- 1School of Mathematics, Hefei University of Technology, Hefei, China
- 2Centre for Invasion Biology, Department of Mathematical Sciences, Stellenbosch University, Stellenbosch, South Africa
- 3African Institute for Mathematical Sciences, Stellenbosch, South Africa
- 4International Initiative for Theoretical Ecology, London, United Kingdom
Ecological communities are composed of different functional guilds that are engaging in multiple types of biotic interactions. We explore how ecological networks fare when confronting infectious diseases according to density-dependent (DD) and frequency-dependent (FD) transmission modes. Our model shows that network compositions can dictate both disease spreading and the relationship between disease and community diversity (including species richness and Shannon’s diversity) as depicted in the dilution effect. The disease becomes more prevalent within communities harboring more mutualistic interactions, generating a positive relationship between disease prevalence and community diversity (i.e., an amplification effect). By contrast, in communities with a fixed proportion of mutualistic interactions, higher diversity from the balance of competition and predation can impede disease prevalence (i.e., the dilution effect). Within-species disease prevalence increases linearly with a species’ degree centrality. These patterns of disease transmission and the diversity-disease relationship hold for both transmission modes. Our analyses highlight the complex effects of interaction compositions in ecological networks on infectious disease dynamics and further advance the debate on the dilution effect of host diversity on disease prevalence.
Introduction
Biotic interactions between species (e.g., trophic and mutualistic), essential for maintaining biodiversity, can steer how pathogens are transmitted and spread in ecological communities (Thébault and Fontaine, 2010; Figueroa et al., 2020). Infectious diseases can often be transmitted within- or cross-species in host communities and, consequently, the composition and the interaction network structure of host communities can strongly influence the disease dynamics (Bowers and Turner, 1997; Rudolf and Antonovics, 2005; Keesing et al., 2010; Salkeld et al., 2013; Johnson et al., 2015; Frainer et al., 2018; Hoi et al., 2020). In the past two decades there have been mounting interests and advances in understanding disease dynamics and the relationship between host community diversity and disease prevalence (LoGiudice et al., 2003; Dobson, 2004; Salkeld et al., 2013; Civitello et al., 2015; Johnson et al., 2015; Huang et al., 2016; Young et al., 2017; Frainer et al., 2018). Meta-analyses have shown that the diversity-disease relationship can be contextual and may depend on many factors, including the architectures of embedded ecological networks within host communities (Salkeld et al., 2013; Rohr et al., 2020) and the transmission mode of the infectious pathogens (Rudolf and Antonovics, 2005; Hatcher et al., 2012). In particular, changes in community composition and interaction network structures can alter the dilution effect, positing that species-rich communities can impede pathogen transmission and dilute disease prevalence (Randolph and Dobson, 2012; O’Regan et al., 2015; Rohr et al., 2015).
Evidence is mounting to show that networks of biotic interactions in communities, typically including competitive, trophic and mutualistic interactions, can profoundly influence community stability and the emerged network architecture (Thébault and Fontaine, 2010; Mougi and Kondoh, 2012; Lurgi et al., 2016; García-Callejas et al., 2018a,b; Qian and Akçay, 2020). Depending on the diversity and composition of different interaction types, infectious diseases could become more prevalent, affecting disproportionately particular functional guilds of a community and consequently creating complex feedbacks on network structures (Keesing et al., 2010; Frainer et al., 2018). For instance, multihost pathogenic diseases can alter direct resource competition between two host species into apparent competition, where a disease outbreak in one host may facilitate pathogens to spill over to the other host species and thus foster the disease spreading across the community (Begon et al., 1999; O’Regan et al., 2015). Disease infections could modify trophic interactions among host species, inhibiting or boosting pathogen transmission under specific ecological context (Packer et al., 2003; Hatcher et al., 2006; Rohr et al., 2015; Su et al., 2020). Structural changes in mutualistic networks can also shape pathogen transmission, with increased connectance from mutualistic interactions diluting the likelihood of disease outbreak (Figueroa et al., 2020). Furthermore, species are often engaging in multiple types of interactions simultaneously and form a complex network; such multiple functional roles a species plays can diversify the possible routes of disease transmission from one species to another. For instance, insect herbivores (e.g., aphids) are often vectors of plant pathogens, while themselves also partake multiple interaction types with a wide range of species such as competitors (caterpillars), predators (lady beetles) and mutualists (ants), which can have predictable effects on the spread of plant pathogens (Salkeld et al., 2013; Clark et al., 2019; Crowder et al., 2019). These studies, thus, highlight the multifarious impacts from the complexity of multiple functional guilds in a community to disease dynamics. Evidently, the structure and composition of the embedded network can affect disease transmission in a community, while disease transmission also alters the structure and composition of these ecological networks.
The way in which pathogens are spilled over to different host species and functional guilds could drastically affect the dynamics and prevalence of the disease in host communities. Transmission mode depicts the function that relates the transmission rate to the densities of susceptible and infected hosts. It is a key factor of pathogen infection and can result in diverse relationships between biodiversity and disease prevalence (McCallum et al., 2001; Rudolf and Antonovics, 2005; Keesing et al., 2006; Hopkins et al., 2020; Silk and Hodgson, 2020). Multiple transmission modes have been proposed to represent real host-pathogen interactions, such as density-dependent (DD), frequency-dependent (FD) and other non-linear functions (McCallum et al., 2001; Hopkins et al., 2020), while most studies on the diversity-disease relationship focus on the DD and FD transmission modes (Keesing et al., 2010). The dilution effect of diversity on the disease prevalence under FD transmission differs fundamentally from those under DD transmission (Hatcher et al., 2012), which may arise from the disparity between inherent transmission mechanisms of these two modes. DD transmission usually implies a positive association between host density and transmission rate, whereby the force of infection is directly proportional to the density of infectious hosts (Anderson and May, 1979; Keesing et al., 2006). In contrast, FD transmission depends on the prevalence of infections, whereby the force of infection is proportional to the fraction of infectious hosts (Begon et al., 1999; Hopkins et al., 2020; Silk and Hodgson, 2020). Recent studies have confirmed the importance of host diversity to the transmission dynamics of generalist pathogens, which is likely to depend on the transmission mode (Rudolf and Antonovics, 2005; Hatcher et al., 2012; Roche et al., 2012). For DD transmission, increasing host community diversity (e.g., species richness) could lift the infection force and enhance pathogen transmission due to the increase of host abundance (Dobson, 2004). However, if the disease transmission is frequency dependent (FD), high diversity can reduce pathogen transmission as the fraction of infected hosts in the force of infection could decline due to the increase of host abundance, even if the total number of infected individuals increases (Rudolf and Antonovics, 2005). Consequently, we expect drastically different transmission dynamics and diversity-disease relationships in communities infected by diseases following FD vs. DD disease transmission.
It remains unclear how the composition and the interaction structure of an ecological network, mediated by disease transmission mode, jointly affect disease spread and prevalence. We therefore see two knowledge gaps for understanding disease transmission in ecological interaction networks. Firstly, multiple types of biotic interactions are rarely considered simultaneously in host communities with regards to disease transmission. Earlier studies have largely focused on the role of a specific type of biotic interactions in the transmission of diseases among host species (Dobson, 2004; Keesing et al., 2010; Orlofske et al., 2012; O’Regan et al., 2015); such roles are often overlaid to compute the net effect size of disease transmission in ecological networks, without considering the eco-evolutionary feedbacks from different types of biotic interactions. Secondly, many studies support the dilution effect hypothesis, while network structures and transmission modes may change the relationship between host diversity and disease prevalence. Furthermore, changes in the composition of different interaction types can alter internal structures of an ecological network, mediating species richness at equilibrium and adjusting interactions between different functional guilds (Lurgi et al., 2014; García-Callejas et al., 2018b; Qian and Akçay, 2020). We have yet to elucidate how such changes in network structures could, in return, affect disease transmission and the diversity-disease relationship under different transmission modes.
