- 1Nees Institute for Biodiversity of Plants, University of Bonn, Bonn, Germany
- 2Facultad de Ciencias Agronómicas and Departamento de Silvicultura y Conservación de la Naturaleza, Universidad de Chile, Santiago, Chile
- 3Botanic Garden and Botanical Museum Berlin (BGBM), Freie Universität Berlin, Berlin, Germany
- 4Escuela Profesional de Ingeniería Ambiental, Universidad Nacional de Moquegua, Moquegua, Peru
In this study we aim at refining our understanding of the floristic connectivity of the loma- and precordillera floras of southern Peru and northern Chile and the parameters determining vegetation cover in this region. We used multivariate analyses to test for floristic- and environmental similarity across 53 precordillera and loma locations in Peru and Chile. We propose the use of predictive modeling in estimating the extent of desert vegetation as a complementary method to remote sensing. We created habitat suitability models for the vegetation on the coast and in the precordillera based on a combination of latent bioclimatic variables and additional environmental predictors using Maxent. We found Peruvian and Chilean lomas to be strongly floristically differentiated, as are the Chilean precordillera and lomas. Conversely, there is clear connectivity between both the Peruvian loma- and precordillera floras on the one hand and the Peruvian and Chilean precordillera floras on the other. Divergent environmental conditions were retrieved as separating the precordillera and lomas, while environmental conditions are not differentiated between Peruvian and Chilean lomas. Peruvian and Chilean precordilleras show a gradual change in environmental conditions. Habitat suitability models of vegetation cover retrieve a gap for the loma vegetation along the coast between Peru and Chile, while a continuous belt of suitable habitats is retrieved along the Andean precordillera. Unsuitable habitat for loma vegetation north and south of the Chilean and Peruvian border likely represents an ecogeographic barrier responsible for the floristic divergence of Chilean and Peruvian lomas. Conversely, environmental parameters change continuously along the precordilleras, explaining the moderate differentiation of the corresponding floras. Our results underscore the idea of the desert core acting as an ecogeographic barrier separating the coast from the precordillera in Chile, while it has a more limited isolating function in Peru. We also find extensive potentially suitable habitats for both loma- and precordillera vegetation so far undetected by methods of remote sensing.
Introduction
The Pacific coast of Peru and Chile is generally considered as a desert comprising a belt of ∼3,500 km of hyperarid climate (Rundel et al., 1991). This hyperaridity is predominantly governed by the strong and stable Pacific Anticyclone and the upwelling cold waters of the Humboldt Current along the western coast of South America (Rodwell and Hoskins, 2001; Garreaud et al., 2010). At the same time, the Andean Cordillera shields the western coast against moist air from entering the northern Chilean Atacama from the east. Precipitation here averages < 1 mm/year (Houston, 2006), which goes back to years of zero precipitation (McKay et al., 2003) interrupted by rare events of torrential rains controlled by the El Niño Southern Oscillation (ENSO; Houston, 2006; Cai et al., 2020).
A closer look at this region, however, reveals a unique altitudinal zonation: Along the coast at elevations of ca. 200–1,000 m heavy sea fog forms during austral winter, caused by the condensation of moist Pacific air masses pushed against the steep slopes of the Coastal Cordillera (Rundel et al., 1991). In these areas of highly seasonal fog, oases develop, the so-called “loma vegetation” (loma—Spanish: “hill”; Ferreyra, 1961; Rundel et al., 1991). This loma vegetation and its typical flora have been extensively studied and documented in the past (e.g., Ferreyra, 1961; Dillon, 2005; Schulz et al., 2011; Muenchow et al., 2013), but taxonomic novelties keep being discovered even in this relatively well studied ecosystem (e.g., Hepp, 2018; Quipuscoa Silvestre and Dillon, 2018; Cáceres de Baldárrago et al., 2019). A recent study employing remote sensing mapped these fog oases with unprecedented precision, arguing that the spatial extent of fog oases is roughly four times as large as previously reported (Moat et al., 2021). This surprisingly indicates that floristic exploration of this peculiar vegetation type is still highly fragmentary. Moat et al. (2021) also concluded that highly ephemeral vegetation and “dormant” fog oasis may not be captured by their analyses. Locations which only develop vegetation at long intervals are thus generally overlooked, but may play a crucial role in providing stepping stones for the loma flora at secular and millennial time levels.
Above the upper limit of fog condensation at ca. 1,000 m there is a full desert, with plant life essentially restricted to phreatophytes (deep rooted plants). This region is called “piso desértico” in Peru (“desert level”; Weberbauer, 1945), respectively, “absolute desert” in Chile (Luebert and Pliscoff, 2017). This “desert level” grades into precordillera vegetation, starting with primarily ephemeral vegetation fed by the occasional spillover of subtropical summer rain at roughly 1,600 m (Schwarzer et al., 2010) to 2,200 m (Ruhm et al., 2020). Precordillera vegetation has been variously referred to as “prepuna” (e.g., Montesinos, 2012; Díaz et al., 2019), “matorral desértico” (Luebert and Pliscoff, 2017), or “sierra vegetation” (Schwarzer et al., 2010). Unlike the loma vegetation of the coast, this precordillera vegetation has been rarely studied and we are not aware of any comprehensive treatment. As our knowledge of this vegetation zone gradually increases, new records are being made and species new to science keep being discovered and described (e.g., Montesinos-Tubée et al., 2016; Quipuscoa Silvestre and Dillon, 2018; Montesinos-Tubée and Chicalla-Rios, 2021). At present, the floristics of the precordillera remains dramatically understudied and no comprehensive overview on the phytodiversity has been provided, neither for the entire Pacific coastal desert nor for either of the two countries. Precordillera vegetation is dominated by short-lived herbs, cacti and shrubs, shows high interannual variability (Díaz et al., 2019) and is characterized by generally low vegetation cover, making it unlikely to be recognized by remote sensing methods (Huete and Tucker, 1991; Escadafal, 2017). A recent study of Chávez et al. (2019) was able to detect flowering desert events based on GIMMS NDVI for the Chilean precordillera between 1981 and 2015 at 8 km pixel resolution. However, outside these events linked to strong precipitation, NDVI values were unable to detect vegetation. We are not aware of any study assessing desert vegetation along the precordillera on a finer scale. Above ca. 3,000 m, precordillera vegetation grades into puna vegetation dominated by shrubs and grasses (Luebert and Gajardo, 2005; Montesinos-Tubée et al., 2015; Luebert and Pliscoff, 2017).
The coastal loma- and Andean precordillera vegetation runs more or less parallel along the Peruvian and Chilean coast over thousands of kilometers, separated by a strip of desert ranging from a few kilometers in width in parts of Peru to over 100 km in northern Chile (Emck et al., 2006). Despite the short geographical distances separating the two formations in some places, they represent contrasting climatic regimes: The precordillera receives scant (austral) summer-rain along the Andean foothills, while the coast is exposed to an (austral) winter-rainfall/fog condensation regime (Weberbauer, 1945; Houston, 2006).
These contrasting climatic conditions are clearly reflected in floristic composition: A first qualitative comparison of the loma- and precordillera floras was recently published for northern Chile and the extreme south of Peru (Ruhm et al., 2020), demonstrating that in this region the precordillera flora is sharply segregated from the loma flora. Qualitative floristic differences between the costal loma- and precordillera floras in northern Chile have been previously reported and ascribed to geographic isolation on both sides of the hyperarid core of the desert (Villagrán et al., 1983; Rundel et al., 1991; Dillon, 2005), but other abiotic factors such as divergent precipitation patterns (Houston, 2006), temperature regimes (Luebert and Pliscoff, 2017) and solar radiation (Molina et al., 2017) likely also play an important role. However, for Peru closer floristic connection between the coastal lomas and Andean precordillera was already proposed by Koepcke (1961) and Sarmiento (1975). More recently, a study of Schwarzer et al. (2010) indicated that elements of typical loma vegetation and even presumed loma endemics may be found in certain habitats along the southern Peruvian precordillera reaching elevations of up to 2,600 m asl. Ruhm et al. (2020) demonstrated a relative floristic coherence along the precordillera from Chile to southern Peru, confirming the presence of a “floristic corridor” along the Andean precordillera of Peru and Chile as previously suggested (Moreno et al., 1994) and shown by phylogenetic studies (e.g., Luebert et al., 2009; Luebert and Weigend, 2014; Böhnert et al., 2022). This is in stark contrast to the “floristic break” between the loma floras of Peru and Chile, respectively, as previously hypothesized in floristic (Rundel et al., 1991; Galán de Mera et al., 1997; Pinto and Luebert, 2009; Manrique et al., 2014) and phylogenetic studies (Gengler-Nowak, 2002; Merklinger et al., 2021). Rundel et al. (1991) suggested that the interruption of the coastal cordillera in the “Pampa de Hospicio” north of Arica at the border between Chile and Peru might be the reason for this floristic discontinuity. In the absence of a steep coastal cordillera humid air pushing inland from the Pacific fails to condense into fog and instead dissipates on the coastal plain.
We here present the most comprehensive dataset to date for the vegetation in southern Peru and northern Chile (below 2,500 m), incorporating floristic data from 53 locations, aiming toward a refined understanding of the spatial extent, connectivity and floristic relationships of the loma- and precordillera in southern Peru and northern Chile. To achieve this, we investigate (a) floristic similarity of loma-and precordillera locations in Chile and Peru, (b) explore the parameters determining vegetation cover in this region and (c) model the current extent and distribution of loma- and precordillera vegetation to understand floristic connectivity and disparity based on the geographical ranges of the distinct vegetation types.
Also, for the analyses of environmental data and habitat suitability modeling, we present an approach combining so-called “latent” PCA derived (Dormann et al., 2013) bioclimatic variables (LBV’s) with additional “original” environmental variables (elevation, inclination and cloud cover) characterizing the habitat of desert vegetation in Peru and Chile. In habitat suitability modeling latent variable approaches have already been applied by several authors (Dupin et al., 2011; Marchi and Ducci, 2018; Senay and Worner, 2019). However, combining latent and original variables has—to the best of our knowledge—rarely been attempted. One of the few examples is Toro-Núñez et al. (2015).
We hypothesize that (1) the loma and precordillera floras in Peru exhibit a higher connectivity compared to the loma and precordillera floras in Chile. In support of this hypothesis, we expect a higher floristic similarity between the loma and precordillera floras in Peru contrasting with higher dissimilarity in Chile, as proposed by Ruhm et al. (2020). Secondly, (2) we expect the floristic composition of the lomas in Peru and Chile to be highly divergent, due to the ecogeographical barrier represented by the interruption of the coastal cordillera at the border between the two countries. We would find this hypothesis supported, if habitat suitability models indicated a pronounced gap for loma vegetation (Rundel et al., 1991; Pinto and Luebert, 2009). Conversely, we propose that (3) the precordillera floras of Peru and Chile show a closer connectivity (Luebert et al., 2009; Böhnert et al., 2022). We here expect a gradual latitudinal floristic change-over in a continuous belt of suitable habitat.
Materials and Methods
Analyses of Floristic Data
In total we analyzed floristic information of 53 coastal and Andean desert locations from southern Peru and northern Chile (Figure 1 and Table 1), including 870 species in 410 genera. All floristic- and subsequent environmental analyses were performed in R-4.0.5 (R Core Team, 2021). Floristic data from 21 mainly southern Peruvian locations, collected between 2009 and 2020, were combined with a previously presented list of floristic data from desert locations in Peru and Chile (Ruhm et al., 2020). From the data analyzed by Ruhm et al. (2020), one location, Rio Ilo-Moquegua, was excluded from the analyses (sampling covers an altitudinal range of more than 4,000 m, which is unsuitable for our objectives). Additional floristic data of coastal lomas and Andean precordillera locations were collated from the literature (León et al., 1997; Roque and Cano, 1999; Jiménez et al., 2008; Galán de Mera et al., 2009; Montesinos-Tubée and Mondragón, 2013; Silvestre et al., 2016) as well as from the online botanical collection of the Field Museum (Chicago, United States accessed: 18/08/2020).1 Floristic data from each location were transformed into a binary data matrix indicating for species occurrences. Coordinates (Figure 1) correspond to centers of the sampling areas or the approximate locations to which species were assigned to by the respective authors. Detailed information on each location, incl. data source, sampling method, altitudinal range and sampling period is provided in Supplementary Appendix 1. Explicit remarks on sampling methods can, if existing, be reviewed in the respective data source. Scientific names of species were assigned and revised according to Brako and Zarucchi (1993) for Peru and Zuloaga et al. (2008) for Chile. We followed Zuloaga et al. (2008) as the more recent source for species listed in both sources under different names. A complete list of the species reported across all locations is given in Supplementary Appendix 2.
