- 1Agriculture Victoria Research, AgriBio Centre, Bundoora, VIC, Australia
- 2School of Applied Systems Biology, La Trobe University, Bundoora, VIC, Australia
The spotted wing drosophila (Drosophila suzukii, Matsumara) is a rapidly spreading global pest of soft and stone fruit production. Due to the similarity of many of its life stages to other cosmopolitan drosophilids, surveillance for this pest is currently bottlenecked by the laborious sorting and morphological identification of large mixed trap catches. DNA metabarcoding presents an alternative high-throughput sequencing (HTS) approach for multi-species identification, which may lend itself ideally to rapid and scalable diagnostics of D. suzukii within unsorted trap samples. In this study, we compared the qualitative (identification accuracy) and quantitative (bias toward each species) performance of four metabarcoding primer pairs on D. suzukii and its close relatives. We then determined the sensitivity of a non-destructive metabarcoding assay (i.e., which retains intact specimens) by spiking whole specimens of target species into mock communities of increasing specimen number, as well as 29 field-sampled communities from a cherry and a stone fruit orchard. Metabarcoding successfully detected D. suzukii and its close relatives Drosophila subpulchrella and Drosophila biarmipes in the spiked communities with an accuracy of 96, 100, and 100% respectively, and identified a further 57 non-target arthropods collected as bycatch by D. suzukii surveillance methods in a field scenario. While the non-destructive DNA extraction retained intact voucher specimens, dropouts of single species and entire technical replicates suggests that these protocols behave more similarly to environmental DNA than homogenized tissue metabarcoding and may require increased technical replication to reliably detect low-abundance taxa. Adoption of high-throughput metabarcoding assays for screening bulk trap samples could enable a substantial increase in the geographic scale and intensity of D. suzukii surveillance, and thus likelihood of detecting a new introduction. Trap designs and surveillance protocols will, however, need to be optimized to adequately preserve specimen DNA for molecular identification.
Introduction
The combined influences of international trade, tourism, and changing climates are increasing the rate at which new insect pests emerge and spread across borders, creating a global burden on food security (Savary et al., 2019). A particularly striking example is the rapid intercontinental spread of Drosophila suzukii, Matsumara (spotted wing drosophila), a significant pest of soft and stone fruits which over the last two decades has expanded from its native range in Southeast Asia (Kanzawa, 1939; Walsh et al., 2011), to Europe, the Americas, and more recently Africa (Cini et al., 2012; Asplen et al., 2015; Boughdad et al., 2021). The pace of this range expansion is attributed to a high fecundity, short generation time, and a broad host range that allows populations to persist throughout the year by alternating between cultivated and wild fruits with different ripening times (Cini et al., 2012). Recent modeling of global climatic suitability predicts rapid establishment of D. suzukii if introduced into regions and continents where it is not yet present, such as Australia and Aotearoa New Zealand (Dos Santos et al., 2017; Maino et al., 2021).
Early detection is critical for containment and eradication of invasive insect populations, with the probability of detecting a new introduction increasing with the intensity of surveillance (Anderson et al., 2017; Reaser et al., 2020). Surveillance for D. suzukii is generally conducted using traps baited with “food attractant” lures such as apple cider vinegar (Landolt et al., 2012), live yeasts (Hamby et al., 2014), or synthetic formulations based on these (Cha et al., 2014). To complement trapping, infested fruit can be crushed and agitated in a salt solution to float any larvae and eggs to the surface, which can then be collected via filtration (Van Timmeren et al., 2017). Neither of these surveillance techniques are specifically selective for D. suzukii, however, often also capturing hundreds of non-target specimens that must be sorted to detect a new introduction (Burrack et al., 2015; Tonina et al., 2018). Rapid morphological identification of D. suzukii is hampered by the characteristic “spotted wings” being present only for male flies, unreliable for juvenile adults, and shared by its sister species Drosophila biarmipes Malloch and Drosophila subpulchrella Takamori and Watabe (Hauser, 2011; Cini et al., 2012). Alternative molecular diagnostic methods such as DNA barcoding (Calabria et al., 2012), quantitative PCR (qPCR) (Dhami and Kumarasinghe, 2014), PCR-Restriction Fragment Length Polymorphism (PCR-RFLP) (Kim et al., 2014), and loop-mediated isothermal amplification (LAMP) (Kim et al., 2016) can provide accurate identifications for D. suzukii at any life stage, but the costly and time-consuming process of conducting single reactions on individual specimens has restricted their use to confirming the identity of specimens already suspected to be D. suzukii (Calabria et al., 2012; Boughdad et al., 2021). The lack of a cost-effective and high-throughput diagnostic method for bulk trap catches remains a major bottleneck for large-scale D. suzukii surveillance. The resulting risk of misidentification and delayed management response has potential to incur considerable costs to individual growers and national economies (Hauser, 2011).
DNA metabarcoding is a broad-scope molecular diagnostic approach that couples DNA barcoding with high-throughput sequencing (HTS) to simultaneously identify all amplified DNA sequences from complex mixed communities (Taberlet et al., 2012). The resulting data can be compared to both lists of regulated species and baseline knowledge of endemic biodiversity to screen not just for target pests, but also other unanticipated taxa that are not being actively searched for Hardulak et al. (2020), Batovska et al. (2021). The ability for metabarcoding to be conducted on mixed trap samples without any prior sorting (Nielsen et al., 2019) is particularly appealing for efficiently handling the large number of specimens likely to be produced by an intensive surveillance program. Nevertheless, ensuring the accuracy of detections must be a priority for applying metabarcoding to invasive species surveillance due to the stringent reporting requirements for regulated taxa and higher economic consequences of a false negative (Piper et al., 2019). During complex metabarcoding protocols, false positive detections can be introduced through laboratory contamination (Nguyen et al., 2015) and index switching (Schnell et al., 2015), while false negatives can arise through insufficient sequencing depth (Smith and Peay, 2014), stochastic sampling of molecules from low abundance specimens (Leray and Knowlton, 2017), and PCR biases (Deagle et al., 2014). Robust metabarcoding protocols thus require both technical replication and use of a detection threshold to resolve true positives from any low-abundance contaminant sequences (Zinger et al., 2019). The required number and type of replicates, and most appropriate manner for deriving this detection threshold remains unclear, however, for protocols which employ non-destructive DNA extractions (i.e., methods which retain morphologically intact specimens) (Carew et al., 2018; Nielsen et al., 2019; Batovska et al., 2021). These recently developed non-destructive protocols allow the high-throughput metabarcoding detections to be confirmed using morphological examination and voucher specimens to be retained according to regulatory requirements (Martins et al., 2019; Batovska et al., 2021), yet come at the expense of reduced DNA concentrations compared to more common destructive tissue-homogenization based protocols (Martoni et al., 2021).
In this study, we evaluated the use of a non-destructive DNA metabarcoding assay for detection of D. suzukii and its close relatives D. subpulchrella and D. biarmipes within unsorted trap samples. The qualitative and quantitative performance of four published primer sets is evaluated, and 6 methods for deriving a detection threshold compared. The diagnostic sensitivity, specificity, and overall accuracy of the protocol, as well as the required number of DNA extraction and PCR replicates is then determined via spiking target species into both mock communities of known composition and field samples collected from a cherry and stone fruit orchard. Analysis of these diverse orchard samples further enabled assessment of the selectivity of different D. suzukii sampling methods, as well as the effects of commonly used attractant lures on DNA quality of trapped specimens. Practical implementation of metabarcoding into D. suzukii surveillance and the wider implications of broad-scope HTS assays for plant pest diagnostics are discussed.
Materials and Methods
Assembling Mock Communities
To assemble mock communities for validating the metabarcoding assay, isofemale lines (David et al., 2005) of Drosophila melanogaster Meigen, Drosophila simulans Sturtevant, Drosophila hydei Sturtevant, and Scaptodrosophila lativittata Malloch were established from individual female drosophila trapped in banana baited traps (Reed, 1938) around Melbourne, Australia. F1 offspring from each isofemale line were identified via DNA barcoding (Hebert et al., 2003) using the LCO1490-HCO2198 primers (Folmer et al., 1994) (data at BOLD project DROSV), and those found to be of the same species combined into ongoing colonies. The identity of S. lativittata was confirmed via comparison with identified material in the Australian Museum (Sydney) due to the barcode matching a mislabeled Drosophila mediostrata sequence on BOLD (accession: BIOUG02267-H05). Drosophila melanogaster, D. simulans, and D. hydei colonies were maintained at 25 ± 0.5°C and 65 ± 5% relative humidity (RH) on a diet of instant drosophila medium (Carolina Biological Supply, United States) and live brewer’s yeast (Fleischmann’s, United States), while S. lativittata was maintained at 20 ± 2°C and 65 ± 5% RH on the diet described by Bock and Parsons (1980). Adult specimens were collected weekly into absolute ethanol, with a randomly selected 5 individuals barcoded every 2 months to confirm colony purity. Voucher specimens for each colony were deposited in the Victorian Agricultural Insect Collection (VAIC) held at the AgriBio Centre, Bundoora, Australia. Additional ethanol preserved specimens of D. suzukii, D. subpulchrella, D. biarmipes, and Drosophila immigrans Sturtevant were obtained from the Cornell Drosophila Stock Centre, United States, the Ehime University Drosophila Species Stock Centre, Japan, and the National Institute of Agricultural Botany East Malling Research Station, United Kingdom. Various numbers of adult or larval specimens were combined to form mock communities with total sizes ranging from 96 to 1,001 individuals (Supplementary Table 1), with each constituent species having relative abundances (RA) between 0 and 55%. Each mock community was suspended in absolute ethanol within 15 mL falcon tubes and stored at −20°C until DNA extraction.
Field Sampling
To obtain samples representative of the insect diversity expected to be encountered in a real surveillance program, 55 red cup traps (Lee et al., 2012) were deployed in a sweet cherry (Prunus avium L.) orchard and 44 traps in a mixed stone fruit (Prunus persica L.) orchard, each located in Mornington and Tatura, Victoria, Australia. To evaluate the selectivity and impacts on DNA quality of different D. suzukii lures, each trap contained one of three lures: (1) Apple cider vinegar as attractant and drowning solution (ACV) (Landolt et al., 2012), (2) the synthetic lure of Cha et al. (2014) as attractant and drowning solution (Syn) or (3) the same synthetic lure with a separate propylene glycol drowning solution and a dichlorvos insecticide cube (SPD). Trap catches were collected every 2 weeks over the course of a 10-week period from January to March 2018, with approximately 1 kg of recently fallen fruits also collected at each timepoint. These fruits were crushed and agitated in a 15% w/v salt solution and larvae collected using methods described in Van Timmeren et al. (2017), apart from the salt solution used here being almost twice the 8.2% w/v concentration of the original study as preliminary experiments showed higher recovery of larvae and eggs at this concentration (unpublished data). All field collected samples were combined by week of collection for each sampling method and orchard. This resulted in a total of 22 trapped samples each containing between 200 and 800 adult insect specimens, as well as seven fruit crush samples containing between 100 and 800 predominantly larval specimens. 13 of these field collected samples (10 trap and 3 fruit crush samples) were then spiked with single specimens (0.1–5% RA) of D. suzukii, D. subpulchrella, or D. biarmipes (Supplementary Table 2). All samples were then suspended in absolute ethanol within 15 mL falcon tubes and stored at −20°C until DNA extraction.
Non-destructive DNA Extraction
The non-destructive Qiagen DNeasy based method of Nielsen et al. (2019) was used to extract DNA from each mixed community in order to retain voucher specimens appropriate for morphological confirmation of any detected exotic species. Ethanol was removed from the samples using a 1,000 μL pipette and specimens dried overnight to ensure all residual ethanol was evaporated. The mixed specimens were suspended in a 10:1 mix of Qiagen ATL tissue lysis buffer and Proteinase K (Qiagen, Germany), with the total volume of buffer varying with the number of specimens to ensure all were fully immersed by at least 1 cm of buffer. Specimens in buffer were then incubated for 24 h at 56°C and 220 rpm in a shaking incubator. Following incubation, lysate was removed from the specimens and manually loaded into Qiagen 96 well DNeasy extraction blocks using a multichannel pipette, and the remainder of the Qiagen DNeasy Blood and Tissue protocol followed within the QiaCube automated DNA purification workstation (Qiagen, Germany). Voucher specimens retained after non-destructive DNA extraction were resuspended in absolute ethanol and stored at −20°C.
