- 1Department of Fish and Wildlife Sciences, University of Idaho, Moscow, ID, United States
- 2Retired, Fairbanks, AK, United States
- 3Institute of Arctic Biology and Department of Biology and Wildlife, University of Alaska Fairbanks, Fairbanks, AK, United States
- 4The Nature Conservancy, Juneau, AK, United States
- 5Department of Fish and Wildlife Biology, University of Montana, Missoula, MT, United States
- 6Department of Soil and Water Systems, University of Idaho, Moscow, ID, United States
- 7Retired, Braintree, VT, United States
Carnivores across much of the world are declining, leading to loss of biodiversity as well as the ecosystem services carnivores provide. In 2020, the Alexander Archipelago (AA) wolf was petitioned for protection under the U.S. Endangered Species Act (ESA) for the third time in 30 years. Concerns included habitat alteration from industrial timber harvest and subsequent declines in prey (deer), human-caused mortality, climate change, and genetic inbreeding. However, the underlying biogeography and ecology of these wolves continues to suggest resiliency across the subspecies’ range, even though local populations may go extinct. If local wolf populations go extinct, it will result in loss of their ecosystem services (e.g., interactions of wolves with their prey, which prevents over-browsing and protects carbon sequestration in soils and trees), which will likely have major consequences for the local social-ecological system. Here, we updated a model we constructed for the last ESA listing process (2015) to examine the dynamics of wolf and deer populations on Prince of Wales Island (the primary geographic focus of all three petitions) in response to future environmental and management scenarios developed with stakeholders. Further, we considered how changes in deer abundance impact predation services (prevention of over-browsing by deer). We found that wolf populations generally persisted over 30 years, but dropped below an effective population size of 50 wolves in 10–98% of years simulated. Low wolf abundance resulted in higher deer abundance, which increased hunting opportunity, but also browsing damages (e.g., 19% of areas would be over-browsed if wolf harvest caps are removed, and >30% of areas would be over-browsed if wolves go extinct). Human harvest of wildlife was a key regulator of abundance and ecosystem services within the coastal rainforest social-ecological system; wolf abundance was most affected by wolf harvest regulations; and deer harvest restrictions increased wolf and deer abundances, but also greatly increased browsing impacts (>70% of areas heavily browsed if hunting ceased). Our findings support an integrated approach to management of this social-ecological system, such that social and ecological sciences are both used to monitor important components of the system (e.g., measuring public sentiment and likelihood of poaching, alongside wolf and deer numbers). Integration and adaptive approaches are needed to ensure that the many ecosystem services humans depend on are valued, conserved, and restored, including the cryptic predation services wolves have historically provided to the timber industry via reduced browsing pressure by deer.
Introduction
Most top carnivore species around the world are in decline (Ripple et al., 2014), with corresponding losses not only of biodiversity but also of “predation services” that regulate the abundance and behavior of prey species that have net negative effects on people and ecosystems (Braczkowski et al., 2018; O’Bryan et al., 2018; Gilbert et al., 2021a). However, in many parts of Europe and North America, large carnivore populations are making a comeback due to increased legal protection, and in some cases increasing tolerance, for predatory wildlife (Treves et al., 2013; Dressel et al., 2015; Manfredo et al., 2021b), leading to heated debate about whether carnivores should be lethally managed to reduce damages, enjoy continued protection under laws such as the U.S. Endangered Species Act (ESA), or be actively re-introduced to new areas (Manfredo et al., 2021a). These divergent desires regarding carnivore recovery are likely due in part to the fact that those who experience or perceive negative effects of predation (e.g., some ranchers and hunters) are not the same people that experience or perceive the positive services of predation (e.g., environmentalists, wildlife viewers, range and forest land managers; Gilbert et al., 2021a; Manfredo et al., 2021a).
In contrast to trends on the mainland of North America, wolves in some portions of the coastal temperate rainforests of Alaska (Canis lupus ligoni) have declined from historical levels due to many of the same factors that are driving global carnivore declines: reductions in prey base following habitat, alteration and human harvest (Person and Brinkman, 2013; Gilbert et al., 2015). As a result, the Alexander Archipelago (AA) wolf has been petitioned for listing under the ESA three times in the past 30 years, including a recent petition filed in 2020 that is currently under 12-month review (U.S. Fish and Wildlife Service [USFWS], 2020), with concerns focused on the population of wolves living on Prince of Wales Island. Over the past century, timber harvest and associated development has dramatically altered coastal temperate rainforests of the Pacific Northwest of North America (DellaSala et al., 2011), a highly productive region that provides many ecosystem services (Brandt et al., 2014). As a result, the coastal temperate rainforest has become a focus of controversy for both the timber industry and conservation interests, with AA wolves one of the most contentious species. As is common with many wolf populations (Musiani and Paquet, 2004), public perceptions of AA wolves range widely, from wolves being undesirable due to negative cultural stereotypes and real and perceived competition with deer hunters (Brinkman et al., 2007; Liberg et al., 2012; Person and Brinkman, 2013; Pohja-Mykrä and Kurki, 2014; Bradley et al., 2015), to high conservation concern for the persistence of AA wolves in the face of various impacts, including a reduction in prey (Person and Brinkman, 2013) and potential genetic inbreeding following heavy human harvest (Center for Biological Diversity, Alaska Rainforest Defenders, and Defenders of Wildlife 2020).
While the causes of AA wolf declines are varied, most pathways directly or indirectly lead back to human actions driven by timber harvest. The impacts to deer habitat have manifested through large scale old-growth logging and subsequent forest succession. Massive old-growth logging in the last decades of the twentieth century and subsequent forest succession to lower-productivity, older second-growth stages (Alaback, 1982) has reduced the habitat carrying capacity for Sitka black-tailed deer (Odocoileus hemionus sitkensis), the primary food source for AA wolves. Timber harvest also increases the density of road networks, providing greater access for wolf hunting and trapping (Person and Russell, 2008; Person and Logan, 2012; Figure 1). In addition, industrial logging compacts soils, which can reduce seedling recruitment and forage quality by disrupting plant-soil-microbe feedbacks. Given strong links to deer and wolf populations, high levels of timber harvest likely reduce the long-term viability of wolf populations, the stability of predator-prey dynamics, and ecosystem resiliency in Southeast Alaska (Person et al., 1996; Person and Brinkman, 2013; Roffler et al., 2018).
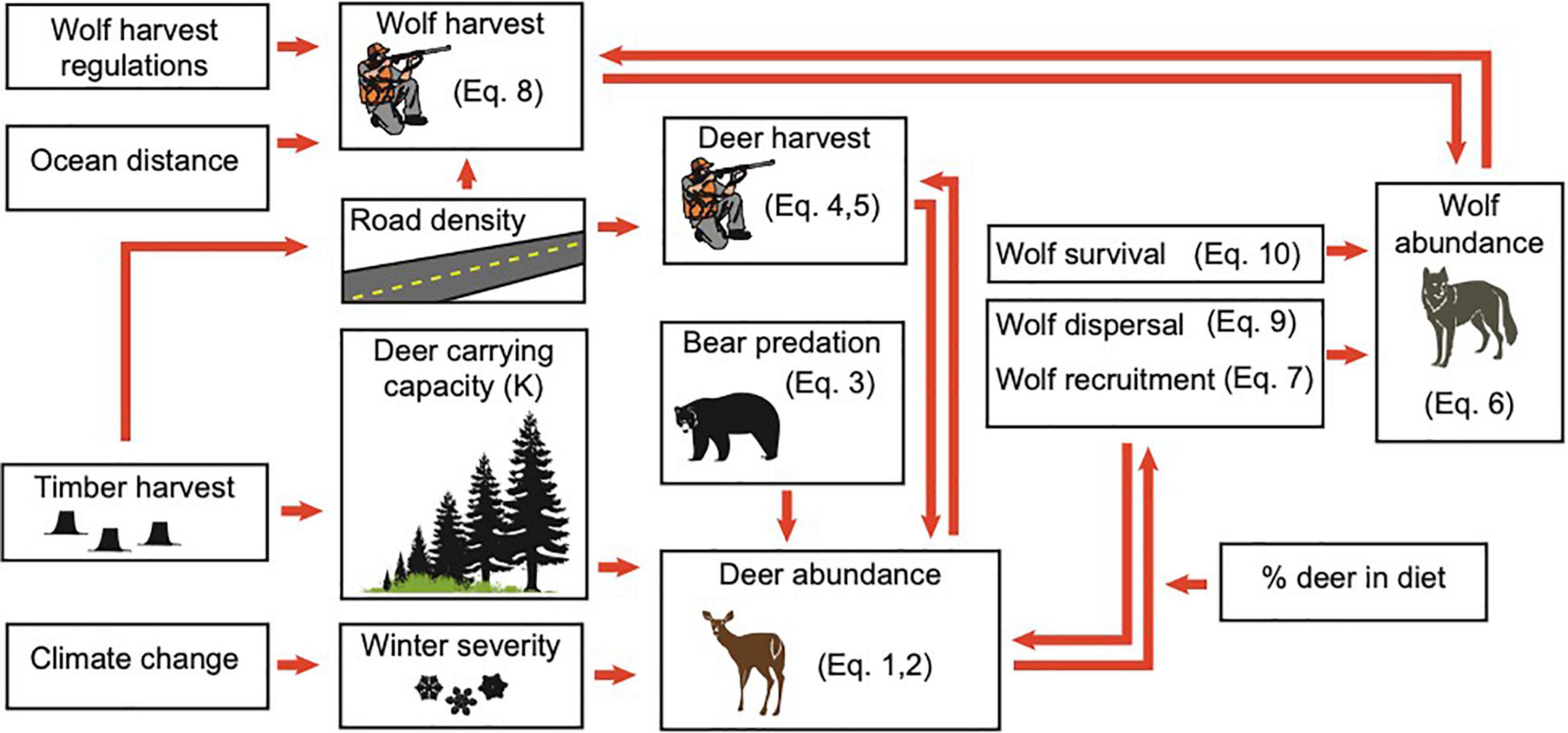
Figure 1. Relationship among primary model components, based on connections among major environmental drivers of wolf abundance in the coastal temperate rainforest of Southeast Alaska. Equation (Eq.) numbers reference Supplementary Appendix A.
Prince of Wales (POW) and adjacent outlying islands support a significant percentage of the wolves in Southeast Alaska (Person et al., 1996), and the POW population has declined sharply in recent years (U.S. Fish and Wildlife Service [USFWS], 1997; Alaska Department of Fish and Game [ADFG], 2015a; Alaska Department of Fish and Game [ADFG], 2017). In 1993, a petition was filed for protection of the AA wolf under the ESA, but ultimately the U.S. Fish and Wildlife Service (USFWS) determined that listing was not warranted because declines would stop within an acceptable level (U.S. Fish and Wildlife Service [USFWS], 1997). In 2011, another ESA petition was filed, citing the same primary threats but adding climate change and inadequate harvest regulatory mechanisms as additional pressures (Center for Biological Diversity and Greenpeace, 2011; U.S. Fish and Wildlife Service [USFWS], 2014).
At the request of the USFWS, we created a population model in 2014–2015 for wolves on POW and the surrounding islands to inform the listing decision (U.S. Fish and Wildlife Service [USFWS], 2014; Gilbert et al., 2015). The purpose of our analysis was to develop a model best representing our current understanding of predator-prey dynamics on POW, then assess the effects of major stressors on future wolf abundance. We evaluated six possible scenarios spanning a range of likely conditions for future timber harvest, silvicultural treatments that affect forest succession, deer abundance, road building and closures, and wolf harvest regulations. We also conducted sensitivity analyses to measure the relative influence of these factors on wolf and deer abundances. While our model identified a number of scenarios in which wolves on POW could decline to low levels in the future, the USFWS declined to list AA wolves in their 12-month finding (2015), because the AA wolf was not deemed threatened through all or a portion of its range (all of Southeast Alaska and northern British Columbia), and the POW population was not deemed ecologically or genetically unique enough to qualify as a distinct population segment under the ESA (U.S. Fish and Wildlife Service [USFWS], 2015). The current petition, rather than focusing on the POW population, requests that AA wolves throughout Southeast Alaska be listed as a threatened, distinct population segment within the broader sub-species range in coastal Canada and Alaska. However, POW’s wolves are again at the heart of the petition, in part because recent intensive harvests wolf harvest in recent years may have led to inbreeding depression (Zarn, 2019).
