- 1Department of Biology, University of British Columbia, Kelowna, BC, Canada
- 2Institute for Biodiversity, Resilience, and Ecosystem Services, University of British Columbia, Kelowna, BC, Canada
- 3Department of Earth, Environmental and Geographic Sciences, University of British Columbia, Kelowna, BC, Canada
Within the Anthropocene the functional diversity of coral communities is changing rapidly, putting the resilience of many coral reef ecosystems in jeopardy. A better understanding of the relationship between coral functional diversity and reef resilience could reveal practical ways to achieve increased resilience. However, manipulating coral diversity experimentally is challenging, and consequently the links between coral functional diversity, resilience, and ecosystem functioning remain obscure. We used an ecologically detailed agent-based model to conduct a virtual experiment in which functional diversity was manipulated over the entire trait space of scleractinian corals. Using an imputed trait dataset of 798 coral species and eight key functional traits, we assembled 245 functionally distinct coral communities, which we subjected to a cyclone and bleaching event. We then measured four different aspects of their resilience and quantified for each measure the respective effect of (i) the functional richness (FRic), and (ii) community-weighted means (CWM) of four types of trait: effect, resistance, recovery, and competitive. FRic represents the volume occupied by a community in the functional space, while CWM indicates the location of the communities’ centroid in the functional space. We found a significant and positive effect of FRic on three measures of resilience: communities with higher FRic recovered surface cover faster and had more rugosity and cover 10 years after the disturbances. In contrast, the resistance of the coral community—i.e., the capacity to maintain surface cover when subjected to the disturbances—was independent of FRic and was determined primarily by the CWM of resistance traits. By analyzing community dynamics and functional trade-offs, we show that FRic increases resilience via the selection and the insurance effects due to the presence of competitive species in the functional space, i.e., those highly dominant species that contribute the most to the complexity of the habitat and recover quickly from disturbances. Building from the results of our experiment and the trait correlation analysis, we discuss the potential for FRic to serve as a proxy measure of resilience and we present a strategy that can provide direction to on-going reef restoration efforts, and pave the way for sustaining coral communities in a context of rapid global change.
Introduction
Understanding the effect of biodiversity on resilience—the capacity of an ecosystem to maintain or recover the processes, functions and structures that define its identity when facing disturbances (Walker et al., 2004)—has been a fundamental objective in ecology (Macarthur, 1955; May, 1972; Pimm, 1984; McCann, 2000) and remains all the more relevant as global change is altering species diversity worldwide (Cardinale et al., 2012; Hooper et al., 2012; Oliver et al., 2015). By linking diversity to processes, trait-based approaches are key to understanding and predicting the response of ecosystems to disturbances (Loreau and de Mazancourt, 2013; Oliver et al., 2015; Madin et al., 2016b). However, this is especially challenging in diverse ecosystems because of the numerous potential ways traits could combine and interact to govern resilience. The prevalence of important trade-offs among functional traits (Grime, 1977; Darling et al., 2012; Díaz et al., 2016) narrows down these possibilities and offers the opportunity to define predictive frameworks (Suding et al., 2008; Carturan et al., 2018). Combining conventional experimental approaches with trait-based frameworks is an optimal strategy to piece together multiple lines of evidence. However, such experiments can be logistically unfeasible for certain ecosystems such as coral reefs. In this study, we conduct a simulation experiment in which coral diversity is manipulated over an entire Scleractinian coral trait space to quantify the relationship between aspects of functional diversity and resilience. We also undertake a novel assessment of coral trait correlations that reveals the key trade-offs and associated mechanisms responsible for the results of our experiment.
Decades of research have revealed a variety of ways (referred to hereafter as “effects”) by which biodiversity can influence the functioning and resilience of ecosystems (McCann, 2000; Walker et al., 2004; Cardinale et al., 2012; Hooper et al., 2012; Oliver et al., 2015; van der Plas, 2019). Species can contribute individually to an ecosystem function via (i) “dominance” (or “mass ratio”) and (ii) “identity” effects—the distinction being abundance-driven versus contribution to functioning, respectively (Grime, 1998; McLaren and Turkington, 2010; Longo et al., 2013). Species can also contribute collectively to functioning via (iii) “complementarity” (or “niche complementarity”) and (iv) “facilitative” effects, which produce a positive diversity-ecosystem functioning relationship and overyielding (Tilman et al., 2014; van der Plas, 2019). Finally, (v) the “selection” (or “sampling”) effect produces a correlation between diversity and ecosystem functioning due to random chance. The correlation is either negative or positive, depending on whether competitiveness is positively or negatively associated with functioning (Loreau, 2000; see Supplementary Table 1 for a definition of these effects). In the diversity-resilience realm, species contribute collectively to resilience via (vi) the “portfolio effect” (or “statistical averaging”), and (vii) the “insurance effect” (or “compensatory fluctuations”), which involves functional redundancy and response diversity (Supplementary Table 1). An important distinction between the two effects is that population fluctuations are independent in the portfolio effect (Griffin et al., 2009; Mori et al., 2013). These effects can occur concurrently and are dependent on the strength of interspecific competition.
Identifying which effects are responsible for observed ecosystem dynamics can be achieved by quantifying the covariation between measures of resilience or ecosystem functions and aspects of functional diversity (e.g., Pillar et al., 2013; Cadotte, 2017). These aspects of functional diversity can be described using single-trait indices, such as community-weighted means (CWM) (Violle et al., 2007), and multiple-trait indices, such as functional richness (FRic), i.e., the volume in functional space filled by a community (Mason et al., 2005; Villéger et al., 2008; Laliberté and Legendre, 2010; Mouchet et al., 2010). For instance, a strong dominance effect should yield a correlation between CWM of effect traits—traits involved in the delivery of an ecosystem function—and ecosystem functioning (Grime, 1998). Multi-trait indices can be used as proxies for effects that require multiple traits, such as the insurance effect (e.g., Laliberté et al., 2010). We reiterate, however, that to be most informative, analyses of functional diversity indices need to be complemented by analyses that ascribe process and mechanism to particular traits, and that also consider how trade-offs may constrain the universe of possible ecosystem responses. This is illustrated in the following section.
Resolving how functional traits drive resilience can be achieved by experiments that manipulate community trait composition. Specifically, the ways by which functional diversity affects resilience can be distinguished by measuring how community resilience depends on the unique and interactive effects of communities’ centroid location (CWM) and volume (FRic) in functional space. To help describe such an experiment and its potential outcomes, we present three scenarios that make simplifying assumptions and involve only two functional traits (Figure 1). Note that different effects can be revealed in each scenario depending on the traits considered and the trade-offs among them. Though simplified, these scenarios help illustrate the complexity inherent to understanding how traits and trade-offs can influence resilience.
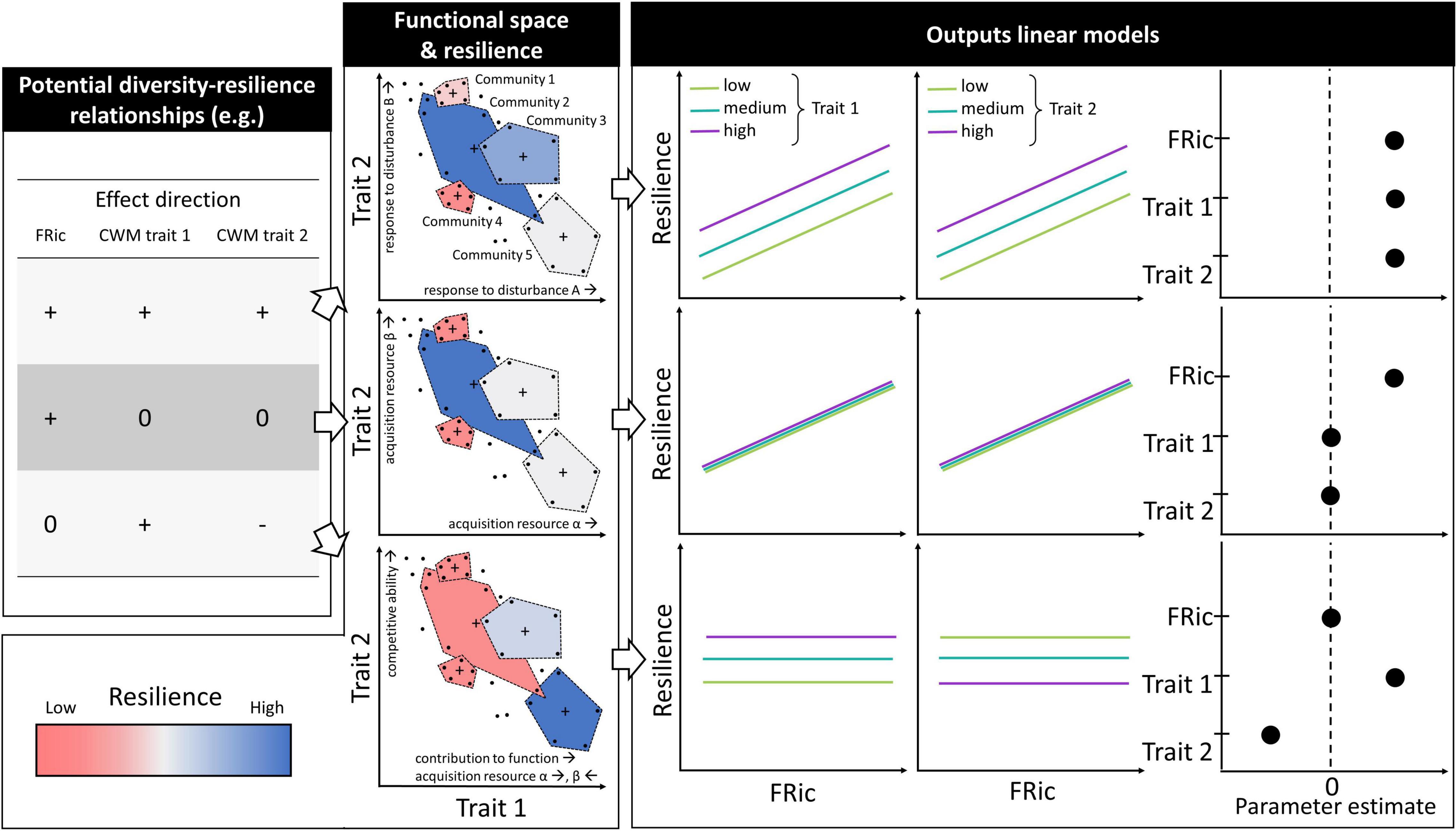
Figure 1. Examples of potential effect directions of functional richness (FRic) and community weighted mean (CWM) of traits on resilience, with corresponding idealized outputs from linear models, including bivariate relationships and parameter estimates. Each of the plots under “Functional space and resilience” show the same four communities (polygons), each of which comprises five species (black dots) and have different CWM of traits (crosses) and FRic (area of polygons). The functional space is defined by only two traits for simplicity, and these two traits are negatively correlated. The color of the community polygons represents their resilience, which depends on the sign of the effect of FRic and the CWM of traits (table on the left). The green, turquoise and purple lines represent idealized model fits with one trait set to a low, medium and high value, respectively, and the other trait set to a medium value. Interactions between FRic and CWM of traits are not depicted here but are included in the results. The figure depicts three scenarios where FRic and CWM of traits have different effects on resilience due to different mechanisms and trait compositions: the insurance effect with two response traits associated to disturbances A and B, respectively (upper panels); the complementarity effect with two traits involved in the acquisition resources α and β, respectively (middle panels); the offsetting combination of the dominance and complementarity effects with trait one involved in the contribution to a function and also in the acquisition of resources α and β (bottom panels), and trait two a competitive trait (see text for details).
In scenario one, FRic and the CWM of traits one and two have a positive effect on resilience. This could be observed under the insurance effect where traits one and two are response traits that are each positively associated with the capacity to withstand a different disturbance, and are themselves negatively correlated. Additionally, species contribute to ecosystem functions in proportion to their abundance, and no trade-off exists between species’ effect and response traits. Communities with higher FRic are more resilient because they have a higher response diversity.
In scenario two, FRic has a positive effect on resilience while the CWM of traits one and two have no effect. This could be observed under a strong complementarity effect where traits one and two positively contribute to the acquisition of different resources, and are themselves negatively correlated. Again, species contribute to ecosystem functions in proportion to their abundance, and there is no trade-off between the species effect and resource acquisition. Assuming that the CWM of traits one and two have equal effect size, the functional trade-off implies that communities with equal FRic have equal resilience. Importantly, communities with higher FRic are more resilient because they have a combination of species that are better at exploiting the resources and have consequently higher abundances.
In scenario three, FRic has no effect on resilience, and the CWM of trait one and trait two have a positive and negative effect, respectively. No effect of FRic could occur due to two offsetting mechanisms where (i) trait one is an effect trait positively associated with the species’ contribution to an ecosystem function, and is also involved in resources acquisition (with different values implying being good at exploiting different resources), and (ii) trait two is positively associated with competitiveness and is negatively correlated with trait one. FRic simultaneously enhances resilience via the complementarity effect and promotes the extirpation of the species that contribute the most to the ecosystem function via competitive exclusion (i.e., the dominance effect).
The experimental design we propose is particularly suitable for ecosystems such as coral reefs, where resilience and functions are governed by notable functional trade-offs and feedback processes. Specifically, the resilience of coral reefs depends directly on the feedback process between habitat provisioning and herbivory (Mumby and Steneck, 2008; Vergés et al., 2011; Bozec et al., 2013). By providing a complex habitat, corals support fish and macroinvertebrate diversity (Holbrook et al., 2002; Fabricius et al., 2014; Nelson et al., 2016; Plass-Johnson et al., 2016), and in particular, diverse populations of herbivores. By mediating the competition between corals and algae (Rasher and Hay, 2010; Adam et al., 2015), herbivory prevents coral reefs from falling into non-coral alternative stable states (Bellwood et al., 2004; Hughes et al., 2010). Given the importance of coral morphological complexity for reef complexity and herbivory (Alvarez-Filip et al., 2011, 2013; Heenan et al., 2016; Darling et al., 2017), and its strong trade-off with the capacity to compete, grow, recruit, and respond to disturbances (Loya et al., 2001; Madin et al., 2014; Álvarez-Noriega et al., 2016; Precoda et al., 2017; Carturan et al., 2018), it is likely that varying coral functional diversity with the relevant traits should yield measurable effect sizes of FRic and CWM on resilience.
