- 1SINTEF Ocean, Trondheim, Norway
- 2The Norwegian College of Fishery Science, UiT The Arctic University of Norway, Tromsø, Norway
- 3DTU Aqua, Technical University of Denmark, Hirtshals, Denmark
- 4Department of Marine Studies, University of Split, Split, Croatia
- 5Centre for Fisheries Ecosystems Research, Fisheries and Marine Institute of Memorial University of Newfoundland, St. John’s, NL, Canada
- 6Department of Arctic Biology, University Centre in Svalbard, Longyearbyen, Norway
- 7Centre for Autonomous Marine Operations and Systems, Department of Biology, Norwegian University of Science and Technology, Trondheim, Norway
Measures of biological diversity (biodiversity) are important for monitoring the state of ecosystems. Several indices and methods are used to describe biodiversity from field observations. Marine faunal biodiversity is often quantified based on analysis of samples collected using a trawl during research surveys. To monitor spatial and temporal changes in biodiversity between surveys, samples are generally collected from a series of stations. Inference regarding changes in biodiversity must account for uncertainties in the estimation of the values for the different biodiversity indices used. Estimation for a single station is affected by spatial-temporal variation in the species composition in the area and by uncertainty due to the finite sample size taken by the trawl. Therefore, variation between stations needs to be accounted for when estimating uncertainty for values of different indices during a survey as total or as mean for the survey. Herein, we present a method based on nested bootstrapping that accounts for uncertainties in the estimation of various indices and which can be used to infer changes in biodiversity. Application of this methodology is demonstrated using data collected in the mesopelagic sound scattering layer in the high Arctic.
Introduction
Loss of biological diversity (biodiversity) negatively affects ecosystem functioning and may ultimately lead to ecosystem collapse (Bland et al., 2018). Several examples in the literature describe and use different indices to quantify species biodiversity in marine ecosystems (Whittaker, 1972; Chao, 2005; Gamfeldt et al., 2014). These indices also allow to detect changes due to, for example, climate change, ocean warming, acidification, loss of habitat and to assess the impact that fisheries have on marine ecosystems (Kaiser et al., 2011).
As biodiversity is a multidimensional concept that includes estimates of both species’ richness and evenness, a single measure of biodiversity is often not sufficient (Daly et al., 2018) and several biodiversity indices are often used. However, using variation in biodiversity indices to infer changes in an ecosystem raises the following questions: (i) How certain are we that changes in biodiversity indices reflect changes in community biodiversity? (ii) Could differences observed in the biodiversity indices be coincidental? (iii) How reliable are biodiversity indices estimated from experimental sampling? To answer these questions, realistic uncertainty limits around biodiversity indices are needed. Furthermore, to be able to monitor spatial or temporal changes in biodiversity, the uncertainties of differences in index values need to be quantified. Survey trawls are one of the methods used to sample data on species biodiversity (Geoffroy et al., 2019). However, the estimation of biodiversity indices based on a single survey trawl haul is affected by spatial-temporal variation in species composition within the area and by uncertainty related to the finite sample size taken by the sampling trawl. Therefore, for a series of sampling hauls (stations) during a survey, the variation between hauls needs to be accounted for when estimating uncertainty of the value for the different indices. This can be done for the survey area as total or as mean for the stations sampled within.
In this study, we present a method based on a nested bootstrapping technique to account for uncertainties when estimating various biodiversity indices. This type of bootstrapping-based method has previously been used to estimate uncertainties (within- and between-station variability) in relation to fishing gear size selectivity studies (Millar, 1993; Herrmann et al., 2017; Larsen et al., 2017, 2018). Our purpose is to adopt this kind of method for assessing uncertainty in biodiversity studies which are subjected to multilevel sources of uncertainties. Further, this novel approach can be used to infer changes in biodiversity. Specifically, we applied this approach to survey trawl data collected in the Arctic ecoregion as a case study of mesopelagic biodiversity with the purpose to investigate and demonstrate the advantages of this new method.
Mesopelagic macrozooplankton and small pelagic fish occupy the zone extending from 200 to 1000 m below the surface of the ocean. These organisms form a sound scattering layer (SSL), which appears on acoustic echograms as echoes that are higher than background noise in the water column (Proud et al., 2015). SSLs were firstly observed and described in the late 1940s (Dietz, 1948) and further explored during the 1960s (Barham, 1966). Mesopelagic SSLs are ubiquitous in the world’s oceans (Irigoien et al., 2014; Proud et al., 2018). However, several knowledge gaps persist regarding the role of these species in the ecosystem and their contribution to biodiversity (St. John et al., 2016; Hidalgo and Browman, 2019).
Materials and Methods
Survey Data for Estimation of Biodiversity in the Sound Scattering Layer of the High Arctic
The data used in this study were collected by Geoffroy et al. (2019) onboard the research vessel “Helmer Hanssen” (63.8 m, 4080 HP) in the northern Barents Sea, north of Svalbard, during three trials conducted from 14 to 16 January 2016 (cruise 1), 28 to 29 August 2016 (cruise 2), and 11 to 14 January 2017 (cruise 3). Bottom depth ranged from < 50 m close to shore to ∼2000 m at the end of the transects. The SSLs were located between 200 and 700 m in August 2016 and from 50 to 500 m in January 2016 and 2017 (Geoffroy et al., 2019). The samples were collected using a Harstad pelagic trawl within the SSL to ground truth the acoustic signal. The mesh size of the inner liner of the trawl’s codend was 10 mm. The Harstad trawl had an opening of 18.28 × 18.28 m with an actual height of 9–11 m and width of 10–12 m at the towing speed of 3 knots (Geoffroy et al., 2019). The towing time was kept between 20 and 80 min (Supplementary Material 1). The catch of the codend was sorted on board, and all organisms were identified to the lowest possible level (species or genus). However, due to time constraints, number of individuals of highly abundant species was estimated from the number in a subsample and then raised to the total number from weight of the subsample to the total weight (Table 1).
Therefore, in this study, we used a dataset consisting of 44 marine faunal species from the Arctic mesopelagic SSL belonging to 9 classes, 21 orders, and 32 families to estimate biodiversity indices. The maximum number of species (n = 33) was recorded during cruise 3. Due to the selectivity of the 10 mm mesh size codend of the Harstad trawl, the samples of the mesopelagic SSL might exclude some of the smaller organisms, such as small euphausiids, thus the dataset does not include the whole community of the mesopelagic SSL.
Description of Biodiversity Indices
Several indices are usually applied when assessing biodiversity (Daly et al., 2018), as restricting the analysis to a single biodiversity index can be uninformative and lead to biased conclusions. The diversity indices estimated in this study were richness (Daly et al., 2018), Shannon (1948); Simpson (1949), Margalef (1958); Menhinick (1964), and Pielou (1966) indices. The value for each of those indices was estimated for each trawl station. For trawl station j, the processed sample consisted of count numbers nij for each species Si, where i is the species index (species ID) that was predefined. We describe below how the value for each of the biodiversity indices was estimated for a single trawl station j from count numbers n1j … nij … nQj, where there are Q species in the list.
Indices of Species Richness
The richness biodiversity index accounts for the absolute number of species within the sample (Daly et al., 2018). This index does not account for species abundance distribution, thus less abundant species and highly abundant ones have equal weight. The richness was estimated as follows:
Margalef (Eq. 2) and Menhinick (Eq. 3) indices also measure species richness but account for a relationship between the number of species and the number of individuals:
Index of Entropy
The Shannon index is a measure of the entropy or disorder of the community of the sample. The Shannon index is sensitive to species richness because it depends on number of species that are sampled (Daly et al., 2018). The value of the Shannon index is zero when there is only one species in a sample, and it increases with each added species. It generally varies between 1.3 and 3.5, but in some cases it can exceed 4.0 (Kanieski et al., 2018). Thus, the lower the value of the Shannon index, the lower the diversity. The Shannon index was estimated by:
Index of Species Evenness
Pielou’s evenness index measures how evenly the number of individuals are distributed among the species. Species evenness in the biodiversity context is the evenness of a community’s species abundance distribution. A community is perfectly even if every species is present in equal proportions and uneven if one species dominates the abundance distribution. The Shannon index is the basis for calculating the Pielou’s index, which was estimated using the following equation:
Pielou’s evenness is the most widely used evenness index in the ecological literature (Daly et al., 2018). The value of Pielou’s evenness index ranges from 0 to 1, with a value of 1 indicating that all species are equally abundant (Kanieski et al., 2018).