Regardless of the outcome of the current ESA listing decision, wolves on POW may go functionally or completely extinct, with potential for profound but poorly recognized impacts to the social-ecological system (the ESA does not require consideration of economic impacts, even if the wolves were listed). What would a “world without wolves” look like on POW? AA wolves clearly are valued by some residents and non-residents, and provide a number of “predation services and disservices” via their consumption of deer, the only significant ungulate herbivore in the ecosystem.
Fortunately, a wolf-free comparison is available for consideration on Haida Gwaii, the large island complex just south of POW in British Columbia. Sitka black-tailed deer are not native to Haida Gwaii, but were introduced to the northern portion of the archipelago ∼100 years ago, after which they spread rapidly across much of the archipelago. They currently sustain densities of ∼15–35 deer/km2(or almost 40 deer/square mile). Despite having drastically depleted understory biomass and impacted soils (Gaston et al., 2006; Le Saout et al., 2014), deer remain at high densities and continue to suppress plant recruitment and growth, potentially by consuming lichens that blow down from old-growth forest canopies in fall and winter, when deer would otherwise be nutritionally limited by terrestrial plant biomass (Le Saout et al., 2014). Palatable plants, such as huckleberry, and commercial and culturally important tree species, such as western red cedar (Thuja plicata), have been almost entirely removed by browsing where deer are present. Even less palatable conifer species, such as Sitka spruce (Picea sitchensis) exhibit stunted growth by up to two decades by browsing pressure compared to deer-free areas, until they are finally able to reach a height where they “escape” browsing (Bachand et al., 2014).
Browsing disservices not only damage the forestry industry by delaying timber rotations for high-value cedar and other conifers, but also impact Haida and Tlingit tribal members living on POW who value cedar and huckleberry for their traditional uses (Norton, 1981; Moss, 2004; Benner et al., 2021). Over-browsing can negatively impact habitat availability, the biodiversity of plants, birds, and invertebrates (Maillard et al., 2021), and ecosystem resiliency. Preferential consumption of higher-quality plant lower (lower C to nitrogen) by deer may also reduce microbial productivity and the delivery of critical nutrients, such as nitrogen, to the soil (Maillard et al., 2021). Forest carbon sequestration could decline if nutrient limitation stimulates microbial respiration, although abundant deer may offset carbon losses through greater soil compaction. In addition to ecosystem services, deer are a regionally prized game species for both sport and subsistence hunters (Brinkman et al., 2009; Colson et al., 2013), and more abundant deer provide more hunting opportunities. As a result, wolves exert both a predation disservice, by reducing hunting opportunities, and a predation service, by increasing the abundance of desirable conifer species and provisioning other ecosystem benefits that are difficult to quantify and often overlooked (Martin et al., 2020; Maillard et al., 2021). Critically, wolves prey on deer wherever they co-occur within the remote, rugged landscapes of the temperate rainforest, whereas human hunters have a strong preference for landscapes with easy boat and road access and high sightability (Brinkman et al., 2009). Therefore, even if deer hunting regulations were drastically loosened or the number of hunters increased greatly, it is unlikely that human hunting could provide an equivalent deer regulatory service over the entirety of the study area. For example, despite extremely generous hunting regulations on wolf-free Haida Gwaii (>15 deer per hunter per year), hunters are unable to regulate deer numbers and negative impacts of deer persist. Indeed, the Canadian government recently spent millions of dollars hiring professional hunters from New Zealand to remove deer from several small islands that are part of the Haida Gwaii archipelago to produce deer-free biodiversity refuges (Anthony, 2019).
Here, we update our 2015 model to consider loss of predation services, via increased browsing pressure, and discuss the implications for AA wolf conservation and social-ecological feedbacks on POW and elsewhere.
Materials and Methods
Study Area
We focus on the POW region (Figure 2) because it has historically supported a large, and relatively isolated (Weckworth et al., 2005; Breed, 2007; Cronin et al., 2015; Zarn, 2019) portion of the AA wolf population in Southeast Alaska (Person et al., 1996) and has a high concentration of stressors that could lead to local extinction, including intensive timber harvest, road density, and easy boat access (Person, 2001). This study region was also a focal area in the status assessment conducted in 2015 by the USFWS for consideration of listing of AA wolves under the Endangered Species Act (Gilbert et al., 2015). The POW region (Figure 2) has a mean annual precipitation of > 300 cm which produces a diversity of temperate rainforest habitats including old-growth forest types, alpine and subalpine vegetation above ∼400 m, and muskeg heaths (Farmer and Kirchhoff, 2007; Alaback and Saunders, 2013). Industrial timber harvest has dramatically altered old-growth forests and removed disproportionate amounts of commercially valuable forest from the study area relative to the region as a whole (Albert and Schoen, 2013). Albert and Schoen (2007) estimate that 40% of the productive forest land on North POW and 9% on South POW has been logged.
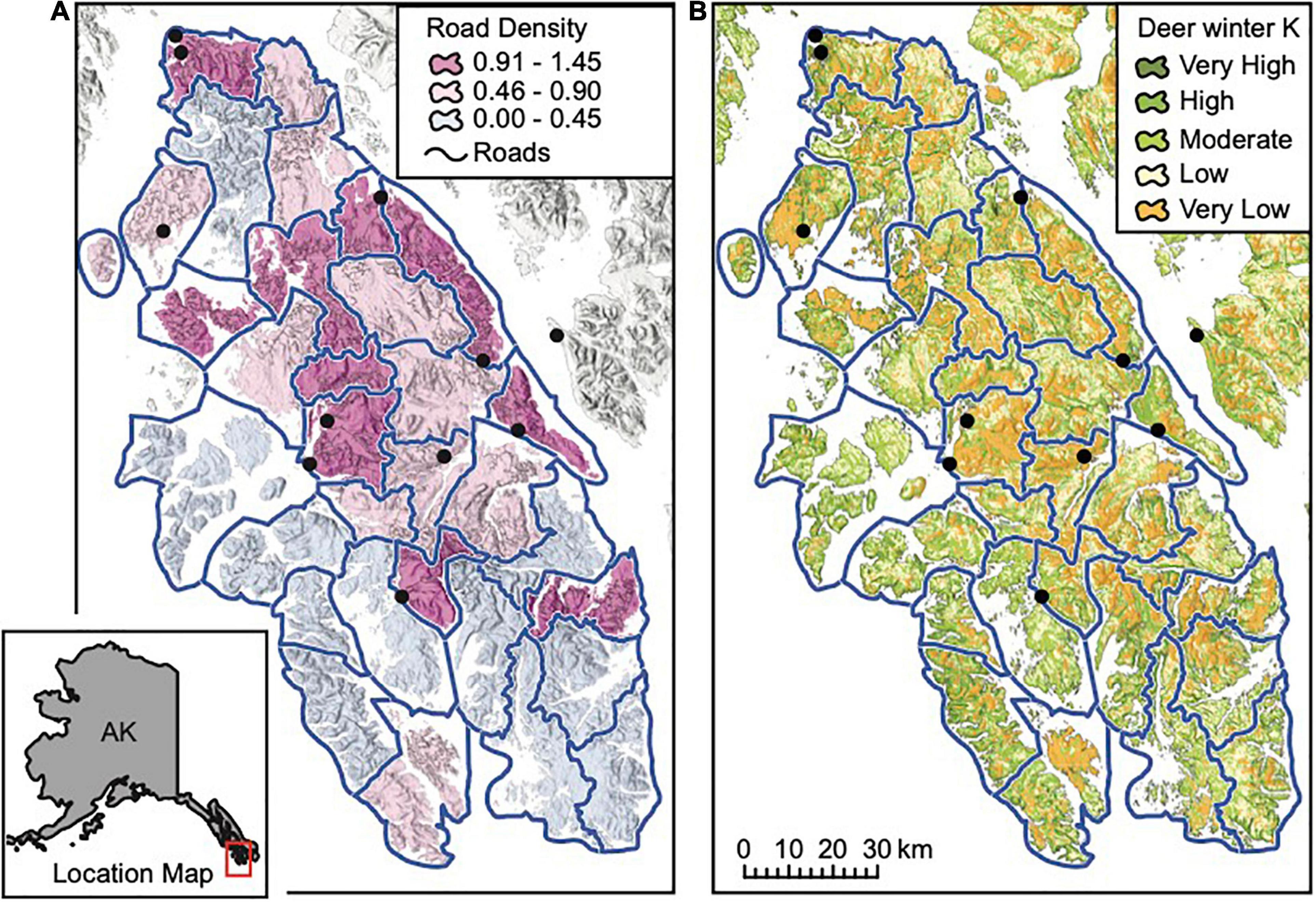
Figure 2. The study area, located on Prince of Wales and outlying islands in Southeast Alaska; pack areas in the wolf population model are outlined in blue and towns are shown as black circles. Maps display (A) road density in km per km2 in each pack area, and (B) deer winter carrying capacity (K) based on the interagency deer habitat suitability index, which ranges from very high (76–130 deer per mi2), to high (43–75 deer per mi2), moderate (23–42 deer per mi2), low (7.1–22 deer per mi2), and very low (0–7 deer per mi2).
The diet of POW wolves is diverse compared to their continental counterparts, and includes a greater marine component, although deer remain the most important prey item (Szepanski et al., 1999). The primary drivers of deer population dynamics in Southeast Alaska include winter severity, habitat quality, predation by wild carnivores, and harvest by humans (Figure 1). During winter, deep snow impacts deer by increasing the cost of movement (Parker et al., 1984), burying forage (Parker et al., 1999; White et al., 2009), reducing landscape connectivity, foraging efficiency, and by habitat carrying capacity (Kirchhoff, 1994; Parker et al., 1999; Hanley et al., 2012; Gilbert et al., 2017). Consequently, deer populations can decline sharply in or following severe winters (Farmer et al., 2006; Person et al., 2009; Brinkman et al., 2011; Gilbert et al., 2020).
Industrial timber harvest has diminished habitat quality for deer by converting structurally diverse old-growth forests to even-aged stands throughout much of Southeast Alaska, and especially in the southern portions of the Alexander Archipelago, including POW. Young and old second-growth has been associated with increased mortality risk for deer due to increased hunting and malnutrition (Farmer et al., 2006; Person et al., 2009) and old second-growth landscapes the lowest deer densities after consecutive severe winters (Brinkman et al., 2011). Landscapes dominated by second-growth habitats are likely to have lower deer populations, which in turn negatively affects wolf densities.
In addition to acting as primary prey of wolves, deer are also an important resource for both humans and bears (Figure 1). Much of the deer harvest in Southeast Alaska is concentrated on POW due to the ease of access on the widespread road system created by timber harvest, relatively high deer densities, and liberal harvest regulations (Brinkman et al., 2009). In addition to human harvest, deer populations experience mortality due to predation on fawns occasionally by eagles (Gilbert, 2016) and more frequently by black bears (Ursus americanus; Gilbert, 2015).
Modeling Approach
We based our model on a pre-existing population model for wolves on POW and surrounding small islands. This model was originally created by Person and Bowyer (1997), refined in 2001 (Person, 2001) henceforth the “2001 model”), and updated via changes to some model parameters to evaluate new scenarios in 2015 (Gilbert et al., 2015). Here, we further update the 2001 model, making our code, data, and approach fully public and reproducible for the first time. Our 2015 efforts were not published in a peer-reviewed journal, although the USFWS did send the model to outside experts for peer review. Therefore, this analysis is in many ways the same as our 2015 efforts, but with parameter improvements based on current best available science and the 2015 outside reviewers’ comments. We also expand the scenarios considered, and include ecosystem service considerations.
