- 1Department of Wildland Resources, Utah State University, Logan, UT, United States
- 2Sierra Nevada Bighorn Sheep Recovery Program, California Department of Fish and Wildlife, Bishop, CA, United States
- 3Department of Wildlife, Fish, and Conservation, University of California, Davis, Davis, CA, United States
Seasonal migration is a behavioral response to predictable variation in environmental resources, risks, and conditions. In behaviorally plastic migrants, migration is a conditional strategy that depends, in part, on an individual’s informational state. The cognitive processes that underlie how facultative migrants understand and respond to their environment are not well understood. We compared perception of the present environment to memory and omniscience as competing cognitive mechanisms driving altitudinal migratory decisions in an endangered ungulate, the Sierra Nevada bighorn sheep (Ovis canadensis sierrae) using 1,298 animal years of data, encompassing 460 unique individuals. We built a suite of statistical models to partition variation in fall migratory status explained by cognitive predictors, while controlling for non-cognitive drivers. To approximate attribute memory, we included lagged attributes of the range an individual experienced in the previous year. We quantified perception by limiting an individual’s knowledge of migratory range to the area and attributes visible from its summer range, prior to migrating. Our results show that perception, in addition to the migratory propensity of an individual’s social group, and an individual’s migratory history are the best predictors of migration in our system. Our findings suggest that short-distance altitudinal migration is, in part, a response to an individual’s perception of conditions on alterative winter range. In long-distance partial migrants, exploration of migratory decision-making has been limited, but it is unlikely that migratory decisions would be based on sensory cues from a remote target range. Differing cognitive mechanisms underpinning short and long-distance migratory decisions will result in differing levels of behavioral plasticity in response to global climate change and anthropogenic disturbance, with important implications for management and conservation of migratory species.
Introduction
Seasonal migration is defined as a regular movement between two or more discrete seasonal ranges separated in space and time (Avgar et al., 2014; Berg et al., 2019). Seasonal migration allows individuals inhabiting environments with temporally predictable variation to track resources, like forage and mates, avoid risks associated with predation and parasitism, and mediate exposure to adverse climatic conditions (Fryxell and Sinclair, 1988; Mysterud et al., 2011; Avgar et al., 2014). Migratory behavior contributes to the increased growth, survival, and reproduction of migratory individuals (Hebblewhite and Merrill, 2011; White et al., 2014). Across a population, the improved demographic performance of migrants contributes to larger equilibrium population sizes than could be achieved through resident behavioral strategies (Fryxell et al., 1988; Fryxell and Sinclair, 1988). The influence of migrants also spans ecosystems as they transport nutrients, disperse seeds, serve as vectors for parasites and disease, and participate in trophic interactions, bridging disjunct habitats (Bauer and Hoye, 2014; Shaw, 2016). The ecological services and ecotourism industries supported by migration generate considerable economic value for the public (Shaw, 2016; Kauffman et al., 2021). The conservation of migratory behavior is important not only to maintain sustainable populations of migratory species, but also to protect the ecological and economic benefits migration confers.
The size of migratory populations and prevalence of migratory behavior has declined globally across terrestrial herbivores, particularly among ungulates (Harris et al., 2009; Tucker et al., 2018; Kauffman et al., 2021). The loss of migrants is primarily attributed to anthropogenic disturbance and global climate change (Lendrum et al., 2013). In species such as mule deer (Odocoileus hemionus) (Sawyer et al., 2019) and caribou (Rangifer tarandus) (Dalerum et al., 2007), migration is a fixed, innate behavior arising from genetics or maternal effects (Møller et al., 2011; Pulido, 2011). However, migration can also be a conditional strategy with individuals exhibiting behavioral plasticity in response to changes in their internal state and external environment, as observed in moose (Alces alces) (Ball et al., 2001; Singh et al., 2012), roe deer (Capreolus capreolus) (Cagnacci et al., 2011), pronghorn (Antilocapra americana) (White et al., 2007), and bighorn sheep (Spitz et al., 2018; Denryter et al., 2021b). Behaviorally plastic migrants make a series of choices with respect to their migratory movements. First individuals decide if they will migrate or remain resident on their present range. If migrating, they also choose when to leave and where to go. We consider only the initial decision to migrate in this manuscript, and refer to this event as a “migratory decision”. Our objective is to understand why individuals choose to migrate, rather than where they go or how they get there. Understanding why terrestrial herbivores decide to migrate is necessary to predict the conditions under which migratory behavior may disappear, allowing for the implementation of proactive, rather than reactive conservation measures.
Plastic migratory behavior is hypothesized to be a response to the physical environment, conditional on an individual’s internal state and social environment. Individuals may decide to migrate to follow ephemeral resources, or avoid risks and unfavorable conditions in portions of their physical environment. The forage maturation hypothesis posits that migrants track phenological gradients of plant growth across the landscape to maximize energy and nutrient intake (Fryxell and Sinclair, 1988; Hebblewhite et al., 2008; Berg et al., 2019). Conversely, the predator avoidance hypothesis states that individuals migrate to escape or mitigate consumptive risk, including predation, parasitism, and hunting pressure (Bergerud et al., 1990; Barten et al., 2001; Skov et al., 2011). Migration may also allow individuals to avoid adverse conditions they cannot tolerate including extreme temperatures, drought, and precipitation events (Ketterson and Nolan, 1976; Sabine et al., 2002; Brinkman et al., 2005). Resources, risks, conditions, and the interactions of these elements are presumably evaluated by an individual, in the context of its internal state and social environment, as it decides whether or not to migrate. Relatively fixed (like age class, sex, or life history strategy) and continuously variable (like body fat or lactation status) attributes of an individual’s physical condition may influence its migratory propensity (Lundberg, 1987; Grayson and Wilbur, 2009; Chapman et al., 2011; Pulido, 2011; Berg et al., 2019). The terminal investment hypothesis predicts that older individuals will be more likely to accept mortality risks associated with migration to access resources that increase the likelihood of successful reproduction, compared to younger individuals who should invest in their future reproductive potential, and hence, their long-term survival (Clutton-Brock, 1984). These hypotheses suggest that migration is a behavioral adaptation to optimize fitness in variable physical environments, and that behavioral plasticity is a response to shifts in the risk-reward tradeoff over the lifetime of an individual.
An individual’s internal state is also shaped by its social environment – here defined as all intraspecific interactions – through density dependent effects and transmission of information between individuals. The dominance or competitive release hypothesis predicts that less dominant individuals will migrate to avoid intraspecific competition for resources when populations reach high densities (Gauthreaux, 1982; Nelson, 1995; Mysterud et al., 2011). On the other hand, positive density dependence (Allee effects) may influence migratory propensity when remaining part of a group confers a fitness advantage (Borrello, 2012) through increased predator vigilance (many eyes) (Lima, 1995; Rieucau and Martin, 2008), reduced predation risk via dilution and confusion effects (Pulliam, 1973; Krause and Ruxton, 2002; Chapman et al., 2011), or transmission of knowledge from experienced migrants (Heyes, 1994; Mueller et al., 2013; Jesmer et al., 2018; Lowrey et al., 2020). The migratory behavior of an individual may mirror the migratory tendencies of its social group – what we term migratory “culture” – because behavior matching maintains group cohesion. These hypothesized mechanisms underpinning migratory plasticity are heavily focused on external drivers, while an individual’s knowledge of its internal state and environment are given little consideration.
An implicit assumption of most research into the environmental drivers of migration is that individuals are omniscient, possessing complete and perfect knowledge of their surroundings near and far [as approximated by remotely-sensed environmental covariates, but see cultural transmission (Jesmer et al., 2018)]. However, behaviorally plastic migrants, particularly long-distance migrants, are likely making migratory decisions with less than complete knowledge of the habitat at their destination. Cognition, the processes concerned with the acquisition, retention, and use of information (Dukas, 1998, 2004; Kashetsky et al., 2021), is the lens through which an individual comes to understand its physical environment. Therefore, migratory behavior arises from an individual’s ‘informational state’- knowledge of its current and alternative environments, rather than the attributes of the environment itself (Blumstein and Bouskila, 1996; Avgar et al., 2013; Merkle et al., 2019).
An individual’s knowledge of its environment may result from previous experience, which when encoded in the brain and retained over time is called memory (Fagan et al., 2013; Kashetsky et al., 2021). Memory encompasses two forms of information: an individual’s experience of its physical environment (attribute memory) and the spatial location associated with that experience (spatial memory) (Fagan et al., 2013). Bracis and Mueller (2017) found that memory was the best cognitive predictor of long-distance migration in zebras. Abrahms et al. (2019) uncovered evidence of blue whale reliance on memory to track algal blooms. Merkle et al. (2019) were unable to replicate the migratory trajectories of mule deer without the inclusion of a spatial memory component in their individual-based simulations. However, all three of these studies focused on the cognitive processes underlying navigation during migration, rather than migratory decision-making. Memory provides behaviorally plastic migrants with a cognitive mechanism to evaluate the profitability of alternative behavioral strategies based on past range conditions. However, memory-driven migration is a response to a predicted state of the migratory range based on past experience, rather than a response to temporally proximate cues. In environments characterized by high interannual variability of resources, risks and conditions, memory-driven migration may lead to a mismatch between the timing of movement and the optimal environmental state of the target range.
Alternatively, individuals could make migratory decisions in response to information gathered about the present state of the physical environment. Individuals perceive their environment through visual, olfactory, auditory, and other sensory cues, but the information available to an organism is constrained by its perceptual range. Therefore, perception may be a more useful cognitive mechanism for guiding local movements and short-distance migrations, than for facilitating long-distance migratory movements (Bracis and Mueller, 2017). Bracis and Mueller (2017), Abrahms et al. (2019), and Merkle et al. (2019) evaluated perception as a mechanism facilitating navigation during migration in their respective systems, but found that individual responses to local forage conditions did not give rise to the observed migratory trajectories. Interestingly, all three studies compared memory and perception as cognitive drivers of migration in species of long-distance migrants (migratory destination outside of the perceptual range of an individual). To our knowledge, a similar comparative analysis has never been conducted in a population of short-distance migrants (migratory destination within the perceptual range of an individual). In focusing on navigation, these previous studies also only included individuals that had previously made the decision to migrate. The cognitive drivers of migratory decision-making have yet to be addressed empirically. Understanding how the cognitive drivers of short and long-distance migration differ will be important for predicting the plasticity of migratory behavior in response to anthropogenic habitat alteration and global climate change.
While cognition likely underpins migratory behavior, particularly in behaviorally plastic migrants, cognitive drivers of migratory decision-making receive much less attention than other hypothesized mechanisms (Kashetsky et al., 2021). Our objective is to determine if cognitive processes – specifically attribute memory and perception – influence the decisions of large herbivores to undertake short-distance, altitudinal migrations. We sought to answer this question by investigating longitudinal location data gathered from Sierra Nevada bighorn sheep (Ovis canadensis sierrae, hereafter SNBS), an ideal study species because SNBS exhibit one of the highest rates of individual switching between resident and migratory behavior among ungulates (Spitz et al., 2018). We built a suite of mixed effect logistic regression models with variation in migratory status explained by cognitive and non-cognitive predictors. Our goal was to isolate the contribution of cognitive processes to migratory decision-making by controlling for the non-cognitive drivers with the most explanatory power, and then introducing the effects of cognition. We modeled attribute memory by including lagged environmental covariates reflecting range conditions experienced by an individual in the previous year. Since bighorn are a highly visual species with good long-distance eyesight (Mooring et al., 2003), we integrated perception into our models by quantifying the area and attributes of alternative ranges a bighorn could see at the time it was making a migratory decision using a viewshed analysis (ESRI, 2020). We eliminated covariates from the global and nested models using stepwise model reduction and evaluated model performance using goodness of fit statistics, finding that perception is the most important cognitive process influencing migratory propensity in SNBS.
Materials and Methods
Focal Species and Study Site
Sierra Nevada bighorn sheep are a subspecies of partially (not all members of the population migrate) and facultatively (individuals are not fixed in their migratory strategy and may switch between years) migratory wild sheep endemic to the southern and central portions of the Sierra Nevada mountain range in eastern California (US Fish and Wildlife Service, 2007). Due to market hunting and disease transmission from domestic sheep, by the 1970s only three native SNBS herds remained (US Fish and Wildlife Service, 2007). Herds are subpopulations defined by boundaries in geographic space outlined in the 2007 SNBS Recovery Plan (US Fish and Wildlife Service, 2007). Between 1979 and 1988, those three herds were used to expand the geographic range of SNBS throughout much of the species’ historic range. There are presently 14 SNBS herds, ranging in size from 9 to 138 animals, for a total population of approximately 600 individuals.
The Sierra Nevada (hereafter Sierra) mountains are 650 km long and between 75 and 125 km wide, with a north-south elevational gradient (Hill, 2006). The tallest peaks, including the highest point in the conterminous United States (Mt. Whitney; 4,421 m), are found in the southern part of the range. The western slope of the Sierra is gentle and mesic in contrast to the steep, xeric escarpment of the eastern slope (Hill, 2006). During the winter, most SNBS herds are found to the east of the Sierra crest in habitat encompassed by the range’s rain shadow. Annual precipitation is highly variable, but generally falls in the form of snow between October and May, with snow persisting at high-elevations until late summer (California Department of Water Resources, 2019). Snow accumulation at high-elevations ranges between 500 and 1,500 cm annually (California Department of Water Resources, 2019). Winds near the crest of the Sierra average 43 km/h and scour snow from the alpine ridges, providing snow-free habitat for SNBS (Bair et al., 2015).
Sierra Nevada bighorn sheep summer in the alpine (elevations > 3,300 m) (Spitz et al., 2018), a habitat spanning the crest of the Sierra characterized by sparse vegetation interspersed with meadows. Average temperatures in the alpine range from daily lows of −2°C to highs of 15°C during the summer, and −14 to −1°C during the winter, with snowfall possible year-round (California Department of Water Resources, 2019). SNBS may overwinter in the alpine or at lower elevations (1,525–2,500 m) (Spitz et al., 2018). The low-elevation winter range is characterized by sage brush-steppe (Artemisia tridentata) plant communities. During the winter, average daily temperatures on these low-elevation ranges are between −2 and 7°C (California Department of Water Resources, 2019). SNBS may travel through mid-elevation (2,500–3,300 m) pinyon-juniper woodlands, coniferous forests, and subalpine meadows when migrating from summer to low-elevation winter range. The straight-line distance between high- and low-elevation ranges is, however, typically <5 km.
