- 1Department of Veterinary Medicine, University of Sassari, Sassari, Italy
- 2Unit for Conservation Genetics (BIO-CGE), Italian Institute for Environmental Protection and Research (ISPRA), Ozzano dell’Emilia, Italy
- 3Faculty of Mathematics, Natural Sciences and Information Technologies, University of Primorska, Koper, Slovenia
- 4Department of Chemistry and Bioscience, Aalborg University, Aalborg, Denmark
Human activities can globally modify natural ecosystems determining ecological, demographic and range perturbations for several animal species. These changes can jeopardize native gene pools in different ways, leading either to genetic homogenization, or conversely, to the split into genetically divergent demes. In the past decades, most European wild boar (Sus scrofa) populations were heavily managed by humans. Anthropic manipulations have strongly affected also Italian populations through heavy hunting, translocations and reintroductions that might have deeply modified their original gene pools. In this study, exploiting the availability of the well-mapped porcine genome, we applied genomic tools to explore genome-wide variability in Italian wild boar populations, investigate their genetic structure and detect signatures of possible introgression from domestic pigs and non-native wild boar. Genomic data from 134 wild boar sampled in six areas of peninsular Italy and in Sardinia were gathered using the Illumina Porcine SNP60 BeadChip (60k Single Nucleotide Polymorphisms – SNPs) and compared with reference genotypes from European specimens and from domestic pigs (both commercial and Italian local breeds), using multivariate and maximum-likelihood approaches. Pairwise FST values, multivariate analysis and assignment procedures indicated that Italian populations were highly differentiated from all the other analyzed European wild boar populations. Overall, a lower heterozygosity was found in the Italian population than in the other European regions. The most diverging populations in Castelporziano Presidential Estate and Maremma Regional Park can be the result of long-lasting isolation, reduced population size and genetic drift. Conversely, an unexpected similarity was found among Apennine populations, even at high distances. Signatures of introgression from both non-Italian wild boar and domestic breeds were very limited. To summarize, we successfully applied genome-wide procedures to explore, for the first time, the genomic diversity of Italian wild boar, demonstrating that they represent a strongly heterogeneous assemblage of demes with different demographic and manipulation histories. Nonetheless, our results suggest that a native component of genomic variation is predominant over exogenous ones in most populations.
Introduction
Animal species can be strongly managed by humans across their natural ranges, being harvested, translocated or sometimes admixed with alien or domesticated forms. All these direct actions can amplify the effects of other ecological perturbations, such as habitat or landscape modifications, contributing to jeopardizing the gene pool of wild populations and their adaptive capacities (Allendorf et al., 2008; Laikre et al., 2010; Boyce et al., 2011; Apollonio et al., 2014).
In Europe, large mammals, that have survived as metapopulations after experiencing demographic and range fluctuations, mainly due to human impacts, are recently spreading to form larger continuous populations (Apollonio et al., 2010a; Chapron et al., 2014; Martin et al., 2020). This apparent new range continuity may underlie genetic gaps which are the legacy of a past fragmentation and that are tricky to be detected. On the opposite, admixture between species, subspecies or populations can take place at suture zones or at release sites, leading to a local increase of genetic variation and to a potential loss of local adaptations.
In the past decades, most genetic investigations to identify cryptic population structure or local admixture in different species were conducted relying on limited sets of autosomal microsatellites (Frantz et al., 2006; Dellicour et al., 2011; Hindrikson et al., 2013; Olano-Marin et al., 2014; Biosa et al., 2015; Silva et al., 2018). However, the genomic era has provided new tools and a higher diagnostic power to detect gene pool differences and admixture signals, creating the conditions to disentangle complicated patterns due to high levels of human disturbance.
The European wild boar (Sus scrofa) population is a good example of the aforementioned changes: after a strong decline resulting in a fragmented and scattered distribution, it is now covering almost continuously all the continent up to the Baltic countries (Apollonio et al., 2010a) with a steadily increasing trend (Massei et al., 2015). In Italy, the species went through demographic declines and range contractions until the first half of the XX century (Ghigi, 1911, 1950), followed by a continuous re-expansion (Apollonio et al., 1988) that was sustained by socio-economic changes, leading to habitat recovery, and by the release of captive-reared or imported individuals (Apollonio et al., 2010b). After a progressive increase, the wild boar today represents one of the most invasive and impacting animals in the Country, likely due also to its genetic make-up which might have played a crucial role in its adaptation to a variety of different environmental and ecological contexts.
During the major range contraction, Italian wild boar survived only in the coastal Tyrrhenian region of Central Italy (named Maremma), in a few central-southern areas of the peninsula, where a relict isolated population persisted within a formerly royal hunting estate that became presidential estate since 1948 (Castelporziano), and in the island of Sardinia (Ghigi, 1911).
Morphometric analyses induced De Beaux and Festa (1927) to propose the Maremma and Sardinian populations as belonging to two different endemic subspecies, respectively, Sus scrofa majori and Sus scrofa meridionalis. However, the population recovery, which mostly followed the World War II, involved both the natural spread from existing populations (like the recolonization of the Western Alps from France) and artificial processes such as reintroductions and population restocking (Monaco et al., 2007; Apollonio et al., 2010b). These latter operations implied a massive release of boars from different sources, that possibly admixed with local wild stocks. In the assessment of the status of Eurasian Sus scrofa populations dated 1993, the IUCN Specialist Group reported the Castelporziano population as likely representing the “only surviving pure-bred native stock” in Italy (Oliver et al., 1993). The first genetic studies on Italian wild boar, based on mitochondrial DNA (mtDNA) sequencing, revealed the existence of an endemic Italian mtDNA lineage, occurring both in historical and modern samples (Randi, 1995; Larson et al., 2005; Scandura et al., 2008, 2009), that ended up to be isolated in Italy during glacial periods (Vilaça et al., 2014; Maselli et al., 2016; Khederzadeh et al., 2019). Further studies confirmed the occurrence of native genetic variation also in autosomal regions, although detecting signatures of local admixture with non-native stocks (Vernesi et al., 2003; Scandura et al., 2008; Veličković et al., 2016). Nonetheless, this information was only partial and not conclusive, since these studies were based on a limited number of molecular markers and the number of sampled populations and individuals analyzed were poorly representative of the variety of situations that are likely to occur in the Italian peninsula. More refined details were obtained on the Sardinian populations, which, despite the intricate pattern of genetic structure and local introgression from non-native wild boar and domestic pigs, still retain a meaningful proportion of endemic diversity, well-distinguishing them from the related peninsular conspecifics (Scandura et al., 2008, 2009, 2011; Iacolina et al., 2016).
However, all studies so far conducted on this species in Italy have experienced the same difficulties to untangle local effects of human manipulation, including the admixture among individuals from multiple wild and domestic sources (Vernesi et al., 2003; Scandura et al., 2011; Veličković et al., 2016; Canu et al., 2018).
The advent of genome-wide single nucleotide polymorphisms (SNPs) can offer practical solutions. Such markers have, indeed, the advantage to be the most widespread source of standing variation at both neutral and non-neutral genomic regions, and being bi-allelic, they permit to easily gather fully comparable data, which can be also merged with already published and publicly available datasets.
Additionally, the development of genome-wide genotyping assays in the domestic pig (Porcine SNP60 BeadChip; Ramos et al., 2009) and the availability of comprehensive genome data for this model species provide a powerful tool to explore genomic variation in wild boar populations and to investigate population history, as well as patterns of differentiation and introgression (Goedbloed et al., 2013a,b; Manunza et al., 2013; Iacolina et al., 2016; Alexandri et al., 2017; Smyser et al., 2020).
Therefore, in this study we genotyped a set of Italian wild boar collected from different peninsular and insular areas using the Porcine SNP60 BeadChip, which were then combined and analyzed together with publicly available genotypes of domestic pigs and wild boar from several European populations. We built an exhaustive genome-wide dataset including a good representation of the overall genomic variation so far available for the Italian population, which was analyzed by multivariate and maximum-likelihood approaches to specifically: (i) assess levels of genomic variation in the Italian wild boar populations; (ii) detect local population structure and quantify the extent of genomic differentiation; (iii) investigate the genomic make-up of the most differentiated local populations, distinguishing between multiple sources of standing variation (native vs. non-native wild boar, and commercial vs. local domestic pig breeds).
Materials and Methods
Sampling, Genotyping and Dataset Building
We analyzed 98 wild boar (WB) individuals collected in six administrative regions spanning the Italian peninsula (Aosta Valley, Liguria, Tuscany, Latium and Calabria, Figure 1). Sampling areas differ in latitude, orography, habitat, human density, and WB presence. Moreover, for comparative purposes we included in the analyses 7 rural domestic pigs (DP) from the same area in Calabria where WB were sampled.
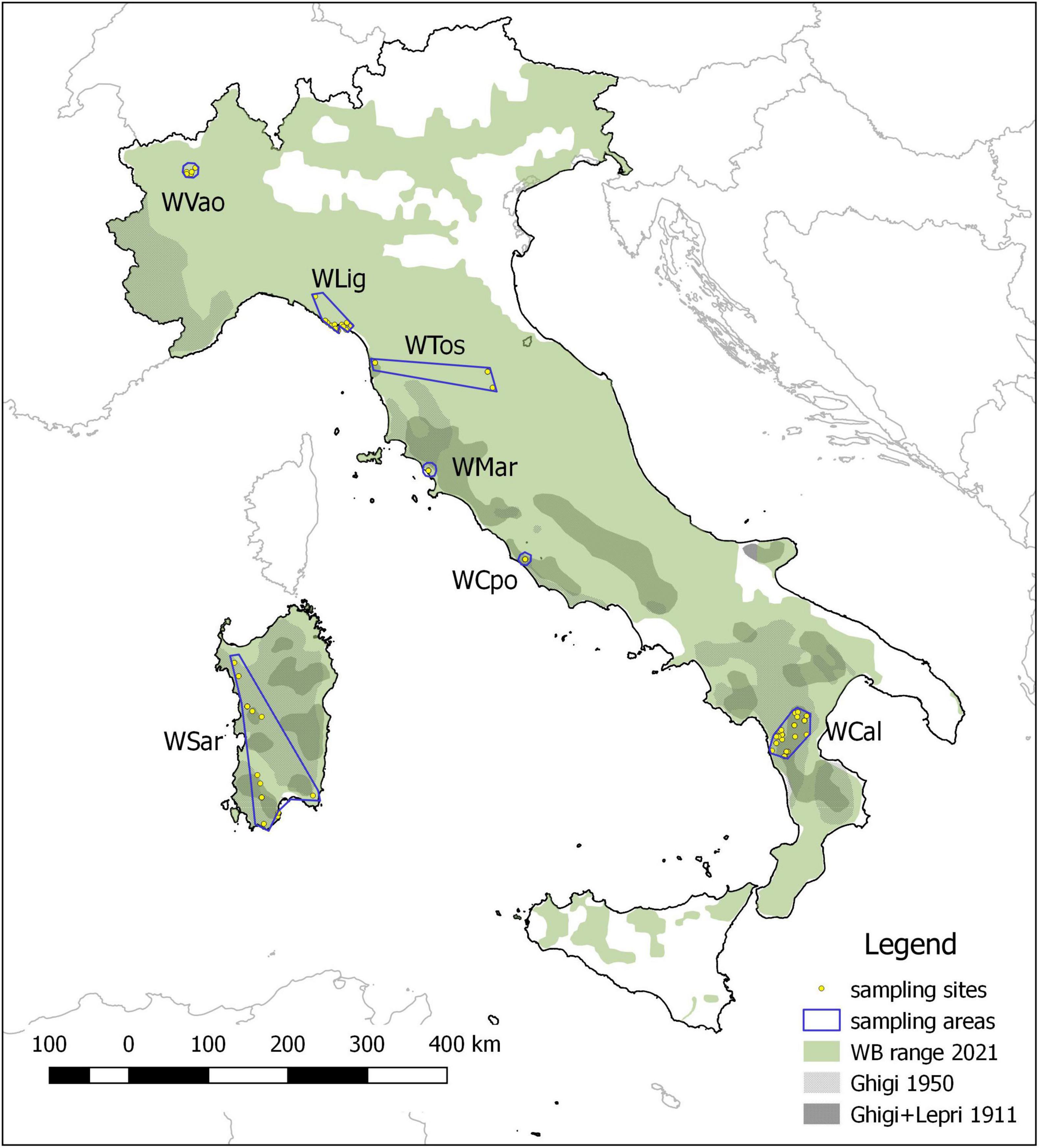
Figure 1. Wild boar historical and current distribution in Italy and sampling areas. The historical range refers to the minimum extension reached at the beginning (Ghigi, 1911; Lepri, 1911) and at the middle of the XX century (Ghigi, 1950), which was followed by a progressive expansion leading to the current distribution.