The 2001 model used data specific to wolves in the study area when available, as well as data and relationships from studies of wolves and deer in other ecosystems when data specific to POW were not available. The 2001 model exhibited good performance for describing population dynamics when validated on other systems with more complete data, such as deer at the George Reserve, wolves on Coronation Island, and wolves and moose at Isle Royale (Person et al., 2001). We updated the 2001 model using new data from Southeast Alaska to better parameterize variables (Supplementary Table 1).
Using our updated model, we simulated the effects of a range of plausible environmental changes on wolf abundance. The basic structure of the model includes the major factors expected to influence future wolf abundance: changes to deer carrying capacity, projections of winter severity, and harvest rates of wolves by humans. Secondary drivers are considerably more complex (Figure 1), resulting in relationships that are either one-way, or involve density-dependent feedback loops. We describe in detail the equations used to structure the wolf population model, as well as updates made to the model’s parameters in Supplementary Appendix A, and list new data sources in Supplementary Table A1. Below, we describe the basic model structures.
We modeled wolf population dynamics as a cumulative sum of dynamics of 31 hypothetical, spatially explicit, contiguous wolf “packs” (Figure 2). Each pack’s home-range was represented by a polygon with a mean size of 303 km2 (SD = 87), which is in line with empirical estimates of wolf home-range sizes, ranging from 260 km2 (SE = 48; Person, 2001) to 535 km2 (Alaska Department of Fish and Game [ADFG], 2015b). Although we use the term “packs” to describe wolves in each polygon, wolves in a polygon do not have to be organized into a single pack, although the dynamics of all packs in a given polygon are linked. We recognize that wolf pack territories and home ranges are dynamic and our pack areas are modeled as static. We used the same pack polygon boundaries established by Person (2001) and assumed a closed population of wolves in the study area, although we allowed wolves to disperse among all pack polygons without geographic restrictions. Dispersal of wolves among packs allowed packs to be more realistically dynamic in terms of recovery from local extinction, because new wolves could colonize from other packs. Demographic rates for wolves in our model, particularly recruitment and mortality, are regulated by the ratio of deer to wolf abundance in pack areas. In addition, those demographic rates are given wide distributions such that they can accommodate hypothetical changes in numbers of packs within pack areas. For example, if the ratio of deer to wolves is high, recruitment to wolves also can be high. In this example, our model would accommodate either a single pack with a large litter of pups, or a situation in which a second breeding pair establish a territory within the pack area, although such social dynamics are not modeled explicitly. We allow a 2-year time lag for the effects of deer/wolf ratios to be fully manifested within a pack to account for asynchrony between deer and wolves. A limitation of our model is it does not model the distribution of those resources at a finer resolution than the pack area. For example, if high quality habitat for deer is concentrated along a border between pack areas, a small change in area boundaries could have a large effect on which group sequesters those deer. Consequently, our model likely underestimates the potential variation in the ratio of prey to wolves and in wolf-deer predator-prey dynamics.
Model Structure
Within each wolf pack area i, annual wolf numbers at time t are described using the formula:
where Pt(i) is the size of the wolf pack prior to parturition in pack area i, Rt(i) is recruitment, Tt(i) is wolves harvested, Dt(i) is dispersal, Mt(i) is natural mortality, and It(i) is immigration from other packs. Recruitment, natural mortality, and dispersal probability were modeled as density dependent, based on the ratio of deer available to deer consumed for each wolf pack (Supplementary Appendix A, Equations A.7, A.9, and A.10). We considered natural mortality and dispersal to be compensatory with mortality from human harvest (i.e., human harvest reduces rates of natural mortality and dispersal; Person and Bowyer, 1997). Human harvest of wolves for each pack was determined based on road density and distance via ocean from the nearest human settlement (Person and Russell, 2008; Supplementary Appendix A, Equation A.8). Individual wolf packs affect overall wolf pack dynamics by contributing dispersing wolves to a population wide “dispersal pool” in a density-dependent manner. Wolves that enter the dispersal pool could colonize vacant pack areas. We modified the 2001 model so that if wolves in the dispersal pool did not colonize a vacant pack area in year t, individuals in this pool had an annual survival probability of 0.34 (SE = 0.17) (Person and Russell, 2008).
The deer sub-model is a component of the wolf population model that represents prey resource dynamics in the pack areas (details in Supplementary Appendix A). The deer population (Ut(i)) at time t was calculated as:
Where Ruti is recruitment into the deer population in pack area i, BAti is predation mortality of adult deer by black bears, CPati is predation mortality of deer by wolves, and Hti is harvest mortality from human hunting. C, the per-capita wolf predation rate (15 deer/wolf/year) was based on a wolf diet estimate for POW from a stable isotope analysis (Szepanski et al., 1999) and thus represents a conservative minimum number of deer killed, given that wolves do not necessarily consume the entire deer carcass. Pati is the average of spring and fall population sizes of wolves in year t. Similar to the wolf model, the deer model was density-dependent. Recruitment scaled with proximity of the deer population in pack area i to the carrying capacity of deer in that pack area (Supplementary Material 1, Equation 2), and was assumed to have failed completely if a severe winter occurred, as severe winters impact fawns far more than adults (Gilbert et al., 2021a,b). Predation of fawns by black bears was also modeled as density-dependent, with the percentage of compensatory mortality increasing as the deer population approached carrying capacity, K (Supplementary Material 1, Equation 3). We predicted deer carrying capacity in each pack area from the deer habitat capability index (deer HCI; originally developed by Suring et al., 1993, and updated by an interagency deer team intermittently), which estimates the maximum number of deer that can be nutritionally supported during winter in a specified area (United States Forest Service, 2008). We treated adult deer mortality due to hunting, and predation by black bears and wolves as completely additive. Hunting mortality on deer was a function of road length, based on a published regression relationship between road length and reported harvest (Person and Bowyer, 1997; Supplementary Material 1, Equation 5), although importantly this equation is not sex or age specific, and specifies no maximum capacity for hunting in terms of demand or number of total hunters and their effort, other than the total population of deer available in a WAA. Person and Bowyer (1997) found no difference in performance of more complex sex- and age-structured models vs. an unstructured model, so we retained their equation. Potential biases produced by this equation are over-estimation of deer hunters’ ability to harvest deer and resulting hunting services and wolf disservices, as real-world harvest is heavily male-biased.
Predation services and disservices via deer reductions were based on impacts to cedar recruitment and delays in conifer regrowth on Haida Gwaii, and predicted levels of hunting (see above for potential biases in hunting). However, because the deer HCI model generally predicts relatively low deer densities, well below the 30 deer/km2 density at which severe browse impacts to conifers were observed on Haida Gwaii, and is a predictor of habitat value to deer rather than absolute deer densities, we instead assumed that deer browse impacts would occur if deer density exceeded 90% of carrying capacity (K).
Scenario Development
We developed six scenarios for analysis that spanned a range of possible future conditions on POW. The conditions for each scenario were based on proposed or planned land use and resource management actions, as well as on future climate possibilities for the region, downscaled from global climate models (i.e., winter severity frequency, Supplementary Material 1). We developed the scenarios during a technical model review workshop (Anchorage, AK, March 18–19, 2015) that included participants from key management agencies, along with technical experts in population modeling, spatial analysis, and wolf ecology.
Scenarios included likely future changes to timber harvest, road building or closures (i.e., decommissioning), effects of climate change on frequency of severe winters, and wolf harvest regulations. We considered changes to vegetation based on five potential timber harvest conditions: (1) no future timber harvest after 2014 (i.e., forest successional change only); (2) a transition to harvest of second-growth forest on Tongass National Forest lands (i.e., the young growth transition currently in planning by the U.S. Forest Service); (3) continued harvest of old-growth at the rates observed from 2000 to 2014; (4) increased harvest of old-growth forest at the rates observed from 1995 to 2000; and (5) maximum harvest of old-growth forest allowable under the 2008 Tongass Land Management Plan. We also varied the rate of future timber harvest assumed to occur on non-federal lands among these alternatives. Details and assumptions associated with these possible future vegetation conditions are included in Supplementary Material 2.
We considered five alternative conditions for road construction and decommission: (1) no change in total road length from 2014 levels; (2) road decommissioning at levels in current management plan (i.e., –2.2% total road length, implemented during 2015–2025; United States Forest Service, 2009); (3) road decommissioning at increased levels (i.e., –28.7% total road length, implemented during 2015–2025); (4) road decommissioning at maximum levels (i.e., –232% total road length, implemented during 2015–2025); and (5) road construction necessary to access new old-growth harvest areas if the maximum old-growth harvest scenario takes place (i.e., 30% increase in total road length). We calculated road construction necessary to access new old-growth based on a regression relationship between existing total road length and acres of timber harvest in the wolf pack areas (United States Forest Service, 2008). We used the resulting slope (i.e., regression coefficient, β = 0.0385, SE = 0.0026, R2 = 0.88), which specifies 0.0385 km of road construction per hectare of new logging.
Wolf harvest regulations included in the scenarios ranged from complete closure of regulated harvest (0% reported harvest) to closure of harvest within a harvest season if reported harvest exceeded a fixed percentage (20 and 30%) of the previous fall population (i.e., a harvest “cap”). In addition, Person and Russell (2008) found that 13 of 31 (42%) wolves harvested by humans were not reported. As a result, we use regression relationships to predict reported harvest based on road density and distance to nearest town via ocean (see Supplementary Material 1, equation 8), then multiplied the result by an unreported harvest scalar of 1.72, equivalent to total harvest (n = 31) divided by reported harvest (n = 18), then applied a harvest cap to the reported portion of the predicted harvest for each pack if the cap threshold for a scenario was exceeded at the population level.
Combining these factors, along with possible future frequencies of severe winters (Supplementary Material 1), we created six scenarios with input from workshop participants for evaluation (Table 1). Across scenarios, we hypothesized that Scenario A would be most favorable for wolf abundance and resilience of the predator-prey community, Scenario B would be the most likely under current agency policy, Scenario E would be least favorable for wolf abundance, and Scenarios C and D are intermediates between A and E in terms of favorability for wolves. We also included a No New Action scenario, which represented ongoing changes in forest succession and habitat values from past logging, with no additional change or management action in the future (Table 1). We chose a 30-year timeframe (2015–2045) for model simulations because it encompassed enough years for the population dynamics of long-lived animals such as deer and wolves to stabilize and respond to environmental change but was short enough to minimize uncertainty associated with future management, climate, and socioeconomics. For each scenario, we conducted 1,000, 30-year simulations of the wolf-deer model.
Sensitivity Analysis
To isolate how scenarios are affected by changes to vegetation, road length, frequency of severe winters, and wolf harvest regulations, we perturbed each of these variables separately across the range of values found in the scenarios, while holding all other variables at Scenario B values. As with the primary scenarios analysis, for each parameter perturbation, we conducted 1,000, 30-year simulations of the wolf-deer model. We also tested model sensitivity to wolf diet composition, examining wolf diets comprised of 15 deer/wolf/year used in scenarios (i.e., 45% deer in the diet) vs. 9.5 deer/wolf/year (i.e., 28% deer in the diet), 20.5 deer/wolf/year (i.e., 60% deer in the diet), or 26 deer/wolf/year (i.e., 77% deer in the diet; the value used by Person, 1996). Finally, we explored the effects of changing deer harvest in the study area, by considering the most extreme alternative: no deer harvest. We included this condition because the model could not realistically quantify the effects of different levels of deer harvest in its current form (i.e., a model with a single sex and adult age class). We included a wolf harvest perturbation of no cap on harvest (i.e., harvest depends on environmental predictors only), because ADFG shifted harvest regulations to a non-capped system in recent years, resulting in high harvest rates (Alaska Department of Fish and Game [ADFG], 2021). As with scenario results, we present results of sensitivity analysis as a percent change in abundance over 30 years from 2014 levels (i.e., total change by 2045) and base interpretation on relative comparisons.