Migratory Delineation
Location data for SNBS are collected by the California Department of Fish and Wildlife (CDFW) via direct capture, ground field surveys, and very high frequency (VHF) and global positioning system (GPS) telemetry (Stephenson et al., 2012). For the purposes of this study, our animal year begins July 1st (post spring migration and lambing) and ends June 30th. Our animal years are further divided into 3-month seasons with summer defined as July–September (months 7–9), fall as October–December (months 10–12), winter as January–March (months 1–3), and spring as April–June (months 4–6). Helicopter net gun captures are conducted in the spring and fall to deploy GPS and VHF collars and for the purposes of translocating individuals between herd units. Annual ground surveys are conducted during the summer or winter seasons for every herd unit (with seasonal variability in survey timing necessary to optimize survey success). Observers undertake a full population census for each herd unit, recording the number, age, and sex of all bighorn, along with the location of marked individuals (Johnson et al., 2010a). Monthly fixed wing telemetry flights gather positional data on VHF-collared bighorn (Stephenson et al., 2012). GPS-collared animals provide between 2 and 24 daily locations, uploaded continuously to CDFW via satellite.
We used these four sources of positional data to classify the migratory behavior of individual SNBS. Migration of SNBS is altitudinal (2,000–2,500 m) over relatively short distances (4–10 km), and its fall bout (from high to low-elevation) can occur any time between November and January of the following year (Spitz et al., 2018). Spitz et al. (2017) developed an altitudinal net squared displacement (NSD) method for classifying the migratory status of SNBS, but the approach is only applicable to individuals with sufficient telemetry data. We developed a new technique to delineate migration in SNBS that can accommodate all forms of positional data, increase sample size, and allow for the fitting of more complex models. We used an elevational cutoff of 2,850 m to demarcate ‘migrant’ and ‘resident’ classes of SNBS. We selected a cutoff of 2,850 m because that elevation provided a clear separation between used high and low-elevation winter habitat across herd units based on GPS data. For any given year, animals detected below 2,850 m one or more times during the winter season (January–March) were classified as ‘migrant,’ while individuals not detected below 2,850 m, but observed at higher elevations were classified as ‘resident.’ We deemed a single detection below the elevational threshold sufficient to classify an individual as migratory based on a sensitivity analysis of GPS collared individuals. Removing individuals with few positional fixes below 2,850 m did not change the number of migrants. We assigned animals known to be alive, but not detected during a given year an “unknown” migratory status.
Given that multiple types of location data are often collected for a single individual during an animal year, we developed a hierarchical classification structure for migratory behavior based on the uncertainty of observing an individual below 2,850 m. We considered data types with the most positional fixes to provide the most reliable migratory classifications because they minimize the risk of failing to detect an individual on low-elevation winter range, if it was present. In our hierarchy, there are four levels; GPS data provides the most accurate migratory classification, followed by VHF, ground survey, and capture data. We found few conflicts between migratory classifications across levels of the hierarchy so we concluded a migratory designation could arise from any data type. We validated our migratory classification via elevational cutoff for GPS-collared bighorn against NSD classifications of migration and found that our categorizations only differed by our treatment of flexible migrants. Our classifier has an intentional migratory bias because our focus is on drivers of seasonal movements to low-elevation winter range. Our use of realized migration as a proxy for migratory decision-making rests on the assumption that all individuals that decide to migrate complete the migration, and are observed on low-elevation winter range. We believe this assumption is reasonable given that SNBS typically complete their fall migration within the span of a few days.
Range Delineation
We developed a habitat suitability metric to delineate available summer, winter high, and winter low-elevation ranges independent of present SNBS use. We chose to define ranges independent of present use to (1) accommodate individuals without GPS data, and (2) to create a temporally-static set of polygons within which we explore temporally dynamic variables. We defined three range classes (summer, high-elevation winter, and low-elevation winter) using the resource selection functions (RSFs) for SNBS presented in Spitz et al. (2020) (Figure 1). RSFs provide a continuous characterization of range-specific habitat based on relative intensity of use. To demarcate summer range, we used ArcGIS (ESRI, 2021) to select the largest 5% of RSF values from a raster describing the relative intensity of summer habitat use, and merged these cells into sub-seasonal range polygons, excluding areas less than 200,000 m2 (Figure 1C). To define the winter ranges, we combined the raw RSF values for high and low-elevation habitat in raster cell x into a single index using the formula:
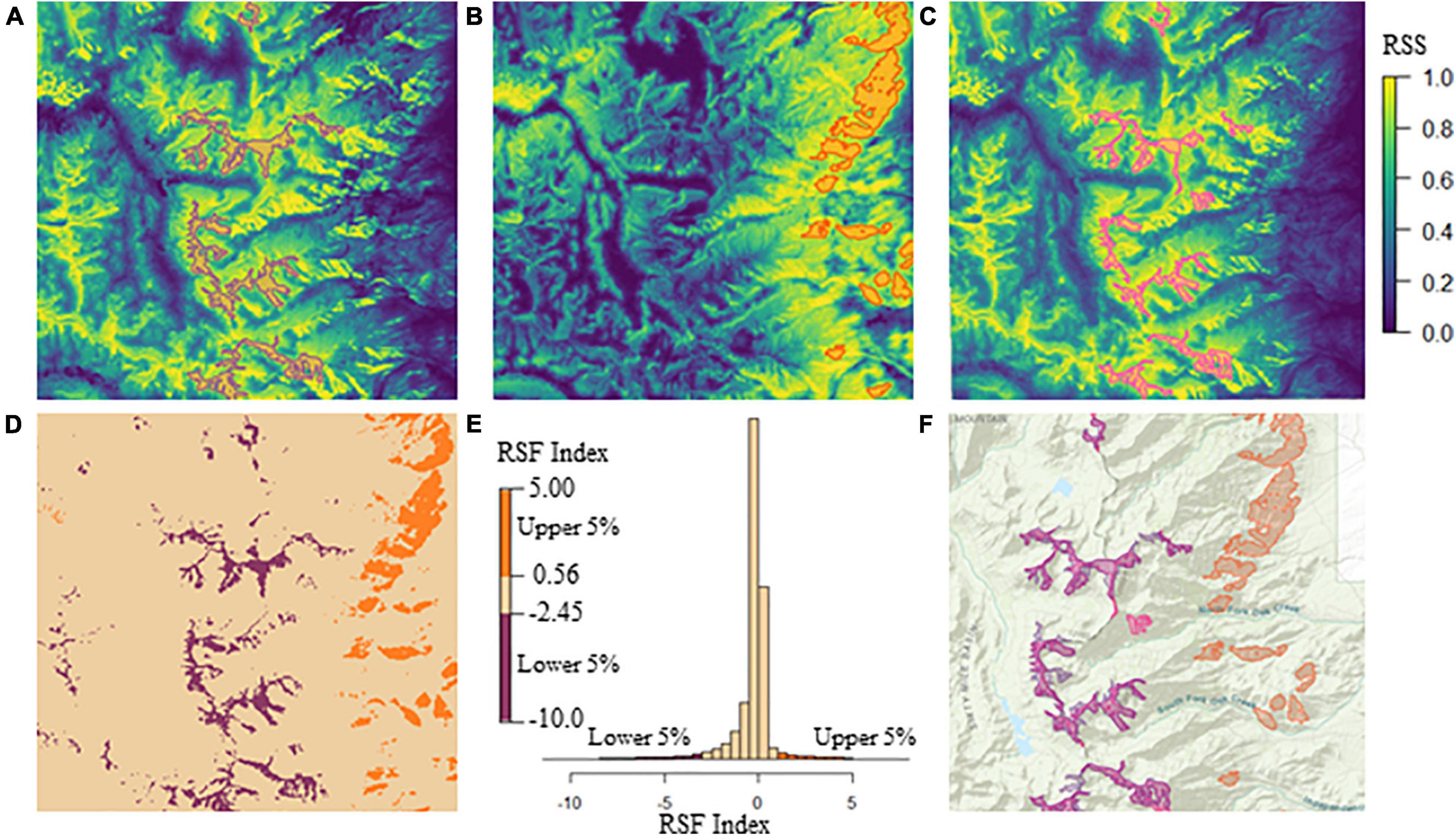
Figure 1. (A) Baxter herd unit high-elevation winter range RSF with blue and yellow colors representing low and high values of relative selection strength (RSS), respectively (RSS colors should be interpreted similarly for panels B,C). The purple polygons delineate the Baxter high-elevation winter range considered in our analysis, and encompass the lowest 5% of RSF index values, excluding polygons with areas less than 200,000 m2. (B) Baxter herd unit low-elevation winter range RSF with orange polygons delineating the low-elevation winter range considered in our analysis. Polygon boundaries encompass the highest 5% of RSF index values, excluding areas less than 200,000 m2 in size. (C) Baxter herd unit summer range RSF with pink polygons delineating the summer range considered in our analysis. Polygon boundaries encompass the highest 5% of RSS values, excluding areas less than 200,000 m2 in size. (D) Baxter herd unit RSF index values plotted spatially. Orange low-elevation and purple high-elevation winter range raster cells contain values that fall within the highest 5% or lowest 5% of the RSF index, respectively. Tan raster cells include all index values outside of the most extreme 10% of the data. This index map does not perfectly match the realized range polygons because areas less than 200,000 m2 are included. (E) A histogram of the RSF index raster values. Orange and purple bars represent the highest and lowest 5% of values, respectively (corresponding with low and high elevation range in panel D). The tan bars include all values outside of the most extreme 10% of the data (corresponding with the landscape matrix in panel D). (F) Composite map of Baxter herd unit high and low-elevation winter range and summer range polygons. High-elevation winter and summer range overlap extensively, hence why the pink and purple polygons are nearly indistinguishable.
where wRSFlow and wRSFhigh are the winter RSF values for low and high elevation habitats in x. The index describes high and low winter habitat in a single raster with high-elevation winter range having the smallest (negative) values and low-elevation winter range having the highest (positive) values (Figure 1). The use of the RSF index, rather than the raw values, ensures that the classifications of high and low-elevation range are mutually exclusive. A raster cell must have both a large combined RSF value (wRSFlow(x) + wRSFhigh(x)), and a large range-specific RSF value (low or high) to be considered a candidate for inclusion in a winter range. We selected the largest and smallest 5% of the values to define the low-elevation and high-elevation winter sub-seasonal ranges, respectively. Both the RSF (summer range) and index (winter ranges) threshold values were chosen because the resultant sub-seasonal ranges encompassed all the GPS points of range resident (non-transient) individuals. Based on this range delineation, each herd unit contains between 1 and 35 sub-seasonal ranges for each combination of season and elevation, that we refer to in aggregate as the seasonal range (summer, high-elevation winter, and low-elevation winter). Given that individuals without GPS data cannot be attributed to a sub-seasonal range polygon, all individual associations are considered to occur at the level of the seasonal range.
Covariates
We attempted to explain variation in migratory status between individuals using cognitive predictors – perception and memory – while controlling for non-cognitive drivers – an individual’s internal state and social and physical (conditions, risks, and resources) environment. The physical environment of an SNBS is characterized by forage biomass, shrub cover, snow-free area, distance to steep terrain, lion predation risk, and distance to migratory range. The biological importance and methods used to derive each covariate are explored in detail below. While change in the physical environment may underlie migration, it is unclear if SNBS are pushed from their present range by unfavorable conditions or pulled to an alternate range by favorable conditions. For an individual to be drawn to an alternative range, it must possess some information about conditions at that location. An individual may remember what it experienced at that location in the previous year (memory) or use its senses to gather information about the present state of the alternate range (perception). Memory and perception provide partial information about conditions at a distant location, but an individual may also possess complete information (omniscience), as assumed by many ecological models. An individual’s propensity to migrate may also be influenced by its internal state (sex and age, as well as previous migratory history) and social environment. An individual’s social environment is comprised of the migratory behavior of its social group (deme culture), the length of time its social group has existed (deme history), and the size of the group (deme size). Our base model encapsulates all the information an individual possesses about itself, its social environment, and present resources, risks, and conditions at the time it makes the decision to migrate. Our three cognitive models (omniscient, perception, memory) represent different degrees and sources of information about alternative ranges, while controlling for important base model covariates. All models and the covariates they include are described in detail below and summarized in Table 1.
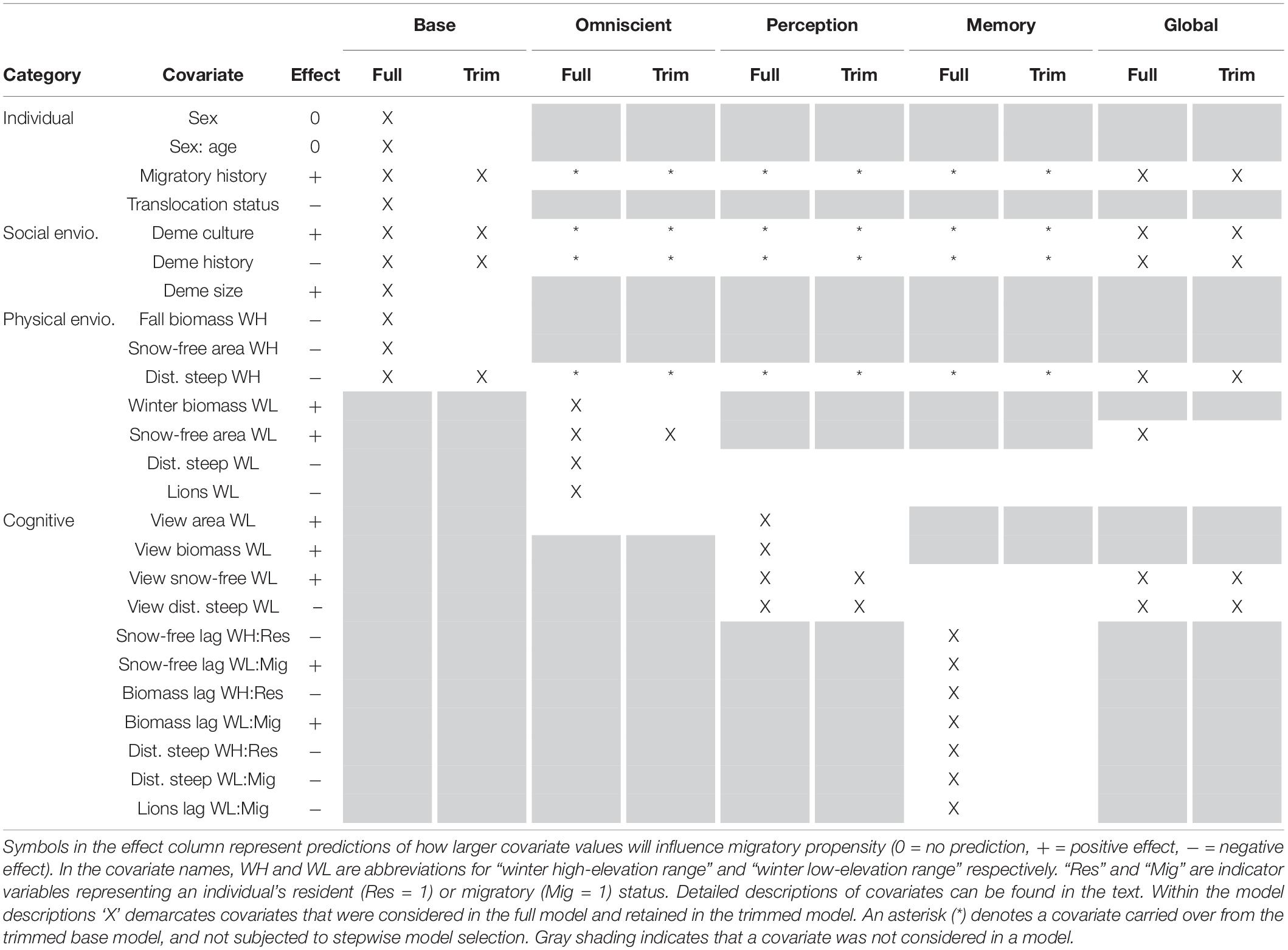
Table 1. Covariate structure of our full and trimmed base, omniscient, perception, memory, and global models.
