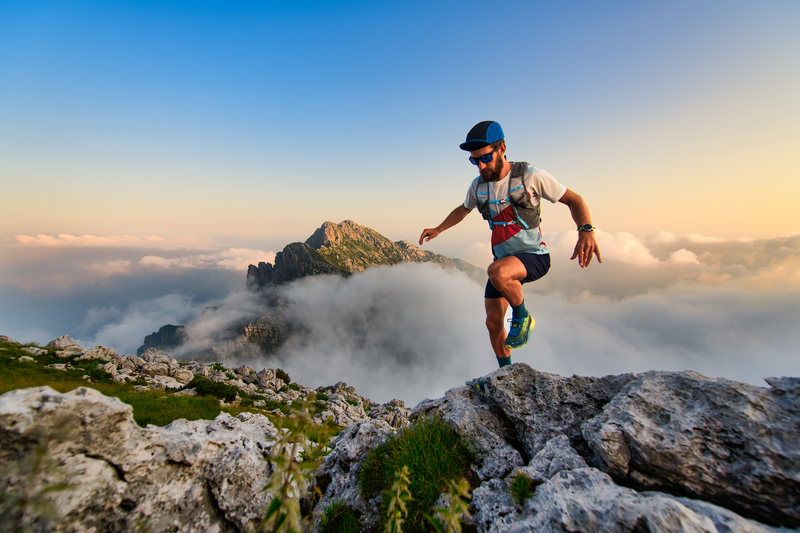
94% of researchers rate our articles as excellent or good
Learn more about the work of our research integrity team to safeguard the quality of each article we publish.
Find out more
BRIEF RESEARCH REPORT article
Front. Ecol. Evol. , 10 February 2022
Sec. Population, Community, and Ecosystem Dynamics
Volume 10 - 2022 | https://doi.org/10.3389/fevo.2022.771490
This paper found the optimization direction of ecological compensation project from the perspective of farmers’ well-being. Based on the data of 2016 China Labor Force Dynamic Survey (CLDS2016), this paper uses the ordered Probit model to investigate the impact of the Grain for Green Program on farmers’ subjective well-being. The study found that the Grain for Green Program significantly reduced farmers’ subjective well-being. Further research found that the Grain for Green Program ultimately inhibited the subjective well-being of farmers by reducing the subjective perception of air, soil and noise pollution, intensifying the subjective perception of water pollution and reducing the per capita income of households. In the new round of the Grain for Green Program in the “14th Five-Year Plan” period, it is advisable to increase the intensity of income compensation, strengthen supervision to ensure the payment of compensation to households, and control the scale of the project based on ecological foundation, so as to improve the well-being of farmers.
Building happy villages is a key task in China’s rural revitalization strategy, and it is also an inevitable choice for China to pursue the quality and efficiency of economic development. It has become the priority governance goal of local governments at all levels. The construction of happy villages is closely related to the construction of ecological compensation projects. In April, 2018, General Secretary Xi Jinping made an significant instruction during his investigation in Zhejiang, which was to “build an ecologically livable beauteous countryside, so that the majority of farmers can have more sense of gain and well-being in rural revitalization.” Therefore, understanding China’s ecological compensation project is the key to building happy villages and implementing the rural revitalization strategy.
Among the global ecological compensation projects, China’s Grain for Green Program has the largest investment scale, the widest coverage and the highest level of public participation. The Grain for Green Program is an ecological compensation project that takes the protection and improvement of ecological environment as the starting point, will step by step stop the cultivation of sloping farmland prone to soil and water loss in a planned way and subsidize farmers to plant trees according to local conditions. In order to further optimize the construction of China’s ecological compensation project and create happy villages, it is urgent to evaluate the impact of China’s Grain for Green Program on farmers’ well-being, and deepen the understanding of its mechanism, so as to optimize the policy choices.
Based on this, this paper will use the 2016 China Labor Force Dynamic Survey (CLDS2016) data to study the impact of the Grain for Green Program on farmers’ well-being. The marginal contributions of this paper may lie in: (1) It provides the direct statistical evidence of relationship between Grain for Green Program and farmers’ well-being for the first time and find that the Grain for Green Program significantly inhibits farmers’ well-being. (2) Based on the survey data at the national level, it provides the evidence that the Grain for Green Program reduces farmers’ income. (3) Policy suggestions were provided to optimize the Grain for Green Program to improve farmers’ well-being.