Resolving the effects of functional diversity on coral reef resilience is critical due to the unprecedented compositional changes happening in coral communities (owing to climate change), which is altering the habitat provisioning function and the associated herbivory, and consequently jeopardizing the resilience of coral reefs (Graham et al., 2014; Hughes et al., 2018; Perry and Alvarez-Filip, 2019; Williams and Graham, 2019). But addressing this objective through empirical experiments is logistically extremely challenging and such attempts are consequently lacking (Brandl et al., 2019). The very few experiments aimed at elucidating the influences of diversity on ecosystem functions and resilience have manipulated a handful of species and traits (McWilliam et al., 2018a; Clements and Hay, 2019). These experiments yield valuable insights but may have limited applicability to the real world, where the diversity of species and traits can be extremely high (Hughes et al., 2002), and their composition varies substantially within and across geographic regions (McWilliam et al., 2018b). Consequently, critical knowledge gaps remain and preclude predictions about the functioning of these “novel” ecosystems (Graham et al., 2014; Hughes et al., 2017a).
We addressed these gaps by conducting a simulation experiment using a validated, ecologically detailed, trait-based agent-based model (Carturan et al., 2020). Informed by imputed trait data for 798 coral species (Appendix 1 in Carturan et al. (2020)), we manipulated functional diversity over an unparalleled extent and examined key responses to disturbance over scales of time and space unmanageable with real-life experiments. We first quantified correlations among the eight functional traits that informed our model (Table 1), which revealed the trade-offs that constrain the ways by which species and their traits contribute to resilience. Next, we untangled the respective contribution of FRic and CWM of traits to resilience by identifying the effects responsible for the biodiversity-resilience associations we found using the results of the trait correlation analysis. Finally, we build on these results to discuss the role of FRic as a reliable indicator of resilience, and how the results of our experiment can inform active restoration practices (Rinkevich, 2014, 2021).
Materials and Methods
Software
We used a spatially explicit agent based model written in the Java object-oriented programming language Repast Simphony 2.5.0 (North et al., 2013) to run simulations and R (version 3.5.0, R Core Team, 2017) to manage the simulations. We launched simulations using the R package rrepast 0.7.0 (Prestes García and Rodríguez-Patón, 2016) and rJava 0.9-10 (Urbanek, 2018). We used R (version 3.6.1, R Core Team, 2019) to manipulate datasets and conduct statistical analyses. Further details of the model, including a full Overview, Design, Concept and Details (ODD) are provided in Appendix 2 in Carturan et al. (2020).
Model Description
We used a spatially explicit, stochastic, demographic, trait-based, and agent-based model that simulates the dynamics of functionally diverse coral communities under different environmental conditions. The model represents processes at different spatiotemporal scales (e.g., larval settlement, spatial interactions, overtopping, colony dislodgement, bleaching, grazing, and larval dispersion), their interactions, and the contribution of species functional diversity to ecosystem dynamics. The model enables manipulation of the intensity and frequency of hydrodynamic (i.e., waves and cyclones) and thermal (i.e., bleaching) disturbances as well as larval connectivity and sedimentation. Importantly, the positive feedback between habitat provisioning by corals and herbivory (see section “Introduction”) is implemented because grazing pressure depends directly on the complexity of the habitat created by coral colonies (expressed as reef rugosity). These different abiotic factors influence species interactions and determine the dominance and exclusion of coral species and algae functional groups as a function of their traits (c.f. the hierarchically structured validation in Appendix 5 in Carturan et al. (2020)). The simulated coral community is completely configurable to represent real or fictitious reef systems and can be assembled by sampling species from their functional trait space. Ten functional traits are used to determine how each species grows, reproduces, competes, and responds to disturbances. Most of the parameter values and submodels of ecological processes implemented are based on empirical studies. The model has been calibrated and validated using empirical datasets of three Caribbean reefs and successfully reproduces interspecies dynamics and reef responses to bleaching and cyclone events [cf. Carturan et al. (2020) for complete description of the ecological processes implementation and the model calibration and validation].
Experimental Design
We assembled communities that had unique trait structure, that is, unique combinations of FRic and CWMs, and simulated scenarios subjecting them to a cyclone and a bleaching event. We determined resilience by measuring resistance and three different aspects of the post-disturbance emergent community dynamics, which we compared among communities (Supplementary Figure 1).
Construction of the Communities
We initiated communities of nine coral species by selecting species from a functional space we defined using the traits that have a direct and clear implication in certain processes: (1) bleaching susceptibility; (2) colony complexity; (3) colony maximum diameter; (4) dislodgement susceptibility; (5) colony fecundity; (6) (vegetative) growth rate; (7) mode of larval development; (8) overtop capacity (cf. Table 1 and Supplementary Material: section 2.2 for more details). In the model these traits determine how a colony resists a disturbance (i.e., dislodgement susceptibility, bleaching susceptibility), recovers from it (i.e., colony fecundity, mode of larval development, and growth rate), competes (overtop capacity and growth rate), and contributes to the habitat provisioning function and consequently grazing (i.e., colony complexity).
We reduced the number of dimensions of the functional space by conducting a principal component analysis (PCA) on the correlation matrix, retaining the first four of eight principal components (Supplementary Material: section 2.4), using the prcomp function from the R package stats 3.6.1. We then developed an algorithm to assemble 245 different communities, each initially comprising nine species, and each exhibiting unique functional trait characteristics; specifically, the communities’ centroids collectively span the entire functional space, and the range of functional volume occupied spans over four orders of magnitude (Supplementary Material: section 2.4).
Model Configuration and Scenarios
We defined a 25 m2 benthic surface and a six-month time step. We activated the feedback process between architectural complexity created by the coral community and grazing pressure data (Appendix 2: section 7.1.2.2 in Carturan et al. (2020)). In all simulations we set the initial cover of each coral species, macroalgae, turf and crustose coralline algae to 7.5% each. We maintained a mild and constant wave hydrodynamic regime (dislodgement mechanical threshold = 200), and triggered an intense pulse disturbance at year four, either a cyclone (dislodgement mechanical threshold = 30; the value corresponds approximatively to the effect on the reef crest of a cyclone of category 3 on the Saffir-Simpson scale accorging to Madin and Connolly (2006)) or a bleaching event (degree heating = 14°C-weeks) depending on the scenario. We simulated the effect of the disturbance on larval connectivity (Connell, 1997; Gilmour et al., 2013) by initially setting the number of external larvae entering the reef at 700 m–2, then suppressing it in the 6 months following the disturbance and letting it increase to 700 m–2 during the subsequent 10 years using the following model:
where larvaeexternal = number of external larvae m–2, time is in year. We ran the simulations for 20 years. Finally, we imposed a minimum of 30% of cover grazed to represent the presence of other grazers, such as sea urchins, which are not dependent on the rugosity created by coral colonies.
Dependent Variables
We defined four dependent variables that represent some of the numerous different definitions of resilience (Carpenter et al., 2001; Desjardins et al., 2015). Resiliencecover and resiliencerugosity are the 10 years post-disturbance total coral cover and reef rugosity, respectively; together they capture the definition of resilience provided by Walker et al. (2004) and provided above, namely, the capacity of the ecosystem to absorb disturbance and to reorganize while undergoing changes so as to still retain essentially the same function, structure, identity, and feedbacks. As it is relevant to estimate resilience by measuring its two complementary aspects resistance and recovery (Nyström et al., 2008; McClanahan et al., 2012; Hodgson et al., 2015), we defined resistancecover—one minus the proportional reduction of total coral cover caused by the pulse disturbance and recoverycover—the rate of recovery during the first 3 years after the disturbance (% cover year–1) represent two complementary aspects of resilience (Supplementary Figure 8). For each measure of resilience, we retained the minimum resilience values obtained between the bleaching and cyclone scenario. We retained the minimum value rather than calculating the mean because it is more useful to know, from a management perspective, the absolute vulnerability of an ecosystem rather than its average vulnerability over several disturbances (Supplementary Figure 1). But for comparison, we also provide the results of the statistical analyses obtained with using the mean values (Supplementary Material: section 2.3). Note that we did not define resistancerugosity and recoveryrugosity because the bleaching disturbance does not directly reduce the rugosity of the reef as the skeleton of dead coral colonies remain after the event.
Independent Variables
We described the trait structure of the communities created in section “Construction of the Communities” with three independent variables: functional richness (FRic)—the convex hull volume index (Villéger et al., 2008; Laliberté and Legendre, 2010)—and two variables, PC.1 and PC.2, representing aggregated CWM of traits. We calculated FRic from the first four principal components of a PCA we conducted on the species × traits dataset (Supplementary Figures 4A,B). We log-transformed FRic to reduce the skewness of its distribution (Supplementary Figure 6). We calculated FRic using the R code provided by Mouillot et al. (2013) and using the convhulln function from the R package geometry 0.4.5 (Habel et al., 2019). PC.1 and PC.2 are the first two principal components of a PCA we conducted using the communities × CWM of traits dataset (Supplementary Figure 4C). We centered and scaled FRic, PC.1 and PC.2 to a mean of zero and unit variance in order to compare their effect sizes (Figure 1); we used the scale function from the R package base 3.6.1.
Statistical Analyses
Trait Correlations
We used the PCA performed on the species × traits dataset (n = 798 species and eight traits; cf. section “Construction of the Communities”) to quantify the overall covariations and trade-offs in the trait space. We determined species density in the trait space defined by the first two principal components using kernel density estimation and the kde function from the R package Ks 1.13.1 (Duong et al., 2021). We performed Spearman rank correlations to quantify associations between two continuous or ordinal traits using the cor.test function from the R package Stats 3.6.1. We calculated the Glass rank biserial correlation to quantify the association between a continuous or ordinal trait and a dichotomous trait (Glass, 1965); we determined 95% confidence limits by bootstrap using the percentile method and 1,000 randomisations; we used the wilcoxonRG function from the R package rcompanion (Mangiafico, 2020).
Quantifying Functional Richness and CWM of Traits to Resilience
For each measure of resilience (resiliencecover, resiliencerugosity, resistancecover, and recoverycover), we first fitted full (i.e., with all three explanatory variables and their interactions) linear model candidates (i.e., with different link functions or variance structures). We selected the best full model using the corrected Akaike information criterion (AICc)—we used the AICc because sample size divided by number of parameters < 40 (Burnham and Anderson, 2002)—and residual diagnostic plots (i.e., to check normality and homoscedasticity of the residuals). From each full model selected, we generated models with all possible combinations of predictors, selected the 95% confidence set using AICc, and calculated model-averaged coefficients and 95% confidence intervals using the “full average” method (Bartoń, 2019). We conducted model averaging using the R package MuMIn 1.43.6 (Bartoń, 2019).
For resiliencecover and resistancecover we fitted beta regressions using the R package betareg 3.1.3 (Cribari-Neto and Zeileis, 2010); we used this approach because beta regressions are appropriate with response variables that represent proportions and two categories (e.g., coral and non-coral cover) (Douma and Weedon, 2019). In order to obtain the best full model, we fitted and compared all possible full model candidates considering each link function for the mean model (i.e., logit, probit, cauchit, log, cloglog, and loglog), and each link function (i.e., identity, log, and sqrt) and each possible combination of predictors (and their interactions) for the precision model (phi). Following Espinheira et al. (2008) recommendation, we used the “standardized weighted residuals 2” for the diagnostic plots. We removed one outlier to meet the assumptions of the full model for resistancecover (Supplementary Figures 15, 16). We verified that the maximum likelihood estimator did not overestimate the parameters of the selected full beta regression models by comparing the parameter estimates of the same models but using bias-corrected and bias-reduced estimators (Grün et al., 2012). The selected full and averaged models for resiliencecover and resistancecover are presented in Supplementary Tables 7, 8, 9, 10, respectively. We computed the confidence interval of the fitted values using bootstrap using the percentile method and 1,000 replications.
For resiliencerugosity we fitted gamma generalized linear models—after subtracting one to the response variable so it belonged to [0,+∞)—and compared full model candidates with different link functions (i.e., inverse, identity, and log). We fitted the models using the glm function from the R package stats 3.6.1. We removed four outliers to meet the models’ assumptions using the outlierTest function from the R package car 3.0.3 (Fox and Weisberg, 2019; Supplementary Figures 10, 11). We used the deviance residuals to check the full models’ assumptions (Zuur et al., 2009b and references therein). We present the selected full and averaged models in Supplementary Tables 5, 6, respectively.
We fitted full linear models for recoverycover and applied residual variance structure to account for identifiable structure in the error residuals (Zuur et al., 2009a). First, we used AICc to determine the best variance structure function to apply to each variable (dependent and independent) by comparing a full linear model and full models with a variance structure applied to one variable, for each variance structure available (i.e., fixed, exponential, constant plus power of the variance covariate functions). We then fitted full model candidates for all the possible variables-variance structure function associations. We present the full linear model, the model with variance structure and the averaged model with variance structure in Supplementary Tables 11, 12, 13, respectively.
To evaluate model performance, we calculated for each model the McFadden’s (1977) pseudo R2 = one minus the ratio between the likelihood values for the fitted and null model. Its value is comprised between 0 and 1 for logistic regression models and tends to be considerably lower than other pseudo R2; for instance, values between 0.2 and 0.4 are considered “an excellent fit.” Note that McFadden’s pseudo R2 is negative if the models’ log likelihood values are positive. For instance, values < −1 correspond cases where the goodness of fit of the model is more than twice larger than the goodness of fit of the null model. We obtained the McFadden’s pseudo R2 of the averaged models by calculating the weighted average of the McFadden’s pseudo R2 of the models in the 95% confidence set using their AICc weights (rescaled to the model set). For comparison, we calculated another pseudo R2, defined as the square of the sample correlation coefficient between the original and predicted values of the response variable on the scale of the link function (Ferrari and Cribari-Neto, 2004).