Amplification and Sequencing
Four candidate primers pairs; BF1-BR1 (Elbrecht and Leese, 2017), fwhF2-fwhR2n (Vamos et al., 2017), fwhF2-HexCOIR4 (Marquina et al., 2019a), and fwhF2-SauronS878 (Rennstam Rubbmark et al., 2018) producing 254–258 bp amplicons appropriate for 2 × 150 bp sequencing were selected for evaluation. These highly degenerated primers amplify a broad range of taxonomic groups, thus providing a measure of attractant selectivity, as well as the ability to incidentally detect other unexpected taxa alongside the targets. The qualitative and quantitative performance of each primer pair was compared on a subset of five mock and four field collected samples, then fwhF2-fwhR2n alone was used for the remaining 20 mock and 18 field samples as it detected the most taxa during primer evaluation. Each 25 μL PCR reaction consisted of 5 μL 5X MyFi reaction buffer (Bioline, United States), 1 μL of 10 nM forward and reverse primers, 0.8 μL MyFi DNA polymerase, 11.2 μL BSA and 2 μL of variable concentration template DNA. Cycling conditions were an initial denaturation at 94°C for 2 min, then 30 cycles of 94°C for 30 s, 50°C for 45 s, and 72°C for 45 s, followed by a final 2 min extension at 72°C. Successful amplification was verified on a 2% w/v agarose gel, then amplicons were diluted 1:10 in ddH20 with no further clean-up step. One microliter of each diluted COI amplicon was further amplified using 7 cycles of qPCR to attach Illumina sequencing adapters containing 8 bp unique-dual indices (Costello et al., 2018). Each 50 μL indexing reaction consisted of 10 μL 5X Phusion HF Buffer (Thermo Fisher Scientific, United States), 4 μL of 2.5 nM forward and reverse primers, 0.5 μL Phusion DNA polymerase (Thermo Fisher Scientific, United States), 32.5 μL ddH20, 1 μL of SYBR Green I Nucleic Acid Gel Stain (Thermo Fisher Scientific, United States) diluted 1:1,000 with ddH20, and 1 μL of template DNA. Cycling conditions for the indexing qPCR were 98°C for 10 s, 65°C for 30 s, and 72°C for 30 s.
While only a single DNA extraction and PCR replicate per sample was used for the initial primer comparison, after the fwhF2-fwhR2n primers were selected all further samples were replicated twice at the DNA extraction stage and three times at the PCR stage. DNA extraction replicates were obtained by taking two 500 μL aliquots of the lysate from the 24 h incubation and running each through the QiaCube on separate 96 well DNA extraction blocks, while PCR replicates were obtained by amplifying three separate 2 μL aliquots of the final DNA extract in different thermocyclers (Supplementary Figure 1). As insufficient unique-dual indices were available for all replicated samples, a “twin-tagging” approach (Axtner et al., 2019) was used where three modified versions of the forward and reverse primers containing an additional 2–4 bp inline tag at the 5′- terminus were used to separately amplify each set of PCR replicates (Supplementary Figure 2). These inline tags differed in length in order to improve phasing during the critical first cycles of the sequencing process (Lundberg et al., 2013; Elbrecht and Steinke, 2019). Two positive control libraries consisting of 13 equimolarly pooled synthetic gBlock gene fragments (Integrated DNA Technologies, United States) were included alongside all real communities after DNA extraction but prior to PCR amplification. Each synthetic gBlock was designed to reflect the COI gene of 13 major insect families (Drosophilidae, Tephritidae, Culicidae, Crambidae, Tortricidae, Apidae, Siricidae, Aphididae, Triozidae, Cerambycidae, Nitidulidae, Thripidae, and Acrididae) in base composition and structure (see Supplementary Material 1 for full details).
Following the indexing qPCR reaction, a melt curve analysis was used to quantify DNA concentrations, ramping from 70 to 90°C in increments of 1°C for 10 s, with a SYBR green fluorescence read (480 nm wavelength) at each increment. The concentrations obtained from the melt curve were then used to pool libraries in equimolar ratios using a Biomek FXP liquid handling robot (Beckman Coulter, United States). Pooled libraries were purified using a 0.8:1 ratio reaction of AMPure XP beads (Beckman Coulter, United States) to DNA and then sized and quantified using a 2,200 TapeStation (Agilent Technologies, United States) and Qubit 3.0 Fluorometer (Thermo Fisher Scientific, United States). Final libraries for the primer comparison were diluted to 7 pM, spiked with 5% PhiX, and sequenced on an Illumina MiSeq V2 flow cell using 2 × 150 bp reads. The remainder of fwhF2-fwhR2n amplified mock and field collected samples were treated with the Free Adapter Blocker reagent (Illumina, United States), and cleaned with a second 0.8:1 ratio reaction of AMPure XP beads. These libraries were then diluted to 100 pM, spiked with 1% PhiX and sequenced on a portion of an Illumina NovaSeq 6000 S2 flow cell lane, again using 2 × 150 bp reads. To minimize the risk of contamination from the laboratory environment, DNA extraction, preparation of PCR master-mix, PCR amplification, and library preparation were each performed in separate rooms using dedicated equipment and pipettes.
Bioinformatics
Sequence reads were demultiplexed using bcl2fastq v.2.20 allowing for zero mismatches to the expected index combinations, followed by a second round of demultiplexing for the inline tags using Seal in BBTools v38.87 (Bushnell et al., 2017). Demultiplexed sequencing reads were trimmed of PCR primer sequences using BBDuK in BBTools v38.87 and any sequences with > 1 expected error (Edgar and Flyvbjerg, 2015), of low complexity (containing < 8 unique k-mers of length 2), or containing any ambiguous “N” bases were removed. Remaining sequences were denoised using DADA2 v1.20 (Callahan et al., 2016), with the error model determined separately for the MiSeq and NovaSeq data. Due to overfitting of the default Loess error model to the binned quality scores provided by the NovaSeq, the model was modified to weight by the log10 of the total nucleotide transitions for each quality score, rather than the total number of nucleotide transitions, which improved the model fit across both runs (Supplementary Figure 3). Following denoising, the Amplicon Sequence Variants (ASVs) inferred separately from each sequencing run were combined into a single table and any chimeric sequences removed de novo using the removeBimeraDenovo function in DADA2 v1.20. The remaining ASVs were aligned to a Profile Hidden Markov Model (PHMM) of the COI barcode region (Piper et al., 2021) using the aphid v1.3.3 R package (Wilkinson, 2019) to remove any non-specific amplification products, then checked for frame shifts or stop codons that commonly indicate pseudogenes (Roe and Sperling, 2007).
Hierarchical taxonomy was assigned to the filtered ASVs with a minimum bootstrap support of 50% using the IDTAXA algorithm in DECIPHER v3.13 (Murali et al., 2018), trained on the curated insect reference database of Piper et al. (2021). This was followed by additional species level assignment using a nucleotide BLAST v2.11 (Altschul et al., 1990) search against the same reference database. To avoid over-classification errors, species identities obtained from BLAST searches were only accepted if the genus matched that predicted by IDTAXA. All species detections were confirmed using occurrence records from the Atlas of Living Australia (accessed 20th August 2021)1 and the Australian Faunal Directory (accessed 20th August 2021).2 Following taxonomic assignment, all samples which received < 1,000 reads, and all ASVs that were not classified to an Arthropod genus were removed, then the sequence read counts for all remaining ASVs were transformed into per-sample relative read abundances (RRA). A maximum likelihood phylogenetic tree was constructed from the remaining ASVs using FastTree v2.1.11 (Price et al., 2009) following the General Time-Reversible (GTR) model (Tavaré, 1986) and gamma distribution of rate variation among sites, then rooted on the edge connecting Insecta to Arachnida. All phylogenetic trees were plotted using the ggtree v3.04 R package (Yu et al., 2017, 2018).
Determining a Detection Threshold
A baseline detection threshold of 0.01% RRA was used to resolve false positive observations within the initial primer comparison, which approximates the expected rate of index-switching of both i5 and i7 indices when using unique-dual indices (Costello et al., 2018; MacConaill et al., 2018). For the later fwhF2-fwhR2n amplified samples, this baseline threshold was compared to five additional methods for empirically deriving a detection threshold: (i) The “unassigned indices” method used the abundance ratio of valid (applied during library preparation) to invalid (pairs that could only arise due to switching) index combinations as per Wilcox et al. (2018). (ii) The “positive control” method used the abundance ratio of synthetic COI sequences that were correctly assigned to the positive control libraries to those that were found in other samples. (iii) The “mock community” method used the abundance ratio of expected to unexpected taxon observations across all mock communities. (iv) The “logistic regression” method fit a logistic model to the log10 transformed per-sample RRA of each detection, trained on the expected and unexpected taxon observations within the mock communities, with the sequencing run included as an additional covariate to account for run-specific variation in contamination rates (Batovska et al., 2021). With this method the predictive equation from the logistic model describes the probability of each observation being a true positive (Coughlin et al., 1992), and all observations with probability ≥ 50% were considered detections. (v) The final method used the same logistic regression model but included both the number of DNA extraction and PCR replicates that each taxon was detected in as additional covariates. To evaluate the predictive performance of each approach, all taxon observations within the mock communities were randomly split into 80% training and 20% test sets and the logistic regression classifiers and all detection thresholds compared for their ability to remove cross contamination within the test dataset. To ensure the comparisons were robust to whichever observations were assigned to the training and test sets, the random splitting, training, and evaluation was repeated 1,000 times and the results averaged.
Statistical Analyses
Overlap in detected species between replicates was quantified using Jaccard’s index (Jaccard, 1908), and the influence of collection method and sequencing depth on replicate dissimilarity tested for significance using Analysis of Variance (ANOVA) and linear regression, respectively. Using the known presence or absence of target specimens spiked into both mock and field collected communities, the diagnostic sensitivity (proportion of known positives that were correctly identified as positives), diagnostic specificity (proportion of known negatives that were correctly identified as negatives), and overall accuracy of the assay (arithmetic mean of the sensitivity and specificity) were calculated separately for each target taxon. Relative amplification efficiency for the four primer sets on each mock community taxa was estimated from the observed (from sequencing) and expected (from specimens) using the compositional least-squares approach implemented in the metacal v0.2 R package (McLaren et al., 2019), with standard errors obtained from 1,000 bootstrap resamples. Following metabarcoding it was discovered that the D. immigrans specimens were contaminated with a small but unknown number of Drosophila albomicans Duda, Drosophila nasuta Lamb, Drosophila hypocausta Osten Sacken, Drosophila pulaua Wheeler, Drosophila kohkoa Wheeler, Drosophila rubida Mather and Drosophila sulfurigaster Duda, so all these species were considered as D. immigrans for calculation of amplification efficiencies and number of detected taxa from the mock communities.
Community Diversity
To ensure comparisons of species diversity (α-diversity) between field collected communities were not confounded by differing sequencing depths between samples (Willis, 2019), the breakaway v4.7.6 R package was used to estimate the number of unobserved species in each sample using the frequency ratios of detected species (Willis and Bunge, 2015). Once it was confirmed that there were no unobserved species in those samples with lower sequencing depths, both the observed species richness and Shannon index (Shannon, 1948) were calculated using the phyloseq v1.36 R package (McMurdie and Holmes, 2013). ANOVA was then used to test whether differences in α-diversity could be explained by the collection method or the orchard the sampling was conducted in, with post hoc pairwise comparisons made using Tukey tests. Differences in species composition (β-diversity) between communities was quantified using the weighted-UniFrac distance, which considers both the phylogenetic relatedness and relative abundance of taxa within each sample (Lozupone and Knight, 2005). The effect of sampling method and orchard type on β-diversity was tested for significance using multivariate generalized linear models as implemented in the manyglm function from the mvabund v4.1.12 R package (Wang et al., 2012). Principal coordinate analysis of the weighted-UniFrac distances was used to visualize the multidimensional clustering of samples. All statistical analyses were conducted within the R v4.1.0 statistical programming environment (R Core Team, 2020) using tidyverse v1.3.1 (Wickham et al., 2019) and tidymodels v0.1.3 (Kuhn and Wickham, 2020) packages, and figures plotted with ggplot2 v3.3.5 (Wickham, 2016).