Index of Species Dominance
Indices of dominance express whether one or more species are more abundant than others in the sampled area. Therefore, these indices account for the dominance of some species within the sampled community and do not qualify as indicators of diversity or richness (Kanieski et al., 2018). The Simpson index represents the probability that two individuals randomly taken from the population represent the same species. High values of the Simpson index (range: 0–1) indicate higher dominance of one or a few species, thus implying lower species diversity. The index can be estimated by:
Dominance Pattern
Apart from reducing the biodiversity data to one summary statistic such as a biodiversity index, dominance curves are often used to quantify information about the pattern of relative species abundances for a given sampling station and can be estimated as follows (Warwick et al., 2008):
Cumulative dominance curves are often used to represent species dominance patterns:
where I is the species ID summed up to in the nominator.
Generally, dominance curves are based on ranking of species in a sample in decreasing order of their abundance (Clarke, 1990). This implies that the species ranking could potentially vary among stations. The advantage of this is that by comparing the steepness of the curves, one obtain an overview on how many species are dominant and the distribution of their relative dominance. However, a more detailed picture in comparison of dominance curves among different stations can be obtained if the species ranking remains fixed according to the species ID (Table 1). In the latter case, the dominance curves will have horizontal parts where specific species are not present in the sample. We propose to have both options available dependent on the objective of the specific research.
Estimating Uncertainty for Biodiversity Measures at the Station Level
The number of individuals of all species identified in the sample from trawl station j was defined as nj:
Because nj is a finite number, a resampling method with replacement (Chernick, 2007) was used to estimate the uncertainty around the value. Obviously, the resulting count numbers nij for the individual species varied from one such resampling to another. By performing 1000 resampling, we obtained a population of data for each nij. After applying equations (1)–(8), we obtained a bootstrap population of 1000 values for each indicator measure, which we used to obtain Efron percentile 95% confidence bands (Efron, 1982) for each indicator measure for each trawl station j. This application of the bootstrap procedure can be viewed as a method for estimating precision using confidence intervals obtained based on creating new datasets from the original dataset by the resampling in each bootstrap iteration and calculating indicator values for each of them (Rowland et al., 2021). This procedure enables estimation of the uncertainty at a station level for each biodiversity index induced by the limited sample size at station level without having to make any prior assumptions regarding the distributions in the samples. This is a well-established procedure for estimation of uncertainty at single sample level such as a station and is being applied in several other branches of science (Manly, 1997; Chernick, 2007). The analysis was conducted using the software tool SELNET (Herrmann et al., 2012), which implements the described method.
Inferring Difference in Biodiversity Between Stations
The difference Δr in a biodiversity index r between the stations x and y was estimated by:
The 95% confidence intervals (CIs) for Δr were obtained based on the two bootstrap population results for rx and ry, respectively, as described above. As they were obtained independently of each other, a new bootstrap population of results for Δr was created using:
where i denotes the bootstrap repetition index. As resampling was random and independent between the two bootstrap populations of results, it is valid to generate the bootstrap population of results for Δr based on (11) using the two independently generated bootstrap files (Moore et al., 2003). Based on the bootstrap population of results for Δr, we were able to obtain Efron percentile 95% confidence bands (Efron, 1982). To determine whether the difference between indices is significant, we compared the overlap among the confidence intervals. If the value 0.0 was not within the obtained confidence bands, then the indicator values for stations x and y differed significantly. In general, the confidence limits for Δr cannot exceed what is spanned by rx and ry together and will often be smaller (Larsen et al., 2018). Therefore, using this approach will increase the power of making inference compared to the simple strategy based on the search for non-overlapping confidence limits between the two confidence bands for rx and ry.
Estimating Mean Biodiversity and Uncertainty for Mean Biodiversity Based on a Group of Stations
First, to estimate the mean value rmean for a biodiversity index r based on a group of trawl stations K (i.e., trawl stations within each cruise), a bootstrap population of results was obtained for it for each trawl station j following the procedure described in section “Estimating Uncertainty for Biodiversity Measures at the Station Level.” The bootstrap population of results was obtained independent for each trawl station. Second, a bootstrap population of results was obtained for rmean by for each of the 1000 bootstrap iterations i independent to form a mean based on resampling K stations with replacement between the K stations available in an outer resampling loop. The mean was then calculated based on the values for r for the stations selected for the specific bootstrap iteration i. This procedure was applied for each of the biodiversity indices described by equations (1)–(8) to provide a bootstrap population of results with 1000 values for the mean value based on the K stations considered. Efron 95% percentile confidence bands were then obtained for the mean values for the biodiversity indices based on this bootstrap population for the mean values.
Estimating Total Biodiversity and Uncertainty for Total Biodiversity Based on a Group of Stations
Section “Estimating Uncertainty for Biodiversity Measures at the Station Level” described how the biodiversity measures were estimated with uncertainties for a single trawl station J. Section “Estimating Mean Biodiversity and Uncertainty for Mean Biodiversity Based on a Group of Stations” described how the mean value with uncertainties can be obtained based on a group of K stations. However, sometimes one would more be interested in estimating the total biodiversity in an area covered based on the K trawl stations rather than estimating the mean based on the stations. To estimate the total value for the biodiversity indices for a group of trawl stations (i.e., trawl stations within each cruise), nij in equations (1)–(8) were replaced with ni which is given by:
where the summation was considered over a group of K stations.
To account for variation between stations when estimating the uncertainties in the total biodiversity, another resampling loop around the one described for single station analysis was applied. This outer resampling loop resampled with replacement K stations over the K stations considered. For each station selected, the inner resampling was conducted accounting for the finite sample size for the specific station according to the resampling procedure described in section “Estimating Uncertainty for Biodiversity Measures at the Station Level.” This nested resampling technique was applied 1000 times, leading to 1000 sets of ni data. We applied equations (1)–(8) to these data to obtain a population of results for the total value of biodiversity indicators to estimate Efron 95% percentile confidence bands for this estimation based on the group of stations within the area considered. The same approach used to compare biodiversity estimates between two stations (section “Inferring Difference in Biodiversity Between Stations”) was applied to compare biodiversity estimates between the groups of stations. The nested bootstrapping technique described here are similar in structure to the well-established method applied for fishing gear size selectivity for estimating the uncertainty (Wileman et al., 1996) when aggregating data over multiple trawl stations (Millar, 1993) except for the structure in the inner resampling which follows the procedure outlined in Larsen et al. (2017). However, its application and adaption to provide realistic uncertainty estimates for overall (total) species biodiversity based on a group of stations is new. With its resampling procedure, the method accounts for both the additional uncertainty induced by variation in sample composition between stations and the uncertainty on station level (section “Estimating Uncertainty for Biodiversity Measures at the Station Level”) caused by the finite sample size in the individual samples.
Figure 1 presents a conceptual diagram on the methodological details of the nested bootstrapping approach described above for estimation of total biodiversity.