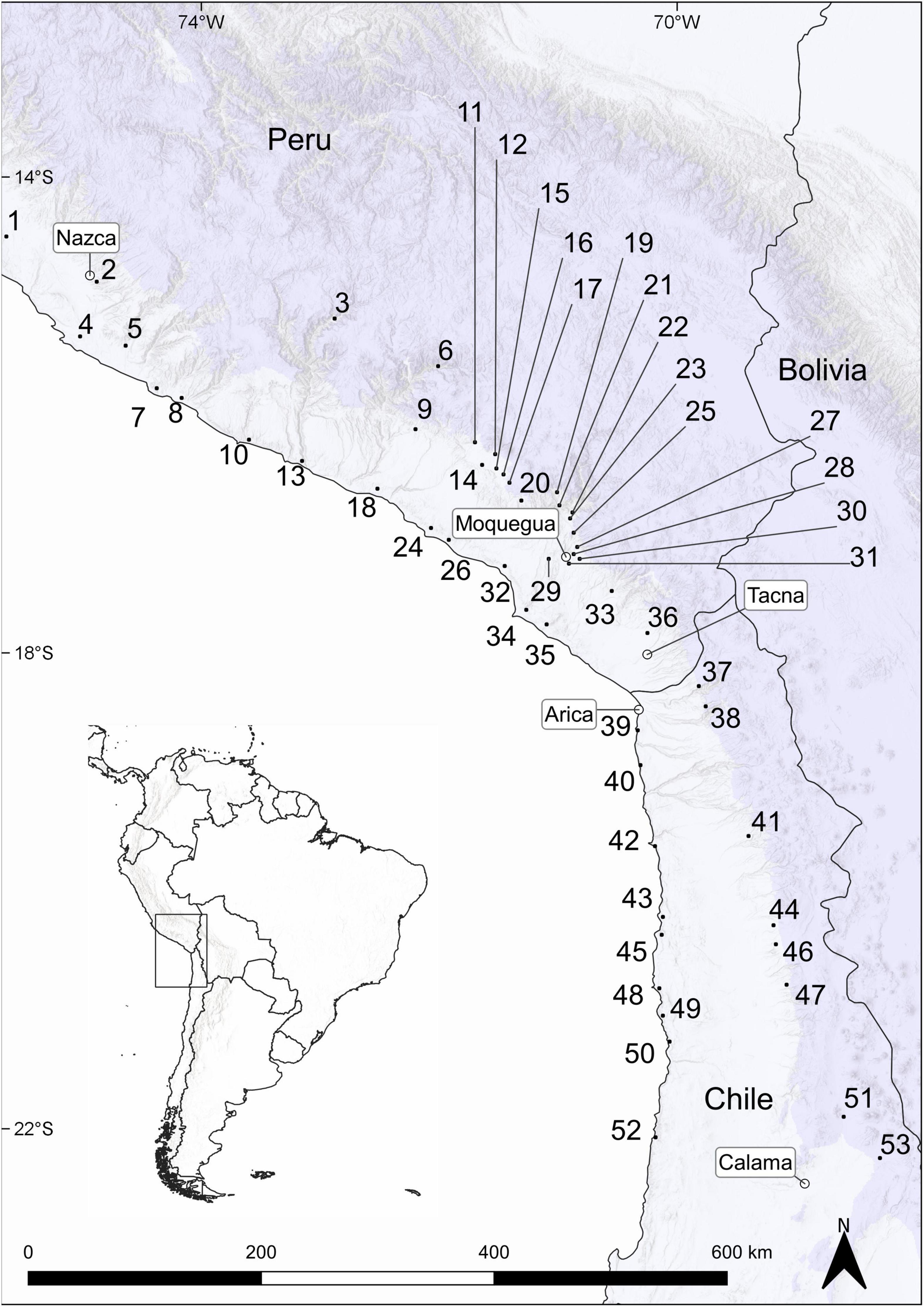
Figure 1. Coastal and Andean desert locations in southern Peru and northern Chile. Map was created in QGIS 3.14.16. Country layers were taken from Natural Earth (www.naturalearthdata.com). City layers were taken from MapCruzin (www.mapcruzin.com). Hillshade layer was taken from ESRI (Environmental System Research Institute [ESRI], 2020). Information on mean elevation of each raster cell (resolution: 30 s) was taken from GMTED2010 (Danielson and Gesch, 2011). For the interactive map see: https://rpubs.com/JRuhm/twosidessamedesert2022.
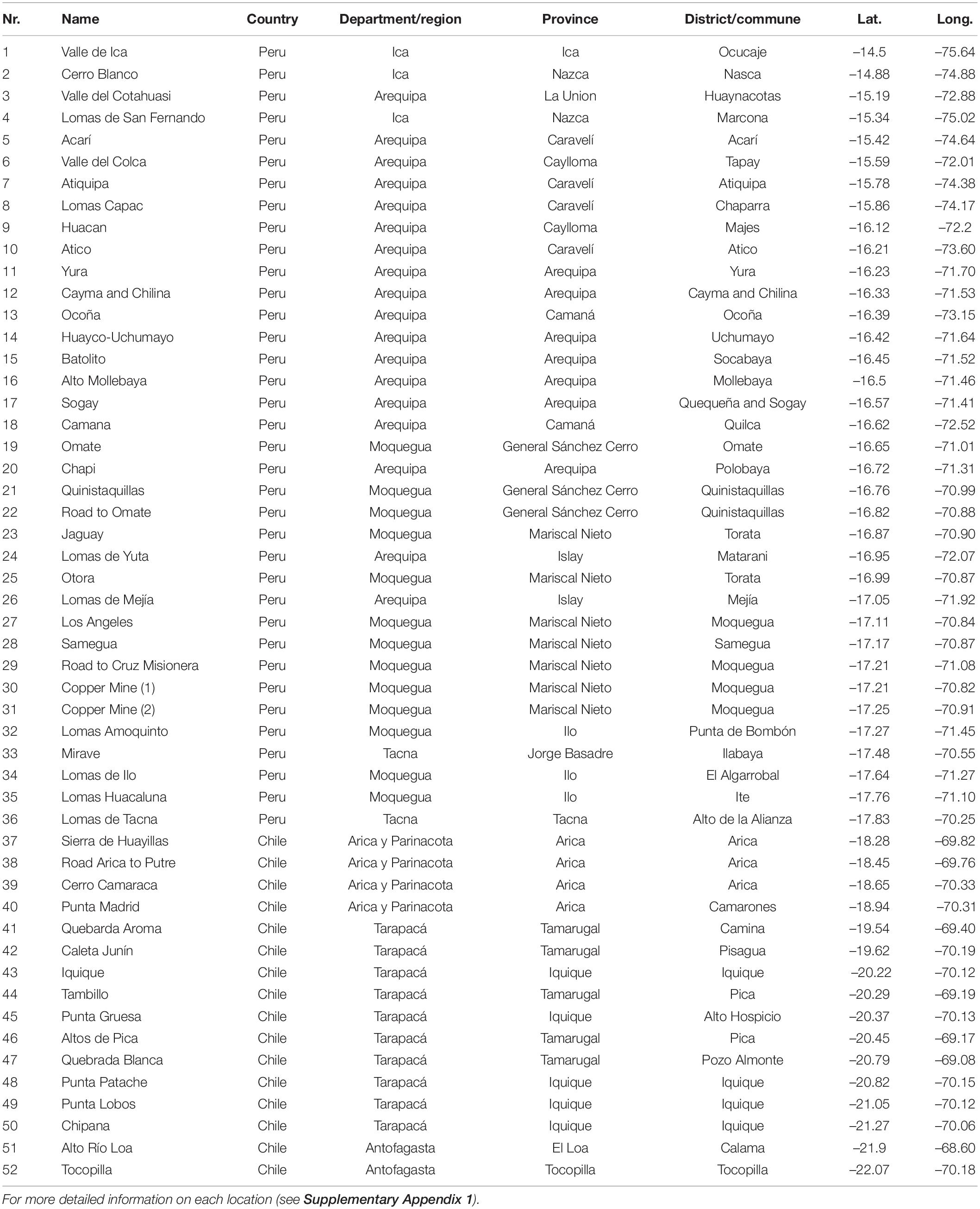
Table 1. List of locations indicated in Figure 1.
Pairwise floristic dissimilarities between the locations, based on presence/absence data of species were calculated using Sørensen dissimilarity employing the R-package “vegan” 2.5–7 (Oksanen et al., 2020). Based on the pairwise dissimilarities of locations we performed hierarchical clustering and inferred clusters from the resulting dendrogram. We peformed cluster analysis for four clustering methods (single linkage, complete linkage, Ward’s minimum variance and UPGMA) and evaluated the resulting dendrograms using cophenetic correlation and Gower’s distance. Based on both metrics, UPGMA produces the best results for our data and was therefore chosen. To display the results of the cluster analysis a final dendrogram was created using the R package “factoextra” 1.0.7 (Kassambara and Mundt, 2020). Complementary ordination analysis of the locations based on the pairwise floristic dissimilarities was conducted using a Principal Coordinates Analysis (PCoA). Results of the cluster analysis and PCoA were subsequently combined and groups were visualized along the first two PCoA axes. For each cluster, we calculated the total number of species and mean species number per location, and species overlap between clusters using Sørensen Similarity. Distances between cluster centroids were calculated making use of the function dist_between_centroids() in the R-package “usedist” 0.4.0 (Bittinger, 2020). Additionally, based on the phi-fielity we also identified sets of diagnostic species for each cluster with significance p < = 0.05 using the R package “indicspecies” (Cáceres and Legendre, 2009).
Analyses of Environmental Data
Raster layers of 19 bioclimatic variables with a resolution of 30 arc sec (ca. 1 × 1 km grid cells) were obtained from the CHELSA online database (Karger et al., 2017, 2018).2 Among those 19 bioclimatic variables,3 variable 15 (precipitation seasonality), had gaps around the southernmost coastal location in Chile and had to be excluded. Main climatic trends were summarized into principal components, conducting Principal Component Analysis (PCA) of the remaining 18 standardized raster layers of bioclimatic variables, which overcomes two issues, multicollinearity and overparameterization, both known to effect multivariable analysis (Warren and Seifert, 2011; Yoo et al., 2014). For that purpose, we utilized the function rasterPCA in the R package “RStoolbox” 0.2.6 (Leutner et al., 2019). Principal components where then used as so-called “latent” (unobserved) variables (Dormann et al., 2013) and are in the following referred to as latent bioclimatic variables (LBVs). Selection of a meaningful number of PCA derived axes is a key issue in ordination and different approaches have been proposed. We used the broken stick model following to Jackson (1993). Here, the first two LBVs were selected, cumulatively explaining more than 78% of the total variance from the original data. A table containing eigenvalues, proportion of total variance and cumulative proportion of total variance for each LBV is provided in Supplementary Appendix 3. An additional table with the coefficients (loadings) of the original variables for each LBV can be found in Supplementary Appendix 4. Figure 2 shows relationships between the 18 original bioclimatic variables and LBV1 (x-axis) and LBV2 (y-axis) derived from PCA. Variables most strongly associated with LBV 1 are primarily related to temperature (red): Min Temperature of Coldest Month (Bio 6), Mean Temperature of Coldest Quarter (Bio 11), Annual Mean Temperature (Bio 1), Mean Temperature of Wettest Quarter (Bio 8), Mean Temperature of Driest Quarter (Bio 9), Mean Temperature of Warmest Quarter (Bio 10). Original variables most strongly associated with the second LBV are primarily related to precipitation (blue): Precipitation of Coldest Quarter (Bio 19), Precipitation of Driest Month (Bio 14), Precipitation of Driest Quarter (Bio 17).