Physical Environment Covariates
Access to resources, exposure to risks, and experienced conditions may influence migratory behavior by either attracting animals to or repelling them from a seasonal range. For each season in each sub-seasonal range (polygons delineated using the approach outlined in Section “Range Delineation”), we extracted all environmental covariates detailed below and calculated their average values. To aggregate the covariates to reflect seasonal range-level measurements (each seasonal range includes 1–35 sub-seasonal polygons), we took the area-weighted means of these averages across all sub-seasonal polygons in a range. We adjusted the spatial scaling of all means to correspond to an average value per hectare of range, and then multiplied by the aggregated range area in hectares to represent the mean seasonal quantity of the covariate.
Increased availability of forage on winter range during the winter season is a resource expected to attract SNBS, and hence positively affect their tendency to migrate. We quantified standing residual forage using the Rangeland Analysis Platform (RAP) biomass tool which combines field, satellite, meteorological, and land surface data to model vegetation productivity across the western United States (Jones et al., 2018). The RAP modeling framework estimates accumulated primary production according to plant functional type every 16 days at a spatial resolution of 30 m (Robinson et al., 2019; Jones et al., 2021). We calculated cumulative annual herbaceous biomass in kilograms per hectare (kg/ha) by summing the forb and grass biomass estimates across seasons, beginning in the spring of the preceding animal year through the winter of the focal animal year. Although the RAP biomass data suggests new growth occurs on some high-elevation winter ranges during the winter, we chose to exclude these values based on expert opinion. We did include winter biomass accumulation values for low-elevation winter range because green-up can occur as early as January given sufficient precipitation (Wehausen, 1992).
Browse comprised of woody shrubs may serve as an important nutritional resource for SNBS during the winter months when forage access is restricted by snow cover. We estimated the annual shrub cover for each sub-seasonal range using RAP’s modeled fractional cover product (Jones et al., 2018; Allred et al., 2021). Our range-aggregated shrub cover index is an estimate of the total hectares of shrub cover within a range based on an area-weighted mean shrub cover across all sub-seasonal ranges. We predicted that increases in forage biomass or shrubs on winter range would increase that range’s attractiveness because of an increase in nutritional resources.
While SNBS survive extreme winter conditions in the Sierra, extensive snow cover can impede movement and restrict access to forage and browse, increasing reliance on body fat reserves to stave off starvation (Monteith et al., 2013; Stephenson et al., 2020). We accounted for the potential role of snow-free area as an attractor to SNBS using daily downscaled 30 m fractional snow cover layers (Rittger et al., 2021) aggregated to produce seasonal average percent snow cover estimates per hectare for each seasonal range. Given that SNBS may migrate during either fall or winter seasons, and the seasons are of equal length, we averaged the range-aggregated mean percent snow cover for the fall and winter to encompass snow attributes at all times of the year when SNBS make migratory decisions. Finally, to calculate the total hectares of snow-free area within a range across the fall and winter seasons, we subtracted the mean percent snow cover from 100% to get the mean percent snow-free area per hectare, and multiplied by the total range area. We anticipated that as a range’s snow-free area increased, SNBS attraction to the range would increase because of access to nutritional resources and reduced costs of locomotion.
Physical characteristics of high-elevation terrain may facilitate snow scouring by strong winter winds, improving forage access for SNBS. Resident SNBS [i.e., those wintering on high-elevation alpine range have been observed to use large, flat windswept plateaus (Stephenson et al., 2020)]. Large plateaus provide access to forage and allow SNBS to distance themselves from precipitous terrain where avalanches and falls down icy slopes result in mortality events (Conner et al., 2018). In contrast, on low-elevation winter range, quick access to steep terrain is important to permit migratory SNBS to escape predators not commonly found at higher elevations (Spitz et al., 2020). Hence, we expect migratory propensity to be negatively associated with increasing distance to steep terrain on both the high and low-elevation winter ranges. We calculated distance to steep terrain in meters for each 30 m DEM pixel in each sub-seasonal range, where steep terrain was defined as any pixel having >31° and <70° slope (Johnson et al., 2007; Spitz et al., 2020). We averaged the distance to steep terrain across each sub-seasonal range and calculated the area-weighted mean of the sub-seasonal ranges to produce a range-aggregated distance. Since our interest was in the amount of a range close to (low-elevation winter range) or far from (high-elevation winter range) steep terrain, we multiplied linear distance in meters by range area in hectares. Given that our seasonal ranges are similar in size and geometric configuration across herd units, large values of our proximity to steep terrain metric represent land area far from escape terrain, while smaller values characterize ranges with land close to steep terrain.
Predator density on low-elevation winter range may also drive SNBS migratory decisions in avoidance of predation risk. SNBS share their low-elevation winter range with mule deer which serve as the primary food source for mountain lions (Puma concolor) in the Sierra (Dellinger et al., 2020). Lions consume migratory SNBS as an alternative prey source on common winter range, making lion depredation one of the primary causes of adult mortality in SNBS (Davis et al., 2012; Conner et al., 2018). As an index of predator abundance, we used annual counts of adult lions (≥18 months) obtained following the methods described in Gammons et al. (2021). Lion counts were conducted at a recovery-unit scale, which encompasses multiple herd units. We assumed that the index applies to each herd unit within the recovery unit, an assumption supported by the extensive home ranges of lions in the Sierra. To account for noise in total lion counts arising from changes in survey effort across time, we used a 5-year moving average to smooth counts. We replaced missing years with linearly interpolated values, except if the missing year was at the beginning or end of the time series, in which case we repeated the first or final value. We approximated predation risk by calculating the predator density of each herd unit, dividing the lion count by the area of the low-elevation winter range with the expectation that predation risk should have a negative effect on SNBS migratory propensity.
The distance SNBS travel between summer and winter range also captures a form of risk incurred moving through unfamiliar or infrequently used portions of the landscape. While high-elevation winter range is a subsection of an SNBS’s summer range, reaching low-elevation winter range requires traversing areas SNBS do not normally inhabit. The landscape matrix between summer and low-elevation winter ranges may contain land cover that SNBS avoid, snow that impedes movement, treacherous topography, predators, or lack resources. We used the straight-line distance, corrected for change in elevation (using the Pythagorean theorem), between the centers of summer range and low-elevation winter range as a proxy for travel-related migratory risk. We expected that larger inter-range distances would have a negative effect on SNBS migratory propensity.
Social Environment Covariates
The social environment, as it is defined here, encompasses all information an individual possesses about itself, its previous behavior, and intra-specific interactions with members of the same social group (deme) or herd unit.
SNBS sexually segregate into demes of rams and ewes, although both may migrate (Schroeder et al., 2010). Males remain with their mothers in ewe demes until they are 2 years of age when they are pushed out of their maternal group and join a ram deme (Geist and Petocz, 1977; Ruckstuhl, 1998; Pelletier, 2005; Schroeder et al., 2010). To account for ram behavioral variation between adolescence and sexual maturity, we included sex as a predictor in our models, with females serving as the reference category, and an interaction of sex and age for males.
An individual’s migratory history may inform its future behavior through knowledge of the location of migratory winter range (spatial memory) and previous range conditions (attribute memory), as well as its innate inclination to migrate. We included a migratory lag covariate to account for past behavior. Bighorn that displayed resident behavior in the previous observation period (within 2 years of the focal animal year, if unobserved the prior year) were assigned a value of −1. Migrants were assigned a value of 1, and individuals previously unobserved were designated 0. We expected this variable to reflect individual behavioral persistence, and hence have a positive effect on the propensity to migrate.
Individuals translocated between ranges during the summer or fall do not possess spatial or attribute memory of migratory winter range in their adopted herd unit. If migration is facilitated by an individual’s prior knowledge of the presence, location, or attributes of low-elevation winter range in their current environment, in the absence of cultural transmission of information, the behavior of translocated animals may be constrained by a lack of information. We accounted for the absence of spatial and attribute memory in translocated individuals in a novel environment by including a binary translocation covariate, with individuals receiving a value of 1 in the year they were placed on summer range in a new herd unit, and a 0 otherwise, with the expectation that this covariate will negatively affect propensity to migrate.
The behavior of an individual’s social group (deme) may dictate the behavior of the individual, particularly if group membership confers a fitness advantage (Festa-Bianchet, 1991). We classified demes through a combination of expert opinion and visual review of mapped positional data for individual bighorn relative to conspecifics and prominent landscape features. In herd units with small populations where only a single social group was present, we replaced the deme designation with the herd unit identifier. To capture group influence on individual behavior, we included a ‘deme culture’ covariate that represents the proportion of migrants in a deme during a given animal year (excluding the focal individual), with the expectation that this covariate would positively affect an individual’s propensity to migrate.
The propensity of a deme to migrate likely changes with time since establishment (typically in a previously unoccupied area) as collective knowledge of available resources, risks, and conditions is accumulated, and deme culture solidifies. We indirectly accounted for this temporal shift by including an inverse time on the landscape covariate for each deme, which we will refer to as ‘deme history.’ We recorded the first year a deme was detected as part of our deme classifications. Any demes present in our reference year, 1978, were assigned a value of 0. Demes emerging later than 1978 were assigned a value equal to the difference between the year they were first documented and the reference year. We anticipated that the length of time a deme has existed on the landscape would be positively correlated with member propensity to migrate due to the cultural transmission of knowledge (Jesmer et al., 2018; Lowrey et al., 2020).
Theory suggests that deme size (equivalent in our systems to local population density) should increase member migratory propensity, as it increases competition for forage (primarily on high-elevation winter range) and decreases per-capita predation risk (primarily on low-elevation winter range) (Avgar et al., 2020). Counts of female SNBS are conducted annually, although the season (winter vs. summer) during which the surveys take place varies between herd units and years. Winter counts are pre-birth pulse surveys and summer counts are post-birth pulse surveys, meaning they are not directly comparable because counts differ by the number of female lambs, assuming adult female mortality is negligible (Johnson et al., 2010b). We adjusted all winter counts to approximate summer counts by adding half of the lamb count (assuming a 50/50 sex ratio given that lambs are not identifiable as male or female) to the female winter count and shifting the count year to reflect the subsequent animal year (because our animal year begins in July). To account for noise arising in total female counts due to changes in survey effort across time, we used a five-year moving average to smooth counts. We replaced missing years with linearly interpolated values, except if the missing year was at the beginning or end of the time series, in which case we repeated the first or final value. To create our deme size index, we divided our smoothed annual female counts for each herd unit by the number of demes present during a given year. We assumed all demes were of equal size because we did not have positional data for all females in a herd to assign them to a deme. We expected deme size to have a positive effect on the propensity of its members to migrate.
Modeling
We built a suite of four binomial generalized linear mixed models (‘base,’ ‘omniscient,’ ‘perception,’ and ‘memory’; detailed below) using Laplace approximations of maximum likelihood in the R package lme4 (Bates et al., 2015). We conducted all analyses described in the methods in R (Version 4.1.1, R Core Team, 2021), unless otherwise noted. Our response variable was the binary classification of whether a given individual migrated in a given year, with our random effects accounting for herd- and individual-level variability in the model’s intercept (the basal migratory propensity). Our model inputs included 1,298 animal years of data, which encompassed 460 unique individuals across 14 herd units. We centered the means of all continuous population-level covariate values at 0 and scaled them in units of standard deviation from the mean to improve convergence and facilitate interpretation. The physical environment experienced by an individual is characterized by cumulative biomass, snow-free area, and distance to steep terrain. We excluded shrub cover and migratory distance covariates from all four of our models because their effects were highly correlated with other covariates that we deemed of greater biological importance in our study system.
We trimmed all four models using stepwise AIC model selection. Beginning with the full models, we excluded a single covariate during each iterative model run and re-calculated the AIC score of the model with a reduced fixed effects structure. The reduced model that received the lowest AIC score served as the starting model for the next step. We continued the stepwise reduction until all remaining models scored at least two △AIC points higher than our starting model for the step, which we designated our best model.
Base Model
Our base model fixed-effects structure captures information an individual possesses about itself, its social environment, and its present physical range at the time it makes the decision to migrate. As such, this base model excludes the effects of perceptual or memorized information about the low-elevation winter range, and hence serves as an appropriate null model. The base model includes sex, an interaction of sex and age, migratory history, and translocation status as covariates representing the state of an individual (Table 1). The social environment experienced by an individual is comprised of deme culture, deme history, and deme size covariates. We assumed that at the time it makes the decision to migrate, an individual is located on its high-elevation winter range (a subsection of its summer range). Therefore, the individual has perfect information about the physical environment on high-elevation winter range at that point in time (i.e., the fall season). After trimming our base model, we carried the remaining fixed effects (and the random-effects structure) forward in our three other models to reflect information an individual possesses in the absence of our cognitive processes of interest. The trimmed base model covariates were not subject to stepwise model reduction in any of the cognitive models.
Omniscient Model
The omniscient model represents a common, but likely unrealistic, ecological assumption – that individuals have perfect knowledge of their environment (near and far) when making decisions about habitat use. While SNBS in our base model had perfect knowledge of the physical environment on high-elevation winter range, individuals in the omniscient model also had perfect knowledge of the low-elevation winter range environment they would experience as migrants in the coming winter. Our omniscient model thus includes all covariates from the trimmed base model, in addition to cumulative biomass, snow-free area, proximity to steep terrain, and predator density on low-elevation winter range for the focal animal year (Table 1).