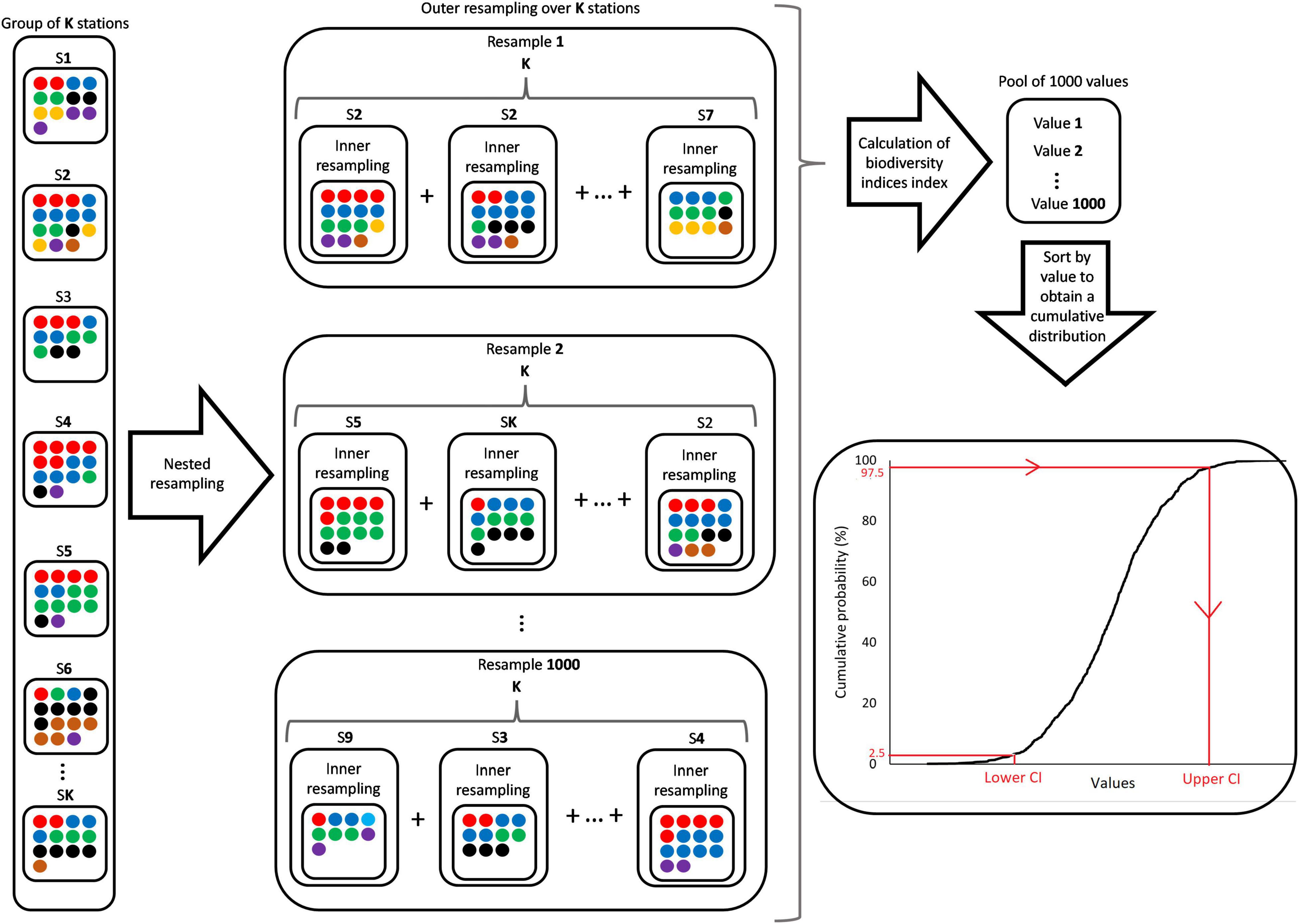
Figure 1. Conceptual diagram on the methodological details of the nested bootstrapping approach applied to estimate uncertainties for the biodiversity indices. Stations S1 to SK have different number of species and number of individuals within each which is represented by colored circles where specific colors represent specific species. The nested bootstrapping consists of an outer resampling loop where the K stations are resampled. The data for each station selected in the outer loop is then resampled in the inner loop. This leads to variation in the composition of numbers of individuals for the same station depictured by the color mixtures for the resampled station data. The inner resampled data belonging to the same outer resampling iteration are summed to produce a pooled population symbolized by the “+.” The obtained data from each pooled population are then used separately to calculate a value for each of the biodiversity indices. Lower and upper confidence limits for each diversity index is subsequently obtained from the cumulative distribution of values. Specifically, the value for the index that corresponds to the 2.5 and 97.5 percentiles represents respectively, lower and upper confidence limits, and thereby forms a 95% percentile confidence interval for the specific index.
Results
Indices of Species Richness
Species richness varied between stations within each cruise (Figure 2). Further, it showed a significant pairwise difference between the cruises regarding total values (Figure 3) and between some of the stations within the cruises (Supplementary Material 2). Total values for species richness differed significantly [pairwise difference (delta)] between cruise 1 and cruises 2 and 3, but the difference between cruises 2 and 3 was not significant (Figure 3). For mean values, there were no significant differences between cruises. Note that the species richness for a station and for a group of stations as total contrary to mean values will have one-sided percentile confidence bands since resampled data cannot contain more species than found in the original sample. This pattern is clear from the results obtained (Figure 2). Contrary, the difference (delta) in species richness will have two-sided percentile confidence bands (Figure 3).
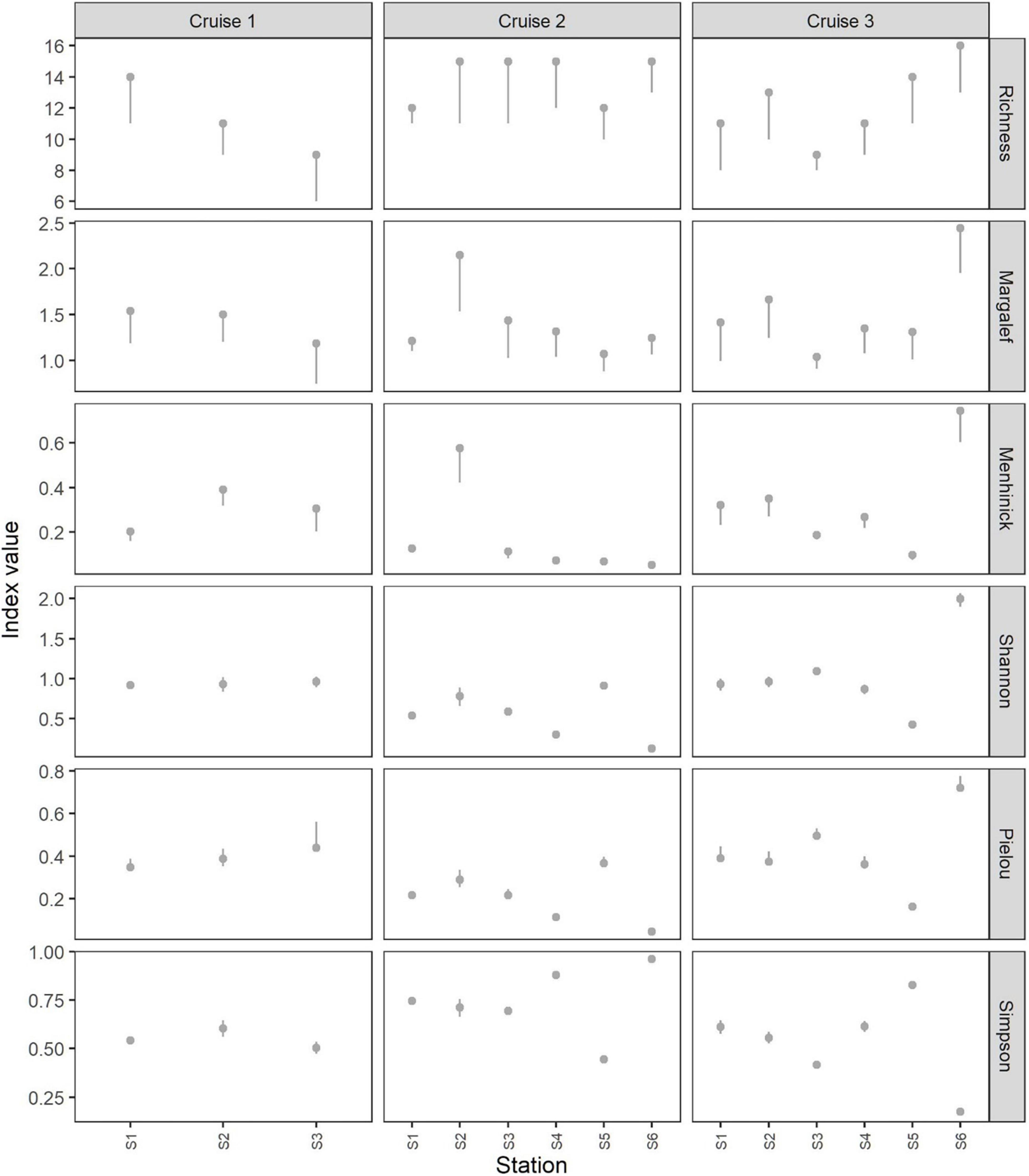
Figure 2. Values of different biodiversity indices estimated for each station and each cruise. Error bars represent 95% confidence intervals.