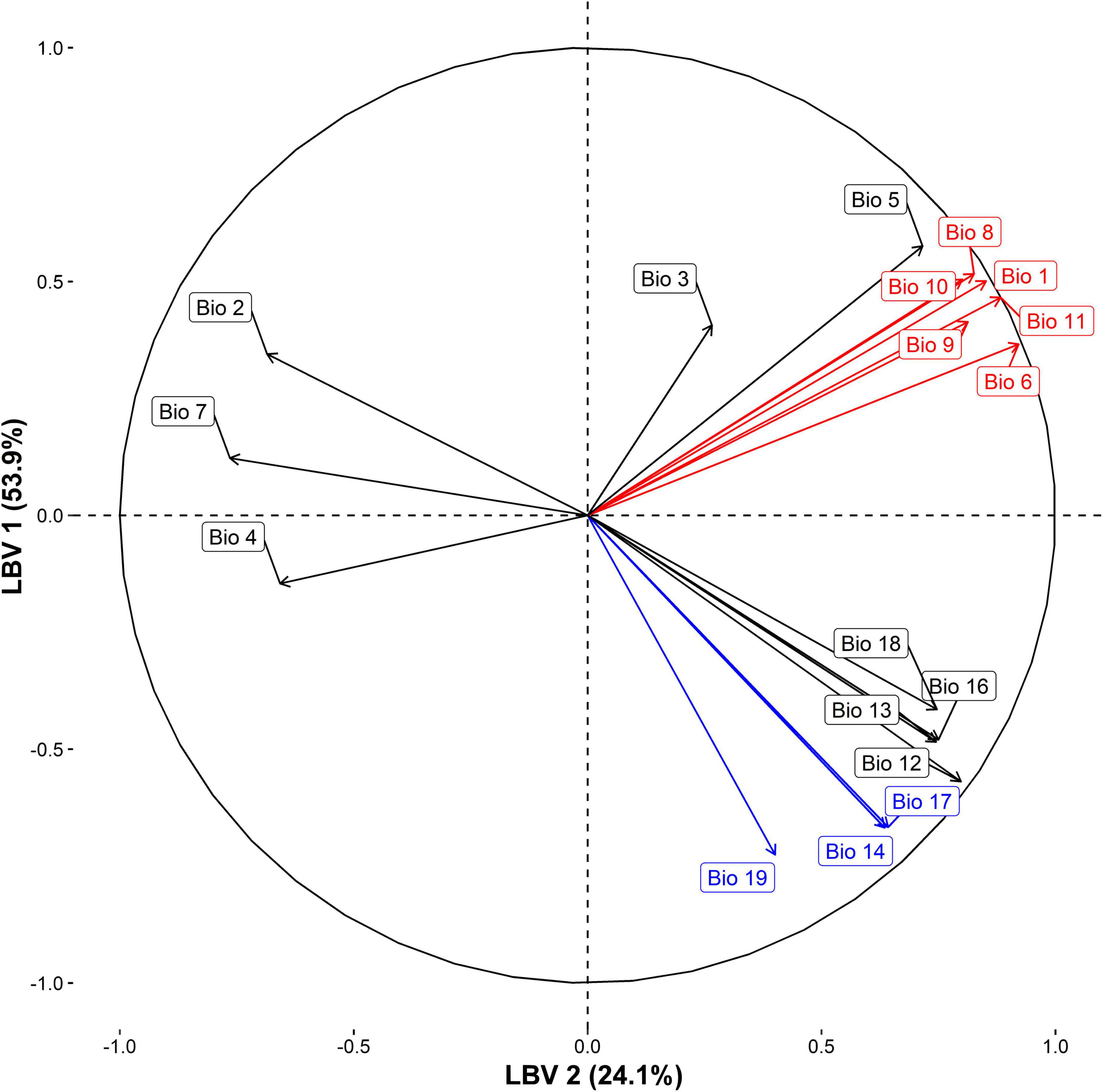
Figure 2. Correlation plot of 18 bioclimatic variables. X- and y-axis correspond to the first two components obtained from principal component analyses of the original bioclimatic variables. Principal components are used as so-called “latent” (unobserved) variables (Dormann et al., 2013) and are referred to as latent bioclimatic variables (LBVs). Direction of variables show associations with LBV1 and LBV2. Distances to the correlation circle indicate how well a variable is represented by LBV1 and LBV2. Bioclimatic variables were taken from the CHELSA online database (chelsa-climate.org; Karger et al., 2017, 2018) and were extracted for Peru, Chile and Bolivia.
The first two LBVs derived from PCA were then combined with additional environmental parameters characterizing the habitats of desert vegetation in Peru and Chile: elevation, inclination and mean August cloud cover, this combination was then used for further analysis. Information on mean elevation per grid cell at a resolution of 30 arc-seconds was derived from the Global Multi-resolution Terrain Elevation Data 2010 (GMTED2010; Danielson and Gesch, 2011). Inclination per grid cell was calculated from raster elevation data using the function in the R package “raster” 3.4–13 (Hijmans, 2021) applying the Horn algorithm with eight neighbors. We found mean August cloud cover best reflecting the differences between the two cloud regimes of the coastal lomas and Andean precordillera; cloud data were acquired from Global 1-km Cloud Cover (Wilson and Jetz, 2016)4 and extracted for Peru and Chile using the R package “raster” 3.4–13.
Parallel to the cluster- and ordination analyses of locations based on the pairwise floristic data we performed cluster- and ordination analysis of the locations based on the combined set of latent bioclimatic variables and the additional environmental parameters (LBV1 LBV2, elevation, inclination, and mean August cloud cover). For a comparison of results we cut the dendrogram into the number of clusters which we had previously inferred from the dendrogram based on the analysis of floristic data. For each location, values for each parameter were extracted from raster files making use of the R-package “raster” 3.4–13. We calculated pairwise similarities for locations using Euclidean distance based on standardized values. Pairwise distances between locations were used for cluster analysis and PCoA using the same methods as described in Analyses of Floristic Data.
Habitat Suitability Models
Maxent (Phillips et al., 2017) has become the most popular algorithm for predictive modeling of species distribution over the last decades. In the present study we make use of the ability of Maxent to predict an object’s range based on predictor variables and presence-only data, applying it to the desert vegetation in northern Chile and southern Peru. Valavi et al. (2022) underscored the high predictive performance of Maxent, particularly outperforming other methods when the number of occurrences is low. We used Maxent’s output “cloglog” because it provides good visual distinction of values within the same scale (0–1; Phillips et al., 2017), facilitating interpretation and comparison of models. Model predictions were based on the combined set of latent bioclimatic variables and the additional environmental parameters (LBV1 LBV2, elevation, inclination, and mean August cloud cover). Model predictions were made inside the calibration range, related to a minimum bounding box including all 53 locations. Habitat suitability models were created for (1) loma vegetation, (2) precordillera vegetation and (3) total extent of the vegetation in the study area combining both datasets. For that purpose, we regarded the locations (Figure 1 and Table 1) as true presences of vegetation and analyzed them in three sets: (1) only coastal locations; n = 25, (2) only precordillera locations; n = 29 and (3) all 53 locations; n = 53. For each of the three sets we created models with different combinations of parameters and selected the best performing model based on AUC and mean absolute error (MAE) as it has been recently suggested by Konowalik and Nosol (2021). We used the average validation AUC calculated across all iterations of cross validation for each model. MAE was applied to measure the goodness of fit for each model prediction compared to the known limits of loma- and precordillera vegetation in Peru and Chile. For that purpose, we mapped the desert core in Peru and Chile as non-habitable zone based on ombrothermicity, thermicity (Rivas-Martínez et al., 2011) and evapotranspiration following the definition of Luebert and Pliscoff (2017). Mean annual evapotranspiration, thermicity and ombrothermicity were calculated based on Chelsa climatic data (see text footnote 2; Karger et al., 2017, 2018). Subsequently, AUC and MAE were plotted against each other on a graph, comparing values for each model. For each set of locations (vegetation type) the model with highest AUC and lowest MAE was selected.
Models were created and evaluated using the R-package “ENMeval” (Kass et al., 2021). For model creation we followed the example of Muscarella et al. (2014) and used eight different values for the regularization multiplier (rm) ranging from 0.5 to 4.0 in steps of 0.5 together with six different combinations of feature classes (fcs), L, LQ, H, LQH, LQHP, LQHPT (L = linear, Q = quadratic; P = product; H = hinge, T = threshold; see also Merow et al., 2013), resulting in 48 models for each vegetation type. We sampled 10,000 background points randomly within a buffer a 100 km around each location, excluding the locations, which represented presence points. Locations were partitioned using the “checkerboard2” method to avoid spatial auto-correlation between locations as advocated by Radosavljevic and Anderson (2014) for training and validation of models for precordillera and total desert vegetation. For training and validation of the models for loma vegetation, n = 25, locations were partitioned using the “jackknife” method. The “jackknife” method is believed to make maximum use of the limited information for small sample sizes (< ca. 25; Shcheglovitova and Anderson, 2013). For modeling we chose the original “maxent.jar” algorithm. A first inspection of model predictions outside the calibration range revealed suitable habitats especially for loma vegetation along the eastern flanks of the Andes and inter-Andean valleys in Peru and Bolivia, which can be attributed to artifacts resulting from the narrow calibration range (100 km around each location) of models. We checked this by increasing the calibration range from which background points were sampled. However, for the sake of specificity (Barbet-Massin et al., 2012), we kept the narrow calibration range and masked model predictions beyond the extent of the Peruvian and Chilean Atacama Desert. For that purpose, we only considered model predictions for ultrahyperarid, hyperarid and arid climates following the classification of Rivas-Martínez et al. (2011). For a quantitative analysis of potentially suitable habitat of the entire loma- and precordillera vegetation in Peru and Chile we used our models to map suitable habitat between 5.8 and 28.5°S following the study extent of Moat et al. (2021). Maxent results were transformed to binary predictions (suitable/unsuitable) using the 10th percentile training presence as thresholding method. Liu et al. (2016) were able to demonstrate that 10th percentile training presence retrieves best results compared to other methods when estimating distribution ranges of mammals. The potential area of each model prediction was calculated based on all raster cells (1 km2) above the threshold using the r package “raster” 3.4–13.
Results
Results can be viewed as interactive map.5 Information and the respective species list are provided for each location and can be obtained by clicking.
Analyses of Floristic Data
The dendrogram based on the cluster analysis of floristic data (Figure 3A) shows an early branching into a cluster uniting precordillera locations and a second cluster uniting loma locations. Both clusters are divided into groups of Chilean and Peruvian locations resulting in a total of four distinct clusters, that can be related to the geographical regions: (1) precordillera Peru, (2) precordillera Chile, (3) lomas Peru and (4) lomas Chile. Within the lomas Peru cluster, the analysis retrieves a northern (1, 2, 4, 5) and a southern subgroup (7, 8, 10, 13, 18, 24, 26, 32, 34, 35, 36). Conversely, there is no clear latitudinal subdivision for the lomas Chile cluster. Within the precordillera Peru cluster a subgroup of locations from higher elevations (11, 12, 15, 17, 20, 21, 22, 23, 25, 27, 31) and a subgroup of locations from lower elevations (14, 19, 28, 29, 30, 33) can be identified. For the Chilean precordillera cluster, the analysis retrieves a core group of locations (38, 41, 44, 46, 47) and a group combining locations north and south of this core group (37, 51, 53). In Figure 3B the combined results of the cluster analysis and the ordination along the first two axes obtained from the PCoA based on floristic pairwise distances between locations are shown (variance explained: A1 = 9.3%, A2 = 7.1%). Low variance of the axes (A1 and A2) in the PCoA (Figure 3B) can be attributed to the binary data structure and high number of species present only in small subsets of locations, without affecting the explanatory power. The ordination shows close relationships between the precordillera Chile and precordillera Peru clusters, mainly separated along A2. The two southernmost Peruvian precordillera locations Nr. 30 (Copper Mine (1) and Nr. 33 (Mirave) are placed closer to the precordillera Chile cluster than to the precordillera Peru cluster, underscoring the close similarity and gradual transition. The lomas Peru cluster is separated mainly along A2 from the precordillera Peru and precordillera Chile clusters, indicating closer floristic proximity than between the lomas Peru and lomas Chile cluster.