There is still controversy in the academic circle about the impact of the Grain for Green Program on the income of farmers. Some scholars believe that the Grain for Green Program may reduce farmers’ income. Based on the field investigation in Mei County, Shaanxi Province, Han and Shi (2010) pointed out that there were cases of local governments misappropriating and embezzling compensation funds during the construction of the Grain for Green Program, which reduced the family income. Zhang et al. (2019) used the CHIPS data in 2013 to explore the effect of the Grain for Green Program in the Yellow River and Yangtze River basins on farmers’ income reduction. The program mainly reduced farmers’ income by reducing labor supply and reducing human capital. Yang et al. (2019) used the data from the rural household follow-up survey sponsored by the Central Ministry of Finance and the Asian Development Bank. Based on the income level of farmers, it was found that the Grain for Green Program has significantly reduced the incidence of poverty, but it varied from year to year and from region to region, and the poverty targeting efficiency was low.
However, some scholars hold the view that the Grain for Green Program is helpful to help the poor and improve the income and welfare of farmers. Li and Tuo (2012) examined the positive impact of the Grain for Green Program on farmers’ income and welfare in Zhouzhi Mountain Area of Shaanxi Province. Liu et al. (2013) studied the dynamic impact trend of the Grain for Green Program in Wuqi County on the relative poverty breadth, intensity and depth of farmers, which first decreased and then increased. Wang and Ximing (2017) analyzed the positive impact of the Grain for Green Program on non-agricultural employment and farmers’ income during the “11th Five-Year Plan” period in 21 provinces in China.
Income is an important factor affecting residents’ well-being. Based on the survey data of Guangdong Province, Zhang and Cai (2011) found that the absolute income increase can significantly strengthen the residents’ well-being, and the absolute income level has an inverted U-shaped relationship with the residents’ well-being. Luo (2009) found that relative income can significantly enhance subjective well-being, and when considering the influence of absolute income and relative income on well-being, the absolute income variable coefficient is not significant. Therefore, the Grain for Green Program is likely to affect farmers’ subjective well-being by affecting their income.
A large number of documents show that the Grain for Green Program will improve the level of environmental quality and reduce environmental pollution. Based on the sediment data of 11 rivers in China, Deng et al. (2012) provided evidence that the Grain for Green Program could solidify soil and improve soil quality. Based on the carbon emission data, Chang et al. (2011) pointed out that the Grain for Green Program has significantly reduced the carbon emission in grassland and forest areas. Xiao (2014) has used the satellite database of the National Aeronautics and Space Administration (NASA) of the United States to show that the Grain for Green Program has significantly improved the air quality in the Loess Plateau region.
However, some scholars still hold negative opinions on the environmental improvement effect of the Grain for Green Program. It is believed that the Grain for Green Program will significantly worsen the water resources situation in arid and semi-arid areas, and large-scale construction of forest land in ecologically fragile areas will aggravate the regional water shortage (Shixiong et al., 2009). Chen et al. (2015) pointed out that the excessive scale of the Grain for Green Program will have negative impact on the community and the environment, including the shortage of food supply and the pollution and shortage of water resources.
And environmental pollution is the decisive factor affecting residents’ well-being. Yang and Zhang (2014) pointed out that air pollution can reduce residents’ health status, affect residents’ subjective mood, and thus inhibit residents’ well-being. Based on CLDS data, Linxiang and Zhang (2020) found that the higher the income, the stronger the inhibition effect of residents’ subjective air pollution on well-being, and the higher the income, the stronger the perception of individual subjective air pollution for the same environmental level, further strengthening the inhibition effect on well-being. Zheng et al. (2015) used CGSS data and found that subjective environmental pollution significantly inhibited residents’ well-being.
Therefore, the Grain for Green Program is likely to strengthen subjective well-being by reducing farmers’ subjective air pollution, subjective noise pollution and subjective soil pollution. However, the Grain for Green Program may also strengthen farmers’ subjective perception of water pollution, Shixiong et al. (2009) provide the statistical evidence in a northern part of China’s Shaanxi Province. Since there might be high demands for water as a consequence of the fast growth of pioneer species, such as hippophae rhamnoides, which may exacerbate soil water shortages, at least in the short term, especially in the arid and semiarid areas and further inhibit their subjective well-being.
Based on the above analysis, this paper puts forward two opposite assumptions:
Ha: Under certain other conditions, the Grain for Green Program inhibits farmers’ subjective well-being.
