- 1Chengdu Institute of Biology, Chinese Academy of Sciences, Chengdu, China
- 2Department of Animal and Biomedical Sciences, School of Life Science, Lanzhou University, Lanzhou, China
- 3College of Life Sciences and Food Engineering, Yibin University, Yibin, China
- 4Department of Integrative Biology, University of Guelph, Guelph, ON, Canada
Upslope range shifting has been documented in diverse species in response to global warming. Plasticity, which refers to the ability of organisms to alter their phenotypes in changing environments, is crucial for the survival of those that newly migrated to a high-altitude environment. The scope and mechanisms of plasticity across biological levels, however, have rarely been examined. We used two agama lizards (genus Phrynocephalus) as model systems and a transplant experiment to comprehensively assess their plasticity on multiple organization levels. Two low-altitude (934 m) agama species, Phrynocephalus axillaris (oviparous) and P. forsythii (viviparous), were transplanted to a high-altitude site (3,400 m). After acclimation for 6 weeks in seminatural enclosures, plasticity was measured from bite force, tail display behavior, gene expression, and metabolome. Both lizards were capable of acclimating to the high-altitude environment without sacrificing their performance in bite force, but they also showed high plasticity in tail display behavior by either decreasing the intensity of a specific display component (P. forsythii) or by the trade-off between display components (P. axillaris). Genes and metabolites associated with lipids, especially fatty acid metabolism, exhibited significant differentiation in expression, compared to individuals from their native habitats. Improved fatty acid storage and metabolism appeared to be a common response among animals at high altitudes. Despite distinct reproductive modes that may differ in response to physiological pressure, the two lizards demonstrated high concordance in plasticity when they faced a novel environment at high altitudes. Taken together, lizards likely acclimate to high-altitude environments by reducing behavioral activity and increasing energy efficiency after range shifting. Our results provide new insights into our understanding of phenotypic plasticity and its importance in today’s changing climate.
Introduction
Upslope range shifting has been documented in diverse species as a response to global warming because cooler regions likely relieve species from overheating and facilitate their survival (Thomas and Lennon, 1999; Parmesan and Yohe, 2003; Root et al., 2003; Thomas et al., 2006). However, moving up along an elevational gradient comes with several other inevitable environmental stressors, including hypobaric hypoxia and intense UV radiation, which may reduce the successful reproduction and individual survival (Scheinfeldt and Tishkoff, 2010; Kouyoumdjian et al., 2019). Endemic high-altitude species have evolved several specific mechanisms that enable them to tolerate these stressors, such as improved O2 uptake and modified metabolism (Qu et al., 2013; Zhang et al., 2014; Li et al., 2018). How newly range shifted species survive in the high-altitude environments remains to be explored.
Phenotypic plasticity plays a crucial role in facilitating organisms to live at high altitudes (Wilson and Franklin, 2002; Seebacher, 2005; Scoville and Pfrender, 2010; Corl et al., 2018). Plasticity in metabolism is particularly important (Seebacher, 2005; Horscroft et al., 2017). Abundant evidence has demonstrated that the oxygen transport system is highly plastic in dealing with severe hypoxia (Storz, 2007; He et al., 2013; Lui et al., 2015), while plasticity in metabolic activities also assists animals in coping with cold and hypoxia (Hammond et al., 2001; Seebacher, 2005). The Australian freshwater turtle Chelodina longicollis, for example, responds to cold stress by increasing the activity of regulatory enzymes (Seebacher et al., 2004). Correspondingly, gene expression, which connects genotype to metabolism, is highly plastic and also shows large differences between individuals living at different altitudes. For example, deer mice from high altitudes show a large-scale upregulation of genes associated with oxygen and metabolic fuel utilization, compared to low elevation individuals (Cheviron et al., 2014; Scott et al., 2015). Plasticity in animal behavior and performance is well known for buffering environmental changes along altitude gradients, especially for ectothermic species (Kearney et al., 2009; Enriquez-Urzelai et al., 2019). In a new environment, individuals often seek proper retreat sites to avoid the overheating risk (Enriquez-Urzelai et al., 2019) or immediately adjust their behavior patterns to match the local environments (Refsnider et al., 2018).
Despite these advancements, a holistic look at how a newly arrived animal may survive in a natural high-altitude environment is lacking. Previous studies were often carried out in laboratory settings with only one stressor considered, typically temperature or oxygen (Seebacher et al., 2004; Seebacher, 2005), but natural high-altitude environments are complex with multiple stressors. Therefore, animal transplant experiments from low- to high-altitude environments with a natural setting are highly desirable. Furthermore, plastic changes in response to high altitudes likely take place at multiple organization levels, including gene expression, metabolism, and performance. Plasticity in metabolic functions is likely essential for organisms to survive at high altitudes. Different pathways, such as oxidative phosphorylation and tricarboxylic acid cycle, have different capacities in heat production and oxygen utility (Voet and Voet, 1995; Cheviron et al., 2012). Several studies have also shown that the fatty acid metabolic pathway is crucial in dealing with high-elevation environments (Cheviron et al., 2012; Qu et al., 2013; Tang et al., 2013; Lui et al., 2015). Other levels of phenotypic variations, such as morphology and behavior, may also contribute to or depend on metabolic functions.
Toad-headed agama lizards (genus Phrynocephalus) provide an excellent system to investigate the plasticity in response to high-altitude environments. As ectothermic species, agama lizards are sensitive to environmental changes. This genus is widely distributed in the Eurasian Arid Belt. Recent studies have shown that they have evolved a series of physiological and behavioral traits that are related to high-altitude habitats (Tang et al., 2013; Yang et al., 2014). In addition, these lizards use tail displays in their social communications, which are regulated by anaerobic metabolism (Zhu et al., 2021; Hu et al., 2022). Also, both oviparity (laying eggs) and viviparity (giving live birth) exist in this group of lizards, and viviparity has often been associated with high-altitude or high-latitude environments (Guo and Wang, 2007). The different reproductive modes may restrict or expand their plasticity in a new high-altitude environment. In this group of lizards, one oviparous species (Phrynocephalus axillaris) and one viviparous species (P. forsythii) are sympatric at low-altitude habitat, and P. forsythii has been assessed to face high extinction risk under global warming (Sinervo et al., 2018). Therefore, a comparison between P. axillaris and P. forsythii would provide an opportunity to reveal both common and specific patterns in response to high-altitude environments for agama lizards.
In this study, we aimed to make a multilevel assessment of the plasticity in response to high-altitude environments for agama lizards. Specifically, we examined (1) whether agama lizards were capable of acclimation from low- to high altitudes and (2) the patterns of plasticity on performance, behavior, gene expression, and metabolome. To achieve these objectives, we transplanted two sympatric agama species P. axillaris (oviparous) and P. forsythii (viviparous), from their original low-altitude habitat to high-altitude environments in the Qinghai-Tibetan Plateau. Their bite force was measured to assess their performance, and their gene expression profiles from heart, liver, and muscle, as well as blood metabolomes, were obtained to examine their patterns of plasticity.
Materials and Methods
Experimental Design
We used a transplanting design to simulate lizards’ upslope range shifting. Two Phrynocephalus species, P. axillaris and P. forsythii from Kuerle, Xinjiang, China (41.50386°N, 86.2290°E, elevation = 934 m a.s.l.), were sampled on June 18–July 2, 2017, and transplanted to a high-altitude environment on July 4, 2017, in Zoige, the eastern part of Qinghai-Tibetan Plateau in Sichuan Province (33.71389°N, 102.48543°E, elevation = 3,400 m a.s.l.). Although a control treatment in the original site would provide consistent conditions for comparison after transplantation, it was difficult to conduct experiments without a well-equipped field workstation in the original site. To minimize bias caused by microhabitat differences, we built six outdoor seminatural enclosures (length × width × height = 5 m × 5 m × 1.5 m, Figure 1A), and the settings resembled the original habitat as much as possible. We used sand from a field site of P. vlangalii, a sister species of P. forsythii, as substrate, and had a fishing net suspended above each enclosure to reduce the risk of bird predation. The lizards were kept in the enclosures for 6 weeks to acclimate to the new environments, and mealworms were provided every 3 days to offset food shortage.