Here, we explore pathogen infections in a multi-host community characterized by an ecological interaction network, to directly address the aforementioned knowledge gaps. We develop a network model with the dynamics of the host community regulated by diverse interaction types, including competition, predation and mutualism. We extend our research focus from previous theoretical studies on how different interaction types affect community stability (Kokoris et al., 1999; Mougi and Kondoh, 2012, 2014; Bachelot et al., 2015; García-Callejas et al., 2018b; Qian and Akçay, 2020) to how the structures of an ecological network, quantified by interaction compositions, species’ degree centrality, population size and host community diversity (including species richness and Shannon’s diversity), affect disease transmission therein. To do so, we first generate an initial interaction network and explore the relationship between disease prevalence and emerged network structures under two disease transmission modes (DD vs. FD). As shown below, the relationship between the diversity and disease prevalence can be greatly affected by the interaction composition of ecological networks, expanding the dilution effect of host diversity on disease prevalence to a comprehensive effect of network structures of host communities on disease dynamics.
Materials and Methods
Ecological Networks
Following the generalized Rosenzweig and MacArthur (1963) model, we used a set of differential equations to track population dynamics of host species that engage with each other in interspecific interactions and are also susceptible to pathogenic infections. Here, infections were formulated using the general SIS epidemic model for ease of exposition (McQuaid and Britton, 2015; Figueroa et al., 2020), while the model can be easily extended to more complex compartmental representations of infection dynamics (e.g., SIR, SEIR). Interactions between host species formed a complex ecological network; specifically, we considered three types of biotic interactions: competition (−, −), predation (+, −) and mutualism (+, +). For compatibility with May (1972) work, we randomly assigned the presence, type, and strength of an interaction between two species; this forms a matrix of interspecific interactions, A = {ax,ij}n×n, with zeros on the diagonal and also for a proportion of 1 − C non-diagonal entries (C defines network connectance). For the paired non-zero entries (ax,ij, ax,ji ≠ 0), we randomly assigned the interaction type to be competition, mutualism and predation according to a proportion vector {pc, pm, pp}, with pc + pm + pp = 1 (Qian and Akçay, 2020). If the interactions between two species are assigned to, say, competition ac,ij, ac,ji ≠ 0, the strength of other interactions between these species automatically become zero (i.e., am,ij = am,ji = ap,ij = ap,ji = 0); i.e., only one interaction type can be assigned between two species (different from a multilayer network where two species can engage in multiple types of interaction). Following the norm, predatory and mutualistic interactions were also assumed to follow a type II functional response, for simplicity shaped by the same half-saturation constanth (Qian and Akçay, 2020).
The generalist pathogen may follow both horizontal transmission (direct transmission through intra- and inter-specific interaction) and vertical transmission (infection through birth to an infected individual), and infected hosts can partake all the biotic interactions in the community (McQuaid and Britton, 2015). Consequently, all biotic interactions can contribute to the net reproductive rate of the disease. Moreover, we took the predation (attack) rate of an uninfected predators on infected prey to be the same as its predation rate on susceptible (uninfected) prey; that is, a predator cannot discern whether a prey individual is infected by the pathogens. The dynamics of this ecological network with multiple interaction types can be described as follows:
where Si, Ii, Hi(i = 1,⋯, n) represent, respectively, the numbers of susceptible, infected and total individuals of host species i, with Hi = Si + Ii and n the number of host species in the ecological network (i.e., network size). Parameter ri is the intrinsic growth rate, δi density-dependent self-regulation, ρi the recovery rate of infected individuals, νi the infection-induced mortality (i.e., pathogen virulence), and ℬij the force of infection between species j and i.
To evaluate how the composition of different interaction types affects the structure of the ecological network, we followed the special case of demographic-neutral interactions (Zhang et al., 2011; Qian and Akçay, 2020); that is, the intrinsic growth rate (ri = r) and density-dependent self-regulation (δi = δ) are identical among species. We also set the recovery rate of infected hosts (ρi = ρ) and infection-induced mortality (νi = ν) to be equal among species. In this way, the dynamics and structures of the ecological networks become mainly determined by biotic interactions and can be numerically simulated using Equation 1. The paired competition coefficients (ac,ij, ac,ji, both are negative), describing the per capita competition impact of species j on i (sensu Dobson, 2004), were drawn independently from a half-normal distribution with scale parameter σ/K. The paired mutualism coefficients (am,ij, am,ji both are positive) were also drawn independently from the same half-normal distribution with scale parameter σ. The paired predation coefficients (ap,ij, ap,ji) were drawn from a half-normal distribution with scale parameter σ but of opposite signs, with one of the two species randomly chosen to be the prey (denoted by ap-,ji < 0) and the other predator (denoted by ap+,ij > 0), assuming full conversion efficiency (ap+,ij = −ap−,ji). Model under less conversion efficiency (ap+,ij = −ε⋅ap−,ji, with 0 < ε < 1) produced qualitatively consistent results and was thus only presented in Supplementary Appendix 1 (Supplementary Figures 1–4).
Under the density-dependent (DD) transmission mode, the infection rate from host species j to i depends solely on the density of infected individuals of species j (McCallum et al., 2001):
where βij is the per capita infection rate (O’Regan et al., 2015). In contrast, under the frequency-dependent (FD) transmission mode, the infection rate depends on the proportion of infected individuals in species j (Begon et al., 1999; McCallum et al., 2001):
The generalist pathogen can transmit through both intraspecific and interspecific interactions, where the per capita infection rate depends on the ecological interaction coefficients between host species (Kirk et al., 2019). Specifically, we assume βij = ωijax,ij for i ≠ j, and βii = ωiiδi, where the transmission coefficient ωij was also drawn from a half-normal distribution with scale parameter σ′ (note, values of parameter σ′ were chosen in the analyses to ensure the total population sizes of all species be equal under DD and FD transmission modes). The model was analyzed under both DD and FD transmission modes, with the results from the DD mode presented in the main text and those from the FD mode compared and presented in Supplementary Appendix 2. All model parameters are listed in Supplementary Table 1.
Network Analyses
We simulated the full spectrum of ecological networks by varying the interaction composition {pc, pm, pp}, with the proportion of each interaction type ranging from 0 to 1 with a step of 0.1 while retaining the sum (pc + pp + pm = 1). Because species in real communities were expected to engage in some levels of interspecific competition, we excluded cases with competition completely absent in modeled networks (Kirk et al., 2019; Qian and Akçay, 2020) and thus set the proportion of competitive interactions to be strictly positive (i.e., pc ranges from 0.1 to 1); this does not affect key conclusions. In total, we explored 55 specific combinations of interaction types. For each interaction composition (specified by vector {pc, pm, pp}) under a specific transmission mode (DD or FD), we ran the model till all populations had reached equilibrium; species with an equilibrial population size below a cut-off value (=0.1) was removed in subsequent analyses (Holland and DeAngelis, 2010). To obtain a robust view of the emerged network structures and disease prevalence therein, we repeated the model 1,000 times for each interaction composition.
To capture the consequence of this community-wide and species-specific disease transmission in the modeled ecological networks, we computed two metrics of disease prevalence: the degree of prevalence at the community level () and the degree of prevalence per species (Ii/Hi). We also quantified three typical community-level structural metrics of the final interaction network at equilibrium, including species richness (final network size; Lau et al., 2017), Shannon’s diversity, and population sizes (specifically, total number of all host individuals, number of susceptible individuals and number of infectious individuals). To understand how disease prevalence varies in response to network structures and explicitly test the dilution effect, we computed the correlations between disease prevalence and these network structural metrics.
To explore how a species’ network topology affects its disease prevalence, we computed the correlation between the species’ disease prevalence and its degree centrality in the ecological network at equilibrium (Supplementary Appendix 2: Supplementary Figures 9, 10). Species i’s degree is simply the number of non-zero entries in the ith row of interspecific interaction matrix A, and the degree centrality is defined as the ratio of a species’ degree to the theoretical maximum degree possible (i.e., the number of sustained species at equilibrium minus one) in the ecological network (Freeman, 1978). The architectures of modeled ecological networks at equilibrium were further measured by nestedness based on the overlap and decreasing fill (NODF; Almeida-Neto et al., 2008) using the software package ANINHADO 3.0 (Guimarães and Guimarães, 2006) and modularity using Newman (2004) algorithm (Supplementary Figure 11). As the effects of transmission modes on network architectures only differ minutely, we only reported these results in Supplementary Appendix 2.
