- 1Chaire de Recherche du Canada en Biodiversité Nordique, Centre d’Études Nordiques, Centre de la science de la biodiversité du Québec, Département de biologie, chimie et géographie, Université du Québec à Rimouski, Rimouski, QC, Canada
- 2Chaire de Recherche du Canada en Écologie Intégrative, Centre d’Études Nordiques, Centre de la science de la biodiversité du Québec, Département de Biologie, Université de Sherbrooke, Sherbrooke, QC, Canada
- 3Chaire de Recherche Sentinelle Nord sur l’impact des migrations animales sur les écosystèmes nordiques, Centre de la science de la biodiversité du Québec, Centre d’Études Nordiques, Département de Biologie, Université Laval, Québec, QC, Canada
- 4Centre d’Études Biologiques de Chizé (CEBC-CNRS), Université de La Rochelle, La Rochelle, France
- 5Centre d’Études Nordiques, Département de Biologie, Université Laval, Québec, QC, Canada
- 6Chaire de Recherche du Canada en Écologie Arctique, Centre de la science de la biodiversité du Québec, Département des Sciences des Ressources Naturelles, Université McGill, Montréal, QC, Canada
- 7Centre for Biodiversity and Environment Research, Department of Genetics, Evolution and Environment, University College London, London, United Kingdom
- 8Acopian Center for Conservation Learning, Hawk Mountain Sanctuary, Orwigsburg, PA, United States
- 9Science and Technology Program, Polar Knowledge Canada, Cambridge Bay, NU, Chaire de Recherche du Canada en Biodiversite Nordique, Centre d’Etudes Nordiques, Departement de biologie, chimie et geographie, Universite du Quebec a Rimouski, Rimouski, QC, Canada
Seasonal migrants transport energy, nutrients, contaminants, parasites and diseases, while also connecting distant food webs between communities and ecosystems, which contributes to structuring meta-communities and meta-ecosystems. However, we currently lack a framework to characterize the structure of the spatial connections maintained by all migratory species reproducing or wintering in a given community. Here, we use a network approach to represent and characterize migratory pathways at the community level and provide an empirical description of this pattern from a High-Arctic terrestrial community. We define community migration networks as multipartite networks representing different biogeographic regions connected with a focal community through the seasonal movements of its migratory species. We focus on the Bylot Island High-Arctic terrestrial community, a summer breeding ground for several migratory species. We define the non-breeding range of each species using tracking devices, or range maps refined by flyways and habitat types. We show that the migratory species breeding on Bylot Island are found across hundreds of ecoregions on several continents during the non-breeding period and present a low spatial overlap. The migratory species are divided into groups associated with different sets of ecoregions. The non-random structure observed in our empirical community migration network suggests evolutionary and geographic constraints as well as ecological factors act to shape migrations at the community level. Overall, our study provides a simple and generalizable framework as a starting point to better integrate migrations at the community level. Our framework is a far-reaching tool that could be adapted to address the seasonal transport of energy, contaminants, parasites and diseases in ecosystems, as well as trophic interactions in communities with migratory species.
1. Introduction
Each year, billions of migratory organisms travel the world back and forth seasonally to complete their life cycle, which leads natural communities and ecosystems to shift their properties periodically (Holdo et al., 2011; Bauer and Hoye, 2014; Furey et al., 2018). By moving from and to different natural systems, migratory species create ecological connections between remote communities and ecosystems, thus creating meta-communities and meta-ecosystems (Bauer and Hoye, 2014; Gounand et al., 2018). Therefore, the effects of local environmental disturbances (hereafter perturbation) such as habitat loss, climate change, invasive species, disease outbreaks and subsidies of contaminants and nutrients can propagate between natural systems at the meta-level.
Local perturbations can modulate the effects of migratory species on communities and ecosystems that are located thousands of kilometers apart, leading to spatial cascades (Bauer and Hoye, 2014). Spatial cascades are defined as the propagation of indirect effects between remote natural systems (García-Callejas et al., 2019). For instance, perturbations on the non-breeding ground of a migratory species can affect population size at a distant breeding ground through carry-over effects (Webster et al., 2002; Norris, 2005; Taylor and Norris, 2010; Wiederholt et al., 2018; Taylor, 2019). The resulting change in breeding population size can influence both the strength of trophic interactions in communities (Jefferies et al., 2004) and the flux of matter in ecosystems (Hessen et al., 2017; Springer et al., 2018) leading to local cascading effects. The effects of migratory species on communities and ecosystems are diverse, including positive, negative, direct and indirect trophic interactions, the dispersal of organisms and diseases and the exchange of energy, nutrients and contaminants (Holdo et al., 2011; Bauer and Hoye, 2014; Viana et al., 2016). In a given community, multiple migratory species maintain several spatial connections with other communities and ecosystems (hereafter migratory pathways) through which spatial cascades can occur. Although spatial cascades via migrations have been empirically described (Jefferies et al., 2004; Hessen et al., 2017; Springer et al., 2018), they have only been addressed theoretically based on dispersal and foraging movements (McCann et al., 2005; García-Callejas et al., 2019).