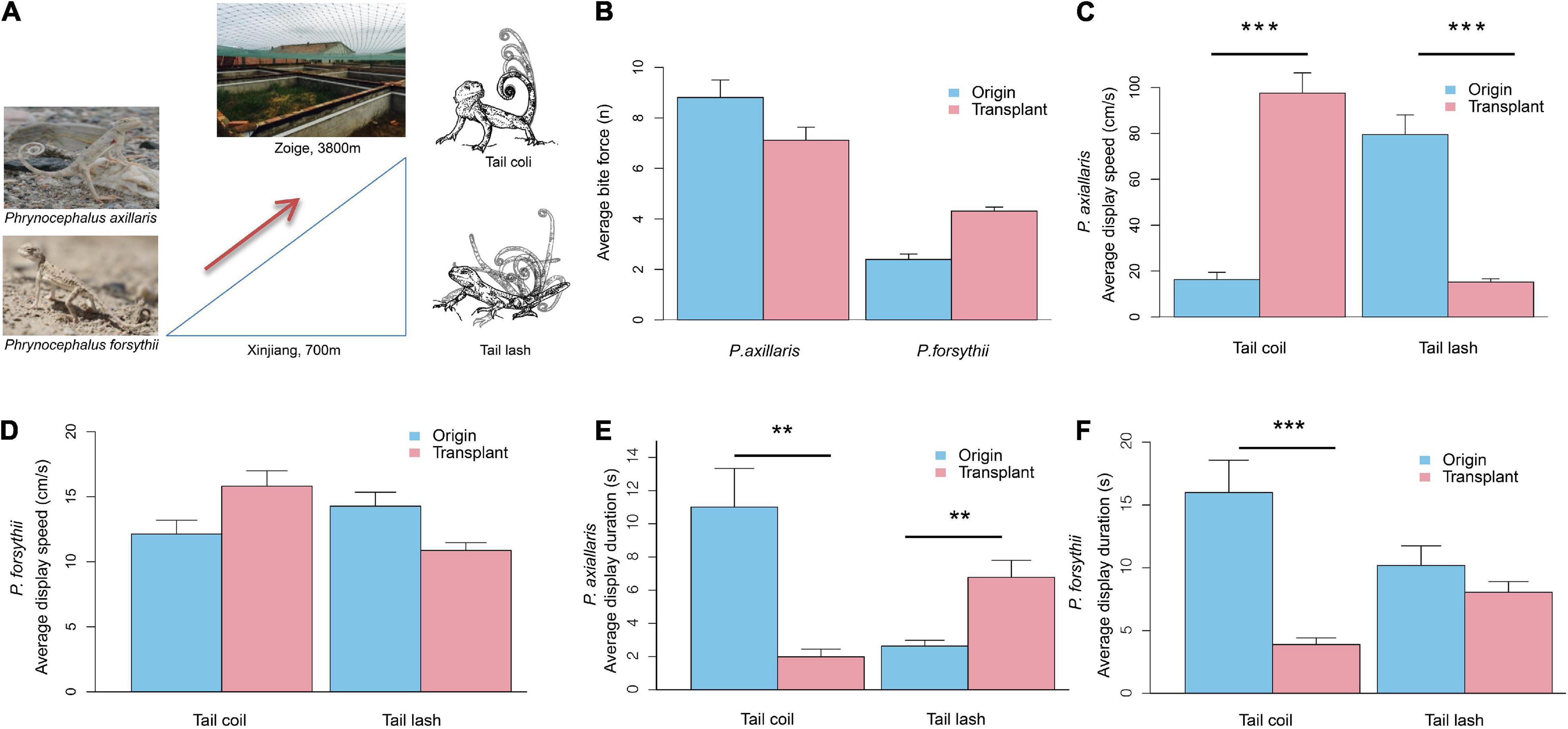
Figure 1. Transplant experimental design and plasticity in performance and behavior for Phrynocephalus axillaris and P. forsythii. (A) Image of P. axillaris and P. forsythii, the outdoor enclosures used for the common garden in Zoige, the eastern part of the Qinghai-Tibetan Plateau in Sichuan Province, and the schematic drawing of tail coil and tail lash for tail displays. (B) Comparison of bite force before and after transplant. (C) Comparison of tail coil speed and tail lash speed before and after transplant for P. axillaris. (D) Comparison of tail coil speed and tail lash speed before and after transplant for P. forsythii. (E) Comparison of tail coil duration and tail lash duration before and after transplant for P. axillaris. (F) Comparison of tail coil duration and tail lash duration before and after transplant for P. forsythii. In (B–F), bar plots represent the mean values, and error bars represent their standard error. Asterisks indicate statistical significance levels: **p < 0.01; ***p < 0.001.
We examined multiple levels of phenotypic plasticity by comparing lizard states before and after transplanting. The bite force was used to assess lizard body condition and performance in the new environments, while the tail display behavior, gene expression, and metabolism were used to assess the plasticity patterns.
Behavioral Data Collection
We collected data of tail display behavior from 25 males of P. axillaris and 26 males of P. forsythii from the original site (Figure 1A). The detailed protocols on display collection are described by Wu et al. (2018). First, we captured intruder males using noose from a different site 3 km away and measured their snout-vent length (SVL) to the nearest 0.01 mm using caliper. Then, the resident male was approached by a size-matched intruder using a 4-m-long fishing rod. Meanwhile, a video camera (HDR PJ670, Sony, Japan) was set up in front of the resident male and recorded the display response. The trial was terminated after the display stopped, or at a maximum of 5 min if no display was observed. At the conclusion of each trial, a ping-pong ball was placed at the location of the resident and recorded as a scale for subsequent analysis. The resident male was captured using a noose after filming. Immediately after capture, we measured the body temperature from the center of its dorsum with an IR thermometer (HT-866, HCJYET, China). The lizards were kept individually during the experiment. We also measured the SVL and bite force of each lizard. The SVL was measured using a caliper to the nearest 0.01 cm. The bite force was recorded using a piezoelectric force transducer (Type 9203, Kistler Inc., Switzerland) that connected to a charge amplifier (Type 5995A, Kistler Inc., Switzerland) and fitted with two metal bite plates (Vanhooydonck et al., 2005). We encouraged the lizards to bite the plates by gently tapping the mouth using a blunt metal probe. To minimize bias due to motivation and physical condition, we repeated the measurement three times and used the maximum value as the final measurement (Vanhooydonck et al., 2005).
A total of 22 male P. axillaris and 23 male P. forsythii were transplanted from the original site to the high-altitude site. Individuals of each species were randomly divided into three groups (7–8 for each) and kept in seminatural enclosures. After acclimation for 6 weeks, we recorded the social display behavior and measured SVL and bite force in the same way. All raw data of the behavioral experiment are shown in Supplementary Table 1.
Bite Force Plasticity Analysis
We analyzed the correlation between bite force and transplant treatments using linear models in the nlme package (Pinheiro et al., 2018) in R version 4.0.5 (R Core Team, 2015). The bite force was log-transformed before modeling to meet the assumption of Gaussian error distribution. We established a linear model with the transplant treatment as the main effect while considering individual body temperature as co-variable.
Display Digitization and Plasticity Analysis
To quantify the tail display, we tracked the motion of tail tips following the methods outlined by Hedrick (2008) in MATLAB 2015b (MathWorks Inc., Natick, MA, United States). The x-y coordinates of tail tips were determined using the DLT dv5 software (Hedrick, 2008) with successive frames. We extracted two tail display variables, the average display speed and display duration. The average display speed was defined as the average distance moved by tail tip within a specific time. The display duration was defined as the time of each display bout. We quantified the two variables for both tail coil display and tail lash display to examine whether tail display plasticity differed between components. As the orientation of the lizard relative to the camera likely affected tail display quantification (Bian et al., 2016), we categorized each display as either facing toward or away from the camera or at the right angle to the camera. Finally, we transformed the grid-based display variables to Euclidean distance in MATLAB using the ping-pong ball as scale.
We analyzed the correlation between tail display and transplant treatments using linear mixed models in the nlme package (Pinheiro et al., 2018) in R version 4.0.5 (R Core Team, 2015). All independent variables were log-transformed to meet the assumptions of the linear mixed model. For average tail display speed, we considered transplant treatment and display orientation as fixed effects, with lizard identity as a random effect and assuming a Gaussian error distribution. For display duration, we considered treatment as fixed effects, with lizard identity as a random effect and assuming a Gaussian error distribution. We included the SVL of residents as covariates in both tail display speed and duration models to account for the potential effect of body size.
Transcriptome Sequencing Analysis
For each species, 6 individuals from the original habitat and 6 transplanted individuals were subjected to part of the examination. Tissues of the heart, liver, and muscle were collected immediately after euthanization on the days of collection or immediately after the completion of the transplant experiments. The sample information is provided in Supplementary Table 2. Total RNA was extracted from each tissue sample according to the TRIzol protocols (Invitrogen, Carlsbad, California). Paired-end sequencing with a read length of 150 base pairs (bp) was carried out on the Illumina HiSeq2500 platform by Novogene (Beijing, China).
Raw sequencing reads were first cleaned by excluding the adapter sequences and low-quality base calls using a Novogene pipeline. Trimmomatic version 0.35 (Bolger et al., 2014) was used to trim the clean reads with LEADING:3, TRAILING:3, SLIDINGWINDOW:4:15, and MINLEN:70. We checked the read quality before and after trimming using FastQC version 0.11.8 (Andrews, 2010). Subsequently, quality-filtered reads of three different tissue samples from one representative individual of each species (A2, G1, and M2 for P. axillaris; C1, I1, and O1 for P. forsythii) were used in de novo assembly via Trinity version 2.8.4 after in silico read normalization (Grabherr et al., 2011; Haas et al., 2013). Since Trinity usually generates a large number of assembled transcripts, among which many may have questionable biological significance, we used kallisto version 0.44.0 (Bray et al., 2016) to quantify the abundance of the assembled transcripts and build expression matrices. Transcripts with “transcripts per million transcripts” (TPM) less than three were removed to generate the final assembly for each species. To obtain orthologous sequences among the two species, a best reciprocal hit (BRH) method was applied for the final assemblies via BLAST + version 2.7.1 (Camacho et al., 2009).