Perception Model
Sierra Nevada bighorn sheep likely do not have perfect information about the conditions on low-elevation winter range when making migratory decisions, but may possess partial information based on the amount of migratory range they can see. While little is known definitively about the eyesight of bighorn sheep, their visual acuity is much to the chagrin of North American hunters who have compared it to a man aided by eight-powered binoculars (Geist, 1971). Bighorn have been observed to react to predators at distances of over 1 km (Geist, 1971). Bighorn also forage more efficiently in habitats with greater visibility, presumably because these landscapes permit early sighting of predators (Risenhoover and Bailey, 1985; Valdez and Krausman, 1999). While bighorn are traditionally considered to be reliant on their vision (which may also reflect a human-centric bias, as we are visual creatures), auditory and olfactory cues may also influence SNBS migratory decisions. However, non-visual sensory cues are difficult to quantify retroactively and cannot be meaningfully interpreted on the seasonal timescale of our data. Although our perceptual model only explicitly accounts for visual information available to SNBS, it generally reflects partial knowledge of the present environment, encompassing non-visual cues indirectly.
We quantified the visibility of low-elevation winter range from the summer range in each herd unit using ESRI’s ArcMap Viewshed Analysis tool (ESRI, 2020) (Figure 2). The tool calculates the visibility of cells in a raster surface from a designated point location given line-of-site barriers imposed by topographic relief, as captured by a digital elevation model (DEM). We assumed that land cover did not restrict line-of-site, which is reasonable in our system given the low profile most vegetation and steepness of the terrain. From our summer range sub-seasonal polygons, we randomly sampled observation points at a density of one point per hectare (Figure 2). Random observation points characterize locations individuals could occupy on their summer range and look down-slope at potential low-elevation winter range. We assumed the eyeline of an SNBS was 1.75 m above the ground, ArcGIS’s default observer height, because we did not have a good estimate of true eyeline height for bighorn. Although an eyeline of 1.75m may overestimate visibility given that SNBS stand, on average, 1 m at the shoulder (CDFW, 2021), the bias is consistent across herd units and should not influence our final results. It is also likely that bighorn use local high-points for visual observation which our overestimate of eyeline may represent. From a random observation point, we assigned all raster cells in our low-elevation winter range polygons a binary classification of 1 (seen) or 0 (not seen). We repeated this classification procedure for each observation point, and calculated a total visibility score for each raster cell by summing the binary classifications. We then summed the visibility score of all raster cells across an entire seasonal range. To account for differences in range size, we created a visibility index that scales between 0 and 1, with 1 indicating all raster cells on low elevation range were visible from every observation point, and 0 representing no visibility of raster cells from any observation point. We calculated our visibility index by dividing a range’s total visibility score (sum of all cell visibility scores) by the total number of raster cells in a range multiplied by the total number of observed points (representing the possibility that every raster cell is seen from every observation point). The visibility index captures the relative variation in visibility between herd units, but given our numerous assumptions may not accurately represent what SNBS actually see. We expected visibility to have a positive effect on migratory propensity as a main effect (SNBS more likely to migrate when they can see more of the low-elevation range), but also to enhance the effects of other low-elevation range attributes.
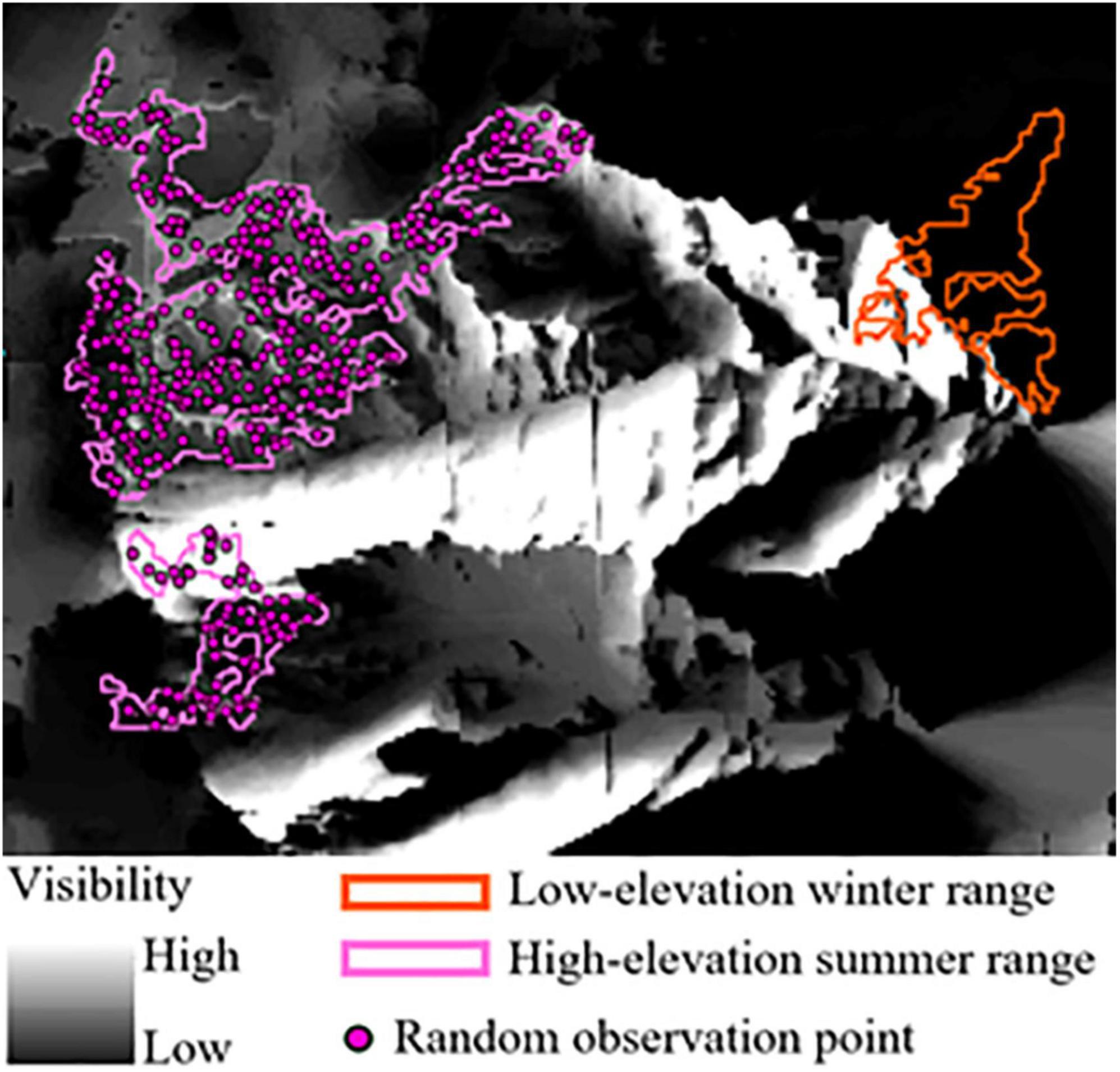
Figure 2. Viewshed analysis performed on a subsection of the Olancha Peak herd unit. Pink high-elevation summer range polygons encompass a series of random observation points on which a hypothetical bighorn stands, looking out over the landscape in all directions (evaluating 360 degrees of visibility). The landscape is represented as a raster, with each raster cell receiving a binary designation of 1 (seen) or 0 (not seen). This process is repeated for every random point, with raster cells being assigned a value that represents the total number of 1 s and 0 s accumulated across all observations. Higher totals (more 1 s) represent greater visibility and are depicted in colors closer to white on the gray scale raster. Lower totals (more 0 s) indicate low visibility and are represented by colors closer to black. Bighorn on the summer range looking down at the orange low-elevation winter range polygon have good potential visibility (unobstructed by topography) of attributes on the left-most edge of the range, but visibility declines moving toward the right-most edge.
In our perception model, the covariates characterizing the low-elevation winter range during the migratory window – cumulative biomass, snow-free area, and proximity to steep terrain – are multiplied by the visibility index (before scaling and centering) to represent visual information about the migratory range available to SNBS at the time they make a migratory decision. We also included (in addition to the trimmed base-model covariates) a range visibility covariate for each herd unit, taking the product of the herd unit visibility index and the area of the low-elevation winter range in hectares to capture the importance of seeing potential migratory range in the absence of specific information characterizing range attributes (Table 1).
Memory Model
While our first three models sought to capture an individual’s knowledge of the present and/or future physical environment, individuals may also remember attributes of the ranges where they overwintered previously, and this information is expected to influence their present migratory behavior. We modeled the influence of attribute memory on migratory behavior by including interactions between last year’s range-specific environmental covariates on either high-elevation (for last year’s resident) or low-elevation range (for last year’s migrants), and migratory history indicator variables. Animals translocated over summer/fall were assumed to rely on attribute information from their natal range. Because this model formulation necessitates excluding animal years where the migratory status of the individual in the previous year was unknown, we fit the memory model using a reduced data set (779 animal years). The memory model includes lagged cumulative biomass, snow-free area, and proximity to steep terrain covariates for both high and low-elevation winter range. We also included a lagged predator density covariate on low-elevation winter range, as well as all covariates from the trimmed base model (Table 1). We trimmed the memory model by removing the high and low-elevation representations of the same environmental covariate in pairs because each pair represents a single hypothesized behavioral driver. We expected that memory of a range would increase range affinity if the conditions experienced last year were good, or encourage the opposite behavior if experienced conditions were poor.
Global Model and Model Goodness of Fit
We then combined the covariates from the trimmed base, perception, omniscient, and memory models into a global model which we again trimmed using stepwise AIC model selection to determine which cognitive processes most strongly influenced migratory behavior (Table 1). We calculated conditional and marginal pseudo-R2 goodness of fit statistics for all trimmed models using the MuMIn package (Barton, 2020). To evaluate the predictive capabilities of the trimmed models, we split our data into five folds based on individual ID, trained our trimmed models using four folds of the data, tested our trained models on the remaining fold, and fit ROC-AUC curves in the R package pROC (Robin et al., 2011) to both the trained and tested models. A higher ROC-AUC value (range 0–1, with 0.5 representing random chance) represents better model performance. We repeated this process until each fold had served as both testing and training data and reported the mean ROC-AUC values across all training and testing iterations. Model predictions on test data arise from the population-level estimates of the fixed effects because the testing data is an out of sample prediction containing new levels of the random effect (unique individuals) not encountered during model training.
Results
The annual percentage of migratory SNBS across the species’ range varied from 56 to 100%, with a mean of 81.6% (SD = 13.6%) (Figure 3A). The percentage of individuals that changed behavioral strategies between subsequent years ranged from 0 to 39.3% with a mean of 15.5% (SD = 10.1%) across years (Figure 3B). We note here that while our study sought to address the drivers of migratory behavior rather than migratory switching, Spitz (2015) considers the latter.
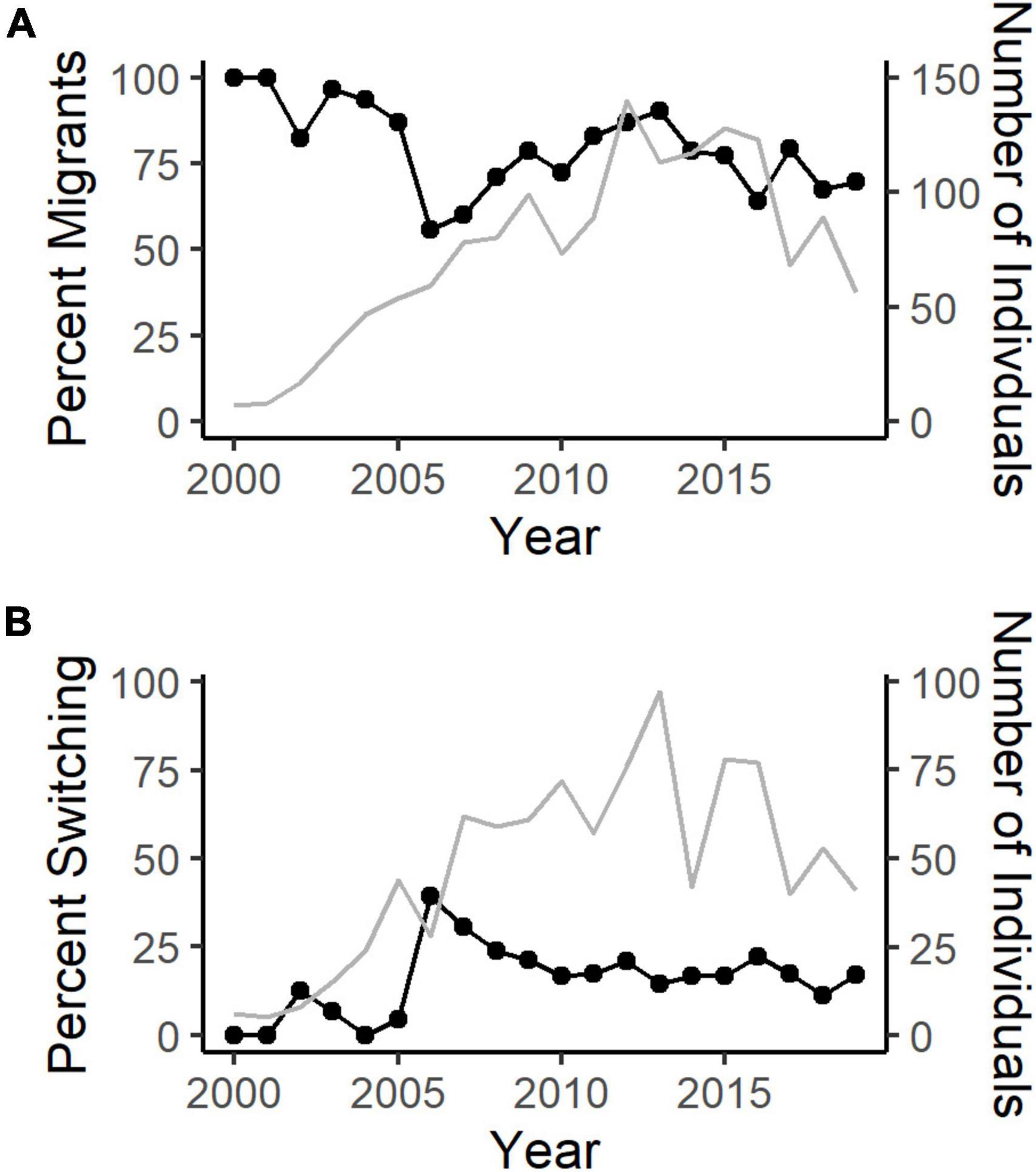
Figure 3. (A) The annual percentage of migratory (detected below elevations of 2,850 m) Sierra Nevada bighorn sheep from 1998 to 2020 based on capture, ground survey, VHF, and GPS telemetry data (black line). The number of individual bighorn monitored each year varies with time and population size (gray line). (B) The annual percentage of Sierra bighorn that switch behavioral strategies (previous resident, present migrant or previous migrant, present resident) between years (black line). The number of individual bighorn with longitudinal data (migratory classification in the previous and present year) varies with time and population size (gray line).