To test the robustness of our main findings, we used a Latin hypercube sampling to explore a region of parameter space for sensitivity analysis (Marino et al., 2008). From a region of the full 7-dimensional parameter space (i.e., seven key parameters δ, C, h, σ, σ′, ν and ρ) defined by ±40% of the reference parameter values used in the main text, 10 samples of parameter combinations were randomly drawn, with each containing 55 interaction compositions and 500 replicates for each interaction composition. We found that the main results were qualitatively robust across all values of self-regulation rate δ, connectivity C, half-saturation constant h, scale parameters σ and σ′, recovery rate ρ and infection-induced mortality ν (Supplementary Appendix 3: Supplementary Figures 12–16).
During the numerical simulations, some species became extinct and were removed before the network equilibrium was reached (Figure 1A), which could alter the initial interaction composition {pc, pm, pp} but only slightly so in our simulations (Figure 1B). Specifically, we observed that the final networks at equilibrium harbor more mutualistic interactions (increasing pm at equilibrium), less competition (reducing pc) and less predation (reducing pp). This is not surprising since predatory and/or competitive interactions could lead to the extinctions of some species, while mutualistic interactions rarely did so in the modeled networks. As the changes to interaction compositions were rather minute (<1% on average), we only refer to initial interaction composition in the following. Specifically, we quantified the direct effects of interaction composition and transmission mode on disease prevalence, as well as the dilution effect mediated by community features at equilibrium (i.e., population size, species richness and Shannon’s diversity). The procedure of the modeling and analyses was illustrated in Figure 1C (the simulation code available on1).
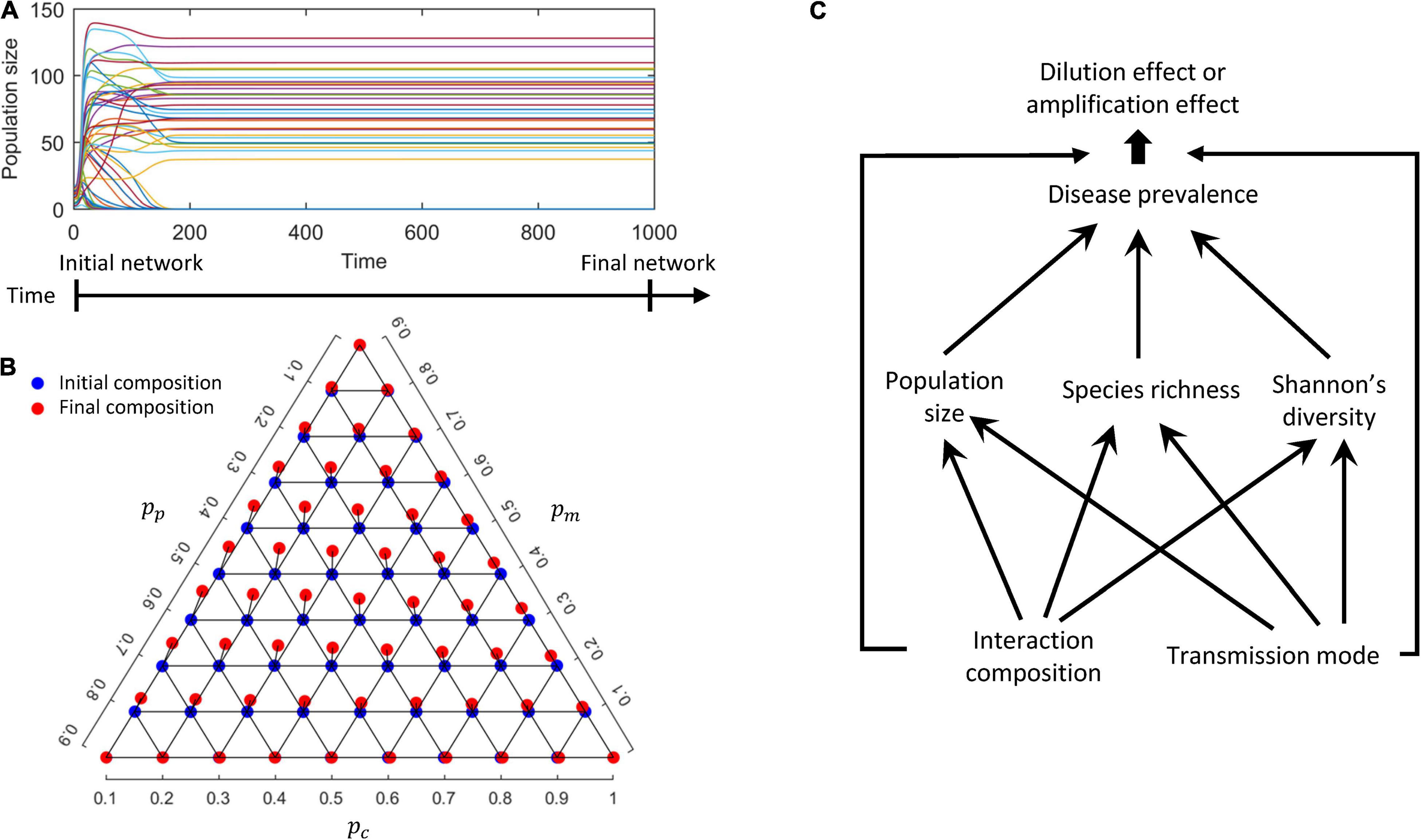
Figure 1. A conceptual diagram of the modeling procedure. (A) Population dynamics of a randomly assembled host network. (B) Interaction compositions of the initial (blue) and final (red) networks when population dynamics have reached equilibrium. (C) Summary diagrams of the effects of interaction composition and transmission mode on community metrics, disease prevalence and the diversity-disease relationship.
Results
Direct Effects of Interaction and Transmission
The interaction composition can greatly affect disease prevalence at the community level regardless of whether the disease follows a DD or FD transmission mode (Figure 2 and Supplementary Figure 5). As we expected, a higher proportion of mutualistic interactions (pm), equivalent to reduced proportions of competition (pc) or predation (pp), led to higher disease prevalence (Figure 2B). Regardless of whether to hold constant the proportion of competitive interactions (pc) or the proportion of predatory interactions (pp), increasing the proportion of mutualistic interactions (pm) can always boost up the disease prevalence monotonically (Figure 2B), suggesting a positive effect of mutualism on disease prevalence. On the contrary, more competitive interactions (higher pc) in communities, accompanied with reduced proportions of mutualism (pm) or predation (pp), led to lower disease prevalence (Figure 2B). Finally, when accompanied with a low proportion of competitive interactions, more predatory interactions can decrease disease prevalence; when accompanied with a low proportion of mutualism, more predatory interactions can increase disease prevalence (Figure 2B). Although the FD transmission mode resulted in less disease prevalence than the DD transmission mode, the responses of disease prevalence to interaction composition were qualitatively similar under these two modes (Supplementary Appendix 2: Supplementary Figure 5A). Specifically, interaction compositions greatly impacted on the population size, but the total population size of all host species under the two transmission modes were found to be largely comparable, whereas the number of infectious individuals was lower under the FD transmission mode than the number under the DD transmission mode (Supplementary Figure 6); this explains the lower disease prevalence under the FD transmission mode than the prevalence under the DD transmission mode (Figure 2 vs. Supplementary Figure 5).