DNA was extracted from muscular tissue samples using the Qiagen DNAEasy Blood and Tissue kit (Qiagen Inc., Hilden, Germany) according to the manufacturer’s instructions, quantified using the Qubit™ Fluorometer (Invitrogen Thermo Fisher Scientific, Waltham, MA, United States) and normalized to a final concentration of 25–50 ng/μl.
DNA samples were genotyped using the Illumina Porcine SNP60 BeadChip v2 (Illumina Inc., San Diego, CA, United States; Ramos et al., 2009) at AIA1 and the results were combined with 164 additional homologous WB genotypes, including 36 samples from the Italian populations of Sardinia and Tuscany and 128 spanning most of the European distribution range. All these additional individuals were previously analyzed in a study focused on the Sardinian WB (Iacolina et al., 2016). A total of 103 porcine genotypes (56 from Italian local breeds – LocDP – and 47 from commercial breeds – ComDP) previously analyzed from Iacolina et al. (2016) and AIA were added to verify possible genomic introgression into WB populations (Table 1).
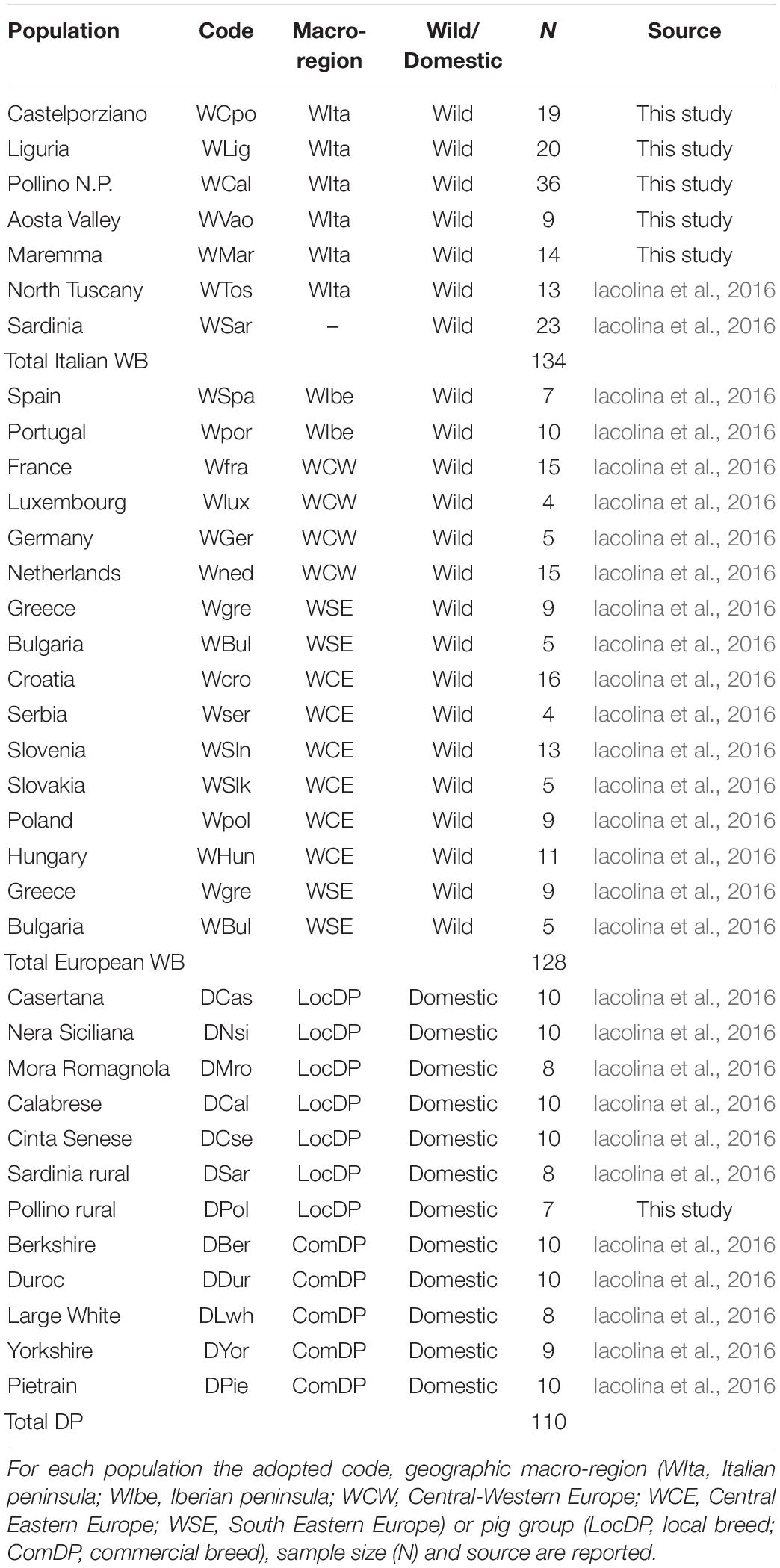
Table 1. List of Italian and European wild boar (WB) and domestic pig (DP) samples used in the study.
An overall dataset of 372 individuals (262 WB and 110 DP, Table 1) genotyped at 48,296 autosomal SNPs was thus obtained.
The number of available genotypes to be included in the dataset was selected so as to limit bias in the analyses introduced by unequal sample sizes. Based on previous studies (Goedbloed et al., 2013b; Alexandri et al., 2017; Iacolina et al., 2018), we grouped European (non-Italian) samples into four macro-regions: Iberia (WIbe), Central-Western Europe (WCW), Central-Eastern Europe (WCE), South-Eastern Europe (WSE, Table 1).
Dataset Filtering
The initial dataset was processed for quality control and filtering in the SNP & Variation Suite 8.0.1 (SVS, Golden Helix Inc., Bozeman, MT, United States) keeping only samples and loci with high genotyping rates (>0.95), and checking for related individuals through Identity By Descent (IBD) pairwise tests to remove one individual per pair where IBD > 0.5. After data filtering for quality and IBD, our dataset consisted of 258 WB, 134 from Italy and 124 from other European countries, and 109 DP from commercial and local breeds, giving a total of 367 individuals genotyped at 47,809 autosomal SNPs (Table 1).
This dataset (hereafter called 48K) was then pruned for linkage disequilibrium (LD, r2 > 0.5 calculated along sliding windows of 50 SNPs) to delve into overall population structure, leaving 30,218 SNPs (30K).
We further explored WB population structure and composition. In PLINK 1.9 (Chang et al., 2015) we created a dataset including all WB (n = 249) and another dataset including only the Italian WB (n = 125) but excluding Aosta Valley because of its genetic composition highlighted by the first analyses (see section “Results”). Both datasets contained SNPs with a minimum minor allele frequency (MAF) of 0.05, in order to exclude rare alleles which can bias structure analysis, in linkage (LE, r2 < 0.3, windows of 50 SNPs) and Hardy-Weinberg equilibrium (HWE, p-value > 0.001), in order to safely make inferences on neutral loci (Jungerius et al., 2005; Purcell et al., 2007; Benestan et al., 2016), thus resulting in 13,218 SNPs (13K) and 14,155 SNPs (14K), respectively.
Finally, specifically for migration analysis in TREEMIX (see below), WB data were merged with 11 publicly available Sus barbatus (SB), used as outgroup, genotyped with the same SNP chip (Yang et al., 2017). We kept only SNPs with no missing values that were pruned for high LD (r2 > 0.5) in PLINK (see Supplementary Material).
Genetic Variation and Differentiation
Genetic variability in our data (48K) was investigated by computing with PLINK observed (Ho) and expected (He) heterozygosity, minor allele frequency (MAF), and number of polymorphic loci for each geographic region and separately for each Italian WB population. All available genotypes were used, aiming at verifying the real genomic variability within the sampled populations, regardless of the cause of their similarities/dissimilarities (i.e., including possible hybrids).
Subsequently, we assessed levels of genetic differentiation within the Italian populations, and between Italian samples and reference WB and DP populations using the 30K dataset filtered for high LD. Among the different parameters that can be estimated for this purpose, we selected two indexes, a fixation index (pairwise FST) and a measure of allelic differentiation (Jost’s D, Jost, 2008), as they can be considered complementary in comparing allele distributions between populations (see Jost et al., 2018). We used ARLEQUIN v3.5 (Excoffier and Lischer, 2010) for pairwise FST calculations and the R package mmod (Winter, 2012) for Jost’s D. With the same dataset, we also explored the overall genetic structure in our samples by a Principal Component Analysis (PCA) performed in SVS, using all WB and DP together and only WB genotypes.
Then, we focused on the Italian WB, using the 14K dataset and running a Discriminant Analysis of Principal Components (DAPC) in the R package adegenet v2.1.1 (Jombart, 2008; Jombart and Ahmed, 2011); the function find.clusters was used to identify the most likely grouping and a cross-validation approach allowed to determine the best number of Principal Components (PCs) to retain. Furthermore, we evaluated the contribution of different populations and groupings to the overall genomic differentiation among Italian populations performing an Analysis of Molecular Variance (AMOVA; Excoffier et al., 1992) using the R package poppr v2.8.5 (Kamvar et al., 2014, 2015) and the method implemented in R package ade4 (Dray and Dufour, 2007).
Admixture Analyses
Once obtained an overview of the overall genetic variation and local differentiation among Italian samples, we investigated the possible impact of anthropogenic drivers on the observed genetic make-up. Indeed, hybridization with DPs or artificial gene flow with distant populations (i.e., translocations) can generate admixed WB populations, either geographically localized or more widespread in case of high levels of human-mediated gene flow; this latter case can lead to “genetic homogenization” (sensu Olden et al., 2004).