The base model covariates retained after stepwise model selection included migratory history, deme culture, deme history, and distance to steep terrain on high-elevation winter range. Individuals that migrated in the recent past were more likely to migrate in the focal animal year. The propensity of individuals to migrate increased as the proportion of migrants in an individual’s social group increased. Individuals that were part of demes with longer histories on the landscape were more likely to migrate than those belonging to ‘younger’ demes. Lastly, increasing distance to steep terrain on high-elevation winter range, capturing the presence of plateaus, had a negative effect on SNBS migratory propensity. These effects all agree with our a-priori expectations based on the ecology of the system.
All cognitive models included the four retained base covariates (to capture variability unexplained by cognitive processes) and the suite of cognitive covariates kept after stepwise model reductions. The only covariate retained in the trimmed omniscient model was the snow-free area on low-elevation winter range. As expected, SNBS were more likely to migrate as the snow-free area on their migratory range increased. The trimmed perception model included visible snow-free area and visible distance to steep terrain on low-elevation winter range. SNBS were more likely to migrate if they could see a greater percentage of snow-free area and shorter distances to escape terrain on their potential migratory range. No cognitive covariates were retained in the trimmed memory model (in addition to the base covariates).
The results of our trimmed global model indicate that perception is the most important cognitive process underlying SNBS migratory decision-making. The omniscient snow-free area covariate was eliminated from the global model during the stepwise model reduction process, resulting in identical trimmed global and perception models. Overall, the trimmed global model reveals that SNBS are more likely to migrate if they recently migrated (Figure 4A), if their social group includes a high percentage of migrants (Figure 4B), and if they are able to see larger amounts of snow-free area on low-elevation winter range (Figure 4E). SNBS are less likely to migrate if they are part of a social group that has existed for a shorter period of time (Figure 4C), if they have access to high-elevation habitat far from steep terrain (Figure 4D), and if they observe a lack of habitat close to steep terrain on potential migratory range (Figure 4F). Lastly, innate differences between individuals explain a considerable amount of residual variability in migratory propensity, as evidenced by the magnitude of our random effects (Table 2).
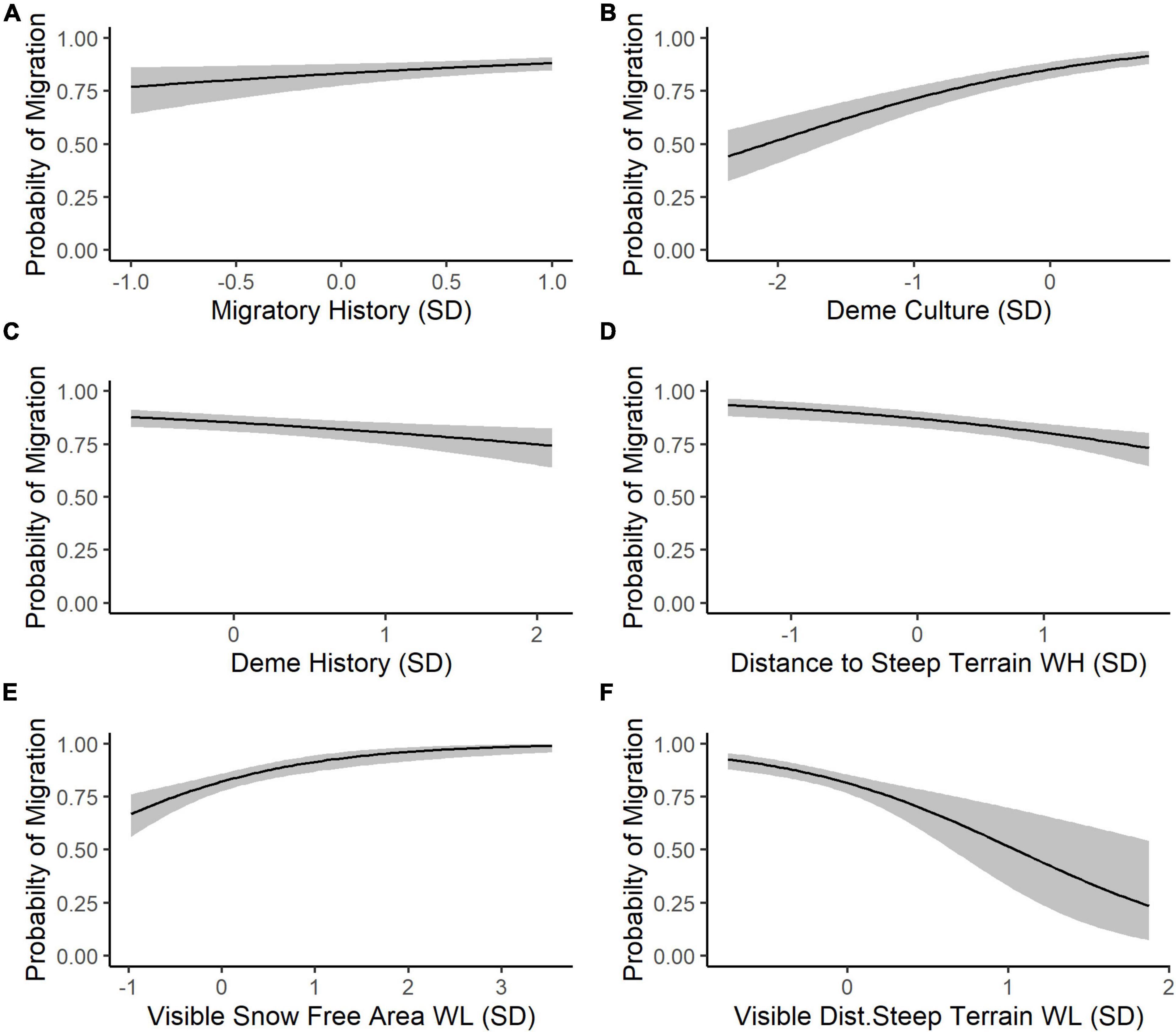
Figure 4. (A) The predicted probability (black line) that an individual SNBS will migrate (with 1 representing certainty that migration will occur) given an individual’s migratory history represented in units of standard deviation (SD) away from mean 0 on the odds ratio scale (back transformed from the logit scale) with the values of all other covariates held at their population mean. Predictions are made only using population-level fixed effects based on coefficient values from our trimmed global model to allow for out of sample predictions (random effect variance is excluded). The gray ribbon represents a 95% confidence interval around the prediction. We allowed each covariate to vary in turn while holding all others at their population mean so (B) captures the probability of migration based on the proportion of migratory individuals in the subpopulation, (C) depicts the probability of migration given the inverse of the length of time that an individual’s subpopulation has existed on the landscape (larger values represent a shorter time on the landscape), (D) represents the probability of migration given the distance to steep terrain on high-elevation winter range, (E) captures the probability of migration based on visible, snow-free area on low-elevation winter range, and (F) shows the probability of migration given the visible distance to steep terrain on low-elevation winter range.
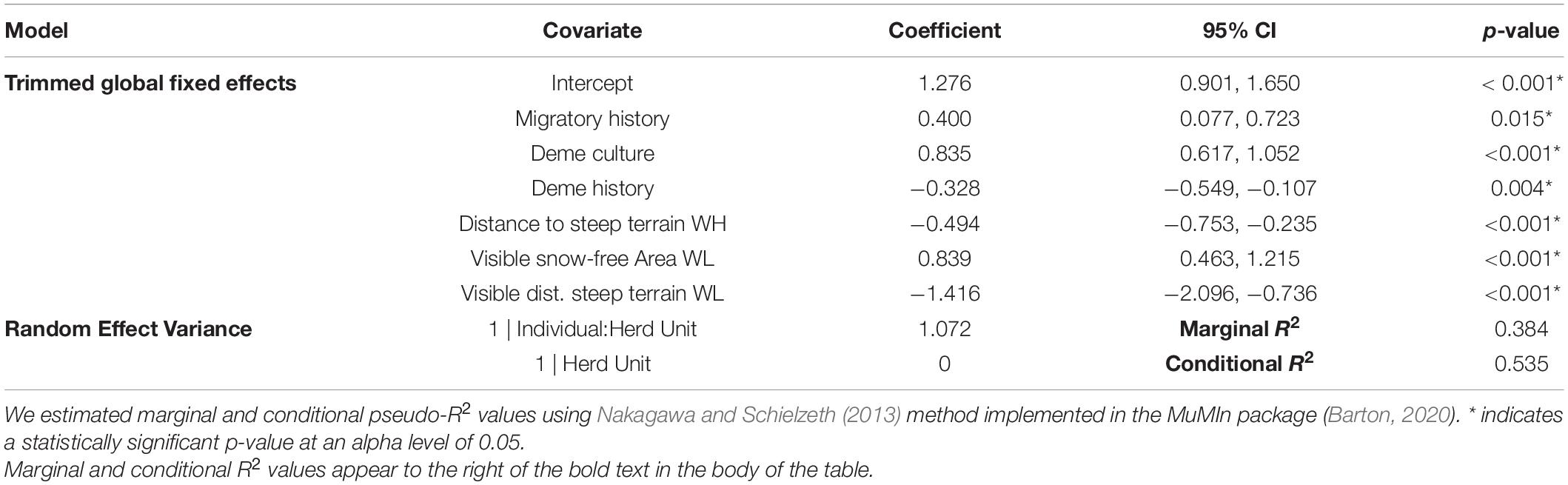
Table 2. Binomial linear mixed effect model results for our trimmed global model, predicting migratory behavior (0 = resident, 1 = migrant) of Sierra Nevada bighorn sheep using a suite of base and cognitive (perceptual) fixed effects and a (1| Herd Unit:Individual) random effect structure in the the R package lme4 (Bates et al., 2015).
A comparison of model performance and goodness of fit statistics across our suite of trimmed models supports perception as the cognitive process that explains the most migratory variability in SNBS (Table 3). However, comparing the marginal R2 values of the perception and base (excluding cognitive covariates) models, cognitive covariates only explain ∼4% of the variation in SNBS migratory behavior compared to ∼34% captured by the base model covariates (Table 3). While our study highlights the influence of cognitive processes on migratory decision making in SNBS, an individual’s previous experiences, and present social and physical environment explain the preponderance of behavioral variation. All models had strong out-of-sample predictive capacity (Table 3).
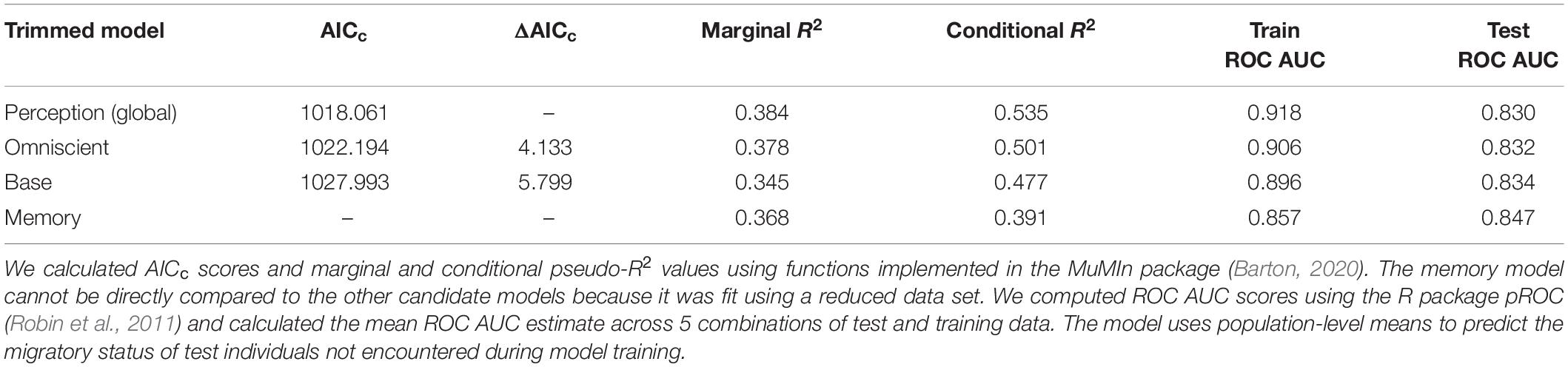
Table 3. Model performance (AICc) and goodness of fit statistics (pseudo-R2 and ROC AUC scores) for the trimmed base and cognitive models.
Discussion
Our study is the first to evaluate cognitive processes that underpin migratory decision-making in a population of short-distance, altitudinal migrants. Our results demonstrate that short-distance altitudinal migration of SNBS is, in part, a behavioral response to an individual’s perception of present environmental conditions on available, low-elevation (migratory) range. An individual’s sensory informational state (perception model) explained more variation in migratory behavior than memorized information (memory model) or perfect environmental knowledge (omniscient model), illustrating that individuals make migratory decisions with only partial information about their target range. While the addition of cognitive processes to our base model improved model performance, the availability of high-elevation winter habitat, an individual’s social environment, and intrinsic factors explained the greatest amount of behavioral variation within the population. We refer to SNBS as perceptually-informed migrants to reflect the limited influence (or our limited ability to detect the influence) of cognitive drivers on migratory decision-making. We believe that our findings are encouraging with respect to the future persistence of SNBS. Perceptually-informed migration may result in greater behavioral plasticity in response to changes in resource phenology and distribution arising from global climate change, while migrants reliant on prediction of future environmental conditions given past experience may exhibit a lagged response to climatic variability.
Perception and Memory
Perception of present conditions on low-elevation migratory range, rather than memory of past conditions or omniscience, was the strongest cognitive predictor of short-distance altitudinal migration in SNBS. SNBS migration occurs over large changes in elevation, but short geographical distances. Steep elevational gradients in the Sierra likely favor perceptually-informed migratory decisions because visual range increases with altitude. Large changes in elevation also give rise to a condensed ecological gradient where resources, risks, and conditions shift drastically over short geographic distances (Lomolino, 2001; John and Post, 2021). Unlike long-distance migrants tracking gradual resource changes over considerable distances, SNBS can likely see a large proportion of the ecological gradient spanning their migratory route to destination range, and assess the profitability of movement. While our model may not accurately represent what bighorn can see, it captures partial sensory information about the present state of alternative ranges, which may include sensory inputs (smell and sound) that we did not explicitly represent. Our findings support our expectation that perception would be the cognitive mechanism favored by short-distance migrants because it is biologically realistic, unlike omniscience, and matches the timing of movement with changes in the environment.
The spatial scale of migratory movement limits the cognitive mechanisms available to support migratory decision-making. By our definition, long distance migrants cannot use perception of alternative range to make a migratory decision because they are moving to a location outside of their perceptual range. Long distance migrants may perceive that the environment is unfavorable on their present range and decide to migrate, but they are being pushed from their current location rather than pulled to a more favorable location, like the SNBS. Long-distance migrants may also use perception to follow a resource gradient to an alternate range (Holdo et al., 2015; Merkle et al., 2019). However, long-distance migrants cannot decide to move to a specific target destination without invoking non-perceptual cognitive processes. Individuals could rely on memory to return to a range they visited previously (Bracis and Mueller, 2017; Abrahms et al., 2019; Merkle et al., 2019), cultural transmission of knowledge from past migrants (Jesmer et al., 2018; Lowrey et al., 2020), or an innate encoding of the migratory destination (Mouritsen, 1998; Kashetsky et al., 2021). None of these cognitive mechanisms provide long-distance migrants with information about the present conditions on their destination range. Global climate change causes phenomenological shifts in events like green-up that may disproportionately impact long-distance migrants because they do not possess the information to match the timing of their movements to environmental changes on their target range.