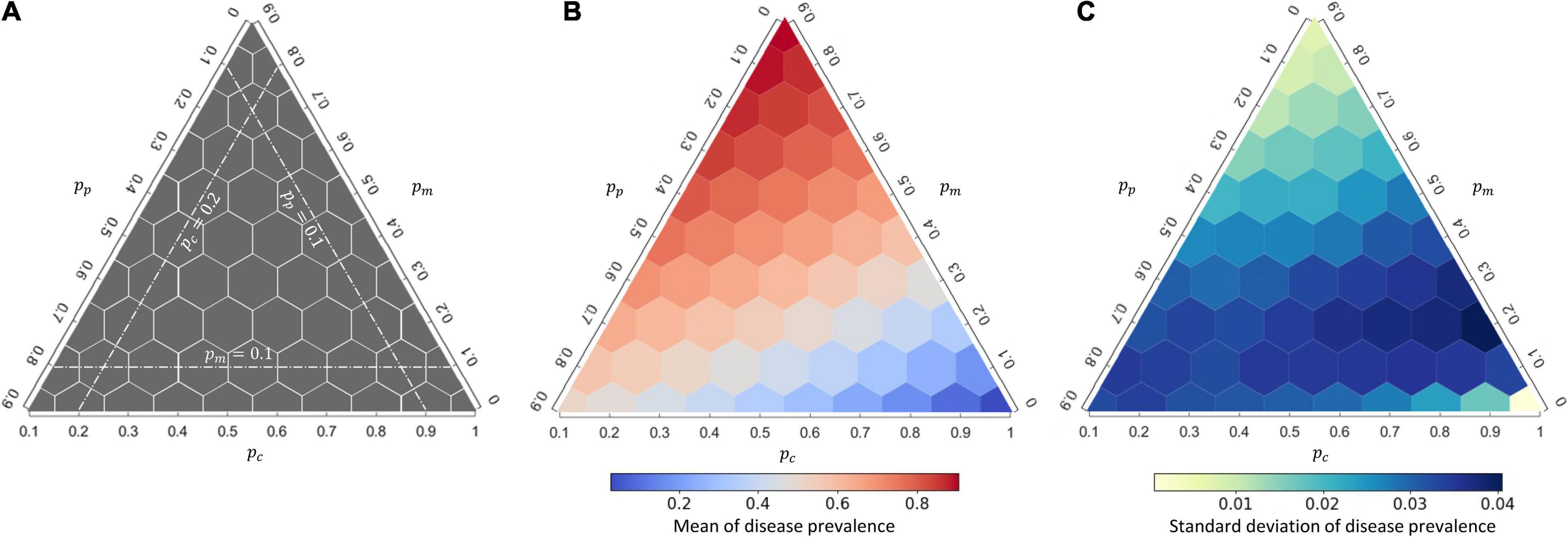
Figure 2. The response of disease prevalence at the community level to interaction composition under density-dependent (DD) disease transmission. (A) A schematic guide to read the ternary plot. The three white dashed lines represent the interaction composition when the proportion of the specified interaction type held constant. The center of each hexagon represents a single interaction composition examined (55 in total); (B,C) the mean and standard deviation of the response for each interaction composition, calculated from 1,000 replicates. See Supplementary Figure 5 for the corresponding figure under frequency-dependent (FD) transmission.
The variation of disease prevalence for each interaction combination, measured by the standard deviation of disease prevalence from 1,000 replicates, was also determined by the interaction composition (Figure 2C and Supplementary Figure 5B). Under the DD transmission mode, the variation of disease prevalence was largely determined by the proportion of mutualism, with more mutualistic interactions in communities (higher pm balanced by either lower pp or lower pc) hampering demographic stochasticity of the disease dynamics (Figure 2C). In contrast, the proportion of mutualism (pm) or competition (pc) did not make a marked impact to the variation of disease prevalence under the FD transmission mode, whereas a higher proportion of predatory interactions (pp) can drastically increase the variation of disease prevalence (Supplementary Figure 5B).
Dilution Effects
As the interaction composition can strongly affect disease prevalence, we further examined how interaction compositions affect the mean and variation of species richness and Shannon’s diversity from the 1,000 replicates (Figure 3) and whether the diversity of a community amplifies or dilutes disease prevalence (Figure 4). At higher proportion of mutualistic interactions (higher pm), equivalent to less proportions of competition (lower pc) or predation (lower pp), mean of species richness was higher (Figure 3A). This resulted in a predominantly positive correlation between species richness and disease prevalence (i.e., an amplification effect; Figure 4A and Supplementary Table 2). In contrast, when holding pm constant, more competitive interactions (higher pc) within communities, accompanied with less predation (lower pp), led to higher species richness (Figure 3A) and lower disease prevalence (Figure 2B), exhibiting a negative correlation between species richness and disease prevalence (i.e., the dilution effect; Figure 4A). Importantly, less competition (lower pc) led to the positive richness-prevalence correlations for all the other combinations of mutualistic and predatory interactions, which indicated that the positive richness-prevalence correlation shifted to the negative with an increasing of competition and then flipped the amplification effect into the dilution effect (Figure 4A and Supplementary Table 2). Finally, changes in the richness-prevalence correlation are small as holding pc constant, and there is no clear pattern varying the proportion of mutualism or the proportion of predation (Figure 4A); indicating the competition effect within communities more pronounced in the relationship between species richness and disease prevalence.
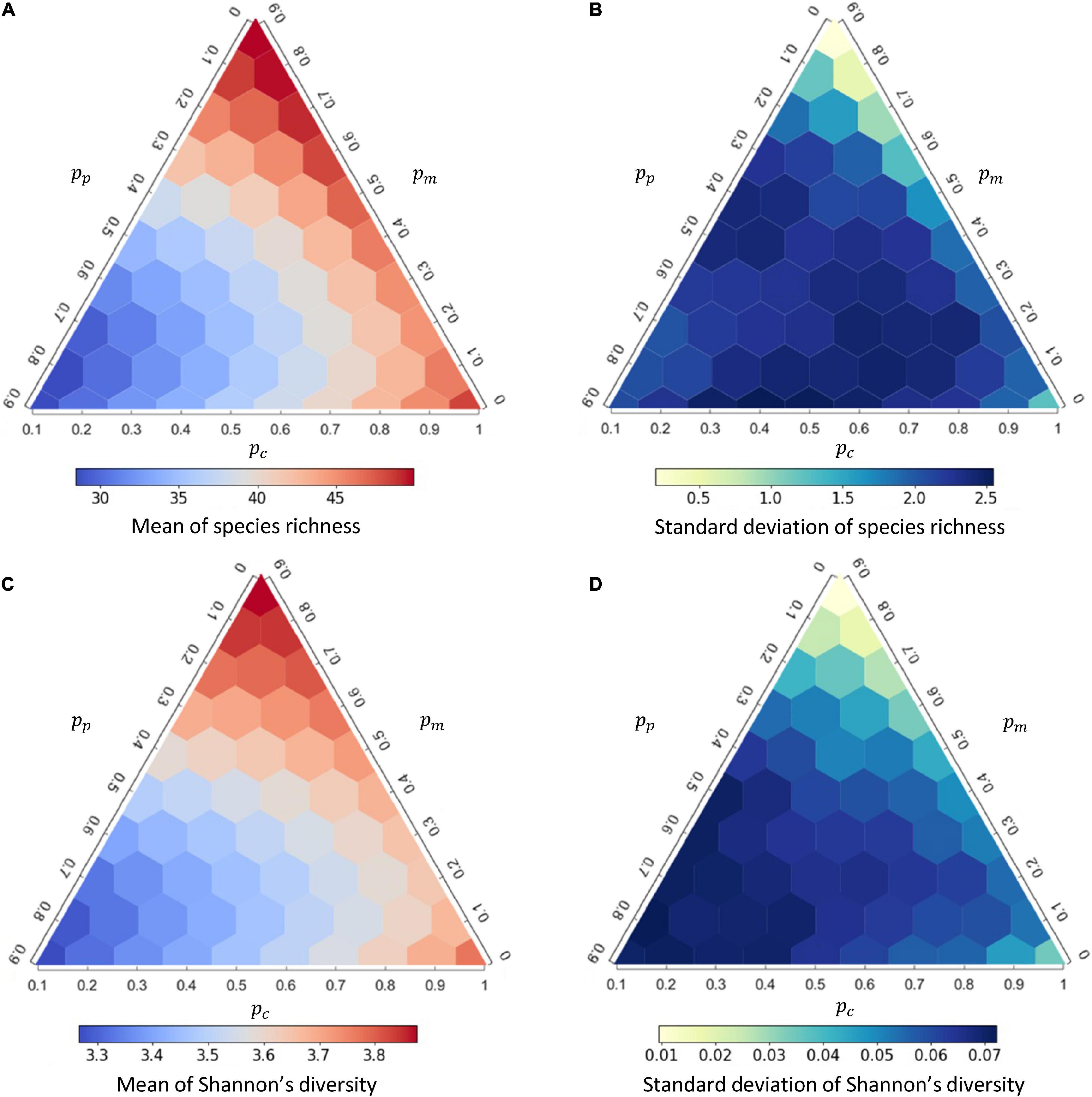
Figure 3. The responses of species richness and Shannon’s diversity, respectively, to interaction composition under density-dependent (DD) disease transmission. (A,B) effects of interaction compositions on the mean and standard deviation of species richness; (C,D) effects of interaction compositions on the mean and standard deviation of Shannon’s diversity. See Supplementary Figure 7 for the corresponding figure under frequency-dependent (FD) transmission.
