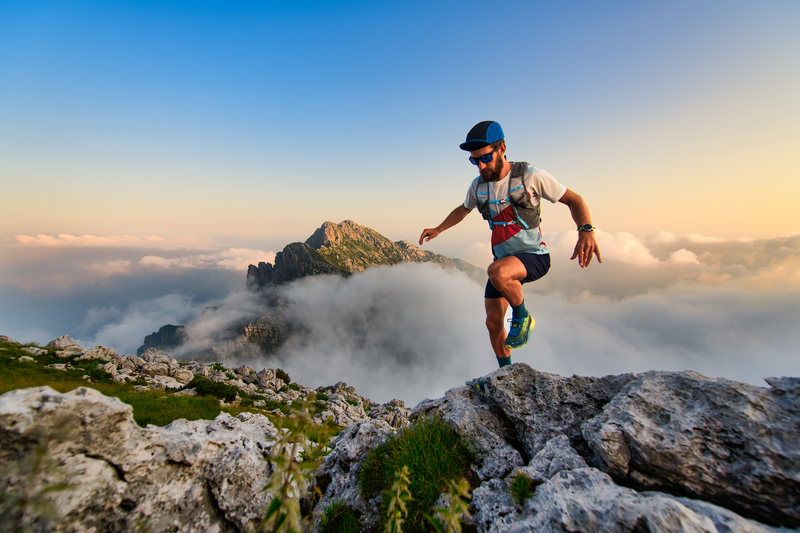
94% of researchers rate our articles as excellent or good
Learn more about the work of our research integrity team to safeguard the quality of each article we publish.
Find out more
ORIGINAL RESEARCH article
Front. Ecol. Evol. , 18 August 2022
Sec. Interdisciplinary Climate Studies
Volume 10 - 2022 | https://doi.org/10.3389/fevo.2022.860745
This article is part of the Research Topic Climate Change and Agricultural System Response View all 22 articles
Poverty caused by disasters poses a great challenge to consolidate the achievements of poverty alleviation. Livelihood resilience is the key factor for farmers to resist risks and get rid of poverty. Therefore, this study used the China Family Panel Studies (CFPS) database. Firstly, we examined the impact of natural disasters on the poverty vulnerability of farmers. Secondly, taking livelihood resilience and its decomposition dimensions as threshold variables, we examined the mechanism of livelihood resilience between natural disasters and poverty. The results show that natural disaster shocks, natural disaster intensity, and natural disaster frequency all had a significant positive effect on farm households’ vulnerability to poverty. The threshold test shows that natural disasters had larger effects on the poverty vulnerability of the farmers with lower buffer capacity, self-organizing capacity, and learning capacity. When the livelihood resilience value exceeded the third threshold, the impact of natural disasters on the poverty vulnerability of farmers turned from positive to negative. When the buffer capacity exceeded the third threshold, the impact of natural disasters on poverty vulnerability turned from positive to negative; when the self-organizing capacity exceeded the first threshold, the impact of natural disasters on poverty vulnerability turned from positive to negative; when the learning capacity exceeded the third threshold, the impact of natural disasters on poverty vulnerability turned from positive to negative. Therefore, it is suggested that appropriate policies should be needed to support farmers’ livelihood resilience and address disaster-induced poverty by improving farmers’ buffer capacity, self-organizing capacity, and learning capacity. Focusing on farmers’ livelihood resilience, government should establish a policy support system aimed at improving farmers’ buffer capacity, self-organizing capacity, and learning capacity, that will help farmers to escape from disaster-induced poverty.
Poverty caused by disasters has always been a huge challenge to consolidate poverty alleviation. Natural disasters directly affect crop production and farmers’ livelihoods, threaten farmers’ sources of income and living conditions, and easily lead non-poor farmers into poverty and those who have been lifted out of poverty back into poverty. According to the World Meteorological Organization’s (WMO) Statement on the State of the Global Climate in 2019, the decade 2010–2019 has been the hottest on record (Kappelle, 2020). Global warming has increased water circulation and caused more catastrophic climate disasters such as floods, droughts, heatwaves, tropical cyclones, and wildfires (Donat et al., 2016; Johnson et al., 2018), with tens of millions of the population to become “climate refugees.” China is a country severely affected by natural disasters. In 2020, various natural disasters in China affected 138 million people, caused 5.891 million people to evacuate, damaged 1.807 million houses, and destroyed 19.958 million hectares of crops. Currently, although China has comprehensively addressed absolute poverty, large population who have escaped from poverty still lack endogenous development incentives and sustainable livelihoods. Some studies show that 15% of people who have escaped from poverty have a high risk of returning to poverty after 3–5 years (Chen et al., 2020), and nearly 2 million people in China are at risk of returning to poverty (Li, 2021). Livelihood resilience plays an important role in helping farm households to resist risks and escape from poverty. Adequate buffer capacity, learning capacity, and self-organizing capacity provide strong support for transforming farm households’ livelihood strategies and stabilizing basic returns when coping with disasters. Therefore, in order to improve farmers’ capacity to resist disasters and consolidate the achievements of poverty alleviation, it is of great significance to explore the impact of natural disasters on farmers’ poverty vulnerability from the perspective of livelihood resilience.