Results
Comparison of 4 Mini-Primer Sets
A MiSeq paired-end sequencing run (2 × 150 bp) was conducted for a subset of five mock and four field collected communities in order to compare the four candidate primer sets, yielding 4,532,936 total reads (mean 116,229 ± 6,092 per sample). All taxa within the mock communities were recovered by the four primer combinations, apart from D. biarmipes which was below the 0.01% RRA detection threshold for BF1-BR1 (Figure 1A). The absence of D. biarmipes for this primer set where it should have been present in two samples at 1% abundance was not related to low total sequencing depth, as these libraries received 66,670 and 146,188 reads, respectively. In addition to the dropout of D. biarmipes, between 6 and 9 false positive detections per primer set were recorded across the mock communities (Figure 1A). Of these, only the D. immigrans and D. hydei false positives were recorded across all primers with > 1% RRA, indicating they may be due to physical cross contamination of a specimen when the mock communities were assembled. In contrast, the remaining false positives were each detected with RRAs between 0.01 and 0.08%, which alongside their presence at high abundance in other sequenced communities suggests they arose through index-switching. Across the four field samples used for primer evaluation, the fwhF2-fwhR2n primers detected 30 taxa, while fwhF2-HexCOIR4 and SauronS878-HexCOIR1 detected 26 and 24 taxa, respectively (Figure 1A). Despite an entire sample amplified with BF1-BR1 receiving insufficient sequence reads to pass through the bioinformatic pipeline, BF1-BR1 still detected 26 distinct taxa. Primer-specific differences were also seen in the identities of detected species (Figure 1A), with Carpophilus truncatus Murray and Carpophilus hemipterus L. only being detected by fwhF2-fwhR2n, and Lonchoptera bifurcata Fallén detected with all primer combinations except BF1-BR1. However, in all cases where a taxon was not detected by every primer combination it was at < 1% RA within the respective physical sample.
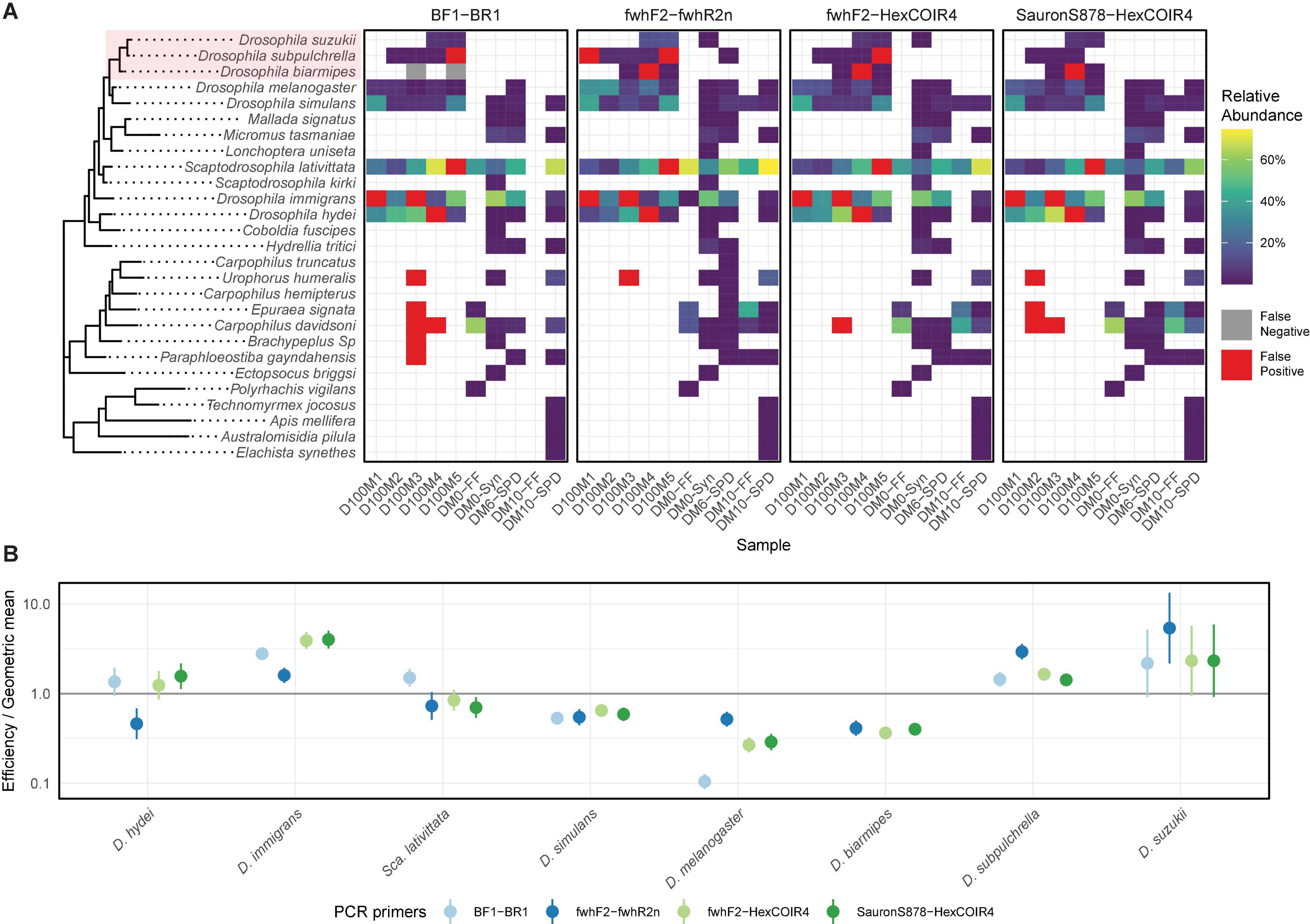
Figure 1. (A) Per-sample relative read abundance (RRA) of detected species across the four evaluated primer sets, with false positive and false negative results within the mock community samples highlighted. (B) Relative primer amplification efficiency of each mock community species compared to the geometric mean efficiency, with standard errors obtained from 1,000 bootstrap resamples. Spiked-in species exotic to Australia highlighted in red.
In addition to qualitative differences in detected taxa, primer specific quantitative biases were also seen across the mock community taxa (Figure 1B). BF1-BR1 showed an above average amplification efficiency for D. hydei, D. immigrans, S. lativittata, D. subpulchrella, and D. suzukii, a below average efficiency for D. simulans, and a very low efficiency for D. melanogaster, while the drop-out of D. biarmipes meant primer efficiency for this taxon was unable to be calculated. fwhF2-HexCOIR4 showed similar quantitative performance to BF1-BR1 across most taxa, except for S. lativittata which showed an average efficiency, and D. melanogaster where efficiency was slightly higher. In contrast, fwhF2-fwhR2n showed close to average efficiency for S. lativittata, a below average efficiency for D. hydei, D. simulans, D. melanogaster, and D. biarmipes, while preferentially amplifying D. immigrans, D. subpulchrella, and D. suzukii. Finally, SauronS878-HexCOIR4 preferentially amplified D. immigrans, D. suzukii, D. subpulchrella, and D. hydei, while showing below average efficiency for D. biarmipes, S. lativittata, D. melanogaster, and D. simulans. Across all primers, D. suzukii and D. hydei showed the largest variation in amplification efficiencies between samples, with the remainder of the mock community taxa having substantially smaller standard errors (Figure 1B). Ultimately, the fwhF2-fwhR2n primer combination was chosen to proceed for the remainder of the study as they identified the most species in the orchard samples (Figure 1A) and showed the highest efficiency for the targets D. suzukii, D. subpulchrella, and D. biarmipes (Figure 1B), which should increase the probability of detecting them at low abundance.
Replicate Similarity
The remaining 22 field collected samples, 20 mock communities, and two synthetic positive control samples were each replicated twice at the DNA extraction and three times at the PCR stage, with the resulting 264 libraries sequenced on a portion of a NovaSeq S2 flow cell lane. This yielded a total of 286.5 million reads following bioinformatic quality control (mean 1,447,221 ± 116,732 per replicate), however, a large number of replicate dropouts occurred across both the mock and field collected communities (Figure 2A). For the mock communities, 78% of the replicates from the adult samples and 67% from the larval samples were successfully sequenced. While for the field samples, 80% of replicates from the SPD treatment, 67% from the fruit crush, 57% from synthetic lure, and only 10% of replicates from the apple cider vinegar samples were successful. Most of these replicate dropouts occurred within the second set of PCR replicates from extraction replicate 1, where 40 of 50 were unsuccessful, including one of the positive controls (Figure 2A). As each set of replicates was processed in a separate microtiter plate and thermocycler (Supplementary Figure 1), this likely indicates a systematic failure during PCR amplification or when these replicates were pooled into the final libraries. Apart from this entire set of replicates, dropouts of single replicates seemed to randomly occur across the samples (Figure 2A). On the other hand, when considering samples where no replicates were successfully sequenced, there were apparent DNA preservation effects relating to collection method used (Table 1). For the field collected samples, all the fruit crush and SPD samples had at least one successfully sequenced replicate which could be analyzed further, while 75% of the synthetic lure samples and only one of the apple cider vinegar samples produced any usable data. For the mock communities, 93% of the adult communities and 80% of the larval communities as well as both positive control samples had at least one successfully sequenced replicate (Figure 2A).
