, C. A. (2017). The numbers of the beast: valuation of jaguar (Panthera onca) tourism and cattle depredation in the Brazilian Pantanal. Global Ecol. Conservation 11, 106–114. doi: 10.1016/j.gecco.2017.05.003
Tumenta, P. N., Kok, J. S., Van Rijssel, J. C., Buij, R., Croes, B. M., Funston, P. J., et al. (2010). Threat of rapid extermination of the lion (Panthera leo leo) in Waza National Park, Northern Cameroon. African J. Ecol. 48, 888–894. doi: 10.1111/j.1365-2028.2009.01181.x
Wilson, G. J., and Delahay, R. J. (2001). A review of methods to estimate the abundance of terrestrial carnivores using field signs and observation. Wildlife Res. 28, 151–164. doi: 10.1071/WR00033
Wultsch, C., Waits, L. P., and Kelly, M. J. (2014). Noninvasive individual and species identification of jaguars (Panthera onca), pumas (Puma concolor) and ocelots (Leopardus pardalis) in Belize, Central America using cross-species microsatellites and faecal DNA. Mol. Ecol. Resources 14, 1171–1182. doi: 10.1111/1755-0998.12266
Zemanova, M. A. (2021). Noninvasive genetic assessment is an effective wildlife research tool when compared with other approaches. Genes 12:1672. doi: 10.3390/genes12111672
Keywords: camera traps, direct photographic surveys, genetic sampling, individual identification, large felids
Citation: Pereira KS, Gibson L, Biggs D, Samarasinghe D and Braczkowski AR (2022) Individual Identification of Large Felids in Field Studies: Common Methods, Challenges, and Implications for Conservation Science. Front. Ecol. Evol. 10:866403. doi: 10.3389/fevo.2022.866403
Received: 31 January 2022; Accepted: 28 March 2022;
Published: 22 April 2022.
Edited by:
Mathew Samuel Crowther, The University of Sydney, AustraliaReviewed by:
Craig Packer, University of Minnesota Twin Cities, United StatesCopyright © 2022 Pereira, Gibson, Biggs, Samarasinghe and Braczkowski. This is an open-access article distributed under the terms of the Creative Commons Attribution License (CC BY). The use, distribution or reproduction in other forums is permitted, provided the original author(s) and the copyright owner(s) are credited and that the original publication in this journal is cited, in accordance with accepted academic practice. No use, distribution or reproduction is permitted which does not comply with these terms.
*Correspondence: Luke Gibson, YmlvZGl2ZXJzaXR5QHN1c3RlY2guZWR1LmNu