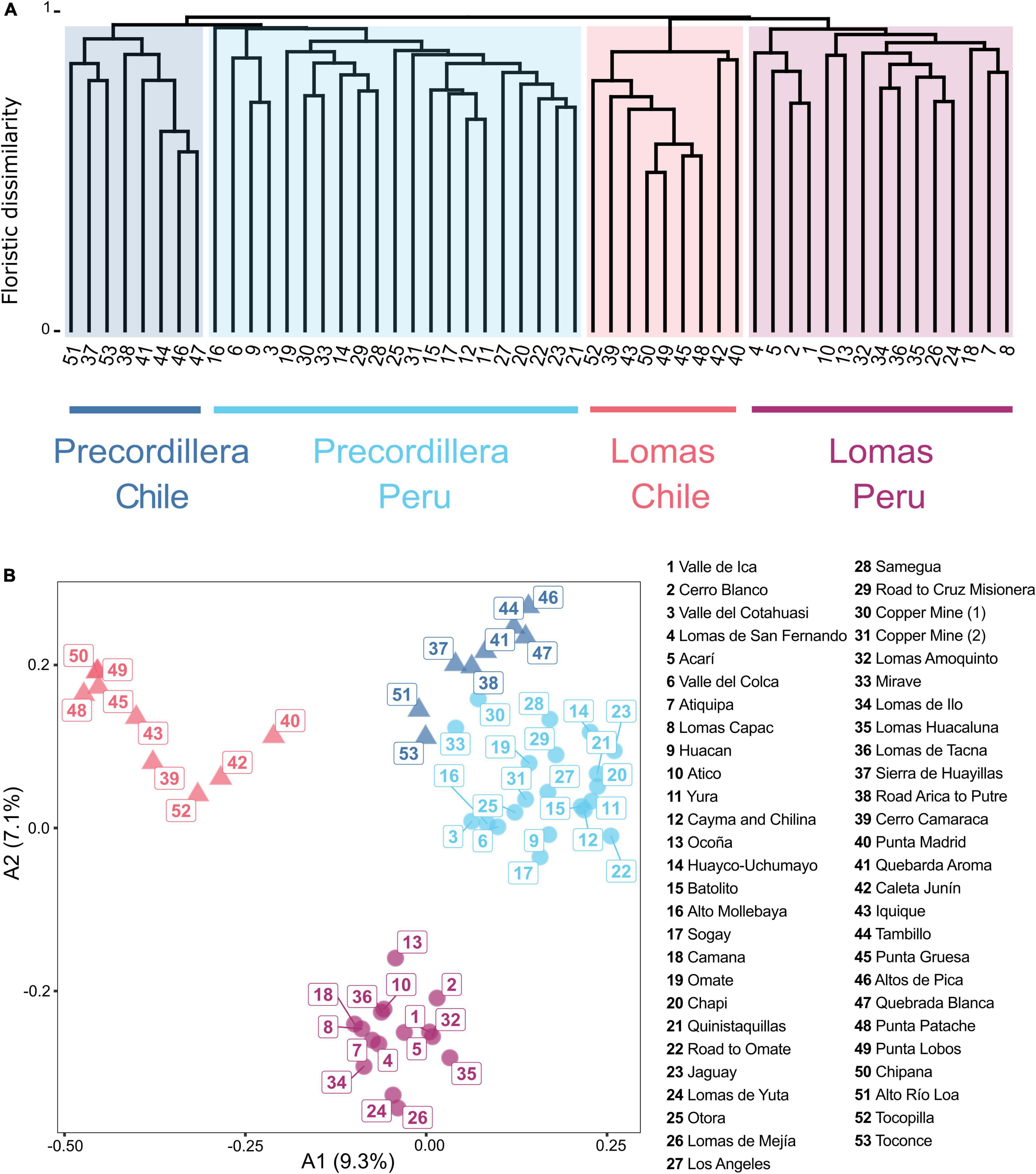
Figure 3. (A) Dendrogram based on cluster analysis of pairwise floristic dissimilarity (Sørensen index) between Peruvian and Chilean desert locations. Clustering is based on UPGMA. Cluster colors code the geographical regions displayed at the bottom. Numbers in square brackets refer to the numbers in the Overview map (Figure 1). (B) Ordination of Peruvian (circle) and Chilean (triangle) desert locations based on pairwise floristic dissimilarities (Sørensen index). X- and y-axis correspond to the first two axes (A1 and A2) of PCoA of floristic data. Values of A1 and A2 indicate proportion of total variance explained by each axis. Colors of locations indicate cluster identities retrieved from cluster analysis related to the geographical region. Separation of the Lomas Peru cluster from the Lomas Chile cluster contrasts with the higher affinities between the Precordillera Chile and the Precordillera Peru cluster.
Overall, we find a decrease in species number from west to east and from north to south (Figure 4) across the entire study area. The Peruvian lomas show the highest number of species, while the Chilean precordillera harbors the fewest species. Within the lomas Peru cluster we report 530 species overall and an average of 84 species per location. This contrasts with the precordillera Chile cluster with 122 species in total and an average of 30 species per location. Different from the cluster analysis (Figure 3A), the similarity analysis of clusters (Figure 4) shows a higher percentage of shared species between the precordillera Peru cluster and the lomas Peru cluster (27.5%, 113 species) than between the precordillera Peru cluster and the precordillera Chile cluster (25.6%, 53 species; Figure 5). Conversely, the dissimilarity between lomas Peru and lomas Chile holds up in the similarity analysis (15.5% shared species, 54 species; Figure 4), as does the dissimilarity between precordillera Chile and lomas Chile (11%, 16 species, Figure 4). A comparison of the distances between cluster centroids also shows the shortest distance between the precordillera Peru and lomas Peru clusters. In total phi-fidelity index identified 250 out of 870 species as characteristic for one of the four clusters. Lists of the characteristic species together with their respective fidelity and significance values are provided in Supplementary Appendix 5.
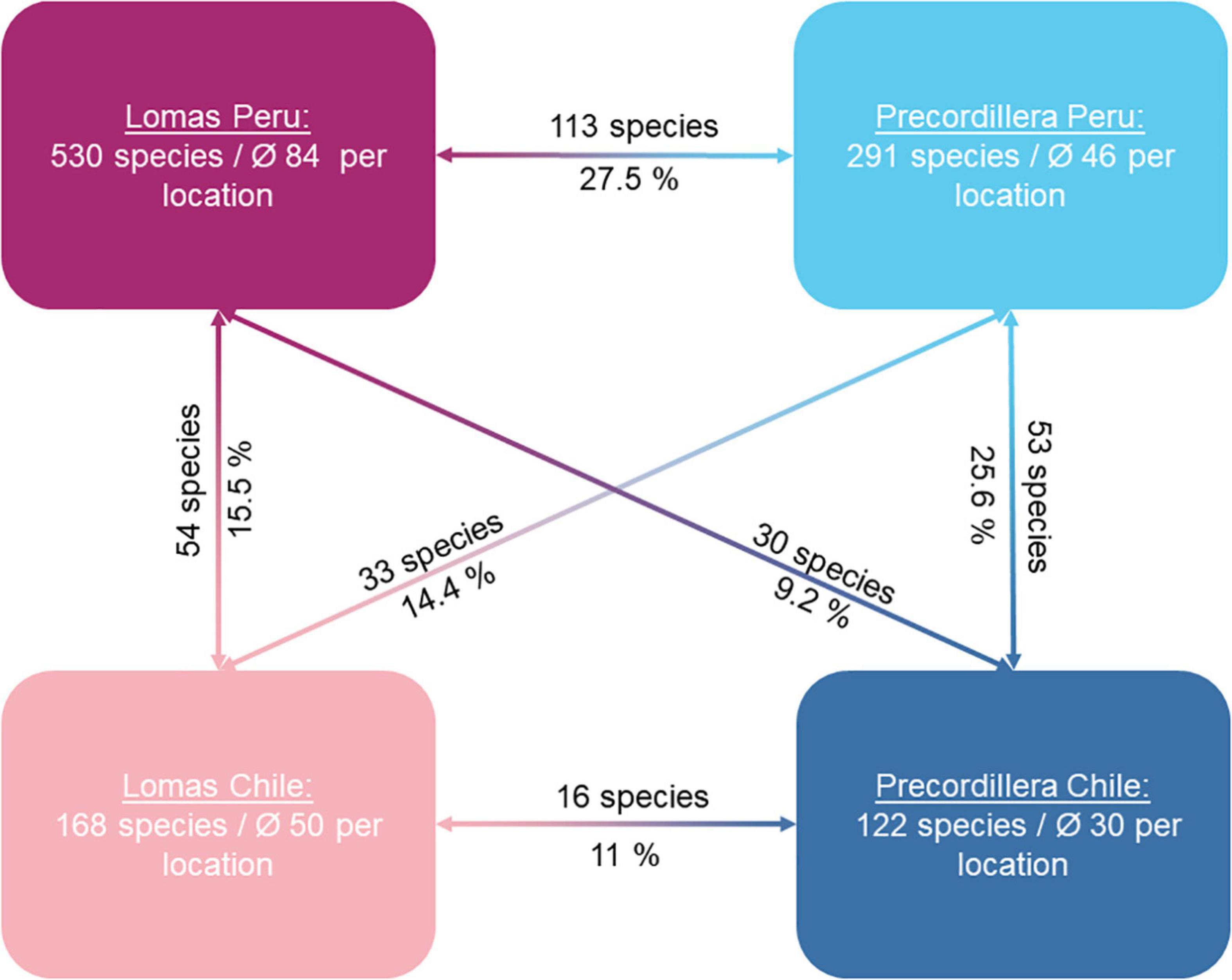
Figure 4. Constellation diagram of clusters indicating total species number per cluster, average species number per location per cluster and overlap of species between clusters (Sørensen Index shown as percent). Pairwise similarity between clusters is highest for the lomas Peru and precordillera Peru cluster while the lomas Peru cluster and precordillera Chile cluster show the lowest similarity. Low similarity between the lomas Peru and lomas Chile cluster is in contrast to higher affinities between the both precordillera clusters.
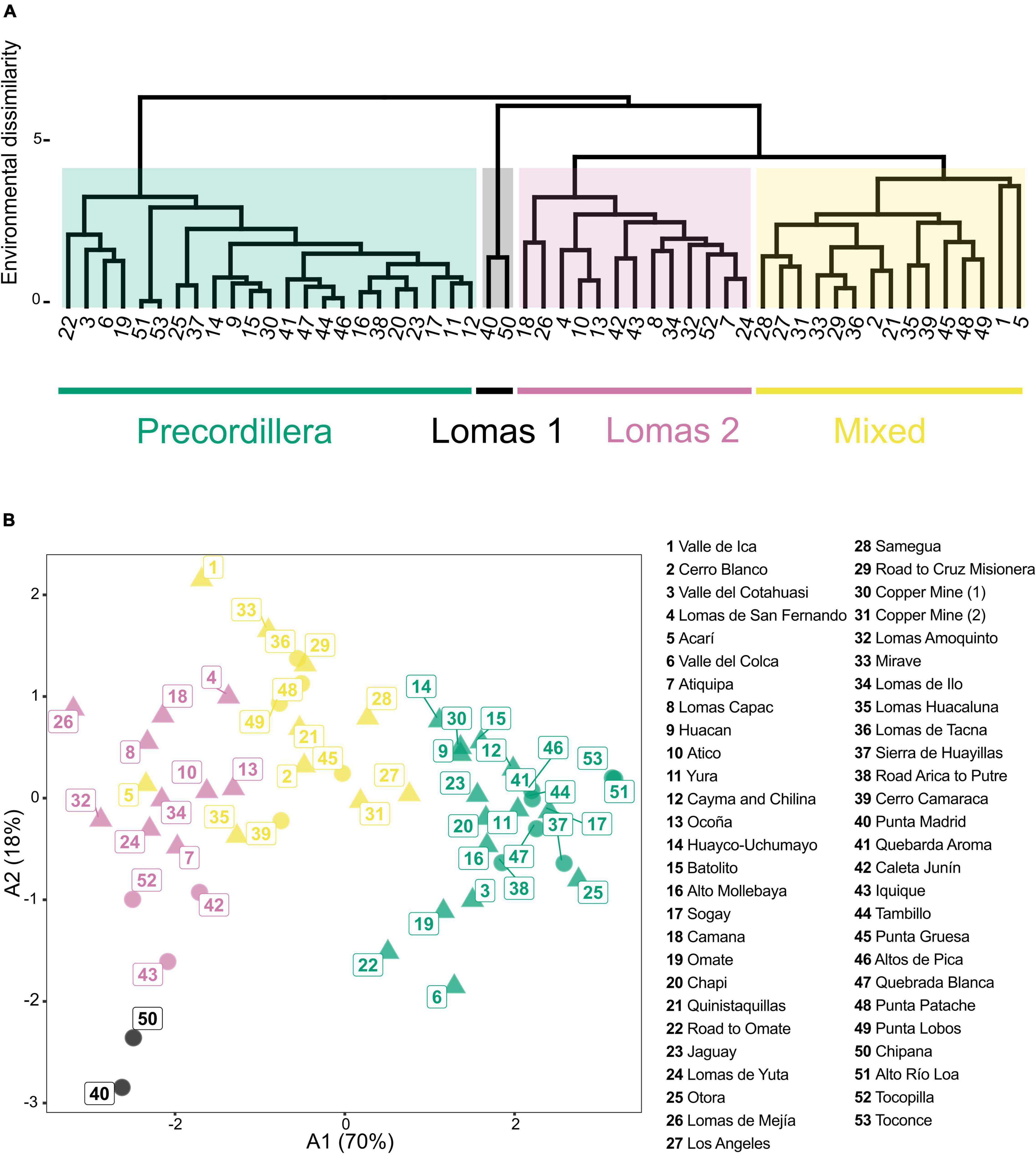
Figure 5. (A) Dendrogram based on cluster analysis of environmental data. between Peruvian and Chilean desert locations. Pairwise distances between locations were calculated using Euclidean distance. Clustering is based on UPGMA. Numbers refer to the numbers in the Overview map (Figure 1). (B) Principal component analysis (PCA) of Peruvian (circle) and Chilean (triangle) desert locations based on environmental data. X- and y-axis correspond to the first two axes (A1 and A2) from PCA of environmental data. Values of A1 and A2 indicate proportion of total variance explained by each axis. Colors of locations indicate cluster identities retrieved from cluster analysis (Figure 6A). Ordination retrieves lomas clusters separated from the precordillera cluster with the mixed cluster in between.