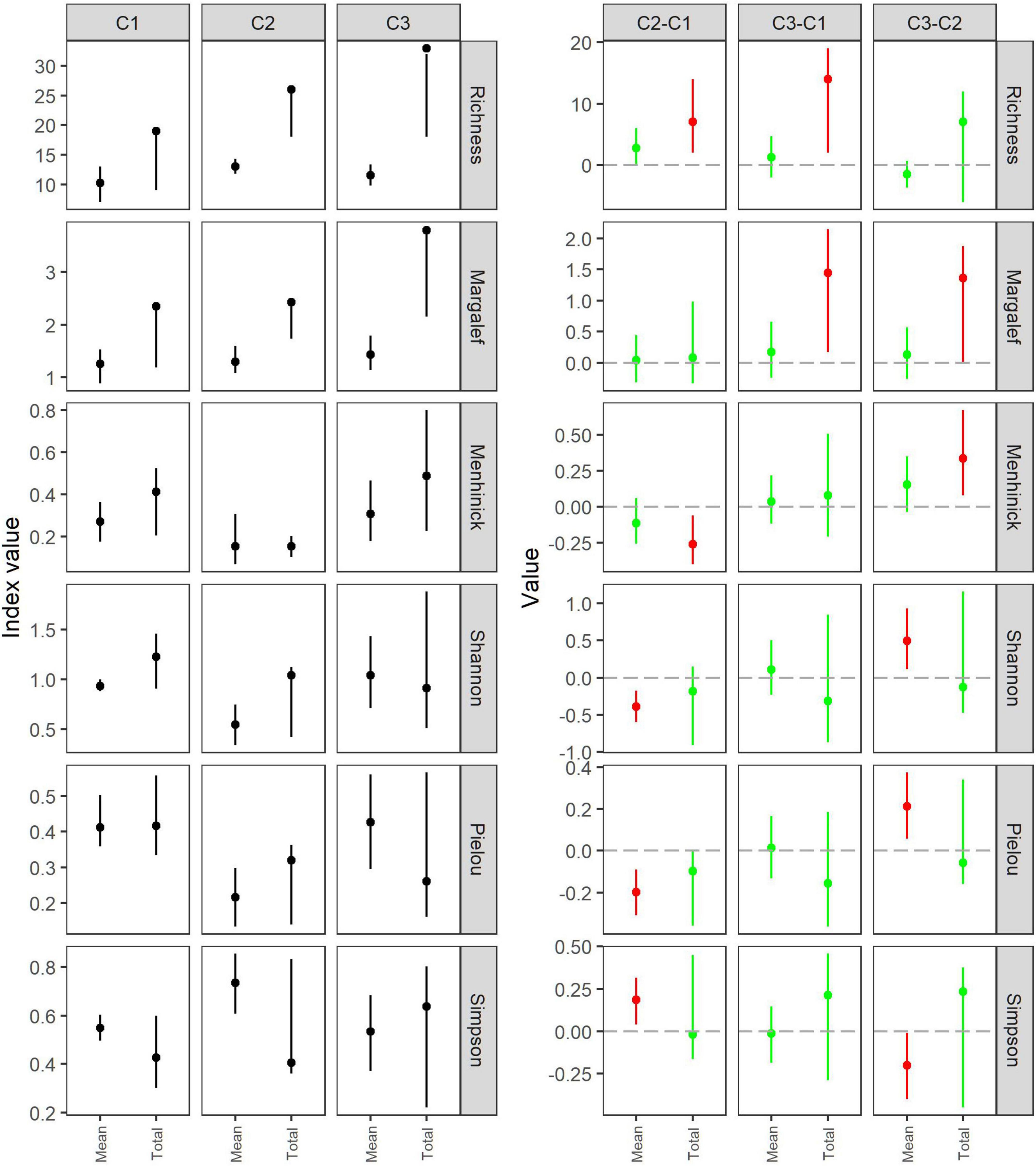
Figure 3. Mean and total values for different biodiversity indices estimated for each cruise (left) and pairwise difference in biodiversity index values (deltas) between cruises (right). Error bars represent 95% confidence intervals (CI). Red circles and corresponding CI highlights significant difference between the two stations, whereas green circles and their CIs represent stations without significant difference in biodiversity index values.
The Margalef and Menhinick indices explain the relationship between the number of species and the number of individuals within each species. Both indices have no upper limit, thus they can be compared between the stations and cruises. In some cases, the values did not display a significant difference in species richness but did differ significantly in terms of the number of individuals within each species (e.g., stations 2 and 3 of cruise 2; Figure 2). Overall, the indices were low (between 1.0 and 1.5 for the Margalef index and between 0.0 and 0.4 for the Menhinick index) compared with two stations displaying higher diversity within each cruise (i.e., station 2 of cruise 2 and station 6 of cruise 3 for both Margalef and Menhinick indices) (Figure 2). At the cruise level, total values for both Margalef and Menhinick indices indicated higher diversity for cruise 3 compared with cruises 1 and 2, but the difference in the Menhinick index between cruise 3 and cruise 1 was not statistically significant (Figure 3). Mean values for those indices did not display any significant differences between cruises (Figure 3).
Index of Entropy
The Shannon index considers not only species richness but also species evenness; therefore, high species richness does not always mirror higher values of the Shannon index (values for stations 2–4 of cruise 2) and vice versa (station 3 of cruise 3) (Figure 2). The variation in Shannon index values between cruises ranged from close to 0.0 at station 6 of cruise 2 to 2.0 at station 6 during cruise 3. There was no significant difference in the Shannon index between cruises regarding total values (Figure 3). Mean values for Shannon index differed significantly between cruise 2 and cruises 1 and 3, but the difference between cruises 1 and 3 was not significant (Figure 3). However, differences in the Shannon index between stations during cruises 2 and 3 were statistically significant (Supplementary Material 2).
Index of Species Evenness
No pairwise difference in the Pielou index was observed when the total values were compared between the three cruises (Figure 3). Mean values for Pielou index differed significantly between cruise 2 and cruises 1 and 3, but the difference between cruises 1 and 3 was not significant (Figure 3). However, the species evenness during each cruise showed significant pairwise differences between stations, with few exceptions (Supplementary Material 2).
Index of Species Dominance
There were significant differences in values of the Simpson index between stations during each cruise, indicating that one or few species were more abundant than the others. The Simpson index exceeded 0.50 at most stations (Figure 2), with exceptions of station 3 of cruise 1, station 5 of cruise 2, and stations 3 and 5 of cruise 3. The dominance index values generally mirrored the values of the Pielou index (higher species dominance of one or few species implies a lower evenness within the sample). In several cases, however, there was a significant difference in species dominance but not in species evenness (pairwise difference between stations 4 and 2 of cruise 3) and vice versa (pairwise difference between stations 2 and 1 of cruise 2) (Supplementary Material 2). In most cases, both the Simpson and Pielou indices exhibited a significant difference in the delta plots, indicating dominance by one or a few species in the sample at the station level. Similar to the Pielou index, the Simpson index did not show significant pairwise difference in the total index values between the cruises (Figure 3). However, the mean values for Simpson index differed significantly between cruise 2 and cruises 1 and 3, while the difference between cruises 1 and 3 was not significant (Figure 3).
Dominance Pattern
Overall, T. inermis (ID 35) was the single species dominating the samples collected during cruises 1 and 2, while T. libellula (ID 34) was also dominant during cruise 3 (43.82% with CI:10.83%–90.71%) (Table 2). Most species were recorded from only a few stations during one of the three cruises. A total of 81.82% of the species were recorded in only one or two cruises across the whole study, and 54.55% of the species were recorded during only one of the cruises. Lanternfish (Myctophidae sp.) (ID 24) did not dominate the samples in any of the cruises, but they were recorded during all three of them, with species dominance values between 0.09 (CI: 0.01–0.25) in cruise 2 and 0.25 (0.08–1.1) in cruise 1.