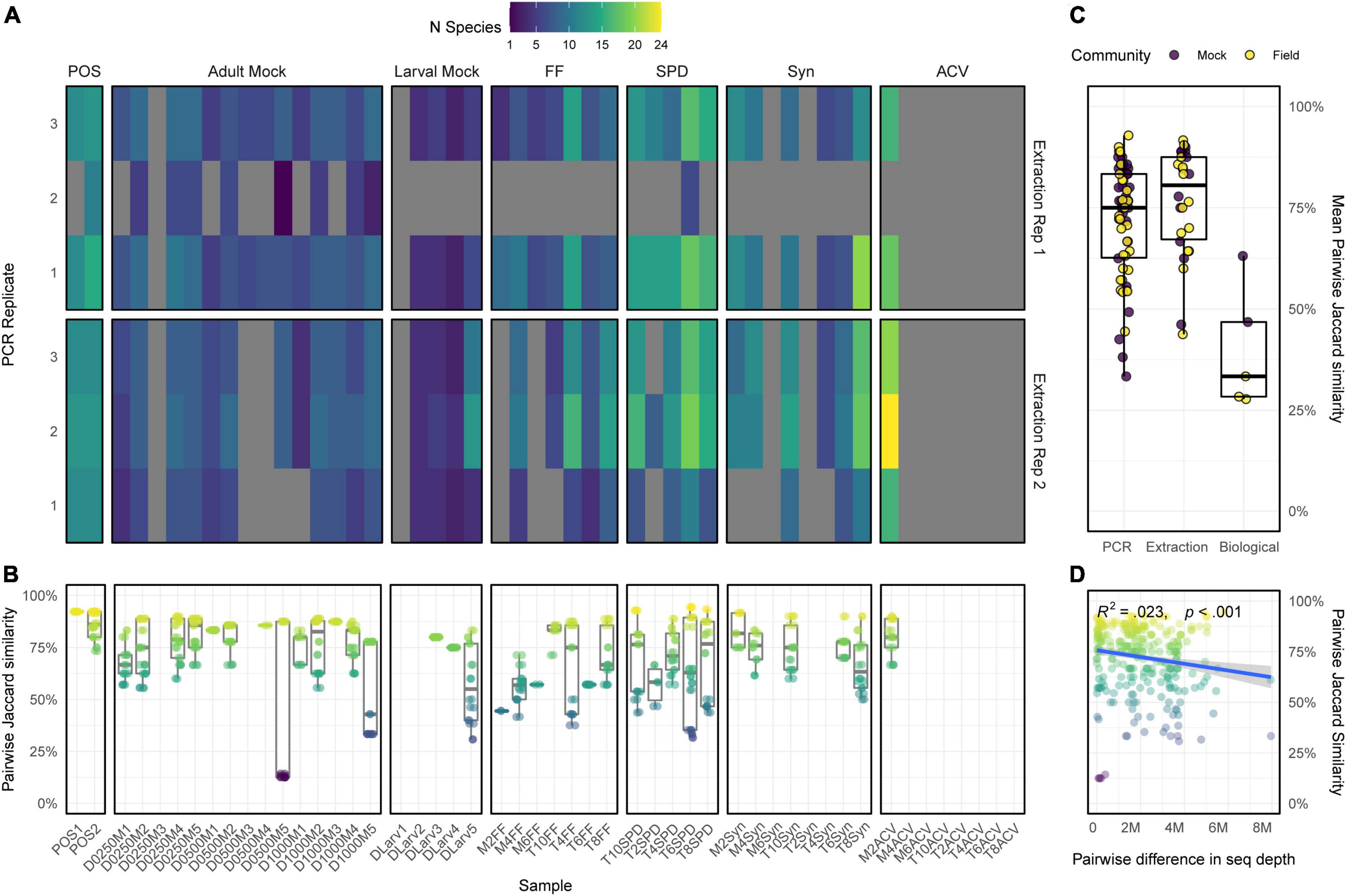
Figure 2. (A) Total number of species observed within each replicated sample prior to application of detection threshold, with complete replicate dropouts indicated in gray. (B) Pairwise Jaccard similarity (presence/absence) coefficients between all replicates from each sample (C) mean Jaccard coefficient between PCR replicates of the same DNA extract, DNA extraction replicates of the same sample, and separate samples obtained using the same collection method (biological replicates). (D) Relationship between pairwise Jaccard similarity and sequencing depth difference for all replicates.

Table 1. Drosophila suzukii surveillance methods used to collect field samples for evaluation of the non-destructive metabarcoding assay, and the number of samples which had at least one successfully sequenced replicate.
While most successfully sequenced replicates had reached saturation in species accumulation (Supplementary Figure 4), the pairwise Jaccard similarity between each ranged from 11 to 98% (Figure 2B), and showed a weak but statistically significant relationship with pairwise differences in sequencing depth (R2 = 0.023, p < 0.001; Figure 2D). A significant relationship was also found between replicate dissimilarity and the community type (field or mock) or collection method used [ANOVA; F(4,533) = 5.39, p < 0.001], which post hoc comparisons revealed to be driven by replicates of the fruit crush being less similar to each other than those from both the synthetic lure or the adult mock communities (Tukey HSD; p < 0.001), and replicates of SPD being less similar to each other than the adult mock communities (p < 0.001). Again, in all cases where a detection occurred in ≤ 50% of the sequenced replicates, the taxon was at ≤ 1% RA within the respective physical sample. Overall, extraction replicates from the same samples were as similar to each other as PCR replicates from the same DNA extraction, but separate samples collected from the same orchard using the same collection method (biological replicates) showed much less overlap in species detected (Figure 2C). There were no significant differences seen in the quantitative performance between the three tagged primer sets on any of the mock community taxa (Supplementary Figure 5), indicating replicate dissimilarity was not due to the twin-tagging approach to multiplexing.
Determining a Detection Threshold
All methods for deriving a detection threshold increased the proportion of true positive detections compared to the uncorrected data, but the degree of improvement varied substantially (Figure 3A). The baseline 0.01% RRA filtering threshold more than halved the number of false positives for the MiSeq and NovaSeq runs, but introduced a substantial number of false negatives in the latter. Surprisingly, the positive control method (included in the NovaSeq run only) performed worse than the baseline threshold, only marginally reducing the number of false positives compared to the uncorrected data. As these two positive control samples were included after DNA extraction, this limited success could indicate that false positives arose through physical cross contamination during or prior to DNA extraction, rather than index switching. Yet the unassigned indices method, which should only account for index switching, removed substantially more false positives than the positive control approach. The mock community method on the other hand did not improve the proportion of true positives above the baseline threshold for the NovaSeq run but performed the best for the MiSeq data (Figure 3A). Both logistic regression classifiers fit to this same mock community abundance information performed equivalently to just the abundance ratio as a threshold on the MiSeq run, while for the NovaSeq data the logistic regression with replicates slightly improved the results. Ultimately, the logistic regression classifier incorporating both abundance and replicate information was chosen for use on the field samples, as it showed consistent performance across both datasets and framing the trade-off between false positives and negatives in terms of the probability of an observation being a true detection provides advantages for interpretation. When this final model was trained again on the full dataset, the most important model covariates were the mean RRA of a taxon across all replicates (t = 10.37), followed by the sequencing run (t = 8.46), and number of DNA extraction replicates it was observed in (t = 4.06), while the total number of PCR replicates it was observed in was the least important (t = 0.13). Following application of the logistic regression model, D. suzukii was successfully detected in all 19 positive samples with a single false positive, giving a diagnostic sensitivity of 100% and specificity of 87.5% (Figure 3B). The false positive occurred in sample D100M2 sequenced on the NovaSeq run, where 593 reads were observed (0.004% RA). The secondary targets, D. subpulchrella and D. biarmipes were detected in 13 of 13 and 11 of 11 positive samples, respectively, with no false positive detections, resulting in a sensitivity and specificity of 100% for both (Figure 3B). The overall accuracy of the assay for the targets was 96.2, 100, and 100% for D. suzukii, D. subpulchrella, and D. biarmipes respectively.
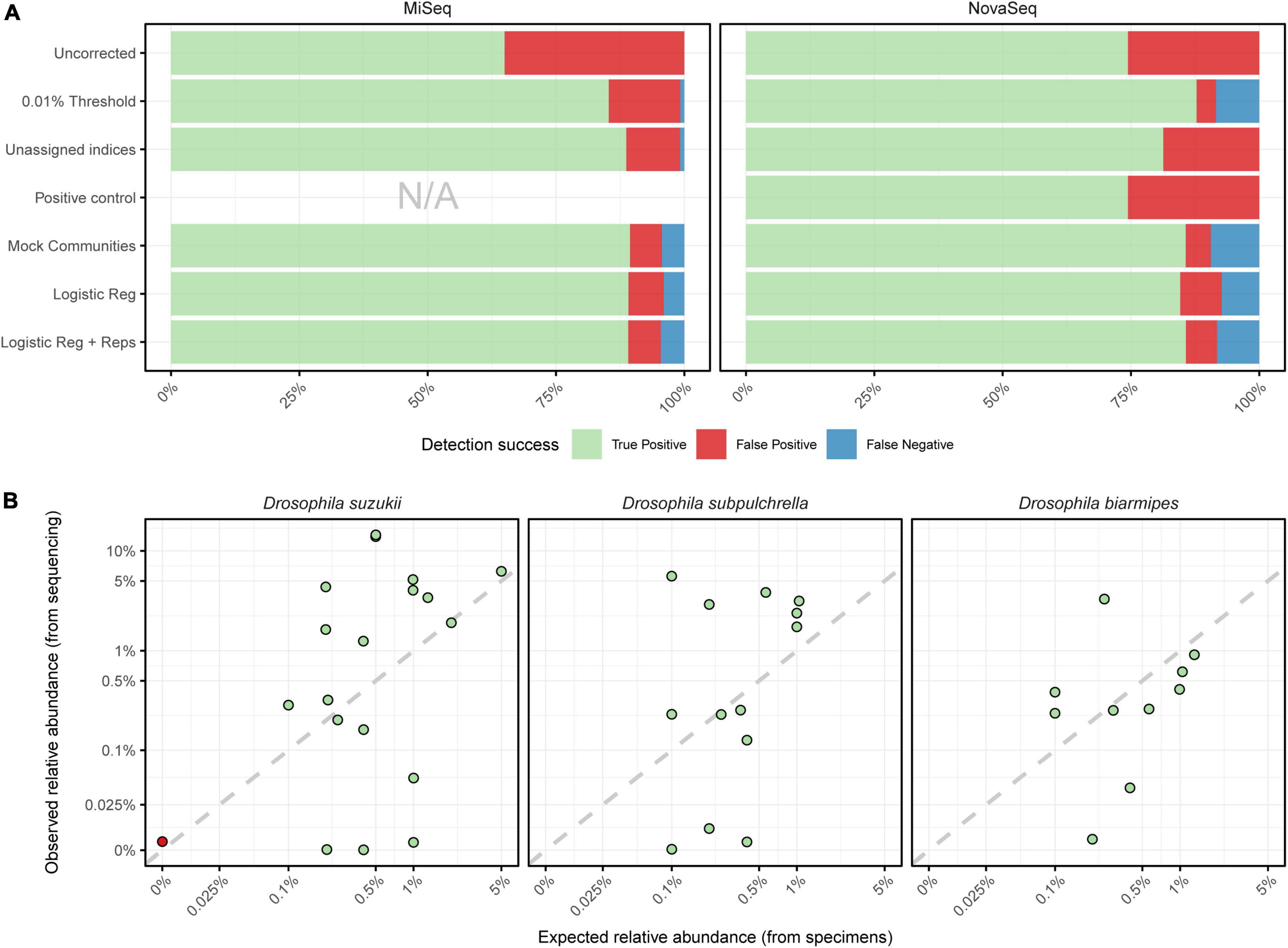
Figure 3. (A) The proportion of true positives, false positives, and false negatives across both the MiSeq and NovaSeq runs following application of various methods for deriving a detection threshold. (B) Relationship between expected (from specimens) and observed (from sequencing reads) relative abundance for target species spiked into mock and trap communities, with each observation colored by whether it was classified as a true positive or false negative by the logistic regression model. Data is displayed on a pseudo-log scale to avoid compressing variation around zero, with dashed lines indicating a perfect relationship between expected and observed relative abundances.
Community Diversity
A total of 1,281 specimens were collected from the cherry orchard, and 4,772 from the stone fruit orchard over the entirety of the 10-week trapping period (Figure 4B). Of these, 654 specimens were caught in the apple cider vinegar (ACV) traps, 1,640 in the synthetic lure (Syn), and 2,224 in the synthetic lure treatment with the propylene glycol and insecticide cube (SPD). On the other hand, fruit crushing and salt flotation (FF) collected at least 1,535 specimens, with the absolute number likely being much higher due to some larvae being too small to accurately count. Following sequencing and application of the logistic regression detection model, a total of 60 unique insect taxa were identified within the trap samples, 59 of which could be successfully assigned to species level taxonomy (Figure 4). This bycatch diversity included 21 Diptera (excluding the three spiked in targets), 11 Coleoptera, 9 Hymenoptera, 5 Hemiptera, 3 Lepidoptera, 2 Psocoptera, 2 Thysanoptera, and a single Orthopteran and Neuropteran species (Figure 4A). Although the PCR primers used were designed to amplify insects (Vamos et al., 2017), the spider species Badumna longinqua L. Koch, Tenuiphantes tenuis Blackwall, and Plebs eburnus Keyserling were also detected in the fruit crush and synthetic lure samples from the cherry orchard. When the identities of bycatch taxa were compared to species occurrence records, all were confirmed to be endemic or previously recorded in Australia.