Many studies have focused on disasters and farm household poverty. Hallegatte et al. (2015) argued that natural disaster shocks to agricultural production and health prevented households from escaping poverty. Yang et al. (2016) found that meteorological disasters significantly reduced farmers’ incomes in specific areas and negatively affected farmers’ agricultural and non-agricultural incomes. Walsh and Hallegatte (2019) incorporated socio-economic resilience into the risk assessment framework and found that floods would immediately lead Sri Lankans into poverty, especially for low-income groups. However, some scholars argued that disasters have a boosting effect on income. Banerjee (2007) analyzed the rise in demand for agricultural labor in Bangladesh after floods, which instead led to an increasing income of rural workers dependent on agricultural wages for their livelihoods. Gignoux and Menéndez (2016) found that individuals affected by the earthquake experienced short-term economic losses, but recovered to their original levels in the medium term (2–5 years) and even achieved long-term gains after 6–12 years.
The concept of resilience first appeared in the study of ecosystems to denote the ability to recover physical characteristics under ecological changes (Holling, 1973). Adger (2000) first introduced the concept of resilience into social science research. Then, resilience has gradually been applied into study the ability of human and social systems to recover livelihoods (Davidson, 2010). In studies of uncertainty and human wellbeing, the concept of resilience has been further expanded. Nowadays, livelihood resilience indicates an ability of a system to maintain its original characteristics after a shock (Tambo, 2016), which is widely used in economic organizational behavior, social-ecological systems and disaster management. In measurement of livelihood resilience, Speranza et al. (2014) built a framework of buffer capacity, self-organizing capacity, and learning capacity. Smith and Frankenberger (2018) classified livelihood resilience into absorptive capacity, adaptive capacity, and transformational capacity. Zhou et al. (2021a) considered livelihood resilience as an important means of addressing disaster shocks, included farmers’ disaster prevention and mitigation capacity into the research framework of livelihood resilience and measured livelihood resilience in four dimensions: buffer capacity, self-organizing capacity, learning capacity, and disaster resilience.
The impact of livelihood resilience on poverty can be discussed in terms of buffer capacity, self-organizing capacity, and learning capacity. Firstly, buffer capacity represents the degree of change or disturbance that a system can withstand to maintain its structure, function, and feedback. In the farmers’ livelihood system, farmers can use existing accessible assets to cope with shocks and capture opportunities for better livelihood outcomes. For example, Wang W. et al. (2021) argued that the initial livelihood capital endowment was critical for farm households to escape from poverty. Some studies show that physical capital such as agricultural means of production, housing and durable goods are effective in reducing poverty occurrence among farm households (Shinn and Gillespie, 1994; Amendola and Vecchi, 2014; Oni and Oyelade, 2014). Secondly, self-organizing capacity describes the impact of institutions, rights and social organizational structures on livelihood resilience. Its impact on poverty can alleviate poverty through institutional systems, social organizations and groups as well as social networks (Jung, 2007; Yan, 2018). Thirdly, learning capacity emphasizes the adaptive management of systems to transform knowledge into productivity. Farmers with higher learning capacity can better participate in the urbanization and industrialization process. Thus, they can achieve a shift in livelihood strategy to escape from poverty by working away from home or starting a business (Yang, 2012; Jia et al., 2017).
In general, a wide variety of research has been conducted on the relationship between disasters and poverty as well as the relationship between livelihood resilience and poverty. However, there are still some shortcomings in existing studies. Firstly, the definition of poverty focuses more on static absolute poverty, which can only reflect the poverty status of rural households in the current period instead of dynamical future trends of poverty (Chaudhuri et al., 2002; Ligon and Schechter, 2003). The poverty vulnerability index measures the likelihood of falling into poverty for rural households in future periods, which is forward-looking for measuring the impact of disaster shocks on farm households’ poverty. Secondly, the impact of natural disasters on farm household poverty has been rarely studied from the perspective of livelihood resilience. Thus, based on the definition of poverty vulnerability and livelihood resilience, livelihood resilience was divided into buffer capacity, self-organizing capacity, and learning capacity in this paper. Furthermore, the threshold role of the three capacities between disaster and poverty was explored. This will provide a theoretical implication and decision-making insight for reducing disaster poverty risk and improving the livelihood sustainability of farm households.
Disasters directly cause poverty, mainly by disaster-causing factors, disaster risks and bearers. Poverty caused by disaster-causing factors and disaster risks involves direct links between disaster and poverty. Poverty caused by disaster factors is manifested as economic losses and casualties of farmers due to natural disasters. Such impacts hit farmers’ income and consumption in the short term, causing them to be vulnerable to poverty. Poverty by disaster risks involves the hidden poverty driven by different disasters. There are differences in the impact of low-frequency and high-loss intensive disasters and high-frequency and low-loss widespread disasters on poverty of farmers. The former type mainly causes economic losses and deaths, while the latter has more cumulative indirect impacts. For example, frequent rainstorms and droughts are major causes of the continuous reduction of farmers’ agricultural income and precarious livelihoods.