Hb: Under certain other conditions, the Grain for Green Program will strengthen farmers’ subjective well-being.
The data in this paper are derived from the CLDS2016 hosted by the Social Science Survey Center of Sun Yat-sen University. The sample covers the labor force aged 15–64 in 29 provinces and cities across the country (except Hong Kong, Macao, Taiwan, Hainan, and Tibet), including three levels of information: village residence, family, and individual labor force, which is representative of the country (Wang et al., 2017). The survey contains detailed information about the participation in the Grain for Green Program, life satisfaction and residents’ subjective well-being, which provides the basis for the study.
The core explained variable in this paper is farmers’ well-being. Interviewees were asked the question “On the whole, do you think your life is happy?” The answer measures of “very happy,” “happy,” “average,” “unhappy,” and “very unhappy” are assigned as 5, 4, 3, 2, and 1 in sequence by interviewees. The higher the value, the better the sense of well-being. The explanatory variable is the participation in the Grain for Green Program, and the CLDS questionnaire provides the situation at the village level. Among the control variables, personal characteristic variables include gender (1 infers male, 0 infers female), age, health (Interviewees were asked the question “How do you feel about your health now?” The answer measures of “very healthy,” “healthy,” “average,” “unhealthy,” and “very unhealthy” are assigned as 5, 4, 3, 2, and 1 in sequence. And it has been suggested that although there is some measurement error in self-rated health as a subjective indicator of health, it is still a good predictor of health status and is even more robust than objective measures of health (Poortinga, 2006), education level, political status (1 present party member, 0 present the other), religious belief (1 infers having religious belief, 0 present the other), family per capita income (get logged measured after calculated with household income and number of family members), marital status (1 infers getting married, 0 infers the other), debt (1 infers own debt, 0 infers the other), trust (very disagree, disagree, agree and very agree are assigned as 1, 2, 3, 4 in sequence). The regional characteristic variables include whether non-agricultural economy exists (1 infers work in non-agricultural occupation, 0 infers the other). It has been found that the socio-economic status, health statues and the high level of trust to society could increase the sense of social equity, which in turn impact the sense of well-being (Linxiang and Zhang, 2020; Ni, 2020). Table 1 shows the descriptive statistics.
Subjective well-being of the explained variable in this paper is a five-item ordered selection variable. Due to the incomparability of the distances between the adjacent options of the ordered variables, it is inappropriate to directly use the ordinary least squares method (OLS). This paper follows the processing method of Liu et al. (2018) and chooses the Ordered Probit model to estimate. This model has been used to study a series of hierarchical levels of residents’ subjective perception variables because it can accurately reflect the marginal changes of ordered variables. The subjective well-being variables in this paper are applicable to this model. The Ordered Probit Model is an extension of Probit Model, which was first developed by McKelvey and Zavoina (1975) and applied to deal with the measurement problem that the explained variables are in discrete order. The model assumes that there is a latent continuous potential variable happy* that can represent the explained variable (redistributing preferences and noting as happyi) but cannot be directly measured to satisfy.
The explained variable happyi in this paper is in discrete form and expressed by a nonlinear function F(⋅):
In the formula (3), k1,k2,k3,k4k5 represent tangent points, and the latent variable represents the interpreted variable happyi in sorted discrete form according to different tangent point sizes.
Where happyi, represents the subjective well-being of the i-th interviewee, greeni indicates whether the village where the i-th interviewee is located is participating in the Grain for Green Program. Xiindicates a series of village-level characteristics and personal characteristics control variables described above, and εi is a random interference term. We mainly focus on the coefficient α1. If α1 is positive, it indicates that the Grain for Green Program can strengthen the well-being of farmers. If α1 is negative, it indicates that the Grain for Green Program can inhibit the well-being of farmers. If α1 is not significant, the Grain for Green Program has nothing to do with the well-being of farmers.