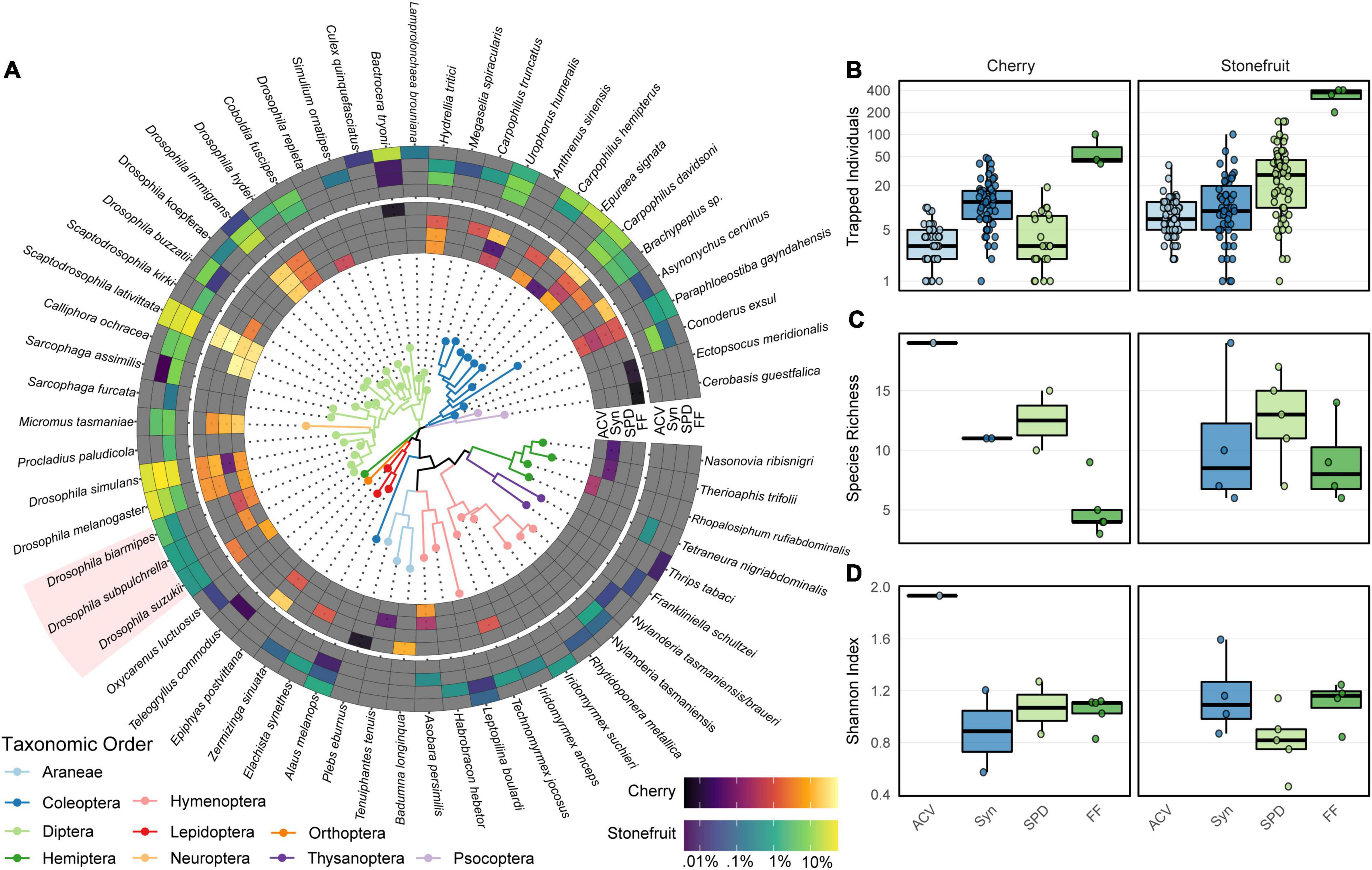
Figure 4. (A) Phylogenetic relationships between all detected taxa in the field samples, with the mean relative read abundance (RRA) of each taxon displayed by sampling method for the cherry (inner heatmap) and stone fruit (outer heatmap) orchards. (B) Number of individual specimens collected from each orchard by each sampling strategy over the total course of the 10-week trapping period, displayed on a pseudo-log scale. (C) Species richness and (D) Shannon index for each community following metabarcoding. Spiked-in target species exotic to Australia highlighted in red.
For the field collected communities, the species richness projections estimated by the breakaway model matched the number of detected species, indicating that all species in each sample had been captured at that sequencing depth. For these communities, the sampling method significantly affected species richness [ANOVA; F(3, 19) = 5.19, p = 0.009] (Figure 4C), with the single successful ACV sample (17 species) containing significantly more species than the fruit crush treatment (mean 6.78 ± 1.15, p = 0.029), but no significant differences were found between any of the other sampling methods (p > 0.05). Significant differences in Shannon diversity were also found between sampling methods [F(3, 19) = 5.23, p = 0.008], primarily driven by the SPD treatment having more taxa at low abundance than the ACV treatment (p < 0.001) (Figure 4B). In contrast, no significant differences were found between the cherry and stone fruit orchards for either of the α-diversity metrics [F(1, 21) = 0.76, 0.49, both p > 0.05]. There were significant effects of both orchard [manyglm; LRT(2, 19) = 126.98, p = 0.002] and sampling method [LRT(1, 21) = 224.53, p < 0.001] on community composition (β-diversity), but a significant interaction effect was also found between the two [LRT(2, 17) = 943229.53, p < 0.001]. Principal coordinate analysis of weighted-UniFrac distances revealed that while the SPD samples from the stone fruit orchard clustered tightly together, and close to the fruit crush samples from the same orchard, the synthetic lure samples showed much higher dispersion (Supplementary Figure 6). In contrast, the synthetic lure, SPD, and fruit crush samples from the cherry orchard were dispersed across both principal coordinates. Taken together, this indicates that differences in β-diversity are primarily driven by a distinct cohort of species occurring in each orchard, as well as between the synthetic lure and fruit crush samples within the cherry orchard. For the stone fruit orchard on the other hand, the differences in species occurrence and relative abundance between sampling methods were much less pronounced (Figure 4A and Supplementary Figure 6).
Discussion
Whilst originally developed for studying biodiversity, metabarcoding approaches are increasingly being applied to the detection of invasive species in aquatic and terrestrial environments (Brown et al., 2016; Piper et al., 2019; Tedersoo et al., 2019). Here, we demonstrated the use of a non-destructive metabarcoding assay to detect the globally significant pest D. suzukii and its close relatives D. biarmipes and D. subpulchrella within large, unsorted trap catches. By circumventing the time-consuming and labor-intensive process of morphological sorting, adoption of metabarcoding assays by diagnostic laboratories could enable a substantial increase in the geographic scale and intensity of D. suzukii surveillance, and thus the likelihood of detecting a new introduction. Nevertheless, our results highlight aspects of trap design and laboratory protocols that may need to be reconsidered if metabarcoding is to be successfully adopted for invasive insect diagnostics.
Apple cider vinegar is the most commonly used attractant and drowning solution for D. suzukii surveillance (Landolt et al., 2012; Hamby et al., 2014; Harris et al., 2014; Mazzetto et al., 2015), yet almost all communities collected using this method failed to produce a sequenceable amplicon. This limited success may be related to trapped specimens being immersed within the highly acidic and watery solution for up to 2 weeks between traps being set and collected, which can cause degradation of DNA molecules (Lindahl, 1993). Even so, if pH or hydrolysis mediated DNA degradation were the only factors involved, a comparable failure rate would be expected for those communities trapped in the more acidic synthetic lure (Table 1). Furthermore, even if the DNA of the trapped specimens were completely degraded, the ethanol preserved D. suzukii, D. subpulchrella, and D. biarmipes specimens that were spiked into these samples should have produced some data. On the other hand, apple cider vinegar is a complex matrix containing various polysaccharides, polyphenolics, and tannins, all of which have PCR inhibiting properties (Jara et al., 2008). While all specimens were rinsed with ethanol, and the DNA extraction method involved two clean-up steps, carry over of some residual inhibitors may have prevented amplification for many of these samples (Martins et al., 2019). In contrast, traps employing the synthetic attractant lure but using a separate propylene glycol drowning solution adequately preserved specimens for metabarcoding analysis. Propylene glycol shows promise for use in Drosophila surveillance traps when molecular methods are to be used for identification, being cheap, non-flammable, non-evaporative, and able to effectively preserve DNA for several months (Martoni et al., 2021; Weigand et al., 2021). To facilitate the use of liquid preservatives such as propylene glycol, new trap designs should physically separate the highly acidic lures from the drowning solution, either in a separate compartment within the trap or a controlled release sachet (Larson et al., 2020). Nevertheless, DNA degradation or PCR inhibition were not the only factors in play, and further optimization of the laboratory protocol may be required to resolve the seemingly random dropouts of single replicates that occurred across both the mock and field collected communities.
Early detection surveillance depends upon swift diagnostic turnaround to ensure that quarantine and intervention procedures are appropriate and effective. In light of this, our study opted for a rapid laboratory protocol which omitted any normalization or purification of DNA between the extraction and both PCRs. While similar rapid protocols have been successfully applied to destructively homogenized specimens (Elbrecht and Steinke, 2019; Steinke et al., 2021), the extra variability introduced by this non-destructive protocol may have contributed to the large number of replicate dropouts observed. Therefore, we suggest that future studies employing non-destructive DNA extractions normalize the resulting extracts to similar concentrations before PCR amplification to avoid disparities in DNA concentration between libraries impacting later stages of the protocol. Although these additional normalization steps will increase laboratory processing time, ultimately it is the sequencing process itself which represents the longest step in a metabarcoding assay, taking between 40 and 56 h depending on the HTS platform (Piper et al., 2019). While the Illumina NovaSeq used in our study is currently the most cost-effective for large numbers of samples, drawing together hundreds of trap samples on a regular basis without in-turn increasing diagnostic turnaround times may prove a logistical challenge for smaller surveillance programs. Therefore, lower throughput platforms such as the Illumina MiSeq will likely remain important into the future, despite their higher cost per gigabase of data and longer runtimes (Elbrecht et al., 2017). The challenge of pooling enough samples for timely and cost-effective metabarcoding on contemporary HTS platforms may limit the immediate utility of this technique for individual growers using monitoring traps for pest management decision making, particularly for those already well versed in identifying male D. suzukii in trap catches. Therefore, in D. suzukii endemic regions, metabarcoding based diagnostics may best be implemented as part of an area wide management approach where a central diagnostic laboratory could process samples for a larger region. Alternatively, emerging nanopore HTS platforms offer more flexible input requirements, substantially lower purchase price, and real time data production (Baloğlu et al., 2021), which may allow for more decentralized adoption of metabarcoding diagnostics in the future.
In addition to dropouts of some replicates, there was also variability in the taxa detected between successfully sequenced replicates. While this replicate dissimilarity showed a slight relationship with sequencing depth differences, with a mean 1.4 million sequence reads per replicate, the sequencing depths obtained in our study were an order of magnitude higher than most metabarcoding studies (Singer et al., 2019). Conversely, all taxa that were detected in 50% or less replicates were below 1% RA within the respective physical community, suggesting that the taxonomic dropouts were not simply a product of insufficient sequencing depth as some have proposed (Smith and Peay, 2014), but instead may be due to stochastic sampling of DNA molecules from low abundance taxa (Leray and Knowlton, 2017). This phenomenon, also known as pipeline noise, increases dissimilarity between replicated samples (Zhou et al., 2013) and can be further exacerbated by taxonomic biases in DNA extraction and PCR efficiency (McLaren et al., 2019). Previous studies conducting metabarcoding on preservative ethanol have found higher variance in taxon detections compared to homogenized tissue, and that results are much more sensitive to exoskeleton hardness and specimen morphology, rather than just specimen biomass (Marquina et al., 2019b; Zizka et al., 2019). The leeching of specimen DNA into ethanol is conceptually similar to the non-destructive DNA extraction used here, and therefore we expect similar issues to have played a role in our study. Further mechanistic research will be required to better understand the specific biases of non-destructive methods; yet our results suggest that these approaches may best be considered closer to environmental DNA metabarcoding, which requires a higher level of replication to maximize species detection (Ficetola et al., 2015). These replicates should be taken at the DNA extraction stage, as this type of replicate was found to be more informative for differentiating true detections from false positives. That said, including technical replicates should not come at the expense of reduced biological samples, as regardless of the effectiveness of metabarcoding or any other diagnostic assay, if an insect is not caught in a trap, it does not necessarily mean it is absent in the area (Low-Choy, 2015).