The impoverishment of bearing bodies is manifested in that different hazard bearing bodies in the same intensity of disasters magnify the losses of disasters due to higher cost value and lower livelihood resilience in the face of disasters. That is, the difference in the livelihood resilience of the carrier may lead to changes in the direction or intensity of the effect of disasters on poverty (Wilhite et al., 2007). Livelihood resilience can be further divided into buffer capacity, self-organizing capacity, and learning capacity according to its roles and functions in anti-poverty. Firstly, as the households’ ability to resist risks, buffer capacity is responsible for smoothing households’ production and consumption, by which the household’s primary welfare is maintained. Low buffer capacity will exacerbate the risk of disaster shocks and prevent farmers from adjusting production and livelihoods in time to adapt to environmental changes. Consequently, farmers will fall into poverty trap. In contrast, for those with sufficient buffer capacity, this short-term impact is too limited to change their livelihood sources. Secondly, self-organizing capacity represents the farmers’ ability to integrate into the local economy, society and institutional environment. When farmers are affected by disasters, components including environmental and institutional factors are critical to helping farmers effectively get rid of the predicament. For example, factors such as convenient transportation and organizational participation are conducive to farmers adopting non-agricultural employment to respond to disaster shocks and mitigate the negative impact of disasters. Thus, these will help farmers get rid of poverty. Farmers with low self-organizing capacity cannot integrate into neighboring systems and organizations when dealing with disasters. It is difficult to avoid disaster risks and thus farmers may fall into poverty. Thirdly, learning capacity represents the farmers’ ability to adapt to disturbances. When the livelihood development of farmers is exposed to short-term disaster shocks, it can help farmers cope with the impact of short-term disasters. However, with the increase of external disturbances and their duration, farmers need to constantly adapt to external disturbances while improving their abilities. In the changing environment, farmers with strong learning capacity can change their livelihood strategies in real time and take adaptive measures such as labor transfer and non-agricultural employment to alleviate the sharp fall in income caused by disaster shocks. Farmers with weak learning ability are unable to cope with disaster shocks by changing their livelihood strategies and can only engage in agricultural production, which aggravates their poverty vulnerability.
Hypothesis 1: Shocks, intensity and frequency of natural disasters lead to poverty vulnerability of farm households.
Hypothesis 2: Natural disaster shocks have non-linear effects on the poverty vulnerability of farm households with a threshold of livelihood resilience.
The adjustment to poverty involves eradicating specific poverty and eliminating sudden poverty vulnerability. Silber and Wan (2016) argued that it is necessary to adjust poverty thresholds according to vulnerability, and the adjusted poverty line can represent the minimum living standard in a vulnerable environment. There are three definitions of poverty vulnerability measures, i.e., poverty vulnerability (VEP), low expected utility vulnerability (VEU), and risk exposure vulnerability (VER). Most scholars adopt the VEP approach (Peng J. et al., 2019; Yang et al., 2021). The main reason is that with α = 0, VEP can be simplified as the probability that consumption is lower than poverty. Therefore, the VEP measure is used in this paper to measure the poverty vulnerability of farm households:
where Vit represents the poverty vulnerability of the farm household i in period t. It refers to the probability that the farm household’s future net consumption (Yi, t+1) per capita is below the poverty line (pl). In the empirical study, the specific calculation method of Vit refers to the three-stage feasible generalized least squares (FGLS) used by Chaudhuri et al. (2002). The regression process is mainly divided into three steps:
Step 1: the model of consumption average and consumption fluctuation is established. The consumption average model is estimated with the OLS method. The square of the residual obtained after regression is used to represent the consumption fluctuation. Then, the OLS method is used to regress the consumption variance model to obtain the heteroscedasticity structure:
where Yi,t denotes the consumption of the household i in period t; Xi,t denotes the household’s observable characteristic variables, including individual characteristic variables such as age, gender, and education of the household head and characteristic variables such as the number of household laborers and health status; βi denotes the vector of household’s characteristic coefficients; μi denotes the disturbance term.
Step 2: by using the heteroscedasticity structure calculated in the first step as the weight, weighted regression (WLS) was performed on the consumption mean and consumption variance model to obtain the required parameter estimates. The future consumption level and its variance according to the parameter estimates can be predicted:
Step 3: The poverty line is selected when the logarithmic variance of consumption and logarithmic expectation of consumption are known, and the vulnerability of households to poverty in the current period is calculated according to the normal distribution function, which can be expressed as:
A household i is vulnerable to poverty if the probability that the household will fall into poverty in the future is larger than the poverty vulnerability threshold. The international poverty criteria adopted by the World Bank for low- and middle-income countries are US$1.9 and US$3.2 per capita per day. Considering that the actual currency purchasing power varies across countries, this paper selected US$3.2 per capita per day as the poverty standard line according to Wang J. et al. (2021) and used the annual purchasing power parity (PPP) index to convert the US dollar indicator into the corresponding RMB measure.1 Poverty vulnerability is usually set in three forms, i.e., poverty incidence (Peng J. et al., 2019), 50% probability value (Sheng and Guo, 2018), and 29% probability value (Günther and Harttgen, 2009). Since using 50% as the vulnerability line will cause the omission of temporarily poor families, in recent years, some scholars began to use the 29% as the vulnerability line, i.e., a household is considered poor and vulnerable if its probability of falling into or remaining poverty in the future is not less than 29%. Therefore, the 29% probability value was selected as the poverty vulnerability line criterion in this paper.
Three core explanatory variables were designed in this paper. (1) Natural disaster shocks. According to the China Family Panel Studies (CFPS) community questionnaire (“During the period from January 1, 2010 to December 31, 2013, has your village suffered from the following natural disasters?”) including nine types of natural disasters in detail such as droughts, floods, and typhoons, if the village suffers a natural disaster, the value of these variables is taken as 1; otherwise, the value is taken as 0. (2) Natural disaster shock intensity. According to the number of types of natural disasters occurring in the area, the natural disaster impact intensity variable was constructed and set as the integer “1–9.” (3) Frequent disasters. According to questionnaire (“Is the area a natural disaster-prone area?”), If the answer is “yes,” the village is considered to be affected by a long-term natural disaster and the value is 1; otherwise, the value is 0.