Quasi-Extinction Calculations
We investigated effects of six possible scenarios on the probability that wolf abundance would fall below a quasi-extinction (QE) threshold (i.e., abundance that is so low that the population is at significant risk of extinction). The 50–500 rule is commonly used in conservation genetics to determine the QE threshold (Franklin, 1980). This rule suggests that an effective population size (Ne; number of animals contributing genes to the next generation) of at least 50 is needed for short-term population viability, whereas an Ne of 500 or more is needed to ensure long-term viability (Laikre et al., 2016). Among wolves, only a fraction of the population breeds, so that Ne is lower than total abundance, N (Aspi et al., 2006; Laikre et al., 2016). We therefore assume a ratio of Ne/N of 0.42 (i.e., only 42% of wolves pass on genes to the next generation), as has been recorded for Finnish wolves, an analogously small and isolated population (Aspi et al., 2006; Laikre et al., 2016). Using this ratio, we calculated that to achieve Ne = 50, an N = 119 was needed, whereas to achieve Ne = 500, N must equal 1,190 wolves, far beyond likely historical wolf abundance on POW. We calculated the percentage of years under each scenario that wolf abundance will drop below 119 wolves to assess how frequently the POW wolf population would drop below the QE threshold. We chose this approach, rather than simply counting number of population realizations where N < QE, because the low initial starting size of the population (i.e., 89 wolves) ensures that almost all populations start below the QE threshold.
Results
Scenario Development
Between 1995 and 2015, the composition of forests logged in the study area shifted from primarily young growth to primarily old second-growth, despite continued but slowed harvest of remaining old-growth forests (Supplementary Table 3). These changes represent a decline of approximately 13% in carrying capacity (K) for deer from 1995 to 2015. By 2045, we project that 100, 86, 89, 90, and 93% of cut forests will have transitioned to old second-growth in No New Action, Scenario A, Scenario B, Scenario C, Scenario D, and Scenario E, respectively (Supplementary Table 3). Under the “No New Action” scenario and Scenario A, we estimated an additional decline of 6% in deer K by 2045, with larger projected declines under Scenario B (–9%), Scenario C (–11%), Scenario D (–14%), and Scenario E (–17%).
Scenario Outcomes
From the low, empirically estimated starting abundance of 89 wolves in fall of 2014 (95% CI = 50, 159; (Alaska Department of Fish and Game [ADFG], 2015a), the projected wolf population in 2045 increased in four scenarios and decreased in two (range: –35 to 284%) and deer abundance declined across all scenarios (range: –9 to –36%; Table 2 and Figures 3, 4). Across scenarios, wolf abundance dropped below an effective population size of 50 wolves in 10–98% of years simulated. Deer browsing damage to conifers varied across scenarios and through time, with 0–16% of wolf home ranges impacted by heavy browsing by 2045, whereas deer hunting opportunity declined across scenarios from 19 to 60% (Table 2), although only some of this loss is attributable to wolves (see sensitivity analysis, below), because deer abundance declined in general due to dwindling habitat carrying capacity.
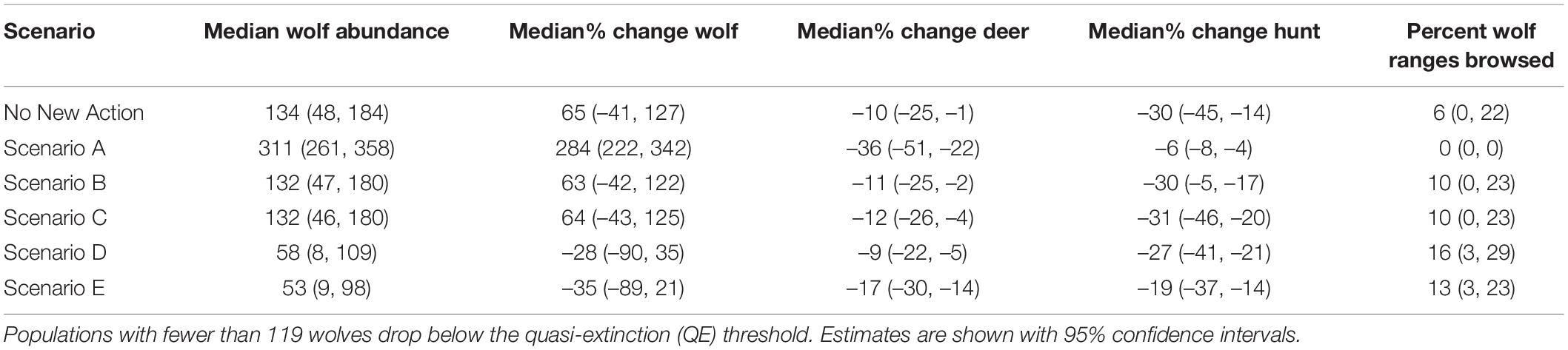
Table 2. Modeled changes from 2015 to 2045 in spring abundance of wolves, deer, and predation services (percentage of wolf pack areas exposed to heavy browsing in 2045) and disservices (change in hunting opportunities for deer) under future scenarios.
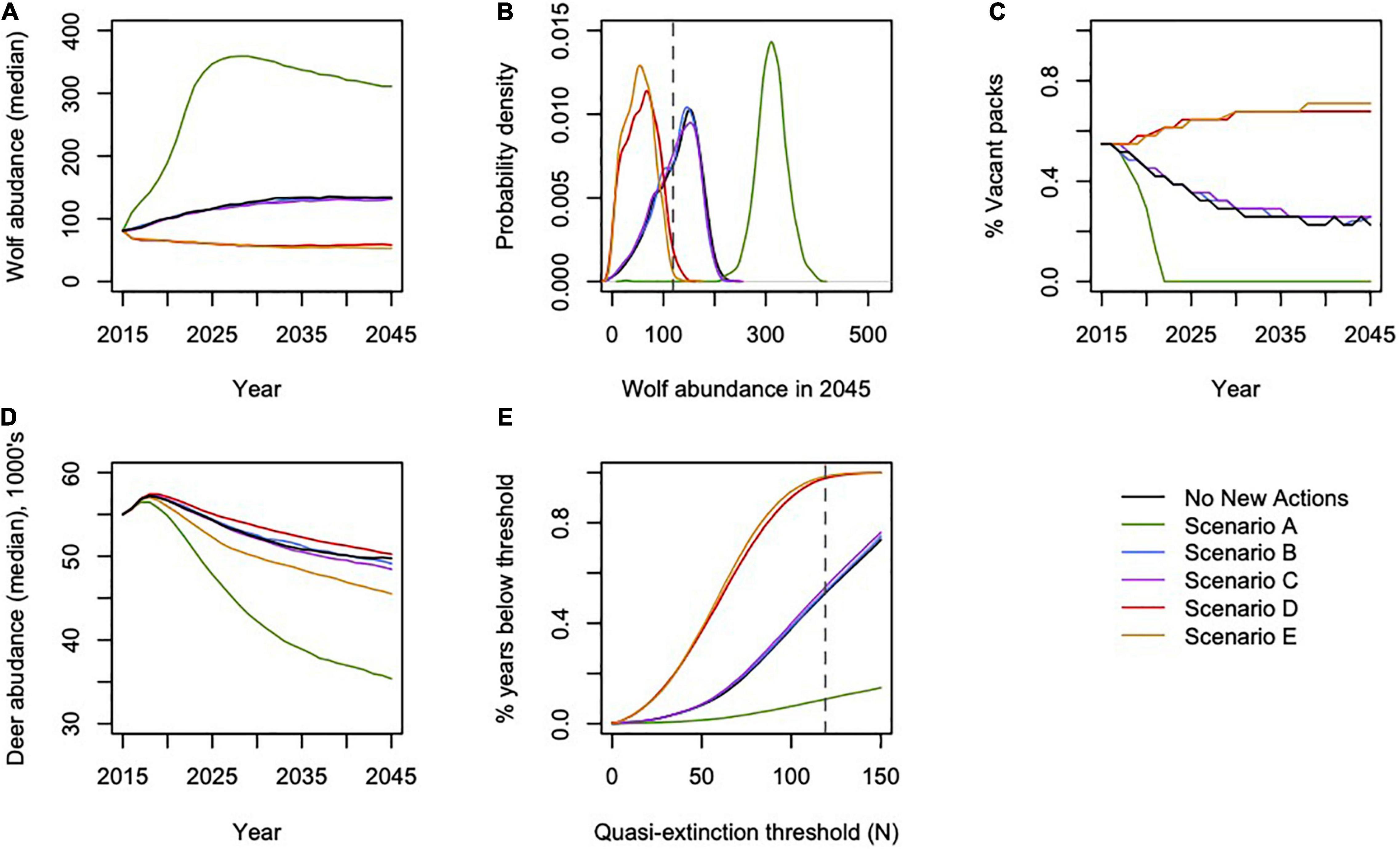
Figure 3. Projected effects of modeled scenarios on (A) annual wolf abundance; (B) distribution of final wolf abundance in 2045; (C) percent of pack areas vacant annually; (D) annual deer abundance; and (E) percentage of years during which wolf abundance dropped below a range of quasi-extinction thresholds. Dashed horizontal lines in (B,E) indicate the quasi-extinction threshold of N = 119 wolves, the abundance needed to ensure an effective population size (Ne) of 50 wolves.
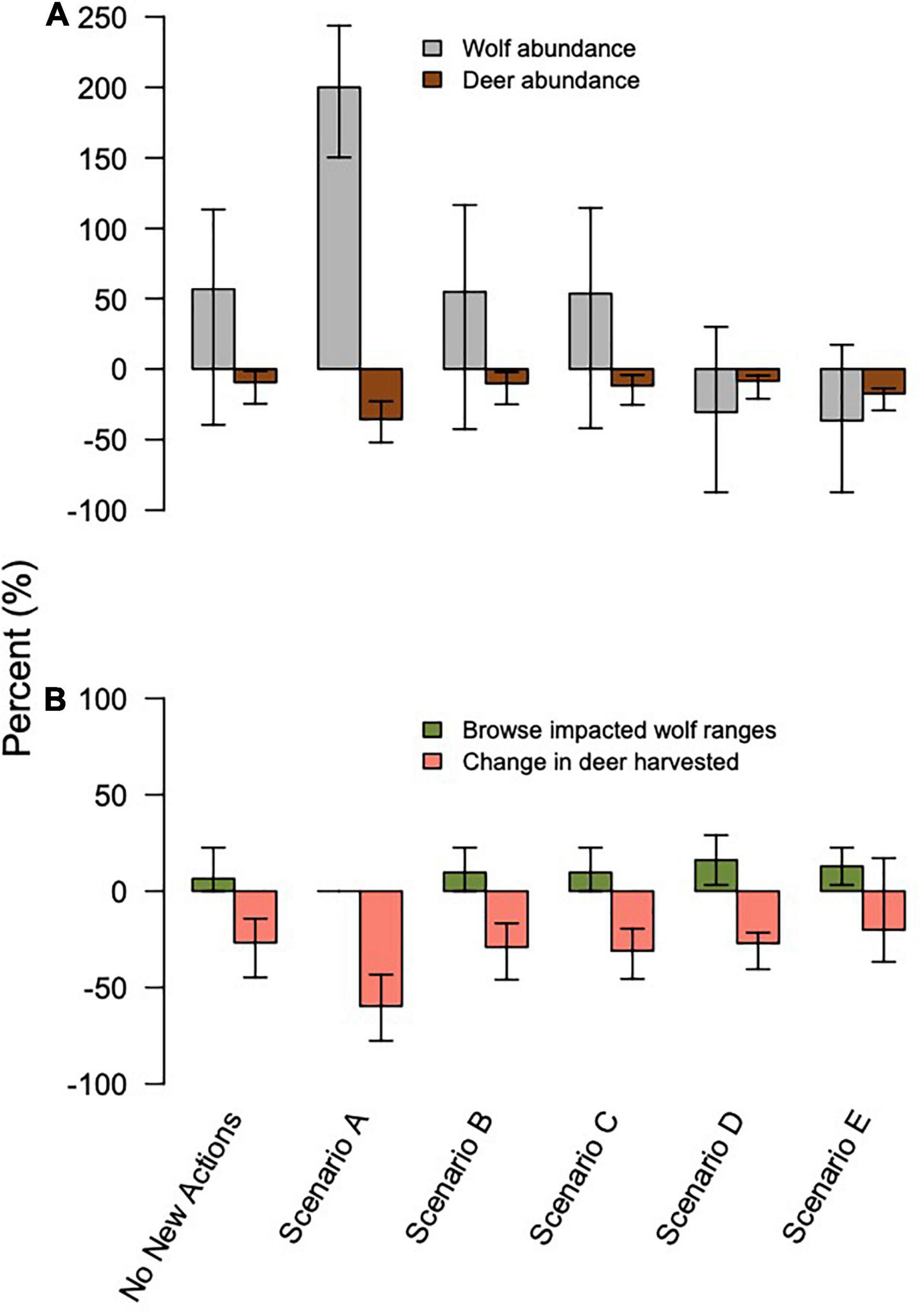
Figure 4. Change from 2014–2045 in (A) percent change of wolf (gray) and deer (brown) abundance, and (B) ecosystem services and disservices across model scenarios, with 95% confidence interval bars. Services and disservices include the % change in deer harvested by hunters and the percentage of pack areas exposed to intensive deer browsing on conifers by 2045.