Meta-community frameworks have been defined for dispersal and foraging movements, but have never been adapted to migratory movements. The traditional meta-community framework defines dispersal and foraging movements as continuous links between communities, where at any time, some individuals relocate, and others forage in other communities. However, seasonal migratory movements are often highly synchronous. All individuals leave one community and seasonally relocate to another within a short period of time, so it is more of a discontinuous on-and-off connection between communities than a continuous one. Moreover, migratory species must access distant communities at different seasons to complete their annual cycle. Unlike resident species, if the movement of migratory species between communities is prevented, the species become extinct. Migratory species can only persist in a meta-community system, unlike resident species in the traditional meta-community framework. Therefore, the way in which dispersal and foraging movements are incorporated into traditional meta-community frameworks does not fit the characteristics of migratory movements.
The typical annual cycle of seasonal migratory species can be divided into periods of breeding, post-breeding migration, stationary non-breeding and pre-breeding migration (Rubenstein and Hobson, 2004; Mueller and Fagan, 2008). We define the breeding period as the length of time individuals are relatively stationary to breed and raise their young. We define the stationary non-breeding period (hereafter non-breeding period) as the portion of the time when individuals are located outside of their breeding ground and perform only local movements, which is often defined as wintering in the northern hemisphere. We define the migration periods as the relocation of individuals from breeding grounds to stationary non-breeding grounds and inversely. Because of their seasonal movements, migratory individuals are present for only part of their annual cycle in the visited communities and ecosystems. Therefore, a perturbation occurring on a non-breeding ground may only spread to a breeding ground in subsequent seasons, and vice versa, generating a seasonal delay in the spatial spread of perturbations.
Due to the characteristics of seasonal migrations, we need to adapt existing frameworks in community ecology to study migrations at the community level. Networks represent how information propagates through interconnected components (Newman, 2018) and are now widely used in ecology (Dale and Fortin, 2010). They are composed of nodes or vertices (circles) connected by edges (lines). In their simplest form, networks present a single type of node (unipartite), such as a food web, where the nodes illustrate the species of a community and the edges represent trophic interactions between those species (Dunne et al., 2002). Networks are represented by an adjacency matrix A where each element consists of pairs of nodes ij (e.g., species i and species j) where 1 represents the presence and 0 the absence of an edge (e.g., trophic interaction) between node i and node j (Newman, 2018). Edges from node i to node j can be directed (unidirectional) or undirected (bidirectional) and unweighted (binary) or weighted (non-binary; e.g., diet matrix). Networks have been used to study ecological interactions within communities (Dunne et al., 2002; Fortuna et al., 2010), migratory pathways at the species level (Iwamura et al., 2013; Knight et al., 2018; Taylor, 2019) and dispersal or foraging movements between set of communities (García-Callejas et al., 2019). However, we still lack a network approach that can highlight the meta-ecosystem and meta-community connections maintained by the migratory species from a focal community, while taking into account the seasonality of migrations.
Through this paper, we aim to (i) propose a framework to represent and characterize the migratory pathways maintained at the community level and (ii) provide an empirical description of the structure of migratory pathways at the community level from a case study. Terrestrial Arctic communities are relevant systems to scale migrations at the community level since they present a relatively low species richness with a high proportion of migratory species (Newton and Dale, 1996; Somveille et al., 2013). We propose the concept of community migration networks based on a simple network approach commonly used in community ecology, but which will be adapted here to the context of community-level migrations. We propose a novel network definition and thus novel interpretations of traditional network metrics, but do not make any mathematical advances. We highlight the potential of the proposed framework by describing the community migration network of the Bylot Island tundra food web for which detailed ecological monitoring information is available over the last 30 years (Gauthier et al., 2011, 2013; Figure 1). For the purpose of the study, all species from the community are considered here (5 mammals and 30 birds). Bylot Island has a relatively simple community for which we have long-term monitoring showing the local influence of migratory species on trophic interactions and the ecosystem dynamic, as well as their global migratory pathways (Therrien et al., 2012; Robillard et al., 2018; Léandri-Breton et al., 2019; Lamarre et al., 2021; Legagneux et al., unpublished data; Seyer et al., 2021). Bylot Island hosts the largest greater snow goose (Chen caerulescens atlantica) colony, which represents the dominant herbivore of the system (Legagneux et al., 2012). We use this species whose migratory pathways and trophic effects have been well documented at our study site to show how our framework can be used to complement existing food web models by illustrating the spread of perturbations at the meta-community level. Due to agricultural changes on the non-breeding and staging grounds, the snow goose population has increased dramatically at the study site (Gauthier et al., 2005). This increase led to important local changes in the community and ecosystem: a reduction in graminoid biomass through grazing (Gauthier et al., 1995), the exportation of nitrogen through young flying out of the island (Gauthier et al., 2011), and an increase in nest predation on other species through apparent competition (Lamarre et al., 2017; Clermont et al., 2021; Duchesne et al., 2021).