Referring to the livelihood resilience measurement framework proposed by Speranza et al. (2014), relevant literature and data (Cao et al., 2016; Gerlitz et al., 2017; Peng L. et al., 2019; Sina et al., 2019; Zhou et al., 2021a) were combined to construct a three-dimensional—livelihood resilience evaluation system of buffer capacity, self-organizing capacity, and learning capacity. Buffer capacity refers to the farmers’ ability to use available assets when coping with shocks. In combination with the sustainable livelihood analysis framework, the indicators reflecting the buffer capacity of farmers were selected, including natural capital (Arable land area), financial capital (per capita income), human capital (dependency ratio and amount of labor force), material capital (housing capital, durable goods value, and production capital), and social capital (social spending). Self-organizing capacity reflects the impact of system, rights and social organization structures on livelihood resilience. The variables including traffic accessibility (Distance to the nearest township and county), social organization participation and economic assistance were selected. Learning capacity emphasizes the adaptive management ability of the system, which refers to the ability of farmers to transform knowledge into productivity. This paper selects variables such as years of education of the head of household, the income of immigrants, the degree of participation in training, and the use of Internet for learning.
Proposed by Wu et al. (2019), the entropy weighting method was used to measure the weights. As an objective assignment method, the entropy method can reduce the interference of artificial elements on the evaluation results and yield more scientific evaluation results (Xu et al., 2018, 2019; Guo et al., 2019; Zhou et al., 2021b). Specific indicators and weights are shown in Table 1.
In addition to disaster shocks, the poverty vulnerability of rural households is also affected by many other factors. In order to ensure the scientific validity and integrity of the model, variables such as household head characteristics, family characteristics and external support were included based on previous research and data availability. Household head characteristics included age, gender (1 = male; 0 = female) and marital status (1 = married; 0 = otherwise). Family characteristics include the proportion of unhealthy people, social services (logarithm of agricultural machinery rental), whether the land was transferred out (1 = land was transferred out; 0 = land was not transferred out) and government subsidies were selected for external support (1 = received government subsidies; 0 = did not receive government subsidies) and social donations (1 = received social donations; 0 = did not receive social donations). In addition, whether the included area was a mine was used as a regional control variable. Table 2 presents the descriptive statistics of the main variables.
The master model is specified as:
where Y represents the poverty vulnerability; X indicates natural disaster impact, natural disaster intensity and natural disaster frequency; β0 is a constant; ε is the error term; β1 and β2 are coefficients to be determined.
When examining the factors that cause heterogeneous effects of explanatory variables on the explained variables, grouping tests or interaction terms are commonly used, but it is difficult to determine the grouping criteria for grouping tests (Xu et al., 2021). Therefore, within the traditional linear model framework, it is impossible to clarify the differential relationship between natural disaster shocks and farmers’ poverty vulnerability at different livelihood resilience levels. In order to overcome the limitations of current research methods, Hansen (1999) established a grouping regression model on the basis of grouping approach in threshold regression:
where qi represents livelihood resilience and its decomposition term, γ represents the critical point of the threshold variable. According to n critical values, the total sample can be divided into n + 1 sample intervals, θ1 and θ2 represents the estimation coefficients of different sample intervals, respectively.
This study used data from the CFPS, a large-scale micro-household survey implemented by the China Social Science Survey Center of Peking University. CFPS is nationally representative and aims to reflect the changes in China from the perspective of society, economy, population, education, and health. It tracks and collects data from individuals, households and communities, covering household microdata from most provinces (municipalities and autonomous regions) across China.
Table 3 shows the regression results of the effects of natural disasters on poverty vulnerability of farm households. Models (1), (2), and (3) are the effects of natural disaster shocks, natural disaster frequency, and natural disaster intensity on the poverty vulnerability of farm households, respectively. Column (1) shows that natural disaster shocks had a positive effect on the poverty vulnerability of farm households and were highly significant at the 5% level; Columns (2) and (3) show that natural disaster frequency and intensity both had a significant positive effect on the poverty vulnerability of farm households and was highly significant at the 10% level, respectively. Thus, Hypothesis 1 was verified. The reason is that intensive disasters (e.g., catastrophes) directly hit farm households and lead to death and asset loss, which in turn leads to poverty vulnerability. The risk of extensive disasters (e.g., recurrent floods and agricultural droughts) is responsible for asset damage and economic losses, and their continuous cumulative indirect effects (e.g., welfare damage, health deterioration, and income decline) contribute to chronic and deep poverty.
Among the control variables, age, marriage, and land transfer had significant negative effects on poverty vulnerability, while gender, the square term of age, social donations, government subsidies, the proportion of unhealthy people, and being in a mining area all have significant positive impacts on the poverty vulnerability of farmers. It can be seen that there was an inverted U-shaped relationship between age and poverty vulnerability. When the household head was in their young and middle age, the farming household had stronger workability and learning capacity, which is more advantageous in making a living and earning income. When the age exceeded the threshold point, problems such as deteriorating health, work difficulty and mobility constraints restrict the income growth and consumption desire of the farming household. Married households can effectively alleviate poverty vulnerability since the income growth brought by marriage facilitates the escape from poverty. Farmers can reasonably allocate labor resources to achieve diversified income growth by farming and working away from home. The increase in the proportion of unhealthy families not only reduces the labor force, but also increases the burden on the existing labor force, thus increasing the possibility of farmers’ poverty vulnerability. The change in livelihood strategy caused by the land transfer effectively contributes to income growth and poverty alleviation. Both social donation and government subsidies have a positive effect on poverty vulnerability, because short-term support policies can help farmers overcome their difficulties, while they may also lead to the attitude of “waiting for, relying on and requesting” aid. This makes it difficult for farmers to escape from poverty in the long term, thus increasing their poverty vulnerability. Farmers in mining areas are more likely to fall into poverty vulnerability. The possible reason may be that the “resource dependence trap” leads to a lack of human capital investment and technological progress for farmers, and thus they fall into poverty.