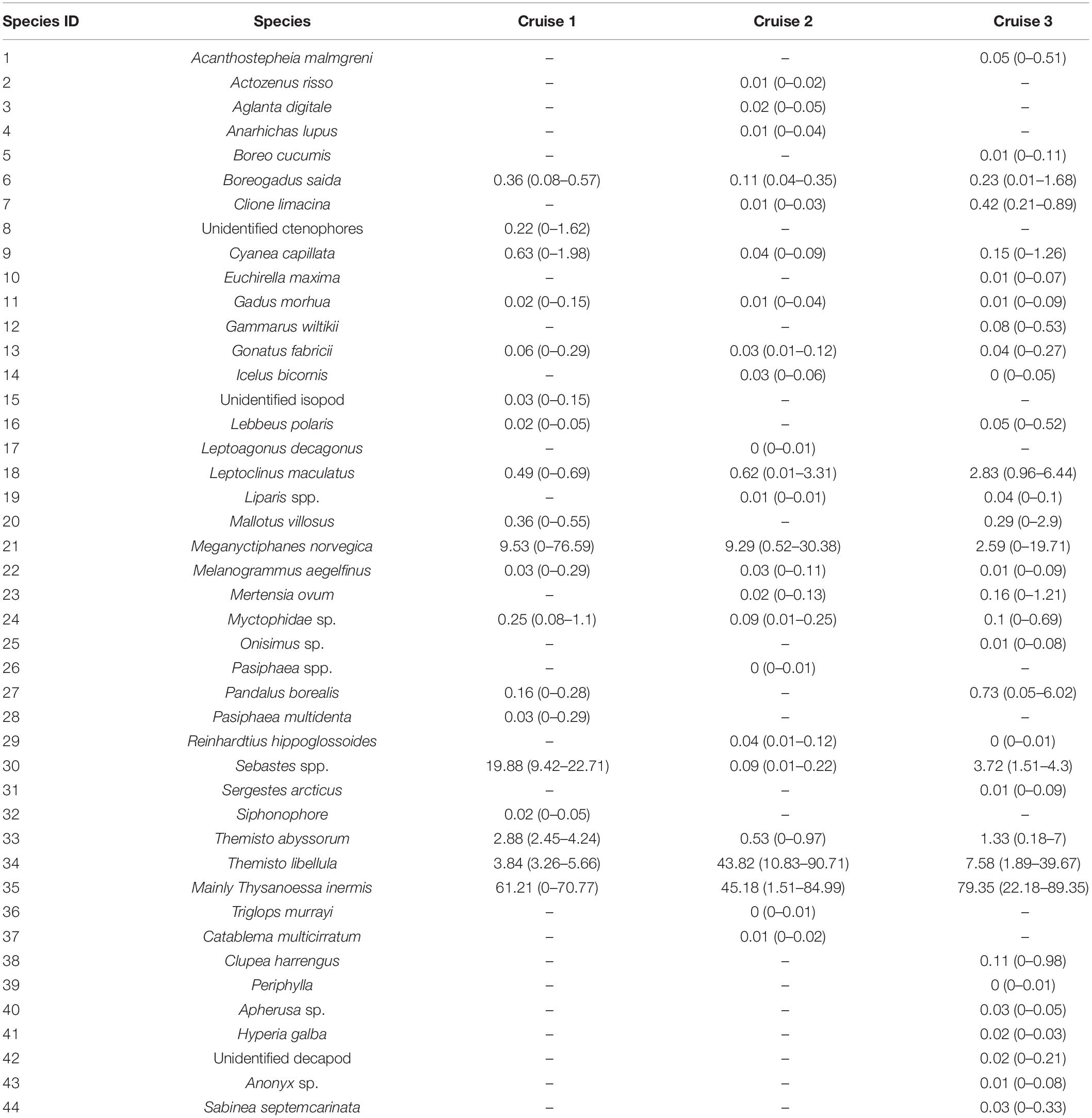
Table 2. Species dominance values dij according to Eq. (8) for each cruise. Values in parentheses represent 95% confidence intervals.
At the station level, organism abundance was dominated by a few species: euphausiids (Meganyctiphanes norvegica) (ID 21) and Thysanoessa inermis (ID 35), the amphipod Themisto libellula, (ID 34) and daubed shanny (Leptoclinus maculatus) (ID 18) (Supplementary Materials 3–5). During cruise 1, krill (M. norvegica) (ID 21) constituted 76.8% (CI: 73.77–79.70%) of the assemblage at station 2, while T. inermis (ID 35) accounted for 66.97% (CI: 63.63–70.09%) to 70.42% (CI: 69.13–71.73%) of all samples (Supplementary Material 3). Cruise 2, which was conducted in summer, showed a different pattern compared with cruises 1 and 3, which were conducted in winter. During cruise 2, T. libellula (ID 34) dominated at five of the six stations, accounting for 78% (CI: 50.22–51.41%) up to 93.68% (CI: 93.45–93.91%) of the mesopelagic community, whereas station 6 was dominated by krill T. inermis (ID 35) (98.00% with CI: 97.9–98.09%) (Supplementary Material 4). During cruise 3, T. inermis (ID 35) dominated the community at five out of six stations [55.85% (CI: 53.79–57.86%) to 90.77% (CI: 90.36%–91.17%)] (Supplementary Material 5).
Figure 4 shows dominance curves for the cumulative dominance values as estimated by Equation (8) for each station within each cruise and as mean and total for the cruises. Note that the species ranking remains fixed. Differences in dominance indicate the dissimilarity among stations sampled in winter (January 2016 and 2017) and summer (August 2016). However, the values within the dominance curves for some stations were larger than in the others for particular species but less so for the others. For example, at stations 3 and 6 of cruise 3, species L. maculatus (ID 18) and M. norvegica (ID 21) were more dominant compared with other stations within the same cruise, which was shown by the incline of the dominance curves for those species. T. libellula (ID 34) and M. norvegica (ID 21) strongly dominated the samples collected in August (cruise 2). In winter, a higher abundance of Thysanoessa spp. (ID 35) was noted (cruises 1 and 3). Redfish species (Sebastes spp.) (ID 30) were more abundant during cruise 1 compared with cruises 2 and 3, and the species was significantly more dominant in cruise 3 compared with cruise 1 (Table 2). The horizontal parts in Figure 4 shows specific species that were not represented in the sample of particular station or groups of stations.