A specific analysis to ascertain possible differences in the local levels of admixture was performed using the software ADMIXTURE v1.2.3 (Alexander et al., 2009). We ran the program with the WB + DP dataset (30K) for K ranging from 1 to 30 and determined the best K according to the lowest cross-validation error (Alexander et al., 2009). At the best K we checked for domestic and extra-Italian genetic components in the Italian populations. As a signature of genomic admixture might appear also in the reference DP breeds, we run the cluster analysis twice, with and without DP, to ascertain whether possible past hybridization events were masked by the variegated nature of some DPs.
Since the interpretation of ADMIXTURE results can be cumbersome (Lawson et al., 2018), on account of the available historical information, we considered the possibility that past introductions of European WB (from multiple sources) to the Italian peninsula had produced a composite genomic make-up, not associable to any of the reference populations. Therefore, we further verified the origin and composition of the Italian populations putatively carrying signs of past admixtures with European WB (see section “Admixture Analyses” in “Results”) using two different approaches. Specifically, we performed a PCA with adegenet on the WB 13K dataset, leaving out the populations that were suspected to be admixed; we subsequently projected their coordinates on the first two axis (i.e., PC1 and PC2) with the function suprow in ade4. This approach eliminates the possible bias in PCA calculation introduced by admixed populations and allows to evaluate their actual origin according to their projection on the PCs estimated from the reference populations (McVean, 2009; see also Smyser et al., 2020).
We also tested for past gene flow from other European populations into one or more Italian WB populations with the program TREEMIX (Pickrell and Pritchard, 2012). The approach used in TREEMIX consists first in drawing a maximum likelihood (ML) tree, and then to add migration edges to verify if the data are better explained by a network-like model. As the program corrects for LD by dividing the whole dataset into blocks of k SNPs (where k is specified by the user), using PLINK we first computed the LD decay as the distance at which r2 < 0.2 (option -r2), and then we obtained the mean number of SNPs in our dataset within that distance with a custom R script. We rooted the ML tree with no migration events on WSE (see Supplementary Material for details) and built the ML tree sampling blocks of 200 SNPs (option -k) and activating the -global option for a global rearrangement after adding all the populations to the tree. We then started to add migration events from 1 to 21 (option -m), supplying at each run the tree with n-1 migration edges (option -g) to save time. We calculated the variance explained by each model thus obtained with the function get_f that comes with the program in an R script; after identifying in m = 5 the knee point (i.e., where the increment of the variance explained started to be less pronounced) we ran 200 bootstrap replicates (option -bootstrap), and then we used the functions provided by Zecca et al. (2020) to visualize the best ML tree and to calculate the migration support (MS) and the extended migration support (MSE) across our replicates for the migration events involving the Italian populations (see Zecca et al., 2020 for the description of the indexes). We finally calculated the f4 statistic with the program fourpop, included in TREEMIX, to formally test for admixture. It requires four populations (A, B, C, and D) and tests if the tree topology (A, B; C, D) is correct; if the Z score is significantly different from zero it means that the history of the four populations is not strictly tree-like (Reich et al., 2009; Pickrell and Pritchard, 2012).
Results
Genomic Diversity
Minor allele frequencies were comparable among the Italian populations (ranging from 0.147 in WCpo to 0.193 in WVao) and appeared slightly lower in Italy than in other European geographic regions (0.169 vs. 0.186–0.194). The number of polymorphic loci varied from the lowest value observed in WCpo (n = 18,544) to the highest in WCal (n = 28,401) for Italy, whereas in the rest of Europe it ranged between n = 26,944 in WIbe and n = 28,598 in WCE (Table 2). Overall, heterozygosity levels were generally higher in DP than in WB. In particular, observed and expected heterozygosity differed significantly, especially in WB, with all Italian populations showing a remarkable deficit of heterozygotes (Wilcoxon test p-value < < 0.001), which was less pronounced in the other WB populations. The lowest values of Ho were observed in WLig and WCpo (0.135 and 0.139, respectively), whilst WSar showed the highest value (0.215, see Table 2).
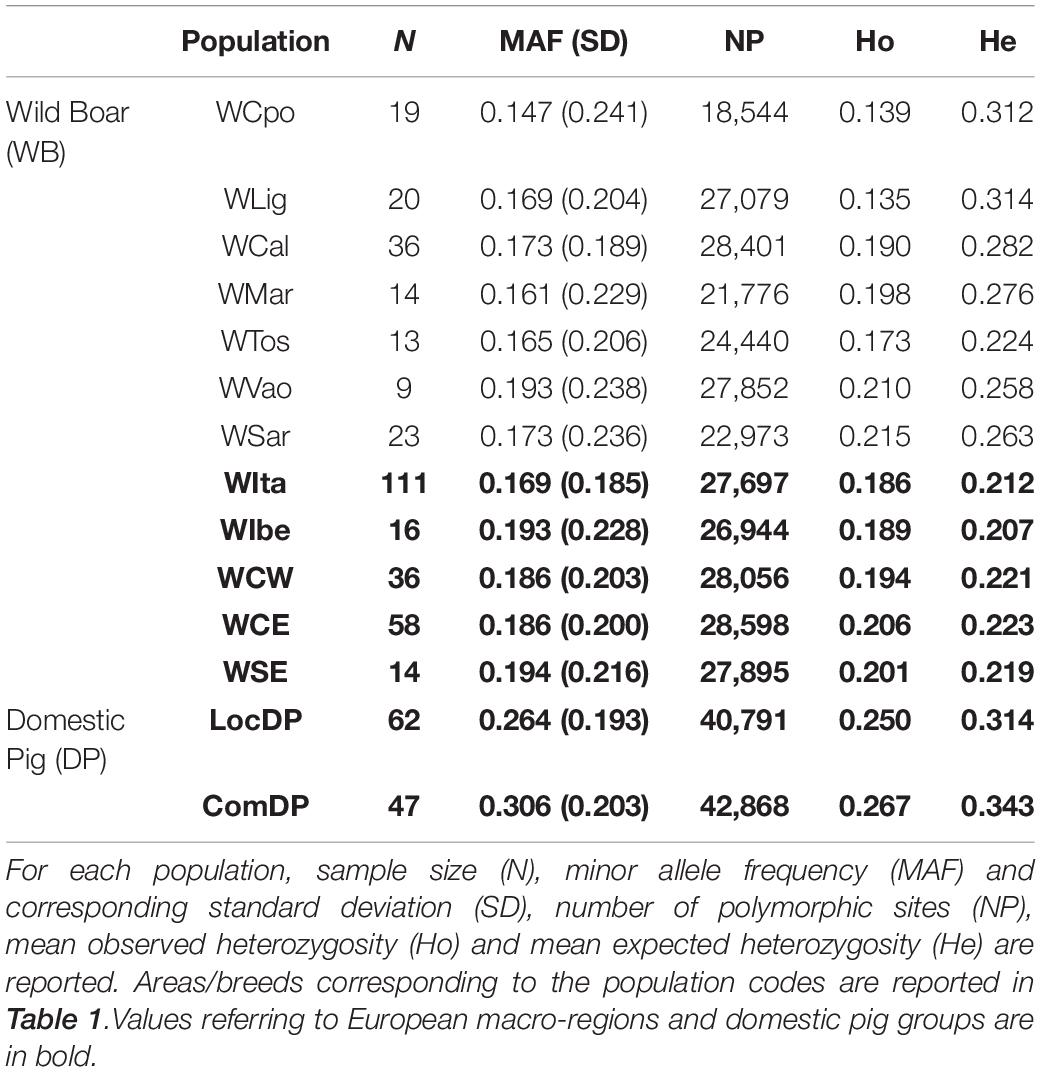
Table 2. Genetic diversity in Italian wild boar populations compared to the reference European macro-regions and domestic pig groups (local and commercial breeds).
Genomic Differentiation
The exploratory PCA clearly separated WB from DP along PC1, and the Italian WB from the European populations along PC2 (Figure 2A). Individuals from the Aosta Valley population (WVao, North Italy) clearly clustered with the European populations (see also ADMIXTURE results). When DP were removed from the analyses, WB population structure became clearer (Figure 2B): the most diverging Italian populations were WCpo and WSar. WMar, WTos, WLig, and WCal clustered in close proximity, but WTos was split into two separate groups, corresponding, respectively, to the western (coastal) and eastern (Apennine) areas in Figure 1. These results are also mirrored by pairwise FST (Figure 3A) and Jost’s D (Supplementary Table 1) values, as well as by the DAPC restricted to the Italian populations (WVao excluded, Figure 3B). The most supported partition of the Italian populations on the basis of BIC values corresponded to four discrete groups (Supplementary Figures 1A,B), specifically: WSar (group 1), WMar (group 2), WCpo (group 3), and WLig-WTos-WCal (group 4). Coherently, the AMOVA showed that this partition was associated with the highest percentage of variance between groups explaining 15.26% of the total variance in the Italian sample (p < 0.001), more than double compared to the percentage of variance between populations within groups (6.88%, Supplementary Table 2).
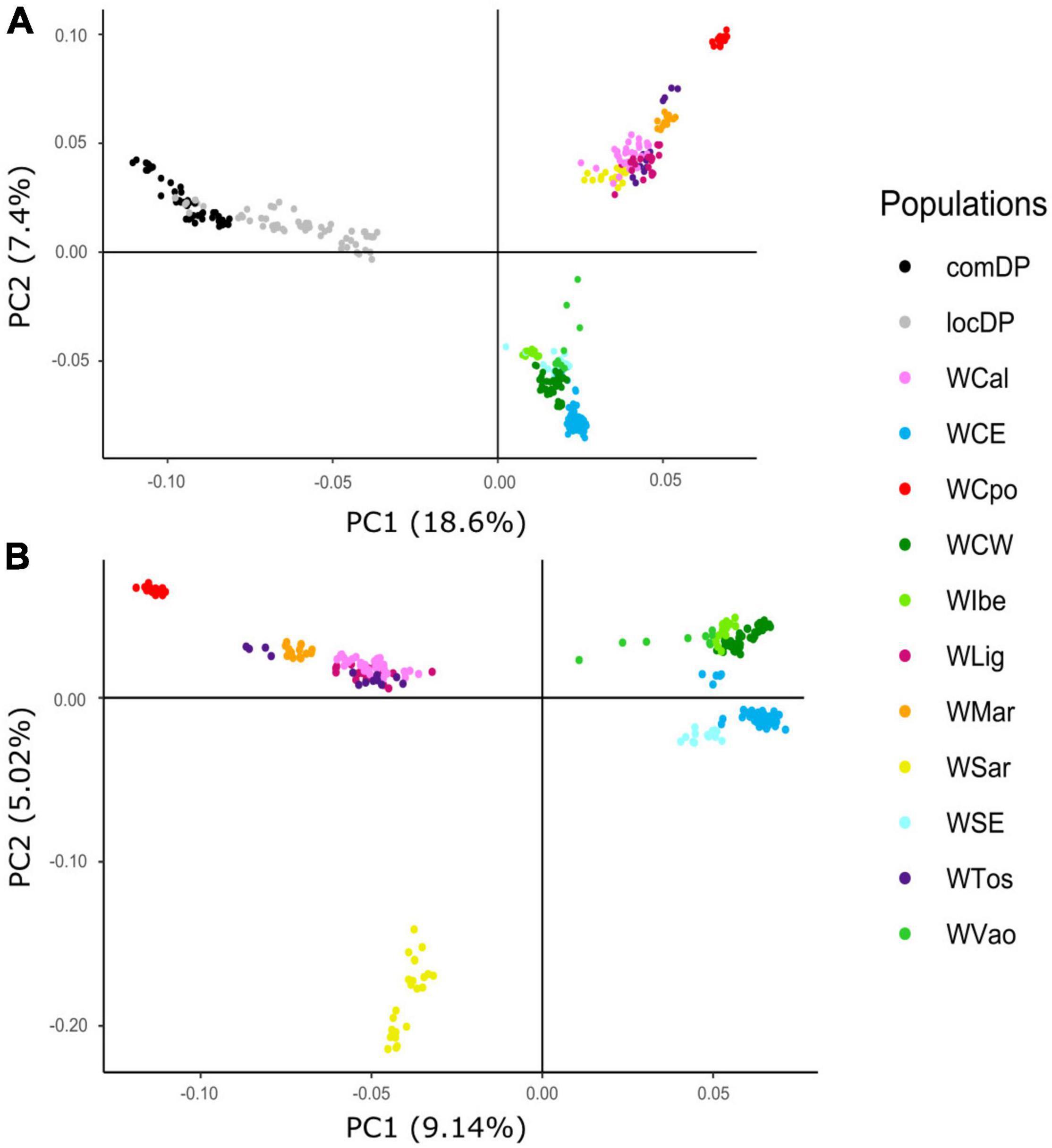
Figure 2. Principal component analysis (PCA) computed using wild boar (WB) and domestic pig (DP) 30K genotypes. Plots show relative genomic distances among sampled individuals in (A) Italian WB, European WB, and local and commercial DP breeds and (B) Italian and European WB only.