The clean reads for each tissue sample were mapped to the transcriptome assemblies for corresponding species by STAR version 2.6 (Dobin et al., 2013). The quantity of reads that matched to the same transcripts was counted using the HTSeq-count tool with the “union” resolution mode (Anders et al., 2015). The expression similarity among samples was calculated by the Euclidean distance and visualized by clustering heatmap after regularized log-transformation (rlog) of normalized counts via DESeq2 version 1.20 (Love et al., 2014). Principal component analysis (PCA) was used to assess the relationship between samples. We compared the samples that belonged to the same species separately to examine the expression divergence among different tissue types within one species. Differentially expressed genes (DEGs) were estimated using generalized linear models in edgeR package version 3.22.5 (Robinson et al., 2010; McCarthy et al., 2012). We used a strict thresold to characterize DEGs, with fold-change ≥ 2 and adjusted p-value < 0.05 [false discovery rate (FDR)].
Functional annotation was applied by aligning the transcripts to the UniProtKB/Swiss-Prot database (release “2018_08”) with BLAST hits E-value cutoff greater than 10–5. For transcripts with annotation information, overrepresentation test of DEGs was calculated using the clusterProfiler package (Yu et al., 2012) in R with annotation to the Gene Ontology (GO) category and the Kyoto Encyclopedia of Genes and Genomes (KEGG) pathway databases. The minimum number of transcripts required for each test of a given category was 10.
Metabolomic Assay Analysis
For each species, 10 individuals from the original habitat and 10 transplanted individuals were subjected to this part of the examination. The sampling of blood plasma was conducted immediately after euthanization on the days of collection or the completion of the transplant experiment. In detail, each lizard was fixed on a polyvinyl board. Then, we used ophthalmic scissors to remove the skin of the neck and make a cut on the Jugular vein. Notably, 40 μl blood plasma was extracted by a capillary (length: 100 mm; inner diameter: 1.55 mm) from the vein. Finally, the plasma was pushed into a 200-μl tube and then immediately stored in liquid nitrogen. The plasma samples were inputted for metabolome assay using the liquid chromatography-mass spectrometry (LC-MS) method (Brown et al., 2011; Dunn et al., 2011) and an ultra-performance liquid chromatography (UPLC)-TripleTOF 5600 system (AB SCIEX, Majorbio, Shanghai, China). Due to the different properties of metabolites, two modes (i.e., negative and positive ion modes) were used to detect metabolites. The raw data from LC-MS passed through a series of quality control phases, including baseline filtering, peak identification, integration, retention time correction, and peak alignment, and finally were normalized using the software XCMS (Tautenhahn et al., 2012). Final data matrices contained retention time, mass-to-charge ratio, and peak intensity. The identification of metabolites was carried out using substance-matching in the METLIN and HMDB databases and an in-house standard library according to the retention time and m/z. The SIMCA-14.1 (Umetrics, Kinnelon, United States) was used to perform orthogonal partial least squares discriminant analysis (OPLS-DA) for obtaining variable influence on projection (VIP) values. Differentially expressed metabolites (DEMs) were defined using the following cutoff criteria: (1) Benjamini-Hochberg-adjusted p-values being less than 0.05; (2) fold-change (expressed as the ratio of average metabolite abundance between groups) being less than 0.5 for downregulation or being larger than 2 for upregulation; (3) VIP being larger than 1.
The Chemical Similarity Enrichment Analysis (ChemRICH; Barupal and Fiehn, 2017) was conducted to classify the metabolites into biochemical clusters from metabolomic database using Chemical Translation Service (CTS) and PubChem Identifier Exchange Service (Barupal et al., 2018). ClassyFire (Feunang et al., 2016) was used to automatedly annotate metabolite classification with default parameters. In addition, to further identify key metabolic processes for various metabolites, null diffusion-based enrichment was conducted with the KEGG pathway database by runDiffusion function using the FELLA package (Picart-Armada et al., 2018).
Results
Plasticity in Bite Force
We found no significant change in bite force in P. axillaris (from 8.81 ± 0.70 n, n = 11 to 7.12 ± 0.51 n, n = 17; t = −0.95, p = 0.35; Figure 1B) and in P. forsythii (from 2.40 ± 0.22 n, n = 14 to 4.31 ± 0.16 n, n = 18; t = 0.74, p = 0.47; Figure 1B) after being acclimated to high-altitude environments.
Plasticity in Tail Display Behavior
We detected clear plasticity in tail display behavior in both species. In P. axillaris, average tail coil speed was significantly increased (from 16.28 ± 3.19 cm/s, n = 23 to 97.55 ± 8.82 cm/s, n = 20; t = 5.06, p = 0.0004; Figure 1C), while average tail lash speed was significantly decreased (from 79.50 ± 8.52 cm/s, n = 22 to 15.19 ± 1.49 cm/s, n = 22; t = −5.28, p = 0.0005; Figure 1C) after being acclimated to high elevation area. Meanwhile, the average tail coil duration decreased significantly from 11.01 ± 2.33 s (n = 23) to 1.98 ± 0.46 s (n = 20; t = −3.31, p = 0.007), while average tail lash duration increased significantly from 2.63 ± 0.35 s (n = 22) to 6.77 ± 1.02 s (n = 22; t = 4.00, p = 0.003; Figure 1E). In P. forsythii, we found no significant change in average tail coil speed (from 12.13 ± 1.06 cm/s, n = 13 to 15.82 ± 1.18 cm/s, n = 22; t = 1.63, p = 0.13; Figure 1D), in average tail lash speed (from 14.28 ± 1.07 cm/s, n = 20 to 10.87 ± 0.60 cm/s, n = 20; t = −0.97, p = 0.35; Figure 1D), and in average tail lash duration (from 10.19 ± 1.55 s, n = 20 to 8.05 ± 0.85 s, n = 20; t = −0.85, p = 0.41; Figure 1F) after being acclimated to high elevation area, but the average tail coil duration decreased significantly from 15.99 ± 2.57 s (n = 13) to 3.89 ± 0.52 s (n = 22; t = −4.73, p = 0.0004; Figure 1F).
Plasticity in Gene Expression
A total of 44,000,068–123,811,250 and 42,179,214–91,710,426 raw reads were generated for P. axillaris and P. forsythia by Illumina sequencing, respectively. After filtering, 41,832,664–118,600,900 and 39,967,100–90,040,782 reads were retained, respectively (Supplementary Table 3). For P. axillaris, 27,894 transcripts were obtained with an N50 size of 2,372 bp and a mean length of 1,224 bp. For P. forsythii, 31,519 transcripts were obtained with an N50 size of 2,185 bp and a mean length of 1,205 bp. By the BRH method, 8,892 orthologous transcripts were identified among the two species.
The PCA plot showed that the tissue-specific expression was very consistent for all types of tissues within the two species, and the expression divergence for any type of tissues between low- and high altitudes was lower than any cross-tissue comparisons (Figures 2A,B). Generally, transcriptomes from heart and muscle were less diverged than from liver for both species. As for DEGs of P. axillaris, 1,612 were identified in heart between low- and high-altitude groups, with 690 upregulated and 922 downregulated, given low-altitude sample as a reference; 2,792 DEGs were identified in liver, with 1,485 upregulated and 1,307 downregulated; 2,993 DEGs were identified in muscle, with 1,365 upregulated and 1,628 downregulated. Similarly, for P. forsythii, 913 DEGs were identified in heart, with 405 upregulated and 408 downregulated; 1,301 DEGs were identified in liver, with 722 upregulated and 579 downregulated; 1,994 DEGs were identified in muscle, with 868 upregulated and 1,126 downregulated.