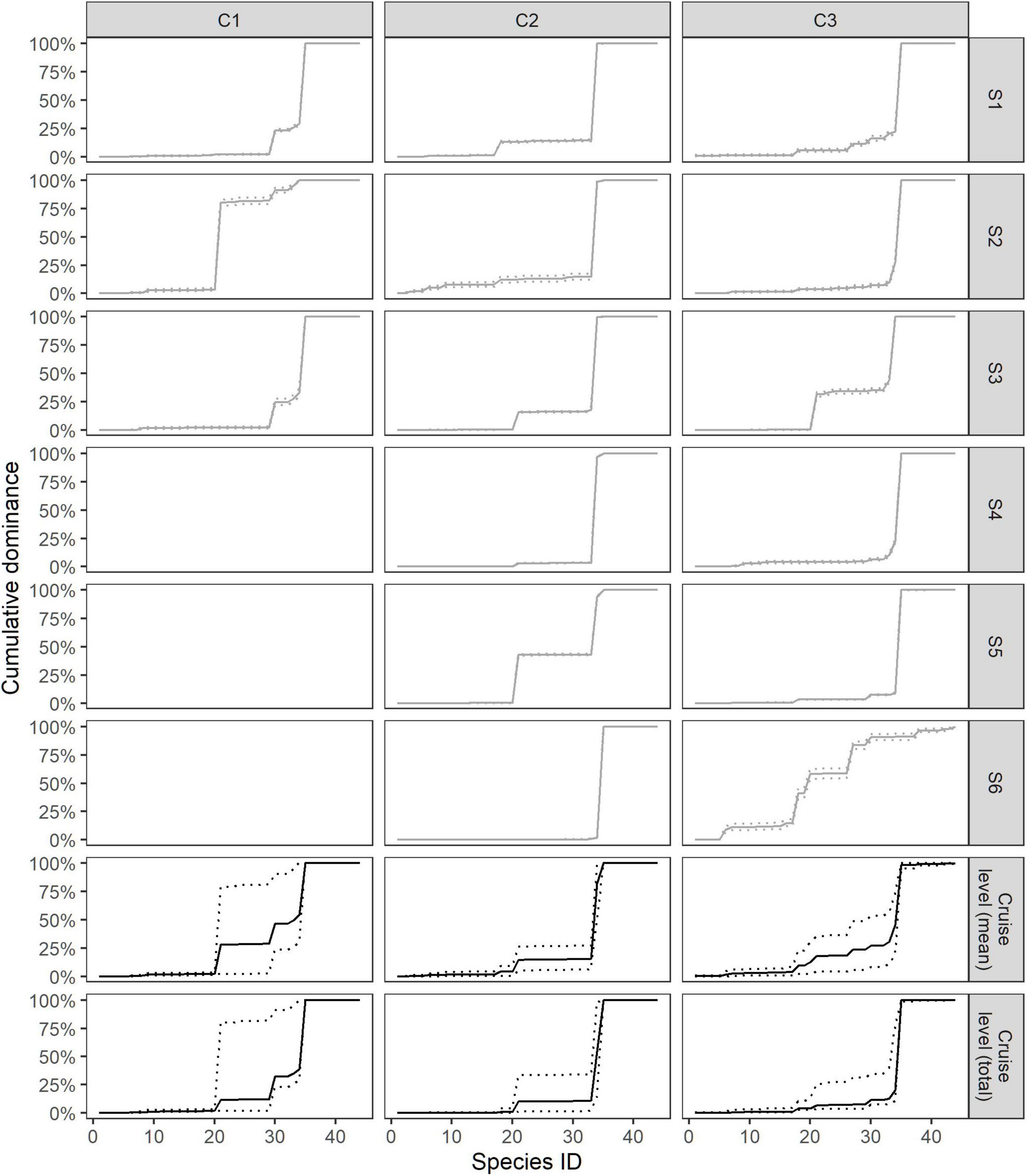
Figure 4. Dominance curves for each station (gray) and mean and total for cruises (black). Dashed lines are 95% confidence intervals. In this case we have made dominance curves where the species ranking remains fixed (see section “Dominance Pattern”).
The pairwise difference in dominance (delta) curves show the difference in species dominance between cruises (Figure 5) and between different stations (Supplementary Materials 6–8). Between cruise differences in species dominance (Figure 5) included increased dominance of redfish (ID30) in cruise 1 compared with the other cruises. Cruise 2 showed increased dominance of T. libellula (ID 34) but lower dominance of T. inermis (ID 35) relative to the cruises conducted in winter (Figure 5).
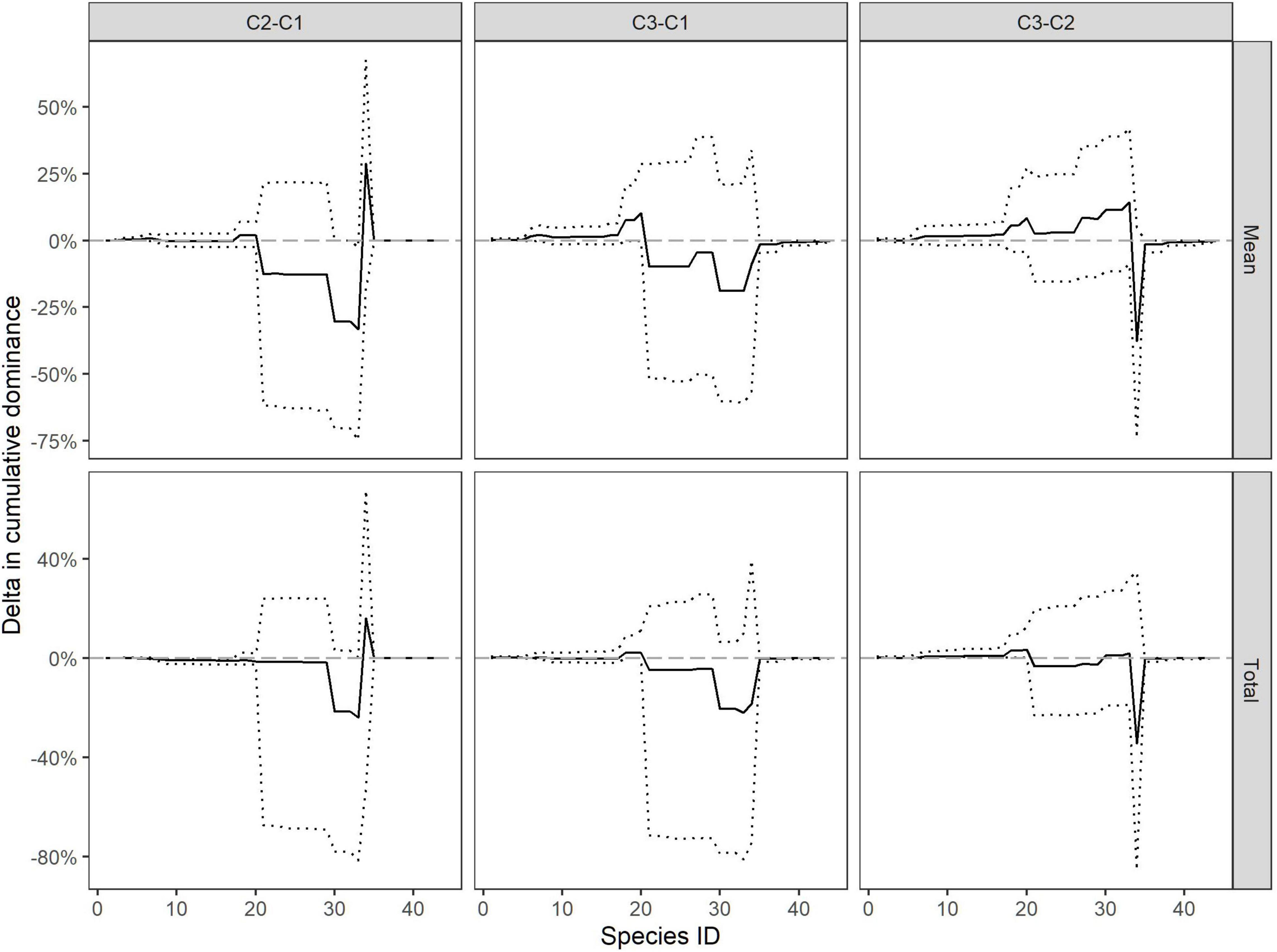
Figure 5. Pairwise difference in dominance curves between cruises. Dashed lines are 95% confidence intervals.
Cruise 1 shows a high dominance of M. norvegica (ID 21) and a lower dominance of redfish (Sebastes spp.) (ID 30) at station 2. Additionally, stations 1 and 3 showed a significantly higher dominance of T. inermis (ID 35) compared with station 2 (Supplementary Material 6). The differences in station level in cruise 2 were mainly associated with the abundance of L. maculatus (ID 18), M. norvegica (ID 21), T. inermis (ID 35), and T. libellula (ID 34). For example, relative to the other stations, station 5 displayed greater dominance of M. norvegica (ID 21), followed by insignificant changes in dominance of the following species (flat curve), which was followed by a decrease in dominance of T. libellula (ID 34) compared with other stations within the cruise. Station 6, however, had a high dominance of T. inermis (ID 35) [98.00% (CI: 97.90–98.09%)] but a significantly lower dominance of T. libellula (ID 34) [0.84% (0.78–0.91%)] compared with the other station in cruise 2 (Supplementary Material 7). In cruise 3, station 6 showed increased dominance of L. maculatus, capelin (Mallotus villosus) (ID 20), and deep-water shrimp (Pandalus borealis) (ID 27) compared with the other stations within the cruise. Both stations 3 and 6 displayed a lower dominance of T. inermis (ID 35) compared with the other stations. Between cruise differences in species dominance (Figure 5) included increased dominance of redfish in cruise 1 compared with the other cruises. Cruise 2 showed increased dominance of T. libellula (ID 34) but lower dominance of T. inermis (ID 35) relative to the cruises conducted in winter (Supplementary Material 8).