Endogeneity problems may exist as survey data were susceptible to respondent bias and recall during collection, and there may also be omitted variables that affect livelihood resilience. In this paper, the instrumental variable measurement by Dell et al. (2014) was used and the disaster incidence in other regions in the same province was selected as the instrumental variable of the IV model to estimate the disaster impact. The natural disaster occurrence recorded in the CFPS 2014 questionnaire spanned from 2010 to 2013, and the probability of natural disaster occurrence in districts and counties within the same province was similar over a longer period, which can reflect the natural disaster occurrence in the village. In addition, the natural disaster occurrence in areas except for one specific village was not directly related to the relative poverty level of local farm households. Therefore, the condition of exogeneity is met.
From the 2SLS estimation results in Table 4, the results of the IV under-identification test indicate that the LM statistic (p-value) of the model significantly rejected the original hypothesis, indicating that the model did not show under-identification of instrumental variables. The Cragg-Donald Wald F-statistic was significantly larger than the threshold value of StockYogo weak instrumental variables, indicating that the model did not have the problem of weak instrumental variables. The first-stage regression results present a positive relationship between the occurrence of natural disasters in the neighboring areas and the occurrence of natural disasters in the area. The coefficient from the second-stage regression was 0.2507 and reached statistical significance at the 1% level, which was a significant increase compared to the coefficient in baseline regression. This shows that ignoring the endogeneity problem will lead to the underestimation of the estimated coefficients.
According to the principle of the threshold effect model, the F statistic and the corresponding p-values at 1%, 5%, and 10% significance levels were compared, as shown in Table 5. Under livelihood resilience, all triple threshold estimates (0.263, 0.329, and 0.371) passed the test at the 1% significance level, with significant differences between these three values. Similarly, the estimates of buffer capacity all passed the test at the 1% significance level, and the three thresholds were 0.021, 0.046, and 0.095, respectively. The double threshold of self-organizing capacity passed the test at the 1% significance level, while the triple threshold did not. Thus, there was a double threshold estimate of self-organizing capacity, and the actual values corresponding to the double threshold were 0.189 and 0.190. The estimated values of learning capacity all passed the test at a 1% significance level, and the three thresholds were 0.031, 0.078, and 0.108, respectively (see Table 6 for details).
From Table 7 we can see that the extent of the impact of natural disaster shocks on farm household poverty vulnerability varied with different livelihood resilience. When the livelihood resilience was below the first threshold, the impact of natural disaster shocks on the poverty vulnerability of farm households was highly significant at the 1% level, with an estimated coefficient of 0.1110; when the livelihood resilience was between the first and second thresholds, the impact was highly significant at the 1% level, with an estimated coefficient of 0.0547; when the livelihood resilience was between the second and third thresholds, the impact of natural disaster shocks on the poverty vulnerability of farmers was not significant; when the livelihood resilience was larger than the third threshold, the impact was highly significant at the 1% level, with an estimated coefficient of −0.1961, and the sign of the impact coefficient changed from positive to negative. In general, it shows that when the livelihood resilience of farmers was at a low level, the natural disaster shock positively affected the poverty vulnerability of farmers; when the livelihood resilience of farmers exceeded 0.371, the natural disaster shock alleviated the poverty vulnerability of farmers instead. Based on our hypotheses, there are two main reasons. Firstly, farmers with high livelihood resilience tend to adopt livelihood transitions in response to reduced agricultural production and income caused by disasters. Secondly, for farmers with high mechanization and disaster resilience, the imbalance between supply and demand of agricultural products after disasters is instead beneficial to the income growth of such farmers.
When the buffer level was below the first threshold, the impact of natural disaster shocks on farm household poverty vulnerability was highly significant at the 1% level, with an estimated coefficient of 0.4291; when the buffer capacity was between the first and second thresholds, the impact was highly significant at the 1% level, with an estimated coefficient of 0.1572; when the buffer capacity was between the second threshold and the third threshold, the impact of natural disaster shocks on the poverty vulnerability of farmers was highly significant at the 1% level, with an estimated coefficient of 0.0654; when the buffer capacity was greater than the third threshold, the impact of natural disaster shocks on the poverty vulnerability of farmers was highly significant at the 10% level, with an estimated coefficient of −0.0381. Overall, when the buffer capacity exceeded the third threshold (0.095), it can effectively alleviate the positive impact of natural disasters on farmers’ poverty. The reason is that buffer capacity can help to withstand disaster shocks and reduce disaster losses. In addition, farmers with higher human and social capital can change their livelihood strategies more rapidly in the face of disaster shocks, thus reducing disaster risks.
When the self-organizing capacity was below the first threshold, the impact of natural disaster shocks on the poverty vulnerability of farm households was highly significant at the 1% level with an estimated coefficient of 0.0652; when the self-organizing capacity was between the first and second thresholds, the impact was highly significant at the 1% level with an estimated coefficient of −0.2412; when the self-organizing capacity was larger than the third threshold, the impact was insignificant. Overall, when the self-organizing capacity reached the threshold value (0.189), the impact of natural disaster shocks on the farmers’ poverty vulnerability turned from negative to positive. Stronger self-organizing capacity of farmers represents a more stable relationship network and higher social quality, which enables farmers to exchange materials, capabilities and information with various subjects of the network in the face of disaster impacts, thus reducing the negative impact of disaster impact.