Scenario A resulted in the largest increase in wolf abundance (284%, 95% CI = 222, 342), which included no further timber harvest (i.e., natural succession only from 2015 onward), planned decommission of roads, a low future frequency of severe winters, and no reported wolf harvest (although unreported wolf harvest continued). Scenario A also produced the lowest percentage of pack areas unoccupied (0% unoccupied by 2045), and lowest percentage of years in which the wolf population dropped below Ne = 50 (10% of years, 95% CI = 0, 32%; Figure 3). Despite less frequent severe winters, Scenario A also resulted in the largest decrease in deer population (–36%, 95% CI = –51, –22) and deer hunting opportunity (–60%, 95% CI = –78, –43) among the scenarios, because wolf numbers were high while deer carrying capacity continued to diminish due to post-logging forest succession, wolf predation, and deer hunting pressure. In contrast, Scenario E resulted in the largest declines in wolf abundance (–35%, 95% CI = –89, –21), which included increased harvest of old growth forest, no change in road density, a 30% cap on wolf harvest, and a high future frequency of severe winters. Old forest logging paired with road access, severe winters, and a high cap on wolf hunting and trapping resulted in the lowest rates of pack occupancy and the highest percentage of years dropping below Ne = 50 (98%, 95% CI = 81, 100). Deer hunting opportunity was the highest across all scenarios (–19%, 95% CI = –37, –14).
Scenario B, which we considered the most likely scenario, resulted in a median increase of 63% in wolf abundance (95% CI = –42, 122), median rate of pack area vacancy of 25% (95% CI = 0, 74), 52% of years with populations below the QE threshold Ne = 50 (95% CI = 0, 100; Figure 3), and a median decline of 11% in deer abundance (95% CI = –25, –2). The resulting predation services included browsing impacts in a median of 10% of pack ranges by 2045 (95% CI = 0, 23), a reduction from starting median values of 19% (95% CI = 9, 30).
Sensitivity Analysis
Deer abundance declined in 22 of the 26 sensitivity models because of ongoing changes in post-logging forest succession; however, wolf abundance increased across all of the sensitivity models, except for wolf harvest regulations with a 30% legal cap or no cap, in part because the starting value for the models was low based on the ADFG empirical estimate of wolf abundance (Supplementary Figure 1). Changes in wolf abundance due to changes in harvest regulations ranged from an increase of 200% (95% CI = 148, 266) if there was no legal or illegal wolf harvest, to a decrease of –30% (95% CI = 30, –90) if there was no cap on harvest. The second most influential variable on wolf abundance was the percentage of deer in wolf diets, which increased by 31–52%. These sensitivity results allow us to evaluate the influence of a variable across a reasonable range of values, but does not allow us to compare the absolute influence among variables on a per-unit basis.
Eliminating deer harvest entirely had a positive effect on both wolf and deer abundance compared to 2015 levels, increasing wolf abundance by 75% (95% CI = –38, 145) and deer abundance by 12%, (95% CI = 5, 16; Supplementary Table 4 and Supplementary Figure 1). Although an extreme and unrealistic perturbation, these results suggest wolves and humans are competing for deer in some watersheds and in some years. Imposing moderate deer harvest regulations could increase wolf and deer abundance, although hunting restrictions would reduce ecosystem services of hunting (i.e., protein provisioning to humans, cultural and recreational values).
The ecosystem disservices of severe deer browsing (deer at 90% of carrying capacity or more within a wolf pack’s home range) were most strongly influenced by wolf harvest regulations and deer hunting (Supplementary Table 5 and Supplementary Figure 2). Starting conditions for the simulation, which represent the lowest estimate of wolf abundance on record, showed that 19% of pack ranges (95% CI = 10, 32) were impacted by severe browsing. When wolf harvest was comprised only of illegal harvest or absent entirely, no pack ranges were heavily browsed by 2045. However, if a 30% cap or no cap on wolf harvest were implemented, 16% (95% CI = 3, 29) of pack ranges were heavily browsed. Without any deer hunting, 71% of pack ranges were heavily browsed (95% CI = 39, 90) compared to only 10% (95% CI = 0, 22) if hunting continued.
Ecosystem services provided by deer hunting were most strongly influenced by wolf harvest regulations and the percentage of deer in wolf diets, whereas disservices created by severe deer browsing were most strongly influenced by trapping regulations and deer hunting (Supplementary Table 5 and Supplementary Figure 2). When no wolf harvest occurred, deer hunting services declined drastically (–80%, 95% CI = –87, –66), but so did browsing disservices (0% pack ranges browsed, 95% CI = 0, 0). Conversely, if wolves went extinct, deer hunting services declined moderately (–18% CI = –29, –14) but browsing disservices rose (29% pack ranges browsed, 95% CI = 10, 32). Wolf diets showed similar, expected inverse relationships between services and disservices: when wolves ate 9.5 deer/year, deer browsing disservices were higher (16% pack ranges browsed, 95% CI = 3, 26), and hunting services declined less (–24%, 95% CI = –38, –16). When wolves ate the maximum of 26 deer/year, browsing disservices were almost eliminated (3% of pack ranges browsed, 95% CI = 0, 16), but hunting services declined considerably (–44%, 95% CI = –63, 23).
Discussion
Our model indicated that wolf populations would likely increase from our initial spring 2015 population for the “No New Action” scenario and scenarios A–C. This prediction was broadly supported by recent population estimates using a DNA-based capture-mark-recapture technique (n = 316 wolves estimated for fall 2019; Supplementary Figure 3), although there is considerable uncertainty around these empirical estimates (Alaska Department of Fish and Game [ADFG], 2017). However, recent wolf harvests have been record-breaking (n = 165 harvested winter 2019–2020; Alaska Department of Fish and Game [ADFG], 2017), and all scenarios except scenario A resulted in predicted negative population growth in a portion of the simulations, indicating some degree of risk of future decline regardless of scenario. Wolf populations fell below the quasi-extinction threshold of Ne = 50 in 10% of years under scenario A, in > 50% of the years simulated for scenarios No New Action, B and C, and by at least 97% of years in scenarios D and E. While our results suggest the potential for modest population growth under current and proposed conditions, wolf recovery likely will be well below historical levels and the potential for inbreeding and resulting population declines will likely remain. Moreover, about 70% of pack areas would be vacant in scenarios D and E and resulting potential for ecosystem disservices via deer browse impacts to conifer regeneration are probable in some areas. Our pack areas were static entities that in reality can be fluid and, as we stated previously, we likely underestimated the variation in predator-prey dynamics that would actually occur. Therefore, our simulation results are likely optimistic.
Mortality of wolves from legal and illegal harvest had the greatest influence on wolf abundance, (Santiago-Ávila et al., 2020; Louchouarn et al., 2021; Musto et al., 2021; Nowak et al., 2021) and also strongly affected deer services (hunting) and disservices (browsing impacts to conifers). Many studies of wolf population dynamics identify human exploitation as a primary driver of population change (Fuller et al., 2003) and the sensitivity of wolf population in our model to harvest is consistent with those studies. The effect of roads on wolves within our study area is also strongly linked to rates of mortality from harvest (Person et al., 1996; Person, 2001; Person and Russell, 2008; Person and Logan, 2012); however, our simulations show little effect of new road construction on future wolf population because the simulated increase in road density was small relative to the existing road extent. Similarly, the effects of forest succession on wolf and deer numbers did not vary much between scenarios because all scenarios included the successional transition to stem exclusion in large areas of second-growth that were harvested prior to 2015. Likewise, the influence of new clearcuts transitioning to old second-growth during the 30-year period covered by our simulations minimizes that same influence from new clearcuts because productive forest sites take 25–40 years to fully transition to low-nutrition old second growth (Alaback, 1982). Overall, the changes in forest conditions and roads that we included are a small fraction of the changes that have already occurred to those components between the initiation of industrial-scale logging in the mid-1950s and 2015.
Number of deer killed/year/wolf also exerted a strong influence on wolf and deer numbers, reducing both as predation rates increased. Person (2001) modeled the predator-prey system on Prince of Wales Island using a simpler version of our model with a higher per capita rate of predation. He estimated mean and variance of predation rate on deer from incidence of occurrence data in scats collected during all seasons (Person et al., 1996; Kohira and Rexstad, 1997), whereas we employed a lower rate of per capita predation based on stable isotope analyses (Szepanski et al., 1999). Both methods likely underestimate actual predation rates because diet composition cannot be easily converted to number of prey killed, particularly when predators such as wolves do not always consume entire carcasses. Severe winters can substantially reduce deer numbers (Brinkman et al., 2011) and increase the ratio of wolves to deer, which may result in wolves suppressing deer population to low levels for multiple years (Ballard et al., 2001; Bowyer et al., 2005). Our simulations failed to express that dynamic, likely because we used low rates of predation and initial population size of wolves. In contrast, model simulations by Person (2001) for conditions similar to our scenarios B and C, showed that severe winter events reduced deer populations below 50% of K in 39% of individual model runs, and that 16% of those remained below that threshold for ≥10 years (Person, 2001). The direct consequence for wolves of suppressed deer populations is not immediate food stress because other prey, particularly salmon and beaver, can be temporarily substituted (Darimont et al., 2008). Rather, the belief that wolves are competing for fewer deer will motivate hunters and trappers to kill more wolves legally and illegally (Person and Brinkman, 2013).
Our focus was constrained mostly to wolves because ESA decisions are species centric. Nonetheless, to sustain a resilient population of wolves, deer and other prey are required in sufficient numbers to also sustain other predators like black bears and satisfy deer hunters. Predation by black bears had little influence on changes in predator-prey dynamics between wolves and deer, but hunter harvest of deer reduced both deer and wolf numbers. If wolves had been listed as threatened, scenario “A” likely best represents the resulting conditions in which wolf harvest is curtailed and a significant percentage of roads closed to vehicular use. However, deer harvest likely would plunge 43–78% to levels well below current harvests (Harper and McCarthy, 2015) and result in a strong backlash against protecting wolves from subsistence and recreational deer hunters. This could introduce substantial instability to the wolf-deer-people system. Most scenarios indicate deer harvests will decline by 20–30% from 2015 levels, raising the risk that retaliatory legal and illegal wolf harvest will increase and suppress wolf populations below our predicted levels.
The results of our analyses suggest that radical changes in system dynamics on POW are possible and highlight the challenge of conserving predator-prey systems, as well as the potential for considerable changes to predation services and disservices. We argue that large mammalian predators are inevitably dependent on complex social-ecological communities and must be considered within the context of a system rather than an individual species population. Ultimately, loss of deer habitat will drive the systemic decline of deer over the coming decades. The proximate threat to wolf viability will likely still be risk of unsustainable legal and illegal wolf harvest rather than prey scarcity. The motivation for hunters and trappers to kill wolves will be driven by their perception of deer abundance, which is influenced not only by relative abundance of deer and wolves but also by landscape changes (Brinkman et al., 2009). As areas dominated by clearcuts transition to thick, even-aged second growth, deer hunters can no longer see or hunt deer in those landscapes and their perception of competition with wolves and other hunters for deer on dwindling lands suitable for hunting will continue to grow.