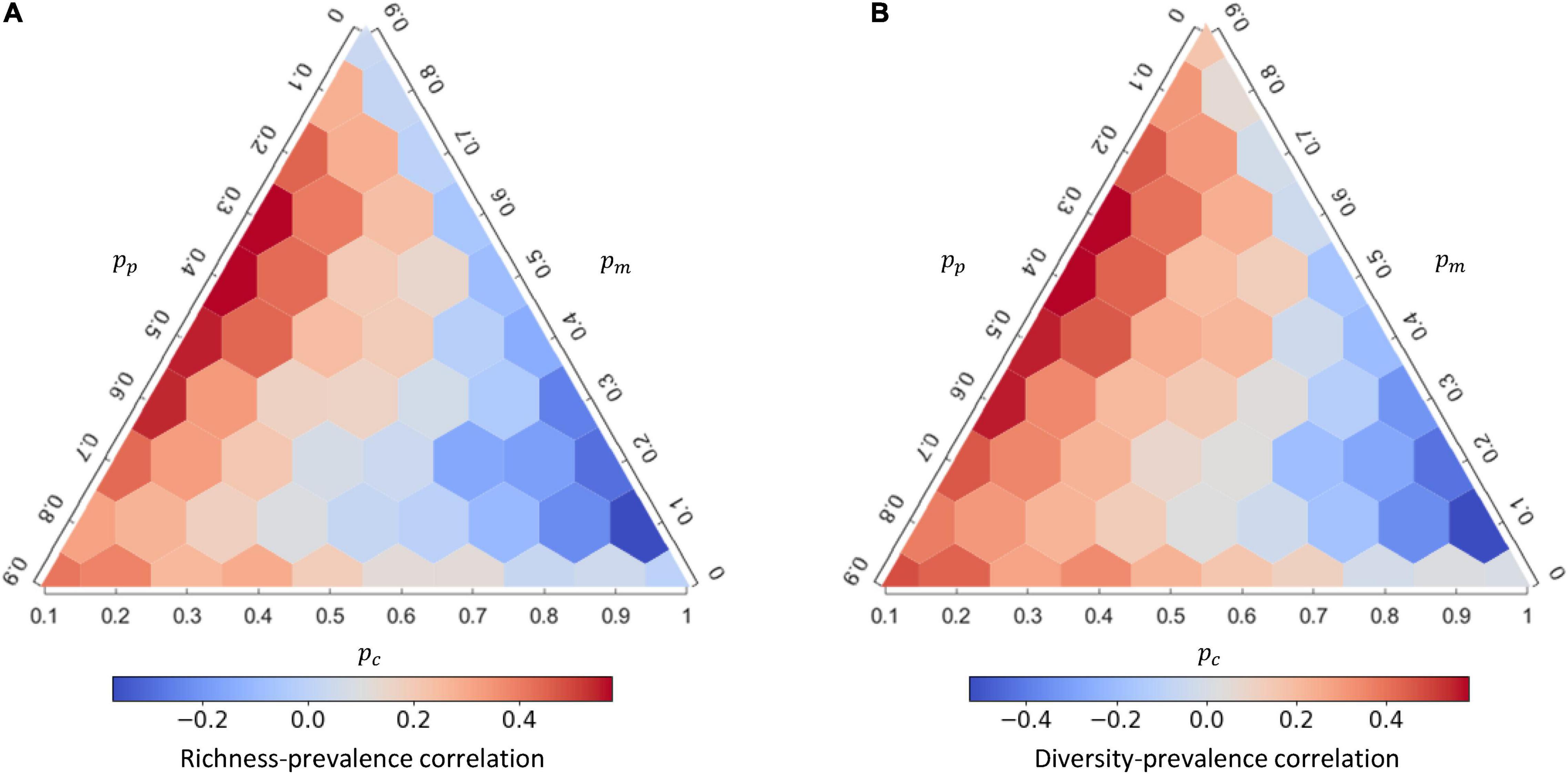
Figure 4. The response of richness-prevalence correlation and diversity-prevalence correlation to interaction composition under density-dependent (DD) disease transmission. Richness-prevalence correlation (A) and diversity-prevalence correlation (B) of the response for each interaction composition, calculated from 1,000 replicates. See Supplementary Figure 8 for the corresponding figure under frequency-dependent (FD) transmission.
In addition, both the mean of Shannon’s diversity (Figure 3C) and the diversity-prevalence correlation (Figure 4B and Supplementary Table 3) showed a similar complex response to changes in interaction compositions. Similar results were also retained under the FD transmission mode (Supplementary Figures 7, 8). The role of interaction compositions in driving the diversity-disease relationship appeared robust under other model parameters (Supplementary Appendix 3: Supplementary Figures 14–16).
Discussion
Predicting the prevalence of pathogenic diseases transmitting across multiple interacting species has proven to be difficult due to the direct and indirect biotic interactions within an ecological network (Keesing et al., 2010; O’Regan et al., 2015; Figueroa et al., 2020). Previous studies have revealed that the composition of different types of biotic interaction plays an important structural role in ecological communities (Mougi and Kondoh, 2012; Bachelot et al., 2015; Coyte et al., 2015; García-Callejas et al., 2018a,b), although how such ecological networks fare when facing infectious diseases has rarely been explored. Our modeled ecological networks were shaped by different compositions of interaction types, which allowed us to explore the complex relationships between disease transmission and biodiversity structures in ecological communities. Results illustrated that biotic interactions can govern disease transmission dynamics and the diversity-disease relationship (Figures 2, 4 and Supplementary Figures 5, 8). These key findings added a new explanation to the list of mechanisms hypothesized to address the diversity-disease relationship in natural communities (i.e., the dilution effect) and clarified the differential effects of interaction types on disease prevalence at both species- and community-level. Our results are therefore relevant to understanding the disease-network relationship in communities consisting of multiple types of biotic interaction.