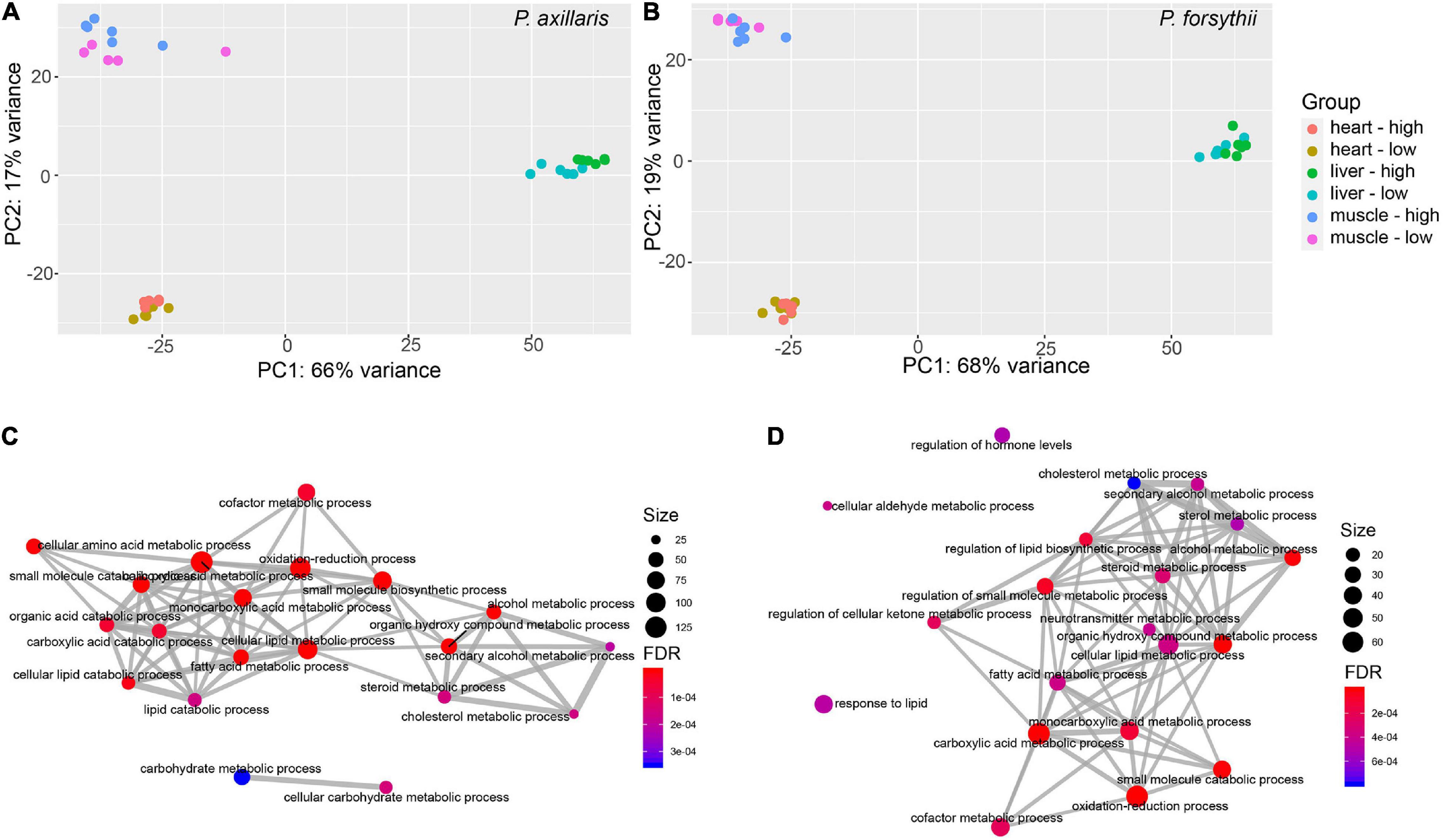
Figure 2. Plasticity in gene expression profiles. (A) Principal component analysis (PCA) plot for expression profile of P. axillaris. The colors of dots represent the experimental groups. (B) PCA plot for expression profile of P. forsythii. (C) Network for overrepresented Gene Ontology (GO) categories for differentially expressed genes (DEGs) in liver of P. axillaris. (D) Network for overrepresented GO categories in the liver for P. forsythii. In the networks, the size of dots represents the number of genes in each category, and the FDR represents the p-values after multiple correction by false discovery rate.
After functional annotation for DEGs of P. axillaris, a total of 32 GO categories and 1 KEGG pathways were identified as overrepresented in heart, most of which were associated with muscle function (e.g., GO: 0006936 muscle contraction, GO: 0003012 muscle system process) and small molecule metabolism (e.g., GO: 0019320 hexose catabolic process, GO:1901135 carbohydrate derivative metabolic process). In muscle, 158 GO categories and 7 KEGG pathways were overrepresented by DEGs, concentrating mostly on muscle function, including GO: 0003012 muscle system process, GO: 0006936 muscle contraction, and GO: 0006941 striated muscle contraction, and also associating with acid metabolism, such as GO: 0043436 oxoacid metabolic process. In liver, different from the other two tissues, 77 GO categories and 13 KEGG pathways were identified as overrepresented by DEGs, which were mostly associated with lipid metabolism, including GO: 0044255 cellular lipid metabolic process, GO: 0006631 fatty acid metabolic process, and GO: 0008202 steroid metabolic process. Similar patterns were also found in overrepresented KEGG pathways, such as map00071 fatty acid degradation and map01212 fatty acid metabolism (Figure 2C and Supplementary Figure 1).
For the DEGs of P. forsythii, four GO categories and one KEGG pathway were overrepresented in heart, which were all related to circadian regulation, including GO: 0032922 circadian regulation of gene expression, GO: 0009649 entrainment of the circadian clock, and pathway hsa04710: circadian rhythm. In muscle, 60 GO categories but no KEGG pathways were overrepresented by DEGs. Those categories were mostly related to cell migration and blood vessel development, including GO: 0030334 regulation of cell migration, GO: 0048514 blood vessel morphogenesis, and GO: 0010594 regulation of endothelial cell migration. In liver, 115 GO categories and 13 KEGG pathways were overrepresented. Similar to P. axillaris, most of those functional categories were also associated with lipid metabolism, including GO: 0008202 steroid metabolic process and GO: 0006631 fatty acid metabolic process, and pathways, such as map00071 fatty acid degradation (Figure 2D and Supplementary Figure 1).
Plasticity in Metabolism
A total of 4,523 features of metabolites were detected in the metabolome assay, among which 4,309 features were retained after filtering. After ChemRICH classification, 1,109 and 985 metabolites were identified with biochemical annotation for positive and negative ion mode of LC-MS, respectively. For P. axillaris, 421 DEMs were identified in positive ion mode with 83 upregulated and 338 downregulated; 482 DEMs were identified in negative ion mode with 80 upregulated and 412 downregulated. Similarly, for P. forsythii, 565 DEMs were identified in positive ion mode with 106 upregulated and 459 downregulated; 554 DEMs were identified in negative ion mode with 117 upregulated and 437 downregulated. Both positive and negative ion modes suggested that the patterns of metabolome for the two species were quite similar. For all biochemical clusters, amino acids, peptides, and analogs contained the most metabolites for both species, among which approximately 55% of the metabolites were differentially expressed. However, clusters associated with lipids were identified, showing a high ratio of DEMs, such as fatty acid esters, fatty acids and conjugates, and glycosphingolipids (Figure 3).
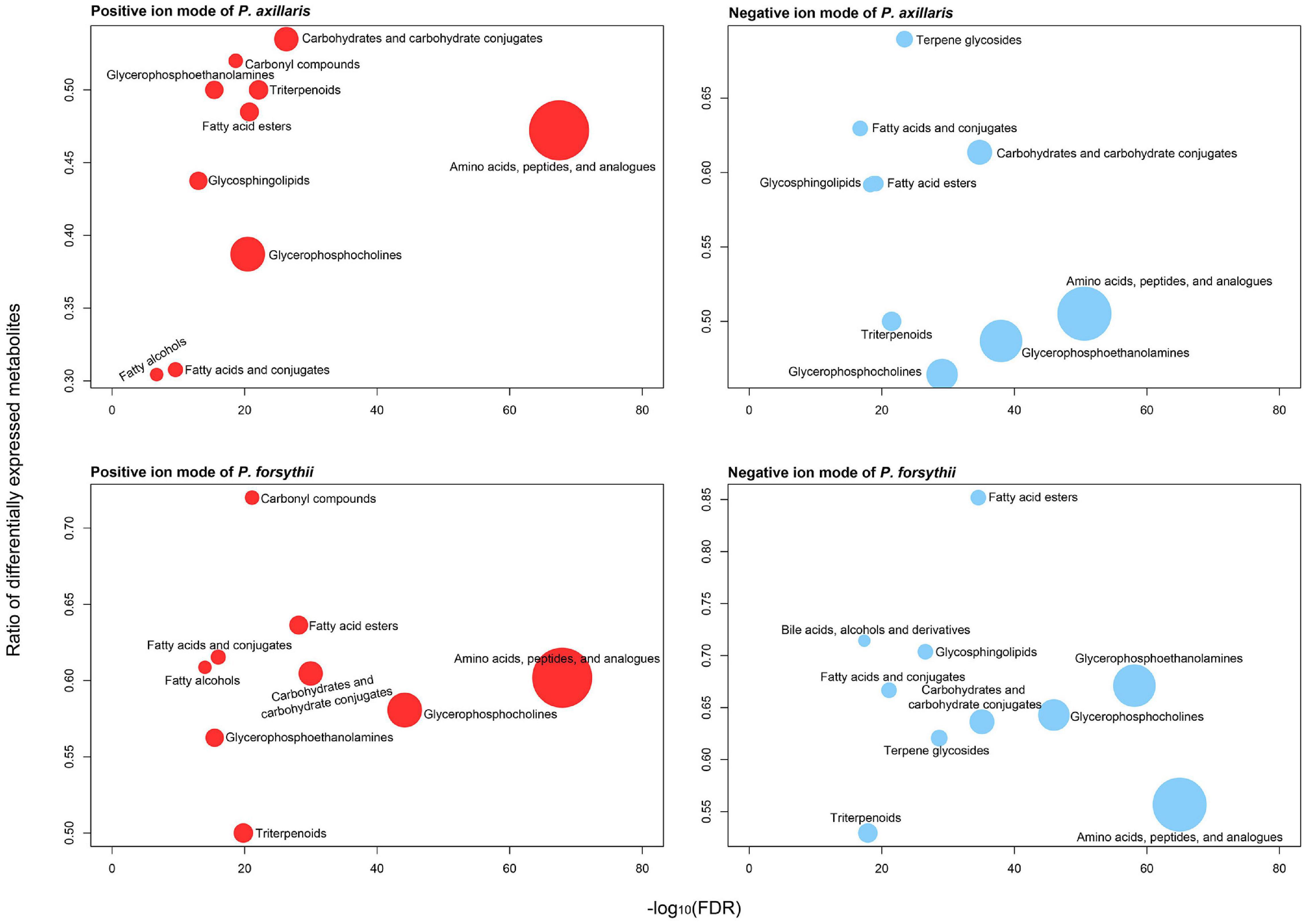
Figure 3. Plasticity in main biochemical clusters of metabolites identified in metabolome assay. Red color denotes metabolites from positive ion mode, and blue color denotes metabolites from negative ion mode. The top panels represent the metabolome in P. axillaris, and the bottom panels represent that in P. forsythii.