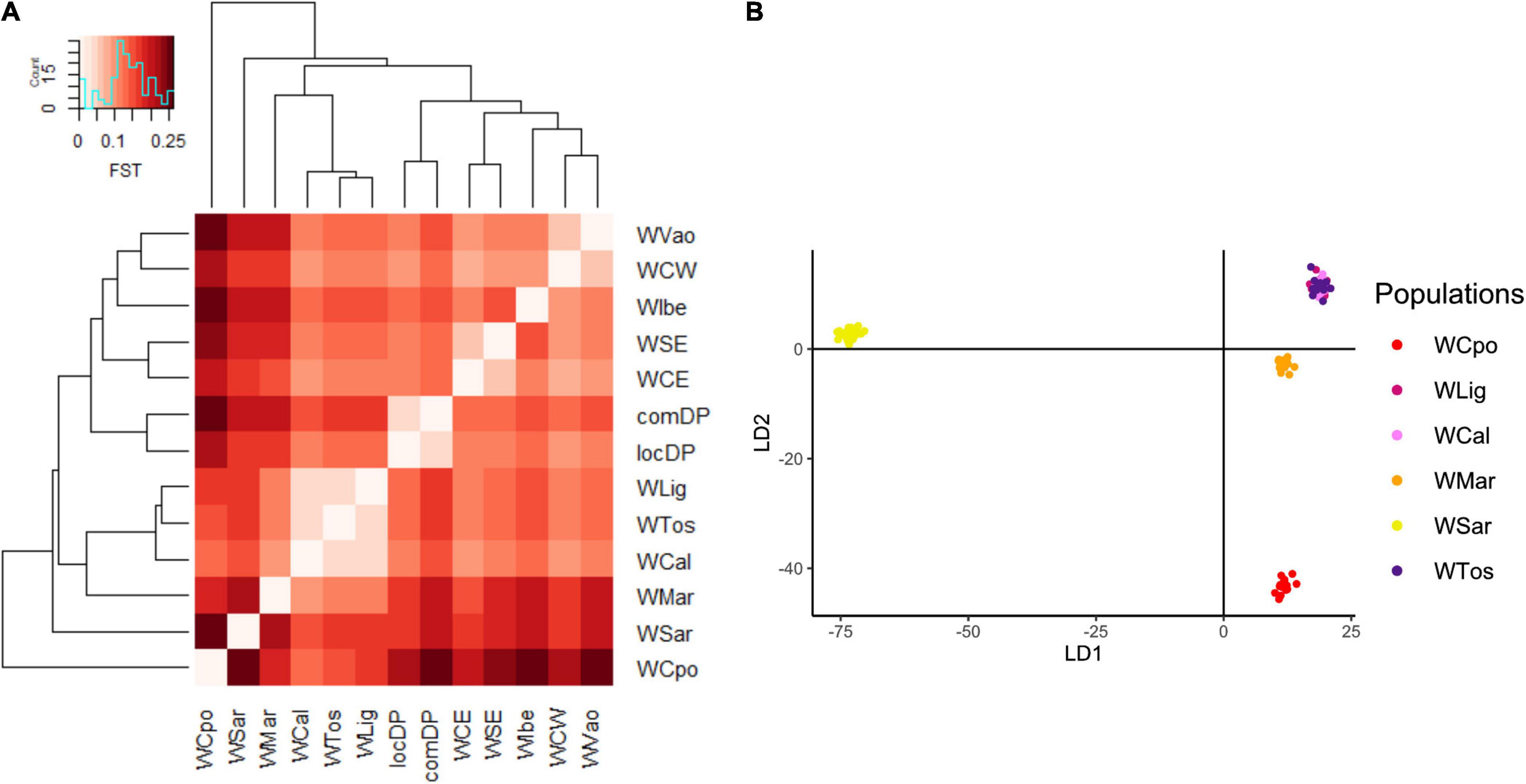
Figure 3. Genomic differentiation among Italian wild boar populations expressed by (A) Pairwise FST heatmap and (B) Discriminant Analysis of Principal Components (DAPC). WVao population was excluded from the DAPC because of its clear assignment to non-Italian populations (Figure 2). The most supported partition is into four groups: WCpo, WMar, WSar and a joint group including WLig, WTos, and WCal. WCpo and WSar represent the populations with the highest genomic divergence.
Admixture Analyses
A contribution to clarify the nature of the identified groupings arose from the admixture analyses. ADMIXTURE results depicted a variegated picture of the Italian populations. The lowest cross validation error was reached at K = 19 but local minima were observed at K = 12 and K = 16 (Figure 4B). At these K values, WVao clearly clustered with the western European populations, consistently with its geographical proximity. In agreement with PCA and FST results, starting from K = 12, the other Italian populations were roughly assigned each to their own clusters, except the group WLig-WCal-WTos (Figure 4A). This group formed a single cluster at K = 12 and was split into two clusters at higher K values. Striking signals of introgression from domestic pigs or from other European populations did not emerged in the Italian samples, with a few exceptions only in WCal and some individuals from WLig and WMar.
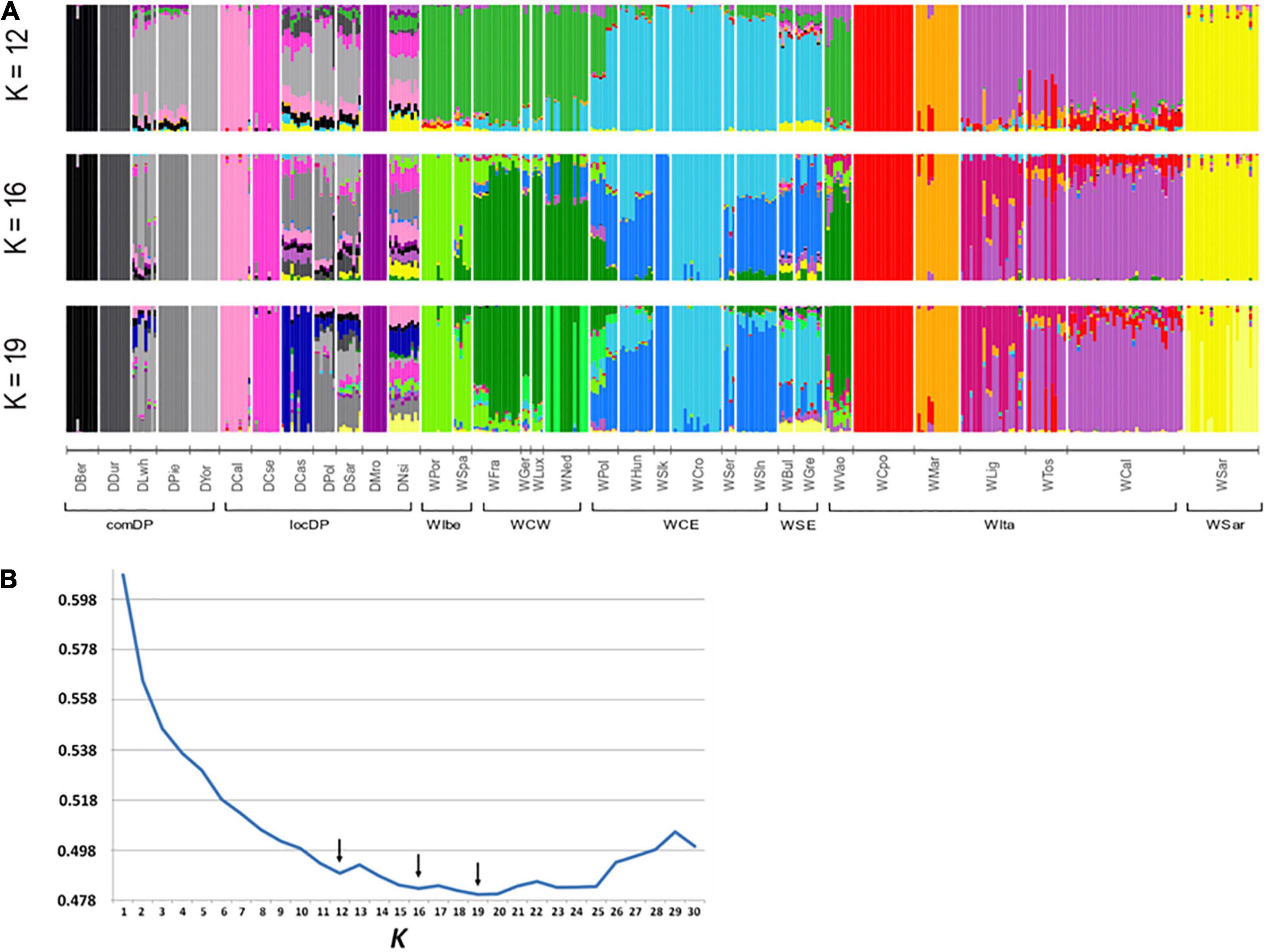
Figure 4. Admixture analyses in wild boar (WB) populations conducted using the software ADMIXTURE. (A) Membership proportions of every genotyped individual to each of the K inferred clusters are shown as different colors. Reference European WB and domestic pig (DP) genotypes are included to test for genomic introgression in Italian WB. Histograms are reported for the best K values indicated by the cross-validation error (B). Arrows in (B) indicate local minima. WB and DP population codes are shown in Table 1.