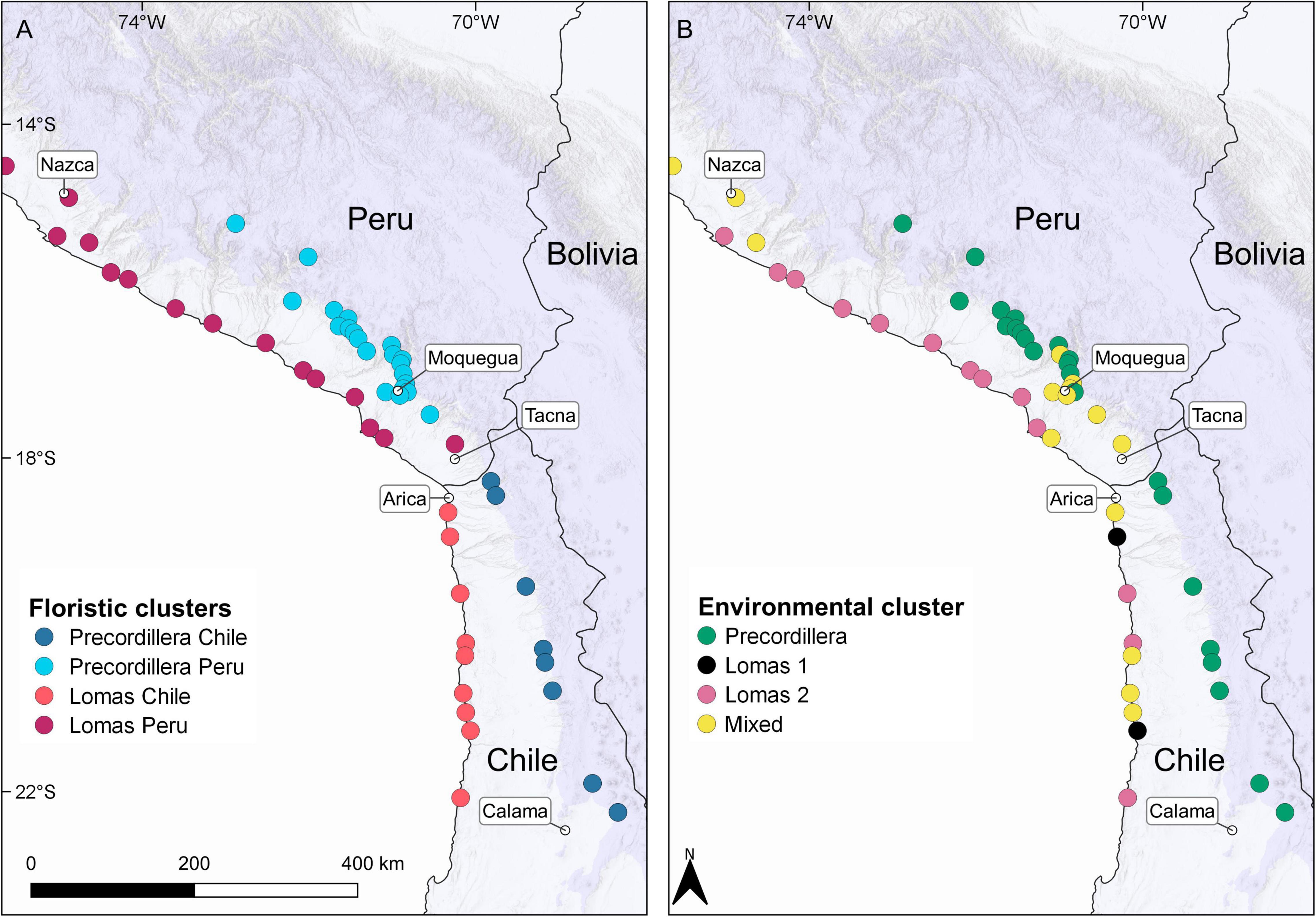
Figure 6. Overview of Locations color coded according to the results from cluster analyses (UPGMA) of (A) floristic data and (B) environmental data. Maps were created in QGIS 3.14.16. Country layers were taken from Natural Earth (www.naturalearthdata.com). City layers were taken from MapCruzin (www.mapcruzin.com). Hillshade layer was taken from ESRI (Environmental System Research Institute [ESRI], 2020). Information on mean elevation of each raster cell (resolution: 30 s) was taken from GMTED2010 (Danielson and Gesch, 2011). For the interactive map see: https://rpubs.com/JRuhm/twosidessamedesert2022.
Analyses of Environmental Data
The dendrogram resulted from cluster analysis of locations based on environmental variables is shown in Figure 5A. Cutting the dendrogram into four groups retrieves the clusters: (1) precordillera, (2) lomas 1, (3) lomas 2, and (4) mixed. The precordillera cluster units Chilean precordillera locations and most of the Peruvian precordillera locations, additionally retrieving a subgroup of Chilean precordillera locations (41, 44, 46, 47). The dendrogram also shows two lomas cluster (lomas 1 and lomas 2) uniting loma locations from both Peru and Chile. The mixed cluster mainly unites Peruvian precordillera locations from lower elevations (compare Supplementary Appendix 4) and Peruvian and Chilean loma locations. Combined results of cluster analysis and ordination along the first two axes (A1 and A2) obtained from PCoA are shown in Figure 5B. The ordination retrieves the precordillera and lomas clusters separated from each other. The mixed cluster is found in between the lomas and precordillera clusters. However, it can be seen that actual coastal locations retrieved in the mixed cluster (e.g., 5, 35, 39, 49) are grouped close or even within the lomas 1 cluster. Additionally, actual precordillera locations (e.g., 27, 28, 31) found in the mixed cluster show closer affinities to the precordillera cluster. Figure 6 shows locations color coded according to their cluster identities based on floristic data (Figure 6A) and environmental data (Figure 6B).
Habitat Suitability Models
Graphs used for model evaluation are shown in Supplementary Appendix 6. Values of AUC and MAE for each model are listed in Supplementary Appendix 7. In general, all models for the three vegetation types had high AUC values between 0.95 and 0.97 and differed mainly in values for MAE. In so far as different models had similar AUC values we selected the one with lower MAE.
For loma vegetation the selected model (fc = LQHP, rm = 1) predicts highly suitable habitat along most of the coast of Peru and Chile with a clear interruption on both sides of the Peruvian/Chilean border (Figure 7A). For this region, the model further shows a fragmentation of suitable loma habitats and a displacement inland toward the Andean Cordillera. Based on the extrapolation of the model predictions for the entire coast of Peru and Northern Chile and applying the 10th percentile training presence the estimated area of potentially suitable habitat of loma vegetation covers more than 31,000 km2 in Peru and Chile.
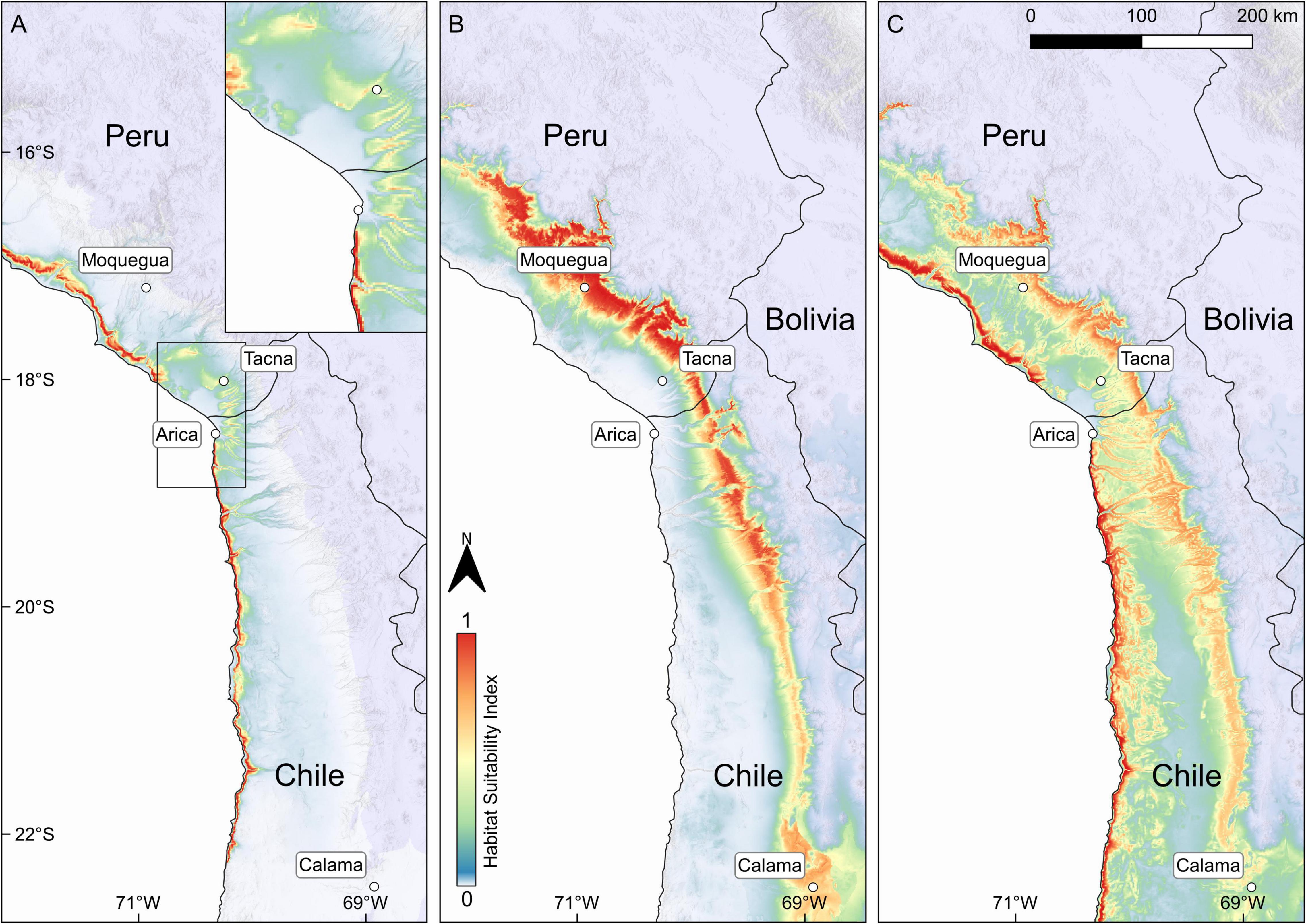
Figure 7. Habitat suitability models of (A) loma- (B) precordillera- and for (C) the entire vegetation in the southern Peruvian- and northern Atacama Desert. For each vegetation type the model with highest AUC and lowest MAE is presented. Final maps were created in QGIS 3.14.16. Country layers were taken from Natural Earth (www.naturalearthdata.com). City layers were taken from MapCruzin (www.mapcruzin.com). Hillshade layer was taken from ESRI (Environmental System Research Institute [ESRI], 2020). Information on mean elevation of each raster cell (resolution: 30 s) was taken from GMTED2010 (Danielson and Gesch, 2011). For the interactive map see: https://rpubs.com/JRuhm/twosidessamedesert2022. Permission to reuse and Copyright.