Molecular recombination of oligonucleotide indices used to label samples during sequencing can cause taxa from one sample to “bleed” into others and must be controlled for using a detection threshold (Piper et al., 2019). Use of positive control samples in the form of synthetic sequences or taxa “alien” to the study environment has been proposed for empirically measuring and accounting for the run-specific contamination rate (Galan et al., 2018; Palmer et al., 2018). In our study, however, we found this approach drastically underestimated cross contamination, underperforming compared to simply placing a minimum abundance threshold of 0.01% RRA across the dataset. Indeed, none of the evaluated methods for empirically deriving a detection threshold were able to increase the proportion of true positives detections above 90%. Ultimately, the similar abundances recorded for taxa close to the limits of detection and false positive observations introduced by index switching means there will always be a trade-off between type I and II error (Alberdi et al., 2019). Despite this, the ability of the logistic regression model to frame this trade-off in terms of a probability that an observation is a true detection provides benefits for practical interpretation of the results. While in our study a simple probability threshold of 50% was used to consider an observation a true detection or not, this threshold could be further tailored to the specific goals and statistical power desired by the surveillance program (Whittle et al., 2013). For instance, a biodiversity survey may prefer a stricter threshold to ensure only the most robust detections are recorded (Alberdi et al., 2019), whilst an invasive species survey may opt for a more lenient threshold to maximize sensitivity, as the economic consequences of a false negative are much higher (Jarrad et al., 2011). Nevertheless, this logistic regression approach, as well as the mock community and positive control methods all require a portion of each sequencing run to be pre-allocated to mock communities or positive controls, which introduces additional sequencing costs. For studies where this may not be practical, both the abundance ratio of correctly assigned to unassigned index combinations, as well as the baseline 0.01% relative abundance threshold adequately controlled the contamination rate here. Alternatively, the twin-tagging approach used in this study to differentiate PCR replicates (Supplementary Figure 1) could be expanded to ensure every library contains a completely unique twin-tag as well as the unique adapter indices. The extra power to identify switched molecules enabled by this approach has recently been shown to alleviate cross contamination issues altogether (Yang et al., 2021), yet comes at the substantial upfront cost of purchasing separate primer oligos for each sample and replicate.
Besides the spiked-in target species, metabarcoding revealed the identity of diverse arthropod communities collected as bycatch through D. suzukii surveillance methods in Australian orchards. Communities extracted from fallen fruit were the least diverse but showed the most variation between weeks as the number of decayed fruits progressively increased throughout the course of the sampling period. In agreement with previous comparisons of D. suzukii attractants, the synthetic lure outperformed the apple cider vinegar in both the number of specimens collected and selectivity for Drosophila species (Burrack et al., 2015; Cha et al., 2018; Tonina et al., 2018). The SPD treatment on the other hand was less selective than the synthetic lure on its own, likely due to the inclusion of the dichlorvos insecticide cube, the effects of which were clearly illustrated by the presence of the ant species Iridomyrmex anceps Roger and Technomyrmex jocosus Forel within these samples. These ants may have entered traps to prey on already collected insects and would have been able to safely exit those traps which solely relied upon flying insects drowning in the attractant solution. Predation of trapped specimens by ants and spiders has been documented previously (Armstrong and Richman, 2007; Lynegaard et al., 2014), and raises an intriguing question about whether predation could be an additional source of false negatives for biosecurity surveillance programs.
Whilst all the bycatch taxa identified in our study were either endemic or previously recorded in Australia, in other cases metabarcoding has revealed the presence of unanticipated or cryptic exotic species that have been missed by previous targeted surveys (Simmons et al., 2016; Batovska et al., 2021). This aspect of metabarcoding is particularly promising for revealing historical introductions that have gone undiscovered due to the lack of targeted surveillance for that species (Essl et al., 2011; Maclachlan et al., 2021). While the ability for metabarcoding to be conducted on unsorted trap catches represents a significant advance in itself, this universal nature of metabarcoding primers could substantially expand the range of organisms within the scope of a diagnostic laboratory (Piper et al., 2021). Adoption of high-throughput metabarcoding assays for screening of mixed trap catches therefore offers a viable method for increasing the geographic scale and intensity of invasive insect surveillance that could be readily adapted to the next emerging threat.
Data Availability Statement
The datasets presented in this study can be found in online repositories. The names of the repository/repositories and accession number(s) can be found below: NCBI SRA, PRJNA722763. Zenodo, http://doi.org/10.5281/zenodo.5726336 and GitHub, https://github.com/alexpiper/Drosophila_metabarcoding.
Author Contributions
AP, JC, and MB conceptualized the study. AP performed all field sampling, laboratory procedures, bioinformatics, and statistical analyses, and wrote draft manuscript with input and supervision from JC, NC, and MB. All authors read and approved the final manuscript.
Funding
Financial support was provided by Agriculture Victoria’s Improved Market Access for Horticulture Programme (CMI105584), and Horticulture Innovation Australia (ST16010) through funding from the Australian Government Department of Agriculture as part of its Rural R&D for Profit program and Grains Research and Development Corporation. AP was further supported by an Australian Government Research Training Program Scholarship.
Conflict of Interest
The authors declare that the research was conducted in the absence of any commercial or financial relationships that could be construed as a potential conflict of interest.
Publisher’s Note
All claims expressed in this article are solely those of the authors and do not necessarily represent those of their affiliated organizations, or those of the publisher, the editors and the reviewers. Any product that may be evaluated in this article, or claim that may be made by its manufacturer, is not guaranteed or endorsed by the publisher.
Acknowledgments
We would like to thank Jessica Henneken, Shahidul Islam, Kevin Farnier and Farrukh Baig for their assistance with field work, Lea Rako and Shane McEvey for their taxonomic support, and Francesco Martoni for assisting to validate the adapter indices used in this study. We further thank the Cornell Drosophila Species Stock Centre, Ehime University Drosophila Species Stock Centre, and the National Institute of Agricultural Botany East Malling Research Centre for providing the exotic Drosophila specimens used in this work. Field sampling for this study was conducted on the traditional lands of the Yorta Yorta and Bunurong people and we pay our respects to elder’s past and present. We would further like to thank Douglas Yu, James Hereward, and four reviewers for their constructive suggestions on previous versions of this manuscripts.
Supplementary Material
The Supplementary Material for this article can be found online at: https://www.frontiersin.org/articles/10.3389/fevo.2022.822648/full#supplementary-material
Footnotes
References
Alberdi, A., Aizpurua, O., Bohmann, K., Gopalakrishnan, S., Lynggaard, C., Nielsen, M., et al. (2019). Promises and pitfalls of using high-throughput sequencing for diet analysis. Mol. Ecol. Resour. 19, 327–348. doi: 10.1111/1755-0998.12960
Altschul, S. F., Gish, W., Miller, W., Myers, E. W., and Lipman, D. J. (1990). Basic local alignment search tool. J. Mol. Biol. 215, 403–410. doi: 10.1016/S0022-2836(05)80360-2
Anderson, C., Low-Choy, S., Whittle, P., Taylor, S., Gambley, C., Smith, L., et al. (2017). Australian plant biosecurity surveillance systems. Crop Prot. 100, 8–20. doi: 10.1016/j.cropro.2017.05.023
Armstrong, J. S., and Richman, D. B. (2007). Interference of Boll Weevil trapping by spiders (Araneida) and an evaluation of trap modification to reduce unwanted Arthropods. J. Entomol. Sci. 42, 392–398. doi: 10.18474/0749-8004-42.3.392
Asplen, M. K., Anfora, G., Biondi, A., Choi, D. S., Chu, D., Daane, K. M., et al. (2015). Invasion biology of spotted wing Drosophila (Drosophila suzukii): a global perspective and future priorities. J. Pest Sci. 88, 469–494. doi: 10.1007/s10340-015-0681-z
Axtner, J., Crampton-platt, A., Lisa, A. H., Mohamed, A., Xu, C. C. Y., Yu, D. W., et al. (2019). An efficient and robust laboratory workflow and tetrapod database for larger scale environmental DNA studies. Gigascience 8:giz029. doi: 10.1093/gigascience/giz029
Baloğlu, B., Chen, Z., Elbrecht, V., Braukmann, T., MacDonald, S., and Steinke, D. (2021). A workflow for accurate metabarcoding using nanopore MinION sequencing. Methods Ecol. Evol. 12, 794–804. doi: 10.1128/AEM.01368-19
Batovska, J., Piper, A. M., Valenzuela, I., Cunningham, J. P., and Blacket, M. J. (2021). Developing a non-destructive metabarcoding protocol for detection of pest insects in bulk trap catches. Sci. Rep. 11:7946. doi: 10.1038/s41598-021-85855-6
Bock, I. R., and Parsons, P. A. (1980). Culture methods for species of the Drosophila (Scaptodrosophila) coracina group. Drosoph. Inf. Serv. 55, 147–148.
Boughdad, A., Haddi, K., El Bouazzati, A., Nassiri, A., Tahiri, A., El Anbri, C., et al. (2021). First record of the invasive spotted wing Drosophila infesting berry crops in Africa. J. Pest Sci. 94, 261–271. doi: 10.1007/s10340-020-01280-0
Brown, E. A., Chain, F. J. J., Zhan, A., MacIsaac, H. J., and Cristescu, M. E. (2016). Early detection of aquatic invaders using metabarcoding reveals a high number of non-indigenous species in Canadian ports. Divers. Distrib. 22, 1045–1059. doi: 10.1111/ddi.12465
Burrack, H. J., Asplen, M., Bahder, L., Collins, J., Drummond, F. A., Guédot, C., et al. (2015). Multistate comparison of attractants for monitoring Drosophila suzukii (Diptera: Drosophilidae) in blueberries and caneberries. Environ. Entomol. 44, 704–712. doi: 10.1093/ee/nvv022
Bushnell, B., Rood, J., and Singer, E. (2017). BBMerge – Accurate paired shotgun read merging via overlap. PLoS One 12:e0185056. doi: 10.1371/journal.pone.0185056
Calabria, G., Máca, J., Bächli, G., Serra, L., and Pascual, M. (2012). First records of the potential pest species Drosophila suzukii (Diptera: Drosophilidae) in Europe. J. Appl. Entomol. 136, 139–147. doi: 10.1111/j.1439-0418.2010.01583.x
Callahan, B. J., McMurdie, P. J., Rosen, M. J., Han, A. W., Johnson, A. J. A., and Holmes, S. P. (2016). DADA2: high-resolution sample inference from Illumina amplicon data. Nat. Methods 13, 581–583. doi: 10.1038/nmeth.3869
Carew, M. E., Coleman, R. A., and Hoffmann, A. A. (2018). Can non-destructive DNA extraction of bulk invertebrate samples be used for metabarcoding? PeerJ 6:e4980. doi: 10.7717/peerj.4980
Cha, D. H., Adams, T., Werle, C. T., Sampson, B. J., Adamczyk, J. J., Rogg, H., et al. (2014). A four-component synthetic attractant for Drosophila suzukii (Diptera: Drosophilidae) isolated from fermented bait headspace. Pest Manag. Sci. 70, 324–331. doi: 10.1002/ps.3568
Cha, D. H., Hesler, S. P., Wallingford, A. K., Zaman, F., Jentsch, P., Nyrop, J., et al. (2018). Comparison of commercial lures and food baits for early detection of fruit infestation risk by Drosophila suzukii (Diptera: Drosophilidae). J. Econ. Entomol. 111, 645–652. doi: 10.1093/jee/tox369
Cini, A., Ioriatti, C., and Anfora, G. (2012). A review of the invasion of Drosophila suzukii in Europe and a draft research agenda for integrated pest management. Bull. Insectol. 65, 149–160.