Discussion
In this study, we adapted a method based on a nested bootstrapping technique that enables us to account for uncertainties when estimating various biodiversity indices resulting from sampling at the station level and at the cruise level as mean and as total (between-station variation) (Figure 1). This method is applicable when sampling is affected by spatial-temporal variation in the species composition in the study area (station level) and by uncertainty due to the finite sample size taken by the trawl (variation between stations; Fryer, 1991). This method has been widely used to estimate uncertainties (within- and between-station variability) in fishing gear size selectivity studies (Millar, 1993; Herrmann et al., 2017). Herein we have demonstrated that it can also be applied to biodiversity studies that are subject to multilevel sources of uncertainties. The increased power in making inference at a station level or between groups of stations when the delta approach based on the bootstrap calculus [Equations (10)-(11)] is applied, is demonstrated in Figure 3 where richness is being compared between cruises. If the simple approach of looking for non-overlapping confidence bands is applied, no significant difference between cruises 1–2 and 1–3 could be detected. Contrary, when the delta approach is used, we are able to detect a significant difference in both cases. These examples demonstrate the usefulness of the bootstrap with the delta approach to increase the power in making inference.
However, while bootstrapping is a powerful technique to obtain realistic estimates for uncertainties even for complex sampling designs, like, for example, estimates on group level (several stations) without requiring several assumptions to be fulfilled, it, of course, cannot account for species that are not present in any of the samples. In species biodiversity analysis this can be a challenge with species of low abundancy. This is a special challenge regarding the species richness (see section “Indices of Species Richness”) and can cause limitations in studies where the species composition is subjected to under-sampling on species. Thus, the result is affected by the sampling effort. However, this should not be considered as a shortcoming of the statistical analysis method applied, in our case the nested bootstrapping presented in this study. Instead, it should be considered more as a shortcoming or challenge regarding sampling methodology and effort. Further, sampling is also affected by net avoidance, gear selectivity and body composition of the organisms (fragile organisms are known to break and extrude through nets).
We applied our methodology to data collected from the mesopelagic SSL in the high Arctic. In this case study, samples were collected in a similar area during summer and winter periods of 2016–2017, thus covering two different seasons. Smaller organisms, such as small euphausiids, were likely undersampled because we used a 10 mm mesh size codend in the Harstad trawl. Eriksen et al. (2016) estimated that most of the euphausiids larger than 15 mm in length would be retained while most of the individuals smaller than 10 mm in length would be lost when using the Harstad trawl. Sampling biases differ among net catches, optical observations, and acoustic sampling, and they have been well reported for macrozooplankton and micronekton (Kaartvedt et al., 2012; Kloser et al., 2016; Hosia et al., 2017). Hence, we cannot be certain that trawling will retain all mesopelagic organisms in the water column or if the proportions are representative of the SSL. However, this bias would have been consistent among cruises, and our aim was to demonstrate the use of our method on the biodiversity estimation of the sampling catch of the mesoplegaic SSL and not that of the complete mesopelagic community.
Our results showed how applying bootstrapping method provided useful information regarding uncertainty of different indicator values and allowed to estimate if the differences among biodiversity indices between the samplings are significant. Values of the biodiversity indices as total differed among the three cruises, with cruise 1 exhibiting significantly less species richness. This can be explained by the smaller number of species collected due to fewer stations sampled during this cruise (three hauls conducted on cruise 1 compared with six hauls during cruises 2 and 3). The Margalef and Menhinick indices showed the same pattern, as they also consider species richness. Lower diversity associated with some of the stations, as described by the Shannon, Margalef, and Menhinick indices, were likely due to fewer number of species. However, the total value of the Menhinick index did not differ significantly between cruises 1 and 3. The Menhinick index values exhibited smaller variation compared with the Margalef index, which explains the differences in the Margalef and Menhinick in total indices values between cruises 1 and 2 (Figure 3; Cozolli et al., 2017). Other indices (Shannon index, Pielou index, and Simpson index) did not differ significantly in the pairwise comparison of the three cruises. There was significant variation in Pielou index at a station level within each of the cruises, which could have been affected by changes in species distribution throughout the sampling.
The nested bootstrapping method provided CIs for our biodiversity index values, thereby accounting for the uncertainty in the estimation resulting from variation between stations and cruises (Figure 1). Therefore, the method allows estimating uncertainties when extrapolating about a population from a limited size of samples. To the best of our knowledge, this is the first time this bootstrap scheme has been adapted and incorporated into biodiversity analysis. However, the method has a broad scope of applicability and importance to address questions regarding biodiversity estimates, especially since biodiversity indices are estimated using limited data sets to draw broader conclusions (Rowland et al., 2021).
Data Availability Statement
The raw data supporting the conclusions of this article will be made available by the authors, without undue reservation.
Author Contributions
BH, KC, JB, and EG contributed to conception and design of this study and wrote the first draft of the manuscript. MG, MD, and JB collected the data used in this study. KC organized the data. BH, KC, and JB performed the statistical analysis. All authors contributed to manuscript revision, read, and approved the submitted version.
Funding
The EU-project SUMMER (817806) provided financial support for the preparation of this article. Sampling was supported by the Research Council of Norway (NFR) through the Arctic ABC project (grant #244319) and the Marine Night project (grant #226417). MD and MG were supported by NFR grant Deep Impact (grant #300333). MG is financially supported by the Ocean Frontier Institute funded through Canada’s First Research Excellence Fund and ArcticNet a Network of Centres of Excellence Canada.
Conflict of Interest
BH, KC, and EG was employed by the company SINTEF Ocean.
The remaining authors declare that the research was conducted in the absence of any commercial or financial relationships that could be construed as a potential conflict of interest.
Publisher’s Note
All claims expressed in this article are solely those of the authors and do not necessarily represent those of their affiliated organizations, or those of the publisher, the editors and the reviewers. Any product that may be evaluated in this article, or claim that may be made by its manufacturer, is not guaranteed or endorsed by the publisher.
Acknowledgments
We are grateful to the academic editor and reviewers for their valuable comments, which we feel have significantly improved our manuscript. We thank the officers and crew of the R/V Helmer Hanssen and the numerous colleagues who helped during sampling.
Supplementary Material
The Supplementary Material for this article can be found online at: https://www.frontiersin.org/articles/10.3389/fevo.2022.775759/full#supplementary-material
References
Barham, E. G. (1966). Deep scattering layer migration and composition: observation from a diving saucer. Science 151, 1399–1403. doi: 10.1126/science.151.3716.1399
Bland, L. M., Rowland, J. A., Regan, T. J., Keith, D. A., Murray, N. J., Lester, R. E., et al. (2018). Developing a standardized definition of ecosystem collapse for risk assessment. Front. Ecol. Environ. 16:29–36. doi: 10.1002/fee.1747
Chao, A. (2005). “Species richness estimation,” in Encyclopedia of Statistical Sciences, eds N. Balakrishnan, C. B. Read, and B. Vidakovic (New York: Wiley), 7909–7916.
Clarke, K. R. (1990). Comparisons of dominance curves. J. Exp. Mar. Biol. Ecol. 138, 143–157. doi: 10.1016/0022-0981(90)90181-B
Cozolli, F., Stanca, E., Selmeczy, G. B., Francé, J., Varkitzi, I., and Basset, A. (2017). Sensitivity of phytoplankton metrics to sample-size: A case study on a large transitional water dataset (WISER). Ecol. Indic. 82, 558–573. doi: 10.1016/j.ecolind.2017.07.022
Daly, A. J., Baetens, J. M., and De Baets, B. (2018). Ecological Diversity: Measuring the Unmeasurable. Mathematics 6, 119. doi: 10.3390/math
Dietz, R. S. (1948). Deep scattering layer in the Pacific and Antarctic oceans. J. Mar.Res. 7, 430–442.