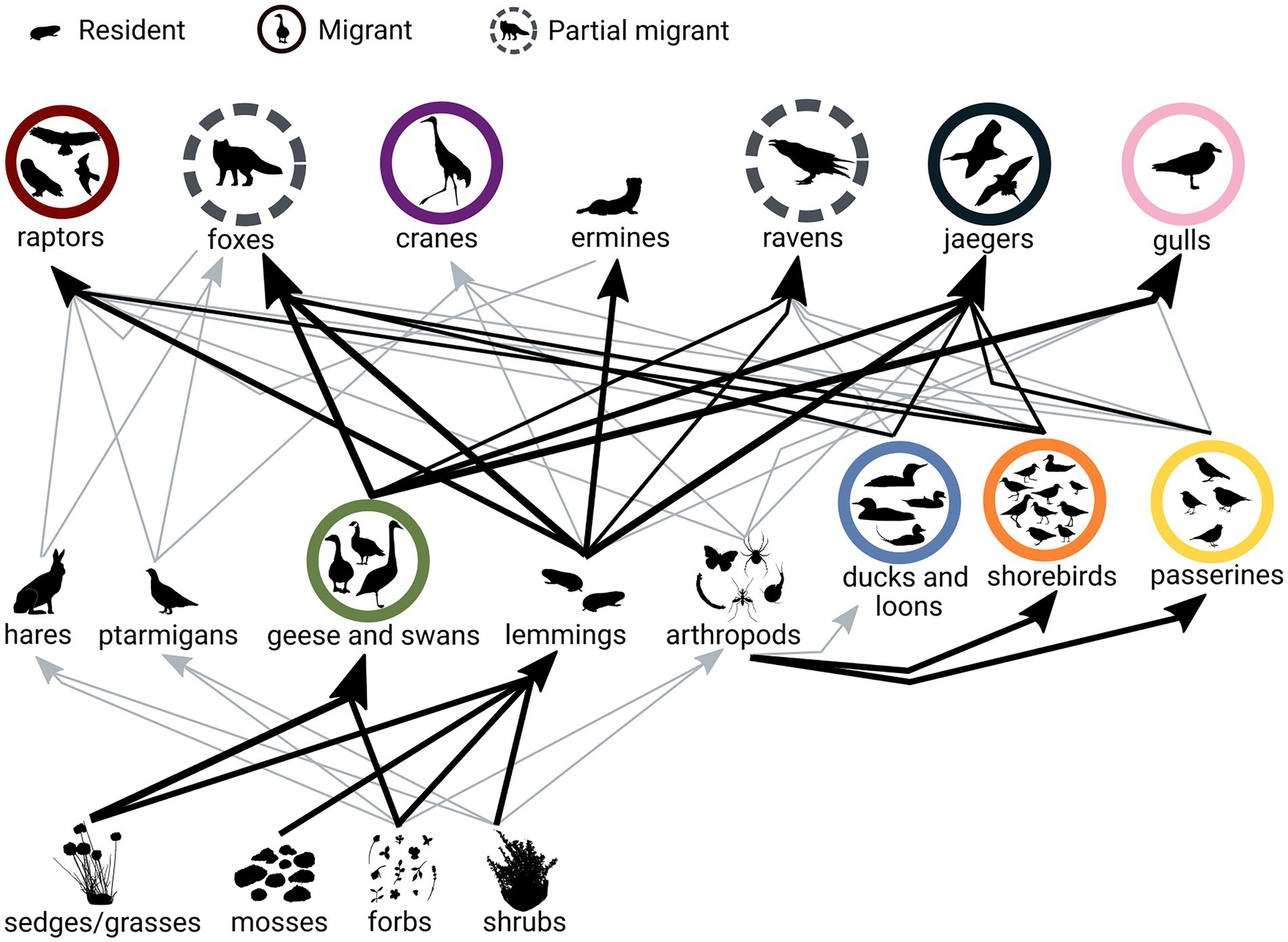
Figure 1. Bylot Island tundra food web adapted from Gauthier et al. (2011). Labels represent the functional groups, and the groups composed of migratory species are colored (red = raptors, pink = gulls, purple = cranes, dark blue = jaegers, light blue = ducks and loons, orange = shorebirds, yellow = passerines, green = geese and swans). The species composition of vertebrate functional groups is represented by icons (e.g., four species of passerines). Arrows represent biomass flow between functional groups and the size of the arrows is relative to the proportion of the diet; gray arrows represent minor portions of the diet.