Results
Distribution of Traits Across Species and Communities
The coral trait space is highly restricted due to strong trait correlations and trade-offs: 72.4% of the species variation within the eight-dimensional functional space is contained in the plane defined by the first two principal components of the PCA (Figure 2). The first principal component represents 48% of variance and discriminates species according to their growth rate, colony fecundity, dislodgement susceptibility, overtop capacity, and colony complexity (cf. loadings in Supplementary Table 3). The first principal component clearly separates competitive from stress-resistant species. The second principal component explains 25% of variance and represents bleaching susceptibility, colony maximum diameter and mode of larval development. Weedy species, which are mostly brooders, are situated at lower values along the second principal component.
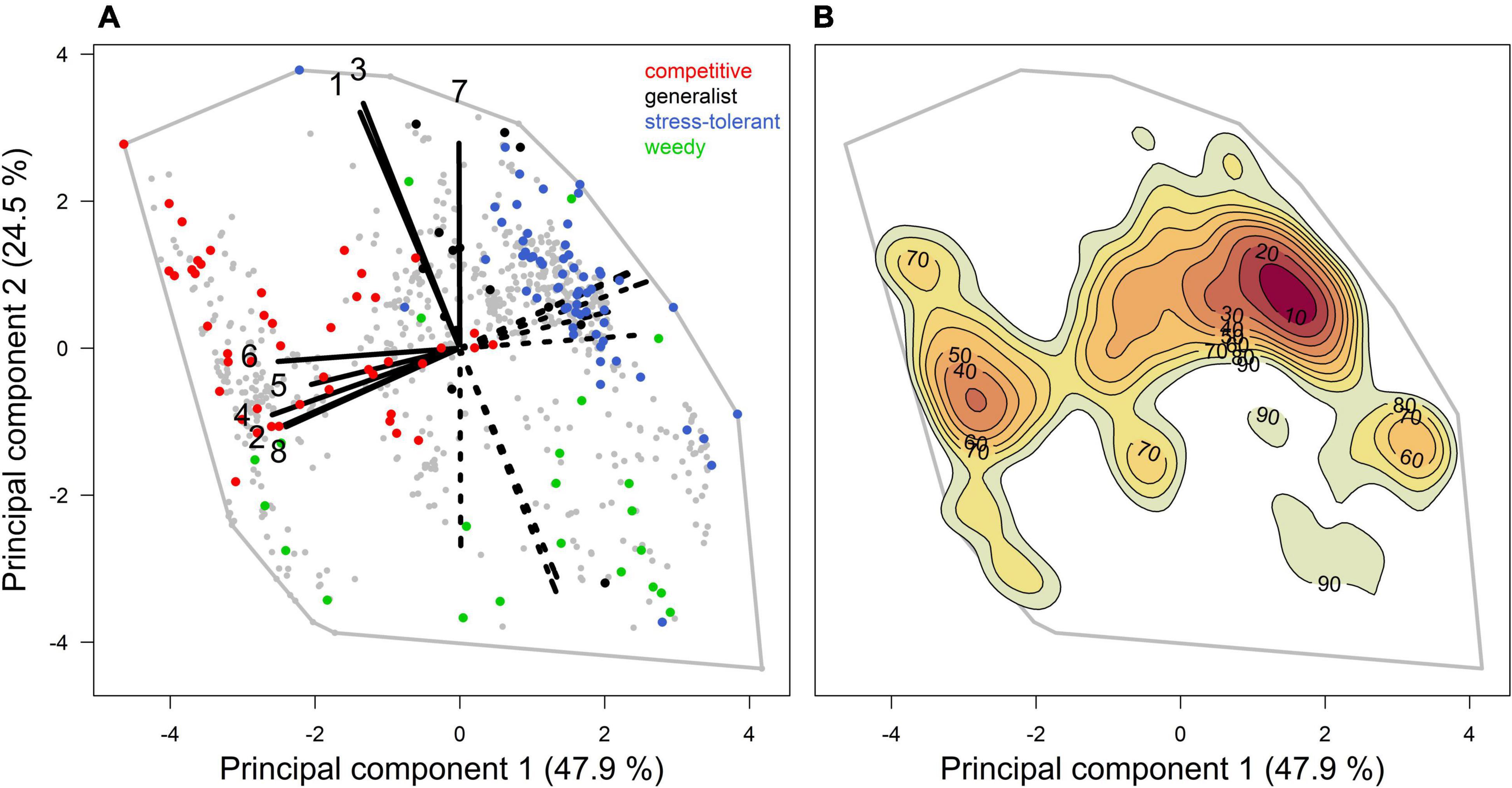
Figure 2. Coral functional space from which we sampled coral species. Biplot (A) of the principal component analysis (PCA) shows the species distribution (n = 798; gray dots in the left panel) and the loadings of the first and second principal components (black segments) which represent the following traits: (1) bleaching susceptibility, (2) colony complexity, (3) colony maximum diameter, (4) dislodgement susceptibility, (5) colony fecundity, (6) growth rate, (7) mode of larval development, and (8) overtop capacity (cf. Supplementary Table 2 for the values of the loadings). The red, black, blue, and green dots represent the competitive, generalist, stress-tolerant, and weedy species, respectively (cf. Darling et al. (2012) classification). Biplot (B) describes the species density with color gradient and contour lines (based on kernel density estimation). Note that we sampled species in the space defined by the first four principal components (cf. Construction of the Communities and Supplementary Figure 4).
The trait correlation analysis reveals key functional trade-offs (Figure 3). First, the species contributing the most to the complexity of the habitat are generally more susceptible to dislodgement (rs = 0.71) and, to a lesser extent, bleaching (rs = 0.14). They are, however, better at recovering via vegetative growth (rs = 0.68) and/or recruitment (rs = 0.64). Importantly, they are strong spatial competitors due to their capacity to overtop other organisms (rg = −0.78), which is explained by the presence of branching and plating species in the trait dataset. Second, non-resistant species are usually able to recover quickly and vice versa. Species susceptible to dislodgement have on average a faster growth rate (rs = 0.76) and a higher colony fecundity (rs = 0.56). Species susceptible to bleaching tend to be susceptible to dislodgment (rs = 0.21) and grow faster (rs = 0.28). Finally, species with a fast growth rate tend to have a high colony fecundity (rs = 0.65).
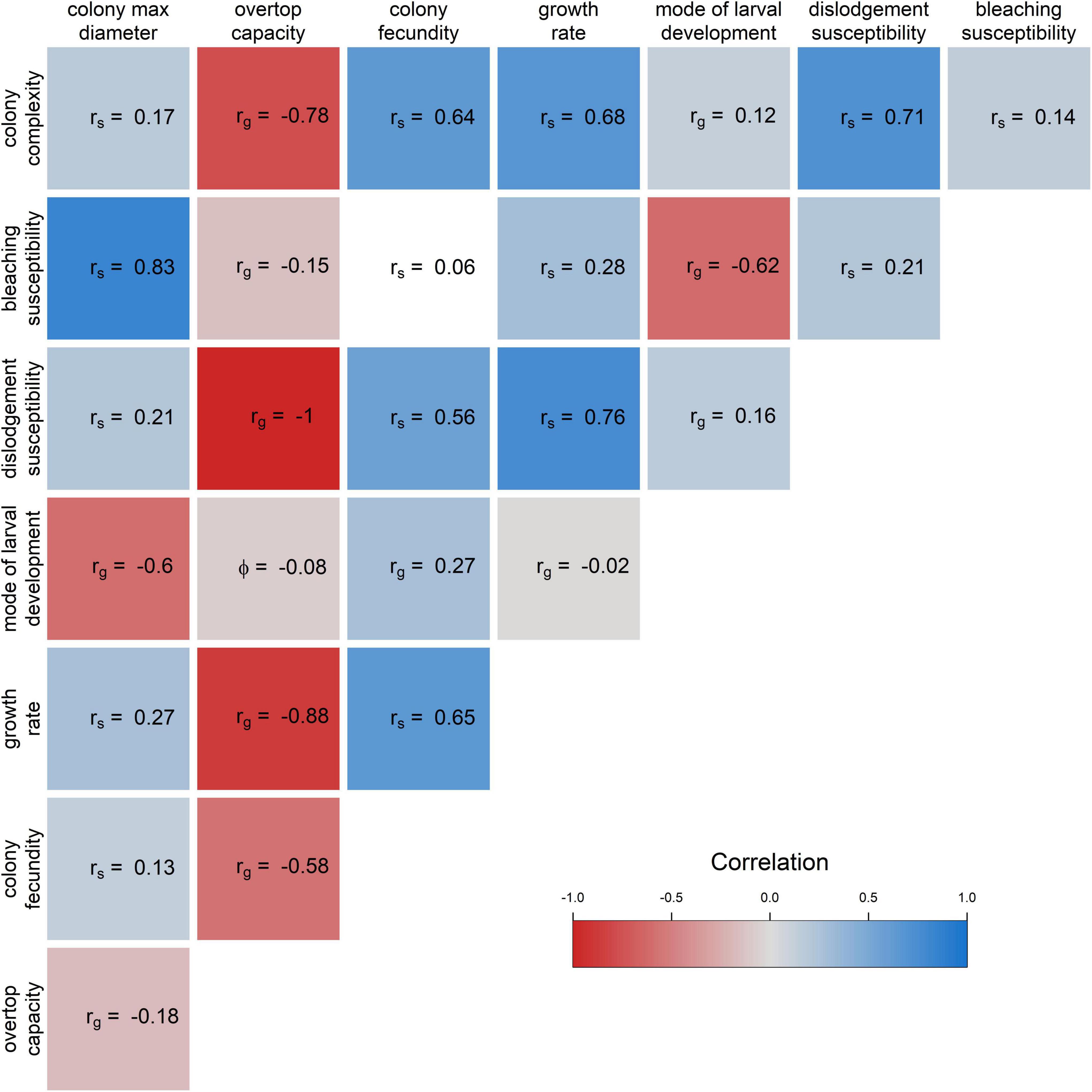
Figure 3. Pairwise correlation matrix of the functional traits (n = 798) (cf. Table 1 for a complete description of these traits) We used the Spearman’s rank correlation statistic (rs), the Glass’ rank biserial correlation statistic (rg) and the mean square contingency coefficient (ϕ) to calculate the correlations between two continuous variables, one continuous and one dichotomous variable and between two dichotomous variables, respectively (cf. Supplementary Figure 9 for a visualization of the data).
Trade-offs and constraints in trait values among species translated to similar trade-offs and constraints in trait CWM across assembled communities. There is a strong similarity between the PCA performed on the species × traits dataset and the one performed on the communities × CWM of traits dataset (Supplementary Figure 4). In the latter, the first principal component (PC.1) represents 53% of variance and is negatively associated with colony complexity, dislodgement susceptibility, colony fecundity, growth rate, overtop capacity. The second principal component (PC.2) represents 27% of variance and is negatively associated with bleaching susceptibility, colony maximum diameter and mode of larval development (cf. loadings in Supplementary Table 4).
Strong Positive Effect of Functional Richness on Overall Resilience and Recovery
The effect sizes on resiliencerugosity, resiliencecover, and recoverycover were positive for FRic and negative for PC.1 (Figure 4). These results are due to the combined effect of the rugosity-grazing feedback process and trait correlations. The feedback process was strong as it permitted communities able to provide sufficient rugosity to recover rapidly from disturbances (both cover and rugosity), while it led those with the lowest levels of rugosity to collapse even before the onset of the disturbances (Supplementary Figures 21, 22). The strong positive correlations between colony complexity, growth rate, and colony fecundity (Figure 3) further granted the communities with higher CWM of these traits (i.e., low PC.1) a stronger capacity to provide and recover rugosity and total cover, which further enhanced rugosity (Supplementary Figures 12, 14, 20). Additionally, the fact that many of the species with complex morphologies and capable of recovering quickly can also overtop other organisms further contributed to the resilience of their communities. PC.1 had a strong negative effect because it captured most of the variation in the CWM of these traits (with lower values of PC.1 corresponding to higher values of these traits).