When learning capacity was below the first threshold, the impact of natural disaster shocks on farmers’ poverty vulnerability was highly significant at the 1% level, with an estimated coefficient of 0.1632; when learning capacity was between the first and second thresholds, the impact was highly significant at the 1% level, with an estimated coefficient of 0.0675; when the learning capacity was between the second threshold and the third threshold, the impact of natural disaster on farmers’ poverty vulnerability was not significant; when the learning capacity was greater than the third threshold, the impact of natural disaster impact on farmers’ poverty vulnerability was highly significant at the level of 1%, and the estimated coefficient was 0.1312. In conclusion, when the learning capacity exceeded the threshold of 0.108, it can effectively alleviate the negative impact of natural disasters on farmers. A stronger learning capacity indicates the stronger transformation ability of farmers’ livelihood. When dealing with the disaster impact, farmers with a strong learning capacity can immediately change their livelihood strategies and transfer resources to production departments with higher utilization efficiency, thus reducing the negative impact of natural disasters.
Based on data from China Family Panel Survey, the impact of natural disasters on poverty vulnerability was first analyzed. It is found that natural disaster shocks, intensity, and frequency all exhibited significant positive effects on farm household poverty vulnerability. Secondly, there was a threshold effect of livelihood resilience in the impact of natural disasters on farm households’ poverty vulnerability, and the impact turned from positive to negative when livelihood resilience exceeded the third threshold. Finally, as is shown in the threshold effect of buffer capacity, self-organizing capacity, and learning capacity, when the buffer capacity exceeded the third threshold, the impact of natural disasters on poverty vulnerability changed from positive to negative; when the self-organizing capacity exceeded the first threshold, the impact of natural disasters on poverty vulnerability changed from positive to negative; when the learning capacity exceeded the third threshold, the impact of natural disasters on poverty vulnerability changed from positive to negative.
Based on the above research conclusions, implications can be drawn as follows: firstly, to improve the rural natural disaster prevention and control system, local governments should prepare and implement plans to prevent, mitigate and relieve disasters in villages. It is recommended to combine technologies such as the 5G network and socialized services to improve the support system of science and technology, especially early warning capabilities. The second is to improve farmers’ buffer capacity and the government’s assistance system for low-income farmers and to cultivate the livelihood capacity of such farmers in a targeted manner, such as promoting the transformation of small-scale farmers into family farms and assisting farmers to engage in non-agricultural employment, so as to improve their buffer capacity. The third is to improve the farmers’ self-organizing capacity by giving full play to the main role of farmers, organizing farmers according to the voluntary principles based on organizations such as farmers’ cooperatives, village collectives, village committees, and other organizations, and guiding scattered farmers to the rural community. Simultaneously, improvement of infrastructure construction is necessary to reduce inter-regional mobility costs and transform farmers’ livelihood strategies. The fourth is to improve farmers’ learning capacity, focus on cultivating the independent learning capacity of farmers and promote small-scale farmers to actively learn various knowledge of agricultural production and operational activities out of their subjective and objective needs. These will help to develop their cognitive structure system for the promotion of agricultural science and technology and production informatization. In addition, it is necessary to enhance the ability of small-scale farmers to practice and learn and strive to improve their ability to recognize, analyze, and solve problems, so as to help solve various problems in agricultural production and operation.
Publicly available datasets were analyzed in this study. This data can be found here: https://opendata.pku.edu.cn/dataverse/CFPS?q=&types=files&sort=dateSort&order==desc&page=1.
JZ and HL: conceptualization, formal analysis, and investigation. HL: methodology and software. HL, HO, YL, and XL: validation. JZ: resources, writing—original draft preparation, review and editing, and project funding acquisition. HO, YL, and XL: data curation. JZ: supervision. All authors read and agreed to the published version of the manuscript.
This study was supported by the 2021 Discipline Construction Coordination Project of Zhongnan University of Economics and Law, “A Study on the Effective Connection between Consolidating and Expanding Poverty Alleviation Achievements and Rural Revitalization” (Project No. XKHJ202118).
The authors declare that the research was conducted in the absence of any commercial or financial relationships that could be construed as a potential conflict of interest.
All claims expressed in this article are solely those of the authors and do not necessarily represent those of their affiliated organizations, or those of the publisher, the editors and the reviewers. Any product that may be evaluated in this article, or claim that may be made by its manufacturer, is not guaranteed or endorsed by the publisher.
Adger, W. N. (2000). Social and ecological resilience: Are they related? Prog. Hum. Geogr. 24, 347–364. doi: 10.1191/030913200701540465
Amendola, N., and Vecchi, G. (2014). Durable Goods and Poverty Measurement. World Bank Policy Research Working Paper No. 7105. Washington, DC: World Bank Group.
Banerjee, L. (2007). Effect of flood on agricultural wages in Bangladesh: an empirical analysis. World Dev. 35, 1989–2009. doi: 10.1016/j.worlddev.2006.11.010
Cao, M., Xu, D., Xie, F., Liu, E., and Liu, S. (2016). The influence factors analysis of households’ poverty vulnerability in southwest ethnic areas of China based on the hierarchical linear model: a case study of Liangshan Yi autonomous prefecture. Appl. Geogr. 66, 144–152. doi: 10.1016/j.apgeog.2015.11.016
Chaudhuri, S., Jalan, J., and Suryahadi, A. (2002). Assessing Household Vulnerability to Poverty from Cross-sectional Data: A Methodology and Estimates from Indonesia. New York, NY: Department of Economics, Columbia University.