A total of 29 DEMs were mapped to KEGG pathways, among which 16 and 26 were from P. axillaris and P. forsythii, respectively. A high proportion (50.0% for P. axillaris and 53.8% for P. forsythii) of DEMs were associated with lipids, including those concordantly regulated in both species, such as the upregulation of 17α-Hydroxypregnenolone (cortisol synthesis and secretion) and sphingosine phosphocholine (sphingolipid metabolism) and the downregulation of cholesterol sulfate (steroid hormones biosynthesis) and L-palmitoylcarnitine (fatty acid degradation). In addition, lipid metabolism-related pathways were overrepresented by DEMs in both species, including fatty acid degradation, primary bile acid biosynthesis, and taurine and hypotaurine metabolism (Supplementary Table 4).
Fatty Acid Metabolism
Fatty acid metabolism appeared to be a major source of plasticity for both DEGs and DEMs, and therefore, we further examined detailed expression patterns for genes and metabolites associated with the fatty acid metabolic pathway (Figure 4A). We identified a total of 13 genes and 4 symbolic metabolites within the pathway that was differentially expressed in both species. Intriguingly, the patterns of up- or downregulation for those genes were identical in the two lizards, suggesting a common acclimation process in response to high-altitude environments for Phrynocephalus species. Among those genes, three play crucial roles in the fatty acid metabolic pathway: sterol regulatory element-binding transcription factor 1 (SREBF1), fatty acid synthase (FASN), and very long-chain-specific acyl-CoA dehydrogenase (ACADVL) (Figure 4B). SREBF1 acts as an inductor of lipogenesis in liver leading to increased storage of fatty acid as triglycerides for organisms. FASN encodes the enzyme FASN that catalyzes the synthesis of fatty acid from acetyl-CoA and is regulated by SREBF1 (Bhuiyan et al., 2009; Bouchard-Mercier et al., 2012). On the opposite side, ACADVL catalyzes the first step of mitochondrial fatty acid beta-oxidation, which digests fatty acid into acetyl-CoA before the citric acid cycle to produce energy, specifically for long-chain fatty acids (Miller et al., 2015). Among the metabolites within the pathway, L-palmitoylcarnitine is an important ester derivative of carnitine, which plays a core role in fatty acid metabolism by transporting long-chain fatty acids into mitochondria (Wood et al., 1984; Mutomba et al., 2000). In both P. axillaris and P. forsythii, SREBF1 and FASN showed significant upregulation, while ACADVL and the L-palmitoylcarnitine showed significant downregulation, which strongly suggested increased storage and decreased digestion of fatty acids.
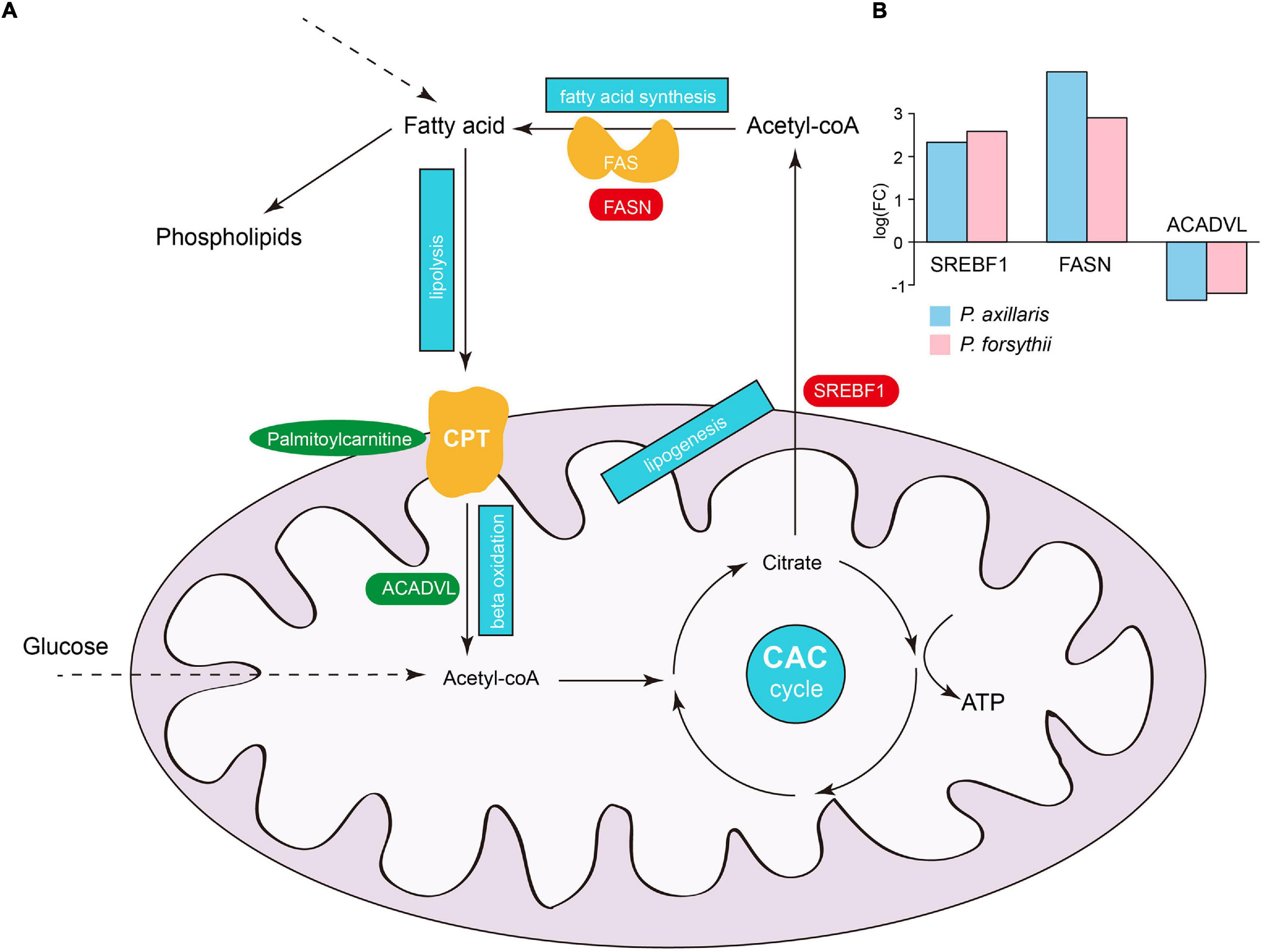
Figure 4. Plasticity in fatty acid metabolism. (A) A schematic illustration of fatty acid metabolism in organisms. The blue frames represent the important biological processes in the pathway: lipolysis, beta-oxidation, lipogenesis, citric acid cycle (CAC), and fatty acid synthesis. Crucial genes and metabolites in the pathway are indicated by red or blue colors, which represent upregulation and downregulation of genes or metabolites, respectively. (B) The expression fold-change of SREBF1, FASN, and ACADVL in P. axillaris and P. forsythii.
Discussion
The upslope range shifting is one of the primary responses for species under climatic changes (Pecl et al., 2017). Animals are predicted to colonize high-altitude regions following the shifting of suitable climatic conditions (Lenoir and Svenning, 2015). At high altitudes, key environmental factors, such as low oxygen availability, high levels of UV radiation, and their interactions, pose severe challenges to organisms, especially ectothermic species (Li et al., 2018; Sun et al., 2018). Our transplant experiments demonstrate that toad-headed lizards (P. axillaris and P. forsythii) from low altitudes are capable of acclimating to high-altitude environments without sacrificing their performance. P. axillaris showed high plasticity in tail display behavior, with increased tail coil speed and tail lash duration, as well as decreased tail coil duration and tail lash speed, while P. forsythii only showed reduced tail coil duration at high altitudes. Genes and metabolites associated with fatty acid metabolism were also identified with significant differentiation in expression when compared to individuals from low-altitude native habitats. Moreover, a large proportion of metabolites showed decreased expression for transplanted groups. Those consistent results imply that toad-headed lizards acclimate to high-altitude environments by reducing the behavioral intensity and increasing energy efficiency in multiple ways. Despite distinct reproductive models, the two lizards have highly concordant plasticity.