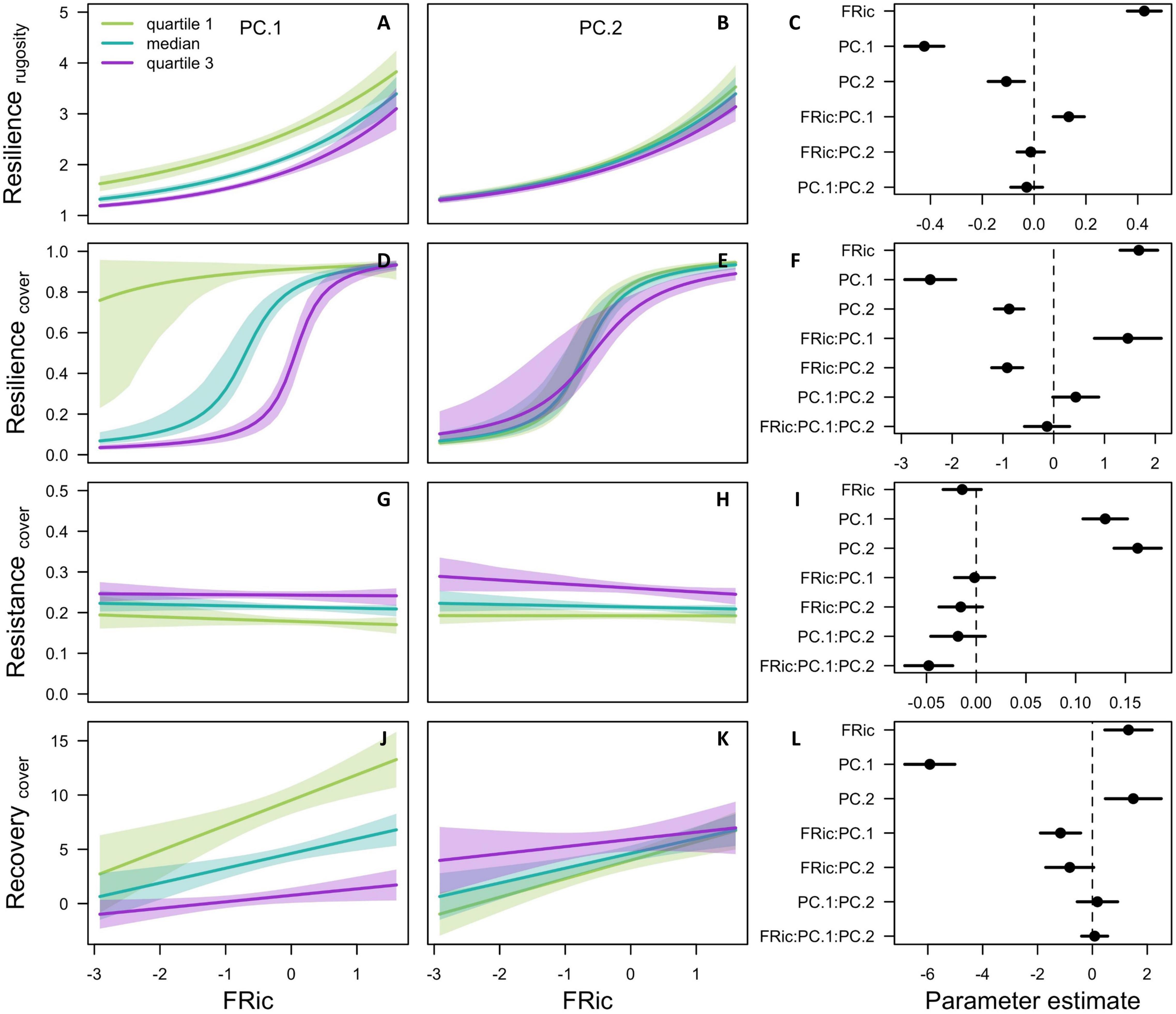
Figure 4. Experimental results depicting interacting effects of FRic and CWM of traits on ecosystem resilience. Left and center panels show conditioning plots of FRic (X-axes) versus one of four measures of resilience (Y-axes), conditioned by CWMs of traits, specifically PC.1 (panels A,D,G,J) and PC.2 (B,E,H,K). Green, turquoise, and purple lines represent model fits conditioned on the 1st, 2nd, and 3rd quartiles, respectively, of the conditioning variable (each n = 245). In all cases the secondary conditioning variable (either PC.1 or PC.2) was held at its median value. Right-hand panels show model-averaged parameter estimates and their 95% confidence intervals (C,F,I,L).
The strong positive effects of FRic on resiliencerugosity, resiliencecover, and recoverycover were due to the occurrence of combined positive selection and dominance effects in most of the resilient communities. Species with complex colonies tend to be competitive due to their capacity to overtop and their fast growth rates and/or colony fecundity. The dominance effect occurred in the communities where at least one such species was present as it occupied a larger surface area and contributed proportionally to the rugosity of the reef, and consequently to its resilience. The positive selection effect occurred because communities with higher FRic were more likely to have such morphologically complex and competitive species (Figure 5). Note that our categorization of competitive species is independent from, but similar to, those of Darling et al. (2012) (Figure 2). The selection effect was particularly important for communities dominated by morphologically simple species (i.e., with high PC.1) because higher FRic provided a chance to avoid total collapse; this is reflected in the significant and positive interaction between FRic and PC.1 for resiliencerugosity and resiliencecover (Figures 4C,F).
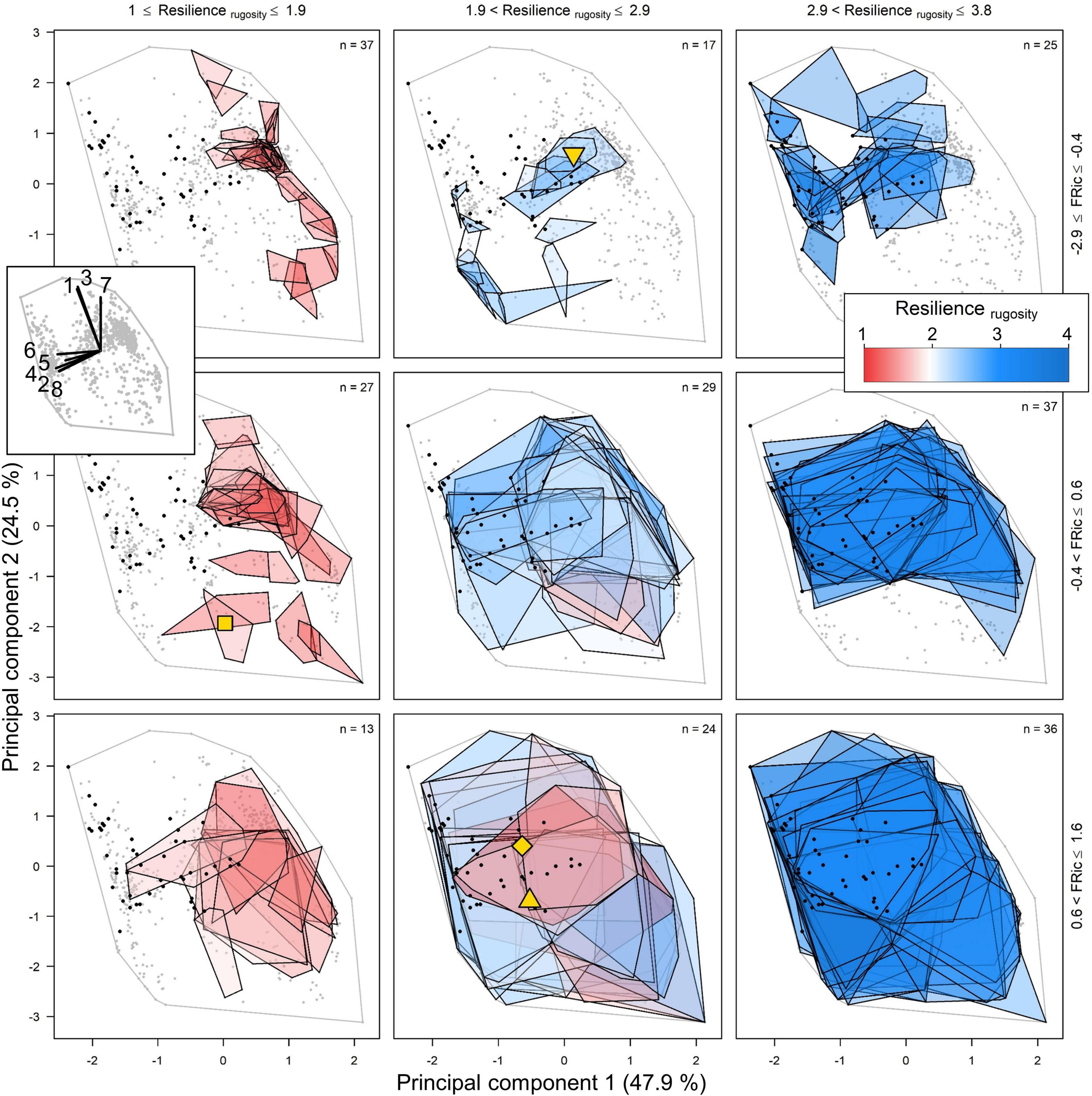
Figure 5. Representation in the functional trait space of the 245 communities and their Resilience rugosity. Trait space is represented by principal component 1 and 2, which are the first two of four PCA axes from our trait analyses (cf. section “Independent Variables”; Supplementary Figure 4A). Each polygon represents a unique community, and its area reflects its value of FRic. The red-blue color gradient reflects community resilience (rugosity). Gray and black dots show the location of all species (n = 798) and those categorized as competitive (n = 46) by Darling et al. (2012), respectively. See Figure 2 for details about the traits defining the functional trait space. The yellow symbols designate the centroids of the resilient communities presented in Figure 7.
The averaged models explained sizeable fractions of the variation in the response variables: for resiliencerugosity (GLM with gamma distribution), pseudo R2 = 0.68 and McFadden’s pseudo R2 = 0.38 ± 0.001 (Supplementary Table 6); for resistancecover (beta regression), pseudo R2 = 0.71 and McFadden’s pseudo R2 = −0.59 ± 0.002 (Supplementary Table 10); for recoverycover (linear model with exponential variance structure), pseudo R2 = 0.60 and McFadden’s pseudo R2 = 0.17 ± 0.000 (Supplementary Table 13); for resiliencecover (beta regression), pseudo R2 = 0.10 and McFadden’s pseudo R2 = −1.20 ± 0.002 (Supplementary Table 8). The low pseudo R2 of the model fitted for resiliencecover was smaller than the ones obtained with other link functions (> 0.7). This was surprising considering that the selected model had a much smaller AICc (delta AICc > 60). This was due to the high number of values close to zero and one. The Cauchit link function accommodated this distribution better compared to the other link functions, according to the AICc. However, several observations on the link scale were much more extreme with the Cauchit function than with other link functions. Consequently, the pseudo R2—which is based on the correlations of observed and fitted values on the link scale—was strongly affected. This measure of pseudo R2 was consequently not a good measure of fit in this case. Comparatively, the McFadden’s pseudo R2 < −1, which suggests that the model fitted the data much better than the null model. We obtained highly similar results by using the mean instead of the minimum resilience (Supplementary Material: Section 3.3).
Overall, communities with higher FRic were more likely composed by a majority of corals 10 years after the disturbances (Figure 6). Additionally, most of these communities were characterized by a strong dominance effect where the dynamics of coral cover was mostly influenced by one morphologically complex species that dominated the community (> 30% relative cover). The positive selection effect is shown by the fact that most of these communities had above-average FRic. The insurance effect occurred to a lesser extent and mostly in communities with high FRic (see examples of community dynamics in Figure 7).
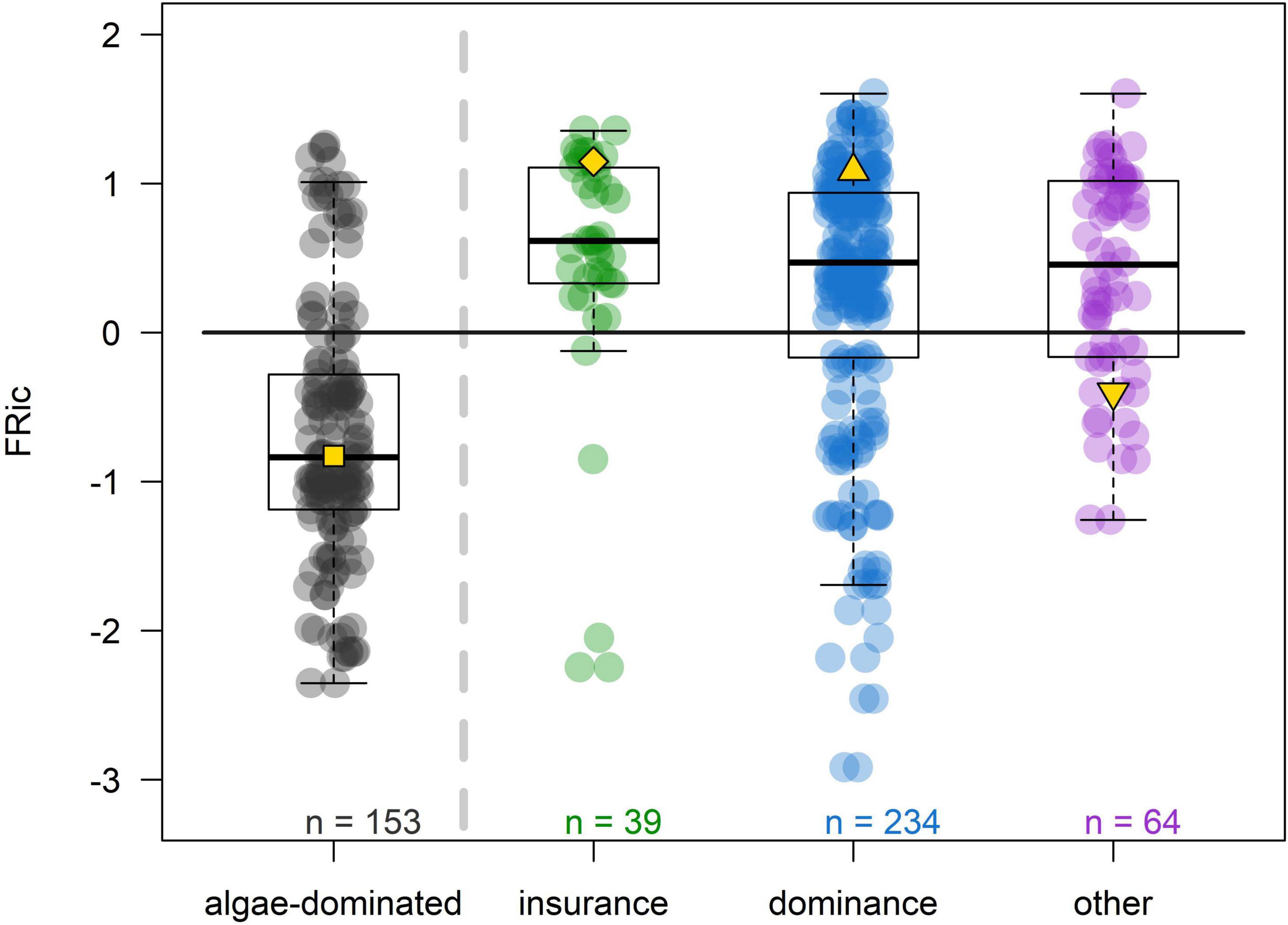
Figure 6. Relationships between FRic and coral-dominance via the insurance or strong dominance effects for both scenarios (each dot represents a community in either the cyclone or bleaching scenario; total n = 2 × 245). Algae-dominated communities (black dots) have < 50% coral cover 10 years after the disturbance. Communities showing the insurance effect (green dots) are defined by having at least two morphologically complex species (i.e., tabular, digitate, corymbose, branching, and columnar) and a switch of dominance among these species and between just before the onset of the disturbance and 3 years after. Communities showing strong dominance effect (blue dots) are characterized by a constant dominance of the same morphologically complex species (i.e., just before the disturbance, 3 and 10 years after) and a relative % cover of at least 30% 10 years after the disturbance. The horizontal line shows the initial FRic averaged across all communities and scenarios. The prevalence of the positive selection effect is shown by the higher number of communities with strong dominance effect and above-average FRic. The yellow symbols designate the communities presented in Figure 7.