Efron, B. (1982). The jackknife, the bootstrap and other resampling plans. Philadelphia: Society for Industrial and Applied Mathematics
Eriksen, E., Skjoldal, H. R., Dolgov, A. V., Dalpadado, P., Orlova, E. L., and Prozorkevich, D. V. (2016). The Barents Sea euphausiids: methodological aspects of monitoring and estimation of abundance and biomass. ICES J. Mar. Sci. 73, 1533–1544. doi: 10.1093/icesjms/fsw022
Fryer, R. (1991). A model of the between-haul variation in selectivity. ICES J. Mar. Sci. 48, 281–290. doi: 10.1093/icesjms/48.3.281
Gamfeldt, L., Lefcheck, J. S., Byrnes, J. E. K., Cardinale, B. J., Duffy, J. E., and Griffin, J. N. (2014). Marine biodiversity and ecosystem functioning: what’s known and what’s next? Oikos 124, 252–265. doi: 10.1111/oik.01549
Geoffroy, M., Daase, M., Cusa, M., Darnis, G., Graeve, M., Hernández, N. S., et al. (2019). Mesopelagic sound scattering layers of the high Arctic: Seasonal variations in biomass, species assemblage, and trophic relationships. Front. Mar. Sci. 6:364. doi: 10.3389/fmars.2019.00364
Herrmann, B., Sistiaga, M. B., Nielsen, K. N., and Larsen, R. B. (2012). Understanding the size selectivity of redfish (Sebastes spp.) in North Atlantic trawl codends. J. Northwest Atl. Fish. Sci. 44, 1–13. doi: 10.2960/J.v44.m680
Herrmann, B., Sistiaga, M., Rindahl, L., and Tatone, I. (2017). Estimation of the effect of gear design changes in catch efficiency: methodology and a case study for a Spanish longline fishery targeting hake (Merluccius merluccius). Fish. Res. 185, 153–160. doi: 10.1016/j.fishres.2016.09.013
Hidalgo, M., and Browman, H. I. (2019). Developing the knowledge base needed to sustainably manage mesopelagic resources. ICES J. Mar. Sci. 76, 609–615. doi: 10.1093/icesjms/fsz067
Hosia, A., Falkenhaug, T., Baxter, E. J., and Pagès, F. (2017). Abundance, distribution and diversity of gelatinous predators along the northern Mid-Atlantic Ridge: A comparison of different sampling methodologies. PLoS One 12:e0187491. doi: 10.1371/journal.pone.0187491
Irigoien, X., Klevjer, T., Røstad, A., Martinez, U., Boyra, G., Acuña, J. L., et al. (2014). Large mesopelagic fishes biomass and trophic efficiency in the open ocean. Nat. Commun. 5:3271. doi: 10.1038/ncomms4271
Kaartvedt, S., Staby, A., and Aksnes, D. L. (2012). Efficient trawl avoidance by mesopelagic fishes causes large underestimation of their biomass. Mar. Ecol. Prog. Ser. 456, 1–6. doi: 10.3354/meps09785
Kaiser, M. J., Attrill, M. J., Jennings, S., Thomas, D. N., Barnes, D. K. A., Brierley, A. S., et al. (2011). Marine ecology: processes, Systems, and Impacts, 2nd Edn. New York: Oxford University Press.
Kanieski, M. R., Longhi, S. J., and Soares, R. C. (2018). “Methods for biodiversity assessment: Case study in an area of Atlantic Forest in Southern Brazil,” in Selected Studies in Biodiversity, ed. B. Şen (London: IntechOpen).
Kloser, R. J., Ryan, T. E., Keith, G., and Gershwin, L. (2016). Deep-scattering layer, gas-bladder density, and size estimates using a two-frequency acoustic and optical probe. ICES J. Mar. Sci. 73, 2037–2048. doi: 10.1093/icesjms/fsv257
Larsen, R. B., Herrmann, B., Sistiaga, M., Brinkhof, J., and Grimaldo, E. (2018). Bycatch reduction in the Norwegian Deep-water Shrimp (Pandalus borealis) fishery with a double grid selection system. Fish. Res. 208, 267–273. doi: 10.1016/j.fishres.2018.08.007
Larsen, R. B., Herrmann, B., Sistiaga, M., Brinkhof, J., Tatone, I., and Langård, L. (2017). New approach for modelling size selectivity in shrimp trawl fisheries. ICES J. Mar. Sci. 75, 351–360. doi: 10.1093/icesjms/fsx117
Manly, B. F. J. (1997). Randomization, Bootstrap and Monte Carlo Methods in Biology—Texts in Statistical Science. Boca Raton, FL: Chapman & Hall.
Menhinick, E. F. (1964). A comparison of some species-individuals diversity indices applied to samples of field insects. Ecology 45, 859–861. doi: 10.2307/1934933
Millar, R. B. (1993). Incorporation of between-haul variation using bootstrapping and nonparametric estimation of selection curves. Fish. Bull. 91, 564–572.
Moore, D. S., McCabe, G. P., Duckworth, W. M., and Sclove, S. L. (2003). The Practice of Business Statistics Using Data for Decisions. New York, NY: W.H. Freeman and Co.
Pielou, E. C. (1966). Species-diversity and pattern-diversity in the study of ecological succession. J. Theor. Biol. 10, 370–383. doi: 10.1016/0022-5193(66)90133-0
Proud, R., Cox, M. J., Le Guen, C., and Brierley, A. S. (2018). Fine-scale depth structure of pelagic communities throughout the global ocean based on acoustic sound scattering layers. Mar. Ecol. Prog. Ser. 598, 35–48. doi: 10.3354/meps12612
Proud, R., Cox, M. J., Wotherspoon, S., and Brierley, A. S. (2015). A method for identifying Sound Scattering Layers and extracting key characteristics. Methods Ecol. Evol. 6, 1190–1198. doi: 10.1111/2041-210X.12396
Rowland, J. A., Bland, L. M., James, S., and Nicholson, E. (2021). A guide to representing variability and uncertainty in biodiversity indicators. Conserv. Biol. 35, 1669-1682. doi: 10.1111/cobi.13699
St. John, M. A., Borja, A., Chust, G., Heath, M., Grigorov, I., Mariani, P., et al. (2016). A Dark Hole in Our Understanding of Marine Ecosystems and Their Services: Perspectives from the Mesopelagic Community. Front. Mar. Sci. 3:31. doi: 10.3389/fmars.2016.00031
Warwick, R. M., Clarke, K. R., and Somerfield, P. J. (2008). “k-Dominance Curve,” in Encyclopedia of Ecology, eds S. E. Jørgensen and B. D. Fath (Oxford: Academic Press), 2055–2057.
Whittaker, R. H. (1972). Evolution and measurement of species diversity. Taxon 21, 213–251. doi: 10.2307/1218190
Keywords: uncertainty, sound scattering layer, mesopelagic, bootstrapping, biodiversity indices, Arctic
Citation: Herrmann B, Cerbule K, Brčić J, Grimaldo E, Geoffroy M, Daase M and Berge J (2022) Accounting for Uncertainties in Biodiversity Estimations: A New Methodology and Its Application to the Mesopelagic Sound Scattering Layer of the High Arctic. Front. Ecol. Evol. 10:775759. doi: 10.3389/fevo.2022.775759
Received: 14 September 2021; Accepted: 07 April 2022;
Published: 27 April 2022.
Edited by:
Jody R. Reimer, The University of Utah, United StatesReviewed by:
Matthew Joseph Michalska-Smith, University of Minnesota Twin Cities, United StatesAndrea Petetta, National Research Council (CNR), Italy
Copyright © 2022 Herrmann, Cerbule, Brčić, Grimaldo, Geoffroy, Daase and Berge. This is an open-access article distributed under the terms of the Creative Commons Attribution License (CC BY). The use, distribution or reproduction in other forums is permitted, provided the original author(s) and the copyright owner(s) are credited and that the original publication in this journal is cited, in accordance with accepted academic practice. No use, distribution or reproduction is permitted which does not comply with these terms.
*Correspondence: Kristine Cerbule, a3Jpc3RpbmUuY2VyYnVsZUB1aXQubm8=
†These authors have contributed equally to this work