2. Community migration networks
We propose the concept of community migration networks to scale migratory pathways at the community level. We consider community migration networks as multipartite networks (i.e., different types of nodes) representing the geographic regions connected with a focal community through the seasonal movements of its migratory species. Although multipartite networks have been widely used in community ecology (host–parasite, plant-pollinator, seed-disperser; Fortuna et al., 2010), they have never been adapted to study the spatial connections made by animal movement at the community level. Community migration networks, in their simplest form are presented as bipartite with the species assemblage of a focal community and the migration destinations represented by geographic regions (either non-breeding, breeding or stopover grounds). For example, an Arctic community where multiple migratory species come to breed (i.e., focal community) can be connected with different regions used as non-breeding grounds by the migratory species. Community migration networks can also be presented as tripartite with the species of the focal community and two types of migration destinations (e.g., non-breeding and staging regions). The focal community can be a breeding, a stopover or a non-breeding ground used by migratory species. However, the framework considers a single type of edge (migratory movements), currently preventing modeling trophic interactions within a given focal community.
The populations of migratory species are considered seasonal nodes in community migration networks (Figure 2). The species nodes are taxonomically defined as populations of the species in the focal community and spatiotemporally delimited by the arrival of the individuals in the focal community until their departure. Therefore, the temporal definition of the species nodes considers the populations of migratory species as seasonal (discontinuous) and populations of resident species as non-seasonal (continuous) since they stay in the community throughout the year. Populations of resident species are presented as unconnected nodes in the network. Partially migratory species with both resident and migratory individuals (Chapman et al., 2011) are represented by a seasonal and a non-seasonal species node, representing the proportion of migratory and resident individuals in the population, respectively. The nodes representing the geographic regions are spatially defined by the boundaries of the regions and are considered temporally continuous since perturbation can happen in the regions even when migratory species are absent (e.g., habitat loss or contaminant subsidies). The temporal definition of nodes and edges does not need to be considered explicitly to highlight the pattern of migrations at the community level, which is the scope of this paper. However, in a dynamic community migration network, the temporal definition of nodes would be essential.
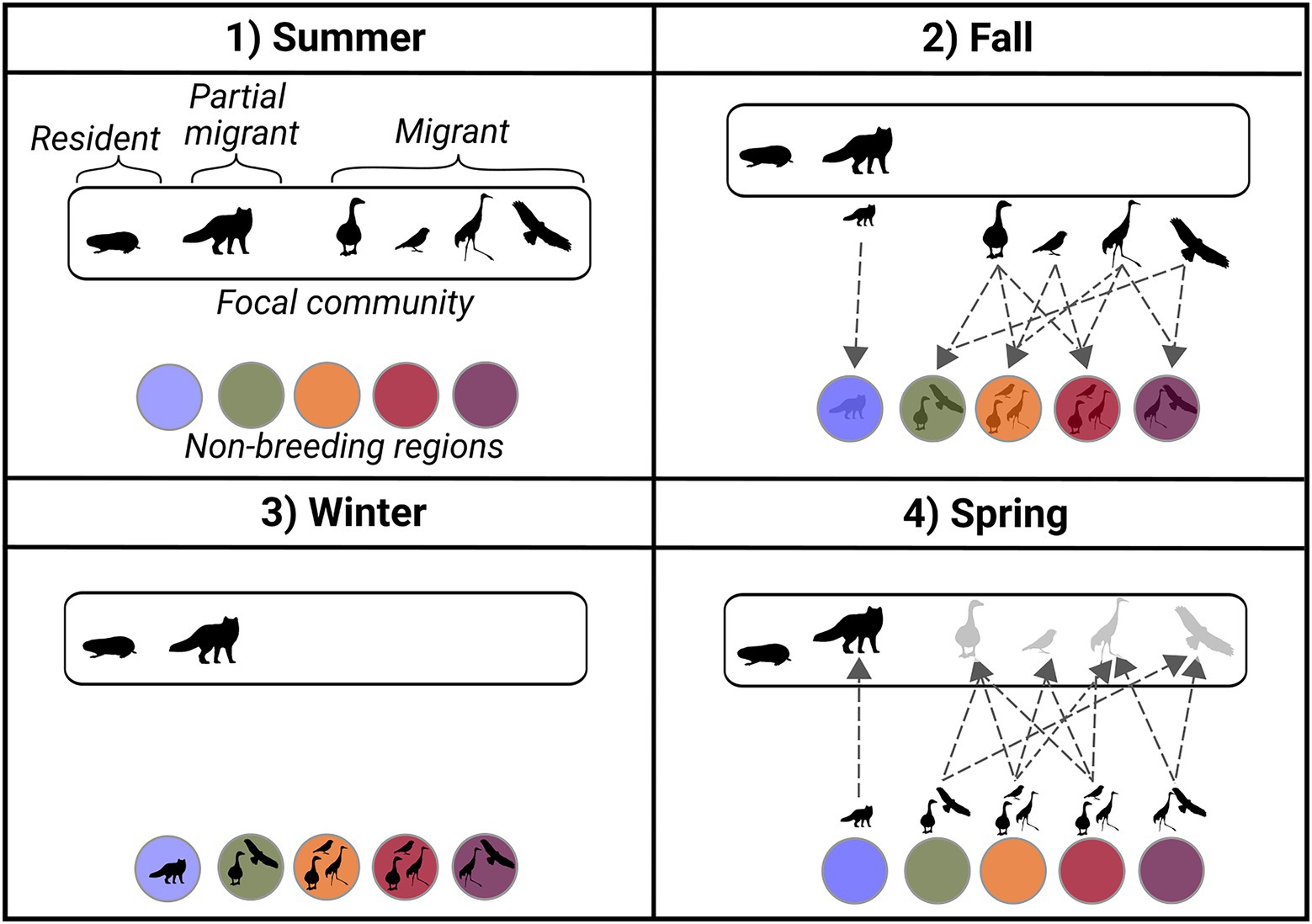