The spatial and temporal predictability of resources, risks, and conditions may also determine which cognitive mechanisms are used by migrants. Memory-informed migrants make predictions about the present/future state of their target range based on past experience (Bracis and Mueller, 2017; Abrahms et al., 2019; Merkle et al., 2019). Effective prediction relies on consistency in the location and timing of environmental variation. High-altitude environments along steep elevational gradients, like those found in the Sierra, are not characterized by predictable variation. Birds, frogs, skinks, snails, and freshwater shrimp have all been found to adjust their life history strategies at high altitudes, producing fewer, but larger offspring partially in response to increased climatic unpredictability (Badyaev and Ghalambor, 2001). Perceptually-informed migration may be an adaptive cognitive response to environmental variability within and between years. The Sierra has the highest variability in snow events of any mountain range in the western United States, low correlation of interannual snowpack, and no discernible directional trend in snow accumulation over a 71-year time series (Cayan, 1996). If the SNBS attraction to snow-free areas observed in our system was a memory-informed response to the previous year’s snow cover, individuals would likely experience a mismatch between the expected timing and location of snow-free area and present snow conditions. The timing of the first major precipitation event that drives green-up on low-elevation winter range also is highly variable between years, ranging from early autumn to mid-winter (Wehausen, 1992). If migrants depart for low-elevation winter range in advance of green-up, they face increased predation risk and are not compensated with greater forage availability. Migration in response to a past state of the environment confers fewer fitness advantages than a behavioral response to present environmental cues when environmental variability is unpredictable.
Variation in the timing of SNBS migration between years (Spitz et al., 2018) suggests that SNBS are responding to inter-annual environmental variability based on proximate cues. The existence of vacillating migration in SNBS, where individuals undertake 2–4 movements between seasonal ranges during the migratory window (Denryter et al., 2021b), also suggests that migration is a response to observed intra-annual variability. Memory may be the cognitive mechanism that underlies migration in predictable environments, while perception facilitates migration when the timing and location of resources, risks, and conditions is variable.
Habitat
An individual’s migratory status is partially determined by the quality and availability of high and low-elevation winter habitat. Our results corroborate the findings of Spitz et al. (2020) who observed that habitat selection by migrant and resident SNBS was predictive of the local prevalence of migratory behavior. Similar to our study, Spitz et al. (2020) found that resident bighorn selected habitats twice as far from steep terrain as migrants. While our top model included a perceptual contextualization of low-elevation range attributes, in concurrence with Spitz et al. (2020) we found that migration is more prevalent in habitat with less snow near escape terrain. However, Spitz et al. (2020) also observed that forage availability on both high and low-elevations ranges helped to explain the frequency of migrant and resident behaviors. They concluded that migrant SNBS prioritize access to forage at the cost of increased predation risk, while residents minimize predation risk but incur increased energetic costs imposed by limited access to forage and greater thermoregulatory demands (Johnson et al., 2013; Spitz et al., 2020; Denryter et al., 2021a). While our results suggest that avoidance of snow may be of greater importance for migrants than access to forage, discrepancies between studies may result from our quantification of vegetative biomass. We used an annual measure of forage biomass on each range. Spitz et al. (2020) did not have access to these new remotely sensed products to incorporate temporally dynamic habitat attributes, and instead relied on 11-year averages of forage and snow cover. Observed habitat selection in response to long-term averages of temporally variable covariates may indicate that bighorn migration has some dependence on memory that we did not capture looking at range conditions experienced only in the previous year. In long-lived species like SNBS, complex, non-Markovian representations of memory merit further evaluation.
One habitat attribute that we did not quantify, but warrants further exploration is connectivity between high and low-elevation ranges. The distance covariate included in our model does not capture resistance to movement based on land cover attributes. Mid-elevations in the Sierra are characterized by pinyon-juniper scrub and coniferous forests. SNBS avoid forested landscapes presumably because tree cover conceals predators and increases predation risk. Contiguous mid-elevation forest cover may restrict migratory movements. However, the migratory corridors of Sierra sheep are difficult to delineate because individuals can move between seasonal ranges within a few hours. The temporal resolution of GPS fixes from collars is often too coarse to capture SNBS mid-migration. Establishing a measure of migratory connectivity in our system is an important step toward understanding the residual variation in migratory behavior between herds.
Culture
Our results show that membership to a social group with a large proportion of migrants is the strongest predictor of migratory behavior in SNBS, but time since establishment of the group determines the group’s propensity to migrate, in line with the findings of Jesmer et al. (2018) and Lowrey et al. (2020). While time since establishment may also capture behavioral variation resulting from changes in group size and experienced density, the independent group density covariate in our analysis did not receive support. The increase in migratory propensity over time has been hypothesized to arise from social learning, a process grounded in shared memory across generations (Jesmer et al., 2018). When SNBS colonize or are translocated to a new area of the landscape, they possess no knowledge of migratory ranges or routes accrued from experience on their new range, other than a general awareness of previously overwintering at a high or low elevation in their natal range (migratory history). As individuals explore new habitat, they accumulate information about their surroundings, which if retained, becomes memory (Fagan et al., 2013). The strong group cohesion of SNBS permits dissemination of information within social groups between individuals. The group’s collective knowledge of the landscape is a compilation of individual knowledge, and increases over time with the contribution of subsequent generations. Social learning provides a form of collective memory that is not represented in our individualistic characterization of memory.
While attribute memory did not inform SNBS migratory decision-making, the importance of a deme’s history on the landscape (representative of collective memory) suggests memory is necessary to support migration. Similar to Bracis and Mueller (2017), Abrahms et al. (2019), and Merkle et al. (2019) who found memory underpinned navigation of migratory mule deer, whales, and zebras, respectively, SNBS may rely on memory to navigate to low-elevation winter range, particularly over longer geographic distances. Although we did not explicitly consider drivers of the navigation process, navigation is implicit in our model. We used an individual’s migratory status in a given year as our response variable which encompasses both halves of the migratory process – decision-making and navigation. By treating a completed migration – a realization of a migratory decision – as representative of a migratory decision, we don’t account for individuals who decide to migrate, but fail to navigate to an alternative range. However, it is possible that SNBS migration in a novel landscape requires numerous failed attempts before enough knowledge is accumulated to permit successful navigation to low-elevation winter range. Translocated bighorn with an abbreviated history on the landscape are less migratory than native populations, a behavioral trend attributed to an absence of collective memory (Lowrey et al., 2020). Given the prevalence of translocation as a management strategy in our system to establish SNBS in historic habitat, further study of the importance of memory for navigation between migratory ranges is warranted.
We sought to represent deme culture by quantifying the proportion of migrants in each social group in the absence of the focal individual, which may serve as a proxy for accumulated knowledge or opportunities for social learning if each migrant individual has previous experience. However, an increase in an individual’s probability of migration as the migratory propensity of the group increases may simply represent the importance of remaining part of a group. Predator vigilance is important for SNBS survival, particularly while foraging, and being part of a group with “many eyes” confers a safety advantage (Lima, 1995; Rieucau and Martin, 2008). Group membership also reduces predation risk through confusion and dilution effects (Pulliam, 1973; Krause and Ruxton, 2002). While there is limited predation risk on high-elevation range during the winter, mountain lion predation events on low-elevation range are the greatest source of adult mortality for SNBS (US Fish and Wildlife Service, 2007; Johnson et al., 2013; Gammons et al., 2021). Migration to low-elevation range may become safer as the number of migrants increases for which proportion of migrants serves as a proxy. However, our deme culture covariate captures all factors common to a migratory or resident portion of a social group that explain some behavioral variation. The cultural effect size from our analysis should be interpreted with caution.
In agreement with Lowrey et al. (2020), our findings indicate that individual variability and the social environment are stronger determinants of migratory behavior than attributes of the physical environment. Our base model covariates capture 47.7% of the variability in our system and the addition of cognitive mechanisms only slightly improves model performance (pseudo-R2 0.535). When translocating animals to establish new herds or augment existing populations, wildlife practitioners should be mindful of who they are moving if the emergence of migratory behavior is a priority. The identity of a translocated individual and migratory proclivity of its new deme are stronger determinants of migratory behavior than habitat. However, a limited understanding of the structure and composition of the social groups in our system means that changes in social dynamics when adding or removing individuals to a herd are unpredictable. If a single dominant individual is responsible for initiating migration, the behavior of a group may change with augmentations made to the social hierarchy. Translocated individuals may adopt or disrupt the social hierarchy of their new herd. Improving our understanding of SNBS social structure and migratory initiation will allow resource managers to optimize their selection of individuals for translocation to achieve desired behavioral outcomes.
Individual History
Our results agree with the findings of Spitz et al. (2018) that migratory behavior in SNBS is highly plastic. Compared to Spitz et al. (2018), we detected a higher frequency of migratory behavior (with an average of 81% vs. 63% of individual SNBS migrating each year) and a lower facultative switching rate (with an average of 15.5% vs. 25% of individuals changing migratory strategies between years). Discrepancies between studies likely arise from differences in the types and quantity of data used, as well as migratory classification techniques. Spitz et al. (2018) analyzed 262 animal years of GPS data collected between 2005 and 2016. Our study incorporated 1,298 animal years of data spanning from 1999 to 2020. We integrated multiple sources of positional data into our analysis including GPS, VHF, visual survey and capture information. Spitz et al. (2018) also used elevation-based net squared displacement to classify migration and chose to categorize individuals that exhibit vacillating or abbreviated migration (Denryter et al., 2021b) as residents. These same individuals are classified as migrants in our study because of their presence below the elevational cutoff during the migratory window. We may have detected a lower migratory switching rate than observed previously because non-traditional migrants who may more readily adopt conventional migratory strategies are already classified as migrants in our study. Our classification would indicate that no behavioral switching occurred. It should be noted that embedded in our data is an inherent migratory bias because visual counts and captures during the migratory window most often take place on low-elevation winter range where all observed individuals are migrants. While this may over-represent the prevalence of migratory behavior in the population relative to GPS-based classifications, we believe it does not affect our ability to quantify the drivers of migration.
The migratory switching rate of SNBS is one of the highest known among ungulates (Spitz et al., 2018) and this behavioral plasticity likely arises because migratory decisions are partially informed by perception of conditions on alternative ranges. SNBS may switch between migrant and resident behaviors more often than other taxa because they possess real-time information about alternative ranges that allows individuals to assess the profitability of migration relative to residency on their current range. SNBS should choose to occupy the range that affords the greatest fitness gains if the risk incurred in moving between ranges is negligible (Fryxell and Sinclair, 1988; Mysterud et al., 2011; Spitz et al., 2018). Given the short distance that SNBS travel between ranges, migration may reflect a comparative evaluation of high and low-elevation range quality based on perceptual information. Repeated comparative evaluations of habitat quality during the migratory window may explain the behavior of vacillating migrants. Interannual variation in range quality may also provide an alternative explanation for low-elevation range abandonment observed in SNBS in the 1980s and attributed to predation (Wehausen, 1996). In comparison, long-distance migrants, like caribou (Rangifer tarandus), have been observed to exhibit strong range fidelity even in habitat that has undergone extensive anthropogenic alteration (Dalerum et al., 2007). Given that caribou rely on spatial memory to navigate the landscape (Avgar et al., 2015), it is likely that this species’ lack of behavioral plasticity in selection of migratory range is due to the predominance of memory as a cognitive driver. Sawyer et al. (2019) also found an absence of migratory plasticity among mule deer, a species reliant on spatial memory to facilitate migration (Merkle et al., 2019). The asymmetry of behavioral plasticity between cognitive drivers means that perceptually-informed migrants will be better able to tolerate the increased climatic variability that accompanies global climate change (assuming no significant loss of habitat). However, additional research is necessary to validate our hypothesized drivers of migratory switching in SNBS because the scope of our study is limited to the drivers of migration.
Our study recorded high migratory switching rates in SNBS in addition to behavioral inertia. Individuals that migrated the previous year were more likely to migrate in the present year. Spitz et al. (2018) also noted that individuals only changed migratory strategies approximately every 4 years. While this periodicity may be driven by environmental factors, it may also be a consequence of an individual’s physiological condition or reproductive status. Some species reproduce and migrate in alternate years to compensate for the energetic demands of reproduction (Morrison and Bolger, 2012). Lactating SNBS ewes enter the winter season with lower body fat reserves than females who lost a lamb early or did not reproduce (Stephenson et al., 2020). Lactation may necessitate migration given the high energetic cost of residency on high-elevation range because of a lack of forage and increased thermoregulatory demands. Presently, we have little information on body condition and lambing status that could be explored in relation to migratory behavior for SNBS. However, those data, when available, should be incorporated into future studies of migratory drivers.
Management
Predicting when migratory behavior will arise in novel landscapes is a challenge for wildlife practitioners as they work to re-establish migratory populations via translocation in unoccupied native range. The frequency of facultative migration is predictable in species where perception and habitat attributes explain significant behavioral variability. For SNBS, resource managers can apply the perception and physical environment covariate predictions from our model to areas of the landscape where they are considering translocation to estimate the probability of migratory behavior emerging in an established herd. However, culture and characteristics of individuals are better predictors of migratory behavior than perception or attributes of the physical environment. Known migrants should be placed with individuals from the same social group into habitat with characteristics corresponding to migratory behavior to maximize chances of behavioral continuity in a new environment, if desirable. Re-establishing “lost” short-distance migration (Wehausen, 1996) will be easier to accomplish in species like SNBS, where perception is the primary cognitive mechanism influencing migratory decisions, because individuals (or social groups) are not reliant on prior knowledge of where and when to move. It may prove more challenging to re-establish long-distance migration to low-elevation winter range on the eastern slope of the Sierra in populations of SNBS translocated to the western slope. The Sierra crest prevents SNBS reliance on perceptual cues to evaluate range quality on the opposite slope and collective memory of this historical migration is absent in translocated populations. Environmental drivers and trial and error may be important for recovering migratory movements that span the Sierra crest. Persistent changes in the resources, risks, and conditions that drive migratory behavior, if detectable through a sensory-perceptual mechanism, may still lead to the disappearance of migration in perceptually-informed species. The disappearance of migration is of concern if it decreases a species’ fitness or results in the loss of collective memory important for future migratory movements.