As deer carrying capacity and resulting deer abundance declines, deer will also likely continue to cause browsing disservices by reducing or eliminating red and yellow cedar and slowing regeneration rates of conifers in young clearcuts, especially if populations are regulated by nutrition rather than predation. As we demonstrate here, wolves are likely providing a predation service to the forestry industry by reducing browsing damage, but this service is currently overlooked, despite its potential high value. In our analysis of potential wolf extinction effects, browsing impacts to conifer occurred in 30% of pack ranges by 2045; this percentage could be much higher if hunting does not keep pace with the deer population, and areas with poor access or sightability for hunters are especially likely to show such effects in the absence of wolves. For example, if deer hunting is absent from the system, over 70% of pack ranges would be impacted by severe conifer browsing, even with continued wolf predation. Long timber rotations are one of the limiting factors affecting the competitiveness of the Southeast Alaskan second-growth timber economy, and the potential for 20 years of delayed regeneration or complete loss of commercially valuable species, as has been documented on wolf-free neighboring Haida Gwaii (where hunters are unable to regulate the deer population in the absence of wolves) could make this marginal industry unfeasible. Given the simplistic and optimistic way that hunting is included in our models, we are likely over-estimating deer harvest and hunters’ abilities to reduce deer browsing. For example, we included no sex structure in our models, implying both sexes are hunted equally, whereas in reality availability of male deer limits hunting opportunity; likewise, we included no habitat limitations to hunting, while in reality older successional forest stages are very difficult habitats in which to spot and successfully hunt deer.
Importantly, deer can provide other important ecosystem services (such as wildlife viewing and existence values) and disservices (such as suppression of berry bushes, reduction of bird and invertebrate biodiversity, and reduction of carbon sequestration) that we do not attempt to estimate (Côté et al., 2004; Martin et al., 2020). While explicitly representing these feedbacks is beyond the scope of our analysis, the effects of predator-prey interactions on ecosystem function are important to consider, especially as worldwide interest in carbon sequestration and biodiversity conservation accelerates (Schmitz et al., 2018). For example, moderate browsing may increase soil organic carbon (SOC) storage by increasing soil bulk density through trampling and compaction and increase plant biodiversity by influencing plant competitiveness and assembly rules (Nishizawa et al., 2016). However, heavy browsing may reduce forest net primary productivity (NPP) and carbon (C) sequestration by removing aboveground biomass, delaying seedling recruitment, and degrading soil structure (Harada et al., 2020). Preferential browsing can also reduce litter quality by removing palatable species (e.g., cedar and huckleberries) and inducing plants to invest in antiherbivore defense compounds and structural tissues (Côté et al., 2004), although high egestion rates could bypass the litter decomposition pathway (Bardgett and Wardle, 2003). Reducing the quality of litter entering the soil ecosystem alters microbial functional traits (Shao et al., 2019), increases SOC mineralization, and decelerates soil nutrient and litter cycling (Harrison and Bardgett, 2004). If microbial communities attack existing stocks to access nutrients (a phenomenon known as priming; Kuzyakov et al., 2000), conversion of SOC to CO2 could flip heavily browed forests from a regional C sink to source.
Wolf predation could help mitigate, or reverse, SOC loss by inducing trophic cascades that release plants from intense browsing pressures (Kirchhoff and Person, 2008; Callaghan et al., 2013; Flagel et al., 2016; Schmitz et al., 2018). As recovering plants allocate C belowground, they gain access to limiting nutrients (including from decomposing carcasses that are not exported from the forest by hunters; Daufresne, 2021). This belowground carbon allocation can in turn stimulate rhizosphere microbial communities (Bardgett et al., 1998). Resulting increases in forage quality could not only help stabilize deer and wolf populations, but may also promote efficient microbial biomass production (Wardle et al., 2002; Liang et al., 2017). The retention of microbial byproducts on soil minerals is now thought to be a primary driver of SOC formation and persistence (Lehmann et al., 2020) and could be especially important in Coastal Alaska where more than 60% of total forest C is held in mineral pools (Yatskov et al., 2019). Wolf-regulated increases in microbial efficiency could thus reinforce the forest C sink. The effects of growing pressure on the soil ecosystem is complex and could exert a positive feedback on SOC (Conant and Paustian, 2002), by increasing microbial metabolic efficiency and the formation of new SOC, or a negative feedback, by increasing priming and SOC loss as CO2. Browsing and predator-prey interactions modulate the balance between litter inputs and heterotrophic respiration, which is difficult to predict but has large consequences for the forest C balance (Pugh et al., 2019). Explicitly incorporating predator-prey dynamics could improve the predictive capacity of ecosystem models and may reveal additional ecosystem services provided by wolves and deer (Schmitz et al., 2018).
In closing, we argue for a new, integrated approach to forest and wildlife management in Southeast Alaska and beyond, in which the “multi-use” mandate of the US Forest Service grows to encompass the wide variety of valuable ecosystem services provided by forests. From providing incredible beauty for tourists and residents, to sequestering carbon, sheltering biodiversity, and providing livelihoods, food, and cultural values to indigenous and rural residents, this multitude of services should be considered and valued in management planning. Clearly, herbivory can strongly affect above- and below-ground carbon stores, and predators can regulate the numbers and behavior of their prey; there should be a corresponding, concerted effort by USFS to quantify and incorporate these dynamics into their research program and management decisions. We also recommend several specific management actions. First, we recommend that state and federal agencies more rigorously monitor wolf and deer and require accurate and timely reporting of wolf and deer harvests, as well as implement parallel rigorous social science to understand people’s attitudes and behaviors in relation to deer and wolves. We also suggest that a cautious approach to management of wolf hunting and trapping within the Prince of Wales Island archipelago is necessary, given the potentially large downsides of wolf extinction for forest regeneration and carbon dynamics, and that explicit inclusion of social science into management planning would be enormously helpful for managing this social-ecological system. For example, if deer harvest is insufficient to meet people’s expectations and subsistence needs, simply tightening wolf harvest will probably not be sufficient to prevent retaliatory killings of wolves if people perceive wolves, rather than habitat, as limiting their deer harvest opportunity. In addition, we urge the U.S. Forest Service to implement existing plans to close roads while preserving access to popular deer hunting areas, and to adopt application of methods to maintain and reestablish understory vegetation in both young and old second-growth stands, such as canopy gaps and small clearcuts (Wolf Technical Committee, 2017). New harvest of second-growth forest should be configured to maximize a broad range of ecosystem services across the landscape, not only board-feet of merchantable timber. Restoration treatments should be well distributed within hunter accessible watersheds as well as those closed to vehicular use, ensuring that hunters can harvest deer and that conifers can re-generate in deer-suppressed areas. Finally, a collaborative approach that includes consideration of all stakeholders’ values, notably traditional values, offers the best strategy to ensure compliance with harvest regulations, meet needs of all stakeholders, and help maintain the long-term stability of this social-ecological system.
Data Availability Statement
The datasets presented in this study can be found in online repositories. The names of the repository/repositories and accession number(s) can be found below: https://github.com/sophielgilbert/alexander-archipelago-wolf.
Ethics Statement
Ethical review and approval was not required for the animal study because we used only existing data from past studies of animals.
Author Contributions
SG, TH, ML, MK, DA, and DP contributed to the analysis. All co-authors contributed to the ideas and framing, writing, and editing. All authors contributed to the article and approved the submitted version.
Funding
This work was supported by the U.S. Fish and Wildlife Service (Agreement: F15AC000206). This work was funded by the University of Idaho – Open Access Publishing Fund.
Author Disclaimer
The views and conclusions contained in this document are those of the authors and should not be interpreted as representing the opinions or policies of the U.S. Government. Mention of trade names or commercial products does not constitute their endorsement by the U.S. Government.
Conflict of Interest
The authors declare that the research was conducted in the absence of any commercial or financial relationships that could be construed as a potential conflict of interest.
Publisher’s Note
All claims expressed in this article are solely those of the authors and do not necessarily represent those of their affiliated organizations, or those of the publisher, the editors and the reviewers. Any product that may be evaluated in this article, or claim that may be made by its manufacturer, is not guaranteed or endorsed by the publisher.
Acknowledgments
We would like to thank all the researchers and managers who provided input and insight at the March 2015 workshop. In addition, the Alaska Department of Fish and Game and the U.S. Forest Service provided assistance with data acquisition, while J. Littell kindly shared unpublished data with us.
Supplementary Material
The Supplementary Material for this article can be found online at: https://www.frontiersin.org/articles/10.3389/fevo.2022.809371/full#supplementary-material
References
Alaback, P. B. (1982). Dynamics of understory biomass in Sitka spruce-western hemlock forests of southeast Alaska. Ecology 63, 1932–1948. doi: 10.2307/1940131
Alaback, P. G., and Saunders, S. (2013). “Disturbance ecology of the temperate rainforests of Southeast Alaska and adjacent British Columbia,” in North Temperate Rainforests: Ecology and Conservation, eds J. W. Shoen and G. Orians (Seattle, WA: University of Washington Press).
Alaska Department of Fish and Game [ADFG] (2015a). GMU 2 Wolf Populaton Estimate Update, Fall 2014. Juneau, AK: ADFG.
Alaska Department of Fish and Game [ADFG] (2015b). Memorandum from Scott Ryan, Region 1 Regional Supervisor, July 13, 2015. Juneau, AK: ADFG.
Alaska Department of Fish and Game [ADFG] (2017). GMU 2 Wolf Population Update, Autumnt 2016. Juneau, AK: ADFG.
Alaska Department of Fish and Game [ADFG] (2021). 2021 GMU 2 Wolf Season Announced. Juneau, AK: ADFG.
Albert, D. M., and Schoen, J. W. (2013). Use of historical logging patterns to identify disproportionately logged ecosystems within temperate rainforests of Southeast Alaska. Conserv. Biol. 27, 774–784. doi: 10.1111/cobi.12109
Albert, D., and Schoen, J. (2007). “A conservation assessment of the coastal forests and mountains ecoregion of Southeast Alaska and the Tongass National Forest,” in A Conservation Assessment of the Coastal Forests and Mountains Ecoregion of Southeast Alaska and the Tongass National Forest, eds J. Schoen and E. Dovichin (Anchorage, AK: Audubon Alaska and The Nature Conservancy).
Aspi, J., Roininen, E., Ruokonen, M., Kojola, I., and Vilà, C. (2006). Genetic diversity, population structure, effective population size and demographic history of the Finnish wolf population. Mol. Ecol. 15, 1561–1576. doi: 10.1111/j.1365-294X.2006.02877.x
Bachand, M., Pellerin, S., Côté, S. D., Moretti, M., De Cáceres, M., Brousseau, P. M., et al. (2014). Species indicators of ecosystem recovery after reducing large herbivore density: comparing taxa and testing species combinations. Ecol. Indicators 38, 12–19. doi: 10.1016/j.ecolind.2013.10.018
Ballard, W. B., Lutz, D., Keegan, T. W., Carpenter, L. H., and deVos, J. C. Jr. (2001). Deer-predator relationships: a review of recent North American studies with emphasis on mule and black-tailed deer. Wildlife Soc. Bull. 29, 99–115.
Bardgett, R. D., and Wardle, D. A. (2003). Herbivore-mediated linkages between aboveground and belowground communities. Ecology 84, 2258–2268. doi: 10.1890/02-0274
Bardgett, R. D., Wardle, D. A., and Yeates, G. W. (1998). Linking above-ground and below-ground interactions: how plant responses to foliar herbivory influence soil organisms. Soil Biol. Biochem. 30, 1867–1878. doi: 10.1016/S0038-0717(98)00069-8
Benner, J., Nielsen, J., and Lertzman, K. (2021). Using traditional ecological knowledge to understand the diversity and abundance of culturally important trees. J. Ethnobiol. 41, 209–228. doi: 10.2993/0278-0771-41.2.209
Bowyer, R. T., Person, D. K., and Pierce, B. M. (2005). Detecting Top-down Versus Bottom-up Regulation of Ungulates by Large Carnivores: Implications for Conservation of Biodiversity. Large Carnivores and the Conservation of Biodiversity (JC Ray, KH Redford, RS Steneck, and Berger, J., eds). Washington, DC: Island Press.