Costello, M., Fleharty, M., Abreu, J., Farjoun, Y., Ferriera, S., Holmes, L., et al. (2018). Characterization and remediation of sample index swaps by non-redundant dual indexing on massively parallel sequencing platforms. BMC Genomics 19:332. doi: 10.1186/s12864-018-4703-0
Coughlin, S. S., Trock, B., Criqui, M. H., Pickle, L. W., Browner, D., and Tefft, M. C. (1992). The logistic modeling of sensitivity, specificity, and predictive value of a diagnostic test. J. Clin. Epidemiol. 45, 1–7. doi: 10.1016/0895-4356(92)90180-U
David, J. R., Gibert, P., Legout, H., Pétavy, G., Capy, P., and Moreteau, B. (2005). Isofemale lines in Drosophila: an empirical approach to quantitative trait analysis in natural populations. Heredity 94, 3–12. doi: 10.1038/sj.hdy.6800562
Deagle, B. E., Jarman, S. N., Coissac, E., Pompanon, F., and Taberlet, P. (2014). DNA metabarcoding and the cytochrome c oxidase subunit I marker: not a perfect match. Biol. Lett. 10:20140562. doi: 10.1098/rsbl.2014.0562
Dhami, M. K., and Kumarasinghe, L. (2014). A HRM real-time PCR assay for rapid and specific identification of the emerging pest spotted-wing Drosophila (Drosophila suzukii). PLoS One 9:e98934. doi: 10.1371/journal.pone.0098934
Dos Santos, L. A., Mendes, M. F., Krüger, A. P., Blauth, M. L., Gottschalk, M. S., and Garcia, F. R. M. (2017). Global potential distribution of Drosophila suzukii (Diptera, Drosophilidae). PLoS One 12:e0174318. doi: 10.1371/journal.pone.0174318
Edgar, R. C., and Flyvbjerg, H. (2015). Error filtering, pair assembly and error correction for next-generation sequencing reads. Bioinformatics 31, 3476–3482. doi: 10.1093/bioinformatics/btv401
Elbrecht, V., and Leese, F. (2017). Validation and development of COI metabarcoding primers for freshwater macroinvertebrate bioassessment. Front. Environ. Sci. 5:11. doi: 10.3389/fenvs.2017.00011
Elbrecht, V., and Steinke, D. (2019). Scaling up DNA metabarcoding for freshwater macrozoobenthos monitoring. Freshw. Biol. 64, 380–387. doi: 10.1111/fwb.13220
Elbrecht, V., Vamos, E. E., Meissner, K., Aroviita, J., and Leese, F. (2017). Assessing strengths and weaknesses of DNA metabarcoding-based macroinvertebrate identification for routine stream monitoring. Methods Ecol. Evol. 8, 1265–1275. doi: 10.1111/2041-210X.12789
Essl, F., Dullinger, S., Rabitsch, W., Hulme, P. E., Hülber, K., Jarošík, V., et al. (2011). Socioeconomic legacy yields an invasion debt. Proc. Natl. Acad. Sci. U. S. A. 108, 203–207. doi: 10.1073/pnas.1011728108
Ficetola, G. F., Pansu, J., Bonin, A., Coissac, E., Giguet-Covex, C., De Barba, M., et al. (2015). Replication levels, false presences and the estimation of the presence/absence from eDNA metabarcoding data. Mol. Ecol. Resour. 15, 543–556. doi: 10.1111/1755-0998.12338
Folmer, O., Black, M., Hoeh, W., Lutz, R., and Vrijenhoek, R. (1994). DNA primers for amplification of mitochondrial Cytochrome C Oxidase subunit I from diverse metazoan invertebrates. Mol. Mar. Biol. Biotechnol. 3, 294–299.
Galan, M., Pons, J. B., Tournayre, O., Pierre, É, Leuchtmann, M., Pontier, D., et al. (2018). Metabarcoding for the parallel identification of several hundred predators and their prey: application to bat species diet analysis. Mol. Ecol. Resour. 18, 474–489. doi: 10.1111/1755-0998.12749
Hamby, K. A., Bolda, M. P., Sheehan, M. E., and Zalom, F. G. (2014). Seasonal monitoring for Drosophila suzukii (Diptera: Drosophilidae) in California commercial raspberries. Environ. Entomol. 43, 1008–1018. doi: 10.1603/EN13245
Hardulak, L. A., Morinière, J., Hausmann, A., Hendrich, L., Schmidt, S., Doczkal, D., et al. (2020). DNA metabarcoding for biodiversity monitoring in a national park: screening for invasive and pest species. Mol. Ecol. Resour. 20, 1542–1557. doi: 10.1111/1755-0998.13212
Harris, D. W., Hamby, K. A., Wilson, H. E., and Zalom, F. G. (2014). Seasonal monitoring of Drosophila suzukii (Diptera: Drosophilidae) in a mixed fruit production system. J. Asia Pac. Entomol. 17, 857–864. doi: 10.1016/j.aspen.2014.08.006
Hauser, M. (2011). A historic account of the invasion of Drosophila suzukii (Matsumura) (Diptera: Drosophilidae) in the continental United States, with remarks on their identification. Pest Manag. Sci. 67, 1352–1357. doi: 10.1002/ps.2265
Hebert, P. D. N., Cywinska, A., Ball, S. L., and DeWaard, J. R. (2003). Biological identifications through DNA barcodes. Proc. R. Soc. B Biol. Sci. 270, 313–321. doi: 10.1098/rspb.2002.2218
Jaccard, P. (1908). Nouvelles recherches sur la distribution florale. Bull. Soc. Vaud. Sci. Nat. 44, 223–270.
Jara, C., Mateo, E., Guillamón, J. M., Torija, M. J., and Mas, A. (2008). Analysis of several methods for the extraction of high quality DNA from acetic acid bacteria in wine and vinegar for characterization by PCR-based methods. Int. J. Food Microbiol. 128, 336–341. doi: 10.1016/j.ijfoodmicro.2008.09.008
Jarrad, F. C., Barrett, S., Murray, J., Stoklosa, R., Whittle, P., and Mengersen, K. (2011). Ecological aspects of biosecurity surveillance design for the detection of multiple invasive animal species. Biol. Invasions 13, 803–818. doi: 10.1007/s10530-010-9870-0
Kanzawa, T. (1939). Studies on Drosophila suzukii Mats. Kofu: Yamanashi Prefecture Agricultural Experimental Station.
Kim, S. S., Tripodi, A. D., Johnson, D. T., and Szalanski, A. L. (2014). Molecular diagnostics of Drosophila suzukii (Diptera?: Drosophilidae) using PCR-RFLP. J. Econ. Entomol. 107, 1292–1294. doi: 10.1603/ec13389
Kim, Y. H., Hur, J. H., Lee, G. S., Choi, M. Y., and Koh, Y. H. (2016). Rapid and highly accurate detection of Drosophila suzukii, spotted wing Drosophila (Diptera: Drosophilidae) by loop-mediated isothermal amplification assays. J. Asia Pac. Entomol. 19, 1211–1216. doi: 10.1016/j.aspen.2016.10.015
Kuhn, M., and Wickham, H. (2020). Tidymodels: a collection of packages for modeling and machine learning using tidyverse principles. Available online at: https://www.tidymodels.org (accessed June 10, 2021).
Landolt, P. J., Adams, T., Davis, T. S., and Rogg, H. (2012). Spotted Wing Drosophila, Drosophila suzukii (Diptera: Drosophilidae), trapped with combinations of wines and vinegars. Fla. Entomol. 95, 326–332. doi: 10.1653/024.095.0213
Larson, N. R., Strickland, J., Shields, V. D. C., and Zhang, A. (2020). Controlled-release dispenser and dry trap developments for Drosophila suzukii detection. Front. Ecol. Evol. 8:45. doi: 10.3389/fevo.2020.00045
Lee, J. C., Burrack, H. J., Barrantes, L. D., Beers, E. H., Dreves, A. J., Hamby, K. A., et al. (2012). Evaluation of monitoring traps for Drosophila suzukii (Diptera: Drosophilidae) in North America. J. Econ. Entomol. 105, 1350–1357. doi: 10.1603/EC12132
Leray, M., and Knowlton, N. (2017). Random sampling causes the low reproducibility of rare eukaryotic OTUs in Illumina COI metabarcoding. PeerJ 5:e3006. doi: 10.7717/peerj.3006
Lindahl, T. (1993). Instability and decay of the primary structure of DNA. Nature 362, 709–715. doi: 10.1038/362709a0
Low-Choy, S. (2015). “Getting the Story Straight: Laying the Foundations for Statistical Evaluation of the Performance of Surveillance,” in Biosecurity Surveillance: Quantitative approaches, eds F. Jarrad, S. Low-Choy, and K. Mengersen (Wallingford: CABI), 43–73. doi: 10.1079/9781780643595.0043
Lozupone, C., and Knight, R. (2005). UniFrac: a new phylogenetic method for comparing microbial communities. Appl. Environ. Microbiol. 71, 8228–8235. doi: 10.1128/AEM.71.12.8228-8235.2005
Lundberg, D. S., Yourstone, S., Mieczkowski, P., Jones, C. D., and Dangl, J. L. (2013). Practical innovations for high-throughput amplicon sequencing. Nat. Methods 10, 999–1002. doi: 10.1038/nmeth.2634
Lynegaard, G. K., Offenberg, J., Fast, T. S., Axelsen, J. A., Mwatawala, M. W., and Rwegasira, G. M. (2014). Using insect traps to increase weaver ant (Oecophylla longinoda) prey capture. J. Appl. Entomol. 138, 539–546. doi: 10.1111/jen.12108
MacConaill, L. E., Burns, R. T., Nag, A., Coleman, H. A., Slevin, M. K., Giorda, K., et al. (2018). Unique, dual-indexed sequencing adapters with UMIs effectively eliminate index cross-talk and significantly improve sensitivity of massively parallel sequencing. BMC Genomics 19:30. doi: 10.1186/s12864-017-4428-5
Maclachlan, M. J., Liebhold, A. M., Yamanaka, T., and Springborn, M. R. (2021). Hidden patterns of insect establishment risk revealed from two centuries of alien species discoveries. Sci. Adv. 7:eabj1012. doi: 10.1126/sciadv.abj1012
Maino, J. L., Schouten, R., and Umina, P. (2021). Predicting the global invasion of Drosophila suzukii to improve Australian Biosecurity Preparedness. J. Appl. Ecol. 58, 789–800. doi: 10.1111/1365-2664.13812
Marquina, D., Andersson, A. F., and Ronquist, F. (2019a). New mitochondrial primers for metabarcoding of insects, designed and evaluated using in silico methods. Mol. Ecol. Resour. 19, 90–104. doi: 10.1111/1755-0998.12942
Marquina, D., Esparza-Salas, R., Roslin, T., and Ronquist, F. (2019b). Establishing arthropod community composition using metabarcoding: surprising inconsistencies between soil samples and preservative ethanol and homogenate from Malaise trap catches. Mol. Ecol. Resour. 19, 1516–1530. doi: 10.1111/1755-0998.13071
Martins, F. M. S., Galhardo, M., Filipe, A. F., Teixeira, A., Pinheiro, P., Paupério, J., et al. (2019). Have the cake and eat it: optimizing nondestructive DNA metabarcoding of macroinvertebrate samples for freshwater biomonitoring. Mol. Ecol. Resour. 19, 863–876. doi: 10.1111/1755-0998.13012
Martoni, F., Nogarotto, E., Piper, A. M., Mann, R., Valenzuela, I., Eow, L., et al. (2021). Propylene glycol and non-destructive DNA extractions enable preservation and isolation of insect and hosted bacterial DNA. Agriculture 11:77. doi: 10.3390/agriculture11010077
Mazzetto, F., Pansa, M. G., Ingegno, B. L., Tavella, L., and Alma, A. (2015). Monitoring of the exotic fly Drosophila suzukii in stone, pome and soft fruit orchards in NW Italy. J. Asia Pac. Entomol. 18, 321–329. doi: 10.1016/j.aspen.2015.04.001
McLaren, M. R., Willis, A. D., and Callahan, B. J. (2019). Consistent and correctable bias in metagenomic sequencing measurements. Elife 8:e46923. doi: 10.7554/eLife.46923
McMurdie, P. J., and Holmes, S. (2013). Phyloseq: an R package for reproducible interactive analysis and graphics of microbiome census data. PLoS One 8:e61217. doi: 10.1371/journal.pone.0061217
Murali, A., Bhargava, A., and Wright, E. S. (2018). IDTAXA: a novel approach for accurate taxonomic classification of microbiome sequences. Microbiome 6:140. doi: 10.1186/s40168-018-0521-5
Nguyen, N. H., Smith, D., Peay, K., and Kennedy, P. (2015). Parsing ecological signal from noise in next generation amplicon sequencing. New Phytol. 205, 1389–1393. doi: 10.1111/nph.12923
Nielsen, M., Gilbert, M. T. P., Pape, T., and Bohmann, K. (2019). A simplified DNA extraction protocol for unsorted bulk arthropod samples that maintains exoskeletal integrity. Environ. DNA 1, 144–154. doi: 10.1002/edn3.16
Palmer, J. M., Jusino, M. A., Banik, M. T., and Lindner, D. L. (2018). Non-biological synthetic spike-in controls and the AMPtk software pipeline improve mycobiome data. PeerJ 6:e4925. doi: 10.7717/peerj.4925
Piper, A. M., Batovska, J., Cogan, N. O. I., Weiss, J., Cunningham, J. P., Rodoni, B. C., et al. (2019). Prospects and challenges of implementing DNA metabarcoding for high-throughput insect surveillance. Gigascience 8:giz092. doi: 10.1093/gigascience/giz092
Piper, A. M., Cogan, N. O. I., Cunningham, J. P., and Blacket, M. J. (2021). Computational evaluation of DNA metabarcoding for universal diagnostics of invasive insect pests. bioRxiv [Preprint]. doi: 10.1101/2021.03.16.435710
Price, M. N., Dehal, P. S., and Arkin, A. P. (2009). Fasttree: computing large minimum evolution trees with profiles instead of a distance matrix. Mol. Biol. Evol. 26, 1641–1650. doi: 10.1093/molbev/msp077
R Core Team (2020). R: A language and environment for statistical computing. Vienna: R Foundation for Statistical Computing.