Plasticity in behavior plays a crucial role in dealing with the challenges of high-elevation environments from an energy cost perspective (Refsnider et al., 2018; Enriquez-Urzelai et al., 2020). As the direct connection between organisms and their environments, animal behavior is intimately correlated with energy metabolism (Ros et al., 2006; Mowles, 2014). We found that both species showed a trend of decreasing their tail display intensity, either by a specific component (e.g., tail coil in P. forsythii) or by a trade-off between different components (e.g., tail coil and tail lash in P. axillaris). Tail displays of Phrynocephalus lizards play important roles in social conflict alleviation and mate assessment (Wu et al., 2018), but they are energetically costly (Zhu et al., 2021). Consistent with our results, high-altitude Phrynocephalus lizards often constrain their activity intensity or reduce the display complexity (Hu et al., 2022). Behavioral traits are intrinsically plastic; lizards and many other ectotherms are well known for adjusting their behavior quickly in a new environment (Refsnider et al., 2018; Enriquez-Urzelai et al., 2020).
Plasticity in nutrient and energy metabolism can balance the requirements of life activities in high-altitude environments (Storz et al., 2010; Zhang et al., 2018). As the primary energy storage for animals, fatty acids play a key role in high-altitude plasticity and adaptation (Cheviron et al., 2012; Lui et al., 2015). Tang et al. (2013) first discovered that high-altitude Phrynocephalus species (Phrynocephalus erythrurus) had high-fat utility compared to its low-altitude counterpart (Phrynocephalus przewalskii). In this study, several core genes and metabolites associated with fatty acid metabolism show concerted patterns of differential expression, suggesting a common plastic response for both P. axillaris and P. forsythii. The SREBF1 gene in both species is also significantly upregulated at high altitudes, which functions to facilitate the process of lipogenesis by transforming other nutrients into fatty acids (Bhuiyan et al., 2009; Bouchard-Mercier et al., 2012). The upregulation of FASN is consistent with this process, which directly catalyzes fatty acid synthesis (Bhuiyan et al., 2009). In addition, ACADVL is downregulated at high altitudes, which suppresses the breaking down of long-chain fatty acids during beta-oxidation (Miller et al., 2015). Some endotherms have similar plastic responses at high altitudes. Yang et al. (2006) found upregulated leptin gene at high altitudes for the plateau pika; leptin facilitates the lipolysis process and is a key regulator for fatty acid metabolism (Pan et al., 2014). All these results suggest a common response of fatty acid metabolism, through lipogenesis, synthesis, and lipolysis, at high altitudes.
Global climatic change has increasingly been a leading cause for biodiversity loss (Sala et al., 2000; Thomas et al., 2004; Valtonen et al., 2017). Studying plasticity in response to high-altitude environments will provide useful insights into how organisms cope with new environments after upslope range shifting. In addition to directly buffering the environmental stress, plasticity may also provide direction for adaptive evolution toward high altitudes. The “plasticity-first evolution” model favors phenotypic plasticity that may lead to adaptation and is supported by an increasing number of cases (Moczek et al., 2011; Jones and Robinson, 2018). Environmental changes may first trigger phenotypic plasticity, and the selection on genotypes that influence the plastic expression of phenotypes will follow. Therefore, elucidating the nature of plasticity will help to predict directions of evolution and to manage conservation projects for organisms under the pressure of climatic change. Clearly, more studies are needed to unravel a comprehensive profile for phenotypic plasticity in behavior, performance, and metabolism for upslope range shifting species in high-altitude environments.
Data Availability Statement
The behavioral data that were collected in this study are available in the Supplementary Material of this article. All the sequencing data are deposited in National Genomics Data Center with accession number PRJCA008159.
Ethics Statement
The sampling and experiment in this study were carried out with permission (No. 2017005) from the Ethical Committee for Animal Experiments in Chengdu Institute of Biology, Chinese Academy of Sciences. All animal sample collection protocols complied with the current laws of China. All applicable guidelines for the care and use of animals were strictly followed.
Author Contributions
YQ, JF, and WY conceived the ideas and designed the experiments. YW, ZY, PP, and XQ conducted the field collection and transplant experiment. YQ and YW collected the behavior, performance, and RNASeq data. TZ and XT measured the metabolome assay. YQ, TZ, JF, and WY analyzed the data and drafted the manuscript. All authors read, edited, and approved the final submitted version of the manuscript.
Funding
This study was supported by the National Natural Science Foundation of China (Project Nos. 31501855 and 31872233) to WY and YQ and the Second Tibetan Plateau Scientific Expedition and Research Program (STEP; Grant Nos. 2019QZKK04020202 and 2019QZKK05010503).
Conflict of Interest
The authors declare that the research was conducted in the absence of any commercial or financial relationships that could be construed as a potential conflict of interest.
Publisher’s Note
All claims expressed in this article are solely those of the authors and do not necessarily represent those of their affiliated organizations, or those of the publisher, the editors and the reviewers. Any product that may be evaluated in this article, or claim that may be made by its manufacturer, is not guaranteed or endorsed by the publisher.
Acknowledgments
We are grateful to Cuoke, Erga, and X. Zhu for their logistic assistance in the field station. We have obtained the permits from the Zoige National Wetland Nature Reserve, where we have a long-term field research station on the ecology and evolution of lizards.
Supplementary Material
The Supplementary Material for this article can be found online at: https://www.frontiersin.org/articles/10.3389/fevo.2022.845072/full#supplementary-material
References
Anders, S., Pyl, P. T., and Huber, W. (2015). HTSeq—a Python framework to work with high-throughput sequencing data. Bioinformatics 31, 166–169. doi: 10.1093/bioinformatics/btu638
Andrews, S. (2010). FastQC: A Quality Control Tool for High Throughput Sequence Data. Available online at: https://raw.githubusercontent.com/s-andrews/FastQC/master/README.txt
Barupal, D. K., Fan, S., and Fiehn, O. (2018). Integrating bioinformatics approaches for a comprehensive interpretation of metabolomics datasets. Curr. Opin. Biotechnol. 54, 1–9. doi: 10.1016/j.copbio.2018.01.010
Barupal, D. K., and Fiehn, O. (2017). Chemical Similarity Enrichment Analysis (ChemRICH) as alternative to biochemical pathway mapping for metabolomic datasets. Sci. Rep. 7:14567. doi: 10.1038/s41598-017-15231-w
Bhuiyan, M. S. A., Yu, S. L., Jeon, J. T., Yoon, D., Cho, Y. M., Park, E. W., et al. (2009). DNA polymorphisms in SREBF1 and FASN genes affect fatty acid composition in Korean cattle (Hanwoo). Asian Australas. J. Anim. Sci. 22, 765–773. doi: 10.5713/ajas.2009.80573
Bian, X., Elgar, M., and Peters, R. (2016). The swaying behavior of Extatosoma tiaratum: motion camouflage in a stick insect? Behav. Ecol. 27, 83–92.