Braczkowski, A. R., O’Bryan, C. J., Stringer, M. J., Watson, J. E. M., Possingham, H. P., and Beyer, H. L. (2018). Leopards provide public health benefits in Mumbai, India. Front. Ecol. Environ. 16:176–182. doi: 10.1002/fee.1776
Bradley, E. H., Robinson, H. S., Bangs, E. E., Kunkel, K., Jimenez, M. D., Gude, J. A., et al. (2015). Effects of Wolf removal on livestock depredation recurrence and Wolf recovery in Montana, Idaho, and Wyoming. J. Wildlife Manag. 79, 1337–1346. doi: 10.1002/jwmg.948
Brandt, P., Abson, D. J., DellaSala, D. A., Feller, R., and von Wehrden, H. (2014). Multifunctionality and biodiversity: ecosystem services in temperate rainforests of the Pacific Northwest, USA. Biol. Conserv. 169, 362–371. doi: 10.1016/j.biocon.2013.12.003
Breed, M. (2007). The tale of the Dire Effects of Hunting on wolves in Coastal Southeast Alaska: Loss of Genetic Diversity, Fragmentation and a Regional Sink. Uppsala: Uppsala University.
Brinkman, T. J., Chapin, T., Kofinas, G., and Person, D. K. (2009). Linking hunter knowledge with forest change to understand changing deer harvest opportunities in intensively logged landscapes. Ecol. Soc. 14:36. doi: 10.5751/ES-02805-140136
Brinkman, T. J., Kofinas, G. P., Chapin, F. S. III, and Person, D. K. (2007). Influence of hunter adaptability on resilience of subsistence hunting systems. J. Ecol. Anthropol. 11, 58–63. doi: 10.5038/2162-4593.11.1.4
Brinkman, T. J., Person, D. K., Chapin, F. S. I. I. I., Smith, W., and Hundertmark, K. J. (2011). Estimating abundance of Sitka black-tailed deer using DNA from fecal pellets. J. Wildlife Manag. 75, 232–242. doi: 10.1002/jwmg.22
Callaghan, T. V., Jonasson, C., Thierfelder, T., Yang, Z., Hedenås, H., Johansson, M., et al. (2013). Ecosystem change and stability over multiple decades in the Swedish subarctic: complex processes and multiple drivers. Philos. Trans. R. Soc. B: Biol. Sci. 368:20120488. doi: 10.1098/rstb.2012.0488
Center for Biological Diversity and Greenpeace (2011). Petition to List the Alexander Archipelago Wolf as Threatened or Endangered Under the United States Endangered Species Act. Tucson, AZ: Center for Biological Diversity and Greenpeace.
Colson, K. E., Brinkman, T. J., Person, D. K., and Hundertmark, K. J. (2013). Fine-scale social and spatial genetic structure in Sitka black-tailed deer. Conserv. Genet. 14, 439–449. doi: 10.1007/s10592-012-0388-0
Conant, R. T., and Paustian, K. (2002). Potential soil carbon sequestration in overgrazed grassland ecosystems. Global Biogeochem. Cycles 16, 90–91. doi: 10.1029/2001GB001661
Côté, S. D., Rooney, T. P., Tremblay, J. P., Dussault, C., and Waller, D. M. (2004). Ecological impacts of deer overabundance. Annu. Rev. Ecol. Evol. Syst. 35, 113–147. doi: 10.1146/annurev.ecolsys.35.021103.105725
Cronin, M. A., Canovas, A., Bannasch, D. L., Oberbauer, A. M., and Medrano, J. F. (2015). Single nucleotide polymorphism (SNP) variation of wolves (Canis lupus) in Southeast Alaska and comparison with wolves, dogs, and coyotes in North America. J. Heredity 106, 26–36. doi: 10.1093/jhered/esu075
Darimont, C. T., Paquet, P. C., and Reimchen, T. E. (2008). Spawning salmon disrupt trophic coupling between wolves and ungulate prey in coastal British Columbia. BMC Ecol. 8:14. doi: 10.1186/1472-6785-8-14
Daufresne, T. (2021). A consumer-driven recycling theory for the impact of large herbivores on terrestrial ecosystem stoichiometry. Ecol. Lett. 24, 2598–2610. doi: 10.1111/ele.13876
DellaSala, D. A., Moola, F., Alaback, P., Paquet, P. C., Schoen, J. W., and Noss, R. F. (2011). “Temperate and boreal rainforests of the Pacific Coast of North America,” in Temperate and Boreal Rainforests of the World: Ecology and Conservation, ed. D. A. DellaSala (Washington, DC: Island Press), 42–81. doi: 10.5822/978-1-61091-008-8_2
Dressel, S., Sandström, C., and Ericsson, G. (2015). A meta-analysis of studies on attitudes toward bears and wolves across Europe 1976–2012. Conserv. Biol. 29, 565–574. doi: 10.1111/cobi.12420
Farmer, C. J., and Kirchhoff, M. D. (2007). Ecological classification of deer habitat in the Tongass National Forest, Alaska. Northwestern Natural. 88, 73–84. doi: 10.1898/1051-1733(2007)88[73:ECODHI]2.0.CO;2
Farmer, C. J., Person, D. K., and Bowyer, R. T. (2006). Risk factors and mortality of black-tailed deer in a managed forest landscape. J. Wildlife Manag. 70, 1403–1415. doi: 10.2193/0022-541X(2006)70[1403:RFAMOB]2.0.CO;2
Flagel, D. G., Belovsky, G. E., and Beyer, D. E. (2016). Natural and experimental tests of trophic cascades: gray wolves and white-tailed deer in a Great Lakes forest. Oecologia 180, 1183–1194. doi: 10.1007/s00442-015-3515-z
Franklin, I. (1980). “Evolutionary change in small populations,” in Conservation Biology: an Evolutionary-ecological Persective, eds M. Soule and B. Wilcox (Sunderland, MA: Sinauer Associates, Inc).
Fuller, T. K., Mech, L. D., and Cochrane, J. F. (2003). Wolf Population Dynamics. Chicago, IL: University of Chicago Press.
Gaston, A. J., Stockton, S. A., and Smith, J. L. (2006). Species-area relationships and the impact of deer-browse in the complex phytogeography of the HaidaGwaii archipelago (Queen Charlotte Islands), British Columbia. Ecoscience 13, 511–522. doi: 10.2980/1195-6860(2006)13[511:SRATIO]2.0.CO;2
Gilbert, S. L. (2015). Environmental Drivers of Deer Population Dynamics and Spatial Selection in Southeast Alaska. Juneau, AK: University of Alaska Fairbanks.
Gilbert, S. L. (2016). Bald eagle predation on sitka black-tailed deer fawns. Northwestern Natural. 97, 66–69. doi: 10.1898/1051-1733-97.1.66
Gilbert, S. L., Haynes, T., Lindberg, M. S., Albert, D. M., and Kissling, M. (2015). Future Population Trends and Drivers of Change for Alexander Archipelago Wolves on and near Prince of Wales Island, Alaska. Anchorage, AK: U.S. Fish and Wildlife Service. Final Report for Cooperative Agreement: F15AC000206. doi: 10.7287/peerj.preprints.1934v1
Gilbert, S. L., Carter, N., and Naidoo, R. (2021a). Predation services: quantifying societal effects of predators and their prey. Front. Ecol. Environ. 19:292–299. doi: 10.1002/fee.2336
Gilbert, S. L., Hundertmark, K. J., Lindberg, M. S., Person, D. K., and Boyce, M. S. (2021b). The importance of environmental variability and transient population dynamics for a northern ungulate. Front. Ecol. Evol. 8:531027. doi: 10.3389/fevo.2020.531027
Gilbert, S. L., Hundertmark, K. J., Lindberg, M. S., Person, D. K., and Boyce, M. S. (2020). The importance of environmental variability and transient population dynamics for a northern ungulate. Front. Ecol. Evol. 8:531027.
Gilbert, S. L., Hundertmark, K. J., Person, D. K., Lindberg, M. S., and Boyce, M. S. (2017). Behavioral plasticity in a variable environment: snow depth and habitat interactions drive deer movement in winter. J. Mammal. 98, 246–259. doi: 10.1093/jmammal/gyw167
Hanley, T. A., Spalinger, D. E., Mock, K. J., Weaver, O. L., and Harris, G. M. (2012). Forage Resource Evaluation System for Habitat–deer: An Interactive Deer Habitat Model. General Technical Report PNW-GTR-858 Portland, OR: Department of Agriculture, Forest Service, Pacific Northwest Research Station. doi: 10.2737/PNW-GTR-858
Harada, K., Ann, J. A. M., and Suzuki, M. (2020). Legacy effects of sika deer overpopulation on ground vegetation and soil physical properties. Forest Ecol. Manag. 474:118346. doi: 10.1016/j.foreco.2020.118346
Harper, P., and McCarthy, L. A. (eds) (2015). Deer Management Report of Survey-inventory Activities, 1 July 2012-30 June 2014. Juneau, AK: Alaska Department of Fish and Game, Division of Wildlife Conservation.
Harrison, K. A., and Bardgett, R. D. (2004). Browsing by red deer negatively impacts on soil nitrogen availability in regenerating native forest. Soil Biol. Biochem. 36, 115–126. doi: 10.1016/j.soilbio.2003.08.022
Kirchhoff, M. D. (1994). Effects of Forest Fragmentation on Deer in Southeast Alaska. Juneau, AK: ADFG.
Kirchhoff, M. D., and Person, D. K. (2008). The Alaska Perspective—Deer Populations in the Presence of Wolves. Lessons from the Islands. Ottawa: Canadian Wildlife Service
Kohira, M., and Rexstad, E. A. (1997). Diets of Wolves, Canis Lupus, in Logged and Unlogged Forests of Southeastern Alaska. Ottawa ON: Canadian field-naturalist.