Chen, Y., Hou, S., and Fu, D. (2020). Temporal paths of rural households out of poverty and their strategic choices in China. China Populat. Sci. 01, 53–64.
Davidson, D. J. (2010). The applicability of the concept of resilience to social systems: some sources of optimism and nagging doubts. Soc. Nat. Resour. 23, 1135–1149. doi: 10.1080/08941921003652940
Dell, M., Jones, B. F., and Olken, B. A. (2014). What do we learn from the weather? The new climate-economy literature. J. Econ. Lit. 52, 740–798. doi: 10.1257/jel.52.3.740
Donat, M. G., Lowry, A. L., Alexander, L. V., O’Gorman, P. A., and Maher, N. (2016). More extreme precipitation in the world’s dry and wet regions. Nat. Clim. Change 6, 508–513. doi: 10.1038/nclimate2941
Gerlitz, J. Y., Macchi, M., Brooks, N., Pandey, R., Banerjee, S., and Jha, S. K. (2017). The multidimensional livelihood vulnerability index–an instrument to measure livelihood vulnerability to change in the Hindu Kush Himalayas. Clim. Dev. 9, 124–140. doi: 10.1080/17565529.2016.1145099
Gignoux, J., and Menéndez, M. (2016). Benefit in the wake of disaster: long-run effects of earthquakes on welfare in rural Indonesia. J. Dev. Econ. 118, 26–44. doi: 10.1016/j.jdeveco.2015.08.004
Günther, I., and Harttgen, K. (2009). Estimating households vulnerability to idiosyncratic and covariate shocks: a novel method applied in Madagascar. World Dev. 37, 1222–1234. doi: 10.1016/j.worlddev.2008.11.006
Guo, S., Lin, L., Liu, S., Wei, Y., Xu, D., Li, Q., et al. (2019). Interactions between sustainable livelihood of rural household and agricultural land transfer in the mountainous and hilly regions of Sichuan, China. Sustain. Dev. 27, 725–742. doi: 10.1002/sd.1937
Hallegatte, S., Bangalore, M., Bonzanigo, L., Fay, M., Kane, T., Narloch, U., et al. (2015). “Poverty and climate change: natural disasters, agricultural impacts and health shocks,” in Towards a Workable and Effective Climate Regime, eds B. Scott, C. Carlo, and D. M. Jaime (London: Centre for Economic Policy Research).
Hansen, B. E. (1999). Threshold effects in non-dynamic panels: estimation, testing, and inference. J. Econ. 93, 345–368. doi: 10.1016/S0304-4076(99)00025-1
Holling, C. S. (1973). Resilience and stability of ecological systems. Ann. Rev. Ecol. Syst. 4, 1–23. doi: 10.1146/annurev.es.04.110173.000245
Jia, P., Du, Y., and Wang, M. (2017). Rural labor migration and poverty reduction in China. China World Econ. 25, 45–64. doi: 10.1111/cwe.12220
Johnson, N. C., Xie, S. P., Kosaka, Y., and Li, X. (2018). Increasing occurrence of cold and warm extremes during the recent global warming slowdown. Nat. Commun. 9:1724. doi: 10.1038/s41467-018-04040-y
Jung, Y. M. (2007). “Institutional responses to poverty: korea’s evolutionary social security system,” in Proceedings of the Australian Social Policy Conference, Sydney.
Kappelle, M. (2020). WMO Statement on the State of the Global Climate in 2019. Geneva: World Meteorological Organization (WMO), doi: 10.13140/RG.2.2.13705.19046
Li, J. (2021). Building a solid line of defense for poverty alleviation. China Civil Affairs 7, 8–10.
Ligon, E., and Schechter, L. (2003). Measuring vulnerability. Econ. J. 113, 95–102. doi: 10.1111/1468-0297.00117
Oni, K. C., and Oyelade, O. A. (2014). Mechanization of cassava for value addition and wealth creation by the rural poor of Nigeria. AMA Agric. Mech. Asia Afr. Latin Am. 45, 66–78.
Peng, J., Wu, H., and Qin, X. D. (2019). Research on the impact of land transfer on poverty vulnerability of farm households. China Land Sci. 33, 67–75. doi: 10.11994/zgtdkx.20190408.084615
Peng, L., Xu, D., and Wang, X. (2019). Vulnerability of rural household livelihood to climate variability and adaptive strategies in landslide-threatened western mountainous regions of the Three Gorges Reservoir Area, China. Clim. Dev. 11, 469–484. doi: 10.1080/17565529.2018.1445613
Sheng, B., and Guo, Z. (2018). Has NPS improved the vulnerability of rural low-income households? –Based on a phase-by-phase analysis. China Rural Econ. 1, 90–107.
Shinn, M., and Gillespie, C. (1994). The roles of housing and poverty in the origins of homelessness. Am. Behav. Sci. 37, 505–521. doi: 10.1177/000276429403700404
Silber, J., and Wan, G. (eds). (2016). The Asian ‘Poverty Miracle’: Impressive Accomplishments or Incomplete Achievements?. Cheltenham: Edward Elgar Publishing.