The model selected for precordillera vegetation (fc = LQH, rm = 1; Figure 7B) retrieves a continuous belt of highly suitable habitat along the Andean precordillera in Peru and Chile. Toward the south habitat suitability decreases and the habitat belt narrows until it reaches the city of Calama, Chile. A broader patch of moderately to highly suitable habitat is retrieved around Calama. Based on model extrapolation we calculated a total area of 51,000 km2 of precordillera vegetation in Peru and Chile.
The model for the entire vegetation cover (fc = LQH, rm = 1; Figure 7C) clearly predicts highly suitable habitat along the coast (and to a lesser degree along the Andean precordillera) separated by low suitability values within the desert core. Additionally, the model retrieves potentially suitable habitat for desert vegetation further inland along the coast and along the slopes of the larger canyons crossing the desert core in northern Chile. Similar to the model for loma vegetation, the model retrieves a clear interruption of suitable habitat on both sides of the Peruvian and Chilean border as well as highly suitable habitat around the slopes of Tacna, Peru. Based on our model we estimated the area occupied by desert vegetation in entire Peru and northern Chile to more than 157,000 km2.
A comparison of variable contribution and permutation importance of variables (Supplementary Appendix 8) shows significant differences between the selected models of different vegetation types. The model of loma vegetation mainly relies on the non-climatic variables: elevation, cloud cover and inclination. In contrast, the model of the precordillera vegetation is largely based on LBV2 (24% proportion of variance explained; Supplementary Appendix 3), essentially associated with precipitation variables. The model for the entire desert vegetation mostly relies on elevation, but also—to some degree—on LBV2, followed by inclination, cloud cover and LBV1 (54% of variance explained; Supplementary Appendix 3) which is mainly associated with variables related to temperature. Comparably high values of permutation importance additionally highlights the significance of LBV2 for the predictive power of the models for precordillera and the entire desert vegetation. In general, it can be seen that cloud cover, elevation and LBV2 have the most significant impact on models, while inclination and LBV1 are of less importance.
Discussion
Floristic Relationships of Loma- and Precordillera Vegetation
We clearly confirm the presence of a close floristic connection between the loma- and precordillera vegetation in Peru as previously proposed (Koepcke, 1961; Sarmiento, 1975; Ruhm et al., 2020). This contrasts with the strong floristic differentiation between the precordillera and lomas of Chile as indicated by Ruhm et al. (2020). We also find clear support for a floristic turnover in the coastal loma vegetation near the border between Peru and Chile, contrasting with the floristic connectivity in the precordillera vegetation between the north of Chile and south of Peru. A continuous transition of environmental conditions along the Peruvian and Chilean precordillera explains floristic differentiation, but also floristic similarity between both regions. Clear connectivity is retrieved despite the relatively low sampling density directly north of the Chilean/Peruvian border, which should overemphasize floristic discontinuity, because clinal changes are inadequately reflected in our sampling. We thus confirm both the floristic isolation of the coastal flora of Chile from that of Peru as previously proposed (Rundel et al., 1991; Pinto and Luebert, 2009; Ruhm et al., 2020) and the existence of an “Andean corridor” connecting precordillera locations (Moreno et al., 1994; Luebert and Weigend, 2014; Ruhm et al., 2020). The similarity of the environmental conditions in the Peruvian and Chilean loma locations clearly contradicts an ecological differentiation between the floras of the respective loma areas, leaving a geographic barrier as the most plausible cause of floristic divergence (Rundel et al., 1991).
We argue that ecogeographic and hence also floristic isolation of the coastal loma- and precordillera vegetation in Peru is less effective due to a much narrower desert core than in northern Chile. The physical extension of the full desert reaches its maximum in northern Chile (Emck et al., 2006; Luebert and Pliscoff, 2017), thus explaining the lack of floristic connectivity between Chilean lomas and precordillera compared to Peruvian lomas and precordillera. However, floristic exchange between coastal lomas and Andean precordillera locations in Peru as well as Chile is probably also restricted by diverging environmental conditions and exchange might be therefore reserved to more generalist taxa (Schwarzer et al., 2010).
The floristic data here used were compiled from a large number of different sources and across decades, which might be the cause of errors based, e.g., on different levels of expertise of the compiling researchers and shifting taxonomic concepts (Jansen and Dengler, 2010). The data sets here included are based on different approaches, varying e.g., in sizes and altitudinal ranges of the study areas and had to be extensively standardized for analysis. The streamlined and critically revised data set here used now represents the most comprehensive floristic inventory of the region to date. There is some degree of bias in the dataset based on data availability: Peruvian and Chilean loma vegetation has been studied extensively in the past decades, not least because of its relative accessibility, whereas especially the precordillera remains generally more poorly explored (compare Montenegro-Hoyos et al., 2022). Mutke and Weigend (2017) demonstrated how much such inconsistent sampling density might influence our knowledge of biodiversity patterns for the tropical Andes. Despite these limitations, our analyses yield robust results, demonstrating a clear division into floristic (sub-) regions.
Spatial Extent of Vegetation Cover
Based on point data of the 53 locations we modeled the spatial distribution of potential vegetation cover along both sides of the desert across the study area. This is the first attempt at modeling the precordillera vegetation, retrieving an extensive—and poorly sampled—vegetation belt at the upper limit of the desert level. Similarly, we reconstruct an extensive loma belt along the coast. A very detailed map of loma vegetation based on MODIS observation data was recently published by Moat et al. (2021) dramatically expanding the spatial extent of known loma vegetation compared to what was previously known from the literature. Moat et al. (2021), however, highlight the phenomenon that their analysis can only reflect the relatively few major vegetation events covered by their 20-year data set: Large-scale emergence of the mostly ephemeral flora is considered a rare phenomenon happening only once in decades and generally linked to ENSO-events (Schulz et al., 2011; Luebert and Pliscoff, 2017; Tovar et al., 2018; Chávez et al., 2019). Our study is based on climatic modeling using point data of desert vegetation and the Chelsa climatic data based on monthly records from 1979 to 2013. We believe that this provides a very good climatic baseline beyond the past three decades.
The results of our habitat suitability models are strikingly complementary with the results of floristic analysis (Figures 3A,B), underscoring the floristic break along the coast while clearly retrieving the relative connectivity along the Andean precordillera and also the relatively low degree of separation between the lomas and precordillera in Peru. Based on the model for loma vegetation, the floristic separation (floristic break) along the Peruvian and Chilean coast can be attributed to unsuitable habitat north and south of the border (Figure 7A) as previously suggested by Rundel et al. (1991) and Pinto and Luebert (2009). Further, we can demonstrate an inland shift of loma vegetation toward the Andean precordillera between the cities of Tacna, Peru and Arica, Chile (Figure 7A). The relatively low habitat suitability retrieved for this region potentially indicates highly ephemeral loma vegetation only emerging under rare, favorable climatic conditions and impossible to detect by remote sensing methods (e.g., Moat et al., 2021). We are not aware of any detailed floristic data from this region other than reports of Tillandsiales (e.g., Pauca-Tanco et al., 2020), which typically occur outside the actual loma vegetation. Along the entire coast of Peru and northern Chile the range of medium to highly suitable habitat (ca. 0.4–1) for loma vegetation here retrieved closely follows the extent and categories of loma vegetation presented by Moat et al. (2021). However, applying the 10th percentile training presence recovers also areas with relatively low habitat suitability as potentially habitable for loma vegetation, significantly increasing the potential extent of loma vegetation. Overall, we can confirm that, based on our analyses, the spatial extent of the coastal loma vegetation is much larger than previous studies suggested and possibly even larger than estimated by Moat et al. (2021) when highly ephemeral vegetation is considered. Floristic connections between the precordilleras of Peru and Chile (Figure 3) are correlated to a continuous belt of highly suitable habitats retrieved along the entire part of the precordillera here studied. In general, Andean precordillera vegetation is extremely sparse (Villagrán et al., 1981) and vegetation cover, even in more humid years, is low (Ruhm et al., 2020). Detection of such widely spaced desert vegetation via remote sensing remains challenging due to the high reflectance value of desert soils (Huete and Tucker, 1991; Salama, 2011), which can be overcome only by time consuming and expensive methods (e.g., Al-Ali et al., 2020). Therefore, we would like to propose habitat suitability models based on environmental parameters as a tool complementary to remote sensing methods for obtaining a detailed picture of the spatial distribution of ephemeral vegetation cover. Our data help retrieving a spatially highly resolved pattern of vegetation on both sides of the Pacific coastal desert. We propose that this improved understanding of vegetation cover has dramatic ramifications for plant conservation in this floristically poorly documented and taxonomically understudied region. Moat et al. (2021) highlighted the high level of endemism for the loma formations and we propose that this is also true for the precordillera, underscoring the recent findings of Montenegro-Hoyos et al. (2022). Highly resolved habitat suitability models provide an important backdrop for evolutionary studies, including phylogeography and historical biogeography. At the same time, a comparison of our floristic analyses and the habitat suitability models clearly retrieve dramatical knowledge gaps along the coast, especially in southern Peru and the extreme north of Chile, but even more so along much of the precordillera. Habitat suitability models predict extensive vegetation cover, but we have few if any scientific collections, biodiversity or floristic plot data from most of these areas. These areas should therefore be prioritized in future floristic and biodiversity studies.
Conclusion
The data here presented clearly indicate a floristic subdivision of the coastal loma and Andean precordillera vegetation in Peru and Chile. The respective regions are defined by both divergent environmental conditions and ecogeographic barriers imposed by full desert. We found support for our hypothesis that loma and precordillera floras in southern Peru exhibit a closer connectivity than loma and precordillera floras in Chile. Environmental parameters could not explain this difference between both countries. We propose that this is the result of a narrowing of the desert core toward the north, rendering it a less effective dispersal barrier in Peru than in Chile. In addition, we provide evidence for highly divergent floras of the lomas in Peru and Chile. We can demonstrate that a floristic break along the coast is not linked to diverging environmental conditions, but is rather the result of a ecogeographic barrier imposed by an interruption of the Coastal Cordillera and a concomitant lack of condensation. Habitat suitability models of loma vegetation identify this region as unhabitable likely effecting the floristic gap observed. This is in stark contrast to the situation in the precordillera where we find a more gradual floristic change-over readily explained by a gradual shift of environmental conditions in the absence of any ecogeographical barriers. Our data can show that vegetation cover – at least ephemeral vegetation cover in the Pacific desert of South America – has a much larger spatial extent than previously documented. Despite considerable progress in the past decades, the floristic composition, diversity patterns and vegetation of the Pacific coastal desert are still only fragmentarily known and future research efforts should be dedicated to fill the extensive knowledge gaps in this region.
Data Availability Statement
Original data used for this study, additional results and further information are included in the Supplementary Material.
Author Contributions
JR, MW, and TB conceived the idea. MW coordinated the project. DM-T collected the data. JR and JM carried out the analyses. JR, FL, and DM-T did the data curation. JR, TB, JM, FL, and MW wrote the manuscript. All authors reviewed and approved the final version.
Conflict of Interest
The authors declare that the research was conducted in the absence of any commercial or financial relationships that could be construed as a potential conflict of interest.
Publisher’s Note
All claims expressed in this article are solely those of the authors and do not necessarily represent those of their affiliated organizations, or those of the publisher, the editors and the reviewers. Any product that may be evaluated in this article, or claim that may be made by its manufacturer, is not guaranteed or endorsed by the publisher.
Acknowledgments
Botanical collections formed part of the permits: N°056–2016-SERFOR/DGGSPFFS, N°045–2017-SERFOR/DGGSPFFS, and N°133-2018-MINAGRI-SERFOR/DGGSPFFS issued by the Servicio Nacional Forestal y de Fauna Silvestre (SERFOR, Ministerio de Agricultura y Riego), Peru.
Supplementary Material
The Supplementary Material for this article can be found online at: https://www.frontiersin.org/articles/10.3389/fevo.2022.862846/full#supplementary-material
Supplementary Appendix 1 | Detailed information on each location (source of data, altitudinal range, sampling period). Note that information for most of the locations is incomplete. Empty cells indicate that no information was available.
Supplementary Appendix 2 | Working table including presence/absence data of 870 species for 53 locations in northern Chile and southern Peru.
Supplementary Appendix 3 | Eigenvalues, proportion of total variance and cumulative proportion of total variance for each LBV derived from Principal Component Analysis of 18 Bioclimatic variables (variable 15 was excluded due to missing data) for the Atacama Desert and Peruvian Desert. Note that LBV numbers do not relate to the original Bioclimatic variables.