Although primary research supports the negative effect of host community diversity on disease prevalence, known as the dilution effect, many natural ecosystems have been reported to experience an amplification effect of diversity on disease prevalence (Schmidt and Ostfeld, 2001; Keesing et al., 2010; Civitello et al., 2015; Rohr et al., 2020). Both theoretical and experimental studies have shown that direct interactions between species pairs can control or possibly promote pathogen transmission in multi-host communities through regulating the fluctuations of host species (Orlofske et al., 2012; Salkeld et al., 2013; Coyte et al., 2015; O’Regan et al., 2015). However, interaction networks are not only prescribed by a handful of pairwise interactions, but also the composition and balance among different functional guilds (García-Callejas et al., 2018a; Qian and Akçay, 2020). Some functional guilds can be disproportionally affected by pathogen infections and thus function as hubs for disease transmission. Specifically, different types of interactions can produce different types of direct and indirect feedbacks (e.g., positive for mutualistic interaction and negative for predatory interaction), rendering the differential effects of interaction types on disease dynamics (Crowder et al., 2019). Species engaging in a high number of positive interactions (i.e., mutualistic interactions) tend to persist and maintain their interactions, whereas species with a high number of negative interactions (e.g., competitive and predatory interactions) tend to go extinct more frequently and, thus, lose their associated interactions, increasing the overall ratio of mutualistic interactions (Figure 1B). Consequently, mutualistic interactions provide net benefits and elevate disease prevalence through stimulating cross-species transmission of pathogenic diseases (Figure 2 and Supplementary Figure 5). Consistent with previous studies, interspecific competition hampers species abundances in the host community, which may lead to a decreased rate of cross-species transmission and in return impede disease transmission (O’Regan et al., 2015). Moreover, predators in a community can typically reduce disease transmission through consuming host individuals that are their prey (Packer et al., 2003; Orlofske et al., 2012; Johnson et al., 2015), while our results indicated that whether predatory interactions can dilute disease spreading depends on the balance of mutualism or competition (Figure 2 and Supplementary Figure 5). Although the competitive interactions play a predominant role in the diversity-prevalence correlation, each interaction type can pose a contrasting role in the dilution or the amplification effect depending on the balance of the other two interaction types in the community (Figure 4 and Supplementary Figure 8); that is, the composition of multiple interaction types, not just a single type of biotic interaction, dictates the diversity-disease relationship.
An ecological network involves multiple types of interaction, making it difficult for a single functional guild to exercise control over community dynamics. Recent studies have shown that interaction compositions can profoundly affect the stability of ecological network, while positive interactions (e.g., mutualism) can play a stabilizing or destabilizing role contingent on other functional guilds in the ecological network (Mougi and Kondoh, 2012; Bachelot et al., 2015). It is the balance of multiple interaction types (competition, mutualism and predation) that determines the stability of an ecological community (García-Callejas et al., 2018a,b; Qian and Akçay, 2020). Our results complement these propositions, indicating that the balance of multiple interactions also determines these other things, apart from stability. Comparing to the facilitative effect of mutualism on disease prevalence, mutualistic interactions can benefit or impede the diversity of an ecological network, depending on the compositions of predation or competition (Figure 3 and Supplementary Figure 7). More mutualistic interactions have been shown to reduce the overall strength of biotic interactions in an ecological network (Lurgi et al., 2016). Indeed, we found that more mutualistic interactions when holding either pc or pp constant can increase community diversity, although this beneficial role of mutualism to diversity breaks down when it provokes intense competition for high pc which imposes a net negative impact on community diversity. Our model makes evident the pivotal effects of interaction compositions on biodiversity maintenance and disease prevalence in ecological networks, which can switch the typical dilution effect of diversity on disease prevalence to an amplification effect.
Beyond the diversity-disease relationship that is mediated by interaction compositions, our results further suggested that the interplay of multiple interaction types can posit profound influence on the species-level disease prevalence. All other conditions being equal, the species-level prevalence is expected to increase significantly with a species’ degree centrality (Supplementary Figures 9, 10). As we adopted May (1972) random network framework, the degree centrality of species in the network distributes around the network connectance. Generalist species with higher centrality degrees are hubs for cross-species transmission of infectious diseases, suggesting a positive correlation between degree centrality and within-species disease prevalence regardless of the interaction compositions and the transmission mode. Furthermore, the coefficients of centrality-prevalence correlation were determined by interaction compositions, with predatory interactions generating opposite effects on predators (positive) vs. prey (negative) with the increase of centrality degree, which also resulted the maximum and the minimum of centrality-prevalence correlation in communities lacking the moderation of predatory interactions (Supplementary Tables 4, 5). Other topological structure of ecological networks may also impact epidemiological dynamics (Rozins et al., 2018; Figueroa et al., 2020) and community stability (Thébault and Fontaine, 2010; Mougi and Kondoh, 2012; Landi et al., 2018). For example, increased connectance can reduce the likelihood of a disease outbreak in mutualistic community, resulting a dilution effect (Figueroa et al., 2020). Our simulations indicated that the reported diversity-disease relationships were quantitatively robust against changes of network connectance (Supplementary Appendix 3). Nevertheless, we reckon that our model only yields the null expectation of a random network that is also demographically neutral; consequently, network architectures might not be capable of imposing markable impacts to disease transmission (Supplementary Figures 9–11). Comparing results from more realistic network models with our results can therefore help identify the role of additional processes and features that are not captured by our null model.
Our simulations indicated that the effects of interaction types on disease transmission were largely generic and robust against transmission modes (Supplementary Appendix 2). Previous studies considered only horizontal transmission through intra- and inter-specific contact, and illustrated that the dilution effect normally occurs in communities under FD disease transmission whilst the amplification effect under DD transmission, highlighting the importance of transmission modes (Dobson, 2004; Rudolf and Antonovics, 2005). By contrast, we found that whether the effect of diversity on disease prevalence is diluting or amplifying depends primarily on interaction compositions, and little on transmission modes (Figure 4 and Supplementary Figure 8). The reasons are multifaceted. Firstly, although pathogens and predators can both serve as suppressors to a host/prey species, the former does not normally lead to the kill of the host (Civitello et al., 2015; Rohr et al., 2020); this non-lethal role of diseases, either according to the linear form of DD transmission (βSI) or the non-linear form of FD transmission (βSI/(S + I)), imposes trivial impacts on species richness and thus community diversity. Secondly, by keeping the horizontal and vertical transmissions simultaneously in our model, pathogens can infect multiple host species and their progenies (McQuaid and Britton, 2015). Classical theory has also shown that disease prevalence under both horizontal and vertical transmissions can be much higher than the prevalence with either transmission form alone (Lipsitch et al., 1995), masking any differences created by the DD and FD transmission modes. Clearly, elucidating whether the dilution effect differs between diverse transmission mechanisms (e.g., between vertical and horizontal transmission, and between direct transmission through interaction and environmental transmission of free-living pathogens) warrants ongoing research.
In conclusion, we extended the existing theory on the diversity-disease relationship by setting the context in an ecological network and consequently obtained a more complex diversity-disease relationship tuned by the balance and composition of multiple types of biotic interactions. Our findings contribute to advance in the debate on the dilution effect by highlighting that diverse interaction types are pivotal to generating the variations in the relationship between disease prevalence and community diversity in an ecological network. Insights on the role of interaction composition in the diversity-disease relationship could potentially lead to better disease control (Rohr et al., 2020). Different interaction types were made compatible in our model “on equal footing” (sensu García-Callejas et al., 2018a), with all expressed by their impacts on the population change rate (Mougi and Kondoh, 2012). We do reckon that each interaction type can be modeled by various functional forms while two species can also engage in more than one type of interactions as in multilayer networks (Pilosof et al., 2017; García-Callejas et al., 2018a); how disease transmission performs in other complex networks demands further investigation. In addition, introductions of alien invasive species could alter the composition, structure and invasibility of ecological interaction networks (Maynard et al., 2018; Qian and Akçay, 2020; Hui and Richardson, 2022) which in turn may regulate the disease transmission (Strauss et al., 2012; Young et al., 2017). Our study thus moves only one step toward how complex interaction networks respond to the stress from pathogenic infections, while understanding how species introductions and invasions affect interaction composition and disease transmission could be a fruitful revenue for future research.
Data Availability Statement
The original contributions presented in the study are included in the article/Supplementary Material, further inquiries can be directed to the corresponding author/s.
Author Contributions
MS: conceptualization, methodology, writing original draft, and review and editing. ZJ: software and methodology. CH: methodology and review and editing. All authors contributed to the article and approved the submitted version.
Conflict of Interest
The authors declare that the research was conducted in the absence of any commercial or financial relationships that could be construed as a potential conflict of interest.