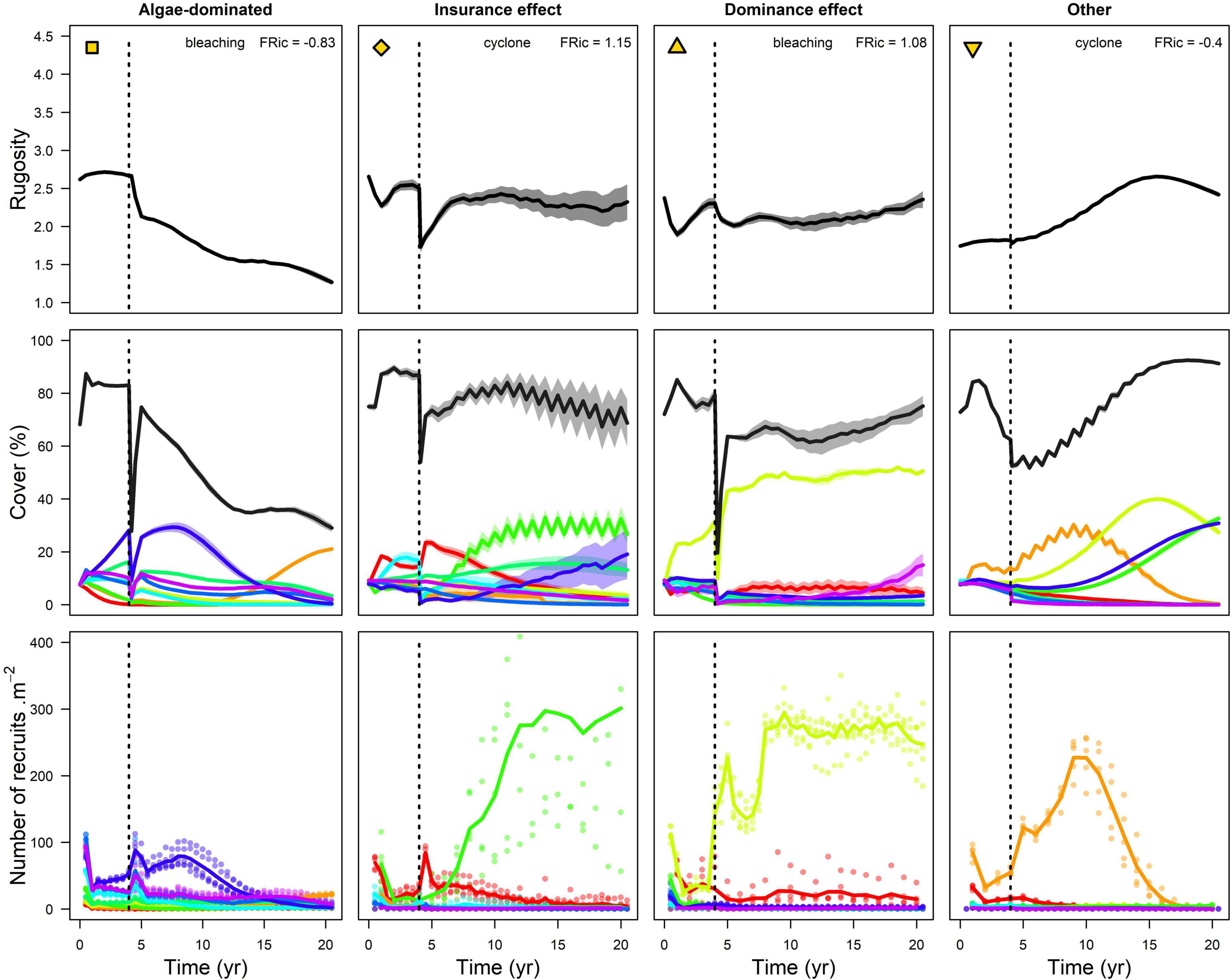
Figure 7. Examples of community dynamics for each category shown in Figure 6. Top row represents the rugosity created by the colonies; the (middle row) displays individual species’ cover in color and total coral cover in black; the (bottom row) shows the density of recruits for each species (solid lines and shaded areas represent mean ± standard error over five replicates; each dote is the number of recruits. m–2 at a given time for a given replicate). Symbols on the top left corner of the plots in the first row are used to indicate where these communities are in Figure 6 and in the variable space in Figure 5 and Supplementary Figures 12, 14, 17, 20.
Weak Negative Effect of Functional Richness on Resistance
Resistancecover depended almost exclusively on CWM of resistance traits (Figure 4), whose variation was captured by PC.1 and PC.2 for dislodgement susceptibility and bleaching susceptibility, respectively (Supplementary Figures 17A,B). Because of the trade-offs between resistance and recovery, the communities with high resistancecover had functional characteristics that differed from those in the communities with high recoverycover, i.e., slow growth rate, low colony fecundity and complexity and incapacity to overtop (hence the positive effect sizes of PC.1 and PC.2).
FRic had a negative effect on recoverycover because of a negative selection effect due to the higher susceptibility of competitive species to disturbances. The effect size was however small (and insignificant) compared to the effect sizes of PC.1 and PC.2, which could be due to the insufficient amount of time between the initialisation of the simulation and the onset of the disturbances (i.e., 4 years) for the competitive species to dominate in their communities.
Note that the trade-off between colony complexity and resistance implies that resistant communities had lower rugosity and total coral cover 10 years after the disturbances compared to communities that recovered quickly but could nevertheless maintain coral dominance (Figure 7).
Discussion
Our extensive analyses of coral trait space and constituent trade-offs, combined with our novel simulation experiment, suggest that FRic is positively associated with resilience, and are the first to clearly highlight the mechanisms at play. We discuss our findings below, then consider potential limitations of our simulation experiment, and finally suggest ways our findings can inform reef conservation and restoration efforts.
How Did Functional Diversity Influence Resilience?
We found that FRic had a positive effect on overall resilience (i.e., resiliencerugosity, resiliencecover) and on recovery (i.e., recoverycover), a weak negative effect on resistance (i.e., resistancecover) (Figure 4) and a positive effect on coral versus algae prevalence after the disturbances (Figure 6). These results are mostly due to the occurrence of a strong dominance effect of species with complex growth forms in communities with above average FRic. To a lesser extent, these results are also due to a positive association between FRic and the insurance effect (Figure 6). These effects occurred because of the rugosity-grazing feedback process and strong correlations and trade-offs among traits. The importance of the dominance effect in the diversity-resilience relationship arose from the superior competitiveness, higher susceptibility and stronger capacity to recover of the species that contributed more to the rugosity of the habitat—those with more complex growth forms—due to their overall faster growth rate and/or colony fecundity and often their capacity to overtop other organisms. When present in a community, these “competitive” species dominated the substrate and consequently drove the dynamics of reef rugosity and the associated grazing regime. The positive association between FRic and this dominance effect corresponds to a positive selection effect, i.e. the increased likelihood of communities with higher FRic to possess at least one competitive species, and arose because these species were situated in a particular location in the functional space (Figure 5).
In at least four important ways, our simulation experiment, results and interpretations align well with empirical evidence and knowledge of coral reef ecology. First, we are confident that the extensive coral trait dataset we used (Appendix 1 in Carturan et al., 2020) accurately captures key functional trade-offs among corals, as attested by the clear segregation the species that have competitive, stress-tolerant, weedy and generalist life-history strategies (Figure 2; Darling et al., 2012). It is also well documented that competitive species tend to grow faster and have higher colony fecundity, are more susceptible to colony breakage (Madin et al., 2014; Álvarez-Noriega et al., 2018) and bleaching (Loya et al., 2001; Wooldridge, 2014), and contribute more to the complexity of the habitat and to fish diversity (Darling et al., 2017).
Second, the dominance effect has the potential for strong influence coral communities. It is reasonable to assume, for example, that coral species contribute to structural complexity proportionally to their abundance (e.g., cover) (Darling et al., 2017) and, consequently, that competitive species drive most of the structural complexity dynamics when present and under favorable conditions (Graham and Nash, 2013). The dynamics we observed in most of the resilient communities (i.e., those with high resiliencerugosity, resiliencecover, and recoverycover) were characterized by strong competitive exclusion and fast recovery after the pulse disturbances (Figure 7). These dynamics are akin to dynamics observed in shallow habitats (where light is not limited) relatively protected from waves and other disturbances, and where communities are usually dominated by one or a few competitive species (Huston, 1985; Cornell and Karlson, 2000), and that show fast recovery after similar disturbances (e.g., Highsmith, 1982; Halford et al., 2004; Diaz-Pulido et al., 2009; Gilmour et al., 2013).
Third, selection effects are likely influential for coral community resilience. Logically, selection effects only happen if dominance or identity effects occur, and dominance effects are potentially strong when competitive species are present in communities (see above). It follows that selection effects are likely when conditions are favorable for competitive species. Evidence consistent with this reasoning comes from protected reefs that, despite being diverse, were generally susceptible to cyclone and bleaching owing to dominance by competitive species (Côté and Darling, 2010; Zhang et al., 2014). This aligns with the observed negative effect size of FRic on resistance (Figure 4I).
Fourth, the insurance effect has been considered a fundamental factor determining coral reef resilience (Bellwood et al., 2004; Nyström, 2006). For instance, the lack of functional redundancy among competitive coral species in the Caribbean is purportedly one important cause of their lower resilience compared to reefs in the Indo-Pacific region (Roff and Mumby, 2012; McWilliam et al., 2018b). Our study is the first to provide a detailed, mechanistic and trait-based explanation of how FRic can foster the insurance effect in coral communities. While it is trivial that FRic is positively correlated with functional redundancy, its association with response diversity depends on the strength of the correlations between effect and response traits. Specifically, response diversity would be limited if species that contributed more to rugosity responded similarly to disturbances. Our trait analysis revealed that there are substantial variations of resistance and recovery traits among species with high colony complexity (Supplementary Figure 9). Consequently, communities with higher FRic were more likely to have multiple species with higher colony complexity (i.e., functional redundancy) that likely responded differently to disturbances (i.e., response diversity). Interestingly, the magnitude of FRic’s effects relative to that of PC.1 and PC.2 is positively associated with the number of trait-related mechanisms involved in the different measures of resilience. The effect of FRic was the weakest with resistance cover, which only depends on one resistance trait in each scenario (either bleaching or dislodgement susceptibility). In comparison, FRic had a stronger effect on recoverycover, which was influenced by several processes associated to the recovery traits growth rate, colony fecundity and mode of larval development (Figure 7). Finally, FRic had the strongest effect on resiliencerugosity and resiliencecover, which accounted for both resistance and recovery processes. This could be a manifestation of the insurance effect, as FRic provided more opportunities for resilience when more responses were potentially available.
Note that these variations of response traits among morphologically complex species suggest that FRic should provide opportunities for the portfolio effect to occur. However, the effect implies that populations fluctuate (quasi) independently from each other (Schindler et al., 2015), which did not occur in our simulations because of the strong competitive spatial interaction among corals. In real reefs, the portfolio effect can be responsible for the stability of communities of mobile species, such as fish (e.g., Mellin et al., 2014). We are not aware of manifestations of this effect with corals, and it seems reasonable to assume that coral populations are rarely independent in their communities because of their strong spatial interactions.
Implication of Certain Model Assumptions
Like all models, ours includes simplifying assumptions, and these bear upon interpretations of our experimental outcomes.
Diversity effects (i.e., complementary and facilitation) did not occur in our simulations because the related processes were not implemented. A complementarity effect requires species to share several resources differently, which involves a complex combination of multiple traits (Kraft et al., 2015; Cadotte, 2017). The model assumes that the landscape is homogeneous and resources such as light, nutrients and dissolved inorganic carbon—necessary for photosynthesis—were unlimited and, consequently, species only compete for space. Like plant species, coral species have different strategies to acquire diverse resources (Darling et al., 2012), which suggests that complementarity effects are possible in real communities. However, attempts to measure these effects are lacking, which prevents us from assessing how critical this model limitation might be. In contrast, facilitation effects have been observed; for instance, coral diversity has been shown to enhance photosynthesis and growth rates and reduce corallivory and disease transmissions (Aeby et al., 2011; Kayal et al., 2011; McWilliam et al., 2018a; Clements and Hay, 2019). We predict that including complementary and facilitation processes could reduce the strength of the competitive exclusion and increase the effect size of FRic on general resilience and recovery, provided that the relevant traits are included in the analysis.
According to our definitions (Supplementary Table 1), the identity effect did not occur because coral colonies contribute to habitat complexity proportionally to their cover (Appendix 2: section 7.1.2.2 in Carturan et al., 2020). This is a reasonable assumption considering that corals contribute to the habitat provisioning functions via the dominance effect (Alvarez-Filip et al., 2011). Brandl et al. (2019) argued that identity effects are prevalent in coral reefs, but we suggest that this best applies to non-coral taxa, such as fish (e.g., Bellwood et al., 2006).
The high prevalence of the dominance and selection effects in our experiment is due in part to the lack of processes that facilitate coexistence, such as frequency-dependent predation and spatial fitness heterogeneity (Chesson, 2000b,a). Additionally, the rugosity-grazing feedback process was purposefully simplistic. In real coral reefs, the feedback process can be moderated by additional processes or features not implemented in our model, such as the contribution of other organisms (e.g., soft corals, sponges) and the reef matrix to structural complexity (Emslie et al., 2008; Halford and Caley, 2009). Other feedback processes can also slow down the recovery of communities, such as the decreased palatability of mature algae stands (van de Leemput et al., 2016). We predict that allowing for more coexistence in our model would have increased the number of communities showing the insurance effect.
Our quantification of rugosity in colonies was approximate because we used simple geometric formulas (Supplementary Material: section 2.2). In particular, in our formulation, the columnar growth form emerged as the most complex one (Supplementary Figure 2), whereas other growth forms, such as branching, should have yielded more complexity. It is notable that this reduced the strength of the correlations of colony complexity with dislodgement and bleaching susceptibility and growth rate (Figure 3).