Supplementary Appendix 4 | Coefficients (Loadings) of the first five LBVs derived from Principal Component Analysis of 18 Bioclimatic variables (variable 15 was excluded due to missing data) for the Atacama Desert and Peruvian Desert. Values above 0.24 (or below –0.24) indicate loadings higher than assumed for equal contribution of variables. Positive loadings indicate positive correlation, negative loadings indict negative correlation.
Supplementary Appendix 5 | Diagnostic species for each cluster based on phi-fidelity index (confidence level p < = 0.05).
Supplementary Appendix 6 | Graphs used for model evaluation. Y-axis, maximum average validation AUC; X-axis, Mean Absolute Error calculated for each model compared to the extent of the desert core. 48 models under evaluation were plotted. Labels indicate regularization multiplier (rm) and feature classes (fc) used for the respective model.
Supplementary Appendix 7 | Values of AUC MAE calculated for 48 models under evaluation for each vegetation type. AUC, maximum average validation AUC; MAE, Mean Absolute Error calculated for each model compared to the extent of the desert core; Rm, regularization multiplier. Fc, feature classes.
Supplementary Appendix 8 | (A) Relative contribution (%) of environmental variables to the Maxent models. (B) Relative permutation importance of environmental variables (%) estimated on randomly permuted values of each variable on training presence and background data.
Footnotes
- ^ https://collections-botany.fieldmuseum.org
- ^ chelsa-climate.org
- ^ https://chelsa-climate.org/bioclim/
- ^ www.earthenv.org//cloud
- ^ https://rpubs.com/JRuhm/twosidessamedesert2022
References
Al-Ali, Z. M., Abdullah, M. M., Asadalla, N. B., and Gholoum, M. (2020). A comparative study of remote sensing classification methods for monitoring and assessing desert vegetation using a UAV-based multispectral sensor. Environ. Monit. Assess. 192:389. doi: 10.1007/s10661-020-08330-1
Barbet-Massin, M., Jiguet, F., Albert, C. H., and Thuiller, W. (2012). Selecting pseudo-absences for species distribution models: how, where and how many? Methods Ecol. Evol. 3, 327–338. doi: 10.1111/j.2041-210X.2011.00172.x
Bittinger, K. (2020). Usedist: Distance Matrix Utilities. R Package Version 0.4.0. Available online at: https://cran.r-project.org/web/packages/usedist/index.html.
Böhnert, T., Luebert, F., Merklinger, F. F., Harpke, D., Stoll, A., Schneider, J. V., et al. (2022). Plant migration under long-lasting hyperaridity – phylogenomics unravels recent biogeographic history in one of the oldest deserts on Earth. New Phytol. 234, 1863–1875. doi: 10.1111/nph.18082
Brako, L., and Zarucchi, J. L. (1993). “Catalogue of the flowering plants and gymnosperms of Peru,” in Monographs in Systematic Botany from the Missouri Botanical Garden, ed. L Bohs Vol. 45. (St. Louis, Mo: Missouri Botanical Garden).
Cáceres de Baldárrago, F., Raimondo, F. M., Poma, I., and Mazzola, P. (2019). Taxonomic studies on the genus Islaya (Cactaceae): Islaya camanaensis a new endemic species from Arequipa region (Peru). Quad. Bot. Ambientale Appl. 30, 33–39.
Cáceres, M., and Legendre, P. (2009). Associations between species and groups of sites: indices and statistical inference. Ecology 90, 3566–3574. doi: 10.1890/08-1823.1
Cai, W., Mcphaden, M. J., Grimm, A. M., Rodrigues, R. R., Taschetto, A. S., Garreaud, R. D., et al. (2020). Climate impacts of the El Niño–Southern oscillation on South America. Nat. Rev. Earth Environ. 1, 215–231. doi: 10.1038/s43017-020-0040-3
Chávez, R. O., Moreira-Muñoz, A., Galleguillos, M., Olea, M., Aguayo, J., Latín, A., et al. (2019). GIMMS NDVI time series reveal the extent, duration, and intensity of “blooming desert” events in the hyper-arid Atacama Desert, northern Chile. Int. J. Appl. Earth Obs. Geoinf. 76, 193–203. doi: 10.1016/j.jag.2018.11.013
Danielson, J. J., and Gesch, D. B. (2011). Global Multi-Resolution Terrain Elevation Data 2010 (GMTED2010). Open-File Report 2011–1073, Reston, VA: United States Geological Survey 1–26. doi: 10.3133/ofr20111073.
Díaz, F. P., Latorre, C., Carrasco-Puga, G., Wood, J. R., Wilmshurst, J. M., Soto, D. C., et al. (2019). Multiscale climate change impacts on plant diversity in the Atacama Desert. Glob. Change Biol. 25, 1733–1745. doi: 10.1111/gcb.14583
Dillon, M. O. (2005). “The solanaceae of the lomas formations of coastal Peru and Chile,” in A Festschrift for William G. D’Arcy. The legacy of a taxonomist. Unter Mitarbeit von William G. D’Arcy Monographs in Systematic Botany from the Missouri Botanical Garden, Vol. 104, eds R. C. Keating, V. C. Hollowell, and T. B. Croat (St. Louis, Mo: Missouri Botanical Garden).
Dormann, C. F., Elith, J., Bacher, S., Buchmann, C., Carl, G., Carré, G., et al. (2013). Collinearity: a review of methods to deal with it and a simulation study evaluating their performance. Ecography 36, 27–46. doi: 10.1111/j.1600-0587.2012.07348.x
Dupin, M., Reynaud, P., Jarošík, V., Baker, R., Brunel, S., Eyre, D., et al. (2011). Effects of the training dataset characteristics on the performance of nine species distribution models: application to Diabrotica virgifera virgifera. PLoS One 6:e20957. doi: 10.1371/journal.pone.0020957
Emck, P., Muñoz-Moreira, A., and Richter, M. (2006). “El clima y sus efectos en la vegetación,” in Botánica Económica de los Andes Centrales. La Paz, S, eds M. Moraes, B. Ollgaard, L. P. Kvist, F. Borchsenius, and H. Balslev 11–36. (Universidad Mayor de San Andrés, La Paz).
Escadafal, R. (2017). Remote sensing of drylands: when soils come into the picture. Ciênc. Tróp. 41, 33–50.
Ferreyra, R. (1961). Las lomas costaneras del extremo sur del Perú. Bol. Soc. Aregnt. Bot. 9, 87–120.
Galán de Mera, A., Linares Perea, E., La Campos, de Cruz, J., and Vicente Orellana, J. A. (2009). Nuevas observaciones sobre la vegetación del sur del Perú. Del Desierto Pacífico al Altiplano. Acta Bot. Malacitana 34, 107–144. doi: 10.24310/abm.v34i0.6904
Galán de Mera, A., Orellana, V. J., and García, J. L. (1997). Phytogeographical sectoring of the Peruvian coast. Glob. Ecol. Biogeogr. Lett. 6, 349–367. doi: 10.2307/2997336
Garreaud, R. D., Molina, A., and Farias, M. (2010). Andean uplift, ocean cooling and Atacama hyperaridity: a climate modeling perspective. Earth Planet. Sci. Lett. 292, 39–50. doi: 10.1016/j.epsl.2010.01.017
Gengler-Nowak, K. (2002). Reconstruction of the biogeographical history of Malesherbiaceae. Bot. Rev. 68, 171–188. doi: 10.1663/0006-8101(2002)068[0171:rotbho]2.0.co;2
Hepp, J. (2018). A new endemic species of Nolana (Solanaceae-Nolaneae) from near Iquique, Chile. Arnaldoa 25, 323–338. doi: 10.22497/arnaldoa.252.25202
Hijmans, R. J. (2021). Raster: Geographic data Analysis and Modeling. R Package Version 3.4-13. Available online at: https://cran.r-project.org/web/packages/raster/index.html
Houston, J. (2006). Variability of precipitation in the Atacama Desert: its causes and hydrological impact. Int. J. Climatol. 26, 2181–2198. doi: 10.1002/joc.1359
Huete, A. R., and Tucker, C. J. (1991). Investigation of soil influences in AVHRR red and near-infrared vegetation index imagery. Int. J. Remote Sens. 12, 1223–1242. doi: 10.1080/01431169108929723
Jackson, D. A. (1993). Stopping rules in principal components analysis: a comparison of heuristical and statistical approaches. Ecology 74, 2204–2214. doi: 10.2307/1939574
Jansen, F., and Dengler, J. (2010). Plant names in vegetation databases – a neglected source of bias. J. Veg. Sci. 21, 1179–1186. doi: 10.1111/j.1654-1103.2010.01209.x
Jiménez, R., La Rosa, R., La Torre, M. I., and Cano, A. (2008). Flora y comunidades vegetales de las lomas de San Fernando y áreas adyacentes. Bol. Soc. Geogr. Lima 117, 31–40.
Karger, D. N., Conrad, O., Böhner, J., Kawohl, T., Kreft, H., Soria-Auza, R. W., et al. (2017). Climatologies at high resolution for the earth’s land surface areas. Sci. Data 4:170122. doi: 10.1038/sdata.2017.122
Karger, D. N., Conrad, O., Böhner, J., Kawohl, T., Kreft, H., Soria-Azua, R. W., et al. (2018). Data from: climatologies at high resolution for the earth’s land surface areas. Sci. Data 4:170122.
Kass, J. M., Muscarella, R., Galante, P. J., Bohl, C. L., Pinilla-Buitrago, G. E., Boria, R. A., et al. (2021). ENMeval 2.0: Redesigned for customizable and reproducible modeling of species’ niches and distributions. Methods Ecol. Evol. 12, 1602–1608. doi: 10.1111/2041-210X.13628
Kassambara, A., and Mundt, F. (2020). Factoextra: Extract and Visualize the Results of Multivariate data Analyses. R Package Version 1.0.7. Available online at: https://CRAN.R-project.org/package=factoextra
Koepcke, H. W. (1961). Synökologische Studien an der Westseite der peruanischen Anden. Aufl–Bonner Geographische Abhandlungen 29. Bonn: Dümmler.
Konowalik, K., and Nosol, A. (2021). Evaluation metrics and validation of presence-only species distribution models based on distributional maps with varying coverage. Sci. Rep. 11:1482. doi: 10.1038/s41598-020-80062-1
León, B., Young, K. R., Cano, A., La Torre, M. I., Arakaki, M., and Roque, J. (1997). Botanical exploration and conservation in Peru: the plants of Cerro Blanco, Nazca. BioLlania 6, 431–448.
Leutner, B., Horning, N., and Schwalb-Willmann, J. (2019). RStoolbox: Tools for Remote Sensing data Analysis. R Package Version 0.2.6. Available online at: https://cran.r-project.org/web/packages/RStoolbox/index.html.
Liu, C., Newell, G., and White, M. (2016). On the selection of thresholds for predicting species occurrence with presence-only data. Ecol. Evol. 6, 337–348. doi: 10.1002/ece3.1878
Luebert, F., and Pliscoff, P. (2017). Sinopsis Bioclimática y Vegetacional de Chile. 2. Aufl. Santiago: Editorial Universitaria (Biodiversidad).