Publisher’s Note
All claims expressed in this article are solely those of the authors and do not necessarily represent those of their affiliated organizations, or those of the publisher, the editors and the reviewers. Any product that may be evaluated in this article, or claim that may be made by its manufacturer, is not guaranteed or endorsed by the publisher.
Acknowledgments
We are grateful to the editor and two reviewers for their constructive comments. MS was supported by the National Natural Science Foundation of China (No. 31770470). CH acknowledged support from the South African Research Chair Initiative and the National Research Foundation of South Africa (Grants 89967 and 109244).
Supplementary Material
The Supplementary Material for this article can be found online at: https://www.frontiersin.org/articles/10.3389/fevo.2022.862986/full#supplementary-material
Footnotes
References
Almeida-Neto, M., Guimarães, P., Guimarães, P. R. Jr., Loyola, R. D., and Ulrich, W. (2008). A consistent metric for nestedness analysis in ecological systems: reconciling concept and measurement. Oikos 117, 1227–1239. doi: 10.1111/j.0030-1299.2008.16644.x
Anderson, R. M., and May, R. M. (1979). Population biology of infectious diseases: Part I. Nature 280, 361–367. doi: 10.1038/280361a0
Bachelot, B., Uriarte, M., and McGuire, K. (2015). Interactions among mutualism, competition, and predation foster species coexistence in diverse communities. Theoretical Ecol. 8, 297–312. doi: 10.1007/s12080-015-0251-2
Begon, M., Hazel, S. M., Baxby, D., Bown, K., Cavanagh, R., Chantrey, J., et al. (1999). Transmission dynamics of a zoonotic pathogen within and between wildlife host species. Proc. R. Soc. B 266, 1939–1945. doi: 10.1098/rspb.1999.0870
Bowers, R. G., and Turner, J. (1997). Community structure and the interplay between interspecific infection and competition. J. Theor. Biol. 187, 95–109. doi: 10.1006/jtbi.1997.0418
Civitello, D. J., Cohen, J., Fatima, H., Halstead, N. T., Liriano, J., McMahon, T. A., et al. (2015). Biodiversity inhibits parasites: broad evidence for the dilution effect. Proc. Natl. Acad. Sci. U S A. 112, 8667–8671. doi: 10.1073/pnas.1506279112
Clark, R. E., Basu, S., Lee, B. W., and Crowder, D. W. (2019). Tri-trophic interactions mediate the spread of a vector-borne plant pathogen. Ecology 100:e02879. doi: 10.1002/ecy.2879
Coyte, K. Z., Schluter, J., and Foster, K. R. (2015). The ecology of the microbiome: networks, competition, and stability. Science 350, 663–666. doi: 10.1126/science.aad2602
Crowder, D. W., Li, J., Borer, E. T., Finke, D. L., Sharon, R., Pattemore, D. E., et al. (2019). Species interactions affect the spread of vector-borne plant pathogens independent of transmission mode. Ecology 100:e02782. doi: 10.1002/ecy.2782
Dobson, A. P. (2004). Population dynamics of pathogens with multiple host species. Am. Nat. 164, S64–S78. doi: 10.1086/424681
Figueroa, L. L., Grab, H., Ng, W. H., Myers, C. R., Graystock, P., McFrederick, Q. S., et al. (2020). Landscape simplification shapes pathogen prevalence in plant-pollinator networks. Ecol. Lett. 23, 1212–1222. doi: 10.1111/ele.13521
Frainer, A., McKie, B. G., Amundsen, P. A., Knudsen, R., and Lafferty, K. D. (2018). Parasitism and the biodiversity-functioning relationship. Trends Ecol. Evol. 33, 260–268. doi: 10.1016/j.tree.2018.01.011
Freeman, L. C. (1978). Centrality in social networks conceptual clarification. Soc. Networks 1, 215–239. doi: 10.1016/0378-8733(78)90021-7
García-Callejas, D., Molowny-Horas, R., and Araújo, M. B. (2018a). Multiple interactions networks: towards more realistic descriptions of the web of life. Oikos 127, 5–22. doi: 10.1111/oik.04428
García-Callejas, D., Molowny-Horas, R., and Araújo, M. B. (2018b). The effect of multiple biotic interaction types on species persistence. Ecology 99, 2327–2337. doi: 10.1002/ecy.2465
Guimarães, P. R. Jr., and Guimarães, P. (2006). Improving the analyses of nestedness for large sets of matrices. Environ. Modell. Software 21, 1512–1513. doi: 10.1016/j.envsoft.2006.04.002
Hatcher, M. J., Dick, J. T. A., and Dunn, A. M. (2006). How parasites affect interactions between competitors and predators. Ecol. Lett. 9, 1253–1271. doi: 10.1111/j.1461-0248.2006.00964.x
Hatcher, M. J., Dick, J. T. A., and Dunn, A. M. (2012). Diverse effects of parasites in ecosystems: linking interdependent processes. Front. Ecol. Environ. 10:186–194. doi: 10.1890/110016
Hoi, A. G., Gilbert, B., and Mideo, N. (2020). Deconstructing the impact of Malaria vector diversity and disease risk. Am. Nat. 196, E61–E70. doi: 10.1086/710005
Holland, J. N., and DeAngelis, D. L. (2010). A consumer-resource approach to the density-dependent population dynamics of mutualism. Ecology 91, 1286–1295. doi: 10.1890/09-1163.1
Hopkins, S. R., Fleming-Davies, A. E., Belden, L. K., and Wojdak, J. M. (2020). Systematic review of modelling assumptions and empirical evidence: does parasite transmission increase nonlinearly with host density? Methods Ecol. Evol. 11, 476–486. doi: 10.1111/2041-210x.13361
Huang, Z. Y. X., van Langevelde, F., Estrada-Peña, A., Suzán, G., and de Boer, W. F. (2016). The diversity-disease relationship: evidence for and criticisms of the dilution effect. Parasitology 143, 1075–1086. doi: 10.1017/S0031182016000536
Hui, C., and Richardson, D. M. (2022). Invading Ecological Networks. Cambridge: Cambridge University Press.
Johnson, P. T. J., Ostfeld, R. S., and Keesing, F. (2015). Frontiers in research on biodiversity and disease. Ecol. Lett. 18, 1119–1133. doi: 10.1111/ele.12479
Keesing, F., Belden, L. K., Daszak, P., Dobson, A., Harvell, C. D., Holt, R. D., et al. (2010). Impacts of biodiversity on the emergence and transmission of infectious diseases. Nature 468, 647–652. doi: 10.1038/nature09575
Keesing, F., Holt, R. D., and Ostfeld, R. S. (2006). Effects of species diversity on disease risk. Ecol. Lett. 9, 485–498. doi: 10.1111/j.1461-0248.2006.00885.x
Kirk, D., Shea, D., and Start, D. (2019). Host traits and competitive ability jointly structure disease dynamics and community assembly. J. Animal Ecol. 88, 1379–1391. doi: 10.1111/1365-2656.13028
Kokoris, G. D., Troumbis, A. Y., and Lawton, J. H. (1999). Patterns of species interaction strength in assembled theoretical competition communities. Ecol. Lett. 2, 70–74. doi: 10.1046/j.1461-0248.1999.22058.x
Landi, P., Minoarivelo, H. O., Brännström, Å, Hui, C., and Dieckmann, U. (2018). Complexity and stability of ecological networks: a review of the theory. Population Ecol. 60, 319–345. doi: 10.1007/s10144-018-0628-3
Lau, M. K., Borrett, S. R., Baiser, B., Gotelli, N. J., and Ellison, A. M. (2017). Ecological network metrics: opportunities for synthesis. Ecosphere 8:e01900.