Finally, we assembled fictional communities based on the location and distances of species in the functional space, without considering the realism of their functional structure, which could have imposed excessively strong fitness differences among species.
Significance of Our Findings for Reefs in the Anthropocene
To the extent that functional diversity indices such as FRic capture mechanistic links between functional traits, community assembly, and ecosystem functioning, they have the potential to inform conservation and restoration decisions that aim to improve the resilience of ecosystems to ongoing anthropogenic stressors (Cadotte et al., 2011). Below we consider the potential for FRic to serve this role in reef ecosystems.
On its own, FRic is not sufficient to inform restoration efforts: our experiment revealed multiple communities that were resilient despite low FRic, and communities with high FRic that were not (Figure 5). It is the combination of FRic and CWMs of the effects, resistance, recovery, and competitive traits that can confer resilience. For instance, our simulation experiment predicts that any community having small FRic and low CWM of colony complexity, growth rate or colony fecundity and overtop capacity will not be resilient in the environmental contexts of our experiment (Figure 5).
Despite revealing significant and somewhat complex interactions between FRic and CWMs, the results of our experiment nevertheless suggest strong utility for FRic in guiding restoration efforts. Specifically, our results suggest the following application of active restoration of coral reefs (Rinkevich, 2014, 2021). If a coral community simultaneously exhibits low FRic and is located far from the optimal trait space location for resilience, adding competitive species to the community could confer greater resilience. In cases where the community has high FRic, or low FRic combined with a favorable location in functional space, resilience would be supported by introducing species situated at a similar location along colony complexity but at distinct locations along the resistance and recovery traits. This would promote resilience through the insurance effect (Figure 8). Broadly, our study aligns with the suggestion to apply effect and response trait-based models to achieve functional targets for ecological restoration (Laughlin, 2014). Our model can help design experiments to (i) compare the efficacy of diverse trait targets for meeting a given restoration goal (e.g., a resilient rugosity level), and (ii) to compare the capacity of different species assemblages to reach a given trait target.
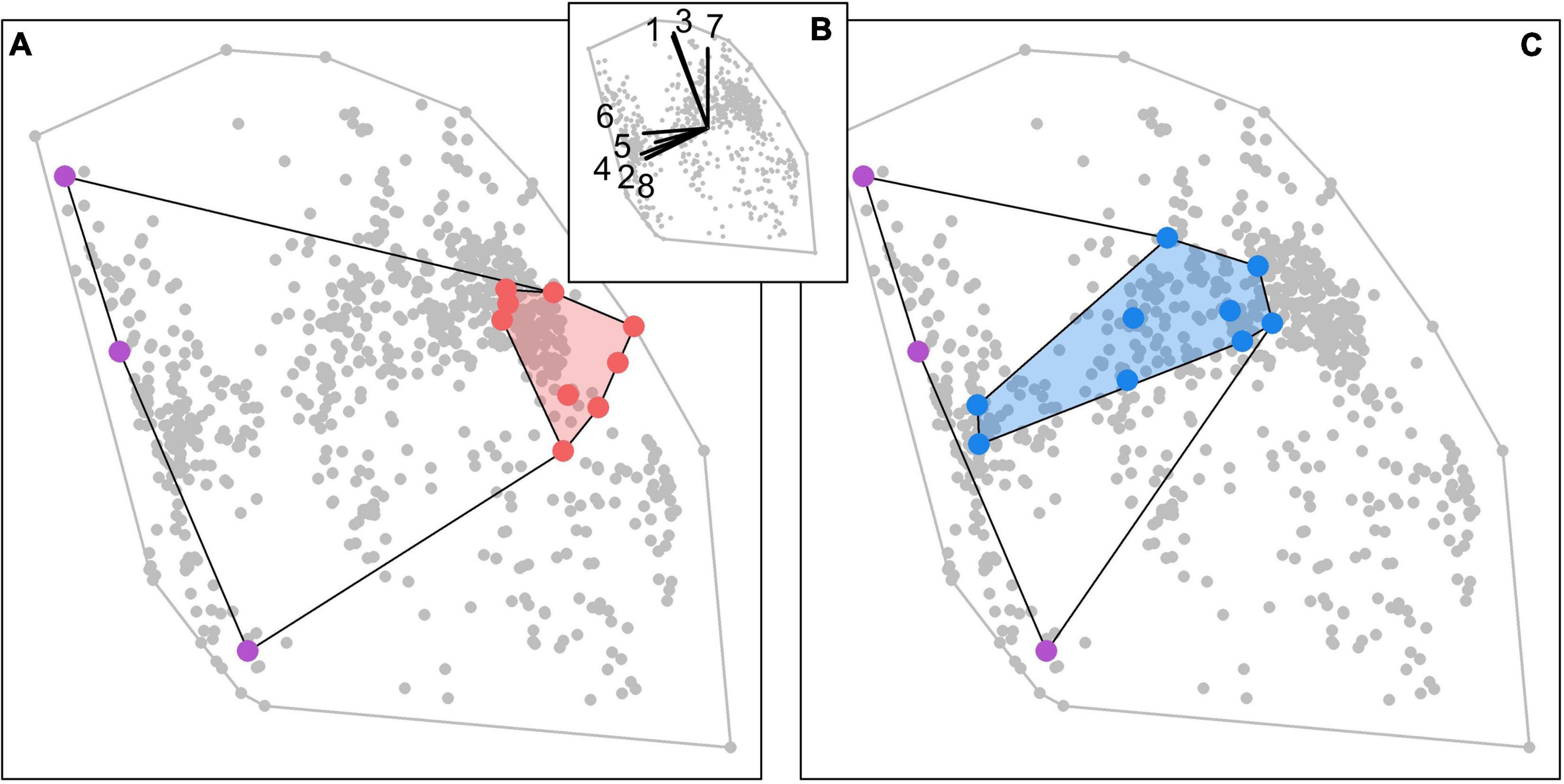
Figure 8. Illustration of resilience enhancement of two coral communities (red and blue polygons) by adding three species (purple dots) that have complex morphologies but different bleaching susceptibilities, growth rates and modes of larval development. Adding the competitive species to the non-resilient community (A) increases its overall resilience via the dominance effect, while adding them to the resilient community (C) enhances its resilience via the insurance effect. Gray dots show the location of all the 798 coral species in the functional space. The traits defining the functional space in panel (B) are: (1) bleaching susceptibility; (2) colony complexity; (3) colony maximum diameter; (4) dislodgement susceptibility; (5) colony fecundity; (6) growth rate; (7) mode of larval development; (8) overtop capacity.
The examples above illustrate how one can combine the results of our experiment with knowledge of traits and their correlations to inform active restoration. They are appropriate for reefs that experience similar environmental conditions, i.e., conditions found in well preserved and protected reefs, and before reefs were strongly affected by climate change and anthropogenic disturbances (e.g., Highsmith, 1982; Halford et al., 2004; Diaz-Pulido et al., 2009; Gilmour et al., 2013). Different strategies might need to be deployed in the majority of contemporary reefs that are affected by the increased frequency and intensity of major bleaching events (Eakin et al., 2010; Hughes et al., 2017b) and other disturbances (Tkachenko, 2015; Pendleton et al., 2016), whose cumulative effects tend to filter out competitive species from local communities (Loya et al., 2001; Van Woesik et al., 2011; McCowan et al., 2012; Hughes et al., 2018). We can reasonably predict that without competitive species the effect of FRic on resilience would have been smaller. Large stress-tolerant species (e.g., Orbicella spp.) are able to provide complex habitats as well (González-Barrios and Álvarez-Filip, 2018) but they might not be able to compete with small opportunistic weedy species (Alvarez-Filip et al., 2013). This suggests that the association of FRic with resilience must be re-evaluated in a similar experiment where competitive species are excluded or where contemporary climatic conditions are simulated.
Several other considerations may limit broader applicability of our findings. First, our experiment highlighted the importance of recovery processes for resilience and overshadowed the role of resistance processes. But in the real world, resistance processes might become the only effective resilience processes due to the increased frequency of climate-related disturbances (Darling and Côté, 2018). This suggests that efforts need to be directed more specifically toward understanding the diversity-resistance relationship. Given the importance of the habitat provisioning-herbivory feedback process for the resilience of the reefs, and the strong trade-off between colony complexity and dislodgement and bleaching susceptibility (Figure 2), assisted evolution efforts might consider focusing on increasing the resistance of coral species that provision high structural complexity to the reefs (Van Oppen et al., 2015; van Oppen et al., 2017). Second, cyclone and bleaching events are only two of the numerous and potentially interacting disturbances that affect contemporary reefs (Pendleton et al., 2016). Third, we considered only a subset of the numerous traits (Madin et al., 2016a) and associated processes involved in coral community dynamics. Further model development effort should be focussed on implementing additional relevant processes. Lastly, CWM and FRic capture only certain aspects of functional diversity, and several other single-trait (e.g., community-weighted variance, or CWV; Sonnier et al., 2010) and multiple-trait indices (e.g., FEve, FDiv, FDis) could serve to shed more light on the processes involved in the diversity-resilience relationship. For instance, CWV can help to further identifying the traits whose intra-community variations are the most influential for resilience.
Conclusion
Many coral reef benthic communities are rapidly changing toward assemblages dominated either by weedy species or by non-coral organisms. Understanding the basis of such changes, and the capacity of different assemblages to respond to disturbances is essential to successfully managing these ecosystems and the services they provide. By manipulating FRic with our ecologically detailed agent-based model and accounting for functional trade-offs between effect, resistance, recovery and competitive coral traits, we found a “sweet spot for resilience” in the functional space, characterized by species that are morphologically complex, competitive and with a good capacity to recover. These findings provide direction to on-going reef restoration efforts by revealing how the resilience of local communities could be managed by importing species that have complementary traits (Figure 8). Future experiments should strive to explore further the role of FRic and other indices on resilience in order to fully comprehend how resilience could be achieved through trait diversity and composition in coral communities.
Data Availability Statement
All data generated and associated scripts have been deposited in OSF under https://doi.org/10.17605/OSF.IO/HEUK3.
Author Contributions
BC, LP, and JP contributed to the conception and design of the study. BC was the principal investigator for the management of the computer simulations, the statistical analyses, and wrote the manuscript. JP contributed importantly to the statistical analyses. JP and LP contributed to manuscript editing and revision. All authors contributed to the article and approved the submitted version.
Funding
JP received funding from the Canada Foundation for Innovation (23065); Natural Sciences and Engineering Research Council of Canada (2014-04176). LP received funding from the Natural Sciences and Engineering Research Council of Canada (2019-05190).
Conflict of Interest
The authors declare that the research was conducted in the absence of any commercial or financial relationships that could be construed as a potential conflict of interest.
Publisher’s Note
All claims expressed in this article are solely those of the authors and do not necessarily represent those of their affiliated organizations, or those of the publisher, the editors and the reviewers. Any product that may be evaluated in this article, or claim that may be made by its manufacturer, is not guaranteed or endorsed by the publisher.
Acknowledgments
We thank Isabelle Côté for helpful feedback on an early version of the manuscript, and two reviewers for constructive comments and suggestions that substantially improved the manuscript.