The benchmark measurement results are given in Table 2. The first three columns are the measurement results using the ordered Probit model. Column (1) is the result of controlling individual covariates, and column (2) is based on column (1), which in turn controls family and community-related covariates. In addition, according to the State Council’s rules for the division of eastern, central and western regions of the PRC, column (3) is based on column (2), which also controls the effect of territorial fixation. In order to list different methods for comparison, column (4) are regression results of OLS model. According to the final results, no matter what kind of measurement model is used or what kind of control variable group is controlled, the Grain for Green Program significantly inhibits farmers’ well-being. According to the descriptive statistics, the average well-being level of farmers in the country is 3.78. As can be seen from column (1), under the condition of controlling individual covariates, participating in the Grain for Green Program reduces farmers’ well-being by 0.094 units, that is, the Grain for Green Program reduces farmers’ well-being by 2.49%, which is believable at the 99% interval level. Column (2) shows that under the condition of controlling the relevant covariates between the family and the community, the Grain for Green Program can reduce farmers’ well-being by 0.081 units, that is, the Grain for Green Program can reduce farmers’ well-being by 2.14%, which is believable at the level of 99%. Both the fixed effect in the controlled area and the regression results using OLS model show that the Grain for Green Program significantly inhibits residents’ well-being.
From the above analysis, it can be concluded that the Grain for Green Program can significantly inhibit farmers’ well-being. This section will further discuss the mechanism of the impact of the Grain for Green Program on farmers’ subjective well-being. This paper considers that the Grain for Green Program affects farmers’ well-being mainly including income mechanism and environmental mechanism.
As for the income mechanism, as the existing research indicates that there is an “inverted U” relationship between income and subjective well-being perception, the conclusion that the income mechanism is tenable cannot be reached only by studying the impact of the Grain for Green Program on income. It can be empirically tested through the intermediary effect model. The intermediate effect model needs to expand the regression of dependent variable to the explained variable (step 1), dependent variable to the intermediate variable (step 2) and intermediate variable to the explained variable (step 3) in turn. If the third measurement result shows that the coefficient of the dependent variable is significant, but there is a significant decrease in absolute value of the coefficient relative to the first measurement result, it can be concluded that the intermediate variable plays a part of the intermediate effect (Wen et al., 2004). And since the individual income is not provided in CLDS database, we use household income to calculate the income per capital data to present income level.
As shown in Table 3, under the condition that other control variables are consistent, column (1) is the regression result between the variable of the Grain for Green Program and the variable of well-being, column (2) is the regression result between the variable of the Grain for Green Program and the intermediate variable of family per capita income, and column (3) is the regression result between the variable of the Grain for Green Program and the variable of family per capita income on well-being. From column (2), it can be seen that the conversion of cropland to forest project has significantly reduced the per capita income level of households by 0.131 units, and it is believable at the level of 99%. Compared with the measurement results in column (1), the column (3)’s absolute value of the regression coefficient of the variable of the Grain for Green Program decreased from 0.051 to 0.039, and the significance of the regression coefficient decreased. Therefore, the Grain for Green Program significantly reduces the per capita income of households, and the variable of per capita income of households plays a part of intermediary effect in the process of reducing farmers’ well-being by the conversion of cropland to forest project.
As for the environmental mechanism, the literature review shows that subjective environmental pollution can significantly and directly reduce farmers’ well-being. If the Grain for Green Program can affect the subjective environmental perception, it can be concluded that the Grain for Green Program can improve farmers’ subjective well-being by reducing the subjective environmental pollution; Or the Grain for Green Program can reduce farmers’ subjective well-being by aggravating subjective environmental pollution.
In order to measure the level of subjective environmental pollution (or subjective environmental quality), this part is based on the questions in the questionnaire about air pollution, water pollution, soil pollution and noise pollution: “How serious do you think the following questions are in the place where you live?” “not serious at all,” “not too serious,” “more serious,” and “not serious at all,” which are assigned as 4, 3, 2, and 1 in sequence according to the answers provided by the farmers. The higher the value, the lower the subjective pollution degree of relevant environmental problems and the higher the subjective environmental quality.
As shown in Table 4, the Grain for Green Program has significantly improved the subjective environmental quality of air and soil and subjective noise-free perception, and significantly reduced the subjective environmental quality of water resources. This is consistent with the conclusion of the existing literature. That is, the Grain for Green Program intensifies the subjective perception of water pollution and reduces the subjective perception of air pollution, soil pollution and noise pollution. Therefore, the Grain for Green Program strengthens the subjective well-being of farmers by reducing the levels of subjective air, soil and noise pollution. At the same time, the Grain for Green Program can reduce the subjective well-being of farmers by strengthening the subjective perception of water pollution.
On the whole, the Grain for Green Program will ultimately inhibit the subjective well-being of farmers by reducing the subjective perception of air, soil, and noise pollution, intensifying the subjective perception of water pollution and reducing the per capita income of households.