Based on the results above and on historical information, we hypothesized that WLig, WTos, and WCal appeared to be similar while diverging from other Italian populations, because they were carrying a unique genomic assemblage influenced by a past introgression from non-Italian gene pools (see section “Discussion”). The projected PCA confirmed, indeed, a much higher proximity of the three tested Italian populations to the European clusters (with respect to Figure 2) and a central position in the plots, providing an indirect support to the hypothesis of past admixture events (Figure 5). Like in the previous PCA and DAPC, most of the genotypes from WLig, WTos, and WCal overlapped, confirming their genomic homogeneity despite their geographical location.
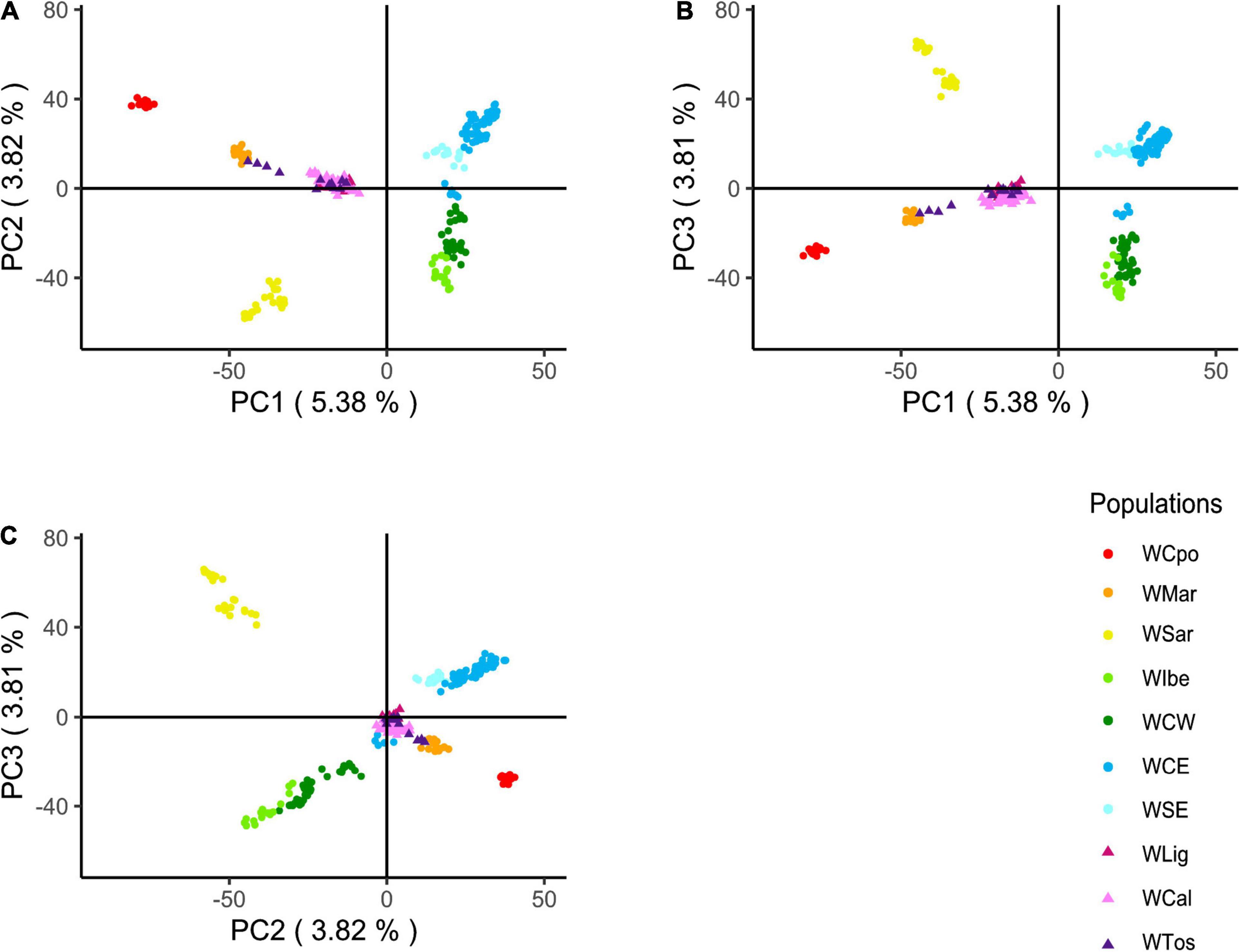
Figure 5. Principal component analysis (PCA) of Italian and European wild boar genotypes. PCA was first calculated excluding the three Italian populations of WLig, WTos, and WCal, whose genotypes were added afterward by projecting their a posteriori estimated coordinates. Plots refer to the following pairwise combinations: PC1 vs. PC2 (A), PC1 vs. PC3 (B), and PC2 vs. PC3 (C).
The ML tree obtained in TREEMIX (Figure 6 and see also Supplementary Figure 2) confirms the overall WB structure. With no migration edges, the ML tree explained 97.7% of the variance. The long branches of WCpo, WMar, and WSar confirmed their high genomic differentiation from the other Italian populations, under the effect of drift. WVao fell in a separate branch and appeared as a sister group to WCW. With five migration edges the percentage of variance explained by the model increased to 99.7%. According to the MS and MSE (Supplementary Tables 3, 4), both WSar and WVao received a migration from populations of central Italy, whereas WCal showed a signature of immigration from the WMar-WCpo lineage. The f4 statistic confirmed that WVao is admixed with the Italian populations (Z > 2, Supplementary Table 5).
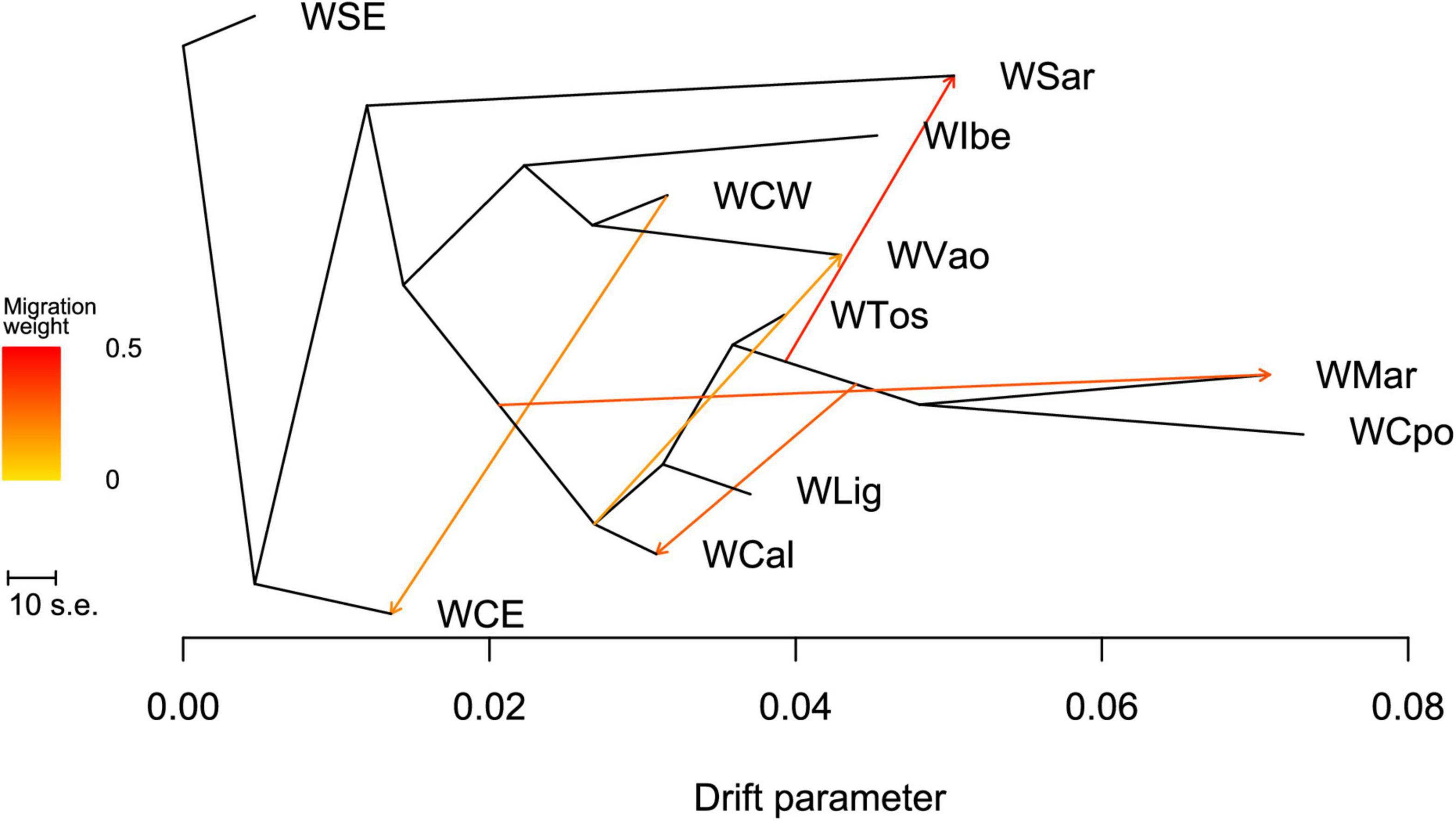
Figure 6. Maximum likelihood tree obtained with TREEMIX describing the relationships among the analyzed wild boar populations sampled in Italy and in the rest of Europe.
Discussion
In the present study, we explored, for the first time, the genome-wide diversity of the Italian WB, analyzing a set of populations sampled from several locations in the Italian peninsula and Sardinia. We expected that the present overall levels of diversity and the genomic make-up of the Italian populations were largely influenced by their demographic histories and human manipulations.
The progressive decline and range contraction of the species in Italy that occurred between the end of the XIX and the beginning of the XX century caused its fragmentation into small isolated demes (Ghigi, 1917, 1950). These nuclei have lasted for decades until the more recent re-expansion, that started after World War II, and have likely suffered bottlenecks accompanied by a loss of genetic variation and changes in allele frequencies. This historical process might explain why the levels of genomic diversity are lower in Italy than in most European populations (Table 2), particularly those recorded in areas that suffered prolonged and strong isolation (e.g., Presidential Estate of Castelporziano).
However, the translocations of wild animals and the release of captive specimens that occurred in the last 60 years, have taken place with no homogeneity throughout the country and have determined a human-mediated gene flow that can be responsible for a fairly higher variation in some zones, where individuals of different origins may have mixed up.
Indeed, for a long time the Italian legislation allowed the release of wild boar for restocking or reintroduction. Yet, since the end of 1980s a ban was introduced, first in single regions and then, only in 2015, at national level.
Genomic Diversity and Differentiation of Italian Wild Boar Populations
Our results on genome-wide heterozygosity levels in WB and DP were consistent with findings from other studies, based on the analyses of similar types and number of genomic markers (Goedbloed et al., 2013b; Alexandri et al., 2017; Iacolina et al., 2018), showing a considerable heterogeneity within and among groups.
However, the low levels of polymorphism we observed in Italian WB, even though comparable with genome-wide values from other European WB populations, should be treated with caution due to the possible ascertainment bias from the applied SNP array. The majority of the SNPs were indeed discovered in commercial pig breeds, and only a pooled set of 31 Central European WB was included in the discovery panel (Ramos et al., 2009). Thus, polymorphisms of the Italian population are likely to be under-represented in the SNPs chip.