Conclusion
Perception is indicated as the primary cognitive mechanism underlying short-distance altitudinal migratory decisions in Sierra Nevada bighorn sheep. Perceptually-informed migrants are behaviorally plastic and able to respond to changes in resource phenology and distribution in unpredictable environments. Migrants that depend on perception will likely be more resilient to climatic unpredictability arising from global climate change than species that must rely on past experience to predict future conditions on migratory range. Perceptually-informed migration allows for the possibility of predicting the emergence of migratory behavior in novel landscapes based on habitat attributes that enhance or limit sensory perception. However, accurate predictions will require a better understanding of the interplay of habitat, culture, and individual behavioral variation and the magnitude of their influence on migratory behavior. Future studies should focus on cognitive drivers of migratory decision-making across taxa to determine if a sensory perceptual mechanism is unique to short-distance, altitudinal migrants.
Data Availability Statement
The datasets presented in this article are not readily available because they pertain to an endangered species. Requests to access the datasets should be directed to the Sierra Nevada Bighorn Sheep Recovery Program (asksnbs@wildlife.ca.gov).
Ethics Statement
Ethical review and approval was not required for the animal study because previously collected data was used.
Author Contributions
DB, DG, TS, and TA contributed to the development of ideas. DB, DG, CJ, and RH conducted the analysis. DB wrote the manuscript with input from all authors.
Funding
Funding for collection of Sierra Nevada bighorn sheep demographic data was provided by the California Department of Fish and Wildlife, Federal Assistance in Wildlife Grants, California Wild Sheep Foundation, the Yosemite Conservancy, the Sierra Nevada Bighorn Sheep Foundation, the Wild Sheep Foundation, and Cooperative Endangered Species Conservation Fund grants to support recovery activities. The Bureau of Land Management, Inyo National Forest, Humboldt-Toiyabe National Forest, Yosemite National Park, and Sequoia and Kings Canyon National Parks are partners in the recovery effort and provided funding. DB was partially funded by the Quinney Doctoral Fellowship through the Wildland Resource Department at USU. DB and TA are partially supported by the Ecology Center at USU. CJ was supported by a NASA FINESST grant (award no. 80NSSC19K1359).
Conflict of Interest
The authors declare that the research was conducted in the absence of any commercial or financial relationships that could be construed as a potential conflict of interest.
Publisher’s Note
All claims expressed in this article are solely those of the authors and do not necessarily represent those of their affiliated organizations, or those of the publisher, the editors and the reviewers. Any product that may be evaluated in this article, or claim that may be made by its manufacturer, is not guaranteed or endorsed by the publisher.
Acknowledgments
Captures were made possible with the assistance from S. D. DeJesus, R. C. Swisher, M. D. Shelton, J. D. Pope, G. M. Pope, M. R. Breiling, and G. M. Schales. Field assistance was provided by A. P. Few, T. F. Calfee, B. E. Hatfield, V. M. Davis, J. L. Fusaro, J. D. Wehausen, D. F. Jensen, K. Anderson, C. P. Massing, S. Forshee, J. Weissman, P. Prentice, D. Gammons, J. M. Runcie, J. A. Erlenbach, K. Ellis, H. E. Johnson, L. E. Greene, C. A. Schroeder, B. A. Gonzales, L. Konde, V. C. Bleich, B. M. Pierce, J. T. Villepique, D. B. Spitz, A. C. Sturgill, M. L. C. Kane, M. A. Kiner, K. M. Ellis, M. Dodd, L. R. Gable, A. H. Peet, G. M. Foote, K. E. Nelson, J. A. Erlenbach, R. J. Spaulding, A. E. Feinberg, A. C. Lawrence, T. M. Glenner, B. A. Teagle, W. O. Livingston, D. E. Rivers, G. L. Cadwallader, W. L. Loeper, C. C. Noear. B. A. Munk, B. Regan. E. Siemion, E. Otto, and J. Leary did visual classification into demes. K. Manlove contributed her knowledge of bighorn biology to enhance our understanding of system dynamics. B. Smith assisted with modeling. We would also like to thank the following agencies and non-profits for their contributions to Sierra bighorn recovery efforts: California Department of Fish and Wildlife, Federal Assistance in Wildlife Grants, California Wild Sheep Foundation, Yosemite Conservancy, Sierra Nevada Bighorn Sheep Foundation, Wild Sheep Foundation, and Cooperative Endangered Species Conservation Fund, Bureau of Land Management, Inyo National Forest, Humboldt-Toiyabe National Forest, Yosemite National Park, and Sequoia and Kings Canyon National Parks.
References
Abrahms, B., Hazen, E. L., Aikens, E. O., Savoca, M. S., Goldbogen, J. A., Bograd, S. J., et al. (2019). Memory and resource tracking drive blue whale migrations. Proc. Natl. Acad. Sci. U.S.A. 116, 5582–5587. doi: 10.1073/pnas.1819031116
Allred, B. W., Bestelmeyer, B. T., Boyd, C. S., Brown, C., Davies, K. W., Duniway, M. C., et al. (2021). Improving Landsat predictions of rangeland fractional cover with multitask learning and uncertainty. Methods Ecol. Evol. 12, 841–849. doi: 10.1111/2041-210X.13564
Avgar, T., Baker, J. A., Brown, G. S., Hagens, J. S., Kittle, A. M., Mallon, E. E., et al. (2015). Space-use behaviour of woodland caribou based on a cognitive movement model. J. Anim. Ecol. 84, 1059–1070. doi: 10.1111/1365-2656.12357
Avgar, T., Betini, G. S., and Fryxell, J. M. (2020). Habitat selection patterns are density dependent under the ideal free distribution. J. Anim. Ecol. 89, 2777–2787. doi: 10.1111/1365-2656.13352
Avgar, T., Deardon, R., and Fryxell, J. M. (2013). An empirically parameterized individual based model of animal movement, perception, and memory. Ecol. Modell. 251, 158–172. doi: 10.1016/j.ecolmodel.2012.12.002
Avgar, T., Street, G., and Fryxell, J. M. (2014). On the adaptive benefits of mammal migration. Can. J. Zool. 92, 481–490. doi: 10.1139/cjz-2013-0076
Badyaev, A. V., and Ghalambor, C. K. (2001). Evolution of life histories along elevational gradients: trade-off between parental care and fecundity. Ecology 82, 2948–2960. doi: 10.1890/0012-9658(2001)082[2948:EOLHAE]2.0.CO;2
Bair, E. H., Dozier, J., Davis, R. E., Colee, M. T., and Claffey, K. J. (2015). CUES—a study site for measuring snowpack energy balance in the Sierra Nevada. Front. Earth Sci. 3:58. doi: 10.3389/feart.2015.00058
Ball, J. P., Nordengren, C., and Wallin, K. (2001). Partial migration by large ungulates: charateristics of seasonal moose Alces alces ranges in northern Sweden. Wildlife Biol. 7, 39–47. doi: 10.2981/wlb.2001.007
Barten, N. L., Bowyer, R., and Jenkins, K. J. (2001). Habitat use by female caribou: tradeoffs associated with parturition. J. Wildlife Manage. 65, 77–92. doi: 10.2307/3803279
Barton, K. (2020). MuMIn: Multi-Model Inference. Available online at: https://CRAN.R-project.org/package=MuMIn (April 04, 2020).
Bates, D., Mächler, M., Bolker, B., and Walker, S. (2015). Fitting linear mixed-effects models using lme4. J. Stat. Softw. 67, 1–48. doi: 10.18637/jss.v067.i01
Bauer, S., and Hoye, B. J. (2014). Migratory animals couple biodiversity and ecosystem functioning worldwide. Science 344:10. doi: 10.1126/science.1242552
Berg, J. E., Hebblewhite, M., St. Clair, C. C., and Merrill, E. H. (2019). Prevalence and mechanisms of partial migration in ungulates. Front. Ecol. Evol. 7:325. doi: 10.3389/fevo.2019.00325
Bergerud, A. T., Ferguson, R., and Butler, H. E. (1990). Spring migration and dispersion of woodland caribou at calving. Anim. Behav. 39, 360–368. doi: 10.1016/S0003-3472(05)80882-6
Blumstein, D. T., and Bouskila, A. (1996). Assessment and decision making in animals: a mechanistic model underlying behavioral flexibility can prevent ambiguity. Oikos 77, 569–576. doi: 10.2307/3545948
Borrello, D. (2012). On the role of allee effect and mass migration in survival and extinction of a species. Ann. Appl. Prob. 22, 670–701. doi: 10.1214/11-AAP782
Bracis, C., and Mueller, T. (2017). Memory, not just perception, plays an important role in terrestrial mammalian migration. Proc. Natl. Acad. Sci. U.S.A. 284:20170449. doi: 10.1098/rspb.2017.0449
Brinkman, T. J., Deperno, C. S., Jenks, J. A., Haroldson, B. S., and Osborn, R. G. (2005). Movement of female white-tailed deer: effects of climate and intensive row-crop agriculture. J. Wildlife Manage. 69, 1099–1111. doi: 10.2193/0022-541X(2005)069[1099:MOFWDE]2.0.CO;2
Cagnacci, F., Focardi, S., Heurich, M., Stache, A., Hewison, A. J. M., Morellet, N., et al. (2011). Partial migration in roe deer: migratory and resident tactics are end points of a behavioural gradient determined by ecological factors. Oikos 120, 1790–1802. doi: 10.1111/j.1600-0706.2011.19441.x
California Department of Water Resources (2019). California Data Exchange Center. Sacramento, CA: California Department of Water Resources.
Cayan, D. R. (1996). Interannual climate variability and snowpack in the western united states. J. Clim. 9, 928–948. doi: 10.1175/1520-0442(1996)009<0928:ICVASI>2.0.CO;2
Chapman, B. B., Brönmark, C., and Nilsson, J. -Å, and Hansson, L.-A. (2011). The ecology and evolution of partial migration. Oikos 120, 1764–1775. doi: 10.1111/j.1600-0706.2011.20131.x
Clutton-Brock, T. H. (1984). Reproductive effort and terminal investment in iteroparous animals. Am. Nat. 123, 212–229. doi: 10.1086/284198
Conner, M. M., Stephenson, T. R., German, D. W., Monteith, K. L., Few, A. P., and Bair, E. H. (2018). Survival analysis: informing recovery of sierra nevada bighorn sheep: bighorn sheep survival and recovery strategies. Jour. Wild. Mgmt. 82, 1442–1458. doi: 10.1002/jwmg.21490
Dalerum, F., Boutin, S., and Dunford, J. S. (2007). Wildfire effects on home range size and fidelity of boreal caribou in Alberta, Canada. Can. J. Zool. 85, 26–32. doi: 10.1139/z06-186
Davis, J. L., Carlson, S. W., Coolahan, C. C., and Orthmeyer, D. L. (2012). Sierra Nevada Bighorn Sheep Recovery Program: the Role of UDA Wildlife Services. Sacramento, CA: CDFW, 1999–2011.
Dellinger, J. A., Cristescu, B., Ewanyk, J., Gammons, D. J., Garcelon, D., Johnston, P., et al. (2020). Using mountain lion habitat selection in management. J. Wildlife Manage. 84, 359–371. doi: 10.1002/jwmg.21798
Denryter, K., Stephenson, T. R., and Monteith, K. L. (2021b). Broadening the migratory portfolio of altitudinal migrants. Ecology 102:e03321. doi: 10.1002/ecy.3321
Denryter, K., German, D. W., Stephenson, T. R., and Monteith, K. L. (2021a). State- and context-dependent applications of an energetics model in free-ranging bighorn sheep. Ecol. Model. 440, 109349. doi: 10.1016/j.ecolmodel.2020.109349
Dukas, R. (1998). Cognitive Ecology: the Evolutionary Ecology of Information Processing and Decision Making. Chicago: University of Chicago Press.
Dukas, R. (2004). Evolutionary biology of animal cognition. Annu. Rev. Ecol. Evol. Syst. 35, 347–374. doi: 10.1146/annurev.ecolsys.35.112202.130152
ESRI (2020). Using Viewshed and Observer Points for Visibility Analysis. Redlands, CA: Emvironmental Systems Research Institute.
Fagan, W. F., Lewis, M. A., Auger-Méthé, M., Avgar, T., Benhamou, S., Breed, G., et al. (2013). Spatial memory and animal movement. Ecol. Lett. 16, 1316–1329. doi: 10.1111/ele.12165
Festa-Bianchet, M. (1991). The social system of bighorn sheep: grouping patterns, kinship and female dominance rank. Anim. Behav. 42, 71–82. doi: 10.1016/S0003-3472(05)80607-4
Fryxell, J. M., Greever, J., and Sinclair, A. R. E. (1988). Why are migratory ungulates so abundant? Am. Nat. 131, 781–798. doi: 10.1086/284822
Fryxell, J. M., and Sinclair, A. R. E. (1988). Causes and consequences of migration by large herbivores. Trends Ecol. Evol. 3, 237–241. doi: 10.1016/0169-5347(88)90166-8
Gammons, D. J., Davis, J. L., German, D. W., and Stephenson, T. R. (2021). Predation impedes recovery of Sierra Nevada bighorn sheep. Calif. Fish Wildlife J. 107, 444–470. doi: 10.51492/cfwj.cesasi.27
Gauthreaux, S. A. Jr. (1982). “The ecology and evolution of avian migration systems,” in Avian Biology, ed. D. S. Farner (Cambridge, MA: Academic Press), 93–168. doi: 10.1016/B978-0-12-249406-2.50011-3
Geist, V. (1971). Mountain Sheep: A Study in Behavior and Evolution. Chicago: The University of Chicago Press.
Geist, V., and Petocz, R. G. (1977). Bighorn sheep in winter: do rams maximize reproductive fitness by spatial and habitat segregation from ewes? Can. J. Zool. 55, 1802–1810. doi: 10.1139/z77-234
Grayson, K. L., and Wilbur, H. M. (2009). Sex- and context-dependent migration in a pond-breeding amphibian. Ecology 90, 306–312. doi: 10.1890/08-0935.1
Harris, G., Thirgood, S., Hopcraft, J., Cromsight, J., and Berger, J. (2009). Gloval decline in aggregated migrations of large terrestrial mammals. Endangered Species Res. 7, 55–76. doi: 10.3354/esr00173
Hebblewhite, M., Merrill, E., and Mcdermid, G. (2008). A multi-scale test of the forage maturation hypothesis in a partially migratory ungulate population. Ecol. Monogr. 78:26. doi: 10.1890/06-1708.1
Hebblewhite, M., and Merrill, E. H. (2011). Demographic balancing of migrant and resident elk in a partially migratory population through forage-predation tradeoffs. Oikos 120, 1860–1870. doi: 10.1111/j.1600-0706.2011.19436.x
Heyes, C. M. (1994). Social learning in animals: categories and mechanisms. Biol. Rev. 69, 207–231. doi: 10.1111/j.1469-185X.1994.tb01506.x
Holdo, R. M., Holt, R. D., and Fryxell, J. M. (2015). Opposing rainfall and plant nutritional gradients best explain the wildebeest migration in the serengeti. The American Naturalist. doi: 10.1086/597229
Jesmer, B. R., Merkle, J. A., Goheen, J. R., Aikens, E. O., Beck, J. L., Courtemanch, A. B., et al. (2018). Is ungulate migration culturally transmitted? Evidence of social learning from translocated animals. Science 361, 1023–1025. doi: 10.1126/science.aat0985
John, C., and Post, E. (2021). Seasonality, niche management and vertical migration in landscapes of relief. Ecography 44, 1–13. doi: 10.1111/ecog.05774
Johnson, H. E., Bleich, V. C., and Stephenson, T. R. (2007). Modeling Sierra Nevada Bighorn sheep Habitat: Applying Resource Selection Functions to Species Recovery. Bishop, CA: California Department of Fish and Game.