Bolger, A. M., Lohse, M., and Usadel, B. (2014). Trimmomatic: a flexible trimmer for Illumina sequence data. Bioinformatics 30, 2114–2120. doi: 10.1093/bioinformatics/btu170
Bouchard-Mercier, A., Paradis, A. M., Pérusse, L., and Vohl, M. C. (2012). Associations between polymorphisms in genes involved in fatty acid metabolism and dietary fat intakes. Lifestyle Genom. 5, 1–12. doi: 10.1159/000336511
Bray, N. L., Pimentel, H., Melsted, P., and Pachter, L. (2016). Near-optimal probabilistic RNA-seq quantification. Nat. Biotechnol. 34, 525–527. doi: 10.1038/nbt.3519
Brown, M., Wedge, D. C., Goodacre, R., Kell, D. B., Baker, P. N., Kenny, L. C., et al. (2011). Automated workflows for accurate mass-based putative metabolite identification in LC/MS-derived metabolomic datasets. Bioinformatics 27, 1108–1112. doi: 10.1093/bioinformatics/btr079
Camacho, C., Coulouris, G., Avagyan, V., Ma, N., Papadopoulos, J., Bealer, K., et al. (2009). BLAST+: architecture and applications. BMC Bioinformatics 10:421. doi: 10.1186/1471-2105-10-421
Cheviron, Z. A., Bachman, G. C., Connaty, A. D., McClelland, G. B., and Storz, J. F. (2012). Regulatory changes contribute to the adaptive enhancement of thermogenic capacity in high altitude deer mice. Proc. Natl. Acad. Sci. U. S. A. 109, 8635–8640. doi: 10.1073/pnas.1120523109
Cheviron, Z. A., Connaty, A. D., McClelland, G. B., and Storz, J. F. (2014). Functional genomics of adaptation to hypoxic cold-stress in high altitude deer mice: transcriptomic plasticity and thermogenic performance. Evolution 68, 48–62. doi: 10.1111/evo.12257
Corl, A., Bi, K., Luke, C., Challa, A. S., Stern, A. J., Sinervo, B., et al. (2018). The genetic basis of adaptation following plastic changes in coloration in a novel environment. Curr. Biol. 28:2970. doi: 10.1016/j.cub.2018.06.075
Dobin, A., Davis, C. A., Schlesinger, F., Drenkow, J., Zaleski, C., Jha, S., et al. (2013). STAR: ultrafast universal RNA-seq aligner. Bioinformatics 29, 15–21. doi: 10.1093/bioinformatics/bts635
Dunn, W. B., Broadhurst, D. I., Atherton, H. J., Goodacre, R., and Griffin, J. L. (2011). Systems level studies of mammalian metabolomes: the roles of mass spectrometry and nuclear magnetic resonance spectroscopy. Chem. Soc. Rev. 40, 387–426. doi: 10.1039/b906712b
Enriquez-Urzelai, U., Bernardo, N., Moreno-Rueda, G., Montori, A., and Llorente, G. (2019). Are amphibians tracking their climatic niches in response to climate warming? A test with Iberian amphibians. Clim. Chang. 154, 289–301. doi: 10.1007/s10584-019-02422-9
Enriquez-Urzelai, U., Tingley, R., Kearney, M. R., Sacco, M., Palacio, A. S., Tejedo, M., et al. (2020). The roles of acclimation and behaviour in buffering climate change impacts along elevational gradients. J. Anim. Ecol. 13, 1722–1734. doi: 10.1111/1365-2656.13222
Feunang, Y. D., Eisner, R., Knox, C., Chepelev, L., Hastings, J., Owen, G., et al. (2016). ClassyFire: automated chemical classification with a comprehensive, computable taxonomy. J. Cheminform. 8:61. doi: 10.1186/s13321-016-0174-y
Grabherr, M. G., Haas, B. J., Yassour, M., Levin, J. Z., Thompson, D. A., Amit, I., et al. (2011). Trinity: reconstructing a full-length transcriptome without a genome from RNA-Seq data. Nat. Biotechnol. 29:644.
Guo, X., and Wang, Y. (2007). Partitioned Bayesian analyses, dispersal-vicariance analysis, and the biogeography of Chinese toad-headed lizards (Agamidae: Phrynocephalus): a re-evaluation. Mol. Phylogenet. Evol. 45, 643–662. doi: 10.1016/j.ympev.2007.06.013
Haas, B. J., Papanicolaou, A., Yassour, M., Grabherr, M., Blood, P. D., Bowden, J., et al. (2013). De novo transcript sequence reconstruction from RNA-seq using the Trinity platform for reference generation and analysis. Nat. Protoc. 8, 1494–1512. doi: 10.1038/nprot.2013.084
Hammond, K. A., Szewczak, J., and Król, E. (2001). Effects of altitude and temperature on organ phenotypic plasticity along an altitudinal gradient. J. Exp. Biol. 204, 1991–2000. doi: 10.1242/jeb.204.11.1991
He, J., Xiu, M., Tang, X., Yue, F., Wang, N., Yang, S., et al. (2013). The different mechanisms of hypoxic acclimatization and adaptation in lizard Phrynocephalus vlangalii living on Qinghai-Tibet Plateau. J. Exp. Zool. A Ecol. Integr. Physiol. 319, 117–123. doi: 10.1002/jez.1776
Hedrick, T. L. (2008). Software techniques for two- and three-dimensional kinematic measurements of biological and biomimetic systems. Bioinspir. Biomim. 3:034001. doi: 10.1088/1748-3182/3/3/034001
Horscroft, J. A., Kotwica, A. O., Laner, V., West, J. A., Hennis, P. J., Levett, D. Z. H., et al. (2017). Metabolic basis to Sherpa altitude adaptation. Proc. Natl. Acad. Sci. U. S. A. 114, 6382–6387. doi: 10.1073/pnas.1700527114
Hu, Q., Lin, Y., Qiu, X., Fu, J., and Qi, Y. (2022). High-elevation adaptation of motion visual display modifications in the toad-headed agamid lizards (Phrynocephalus). Asian Herpetol. Res. (in press)
Jones, B. M., and Robinson, G. E. (2018). Genetic accommodation and the role of ancestral plasticity in the evolution of insect eusociality. J. Exp. Biol. 221:jeb153163. doi: 10.1242/jeb.153163
Kearney, M., Shine, R., and Porter, W. P. (2009). The potential for behavioral thermoregulation to buffer “cold-blooded” animals against climate warming. Proc. Natl. Acad. Sci. U. S. A. 106, 3835–3840. doi: 10.1073/pnas.0808913106
Kouyoumdjian, L., Gangloff, E. J., Souchet, J., Cordero, G. A., Dupoué, A., and Aubret, F. (2019). Transplanting gravid lizards to high elevation alters maternal and embryonic oxygen physiology, but not reproductive success or hatchling phenotype. J. Exp. Biol. 222:16. doi: 10.1242/jeb.206839
Lenoir, J., and Svenning, J. C. (2015). Climate-related range shifts–a global multidimensional synthesis and new research directions. Ecography 38, 15–28.
Li, J. T., Gao, Y. D., Xie, L., Deng, C., Shi, P., Guan, M. L., et al. (2018). Comparative genomic investigation of high-elevation adaptation in ectothermic snakes. Proc. Natl. Acad. Sci. U. S. A. 115, 8406–8411. doi: 10.1073/pnas.1805348115
Love, M. I., Huber, W., and Anders, S. (2014). Moderated estimation of fold change and dispersion for RNA-seq data with DESeq2. Genome Biol. 15:550. doi: 10.1186/s13059-014-0550-8
Lui, M. A., Mahalingam, S., Patel, P., Connaty, A. D., Ivy, C. M., Cheviron, Z. A., et al. (2015). High altitude ancestry and hypoxia acclimation have distinct effects on exercise capacity and muscle phenotype in deer mice. Am. J. Physiol. Regul. Integr. Comp. Physiol. 308:R779. doi: 10.1152/ajpregu.00362.2014
McCarthy, D. J., Chen, Y., and Smyth, G. K. (2012). Differential expression analysis of multifactor RNA-Seq experiments with respect to biological variation. Nucleic Acids Res. 40, 4288–4297. doi: 10.1093/nar/gks042
Miller, M. J., Burrage, L. C., Gibson, J. B., Strenk, M. E., Lose, E. J., Bick, D. P., et al. (2015). Recurrent ACADVL molecular findings in individuals with a positive newborn screen for very long chain acyl-coA dehydrogenase (VLCAD) deficiency in the United States. Mol. Genet. Metab. 116, 139–145. doi: 10.1016/j.ymgme.2015.08.011
Moczek, A. P., Sultan, S., Foster, S., Ledón-Rettig, C., Dworkin, I., Nijhout, H. F., et al. (2011). The role of developmental plasticity in evolutionary innovation. Proc. R. Soc. B Biol. Sci. 278, 2705–2713. doi: 10.1098/rspb.2011.0971
Mowles, S. L. (2014). The physiological cost of courtship: field cricket song results in 408 anaerobic metabolism. Anim. Behav. 89, 39–43.
Mutomba, M. C., Yuan, H., Konyavko, M., Adachi, S., Yokoyama, C. B., Esser, V., et al. (2000). Regulation of the activity of caspases by L-carnitine and palmitoylcarnitine. FEBS Lett. 478, 19–25. doi: 10.1016/s0014-5793(00)01817-2
Pan, H., Guo, J., and Su, Z. (2014). Advances in understanding the interrelations between leptin resistance and obesity. Physiol. Behav. 130, 157–169. doi: 10.1016/j.physbeh.2014.04.003
Parmesan, C., and Yohe, G. (2003). A globally coherent fingerprint of climate change impacts across natural systems. Nature 421, 37–42. doi: 10.1038/nature01286
Pecl, G. T., Araújo, M. B., Bell, J. D., Blanchard, J., Bonebrake, T. C., Chen, I. C., et al. (2017). Biodiversity redistribution under climate change: impacts on ecosystems and human well-being. Science 355:eaai9214. doi: 10.1126/science.aai9214
Picart-Armada, S., Fernández-Albert, F., Vinaixa, M., Yanes, O., and Perera-Lluna, A. (2018). FELLA: an R package to enrich metabolomics data. BMC Bioinformatics 19:538. doi: 10.1186/s12859-018-2487-5
Pinheiro, J., Bates, D., DebRoy, S., and Sarkar, D. R Core Team (2018). Nlme: linear and nonlinear mixed effects models. R package version 3.1-137.
Qu, Y., Zhao, H., Han, N., Zhou, G., Song, G., Gao, B., et al. (2013). Ground tit genome reveals avian adaptation to living at high altitudes in the Tibetan plateau. Nat. Commun. 4:2071. doi: 10.1038/ncomms3071
R Core Team (2015). R: A Language and Environment for Statistical Computing. Vienna: R Foundation for Statistical Computing.