The most diverging population turned out to be the one living in the Castelporziano Presidential Estate (WCpo), which was deemed in the past the only pure indigenous nucleus of Italian WB remaining in the peninsula (Oliver et al., 1993). Such a pronounced divergence of this population can be the result of long-lasting isolation and limited effective size, which have likely shaped its allele frequencies by genetic drift. A similar interpretation can be given for WB living in the Maremma Regional Park (WMar), which also showed a certain level of divergence from the other Italian populations, yet lower than WCpo. Similar results had been already found by Vernesi et al. (2003), who included these two populations in their analyses based on microsatellite markers, detecting a certain level of differentiation that was interpreted as a possible expression of two native lineages. These populations were also included in previous phylogeographic analyses (Scandura et al., 2008; Vilaça et al., 2014), which detected a high proportion of endemic diversity both at autosomal microsatellite and mitochondrial D-loop regions. Specifically, Castelporziano samples showed exclusively mtDNA haplotypes belonging to the Italian lineage E2, which were also detected in museum specimens of Maremma WB (Larson et al., 2005). The observed divergence of WCpo and WMar might thus, in principle, be determined also by a different level of introgression with respect to other (Apennine) populations. The artificial selection for “native morphotypes” that was carried out in the Maremma Natural Park in the past might have contributed to the lower introgression of the individuals sampled in this area, while the isolation of WCpo should have prevented any genetic contamination (see Supplementary Material).
All analyses confirmed the high genomic differentiation of Sardinian WB from all mainland populations. This situation had been already described by Iacolina et al. (2016), who were also able to detect a major proximity with the mainland Italy populations by analyzing a larger sample of Sardinian specimens. This was attributed to a likely shared ancestry, but also at least in part to a recent genetic introgression from mainland populations, due to translocations for hunting purposes. Our results are also coherent with recent findings described by Petrelli et al. (2022) who investigated population genomic and selection patterns in WB living on the island.
The uniqueness of Castelporziano and Sardinian WB had been detected also in a study using microsatellite loci to assess the differentiation among European WB populations (Veličković et al., 2016). Both populations showed significant evidences of past bottlenecks, thus calling into question the main role of genetic drift in determining their differentiation.
The Aosta Valley population clearly showed a different make-up from the other Italian samples. It clustered with Central-Western European populations (WCW), which is consistent with an origin by range expansion from neighboring areas, as known for the Piedmont population (De Beaux and Festa, 1927; Monaco et al., 2007) and suggested by spatial information on the recolonization process in the region (Peracino and Bassano, 1995). This result, however, contrasts with the presumed release of captive hybrid stocks in the region (Apollonio et al., 2010b). Our genomic data thus suggest that natural processes (i.e., expansion from surrounding areas) have prevailed over human-mediated causes (i.e., releases).
An outstanding unexpected outcome of our study was represented by the mismatch between genetic and spatial distance we found. Wild boar from WTos, WLig, and WCal populations showed indeed a high similarity in all the analyses conducted, despite their geographical position. These populations are likely to represent the most manipulated WB populations in our sample. Their genomic composition could be affected by a number of human interventions (reintroductions, translocations) that have mixed up and spread an admixed gene pool, appearing as a separate cluster in ADMIXTURE analyses. This interpretation is confirmed by the central position of these three WB populations in the projected PCA (Figure 5). Within the WTos population, we found a different genetic composition between localities: a group of individuals sampled in the San Rossore estate, on the Tyrrhenian coast, slightly deviated from the other WTos individuals from eastern Tuscany and also from the nearby WLig population, showing similarities with individuals from WMar (Figures 2, 5). According to its history, this coastal nucleus has probably a higher proportion of native variation than the other individuals sampled in northern Tuscany (see Supplementary Material).
Genomic Introgression in Italian Wild Boar Populations
Despite human intervention has to be invoked to interpret the observed patterns of genomic variation in Italy, the detected signals of admixture with DP and non-Italian WB in the sampled populations were lower than expected based on prior knowledge (Oliver et al., 1993; Apollonio et al., 2010b). Both ADMIXTURE and f3 analyses did not show relevant signals of introgression from external sources into the Italian populations, contrasting with patterns emerging from other studies based on microsatellite and MC1R analyses (Vernesi et al., 2003; Scandura et al., 2008, 2011; Canu et al., 2016).
Despite close proximity of WTos, WLig, and WCal to European populations in the projected PCA, only weak signatures of introgression from other European macro-areas were detected by ADMIXTURE and f3 analyses. Two possible explanations could be proposed for our findings: (1) introgression from multiple European WB and DP populations was relevant but it occurred many generations ago and was followed by a local divergence of the admixed gene pool from all parental populations; (2) releases of WB of foreign origin or WB × DP hybrids took place locally but involved a limited number of individuals, which were outnumbered by translocated individuals of Italian origin and/or by naturally expanding native populations (so non-native alleles were diluted by the native ones). In the former situation, the novel gene pool would appear as a different exclusive cluster in the ADMIXTURE analyses (see Figure 4A, K = 12), as it would not be possible to track back the contribution of the parental populations. In the second case, the prevailing native origin of the individuals would explain the observed lack of introgression; however, the origin by translocation from multiple areas in Italy would still generate an admixed and unique gene pool.
Historical information available for our sampling areas (see Supplementary Material) and results of f3 analyses support the second line of interpretation, considering the expansion of remnant native populations sustained by human restocking with native boars, as main drivers of WB recovery in the peninsula. Both past and more recent translocations in fact, although partly undocumented, were carried out using free-ranging or captive animals from central Italy, that derived in large part from the Tyrrhenian populations (like Castelporziano estate or Maremma).
The low rate of introgression from DP detected in this study is consistent with previous findings. Using the same set of markers, no hybrid was indeed detected by Iacolina et al. (2018) in a subsample of 19 Italian WB (from Tuscany) among those considered in the present study, whereas 3 hybrids out of 25 individuals (12%) were detected in a random sample of Sardinian WB. Conversely, using a multiple-marker approach (coat color, MC1R, SNPs), (Petrelli et al., 2022) found a complete overlap among the genomes of Southern Italian known hybrids with those of the sympatric WB, likely as a result of deep introgression into the latter.
Interestingly, though in the present work signals of human-caused introgression were not expected for WSar, as the used Sardinian genotypes had been selected among the less introgressed individuals detected in a previous study focused on the insular population (Iacolina et al., 2016), f3 analyses detected a migration from mainland WB to Sardinia (Figure 6), thus supporting a recent gene flow.
Evidence of a recent introgression from mainland Italy, as well as from DPs, had been also detected by microsatellite analyses (Scandura et al., 2011), while reciprocal introgression with Sardinian DPs was proven by the analysis of allelic variation at nuclear genes (MC1R and NR6A1, Canu et al., 2016).
Conclusion
Despite the prevalent opinion among hunters and managers depicted the native Italian WB as almost genetically extinct, the present study reveals that Italian populations may still maintain a high proportion of native genomic diversity. The strong divergence that Italian populations showed from all other European WB and from DPs, as well as the occurrence of an exclusive mtDNA lineage, are clear indicators of a retained endemic variation.
Our results suggest that the impact of long-term natural processes (such as Quaternary climatic fluctuations, range expansions, natural selection), as well as the prolonged geographic isolation of Italy determined by the Alps and the Mediterranean Sea, might still affect the phylogeographic structure of a number of species, prevailing over the effects of human manipulations in shaping WB genetic diversity patterns. Similar results were recently found in a genomic study on managed populations of red-legged partridges (Alectoris rufa, Forcina et al., 2021).
The most preserved Italian WB populations appear to occur along the central Tyrrhenian coast of the peninsula (WCpo and WMar). The high genomic differentiation of these WB populations, together with their morphometric uniqueness (Apollonio et al., 1988; Tinelli et al., 1999), would call for a re-evaluation of the subspecies Sus scrofa majori (De Beaux and Festa, 1927), as recently already proposed for other highly differentiated Italian mammal populations, such as the Mesola red deer (Cervus elaphus italicus; Zachos et al., 2014), the Italian roe deer (Capreolus capreolus italicus; Randi et al., 2004), and the Apennine wolf (Canis lupus italicus; Montana et al., 2017).
However, the Italian WB populations we investigated, yet contributing to the overall genetic variation of the Italian WB, represent only part of it. Populations of north-eastern Italy, not included in the present study, showed a different composition from those living in central Italy, revealing higher similarities with eastern European populations (Scandura et al., 2008). Similarly, in southern Italy, a highly detectable rate of introgression from DPs was found in WB living north to WCal population (Cilento area), where a high morphological variation was also described (Fulgione et al., 2016; Petrelli et al., 2022). Unfortunately, no genetic nor phenotypical information is instead available for reintroduced WB that are spreading across Sicily, as well as for other areas of more recent recolonization.
It is therefore evident that the Italian WB population represents a strongly heterogeneous assemblage of demes with different histories and genomic make-up, determined by a combination of human-mediated factors such as reintroductions, translocations, restocking activities with farmed (sometimes hybrid) individuals, range fragmentation and demographic events, resulting in a genomic heterogeneity much higher than in any other European region.
Evidence from the present work clearly acknowledges the endemic value of Italian WB native genomic diversity. Thus our findings strongly recommend its conservation (a) by preventing further admixture among WB populations, strictly regulating WB farming and permanently banning wild boar import (as currently regulated due to sanitary reasons, i.e., African Swine Fever prevention), as well as (b) by minimizing domestic introgression by carefully preventing the interaction with pig breeds, especially when raised in a semi-natural state, and with released captive wild boar stocks, possibly deliberately introgressed by domestic swine.
Future studies based on whole genome sequencing could contribute to definitively clarifying the evolutionary histories and divergence timing of Italian WB populations through the analyses of demographic trajectories and past gene flow patterns with other European wild populations and domestic breeds. Paleogenomic and ancient DNA approaches could additionally help assess how much of the current diversity in WB local stocks can be ascribed to a native gene pool comparing modern and historical samples (i.e., museum specimens or stuffed trophies) dated before 1950, thus prior to most human-mediated gene flow.
Moreover whole-genome and transcriptome analyses could help identify further evidences of genetic differentiation and adaptive variation in Italian WB, which could not be detected by the Porcine SNP60 chip due to limitations in its development (not including Italian animals). Such additional genomic regions could allow us to investigate possible selection or adaptive patterns in Italian WB populations, potentially hosting genes responsible for their local adaptation to the Mediterranean environment, and, theoretically, for their high phenotypic divergence. Finally, the availability of entire genomes could also shed lights on the long-term evolutionary potential of Italian WB populations, their ability to actively face the ongoing climate changes and their future ecological role in the Anthropocene.
Data Availability Statement
The original contributions presented in the study are publicly available. This data can be found here: doi: 10.5061/dryad.hmgqnk9jm.
Ethics Statement
Ethical review and approval was not required for this study in accordance with the local legislation and institutional requirements.
Author Contributions
MS, NM, and MA conceived the study. NM, CM, and LI contributed to sampling and lab analyses. GF, RC, FM, GP, and MS performed the data analyses. MS, GF, RC, FM, and NM wrote the manuscript. All authors contributed to the discussion of results, manuscript revision, read, and approved the submitted version.