Kuzyakov, Y., Friedel, J. K., and Stahr, K. (2000). Review of mechanisms and quantification of priming effects. Soil Biol. Biochem. 32, 1485–1498. doi: 10.1016/S0038-0717(00)00084-5
Laikre, L., Olsson, F., Jansson, E., Hössjer, O., and Ryman, N. (2016). Metapopulation effective size and conservation genetic goals for the Fennoscandian wolf (Canis lupus) population. Heredity 117, 279–289. doi: 10.1038/hdy.2016.44
Le Saout, S., Chollet, S., Chamaillé-Jammes, S., Blanc, L., Padié, S., Verchere, T., et al. (2014). Understanding the paradox of deer persisting at high abundance in heavily browsed habitats. Wildlife Biol. 20, 122–135. doi: 10.2981/wlb.13048
Lehmann, J., Hansel, C. M., Kaiser, C., Kleber, M., Maher, K., Manzoni, S., et al. (2020). Persistence of soil organic carbon caused by functional complexity. Nat. Geosci. 13, 529–534. doi: 10.1038/s41561-020-0612-3
Liang, C., Schimel, J. P., and Jastrow, J. D. (2017). The importance of anabolism in microbial control over soil carbon storage. Nat. Microbiol. 2, 1–6. doi: 10.1038/nmicrobiol.2017.105
Liberg, O., Chapron, G., Wabakken, P., Pedersen, H. C., Hobbs, N. T., and Sand, H. (2012). Shoot, shovel and shut up: cryptic poaching slows restoration of a large carnivore in Europe. Proc. R. Soc. B 279, 910–915. doi: 10.1098/rspb.2011.1275
Louchouarn, N. X., Santiago-Ávila, F. J., Parsons, D. R., and Treves, A. (2021). Evaluating how lethal management affects poaching of Mexican wolves. R. Soc. Open Sci. 8:200330. doi: 10.1098/rsos.200330
Maillard, M., Martin, J. L., Chollet, S., Catomeris, C., Simon, L., and Grayston, S. J. (2021). Belowground effects of deer in a temperate forest are time-dependent. Forest Ecol. Manag. 493:119228. doi: 10.1016/j.foreco.2021.119228
Manfredo, M. J., Berl, R. E. W., Teel, T. L., and Bruskotter, J. T. (2021a). Bringing social values to wildlife conservation decisions. Front. Ecol. Environ. 19:355–362. doi: 10.1002/fee.2356
Manfredo, M. J., Teel, T. L., Berl, R. E. W., Bruskotter, J. T., and Kitayama, S. (2021b). Social value shift in favour of biodiversity conservation in the United States. Nat. Sustainabil. 4, 323–330. doi: 10.1038/s41893-020-00655-6
Martin, J. L., Chamaillé-Jammes, S., and Waller, D. M. (2020). Deer, wolves, and people: costs, benefits and challenges of living together. Biol. Rev. 95, 782–801. doi: 10.1111/brv.12587
Moss, M. L. (2004). The status of archaeology and archaeological practice in Southeast Alaska in relation to the larger Northwest Coast. Arctic Anthropol. 41, 177–196. doi: 10.1353/arc.2011.0001
Musiani, M., and Paquet, P. C. (2004). The practices of wolf persecution, protection, and restoration in Canada and the United States. BioScience 54, 50–60. doi: 10.1641/0006-3568(2004)054[0050:TPOWPP]2.0.CO;2
Musto, C., Cerri, J., Galaverni, M., Caniglia, R., Fabbri, E., Apollonio, M., et al. (2021). Men and wolves: anthropogenic causes are an important driver of wolf mortality in human-dominated landscapes in Italy. Global Ecol. Conserv. 32:e01892. doi: 10.1016/j.gecco.2021.e01892
Nishizawa, K., Tatsumi, S., Kitagawa, R., and Mori, A. S. (2016). Deer herbivory affects the functional diversity of forest floor plants via changes in competition-mediated assembly rules. Ecol. Res. 31, 569–578. doi: 10.1007/s11284-016-1367-6
Norton, H. H. (1981). Plant use in KaiganiHaida culture: correction of an ethnohistorical oversight. Econ. Botany 35, 434–449. doi: 10.1007/BF02858592
Nowak, S., Żmihorski, M., Figura, M., Stachyra, P., and Mysłajek, R. W. (2021). The illegal shooting and snaring of legally protected wolves in Poland. Biol. Conserv. 264:109367. doi: 10.1016/j.biocon.2021.109367
O’Bryan, C. J., Braczkowski, A. R., Beyer, H. L., Carter, N. H., Watson, J. E. M., and McDonald-Madden, E. (2018). The contribution of predators and scavengers to human well-being. Nat. Ecol. Evol. 2, 229–236. doi: 10.1038/s41559-017-0421-2
Parker, K. L., Gillingham, M. P., Hanley, T. A., and Robbins, C. T. (1999). Energy and protein balance of free-ranging black-tailed deer in a natural forest environment. Wildlife Monographs 143, 1–48.
Parker, K. L., Robbins, C. T., and Hanley, T. A. (1984). Energy expenditures for locomotion by mule deer and elk. J. Wildlife Manag. 48, 474–488. doi: 10.2307/3801180
Person, D. K. (1996). The Alexander Archipelago Wolf: a Conservation Assessment. Washington, D.C: US Department of Agriculture, Forest Service, Pacific Northwest Research Station. doi: 10.2737/PNW-GTR-384
Person, D. K. (2001). Alexander Archipelago Wolves: Ecology and Population Viability in a Disturbed, Insular Landscape. Juneau, AK: University of Alaska Fairbanks.
Person, D. K., and Bowyer, R. T. (1997). Population Viability Analysis of Wolves on Prince of Wales and Kosciusko Islands, Alaska. Juneau, AK: ADFG.
Person, D. K., and Bowyer, R. T.Van Ballenberghe, V. (2001). Density dependence of ungulates and functional responses of wolves: effects on predator-prey ratios. Alces: A Journal Devoted to the Biology and Management of Moose. 37, 253–273.
Person, D. K., and Brinkman, T. J. (2013). “Succession debt and roads: short- and long-term effects of timber harvest on a large-mammal predator-prey community in Southeast Alaska,” in North Pacific Temperate Rainforests, eds G. H. Orians and J. W. Schoen (Seattle, WA: University of Washington Press).
Person, D. K., and Logan, B. D. (2012). A Spatial Analysis of Wolf Harvest and Harvest Risk on Prince of Wales and Associated Islands, Southeast Alaska. Final Wildlife Research Report, WRR-2012–2016. Juneau, AK: Alaska Department of Fish and Game
Person, D. K., and Russell, A. L. (2008). Correlates of mortality in an exploited wolf population. J. Wildlife Manag. 72, 1540–1549.
Person, D. K., Farmer, C., O’Connor, P., and Doerr, J. (2009). Habitat use and Survivorship of Sitka Black-tailed Deer in Southeast Alaska: a Regional Meta-analysis and Synthesis. Juneau, AK: ADFG.
Person, D. K., Kirchhoff, M., Van Ballenberghe, V., and Iverson, G. C. (1996). The Alexander Archipelago Wolf: a Conservation Assessment. Portland. General Technical Report PNW-GTR-384.
Pohja-Mykrä, M., and Kurki, S. (2014). Strong community support for illegal killing challenges wolf management. Eur. J. Wildlife Res. 60, 759–770. doi: 10.1007/s10344-014-0845-9
Pugh, T. A., Lindeskog, M., Smith, B., Poulter, B., Arneth, A., Haverd, V., et al. (2019). Role of forest regrowth in global carbon sink dynamics. Proc. Natl. Acad. Sci. U S A. 116, 4382–4387. doi: 10.1073/pnas.1810512116
Ripple, W. J., Estes, J. A., Beschta, R. L., Wilmers, C. C., Ritchie, E. G., Hebblewhite, M., et al. (2014). Status and ecological effects of the world’s largest carnivores. Science 343, 151–162. doi: 10.1126/science.1241484
Roffler, G. H., Gregovich, D. P., and Larson, K. R. (2018). Resource selection by coastal wolves reveals the seasonal importance of seral forest and suitable prey habitat. Forest Ecol. Manag. 409, 190–201. doi: 10.1016/j.foreco.2017.11.025
Santiago-Ávila, F. J., Chappell, R. J., and Treves, A. (2020). Liberalizing the killing of endangered wolves was associated with more disappearances of collared individuals in Wisconsin, USA. Sci. Rep. 10:13881. doi: 10.1038/s41598-020-70837-x
Schmitz, O. J., Wilmers, C. C., Leroux, S. J., Doughty, C. E., Atwood, T. B., Galetti, M., et al. (2018). Animals and the zoogeochemistry of the carbon cycle. Science 362:eaar3213. doi: 10.1126/science.aar3213
Shao, P., Liang, C., Lynch, L., Xie, H., and Bao, X. (2019). Reforestation accelerates soil organic carbon accumulation: evidence from microbial biomarkers. Soil Biol. Biochem. 131, 182–190. doi: 10.1016/j.soilbio.2019.01.012
Suring, L. H., Degayner, E. J., Flynn, R. W., Kirchhoff, M. D., Schoen, J. W., and Shea, L. C. (1993). “Habitat capability model for Sitka black-tailed deer in Southeast Alaska: winter habitat,” in Habitat Capability Models for Wildlife in Southeast Alaska, ed. L. H. Suring (Juneau, AK: USDA Forest Service, Alaska Region).
Szepanski, M. M., Ben-David, M., and Van Ballenberghe, V. (1999). Assessment of anadromous salmon resources in the diet of the Alexander Archipelago wolf using stable isotope analysis. Oecologia 120, 327–335. doi: 10.1007/s004420050866
Treves, A., Naughton-Treves, L., and Shelley, V. (2013). Longitudinal analysis of attitudes toward wolves. Conserv. Biol. 27, 315–323. doi: 10.1111/cobi.12009
U.S. Fish and Wildlife Service [USFWS] (2020). Endangered and Threatened Wildlife and Plants; 90-day Findings for Three Species. Washington, DC: USFWS. Federal Register (86) 141.
U.S. Fish and Wildlife Service [USFWS] (1997). 12-month Finding for a Petition to List the Alexander Archipelago Wolf as Threatened and to Designate Critical Habitat. Washington, DC: USFWS. Federal Register (62) 46710.
U.S. Fish and Wildlife Service [USFWS] (2014). 90-day Finding on a Petition to List the Alexander Archipelago Wolf as Threatened or Endangered. Washington, DC: USFWS. Federal Register (79) 17993.
U.S. Fish and Wildlife Service [USFWS] (2015). Species Status Assessment for the Alexander Archipelago Wolf (Canis lupus ligoni). Version 1.0. Anchorage, AK: Alaska Region.
United States Forest Service (2008). Tongass Land and Resource Management Plan Amendment. 2008 Environmental Impact Statement Record of Decision. Juneau, AK: United States Forest Service.
United States Forest Service (2009). Access Travel Management Plan Environmental Assessment Prince of Wales and Surrounding Islands. Juneau, AK: United States Forest Service.
Wardle, D. A., Bonner, K. I., and Barker, G. M. (2002). Linkages between plant litter decomposition, litter quality, and vegetation responses to herbivores. Funct. Ecol. 16, 585–595. doi: 10.1046/j.1365-2435.2002.00659.x
Weckworth, B. V., Talbot, S., Sage, G. K., Person, D. K., and Cook, J. (2005). A signal for independent coastal and continental histories among North American wolves. Mol. Ecol. 14, 917–931. doi: 10.1111/j.1365-294X.2005.02461.x
White, K. S., Pendleton, G. W., and Hood, E. (2009). Effects of snow on Sitka black-tailed deer browse availability and nutritional carrying capacity in Southeast Alaska. J. Wildlife Manag. 73, 481–487. doi: 10.2193/2007-499
Wolf Technical Committee (2017). Interagency Wolf Habitat Management Program: Recommendations for Game Management Unit 2. Management Bulletin R10-MB-822. Juneau, AK: USDA Forest Service, USDI Fish and Wildlife Service, and Alaska Department of Fish and Game
Yatskov, M. A., Harmon, M. E., Barrett, T. M., and Dobelbower, K. R. (2019). Carbon pools and biomass stores in the forests of Coastal Alaska: uncertainty of estimates and impact of disturbance. Forest Ecol. Manag. 434, 303–317. doi: 10.1016/j.foreco.2018.12.014
Keywords: Tongass National Forest, Alaska (United States), ecosystem service (ES) values, predator-prey interactions, social-ecological systems (SES), Endangered Species Act, hunting, Canis lupus ligoni
Citation: Gilbert SL, Haynes T, Lindberg MS, Albert DM, Kissling M, Lynch L and Person D (2022) Potential Futures for Coastal Wolves and Their Ecosystem Services in Alaska, With Implications for Management of a Social-Ecological System. Front. Ecol. Evol. 10:809371. doi: 10.3389/fevo.2022.809371
Received: 04 November 2021; Accepted: 04 February 2022;
Published: 29 April 2022.
Edited by:
Bridget Borg, Denali National Park and Preserve, United StatesReviewed by:
Robert W. Myslajek, University of Warsaw, PolandPerry S. Barboza, Texas A&M University, United States
Copyright © 2022 Gilbert, Haynes, Lindberg, Albert, Kissling, Lynch and Person. This is an open-access article distributed under the terms of the Creative Commons Attribution License (CC BY). The use, distribution or reproduction in other forums is permitted, provided the original author(s) and the copyright owner(s) are credited and that the original publication in this journal is cited, in accordance with accepted academic practice. No use, distribution or reproduction is permitted which does not comply with these terms.
*Correspondence: Sophie L. Gilbert, c29waGllZ2lsYmVydEB1aWRhaG8uZWR1