Supplementary Material
The Supplementary Material for this article can be found online at: https://www.frontiersin.org/articles/10.3389/fevo.2022.780406/full#supplementary-material
References
Adam, T. C., Burkepile, D. E., Ruttenberg, B. I., and Paddack, M. J. (2015). Herbivory and the resilience of Caribbean coral reefs: knowledge gaps and implications for management. Mar. Ecol. Prog. Ser. 520, 1–20. doi: 10.3354/meps11170
Aeby, G. S., Bourne, D. G., Wilson, B., and Work, T. M. (2011). Coral diversity and the severity of disease outbreaks: a cross-regional comparison of Acropora white syndrome in a species-rich region (American Samoa) with a species-poor region (Northwestern Hawaiian Islands). J. Mar. Biol. 2011, 1–8. doi: 10.1155/2011/490198
Alvarez-Filip, L., Carricart-Ganivet, J. P., Horta-Puga, G., and Iglesias-Prieto, R. (2013). Shifts in coral-assemblage composition do not ensure persistence of reef functionality. Sci. Rep. 3:3486. doi: 10.1038/srep03486
Alvarez-Filip, L., Dulvy, N. K., Côté, I. M., Watkinson, A. R., and Gill, J. A. (2011). Coral identity underpins architectural complexity on Caribbean reefs. Ecol. Appl. 21, 2223–2231. doi: 10.1890/10-1563.1
Álvarez-Noriega, M., Baird, A. H., Dornelas, M., Madin, J. S., and Connolly, S. R. (2018). Negligible effect of competition on coral colony growth. Ecology 99, 1347–1356. doi: 10.1002/ecy.2222
Álvarez-Noriega, M., Baird, A. H., Dornelas, M., Madin, J. S., Cumbo, V. R., and Connolly, S. R. (2016). Fecundity and the demographic strategies of coral morphologies. Ecology 97, 3485–3493. doi: 10.1002/ecy.1588
Bellwood, D. R., Hughes, T. P., Folke, C., and Nyström, M. (2004). Confronting the coral reef crisis. Nature 429, 827–833. doi: 10.1038/nature02691
Bellwood, D. R., Hughes, T. P., and Hoey, A. S. (2006). Sleeping functional group drives coral-reef recovery. Curr. Biol. 16, 2434–2439. doi: 10.1016/j.cub.2006.10.030
Bozec, Y.-M., Yakob, L., Bejarano, S., and Mumby, P. J. (2013). Reciprocal facilitation and non-linearity maintain habitat engineering on coral reefs. Oikos 122, 428–440. doi: 10.1111/j.1600-0706.2012.20576.x
Brandl, S. J., Rasher, D. B., Côté, I. M., Casey, J. M., Darling, E. S., Lefcheck, J. S., et al. (2019). Coral reef ecosystem functioning: eight core processes and the role of biodiversity. Front. Ecol. Environ. 17, 445–454. doi: 10.1002/fee.2088
Burnham, K. P., and Anderson, D. R. (2002). Model selection and multimodel inference: a practical information-theoretic approach, 2nd Edn. New York: Springer-Verlag, doi: 10.1007/b97636
Cadotte, M. W. (2017). Functional traits explain ecosystem function through opposing mechanisms. Ecol. Lett. 20, 989–996. doi: 10.1111/ele.12796
Cadotte, M. W., Carscadden, K., and Mirotchnick, N. (2011). Beyond species: functional diversity and the maintenance of ecological processes and services. J. Appl. Ecol. 48, 1079–1087. doi: 10.1111/j.1365-2664.2011.02048.x
Cardinale, B. J., Duffy, J. E., Gonzalez, A., Hooper, D. U., Perrings, C., Venail, P., et al. (2012). Biodiversity loss and its impact on humanity. Nature 486, 59–67. doi: 10.1038/nature11373
Carpenter, S., Walker, B., Anderies, J. M., and Abel, N. (2001). From metaphor to measurement: resilience of what to what? Ecosystems 4, 765–781. doi: 10.1007/s10021-001-0045-9
Carturan, B. S., Parrott, L., and Pither, J. (2018). A modified trait-based framework for assessing the resilience of ecosystem services provided by coral reef communities. Ecosphere 9:24. doi: 10.1002/ecs2.2214
Carturan, B. S., Pither, J., Maréchal, J.-P., Bradshaw, C. J. A., and Parrott, L. (2020). Combining agent-based, trait-based and demographic approaches to model coral-community dynamics. Elife 9:e55993. doi: 10.7554/eLife.55993
Chesson, P. (2000a). General theory of competitive coexistence in spatially-varying environments. Theor. Popul. Biol. 58, 211–237. doi: 10.1006/tpbi.2000.1486
Chesson, P. (2000b). Mechanisms of maintenance of species diversity. Annu. Rev. Ecol. Syst. 31, 343–366. doi: 10.1146/annurev.ecolsys.31.1.343
Clements, C. S., and Hay, M. E. (2019). Biodiversity enhances coral growth, tissue survivorship and suppression of macroalgae. Nat. Ecol. Evol. 3, 178–182. doi: 10.1038/s41559-018-0752-7
Connell, J. H. (1997). Disturbance and recovery of coral assemblages. Coral Reefs 16, S101–S113. doi: 10.1007/s003380050246
Cornell, H. V., and Karlson, R. H. (2000). Coral species richness: ecological versus biogeographical influences. Coral Reefs 19, 37–49. doi: 10.1007/s003380050224
Côté, I. M., and Darling, E. S. (2010). Rethinking ecosystem resilience in the face of climate change. PLoS Biol. 8:e1000438. doi: 10.1371/journal.pbio.1000438
Cribari-Neto, F., and Zeileis, A. (2010). Beta Regression in R. J. Stat. Softw. 34, 1–24. doi: 10.18637/jss.v034.i02
Darling, E. S., Alvarez-Filip, L., Oliver, T. A., McClanahan, T. R., and Côté, I. M. (2012). Evaluating life-history strategies of reef corals from species traits. Ecol. Lett. 15, 1378–1386. doi: 10.1111/j.1461-0248.2012.01861.x
Darling, E. S., and Côté, I. M. (2018). Seeking resilience in marine ecosystems. Science 359, 986–987. doi: 10.1126/science.aas9852
Darling, E. S., Graham, N. A. J., Januchowski-Hartley, F. A., Nash, K. L., Pratchett, M. S., and Wilson, S. K. (2017). Relationships between structural complexity, coral traits, and reef fish assemblages. Coral Reefs 36, 561–575. doi: 10.1007/s00338-017-1539-z
Desjardins, E., Barker, G., Lindo, Z., Dieleman, C., and Dussault, A. C. (2015). Promoting resilience. Q. Rev. Biol. 90, 147–165. doi: 10.1086/681439
Díaz, S., Kattge, J., Cornelissen, J. H. C., Wright, I. J., Lavorel, S., Dray, S., et al. (2016). The global spectrum of plant form and function. Nature 529, 167–171. doi: 10.1038/nature16489
Diaz-Pulido, G., McCook, L. J., Dove, S., Berkelmans, R., Roff, G., Kline, D. I., et al. (2009). Doom and boom on a resilient reef: climate change, algal overgrowth and coral recovery. PLoS One 4:e5239. doi: 10.1371/journal.pone.0005239
Douma, J. C., and Weedon, J. T. (2019). Analysing continuous proportions in ecology and evolution: a practical introduction to beta and Dirichlet regression. Methods Ecol. Evol. 10, 1412–1430. doi: 10.1111/2041-210X.13234
Duong, T., Wand, M., Chacon, J., and Gramacki, A. (2021). Ks: Kernel Smoothing. R Packag. version 1.13. doi: 10.1201/9780429485572
Eakin, C. M., Morgan, J. A., Heron, S. F., Smith, T. B., Liu, G., Alvarez-Filip, L., et al. (2010). Caribbean corals in crisis: record thermal stress, bleaching, and mortality in 2005. PLoS One 5:e13969. doi: 10.1371/journal.pone.0013969
Emslie, M. J., Cheal, A. J., Sweatman, H., and Delean, S. (2008). Recovery from disturbance of coral and reef fish communities on the Great Barrier Reef, Australia. Mar. Ecol. Prog. Ser. 371, 177–190. doi: 10.3354/meps07657
Espinheira, P. L., Ferrari, S. L. P., and Cribari-Neto, F. (2008). On beta regression residuals. J. Appl. Stat. 35, 407–419. doi: 10.1080/02664760701834931
Fabricius, K. E., De’ath, G., Noonan, S., and Uthicke, S. (2014). Ecological effects of ocean acidification and habitat complexity on reef-associated macroinvertebrate communities. Proc. R. Soc. B Biol. Sci. 281:8. doi: 10.1098/rspb.2013.2479
Ferrari, S., and Cribari-Neto, F. (2004). Beta regression for modelling rates and proportions. J. Appl. Stat. 31, 799–815. doi: 10.1080/0266476042000214501
Fox, J., and Weisberg, S. (2019). An R companion to applied regression, 3rd Edn. Thousand Oaks CA: Sage.
Gilmour, J. P., Smith, L. D., Heyward, A. J., Baird, A. H., and Pratchett, M. S. (2013). Recovery of an isolated coral reef system following severe disturbance. Science 340, 69–71. doi: 10.1126/science.1232310
Glass, G. V. (1965). A ranking variable analogue of biserial correlation: implications for short-cut item analysis. J. Educ. Meas. Educ. 2, 91–95. doi: 10.1111/j.1745-3984.1965.tb00396.x
González-Barrios, F. J., and Álvarez-Filip, L. (2018). A framework for measuring coral species-specific contribution to reef functioning in the Caribbean. Ecol. Indic. 95, 877–886. doi: 10.1016/j.ecolind.2018.08.038
Graham, N. A. J., Cinner, J. E., Norström, A. V., and Nyström, M. (2014). Coral reefs as novel ecosystems: embracing new futures. Curr. Opin. Environ. Sustain. 7, 9–14. doi: 10.1016/j.cosust.2013.11.023
Graham, N. A. J., and Nash, K. L. (2013). The importance of structural complexity in coral reef ecosystems. Coral Reefs 32, 315–326. doi: 10.1007/s00338-012-0984-y
Griffin, J. N., O’Gorman, E. J., Emmerson, M. C., Jenkins, S. R., Klein, A. M., Loreau, M., et al. (2009). “Biodiversity and the stability of ecosystem functioning,” in Biodiversity, Ecosystem Functioning, and Human Wellbeing: An Ecological and Economic Perspective, eds S. Naeem, D. E. Bunker, A. Hector, M. Loreau, and C. Perrings (Oxford University Press: New York), 78–93. doi: 10.1093/acprof:oso/9780199547951.003.0006
Grime, J. P. (1977). Evidence for the existence of three primary strategies in plants and its relevance to ecological and evolutionary theory. Am. Nat. 111, 1169–1194. doi: 10.1086/283244
Grime, J. P. (1998). Benefits of plant diversity to ecosystems: immediate, filter and founder effects. J. Ecol. 86, 902–910. doi: 10.1046/j.1365-2745.1998.00306.x
Grün, B., Kosmidis, I., and Zeileis, A. (2012). Extended beta regression in R: shaken, stirred, mixed, and partitioned. J. Stat. Softw. 48, 128–129. doi: 10.18637/jss.v048.i11
Habel, K., Grasman, R., Gramacy, R. B., Mozharovskyi, P., and Sterratt, D. C. (2019). geometry: Mesh Generation and Surface Tessellation. R Packag. version 0.4.5.
Halford, A., Cheal, A. J., Ryan, D., and Williams, D. M. (2004). Resilience to large-scale disturbance in coral and fish assemblages on the Great Barrier Reef. Ecology 85, 1892–1905. doi: 10.1890/03-4017
Halford, A. R., and Caley, M. J. (2009). Towards an understanding of resilience in isolated coral reefs. Glob. Chang. Biol. 15, 3031–3045. doi: 10.1111/j.1365-2486.2009.01972.x
Heenan, A., Hoey, A. S., Williams, G. J., and Williams, I. D. (2016). Natural bounds on herbivorous coral reef fishes. Proc. R. Soc. B Biol. Sci. 283:20161716. doi: 10.1098/rspb.2016.1716
Highsmith, R. C. (1982). Reproduction by fragmentation in corals. Mar. Ecol. Prog. Ser. 7, 207–226. doi: 10.3354/meps007207
Hodgson, D., McDonald, J. L., and Hosken, D. J. (2015). What do you mean, ‘resilient’? Trends Ecol. Evol. 30, 503–506. doi: 10.1016/j.tree.2015.06.010
Holbrook, S. J., Brooks, A. J., and Schmitt, R. J. (2002). Variation in structural attributes of patch-forming corals and in patterns of abundance of associated fishes. Mar. Freshw. Res. 53, 1045–1053. doi: 10.1071/MF02063
Hooper, D. U., Adair, E. C., Cardinale, B. J., Byrnes, J. E. K., Hungate, B. A., Matulich, K. L., et al. (2012). A global synthesis reveals biodiversity loss as a major driver of ecosystem change. Nature 486, 105–108. doi: 10.1038/nature11118
Hughes, T. P., Barnes, M. L., Bellwood, D. R., Cinner, J. E., Cumming, G. S., Jackson, J. B. C., et al. (2017a). Coral reefs in the Anthropocene. Nature 546, 82–90. doi: 10.1038/nature22901
Hughes, T. P., Kerry, J. T., Álvarez-Noriega, M., Álvarez-Romero, J. G., Anderson, K. D., Baird, A. H., et al. (2017b). Global warming and recurrent mass bleaching of corals. Nature 543, 373–377. doi: 10.1038/nature21707
Hughes, T. P., Bellwood, D. R., and Connolly, S. R. (2002). Biodiversity hotspots, centres of endemicity, and the conservation of coral reefs. Ecol. Lett. 5, 775–784. doi: 10.1046/j.1461-0248.2002.00383.x
Hughes, T. P., Graham, N. A. J., Jackson, J. B. C., Mumby, P. J., and Steneck, R. S. (2010). Rising to the challenge of sustaining coral reef resilience. Trends Ecol. Evol. 25, 633–642. doi: 10.1016/j.tree.2010.07.011
Hughes, T. P., Kerry, J. T., Baird, A. H., Connolly, S. R., Dietzel, A., Eakin, C. M., et al. (2018). Global warming transforms coral reef assemblages. Nature 556, 492–496. doi: 10.1038/s41586-018-0041-2
Huston, M. A. (1985). Patterns of species diversity on coral reefs. Annu. Rev. Ecol. Syst. 16, 149–177. doi: 10.1146/annurev.es.16.110185.001053
Kayal, M., Lenihan, H. S., Pau, C., Penin, L., and Adjeroud, M. (2011). Associational refuges among corals mediate impacts of a crown-of-thorns starfish Acanthaster planci outbreak. Coral Reefs 30, 827–837. doi: 10.1007/s00338-011-0763-1
Kraft, N. J. B., Godoy, O., and Levine, J. M. (2015). Plant functional traits and the multidimensional nature of species coexistence. Proc. Natl. Acad. Sci. U. S. A. 112, 797–802. doi: 10.1073/pnas.1413650112
Laliberté, E., and Legendre, P. (2010). A distance-based framework for measuring functional diversity from multiple traits. Ecology 91, 299–305. doi: 10.1890/08-2244.1
Laliberté, E., Wells, J. A., Declerck, F., Metcalfe, D. J., Catterall, C. P., Queiroz, C., et al. (2010). Land-use intensification reduces functional redundancy and response diversity in plant communities. Ecol. Lett. 13, 76–86. doi: 10.1111/j.1461-0248.2009.01403.x
Laughlin, D. C. (2014). Applying trait-based models to achieve functional targets for theory-driven ecological restoration. Ecol. Lett. 17, 771–784. doi: 10.1111/ele.12288
Longo, G., Seidler, T. G., Garibaldi, L. A., Tognetti, P. M., and Chaneton, E. J. (2013). Functional group dominance and identity effects influence the magnitude of grassland invasion. J. Ecol. 101, 1114–1124. doi: 10.1111/1365-2745.12128
Loreau, M. (2000). Biodiversity and ecosystem functioning: recent theoretical advances. Okios 91, 3–17. doi: 10.1034/j.1600-0706.2000.910101.x
Loreau, M., and de Mazancourt, C. (2013). Biodiversity and ecosystem stability: a synthesis of underlying mechanisms. Ecol. Lett. 16, 106–115. doi: 10.1111/ele.12073
Loya, Y., Sakai, K., Yamazato, K., Nakano, Y., Sambali, H., and van Woesik, R. (2001). Coral bleaching: the winners and the losers. Ecol. Lett. 4, 122–131. doi: 10.1046/j.1461-0248.2001.00203.x
Macarthur, R. (1955). Fluctuations of animal populations and a measure of community stability. Ecology 36, 533–536. doi: 10.2307/1929601
Madin, J. S., Hoogenboom, M. O., Connolly, S. R., Darling, E. S., Falster, D. S., Huang, D., et al. (2016b). A trait-based approach to advance coral reef science. Trends Ecol. Evol. 31, 419–428. doi: 10.1016/j.tree.2016.02.012
Madin, J. S., Anderson, K. D., Andreasen, M. H., Bridge, T. C. L., Cairns, S. D., Connolly, S. R., et al. (2016a). The Coral Trait Database, a curated database of trait information for coral species from the global oceans. Sci. Data 3:160017. doi: 10.1038/sdata.2016.17
Madin, J. S., Baird, A. H., Dornelas, M., and Connolly, S. R. (2014). Mechanical vulnerability explains size-dependent mortality of reef corals. Ecol. Lett. 17, 1008–1015. doi: 10.1111/ele.12306
Madin, J. S., and Connolly, S. R. (2006). Ecological consequences of major hydrodynamic disturbances on coral reefs. Nature 444, 477–480. doi: 10.1038/nature05328
Mangiafico, S. (2020). rcompanion: functions to support extension education program evaluation. Packag. version 2.3.25. AvailableOnline at: http://rcompanion.org (accessed September 24, 2020).