Funding
This research was supported by the Ministry of Environment, Pollino National Park, the program FSC 2014-2020 – “Patto per lo Sviluppo della Regione Sardegna” (project RC_CRP_013), and the program “Fondo di Ateneo per la Ricerca 2019.” GF had a Ph.D. grant funded by the European Social Fund – Operational Program 2014/2020 (POR FSE 2014/2020).
Conflict of Interest
The authors declare that the research was conducted in the absence of any commercial or financial relationships that could be construed as a potential conflict of interest.
Publisher’s Note
All claims expressed in this article are solely those of the authors and do not necessarily represent those of their affiliated organizations, or those of the publisher, the editors and the reviewers. Any product that may be evaluated in this article, or claim that may be made by its manufacturer, is not guaranteed or endorsed by the publisher.
Acknowledgments
For the collection of biological samples we are grateful to B. Franzetti (Castelporziano), E. Landini (Liguria), the Istituto Zooprofilattico Sperimentale del Piemonte, Liguria e Valle d’Aosta (Valle d’Aosta), A. Sforzi and Maremma Natural Park (Maremma), Pollino National Park (Pollino), the staff of the San Rossore Estate, and the hunting groups of Chitignano (Tuscany). We thank G. Zecca for useful suggestions on data analyses and two reviewers for their valuable comments.
Supplementary Material
The Supplementary Material for this article can be found online at: https://www.frontiersin.org/articles/10.3389/fevo.2022.833081/full#supplementary-material
Footnotes
References
Alexander, D. H., Novembre, J., and Lange, K. (2009). Fast model-based estimation of ancestry in unrelated individuals. Genome Res. 19, 1655–1664. doi: 10.1101/gr.094052.109
Alexandri, P., Megens, H.-J., Crooijmans, R. P. M. A., Groenen, M. A. M., Goedbloed, D. J., Herrero-Medrano, J. M., et al. (2017). Distinguishing migration events of different timing for wild boar in the Balkans. J. Biogeog. 44, 259–270. doi: 10.1111/jbi.12861
Allendorf, F. W., England, P. R., Luikart, G., Ritchie, P. A., and Ryman, N. (2008). Genetic effects of harvest on wild animal populations. Trends Ecol. Evol. 23, 327–337. doi: 10.1016/j.tree.2008.02.008
Apollonio, M., Andersen, R., and Putman, R. (2010a). European Ungulates and Their Management in the 21st century. Cambridge: Cambidge University Press.
Apollonio, M., Ciuti, S., Pedrotti, L., and Banti, P. (2010b). “Ungulates and their management in Italy,” in European Ungulates and Their Management in the 21st century, eds M. Apollonio, R. Andersen, and R. Putman (Cambridge, UK: Cambridge University Press), 475–506.
Apollonio, M., Randi, E., and Toso, S. (1988). The systematics of the wild boar (Sus scrofa L.) in Italy. Boll. Zool. 3, 213–221.
Apollonio, M., Scandura, M., and Sprem, N. (2014). “Reintroductions as a Management tool for European Ungulates,” in Behaviour and Management of European Ungulates, eds R. Putman and M. Apollonio (Dunbeath: Whittles Publishing), 46–77.
Benestan, L. M., Ferchaud, A. L., Hohenlohe, P. A., Garner, B. A., Naylor, G. J., Baums, I. B., et al. (2016). Conservation genomics of natural and managed populations: building a conceptual and practical framework. Mol. Ecol. 25, 2967–2977. doi: 10.1111/mec.13647
Biosa, D., Scandura, M., Tagliavini, J., Luccarini, S., Mattioli, L., and Apollonio, M. (2015). Patterns of genetic admixture between roe deer of different origin in central Italy. J. Mammal. 96, 827–838. doi: 10.1093/jmammal/gyv098
Boyce, W. M., Weisenberger, M. E., Penedo, M. C. T., and Johnson, C. K. (2011). Wildlife translocation: the conservation implications of pathogen exposure and genetic heterozygosity. BMC Ecol. 11:5. doi: 10.1186/1472-6785-11-5
Canu, A., Apollonio, M., and Scandura, M. (2018). Unmasking the invader: genetic identity of invasive wild boar from three minor islands off Sardinia (Italy). Mamm. Biol. 93, 29–37. doi: 10.1016/j.mambio.2018.07.008
Canu, A., Vilaça, S. T., Iacolina, L., Apollonio, M., Bertorelle, G., and Scandura, M. (2016). Lack of Polymorphism at the MC1R Wild-Type Allele and Evidence of Domestic Allele Introgression across European Wild Boar Populations. Mamm. Biol. 81, 477–479. doi: 10.1016/j.mambio.2016.01.003
Chang, C. C., Chow, C. C., Tellier, L. C., Vattikuti, S., Purcell, S. M., and Lee, J. J. (2015). Second-generation PLINK: rising to the challenge of larger and richer datasets. GigaScience 4:7. doi: 10.1186/s13742-015-0047-8
Chapron, G., Kaczensky, P., Linnell, J. D. C., Arx, M., von Huber, D., Andrén, H., et al. (2014). Recovery of large carnivores in Europe’s modern human-dominated landscapes. Science 346, 1517–1519. doi: 10.1126/science.1257553
De Beaux, O., and Festa, E. (1927). La ricomparsa del Cinghiale nell’Italia settentrionale. Mem. Soc. Ital. Scie. Nat. Mus. Civ. Sto. Nat. Milano 9, 265–342.
Dellicour, S., Frantz, A. C., Colyn, M., Bertouille, S., Chaumont, F., and Flamand, M. C. (2011). Population structure and genetic diversity of red deer (Cervus elaphus) in forest fragments in north-western France. Conserv. Genet. 12, 1287–1297. doi: 10.1007/s10592-011-0230-0
Dray, S., and Dufour, A. B. (2007). The ade4 Package: implementing the Duality Diagram for Ecologists. J. Stat. Softw. 22, 1–20. doi: 10.18637/jss.v022.i04
Excoffier, L., and Lischer, H. E. L. (2010). Arlequin suite ver 3.5: a new series of programs to perform population genetics analyses under Linux and Windows. Mol. Ecol. Res. 10, 564–567. doi: 10.1111/j.1755-0998.2010.02847.x
Excoffier, L., Smouse, P. E., and Quattro, J. M. (1992). Analysis of molecular variance inferred from metric distances among DNA haplotypes: application to human mitochondrial DNA restriction data. Genetics 131, 479–491. doi: 10.1093/genetics/131.2.479
Forcina, G., Tang, Q., Cros, E., Guerrini, M., Rheindt, F. E., and Barbanera, F. (2021). Genome-wide markers redeem the lost identity of a heavily managed gamebird. Proc. Roy. Soc. B. 288:20210285. doi: 10.1098/rspb.2021.0285
Frantz, A. C., Pourtois, J. T., Heuertz, M., Schley, L., Flamand, M. C., Krier, A., et al. (2006). Genetic structure and assignment tests demonstrate illegal translocation of red deer (Cervus elaphus) into a continuous population. Mol. Ecol. 15, 3191–3203. doi: 10.1111/j.1365-294X.2006.03022.x
Fulgione, D., Rippa, D., Buglione, M., Trapanese, M., Petrelli, S., and Maselli, V. (2016). Unexpected but Welcome. Evol. Appl. 9, 769–776. doi: 10.1111/eva.12383
Ghigi, A. (1911). Ricerche faunistiche e sistematiche sui Mammiferi d’Italia che formano oggetto. Caccia. Natura. II, 289–337.
Ghigi, A. (1917). I Mammiferi d’Italia considerati nei loro rapporti con l’agricoltura. Natura VIII, 85–137.
Ghigi, A. (1950). La Vita Degli animali, II Mammiferi ed Uccelli Sedentari Delle Terre Continentali. Mlano: UTET.
Goedbloed, D. J., Megens, H. J., Van Hooft, P., Herrero-Medrano, J. M., Lutz, W., Alexandri, P., et al. (2013a). Genome-wide single nucleotide polymorphism analysis reveals recent genetic introgression from domestic pigs into Northwest European wild boar populations. Mol. Ecol. 22, 856–866. doi: 10.1111/j.1365-294X.2012.05670.x
Goedbloed, D. J., van Hooft, P., Megens, H.-J., Langenbeck, K., Lutz, W., Crooijmans, R. P., et al. (2013b). Reintroductions and genetic introgression from domestic pigs have shaped the genetic population structure of Northwest European wild boar. BMC Genet. 14:43. doi: 10.1186/1471-2156-14-43
Hindrikson, M., Remm, J., Männil, P., Ozolins, J., Tammeleht, E., and Saarma, U. (2013). Spatial Genetic Analyses Reveal Cryptic Population Structure and Migration Patterns in a Continuously Harvested Grey Wolf (Canis lupus) Population in North-Eastern Europe. PLoS One 8:e75765. doi: 10.1371/journal.pone.0075765
Iacolina, L., Pertoldi, C., Amills, M., Kusza, S., Megens, H.-J., Bâlteanu, V. A., et al. (2018). Hotspots of Recent Hybridization between Pigs and Wild Boars in Europe. Sci. Rep. 8:17372. doi: 10.1038/s41598-018-35865-8
Iacolina, L., Scandura, M., Goedbloed, D. J., Alexandri, P., Crooijmans, R. P. M. A., Larson, G., et al. (2016). Genomic diversity and differentiation of a managed island wild boar population. Heredity 116, 60–67. doi: 10.1038/hdy.2015.70
Jombart, T. (2008). adegenet: a R package for the multivariate analysis of genetic markers. Bioinformatics 24, 1403–1405. doi: 10.1093/bioinformatics/btn129
Jombart, T., and Ahmed, I. (2011). adegenet 1.3-1: new tools for the analysis of genome-wide SNP data. Bioinformatics 27, 3070–3071. doi: 10.1093/bioinformatics/btr521
Jost, L. (2008). GST and its relatives do not measure differentiation. Mol. Ecol. 17, 4015–4026. doi: 10.1111/j.1365-294X.2008.03887.x
Jost, L., Archer, F., Flanagan, S., Gaggiotti, O., Hoban, S., and Latch, E. (2018). Differentiation measures for conservation genetics. Evol. Appl. 11, 1139–1148. doi: 10.1111/eva.12590
Jungerius, B. J., Gu, J., Crooijmans, R. P., der Poelm, J. J., Groenen, M. A., van Oost, B. A., et al. (2005). Estimation of the extent of linkage disequilibrium in seven regions of the porcine genome. Anim. Biotechnol. 16, 41–54. doi: 10.1081/ABIO-200053402
Kamvar, Z. N., Brooks, J. C., and Grünwald, N. J. (2015). Novel R tools for analysis of genome-wide population genetic data with emphasis on clonality. Front. Genet. 6:208. doi: 10.3389/fgene.2015.00208
Kamvar, Z. N., Tabima, J. F., and Grünwald, N. J. (2014). Poppr: an R package for genetic analysis of populations with clonal, partially clonal, and/or sexual reproduction. PeerJ 2:e281. doi: 10.7717/peerj.281
Khederzadeh, S., Kusza, S., Huang, C.-P., Markov, N., Scandura, M., Babaev, E., et al. (2019). Maternal genomic variability of the wild boar (Sus scrofa) reveals the uniqueness of East-Caucasian and Central Italian populations. Ecol. Evol. 9, 9467–9478. doi: 10.1002/ece3.5415
Laikre, L., Schwartz, M. K., Waples, R. S., and Ryman, N. (2010). Compromising genetic diversity in the wild: unmonitored large-scale release of plants and animals. Trends Ecol. Evol. 25, 520–529. doi: 10.1016/j.tree.2010.06.013
Larson, G., Dobney, K., Albarella, U., Fang, M., Matisoo-Smith, E., Robins, J., et al. (2005). Worldwide Phylogeography of Wild Boar Reveals Multiple Centers of Pig Domestication. Science 307, 1618–1621. doi: 10.1126/science.1106927
Lawson, D. J., van Dorp, L., and Falush, D. (2018). A Tutorial on How Not to Over-Interpret STRUCTURE and ADMIXTURE Bar Plots. Nat. Comm. 9:3258. doi: 10.1038/s41467-018-05257-7
Lepri, G. (1911). Aggiunte alle “Ricerche faunistiche e sistematichesui Mammiferi d’Italia che formano oggetto di caccia” del prof. A. Ghigi. Boll. Soc. Zool. Ital. Series II XII, 241–250.