Johnson, H. E., Hebblewhite, M., Stephenson, T. R., German, D. W., Pierce, B. M., and Bleich, V. C. (2013). Evaluating apparent competition in limiting the recovery of an endangered ungulate. Oecologia 171, 295–307. doi: 10.1007/s00442-012-2397-6
Johnson, H. E., Mills, L. S., Stephenson, T. R., and Wehausen, J. D. (2010a). Population-specific vital rate contributions influence management of an endangered ungulate. Ecol. Appl. 20, 1753–1765. doi: 10.1890/09-1107.1
Johnson, H. E., Scott Mills, L., Wehausen, J. D., and Stephenson, T. R. (2010b). Combining ground count, telemetry, and mark-resight data to infer population dynamics in an endangered species: combining data types to infer dynamics. J. Appl. Ecol. 47, 1083–1093. doi: 10.1111/j.1365-2664.2010.01846.x
Jones, M. O., Allred, B. W., Naugle, D. E., Maestas, J. D., Donnelly, P., Metz, L. J., et al. (2018). Innovation in rangeland monitoring: annual, 30 m, plant functional type percent cover maps for U.S. rangelands, 1984–2017. Ecosphere 9:e02430. doi: 10.1002/ecs2.2430
Jones, M. O., Robinson, N. P., Naugle, D. E., Maestas, J. D., Reeves, M. C., Lankston, R. W., et al. (2021). Annual and 16-Day Rangeland Production Estimates for the Western United States. Rangeland Ecol. Manage. 77, 112–117. doi: 10.1016/j.rama.2021.04.003
Kashetsky, T., Avgar, T., and Dukas, R. (2021). The cognitive ecology of animal movement. Front. Ecol. Evol. 9:724887. doi: 10.3389/fevo.2021.724887
Kauffman, M. J., Cagnacci, F., Chamaillé-Jammes, S., Hebblewhite, M., Hopcraft, J. G. C., Merkle, J. A., et al. (2021). Mapping out a future for ungulate migrations. Science 372, 566–569. doi: 10.1126/science.abf0998
Ketterson, E. D., and Nolan, V. (1976). Geographic variation and its climatic correlates in the sex ratio of eastern-wintering dark-eyed juncos (junco hyemalis hyemalis). Ecology 57, 679–693. doi: 10.2307/1936182
Lendrum, P. E., Anderson, C. R., Monteith, K. L., Jenks, J. A., and Bowyer, R. T. (2013). Migrating mule deer: effects of anthropogenically altered landscapes. PLoS One 8:e64548. doi: 10.1371/journal.pone.0064548
Lima, S. L. (1995). Back to the basics of anti-predatory vigilance: the group-size effect. Anim. Behav. 49, 11–20. doi: 10.1016/0003-3472(95)80149-9
Lomolino, M. V. (2001). Elevation gradients of species-density: historical and prospective views. Glob. Ecol. Biogeogr. 10, 3–13. doi: 10.1046/j.1466-822x.2001.00229.x
Lowrey, B., McWhirter, D. E., Proffitt, K. M., Monteith, K. L., Courtemanch, A. B., White, P. J., et al. (2020). Individual variation creates diverse migratory portfolios in native populations of a mountain ungulate. Ecol. Appl. 30:e2106. doi: 10.1002/eap.2106
Lundberg, P. (1987). Partial bird migration and evolutionarily stable strategies. J. Theor. Biol. 125, 351–360. doi: 10.1016/S0022-5193(87)80067-X
Merkle, J. A., Sawyer, H., Monteith, K. L., Dwinnell, S. P. H., Fralick, G. L., and Kauffman, M. J. (2019). Spatial memory shapes migration and its benefits: evidence from a large herbivore. Ecol. Lett. 22, 1797–1805. doi: 10.1111/ele.13362
Møller, A., Biard, C., Karadas, F., Rubolini, D., Saino, N., and Surai, P. (2011). Maternal effects and changing phenology of bird migration. Clim. Res. 49, 201–210. doi: 10.3354/cr01030
Monteith, K. L., Stephenson, T. R., Bleich, V. C., Conner, M. M., Pierce, B. M., and Bowyer, R. T. (2013). Risk-sensitive allocation in seasonal dynamics of fat and protein reserves in a long-lived mammal. J. Anim. Ecol. 82, 377–388. doi: 10.1111/1365-2656.12016
Mooring, M. S., Fitzpatrick, T. A., Benjamin, J. E., Fraser, I. C., Nishihira, T. T., Reisig, D. D., et al. (2003). Sexual segregation in desert bighorn sheep (Ovis canadensis mexicana). Behaviour 140, 183–207. doi: 10.1163/156853903321671497
Morrison, T. A., and Bolger, D. T. (2012). Wet season range fidelity in a tropical migratory ungulate. J. Anim. Ecol. 81, 543–552. doi: 10.1111/j.1365-2656.2011.01941.x
Mouritsen, H. (1998). Modelling migration: the clock-and-compass model can explain the distribution of ringing recoveries. Anim. Behav. 56, 889–907. doi: 10.1006/anbe.1998.0826
Mueller, T., O’Hara, R. B., Converse, S. J., Urbanek, R. P., and Fagan, W. F. (2013). Social learning of migratory performance. Science 341, 999–1002. doi: 10.1126/science.1237139
Mysterud, A., Loe, L. E., Zimmermann, B., Bischof, R., Veiberg, V., and Meisingset, E. (2011). Partial migration in expanding red deer populations at northern latitudes - a role for density dependence? Oikos 120, 1817–1825. doi: 10.1111/j.1600-0706.2011.19439.x
Nakagawa, S., and Schielzeth, H. (2013). A general and simple method for obtaining R2 from generalized linear mixed-effects models. Methods Ecol. Evol. 4, 133–142. doi: 10.1111/j.2041-210x.2012.00261.x
Nelson, M. E. (1995). Winter range arrival and departure of white-tailed deer in northeastern Minnesota. Can. J. Zool. 73, 1069–1076. doi: 10.1139/z95-127
Pelletier, F. (2005). Foraging time of rutting bighorn rams varies with individual behavior, not mating tactic. Behav. Ecol. 16, 280–285. doi: 10.1093/beheco/arh162
Pulido, F. (2011). Evolutionary genetics of partial migration – the threshold model of migration revis(it)ed. Oikos 120, 1776–1783. doi: 10.1111/j.1600-0706.2011.19844.x
Pulliam, H. R. (1973). On the advantages of flocking. J. Theor. Biol. 38, 419–429. doi: 10.1016/0022-5193(73)90184-7
R Core Team (2021). R: A Language Environment for Statistical Computing. Vienna: R foundation for Statistical Computing.
Rieucau, G., and Martin, J. G. A. (2008). Many eyes or many ewes: vigilance tactics in female bighorn sheep Ovis canadensis vary according to reproductive status. Oikos 117, 501–506. doi: 10.1111/j.0030-1299.2008.16274.x
Risenhoover, K. L., and Bailey, J. A. (1985). Foraging ecology of mountain sheep: implications for habitat management. J. Wildlife Manage. 49, 797–804. doi: 10.2307/3801714
Rittger, K., Bormann, K. J., Bair, E. H., Dozier, J., and Painter, T. H. (2021). Evaluation of VIIRS and MODIS snow cover fraction in high-mountain Asia using landsat 8 OLI. Front. Remote Sens. 2:647154. doi: 10.3389/frsen.2021.647154
Robin, X., Turck, N., Hainard, A., Tiberti, N., Lisacek, F., Sanchez, J.-C., et al. (2011). pROC: an open-source package for R and S+ to analyze and compare ROC curves. BMC Bioinform. 12:77. doi: 10.1186/1471-2105-12-77
Robinson, N. P., Jones, M. O., Moreno, A., Erickson, T. A., Naugle, D. E., and Allred, B. W. (2019). Rangeland productivity partitioned to sub-pixel plant functional types. Remote Sens. 11:1427. doi: 10.3390/rs11121427
Ruckstuhl, K. E. (1998). Foraging behaviour and sexual segregation in bighorn sheep. Anim. Behav. 56, 99–106. doi: 10.1006/anbe.1998.0745
Sabine, D. L., Morrison, S. F., Whitlaw, H. A., Ballard, W. B., Forbes, G. J., and Bowman, J. (2002). Migration behavior of white-tailed deer under varying winter climate regimes in new brunswick. Journal of Wildlife Manage. 66, 718–728. doi: 10.2307/3803137
Sawyer, H., Merkle, J. A., Middleton, A. D., Dwinnell, S. P. H., and Monteith, K. L. (2019). Migratory plasticity is not ubiquitous among large herbivores. J. Anim. Ecol. 88, 450–460. doi: 10.1111/1365-2656.12926
Schroeder, C. A., Bowyer, R. T., Bleich, V. C., and Stephenson, T. R. (2010). Sexual segregation in sierra nevada bighorn sheep, Ovis canadensis sierrae: ramifications for conservation. Arctic Antarctic Alpine Res. 42, 476–489. doi: 10.1657/1938-4246-42.4.476
Shaw, A. K. (2016). Drivers of animal migration and implications in changing environments. Evol. Ecol. 30, 991–1007. doi: 10.1007/s10682-016-9860-5
Singh, N. J., Börger, L., Dettki, H., Bunnefeld, N., and Ericsson, G. (2012). From migration to nomadism: movement variability in a northern ungulate across its latitudinal range. Ecol. Appl. 22, 2007–2020. doi: 10.1890/12-0245.1
Skov, C., Baktoft, H., Brodersen, J., Brönmark, C., Chapman, B. B., Hansson, L.-A., et al. (2011). Sizing up your enemy: individual predation vulnerability predicts migratory probability. Proc. Natl. Acad. Sci. U.S.A. 278, 1414–1418. doi: 10.1098/rspb.2010.2035
Spitz, D. B. (2015). Does Migration Matter? Causes and Consequences of Migratory Behavior in Sierra Nevada Bighorn Sheep. Ph.D. dissertation. Missoula, MT: University of Montana.
Spitz, D. B., Hebblewhite, M., and Stephenson, T. R. (2017). ‘MigrateR’: extending model-driven methods for classifying and quantifying animal movement behavior. Ecography 40, 788–799. doi: 10.1111/ecog.02587
Spitz, D. B., Hebblewhite, M., and Stephenson, T. R. (2020). Habitat predicts local prevalence of migratory behaviour in an alpine ungulate. J. Anim. Ecol. 89, 1032–1044. doi: 10.1111/1365-2656.13167
Spitz, D. B., Hebblewhite, M., Stephenson, T. R., and German, D. W. (2018). How plastic is migratory behavior? Quantifying elevational movement in a partially migratory alpine ungulate, the Sierra Nevada bighorn sheep (Ovis canadensis sierrae). Can. J. Zool. 96, 1385–1394. doi: 10.1139/cjz-2017-0367
Stephenson, T. R., German, D. W., Cassirer, E. F., Walsh, D. P., Blum, M. E., Cox, M., et al. (2020). Linking population performance to nutritional condition in an alpine ungulate. J. Mammal. 101, 1244–1256. doi: 10.1093/jmammal/gyaa091
Stephenson, T. R., Wehausen, J. D., Few, A. P., German, D. W., Jensen, D. F., Spitz, D. B., et al. (2012). 2001-2011 Annual Report of the Sierra Nevada Bighorn Sheep Recovery Program: A Decade in Review. Bishop, CA: California Department of Fish and Wildlife.
Tucker, M. A., Böhning-Gaese, K., Fagan, W. F., Fryxell, J. M., Van Moorter, B., Alberts, S. C., et al. (2018). Moving in the anthropocene: global reductions in terrestrial mammalian movements. Science 359, 466–469. doi: 10.1126/science.aam9712
US Fish and Wildlife Service (2007). Recovery Plan for the Sierra Nevada Bighorn Sheep. Washington, DC: US Fish and Wildlife Service.
Valdez, R., and Krausman, P. (1999). Mountain Sheep of North America. Tucson: The University of Arizona Press.
Wehausen, J. D. (1992). “The role of precipitation and temperature in the winter range diet quality of mountain sheep of the Mount Baxter herd, Sierra Nevada,” in Proceedings of the Biennial Symposium of the Northern Wild Sheep and Goat Council, Ventura, CA, 279–292.
Wehausen, J. D. (1996). Effects of mountain lion predation on bighorn sheep in the sierra nevada and granite mountains of California. Wildlife Soc. Bull. 24, 471–479.
White, K. S., Barten, N. L., Crouse, S., and Crouse, J. (2014). Benefits of migration in relation to nutritional condition and predation risk in a partially migratory moose population. Ecology 95, 225–237. doi: 10.1890/13-0054.1
Keywords: memory, perception, culture, facultative migration, partial migration, ungulates, reintroduction, cognitive movement ecology
Citation: Berger DJ, German DW, John C, Hart R, Stephenson TR and Avgar T (2022) Seeing Is Be-Leaving: Perception Informs Migratory Decisions in Sierra Nevada Bighorn Sheep (Ovis canadensis sierrae). Front. Ecol. Evol. 10:742275. doi: 10.3389/fevo.2022.742275
Received: 15 July 2021; Accepted: 13 January 2022;
Published: 21 February 2022.
Edited by:
Andrew James Jonathan MacIntosh, Kyoto University, JapanReviewed by:
Iain James Gordon, Australian National University, AustraliaJerod Merkle, University of Wyoming, United States
Tana Verzuh, University of Wyoming, United States, in collaboration with reviewer JM
Copyright © 2022 Berger, German, John, Hart, Stephenson and Avgar. This is an open-access article distributed under the terms of the Creative Commons Attribution License (CC BY). The use, distribution or reproduction in other forums is permitted, provided the original author(s) and the copyright owner(s) are credited and that the original publication in this journal is cited, in accordance with accepted academic practice. No use, distribution or reproduction is permitted which does not comply with these terms.
*Correspondence: Danielle J. Berger, danielle.berger@usu.edu
†ORCID: Christian John, orcid.org/0000-0002-6249-040X