Based on the data of CLDS2016, this paper uses the ordered Probit regression model to make an empirical study and draws the following conclusions: the Grain for Green Program has significantly inhibited the well-being of farmers. The Grain for Green Program has suppressed the farmers’ subjective well-being by reducing the farmers’ income, and the reduction of farmers’ income is the intermediary path to suppress the farmers’ subjective well-being; The Grain for Green Program also suppresses farmers’ subjective well-being by increasing farmers’ subjective perception of water pollution; However, the Grain for Green Program can strengthen farmers’ subjective well-being by reducing their subjective perception of soil, air and noise pollution. Under the comprehensive effect of various paths, the Grain for Green Program finally suppressed the subjective well-being of farmers.
Based on the above conclusions, in order to reverse the negative effect of the Grain for Green Program on farmers’ well-being as a positive effect, this paper puts forward the following suggestions:
Firstly, raise the subsidy standard for the Grain for Green Program. When the subsidy standard is raised, farmer’s income get directly increased, witch could mitigate the negative impact from income decrease caused by Grain for Green program. In order to better enhance the subjective well-being of farmers. For instance, those ecological forests whose subsidies have expired are included in the scope of subsidies for public welfare forests now, but there is still a big gap between their subsidy standards and the real needs for farmers, and there is an urgent need for government to raise the subsidy standard as to integrate the Green for Green Program into the system of governance of mountains, rivers, forests, farmlands, lakes, and grasslands, which can also optimize the use of support funds for agriculture and forestry industry.
Secondly, according to the local ecological foundation, the scale of the Grain for Green Program should be expanded reasonably. In areas with fragile ecological foundation, the large-scale Grain for Green Program will lead to the shortage of water resources and environmental damage instead since there might be high demands for water as a consequence of the fast growth of pioneer species which may exacerbate soil water shortages. It is appropriate to implement the differentiated policy of the Grain for Green Program in some arid and semi-arid areas to consolidate the achievements of ecological construction, so as to enhance the well-being of farmers. For instance, reducing uptake of limited soil water by vegetation should be highly concerned for management of Grain for Green Project in some arid and semi-arid areas, those species that need large amounts of water should be replaced with more suitable local species, for instance, in the arid/semi-arid area of northern Shaanxi Province, the local species such as dwarf shrubs have better afforestation performance than those non-native pioneer species such as hippophae rhamnoides (Shixiong et al., 2009), which can also reduce the transport cost and planning cost.
The original contributions presented in the study are included in the article/supplementary materials, further inquiries can be directed to the corresponding authors.
ZY and TW designed the study, analyzed the data, and wrote the manuscript. MG and SZ collected the data and coordinated the data analysis. JC and ZY revised the manuscript. All authors have read and agreed to the published version of the manuscript.
This research was supported by grants from National Natural Science Foundation of China (Grants 72104189 and 71991482).
The authors declare that the research was conducted in the absence of any commercial or financial relationships that could be construed as a potential conflict of interest.
All claims expressed in this article are solely those of the authors and do not necessarily represent those of their affiliated organizations, or those of the publisher, the editors and the reviewers. Any product that may be evaluated in this article, or claim that may be made by its manufacturer, is not guaranteed or endorsed by the publisher.
Chang, R., Fu, B., Liu, G., and Liu, S. (2011). Soil carbon sequestration potential for “grain for green” project in Loess Plateau, China. Environ. Manag. 48, 1158–1172. doi: 10.1007/s00267-011-9682-8
Chen, Y., Wang, K., Lin, Y., Shi, W., Song, Y., and He, X. (2015). Balancing green and grain trade. Nat. Geosci. 8, 739–741. doi: 10.1038/ngeo2544
Deng, L., Shangguan, Z.-p, and Li, R. (2012). Effects of the grain-for-green program on soil erosion in China. Int. J. Sediment Res. 27, 120–127. doi: 10.1016/S1001-6279(12)60021-3
Han, H., and Shi, Z. (2010). Economic sustainability analysis of china’s grain for green project: an empirical study based on Mei County, Shaanxi Province. J. Agrotech. Econ. 4, 85–91.
Li, J., and Tuo, H. (2012). Analysis on the welfare status of farmers in the farmland conversion area in Zhouzhi, Shaanxi Province based on feasible ability. Manag. Rev. 24, 66–72+101.