Lipsitch, M., Nowak, M., Ebert, D., and May, R. (1995). The population dynamics of vertically and horizontally transmitted parasites. Proc. R. Soc. B 260, 321–327. doi: 10.1098/rspb.1995.0099
LoGiudice, K., Ostfeld, R. S., Schmidt, K. A., and Keesing, F. (2003). The ecology of infectious disease: effects of host diversity and community composition on Lyme disease risk. Proc. Natl. Acad. Sci. U S A. 100, 567–571. doi: 10.1073/pnas.0233733100
Lurgi, M., Galiana, N., López, B. C., Joppa, L. N., and Montoya, J. M. (2014). Networks complexity and species traits mediate the effects of biological invasions on dynamic food webs. Front. Ecol. Evol. 2:36. doi: 10.3389/fevo.2014.00036
Lurgi, M., Montoya, D., and Montoya, J. M. (2016). The effects of space and diversity of interaction types on the stability of complex ecological networks. Theoretical Ecol. 9, 3–13. doi: 10.1007/s12080-015-0264-x
Marino, S., Hogue, I. B., Ray, C. J., and Kirschner, D. E. (2008). A methodology for performing global uncertainty and sensitivity analysis in systems biology. J. Theor. Biol. 254, 178–196. doi: 10.1016/j.jtbi.2008.04.011
May, R. M. (1972). Will a large complex system be stable? Nature 238, 413–414. doi: 10.1038/238413a0
Maynard, D. S., Serván, C. A., and Allesina, S. (2018). Network spandrels reflect ecological assembly. Ecol. Lett. 21, 324–334. doi: 10.1111/ele.12912
McCallum, H., Barlow, N., and Hone, J. (2001). How should pathogen transmission be modeled? Trends Ecol. Evol. 16, 295–300. doi: 10.1016/s0169-5347(01)02144-9
McQuaid, C. F., and Britton, N. F. (2015). Parasite species richness and its effect on persistence in food webs. J. Theor. Biol. 364, 377–382. doi: 10.1016/j.jtbi.2014.09.035
Mougi, A., and Kondoh, M. (2012). Diversity of interaction types and ecological community stability. Science 337, 349–351. doi: 10.1126/science.1220529
Mougi, A., and Kondoh, M. (2014). Stability of competition-antagonism mutualism hybrid community and the role of community network structure. J. Theor. Biol. 360, 54–58. doi: 10.1016/j.jtbi.2014.06.030
Newman, M. E. J. (2004). Fast algorithm for detecting community structure in networks. Phys. Rev. E 69:066133. doi: 10.1103/PhysRevE.69.066133
O’Regan, S. M., Vinson, J. E., and Park, A. W. (2015). Interspecific contact and competition may affect the strength and direction of disease-diversity relationships for directly transmitted microparasites. Am. Nat. 186, 480–494. doi: 10.1086/682721
Orlofske, S. A., Jadin, R. C., Preston, D. L., and Johnson, P. T. J. (2012). Parasite transmission in complex communities: predators and alternative hosts alter pathogenic infections in amphibians. Ecology 93, 1247–1253. doi: 10.1890/11-1901.1
Packer, C., Holt, R. D., Dobson, A. P., and Hudson, P. (2003). Keeping the herds healthy and alert: impacts of predation upon prey with specialist pathogens. Ecol. Lett. 6, 797–802. doi: 10.1046/j.1461-0248.2003.00500.x
Pilosof, S., Porter, M. A., Pascual, M., and Kéfi, S. (2017). The multilayer nature of ecological networks. Nat. Ecol. Evol. 1:0101. doi: 10.1038/s41559-017-0101
Qian, J. J., and Akçay, E. (2020). The balance of interaction types determines the assembly and stability of ecological communities. Nat. Ecol. Evol. 4, 356–365. doi: 10.1038/s41559-020-1121-x
Randolph, S. E., and Dobson, A. D. M. (2012). Pangloss revisited: a critique of the dilution effect and the biodiversity-buffers disease paradigm. Parasitology 139, 847–863. doi: 10.1017/S0031182012000200
Roche, B., Dobson, A. P., Guégan, J. F., and Rohani, P. (2012). Linking community and disease ecology: the impact of biodiversity on pathogen transmission. Philos. Trans. R. Soc. B 367, 2807–2813. doi: 10.1098/rstb.2011.0364
Rohr, J. R., Civitello, D. J., Crumrine, P. W., Halstead, N. T., Miller, A. D., Schotthoefer, A. M., et al. (2015). Predator diversity, intraguild predation, and indirect effects drive parasite transmission. Proc. Natl. Acad. Sci. U S A. 112, 3008–3013. doi: 10.1073/pnas.1415971112
Rohr, J. R., Civitello, D. J., Halliday, F. W., Hudson, P. J., Lafferty, K. D., Wood, C. L., et al. (2020). Towards common ground in the biodiversity-disease debate. Nat. Ecol. Evol. 4, 24–33. doi: 10.1038/s41559-019-1060-6
Rosenzweig, M. L., and MacArthur, R. H. (1963). Graphical representation and stability conditions of predator-prey interactions. Am. Nat. 97, 209–223. doi: 10.1086/282272
Rozins, C., Silk, M. J., Croft, D. P., Delahay, R. J., Hodgson, D. J., McDonald, R. A., et al. (2018). Social structure contains epidemics and regulates individual roles in disease transmission in a group-living mammal. Ecol. Evol. 8, 12044–12055. doi: 10.1002/ece3.4664
Rudolf, V. H., and Antonovics, J. (2005). Species coexistence and pathogens with frequency dependent transmission. Am. Nat. 166, 112–118. doi: 10.1086/430674
Salkeld, D. J., Padgett, K. A., and Jones, J. H. (2013). A meta-analysis suggesting that the relationship between biodiversity and risk of zoonotic pathogen transmission is idiosyncratic. Ecol. Lett. 16, 679–686. doi: 10.1111/ele.12101
Schmidt, K. A., and Ostfeld, R. S. (2001). Biodiversity and the dilution effect in disease ecology. Ecology 82, 609–619. doi: 10.1890/0012-9658(2001)082[0609:batdei]2.0.co;2
Silk, M. J., and Hodgson, D. J. (2020). Life history and population regulation shape demographic competence and influence the maintenance of endemic disease. Nat. Ecol. Evol. 318, 1–10. doi: 10.1038/s41559-020-01333-8
Strauss, A., White, A., and Boots, M. (2012). Invading with biological weapons: the importance of disease-mediated invasions. Funct. Ecol. 26, 1249–1261. doi: 10.1111/1365-2435.12011
Su, M., Yang, Y. Q., and Hui, C. (2020). How intraguild predation affects the host diversity-disease relationship in a multihost community. J. Theor. Biol. 490:110174. doi: 10.1016/j.jtbi.2020.110174
Thébault, E., and Fontaine, C. (2010). Stability of ecological communities and the architecture of mutualistic and trophic networks. Science 329, 853–856. doi: 10.1126/science.1188321
Young, H. S., Parker, I. M., Gilbert, G. S., Guerra, A. S., and Nunn, C. L. (2017). Introduced species, disease ecology, and biodiversity-disease relationships. Trends Ecol. Evol. 32, 41–54. doi: 10.1016/j.tree.2016.09.008
Keywords: ecological network, functional guild, species interactions, disease transmission, dilution effect
Citation: Su M, Jiang Z and Hui C (2022) How Multiple Interaction Types Affect Disease Spread and Dilution in Ecological Networks. Front. Ecol. Evol. 10:862986. doi: 10.3389/fevo.2022.862986
Received: 26 January 2022; Accepted: 28 April 2022;
Published: 18 May 2022.
Edited by:
Miguel Lurgi, Swansea University, United KingdomReviewed by:
Matthew Joseph Michalska-Smith, University of Minnesota Twin Cities, United StatesVirginia Dominguez-Garcia, Doñana Biological Station (CSIC), Spain
Copyright © 2022 Su, Jiang and Hui. This is an open-access article distributed under the terms of the Creative Commons Attribution License (CC BY). The use, distribution or reproduction in other forums is permitted, provided the original author(s) and the copyright owner(s) are credited and that the original publication in this journal is cited, in accordance with accepted academic practice. No use, distribution or reproduction is permitted which does not comply with these terms.
*Correspondence: Min Su, c3VtMDRAMTYzLmNvbQ==; Cang Hui, Y2h1aUBzdW4uYWMuemE=