Mason, N. W. H., Mouillot, D., Lee, W. G., and Wilson, J. B. (2005). Functional richness, functional evenness and functional divergence: the primary components of functional diversity. Okios 111, 112–118. doi: 10.1111/j.0030-1299.2005.13886.x
May, R. M. (1972). Will a large complex system be stable? Nature 238, 413–414. doi: 10.1038/238413a0
McClanahan, T. R., Donner, S. D., Maynard, J. A., MacNeil, M. A., Graham, N. A. J., Maina, J., et al. (2012). Prioritizing key resilience indicators to support coral reef management in a changing climate. PLoS One 7:e42884. doi: 10.1371/journal.pone.0042884
McCowan, D., Pratchett, M., and Baird, A. H. (2012). “Bleaching susceptibility and mortality among corals with differing growth forms,” in Proceedings of the 12th International Coral Reef Symposium, (Cairns, QLD, Australia: James Cook University).
McFadden, D. (1977). Quantitative methods for analyzing travel behaviour of individuals: some recent developments. Cowles Foundation Discussion Papers 474, Cowles Foundation for Research in Economics. Connecticut: Yale University.
McLaren, J. R., and Turkington, R. (2010). Ecosystem properties determined by plant functional group identity. J. Ecol. 98, 459–469. doi: 10.1111/j.1365-2745.2009.01630.x
McWilliam, M., Chase, T. J., and Hoogenboom, M. O. (2018a). Neighbor diversity regulates the productivity of coral assemblages. Curr. Biol. 28, 3634–3639.e3. doi: 10.1016/j.cub.2018.09.025
McWilliam, M., Hoogenboom, M. O., Baird, A. H., Kuo, C., Madin, J. S., and Hughes, T. P. (2018b). Biogeographical disparity in the functional diversity and redundancy of corals. Proc. Natl. Acad. Sci. U. S. A. 115, 3084–3089. doi: 10.1073/pnas.1716643115
Mellin, C., Bradshaw, C. J. A., Fordham, D. A., and Caley, M. J. (2014). Strong but opposing β-diversity – stability relationships in coral reef fish communities. Proc. R. Soc. B 281:20131993. doi: 10.1098/rspb.2013.1993
Mori, A. S., Furukawa, T., and Sasaki, T. (2013). Response diversity determines the resilience of ecosystems to environmental change. Biol. Rev. 88, 349–364. doi: 10.1111/brv.12004
Mouchet, M. A., Villéger, S., Mason, N. W. H., and Mouillot, D. (2010). Functional diversity measures: an overview of their redundancy and their ability to discriminate community assembly rules. Funct. Ecol. 24, 867–876. doi: 10.1111/j.1365-2435.2010.01695.x
Mouillot, D., Graham, N. A. J., Villéger, S., Mason, N. W. H., and Bellwood, D. R. (2013). A functional approach reveals community responses to disturbances. Trends Ecol. Evol. 28, 167–177. doi: 10.1016/j.tree.2012.10.004
Mumby, P. J., and Steneck, R. S. (2008). Coral reef management and conservation in light of rapidly evolving ecological paradigms. Trends Ecol. Evol. 23, 555–563. doi: 10.1016/j.tree.2008.06.011
Nelson, H. R., Kuempel, C. D., and Altieri, A. H. (2016). The resilience of reef invertebrate biodiversity to coral mortality. Ecosphere 7, 1–14. doi: 10.1002/ecs2.1399
North, M. J., Collier, N. T., Ozik, J., Tatara, E. R., Macal, C. M., Bragen, M., et al. (2013). Complex adaptive systems modeling with Repast Simphony. Complex Adapt. Syst. Model. 1:3. doi: 10.1186/2194-3206-1-3
Nyström, M. (2006). Redundancy and response diversity of functional groups: implications for the resilience of coral reefs. Ambio 35, 30–35. doi: 10.1579/0044-7447-35.1.30
Nyström, M., Graham, N. A. J., Lokrantz, J., and Norström, A. V. (2008). Capturing the cornerstones of coral reef resilience: linking theory to practice. Coral Reefs 27, 795–809. doi: 10.1007/s00338-008-0426-z
Oliver, T. H., Heard, M. S., Isaac, N. J. B., Roy, D. B., Procter, D., Eigenbrod, F., et al. (2015). Biodiversity and resilience of ecosystem functions. Trends Ecol. Evol. 30, 673–684. doi: 10.1016/j.tree.2015.08.009
Pendleton, L. H., Hoegh-Guldberg, O., Langdon, C., and Comte, A. (2016). Multiple stressors and ecological complexity require a new approach to coral reef research. Front. Mar. Sci. 3:36. doi: 10.3389/fmars.2016.00036
Perry, C. T., and Alvarez-Filip, L. (2019). Changing geo-ecological functions of coral reefs in the Anthropocene. Funct. Ecol. 33, 976–988. doi: 10.1111/1365-2435.13247
Pillar, V. D., Blanco, C. C., Müller, S. C., Sosinski, E. E., Joner, F., and Duarte, L. D. S. (2013). Functional redundancy and stability in plant communities. J. Veg. Sci. 24, 963–974. doi: 10.1111/jvs.12047
Pimm, S. L. (1984). The complexity and stability of ecosystems. Nature 307, 321–326. doi: 10.1038/307321a0
Plass-Johnson, J. G., Taylor, M. H., Husain, A. A. A., Teichberg, M. C., and Ferse, S. C. A. (2016). Non-random variability in functional composition of coral reef fish communities along an environmental gradient. PLoS One 11:e0154014. doi: 10.1371/journal.pone.0154014
Precoda, K., Allen, A. P., Grant, L., and Madin, J. S. (2017). Using traits to assess nontransitivity of interactions among coral species. Am. Nat. 190, 420–429. doi: 10.1086/692758
Prestes García, A.Rodríguez-Patón, A. (2016). Sensitivity analysis of Repast computational ecology models with R/Repast. Ecol. Evol. 6, 8811–8831. doi: 10.1002/ece3.2580
R Core Team (2017). R: a language and environment for statistical computing. Vienna, Austria: R Foundation for Statistical Computing.
R Core Team (2019). R: a language and environment for statistical computing. Vienna, Austria: R Foundation for Statistical Computing.
Rasher, D. B., and Hay, M. E. (2010). Chemically rich seaweeds poison corals when not controlled by herbivores. Proc. Natl. Acad. Sci. U. S. A. 107, 9683–9688. doi: 10.1073/pnas.0912095107
Rinkevich, B. (2014). Rebuilding coral reefs: does active reef restoration lead to sustainable reefs? Curr. Opin. Environ. Sustain. 7, 28–36. doi: 10.1016/j.cosust.2013.11.018
Rinkevich, B. (2021). Ecological engineering approaches in coral reef restoration. ICES J. Mar. Sci. 78, 410–420. doi: 10.1093/icesjms/fsaa022
Roff, G., and Mumby, P. J. (2012). Global disparity in the resilience of coral reefs. Trends Ecol. Evol. 27, 404–413. doi: 10.1016/j.tree.2012.04.007
Schindler, D. E., Armstrong, J. B., and Reed, T. E. (2015). The portfolio concept in ecology and evolution. Front. Ecol. Environ. 13, 257–263. doi: 10.1890/140275
Sonnier, G., Shipley, B., and Navas, M. L. (2010). Quantifying relationships between traits and explicitly measured gradients of stress and disturbance in early successional plant communities. J. Veg. Sci. 21, 1014–1024. doi: 10.1111/j.1654-1103.2010.01210.x
Suding, K. N., Lavorel, S., Chapin, F. S., Cornelissen, J. H. C., Díaz, S., Garnier, E., et al. (2008). Scaling environmental change through the community-level: a trait-based response-and-effect framework for plants. Glob. Chang. Biol. 14, 1125–1140. doi: 10.1111/j.1365-2486.2008.01557.x
Tilman, D., Isbell, F., and Cowles, J. M. (2014). Biodiversity and ecosystem functioning. Annu. Rev. Ecol. Evol. Syst. 45, 471–493. doi: 10.1146/annurev-ecolsys-120213-091917
Tkachenko, K. S. (2015). Coral reefs in the face of ecological threats of 21st century. Biol. Bull. Rev. 7, 390–414. doi: 10.1134/S2079086416050091
Urbanek, S. (2018). rJava: low-level R to Java interface. R Packag. version 0.9-10. Available Online at: https://cran.r-project.org/package=rJava (accessed July 15, 2018).
van de Leemput, I. A., Hughes, T. P., van Nes, E. H., and Scheffer, M. (2016). Multiple feedbacks and the prevalence of alternate stable states in coral reefs. Coral Reefs 35, 857–865. doi: 10.1007/s00338-016-1439-7
van der Plas, F. (2019). Biodiversity and ecosystem functioning in naturally assembled communities. Biol. Rev. 94, 1220–1245. doi: 10.1111/brv.12499
van Oppen, M. J. H., Gates, R. D., Blackall, L. L., Cantin, N., Chakravarti, L. J., Chan, W. Y., et al. (2017). Shifting paradigms in restoration of the world’s coral reefs. Glob. Chang. Biol. 23, 1–12. doi: 10.1111/gcb.13647
Van Oppen, M. J. H., Oliver, J. K., Putnam, H. M., and Gates, R. D. (2015). Building coral reef resilience through assisted evolution. Proc. Natl. Acad. Sci. U. S. A. 112, 1–7. doi: 10.1073/pnas.1422301112
Van Woesik, R., Sakai, K., Ganase, A., and Loya, Y. (2011). Revisiting the winners and the losers a decade after coral bleaching. Mar. Ecol. Prog. Ser. 434, 67–76. doi: 10.3354/meps09203
Vergés, A., Vanderklift, M. A., Doropoulos, C., and Hyndes, G. A. (2011). Spatial patterns in herbivory on a coral reef are influenced by structural complexity but not by algal traits. PLoS One 6:e17115. doi: 10.1371/journal.pone.0017115
Villéger, S., Mason, N. W. H., and Mouillot, D. (2008). New multidimensional functional diversity indices for a multifaceted framework in functional ecology. Ecology 89, 2290–2301. doi: 10.1890/07-1206.1
Violle, C., Navas, M. L., Vile, D., Kazakou, E., Fortunel, C., Hummel, I., et al. (2007). Let the concept of trait be functional! Oikos 116, 882–892. doi: 10.1111/j.2007.0030-1299.15559.x
Walker, B., Holling, C. S., Carpenter, S. R., and Kinzig, A. (2004). Resilience, adaptability and transformability in social – ecological systems. Ecol. Soc. 9:5.
Williams, G. J., and Graham, N. A. J. (2019). Rethinking coral reef functional futures. Funct. Ecol. 33, 942–947. doi: 10.1111/1365-2435.13374
Wooldridge, S. A. (2014). Differential thermal bleaching susceptibilities amongst coral taxa: re-posing the role of the host. Coral Reefs 33, 15–27. doi: 10.1007/s00338-013-1111-4
Zhang, S. Y., Speare, K. E., Long, Z. T., McKeever, K. A., Gyoerkoe, M., Ramus, A. P., et al. (2014). Is coral richness related to community resistance to and recovery from disturbance? PeerJ 2:e308. doi: 10.7717/peerj.308
Zuur, A. F., Leno, E. N., Walker, N. J., Saveliev, A. A., Smith, G. M. (2009a). “Ch. 4. Dealing with heterogeneity,” in Mixed Effects Models and Extensions in Ecology with R, eds Gail, M., Krickeberg, K., Samet, J. M., Tsiatis, A., and Wong, W. (New York, NY: Springer-Verlag). doi: 10.1016/B978-0-12-387667-6.00013-0
Zuur, A. F., Leno, E. N., Walker, N. J., Saveliev, A. A., Smith, G. M. (2009b). “Ch. 9. GLM and GAM for count data,” in Mixed Effects Models and Extensions in Ecology with R, eds Gail, M., Krickeberg, K., Samet, J. M., Tsiatis, A., and Wong, W. (New York, NY: Springer-Verlag). doi: 10.1016/B978-0-12-387667-6.00013-0
Keywords: FRic, CWM, resilience, function, coral, trait framework, ABM
Citation: Carturan BS, Parrott L and Pither J (2022) Functional Richness and Resilience in Coral Reef Communities. Front. Ecol. Evol. 10:780406. doi: 10.3389/fevo.2022.780406
Received: 21 September 2021; Accepted: 04 May 2022;
Published: 02 June 2022.
Edited by:
Bradley S. Case, Auckland University of Technology, New ZealandCopyright © 2022 Carturan, Parrott and Pither. This is an open-access article distributed under the terms of the Creative Commons Attribution License (CC BY). The use, distribution or reproduction in other forums is permitted, provided the original author(s) and the copyright owner(s) are credited and that the original publication in this journal is cited, in accordance with accepted academic practice. No use, distribution or reproduction is permitted which does not comply with these terms.
*Correspondence: Bruno S. Carturan, bruno.carturan@alumni.ubc.ca