Manunza, A., Zidi, A., Yeghoyan, S., Balteanu, V. A., Carsai, T. C., Scherbakov, O., et al. (2013). A High Throughput Genotyping Approach Reveals Distinctive Autosomal Genetic Signatures for European and Near Eastern Wild Boar. PLoS One 8:e55891. doi: 10.1371/journal.pone.0055891
Martin, J.-L., Chamaillé-Jammes, S., and Waller, D. M. (2020). Deer, wolves, and people: costs, benefits and challenges of living together. Biol. Rev. 95, 782–801. doi: 10.1111/brv.12587
Maselli, V., Rippa, D., Deluca, A., Larson, G., Wilkens, B., Linderholm, A., et al. (2016). Southern Italian wild boar population, hotspot of genetic diversity. Hystrix 27, 137–144. doi: 10.4404/hystrix-27.2-11489
Massei, G., Kindberg, J., Licoppe, A., Gačić, D., Šprem, N., Kamler, J., et al. (2015). Wild boar populations up, numbers of hunters down? A review of trends and implications for Europe. Pest Manag. Sci. 71, 492–500. doi: 10.1002/ps.3965
McVean, G. (2009). A genealogical interpretation of principal components analysis. PLoS Genet. 5:e1000686. doi: 10.1371/journal.pgen.1000686
Monaco, A., Carnevali, L., Riga, F., and Toso, S. (2007). “Il cinghiale sull’arco alpino: status e gestione delle popolazioni,” in Il Cinghiale sull’arco alpino: status e gestione, eds H. C. Hauffe, B. Crestanello, and A. Monaco (Italy: Centro di Ecologia Alpina), 5–23. doi: 10.1007/bf02906453
Montana, L., Caniglia, R., Galaverni, M., Fabbri, E., and Randi, E. (2017). A new mitochondrial haplotype confirms the distinctiveness of the Italian wolf (Canis lupus) population. Mamm. Biol. 84, 30–34. doi: 10.1016/j.mambio.2017.01.005
Olano-Marin, J., Plis, K., Sönnichsen, L., Borowik, T., Niedziałkowska, M., and Jędrzejewska, B. (2014). Weak Population Structure in European Roe Deer (Capreolus capreolus) and Evidence of Introgressive Hybridization with Siberian Roe Deer (C. pygargus) in Northeastern Poland. PLoS One 9:e109147. doi: 10.1371/journal.pone.0109147
Olden, J. D., Leroy Poff, N., Douglas, M. R., Douglas, M. E., and Fausch, K. D. (2004). Ecological and evolutionary consequences of biotic homogenization. Trends Ecol. Evol. 19, 18–24. doi: 10.1016/j.tree.2003.09.010
Oliver, W. L. R., Brisbin, L. I., and Takahashi, S. (1993). “The Eurasian wild pig, Sus scrofa,” in Pigs, Peccaries and Hippos?: Status Survey and Conservation Action Plan, ed. W. L. R. Oliver (Switzerland: IUCN), 112–121.
Peracino, V., and Bassano, B. (1995). The wild boar (Sus scrofa) in the Gran Paradiso National Park (Italy): presence and distribution. Ibex J. Mount. Ecol. 3, 145–146.
Petrelli, S., Buglione, M., Maselli, V., Troiano, C., Larson, G., Frantz, L., et al. (2022). Population genomic, olfactory, dietary, and gut microbiota analyses demonstrate the unique evolutionary trajectory of feral pigs. Mol. Ecol. 31, 220–237. doi: 10.1111/mec.16238
Pickrell, J. K., and Pritchard, J. K. (2012). Inference of Population Splits and Mixtures from Genome-Wide Allele Frequency Data. PLoS Genet. 8:e1002967. doi: 10.1371/journal.pgen.1002967
Purcell, S., Neale, B., Todd-Brown, K., Thomas, L., Ferreira, M. A. R., Bender, D., et al. (2007). PLINK:a Tool Set for Whole-Genome Association and Population-Based Linkage Analyses. Am. J. Hum. Genet. 81, 559–575. doi: 10.1086/519795
Ramos, A. M., Crooijmans, R. P. M. A., Affara, N. A., Amaral, A. J., Archibald, A. L., Beever, J. E., et al. (2009). Design of a high density SNP genotyping assay in the pig using SNPs identified and characterized by next generation sequencing technology. PLoS One 4:e6524. doi: 10.1371/journal.pone.0006524
Randi, E., Alves, P. C., Carranza, J., Milosevic-Zlatanovi, S., Sfougaris, A., and Mucci, N. (2004). Phylogeography of roe deer (Capreolus capreolus) populations: the effectsof historical genetic subdivisions and recent nonequilibrium dynamics. Mol. Ecol. 13, 3071–3083. doi: 10.1111/j.1365-294X.2004.02279.x
Reich, D., Thangaraj, K., Patterson, N., Price, A. L., and Singh, L. (2009). Reconstructing Indian population history. Nature 461, 489–494. doi: 10.1038/nature08365
Scandura, M., Iacolina, L., Cossu, A., and Apollonio, M. (2011). Effects of human perturbation on the genetic make-up of an island population: the case of the Sardinian wild boar. Heredity 106, 1012–1020. doi: 10.1038/hdy.2010.155
Scandura, M., Iacolina, L., Crestanello, B., Pecchioli, E., Bertorelle, G., and Apollonio, M. (2009). “Occurrence of a private mtDNA clade in Italian wild boars,” in Proceedings of the 7th International Symposium on Wild Boar (Sus scrofa) and on sub-order Suiformes, eds A. Nahlik and T. Tari (Hungary: Lover), 96–97.
Scandura, M., Iacolina, L., Crestanello, B., Pecchioli, E., Di Benedetto, M. F., Russo, V., et al. (2008). Ancient vs. recent processes as factors shaping the genetic variation of the European wild boar: are the effects of the last glaciation still detectable? Mol. Ecol. 17, 1745–1762. doi: 10.1111/j.1365-294X.2008.03703.x
Silva, P., López-Bao, J. V., Llaneza, L., Álvares, F., Lopes, S., Blanco, J. C., et al. (2018). Cryptic population structure reveals low dispersal in Iberian wolves. Sci. Rep. 8:14108. doi: 10.1038/s41598-018-32369-3
Smyser, T. J., Tabak, M. A., Slootmaker, C., Robeson, M. S., Miller, R. S., Bosse, M., et al. (2020). Mixed ancestry from wild and domestic lineages contributes to the rapid expansion of invasive feral swine. Mol. Ecol. 29, 1103–1119. doi: 10.1111/mec.15392
Tinelli, A., Pietrelli, L., and Focardi, S. (1999). Dati biometrici della popolazione autoctona di cinghiale (Sus scrofa L.) di Castelporziano. Proc. Soc. Ital. Sc. Nat. Mus.Civ. Sto. Nat. Milano 2, 171–177.
Veličković, N., Ferreira, E., Djan, M., Ernst, M., Obreht Vidaković, D., Monaco, A., et al. (2016). Demographic history, current expansion and future management challenges of wild boar populations in the Balkans and Europe. Heredity 117, 348–357. doi: 10.1038/hdy.2016.53
Vernesi, C., Crestanello, B., Pecchioli, E., Tartari, D., Caramelli, D., Hauffe, H., et al. (2003). The genetic impact of demographic decline and reintroduction in the wild boar (Sus scrofa):a microsatellite analysis. Mol. Ecol. 12, 585–595. doi: 10.1046/j.1365-294X.2003.01763.x
Vilaça, S. T., Biosa, D., Zachos, F., Iacolina, L., Kirschning, J., Alves, P. C., et al. (2014). Mitochondrial phylogeography of the European wild boar: the effect of climate on genetic diversity and spatial lineage sorting across Europe. J. Biogeog. 41, 987–998. doi: 10.1111/jbi.12268
Winter, D. J. (2012). mmod: an R library for the calculation of population differentiation statistics. Mol. Ecol. Res. 12, 1158–1160. doi: 10.1111/j.1755-0998.2012.03174.x
Yang, B., Cui, L., Perez-Enciso, M., Traspov, A., Crooijmans, R. P. M. A., Zinovieva, N., et al. (2017). Genome-wide SNP data unveils the globalization of domesticated pigs. Genet. Sel. Evol. 49:71. doi: 10.1186/s12711-017-0345-y
Zachos, F. E., Mattioli, S., Ferretti, F., and Lorenzini, R. (2014). The unique Mesola red deer of Italy: taxonomic recognition (Cervus elaphus italicus nova ssp., Cervidae) would endorse conservation. Ital. J. Zool. 81, 136–143. doi: 10.1080/11250003.2014.895060
Keywords: Sus scrofa, SNPs, endemic diversity, genetic structure, admixture, genomic introgression, translocation, wild pigs
Citation: Scandura M, Fabbri G, Caniglia R, Iacolina L, Mattucci F, Mengoni C, Pante G, Apollonio M and Mucci N (2022) Resilience to Historical Human Manipulations in the Genomic Variation of Italian Wild Boar Populations. Front. Ecol. Evol. 10:833081. doi: 10.3389/fevo.2022.833081
Received: 10 December 2021; Accepted: 19 January 2022;
Published: 23 February 2022.
Edited by:
Thales Renato Ochotorena De Freitas, Federal University of Rio Grande do Sul, BrazilReviewed by:
Mariano Lisandro Merino, Comisión de Investigaciones Científicas, ArgentinaPavel Munclinger, Charles University, Czechia
Copyright © 2022 Scandura, Fabbri, Caniglia, Iacolina, Mattucci, Mengoni, Pante, Apollonio and Mucci. This is an open-access article distributed under the terms of the Creative Commons Attribution License (CC BY). The use, distribution or reproduction in other forums is permitted, provided the original author(s) and the copyright owner(s) are credited and that the original publication in this journal is cited, in accordance with accepted academic practice. No use, distribution or reproduction is permitted which does not comply with these terms.
*Correspondence: Massimo Scandura, scandura@uniss.it