Luebert, F., and Gajardo, R. (2005). Vegetación alto andina de Parinacota (norte de Chile) y una sinopsis de la vegetación de la Puna meridional. Phytocoenologia 35, 79–128. doi: 10.1127/0340-269X/2005/0035-0079
Luebert, F., and Weigend, M. (2014). Phylogenetic insights into Andean plant diversification. Front. Ecol. Evol. 2:27. doi: 10.3389/fevo.2014.00027
Luebert, F., Wen, J., and Dillon, M. O. (2009). Systematic placement and biogeographical relationships of the monotypic genera Gypothamnium and Oxyphyllum (Asteraceae: Mutisioideae) from the Atacama Desert. Bot. J. Linn. Soc. 159, 32–51. doi: 10.1111/j.1095-8339.2008.00926.x
Manrique, R., Ricotta, C., Ferrari, C., and Pezzi, G. (2014). Latitudinal pattern in plant composition along the Peruvian and Chilean fog oases. Plant Biosyst. Int. J. Dealing Aspects Plant Biol. 148, 1002–1008. doi: 10.1080/11263504.2014.918059
Marchi, M., and Ducci, F. (2018). Some refinements on species distribution models using tree-level National Forest inventories for supporting forest management and marginal forest population detection. IForest 11, 291–299. doi: 10.3832/ifor2441-011
McKay, C. P., Friedmann, E. I., Gómez-Silva, B., Cáceres-Villanueva, L., Andersen, D. T., and Landheim, R. (2003). Temperature and moisture conditions for life in the extreme arid region of the Atacama Desert: four years of observations including the El Niño of 1997-1998. Astrobiology 3, 393–406. doi: 10.1089/153110703769016460
Merklinger, F. F., Böhnert, T., Arakaki, M., Weigend, M., Quandt, D., and Luebert, F. (2021). Quaternary diversification of a columnar cactus in the driest place on earth. Am. J. Bot. 108, 184–199. doi: 10.1002/ajb2.1608
Merow, C., Smith, M. J., and Silander, J. A. (2013). A practical guide to MaxEnt for modeling species’ distributions: what it does, and why inputs and settings matter. Ecography 36, 1058–1069. doi: 10.1111/j.1600-0587.2013.07872.x
Moat, J., Orellana-Garcia, A., Tovar, C., Arakaki, M., Arana, C., Cano, A., et al. (2021). Seeing through the clouds – mapping desert fog oasis ecosystems using 20 years of MODIS imagery over Peru and Chile. Int. J. Appl. Earth Obs. Geoinf. 103:102468. doi: 10.1016/j.jag.2021.102468
Molina, A., Falvey, M., and Rondanelli, R. (2017). A solar radiation database for Chile. Sci. Rep. 7:14823. doi: 10.1038/s41598-017-13761-x
Montenegro-Hoyos, A., Vega, N., and Linares-Palomino, R. (2022). Plant diversity and structure in desert communities of the Andean piedmont in Ica, Peru. Veg. Classif. Surv. 3, 53–66. doi: 10.3897/VCS.68006
Montesinos, D. B. (2012). Andean shrublands of Moquegua, South Peru: prepuna plant communities. Phytocoenologia 42, 29–55. doi: 10.1127/0340-269X/2012/0042-0516
Montesinos-Tubée, D. B., and Mondragón, L. P. (2013). Flora y vegetación en tres localidades de una cuenca costeña: río Acarí, provincia de Caravelí (Arequipa, Perú). Zonas Áridas 15, 11–30. doi: 10.21704/za.v15i1.106
Montesinos-Tubée, D. B., Cleef, A. M., and Sýkora, K. V. (2015). The Puna vegetation of Moquegua, South Peru: chasmophytes, grasslands and Puya raimondii stands. Phytocoenologia 45, 365–397. doi: 10.1127/phyto/2015/0006
Montesinos-Tubée, D. B., Pauca, A., and Revilla, I. (2016). Tigridia arequipensis (Iridaceae Tigridieae), a new species from South Peru. Blumea Biodivers. Evol. Biogeogr. Plants 61, 4–7. doi: 10.3767/000651916X690962
Montesinos-Tubée, D., and Chicalla-Rios, K. J. (2021). Senecio huaynaputinaensis (Compositae), una especie nueva de las faldas del volcán Huaynaputina en el departamento de Moquegua, sur de Perú. Bol. Soc. Argent. Bot. 56, 33–43. doi: 10.31055/1851.2372.v56.n1.29299
Moreno, P., Villagrán, C., Marquet, P. A., and Marshall, L. G. (1994). Quaternary paleobiogeography of northern and central Chile. Rev. Chil. Hist. Nat. 67, 487–502.
Muenchow, J., Hauenstein, S., Bräuning, A., Bäumler, R., Rodríguez, E. F., and von Wehrden, H. (2013). Soil texture and altitude, respectively, largely determine the floristic gradient of the most diverse fog oasis in the Peruvian desert. J. Trop. Ecol. 29, 427–438. doi: 10.1017/S0266467413000436
Muscarella, R., Galante, P. J., Soley-Guardia, M., Boria, R. A., Kass, J. M., Uriarte, M., et al. (2014). ENMeval: an R package for conducting spatially independent evaluations and estimating optimal model complexity for Maxent ecological niche models. Methods Ecol. Evol. 5, 1198–1205. doi: 10.1111/2041-210X.12261
Mutke, J., and Weigend, M. (2017). Mesoscale patterns of plant diversity in Andean South America based on combined checklist and GBIF data. Ber. d. Reinh Tüxen Ges 23, 83–97.
Oksanen, J., Blanchet, F. G., Kindt, R., Legendre, P., O’Hara, R. O., Simpson, G. L., et al. (2020). Vegan: Community Ecology Package. R Package Version 2.5-7. Available online at: https://CRAN.R-project.org/package=vegan.
Pauca-Tanco, G. A., Villasante Benavides, J. F., Villegas Paredes, L., Luque Fernandez, C. R., and Del Quispe Turpo, J. P. (2020). Distribution and characterization of the communities of Tillandsia (Bromeliaceae) in southern Peru and their relationship with altitude, slope and orientation. Ecosistemas 29:2035. doi: 10.7818/ECOS.2035
Phillips, S. J., Anderson, R. P., Dudík, M., Schapire, R. E., and Blair, M. E. (2017). Opening the black box: an open-source release of Maxent. Ecography 40, 887–893. doi: 10.1111/ecog.03049
Pinto, R., and Luebert, F. (2009). Datos sobre la flora vascular del desierto costero de Arica y Tarapacá, Chile, y sus relaciones fitogeográficas con el sur de Perú. Gayana Bot. 66, 28–49. doi: 10.4067/S0717-66432009000100004
Quipuscoa Silvestre, V., and Dillon, M. O. (2018). Four new endemic species of Nolana (Solanaceae-Nolaneae) from Arequipa, Peru. Arnaldoa 25, 295–322. doi: 10.22497/arnaldoa.252.25201
R Core Team (2021). R. A Language and Environment for Statistical Computing. Vienna: R Foundation for Statistical Computing.
Radosavljevic, A., and Anderson, R. P. (2014). Making better Maxent models of species distributions: complexity, overfitting and evaluation. J. Biogeogr. 41, 629–643. doi: 10.1111/jbi.12227
Rivas-Martínez, S., Rivas-Sáenz, S., and Penas, A. (2011). Worldwide bioclimatic classification system. Glob. Geobot. 1, 1–634. doi: 10.5616/gg110001
Rodwell, M. J., and Hoskins, B. J. (2001). Subtropical anticyclones and summer monsoons. J. Clim. 14, 3192–3211. doi: 10.1175/1520-04422001014<3192:SAASM<2.0.CO;2
Roque, J., and Cano, A. (1999). Flora vascular y vegetación del Valle de Ica, Perú. Rev. Peru. Biol. 6, 185–195.
Ruhm, J., Böhnert, T., Weigend, M., Merklinger, F. F., Stoll, A., Quandt, D., et al. (2020). Plant life at the dry limit-Spatial patterns of floristic diversity and composition around the hyperarid core of the Atacama Desert. PLoS One 15:e0233729. doi: 10.1371/journal.pone.0233729
Rundel, P. W., Dillon, M. O., Palma, B., Mooney, H. A., Gulmon, S. L., and Ehleringer, J. R. (1991). The phytogeography and ecology of the coastal Atacama and Peruvian deserts. J. Syst. Evol. Bot. 13, 1–49. doi: 10.5642/aliso.19911301.02
Salama, R. B. (2011). “Remote sensing of soils and plants imagery,” in Encyclopedia of Agrophysics, eds J. Gliński, J. Horabik, and J. Lipiec (Dordrecht: Springer), 681–693. doi: 10.1007/978-90-481-3585-1_132
Sarmiento, G. (1975). The dry plant formations of South America and their floristic connections. J. Biogeogr. 2, 233–251.
Schulz, N., Aceituno, P., and Richter, M. (2011). Phytogeographic divisions, climate change and plant dieback along the coastal desert of northern Chile. Erdkunde 65, 169–187. doi: 10.3112/erdkunde.2011.02.05
Schwarzer, C., Cáceres Huamaní, F., Cano, A., La Torre, M. I., and Weigend, M. (2010). 400 years for long-distance dispersal and divergence in the northern Atacama Desert – insights from the Huaynaputina pumice slopes of Moquegua, Peru. J. Arid Environ. 74, 1540–1551. doi: 10.1016/j.jaridenv.2010.05.034
Senay, S. D., and Worner, S. P. (2019). Multi-scenario species distribution modeling. Insects 10:65. doi: 10.3390/insects10030065
Shcheglovitova, M., and Anderson, R. P. (2013). Estimating optimal complexity for ecological niche models: a jackknife approach for species with small sample sizes. Ecol. Model. 269, 9–17. doi: 10.1016/j.ecolmodel.2013.08.011
Silvestre, V. Q., Pérez, C. T., Ardiles, C. F., Tanco, A. P., Vera, K. D., and Dillon, M. O. (2016). Diversidad de plantas vasculares de las Lomas de Yuta, provincia de Islay, Arequipa Perú, 2016. Arnaldoa 23, 517–546.
Toro-Núñez, O., Al-Shehbaz, I. A., and Mort, M. E. (2015). Phylogenetic study with nuclear and chloroplast data and ecological niche reveals Atacama (Brassicaceae), a new monotypic genus endemic from the Andes of the Atacama Desert, Chile. Plant Syst. Evol. 301, 1377–1396. doi: 10.1007/s00606-014-1157-y
Tovar, C., Sánchez Infantas, E., and Teixeira Roth, V. (2018). Plant community dynamics of lomas fog oasis of central Peru after the extreme precipitation caused by the 1997-98 El Niño event. PLoS One 13:e0190572. doi: 10.1371/journal.pone.0190572
Valavi, R., Guillera-Arroita, G., Lahoz-Monfort, J. J., and Elith, J. (2022). Predictive performance of presence-only species distribution models: a benchmark study with reproducible code. Ecol. Monogr. 92:e01486. doi: 10.1002/ecm.1486
Villagrán, C., Armesto, J. J., and Arroyo, M. (1981). Vegetation in a high Andean transect between Turi and Cerro León in northern Chile. Vegetatio 48, 3–16. doi: 10.1007/BF00117356
Villagrán, C., Arroyo, M., and Marticorena, C. (1983). Effects of natural desertification on the distribution of the Andean flora in Chile. Rev. Chil. Hist. Nat. 56, 137–157.
Warren, D. L., and Seifert, S. N. (2011). Ecological niche modeling in Maxent: the importance of model complexity and the performance of model selection criteria. Ecol. Appl. 21, 335–342. doi: 10.1890/10-1171.1
Weberbauer, A. (1945). El Mundo Vegetal de los Andes Peruanos. Lima: Ministerio de Agricultura, Dirección de Agricultura.
Wilson, A. M., and Jetz, W. (2016). Remotely sensed high-resolution global cloud dynamics for predicting ecosystem and biodiversity distributions. PLoS Biol. 14:e1002415. doi: 10.1371/journal.pbio.1002415
Yoo, W., Mayberry, R., Bae, S., Singh, K., Peter He, Q., and Lillard, J. W. (2014). A study of offects of multicollinearity in the multivariable analysis. Int. J. Appl. Sci. Technol. 4, 9–19.
Zuloaga, F. O., Morrone, O., and Belgrano, J. (2008). “Catálogo de las plantas vasculares del Cono Sur. (Argentina, Sur de Brasil, Chile, Paraguay y Uruguay),” in Monographs in Systematic Botany from the Missouri Botanical Garden, eds A. M Anton and F. O Zuloaga Vol. 107. (St. Louis, MO: Missouri Botanical Garden).
Keywords: Atacama Desert, floristic connectivity, habitat suitability modeling, hyperaridity, loma vegetation, Peruvian Desert, precordillera, prepuna
Citation: Ruhm J, Böhnert T, Mutke J, Luebert F, Montesinos-Tubée DB and Weigend M (2022) Two Sides of the Same Desert: Floristic Connectivity and Isolation Along the Hyperarid Coast and Precordillera in Peru and Chile. Front. Ecol. Evol. 10:862846. doi: 10.3389/fevo.2022.862846
Received: 26 January 2022; Accepted: 07 April 2022;
Published: 20 May 2022.
Edited by:
Mauro Fois, University of Cagliari, ItalyReviewed by:
Gwendolyn Peyre, University of Los Andes, ColombiaEmad Kaky, Sulaimani Polytechnic University, Iraq
Copyright © 2022 Ruhm, Böhnert, Mutke, Luebert, Montesinos-Tubée and Weigend. This is an open-access article distributed under the terms of the Creative Commons Attribution License (CC BY). The use, distribution or reproduction in other forums is permitted, provided the original author(s) and the copyright owner(s) are credited and that the original publication in this journal is cited, in accordance with accepted academic practice. No use, distribution or reproduction is permitted which does not comply with these terms.
*Correspondence: Jonathan Ruhm, anJ1aG1AdW5pLWJvbm4uZGU=