Sina, D., Chang-Richards, A. Y., Wilkinson, S., and Potangaroa, R. (2019). A conceptual framework for measuring livelihood resilience: relocation experience from aceh, indonesia. World Dev. 117, 253–265. doi: 10.1016/j.worlddev.2019.01.003
Smith, L. C., and Frankenberger, T. R. (2018). Does resilience capacity reduce the negative impact of shocks on household food security? Evidence from the 2014 floods in Northern Bangladesh. World Dev. 102, 358–376. doi: 10.1016/j.worlddev.2017.07.003
Speranza, C. I., Wiesmann, U., and Rist, S. (2014). An indicator framework for assessing livelihood resilience in the context of social–ecological dynamics. Glob. Environ. Change 28, 109–119. doi: 10.1016/j.gloenvcha.2014.06.005
Tambo, J. A. (2016). Adaptation and resilience to climate change and variability in north-east Ghana. Int. J. Disaster Risk Reduct. 17, 85–94. doi: 10.1016/j.ijdrr.2016.04.005
Walsh, B. J., and Hallegatte, S. (2019). Socioeconomic Resilience in Sri Lanka: Natural Disaster Poverty and Wellbeing Impact Assessment. World Bank policy Research Working Paper, 9015. Washington, DC: World Bank.
Wang, J., He, B., and Bi, J. (2021). The impact of new agricultural insurance on the consumption of poor and vulnerable farmers. Consum. Econ. 37, 57–68.
Wang, W., Lan, Y., and Wang, X. (2021). Impact of livelihood capital endowment on poverty alleviation of households under rural land consolidation. Land Use Policy 109:28. doi: 10.1016/j.landusepol.2021.105608
Wilhite, D. A., Svoboda, M. D., and Hayes, M. J. (2007). Understanding the complex impacts of drought: a key to enhancing drought mitigation and preparedness. Water Resour. Manag. 21, 763–774. doi: 10.1007/s11269-006-9076-5
Wu, S., Ye, Y., Zhang, C., Suo, Y., and Wen, G. (2019). The impact of land ownership adjustment on household livelihood capital under the framework of sustainable livelihood. China Land Sci. 33, 79–88.
Xu, D., Deng, X., Guo, S., and Liu, S. (2019). Sensitivity of livelihood strategy to livelihood capital: an empirical investigation using nationally representative survey data from rural China. Soc. Indicat. Res. 144, 113–131. doi: 10.1007/s11205-018-2037-6
Xu, D., Liu, E., Wang, X., Tang, H., and Liu, S. (2018). Rural households’ livelihood capital, risk perception, and willingness to purchase earthquake disaster insurance: evidence from southwestern China. Int. J. Environ. Res. Public Health 15:1319. doi: 10.3390/ijerph15071319
Xu, L., Jin, W., and Chen, K. (2021). External constraints of breeding scale and adoption of pro-environment behavior by pig producers. Resour. Environ. Arid Reg. 35, 46–53. doi: 10.13448/j.cnki.jalre.2021.097
Yan, H. (2018). Fuel in the snowy weather or icing on the cake? exploration of social network’s value in alleviation of digital poverty. J. Lib. Sci. China 44, 17–26.
Yang, H., Chen, G., Zhuang, T., and Wang, S. (2016). The impact of meteorological disasters on poverty in special types of areas in China. Resour. Sci. 38, 676–689.
Yang, S. (2012). Poverty reduction in China: the contribution of popularizing primary education. China World Econ. 20, 105–122. doi: 10.1111/j.1749-124x.2012.01275.x
Yang, X., Guo, S., Deng, X., and Xu, D. (2021). Livelihood adaptation of rural households under livelihood stress: evidence from sichuan province, China. Agriculture 11:506. doi: 10.3390/agriculture11060506
Zhou, W., Guo, S., Deng, X., and Xu, D. (2021a). Livelihood resilience and strategies of rural residents of earthquake-threatened areas in Sichuan Province, China. Nat. Hazards 106, 255–275. doi: 10.1007/s11069-020-04460-4
Keywords: livelihood resilience, threshold effect, poverty vulnerability, natural disaster, farm household
Citation: Lu H, Zheng J, Ou H, Liu Y and Li X (2022) Impact of Natural Disaster Shocks on Farm Household Poverty Vulnerability—A Threshold Effect Based on Livelihood Resilience. Front. Ecol. Evol. 10:860745. doi: 10.3389/fevo.2022.860745
Received: 23 January 2022; Accepted: 05 May 2022;
Published: 18 August 2022.
Edited by:
Dingde Xu, Sichuan Agricultural University, ChinaReviewed by:
Rongrong Xiao, Tianjin University of Technology, ChinaCopyright © 2022 Lu, Zheng, Ou, Liu and Li. This is an open-access article distributed under the terms of the Creative Commons Attribution License (CC BY). The use, distribution or reproduction in other forums is permitted, provided the original author(s) and the copyright owner(s) are credited and that the original publication in this journal is cited, in accordance with accepted academic practice. No use, distribution or reproduction is permitted which does not comply with these terms.
*Correspondence: Jiaxi Zheng, NDcwNTA3MzU1QHFxLmNvbQ==
Disclaimer: All claims expressed in this article are solely those of the authors and do not necessarily represent those of their affiliated organizations, or those of the publisher, the editors and the reviewers. Any product that may be evaluated in this article or claim that may be made by its manufacturer is not guaranteed or endorsed by the publisher.
Research integrity at Frontiers
Learn more about the work of our research integrity team to safeguard the quality of each article we publish.