Linxiang, Y., and Zhang, W. (2020). Subjective air pollution, income level and residents’ happiness. J. Financ. Econ. 46, 126–140.
Liu, X., Si, H., and Pang, J. (2018). Regional intergenerational mobility and residents’ well-being: an investigation based on intergenerational education mobility. J. World Econ. 41, 171–192.
Liu, Z., Yao, S., and Qu, M. (2013). Dynamic evolution of farmers’ relative poverty in Wuqi: 1998-2011. China Popul. Resour. Environ. 23, 56–62.
Luo, C. (2009). Absolute income, relative income and subjective well-being: an empirical analysis from china’s urban and rural household survey data. J. Financ. Econ. 35, 79–91.
McKelvey, R. D., and Zavoina, W. (1975). A statistical model for the analysis of ordinal level dependent variables. J. Math. Sociol. 4, 103–120.
Poortinga, W. (2006). Social relations or social capital? Individual and community health effects of bonding social capital. Soc. Sci. Med. 63, 255–270. doi: 10.1016/j.socscimed.2005.11.039
Shixiong, C., Chen, L., and Yu, X. (2009). Impact of China’s grain for green project on the landscape of vulnerable arid and semi-arid agricultural regions: a case study in Northern Shaanxi Province. J. Appl. Ecol. 46, 536–543. doi: 10.1111/j.1365-2664.2008.01605.x
Wang, J., Zhou, Y., and Liu, S. (2017). China labor-force dynamics survey: design and practice. Chin. Sociol. Dialogue 2, 83–97. doi: 10.1177/2397200917735796
Wang, S., and Ximing, Y. (2017). Grain for green, non-agricultural employment and farmers’ income increase: a double difference analysis based on panel data of 21 provinces. Econ. Res. J. 52, 106–119.
Wen, Z., Zhang, L., Hou, J., and Liu, H. (2004). Intermediary effect test procedure and its application. Acta Psychol. Sin. 5, 614–620.
Xiao, J. (2014). Satellite evidence for significant biophysical consequences of the “grain for green” program on the Loess Plateau in China. J. Geophys. Res. Biogeosci. 119, 2261–2275. doi: 10.1002/2014jg002820
Yang, J., and Zhang, Y. (2014). Pricing of air pollution: an analysis based on happiness data. J. World Econ. 37, 162–188. doi: 10.1177/0890117118763742
Yang, J., Liu, C., and Li, H. (2019). Measurement and analysis of precision poverty alleviation effect of grain for green project. J. Quant. Tech. Econ. 36, 64–86.
Zhang, W., Xue, J., and Zhang, X. (2019). Effects of the policy of grain gor green on farmers’ income and its mechanism. Rural Econ. 6, 130–136.
Zhang, X., and Cai, G. (2011). Income, values and residents’ happiness: empirical evidence from Guangdong adult survey data. Manag. World 9, 63–73.
Keywords: Grain for Green Program, well-being, ecological compensation, ordered Probit model, mechanism
Citation: You Z, Wu T, Gong M, Zhen S and Cheng J (2022) The Impact of the Grain for Green Program on Farmers’ Well-Being and Its Mechanism—Empirical Analysis Based on CLDS Data. Front. Ecol. Evol. 10:771490. doi: 10.3389/fevo.2022.771490
Received: 06 September 2021; Accepted: 06 January 2022;
Published: 10 February 2022.
Edited by:
Christos Athanassiou, University of Thessaly, GreeceReviewed by:
Efstathios Kaloudis, University of the Aegean, GreeceCopyright © 2022 You, Wu, Gong, Zhen and Cheng. This is an open-access article distributed under the terms of the Creative Commons Attribution License (CC BY). The use, distribution or reproduction in other forums is permitted, provided the original author(s) and the copyright owner(s) are credited and that the original publication in this journal is cited, in accordance with accepted academic practice. No use, distribution or reproduction is permitted which does not comply with these terms.
*Correspondence: Mengqi Gong, Z29uZ21lbmdxaUB3aXQuZWR1LmNu
Disclaimer: All claims expressed in this article are solely those of the authors and do not necessarily represent those of their affiliated organizations, or those of the publisher, the editors and the reviewers. Any product that may be evaluated in this article or claim that may be made by its manufacturer is not guaranteed or endorsed by the publisher.
Research integrity at Frontiers
Learn more about the work of our research integrity team to safeguard the quality of each article we publish.