Reaser, J. K., Burgiel, S. W., Kirkey, J., Brantley, K. A., Veatch, S. D., and Burgos-Rodríguez, J. (2020). The early detection of and rapid response (EDRR) to invasive species: a conceptual framework and federal capacities assessment. Biol. Invasions 22, 1–19. doi: 10.1007/s10530-019-02156-w
Reed, M. R. (1938). The Olfactory Reactions of Drosophila melanogaster Meigen to the products of Fermenting Banana. Physiol. Zool. 11, 317–325. doi: 10.1086/physzool.11.3.30151465
Rennstam Rubbmark, O., Sint, D., Horngacher, N., and Traugott, M. (2018). A broadly applicable COI primer pair and an efficient single-tube amplicon library preparation protocol for metabarcoding. Ecol. Evol. 8, 12335–12350. doi: 10.1002/ece3.4520
Roe, A. D., and Sperling, F. A. H. (2007). Patterns of evolution of mitochondrial cytochrome c oxidase I and II DNA and implications for DNA barcoding. Mol. Phylogenet. Evol. 44, 325–345. doi: 10.1016/j.ympev.2006.12.005
Savary, S., Willocquet, L., Pethybridge, S. J., Esker, P., McRoberts, N., and Nelson, A. (2019). The global burden of pathogens and pests on major food crops. Nat. Ecol. Evol. 3, 430–439. doi: 10.1038/s41559-018-0793-y
Schnell, I. B., Bohmann, K., and Gilbert, M. T. P. (2015). Tag jumps illuminated - reducing sequence-to-sample misidentifications in metabarcoding studies. Mol. Ecol. Resour. 15, 1289–1303. doi: 10.1111/1755-0998.12402
Shannon, C. E. (1948). A mathematical theory of communication. Bell Syst. Tech. J. 27, 379–423. doi: 10.1002/j.1538-7305.1948.tb01338.x
Simmons, M., Tucker, A., Chadderton, W. L., Jerde, C. L., Mahon, A. R., and Taylor, E. (2016). Active and passive environmental DNA surveillance of aquatic invasive species. Can. J. Fish. Aquat. Sci. 73, 76–83. doi: 10.1139/cjfas-2015-0262
Singer, G. A. C., Fahner, N. A., Barnes, J. G., McCarthy, A., and Hajibabaei, M. (2019). Comprehensive biodiversity analysis via ultra-deep patterned flow cell technology: a case study of eDNA metabarcoding seawater. Sci. Rep. 9:5991. doi: 10.1038/s41598-019-42455-9
Smith, D. P., and Peay, K. G. (2014). Sequence depth, not PCR replication, improves ecological inference from next generation DNA sequencing. PLoS One 9:e90234. doi: 10.1371/journal.pone.0090234
Steinke, D., Braukmann, T. W. A., Manerus, L., Woodhouse, A., and Elbrecht, V. (2021). Effects of Malaise trap spacing on species richness and composition of terrestrial arthropod bulk samples. Metabarcoding Metagenom. 5:e59201. doi: 10.1101/2020.09.30.321430
Taberlet, P., Coissac, E., Pompanon, F., Brochmann, C., and Willerslev, E. (2012). Towards next-generation biodiversity assessment using DNA metabarcoding. Mol. Ecol. 21, 2045–2050. doi: 10.1111/j.1365-294X.2012.05470.x
Tavaré, S. (1986). Some probabilistic and statistical problems in the analysis of DNA sequences. Am. Math. Soc. Lect. Math. Life Sci. 17, 57–86.
Tedersoo, L., Drenkhan, R., Anslan, S., Morales-Rodriguez, C., and Cleary, M. (2019). High-throughput identification and diagnostics of pathogens and pests: overview and practical recommendations. Mol. Ecol. Resour. 19, 47–76. doi: 10.1111/1755-0998.12959
Tonina, L., Grassi, A., Caruso, S., Mori, N., Gottardello, A., Anfora, G., et al. (2018). Comparison of attractants for monitoring Drosophila suzukii in sweet cherry orchards in Italy. J. Appl. Entomol. 142, 18–25. doi: 10.1111/jen.12416
Vamos, E. E., Elbrecht, V., and Leese, F. (2017). Short COI markers for freshwater macroinvertebrate metabarcoding. Metabarcoding Metagenom. 1:e14625. doi: 10.3897/mbmg.1.14625
Van Timmeren, S., Diepenbrock, L. M., Bertone, M. A., Burrack, H. J., and Isaacs, R. (2017). A filter method for improved monitoring of Drosophila suzukii (Diptera: Drosophilidae) larvae in fruit. J. Integr. Pest Manag. 8:23. doi: 10.1093/jipm/pmx019
Walsh, D. B., Bolda, M. P., Goodhue, R. E., Dreves, A. J., Lee, J., Bruck, D. J., et al. (2011). Drosophila suzukii (Diptera: Drosophilidae): invasive pest of ripening soft fruit expanding its geographic range and damage potential. J. Integr. Pest Manag. 2, G1–G7. doi: 10.1603/ipm10010
Wang, Y., Naumann, U., Wright, S. T., and Warton, D. I. (2012). Mvabund- an R package for model-based analysis of multivariate abundance data. Methods Ecol. Evol. 3, 471–474. doi: 10.1111/j.2041-210X.2012.00190.x
Weigand, A. M., Desquiotz, N., Weigand, H., and Szucsich, N. (2021). Application of propylene glycol in DNA-based studies of invertebrates. Metabarcoding Metagenom. 5:e57278. doi: 10.3897/mbmg.5.57278
Whittle, P. J. L., Stoklosa, R., Barrett, S., Jarrad, F. C., Majer, J. D., Martin, P. A. J., et al. (2013). A method for designing complex biosecurity surveillance systems: detecting non-indigenous species of invertebrates on Barrow Island. Divers. Distrib. 19, 629–639. doi: 10.1111/ddi.12056
Wickham, H., Averick, M., Bryan, J., Chang, W., McGowan, L., François, R., et al. (2019). Welcome to the Tidyverse. J. Open Source Softw. 4:1686. doi: 10.21105/joss.01686
Wilcox, T. M., Zarn, K. E., Piggott, M. P., Young, M. K., McKelvey, K. S., and Schwartz, M. K. (2018). Capture enrichment of aquatic environmental DNA: a first proof of concept. Mol. Ecol. Resour. 18, 1392–1401. doi: 10.1111/1755-0998.12928
Wilkinson, S. (2019). aphid: an R package for analysis with profile hidden Markov models. Bioinformatics 35, 3829–3830. doi: 10.1093/bioinformatics/btz159
Willis, A., and Bunge, J. (2015). Estimating diversity via frequency ratios. Biometrics 71, 1042–1049. doi: 10.1111/biom.12332
Willis, A. D. (2019). Rarefaction, alpha diversity, and statistics. Front. Microbiol. 10:2407. doi: 10.3389/fmicb.2019.02407
Yang, C., Bohmann, K., Wang, X., Cai, W., Wales, N., Ding, Z., et al. (2021). Biodiversity Soup II: a bulk-sample metabarcoding pipeline emphasizing error reduction. Methods Ecol. Evol. 2021, 1252–1264. doi: 10.1111/2041-210X.13602
Yu, G., Lam, T. T. Y., Zhu, H., and Guan, Y. (2018). Two methods for mapping and visualizing associated data on phylogeny using ggtree. Mol. Biol. Evol. 35, 3041–3043. doi: 10.1093/molbev/msy194
Yu, G., Smith, D. K., Zhu, H., Guan, Y., and Lam, T. T. Y. (2017). ggtree: an R package for visualization and annotation of phylogenetic trees with their covariates and other associated data. Methods Ecol. Evol. 8, 28–36. doi: 10.1111/2041-210X.12628
Zhou, J., Jiang, Y. H., Deng, Y., Shi, Z., Zhou, B. Y., Xue, K., et al. (2013). Random sampling process leads to overestimation of β-diversity of microbial communities. mBio 4, e00324–13. doi: 10.1128/mBio.00324-13
Zinger, L., Bonin, A., Alsos, I. G., Bálint, M., Bik, H., Boyer, F., et al. (2019). DNA metabarcoding-Need for robust experimental designs to draw sound ecological conclusions. Mol. Ecol. 28, 1857–1862. doi: 10.1111/mec.15060
Keywords: biosurveillance, biosecurity, invasive species, DNA barcoding, non-destructive, early detection, metabarcoding, Drosophila suzukii
Citation: Piper AM, Cunningham JP, Cogan NOI and Blacket MJ (2022) DNA Metabarcoding Enables High-Throughput Detection of Spotted Wing Drosophila (Drosophila suzukii) Within Unsorted Trap Catches. Front. Ecol. Evol. 10:822648. doi: 10.3389/fevo.2022.822648
Received: 26 November 2021; Accepted: 31 January 2022;
Published: 04 March 2022.
Edited by:
Cesar Rodriguez-Saona, Rutgers, The State University of New Jersey, United StatesReviewed by:
Nicholas R. Larson, Beltsville Agricultural Research Center, Agricultural Research Service (USDA), United StatesKwaku Aduse-Poku, Georgia State University, United States
Copyright © 2022 Piper, Cunningham, Cogan and Blacket. This is an open-access article distributed under the terms of the Creative Commons Attribution License (CC BY). The use, distribution or reproduction in other forums is permitted, provided the original author(s) and the copyright owner(s) are credited and that the original publication in this journal is cited, in accordance with accepted academic practice. No use, distribution or reproduction is permitted which does not comply with these terms.
*Correspondence: Alexander M. Piper, alexander.piper@agriculture.vic.gov.au