Refsnider, J. M., Qian, S. S., Streby, H. M., Carter, S. E., Clifton, I. T., Siefker, A. D., et al. (2018). Reciprocally transplanted lizards along an elevational gradient match light environment use of local lizards via phenotypic plasticity. Funct. Ecol. 32, 1227–1236.
Robinson, M. D., McCarthy, D. J., and Smyth, G. K. (2010). edgeR: a Bioconductor package for differential expression analysis of digital gene expression data. Bioinformatics 26, 139–140. doi: 10.1093/bioinformatics/btp616
Root, T. L., Price, J. T., Hall, K. R., Schneider, S. H., Rosenzweig, C., and Pounds, J. A. (2003). Fingerprints of global warming on wild animals and plants. Nature 421, 57–60. doi: 10.1038/nature01333
Ros, A. F. H., Becker, K., and Oliveira, R. F. (2006). Aggressive behaviour and energy metabolism in a cichlid fish, Oreochromis mossambicus. Physiol. Behav. 89, 164–170. doi: 10.1016/j.physbeh.2006.05.043
Sala, J. Q., Olcina, A. G., Cuevas, A. P., Cantos, J. O., Amoros, A. R., and Chiva, E. M. (2000). Climatic warming in the Spanish Mediterranean: natural trend or urban effect. Clim. Chang. 46, 473–483.
Scheinfeldt, L., and Tishkoff, S. (2010). Living the high life: high altitude adaptation. Genome Biol. 11:133.
Scott, G. R., Elogio, T. S., Lui, M. A., Storz, J. F., and Cheviron, Z. A. (2015). Adaptive modifications of muscle phenotype in high altitude deer mice are associated with evolved changes in gene regulation. Mol. Biol. Evol. 32, 1962–1976. doi: 10.1093/molbev/msv076
Scoville, A. G., and Pfrender, M. E. (2010). Phenotypic plasticity facilitates recurrent rapid adaptation to introduced predators. Proc. Natl. Acad. Sci. U. S. A. 107, 4260–4263. doi: 10.1073/pnas.0912748107
Seebacher, F. (2005). A review of thermoregulation and physiological performance in reptiles: what is the role of phenotypic flexibility? J. Comp. Physiol. B 175, 453–461. doi: 10.1007/s00360-005-0010-6
Seebacher, F., Sparrow, J., and Thompson, M. B. (2004). Turtles (Chelodina longicollis) regulate muscle metabolic enzyme activity in response to seasonal variation in body temperature. J. Comp. Physiol. B 174, 205–210. doi: 10.1007/s00360-003-0331-2
Sinervo, B., Miles, D. B., Wu, Y., Cruz, F. R. M. D. L., Kirchhof, S., and Qi, Y. (2018). Climate change, thermal niches, extinction risk and maternal-effect rescue of toad-headed lizards, Phrynocephalus, in thermal extremes of the Arabian Peninsula to the Qinghai—Tibetan Plateau. Integr. Zool. 13, 450–470. doi: 10.1111/1749-4877.12315
Storz, J. F. (2007). Hemoglobin function and physiological adaptation to hypoxia in high altitude mammals. J. Mammal. 88, 24–31. doi: 10.1644/06-mamm-s-199r1.1
Storz, J. F., Scott, G. R., and Cheviron, Z. A. (2010). Phenotypic plasticity and genetic adaptation to high altitude hypoxia in vertebrates. J. Exp. Biol. 213, 4125–4136. doi: 10.1242/jeb.048181
Sun, Y. B., Fu, T. T., Jin, J. Q., Murphy, R. W., Hillis, D. M., Zhang, Y. P., et al. (2018). Species groups distributed across elevational gradients reveal convergent and continuous genetic adaptation to high elevations. Proc. Natl. Acad. Sci. U. S. A. 115, E10634–E10641. doi: 10.1073/pnas.1813593115
Tang, X., Xin, Y., Wang, H., Li, W., Zhang, Y., Liang, S., et al. (2013). Metabolic characteristics and response to high altitude in Phrynocephalus erythrurus (Lacertilia: Agamidae), a lizard dwell at altitudes higher than any other living lizards in the world. PLoS One 8:e71976. doi: 10.1371/journal.pone.0071976
Tautenhahn, R., Patti, G. J., Rinehart, D., and Siuzdak, G. (2012). XCMS Online: a web-based platform to process untargeted metabolomic data. Anal. Chem. 84, 5035–5039. doi: 10.1021/ac300698c
Thomas, C. D., Cameron, A., Green, R. E., Bakkenes, M., Beaumont, L. J., Collingham, Y. C., et al. (2004). Extinction risk from climate change. Nature 427, 145–148.
Thomas, C. D., Franco, A. M., and Hill, J. K. (2006). Range retractions and extinction in the face of climate warming. Trends Ecol. Evol. 21, 415–416. doi: 10.1016/j.tree.2006.05.012
Thomas, C. D., and Lennon, J. J. (1999). Birds extend their ranges northwards. Nature 399, 213–213. doi: 10.1038/20335
Valtonen, A., Latja, R., Leinonen, R., and Pöysä, H. (2017). Arrival and onset of breeding of three passerine birds in eastern Finland tracks climatic variation and phenology of insects. J. Avian Biol. 48, 785–795. doi: 10.1111/jav.01128
Vanhooydonck, B., Herrel, A., Van Damme, R., and Irschick, D. (2005). Does dewlap size predict male bite performance in Jamaican Anolis lizards? Funct. Ecol. 19, 38–42.
Wilson, R. S., and Franklin, C. E. (2002). Testing the beneficial acclimation hypothesis. Trends Ecol. Evol. 17, 66–70.
Wood, C., Jalil, M. N. H., McLaren, I., Yong, B. C. S., Ariffin, A., McNeil, P. H., et al. (1984). Carnitine long-chain acyltransferase and oxidation of palmitate, palmitoyl coenzyme A and palmitoylcarnitine by pea mitochondria preparations. Planta 161, 255–260. doi: 10.1007/BF00982922
Wu, Y., Ramos, J. A., Qiu, X., Peters, R. A., and Qi, Y. (2018). Female–female aggression functions in mate defence in an Asian agamid lizard. Anim. Behav. 135, 215–222.
Yang, J., Zhao, X. Q., Guo, S. C., Li, H. G., Qi, D. L., Wang, D. P., et al. (2006). Leptin cDNA cloning and its mRNA expression in plateau pikas (Ochotona curzoniae) from different altitudes on Qinghai-Tibet Plateau. Biochem. Biophys. Res. Commun. 345, 1405–1413. doi: 10.1016/j.bbrc.2006.05.052
Yang, W., Qi, Y., and Fu, J. (2014). Exploring the genetic basis of adaptation to high elevations in reptiles: a comparative transcriptome analysis of two toad-headed agamas (Genus Phrynocephalus). PLoS One 9:e112218. doi: 10.1371/journal.pone.0112218
Yu, G., Wang, L. G., Han, Y., and He, Q. Y. (2012). clusterProfiler: an R package for comparing biological themes among gene clusters. OMICS 16, 284–287. doi: 10.1089/omi.2011.0118
Zhang, L., Li, J., Meng, J., Du, H., Lv, M., and Zhu, W. (2018). Thermal performance analysis of a high altitude solar-powered hybrid airship. Renew. Energy 125, 890–906.
Zhang, W., Fan, Z., Han, E., Hou, R., Zhang, L., Galaverni, M., et al. (2014). Hypoxia adaptations in the grey wolf (Canis lupus chanco) from Qinghai-Tibet Plateau. PLoS Genet. 10:e1004466. doi: 10.1371/journal.pgen.1004466
Keywords: high altitude, lizards, behavior, performance, gene expression, metabolome, plasticity
Citation: Qi Y, Zhang T, Wu Y, Yao Z, Qiu X, Pu P, Tang X, Fu J and Yang W (2022) A Multilevel Assessment of Plasticity in Response to High-Altitude Environment for Agama Lizards. Front. Ecol. Evol. 10:845072. doi: 10.3389/fevo.2022.845072
Received: 29 December 2021; Accepted: 11 February 2022;
Published: 24 March 2022.
Edited by:
Bao-jun Sun, Institute of Zoology (CAS), ChinaReviewed by:
Nicholas Wu, Western Sydney University, AustraliaHong-Liang Lu, Hangzhou Normal University, China
Copyright © 2022 Qi, Zhang, Wu, Yao, Qiu, Pu, Tang, Fu and Yang. This is an open-access article distributed under the terms of the Creative Commons Attribution License (CC BY). The use, distribution or reproduction in other forums is permitted, provided the original author(s) and the copyright owner(s) are credited and that the original publication in this journal is cited, in accordance with accepted academic practice. No use, distribution or reproduction is permitted which does not comply with these terms.
*Correspondence: Weizhao Yang, eWFuZ3d6QGNpYi5hYy5jbg==
†These authors have contributed equally to this work