Figure 2. Schematic representation and definition of the seasonal nature of community migration networks through a fictive community representing a summer breeding ground used by multiple migratory species (white rectangle) and their migratory pathways (dotted lines) to non-breeding regions (colored circles). (1) Summer: populations of diverse migratory, partially migratory and resident species (black icons) co-exist at the same location, which defines the focal community (white rectangle) (2) Fall: the migratory and a part of the partially migratory populations leave the focal community through the migratory pathways (dotted lines) to reach non-breeding regions (colored circles) (3) Winter: the migratory and a part of partially migratory populations are distributed across diverse non-breeding regions, and the resident and a part of partially migratory populations stayed at the focal community location (4) Spring: the migratory and a part of the partially migratory populations from the focal community leave the non-breeding regions to reach the focal community location through the migratory pathways.
The edges of community migration networks represent the seasonal movements of migratory species between geographical locations used at each period of their annual cycle. Edges can be either undirected or directed depending on whether the species use the same migratory pathways back and forth between nodes of different types (period of the annual cycle) or not. The edges can be weighted to represent the biomass, abundance or proportion of individuals of each species between the focal community and the connected regions. Considering that edges are seasonal, it implies that a perturbation on a distant region would not propagate directly to the focal community but would reach the community in the next season, thus necessarily implying a delayed propagation of perturbations.
Community migration networks are unique relative to other types of networks because (i) some nodes (migratory species) and all edges are seasonal, (ii) during migration periods, all edges have the same direction, (iii) some of the nodes (migratory species) depend on nodes of another type (distant regions), but not the opposite, and (iv) the framework focuses on a local community at a global scale. The unique nature of community migration networks highlights a theoretical challenge in modeling dynamical community migration networks, but those characteristics are essential to represent the biology of migratory species.
Community migration networks can be described with multipartite network metrics at the node and network levels to highlight the structure of the migratory pathways at the species and community levels (Figure 3). At the node level, the species degree (number of regions connected with a species) reflects the population spread, defined as the mean distance in the location of individuals from the same population between different seasons (Finch et al., 2017). Highly spread populations will be found in more geographical regions. In a bipartite community migration network, the region degree represents the number of migratory species from the focal community hosted by a region in another annual cycle period (i.e., non-breeding region). The region degree could potentially be used to identify ecological hot spots thousands of kilometers away from a focal community.
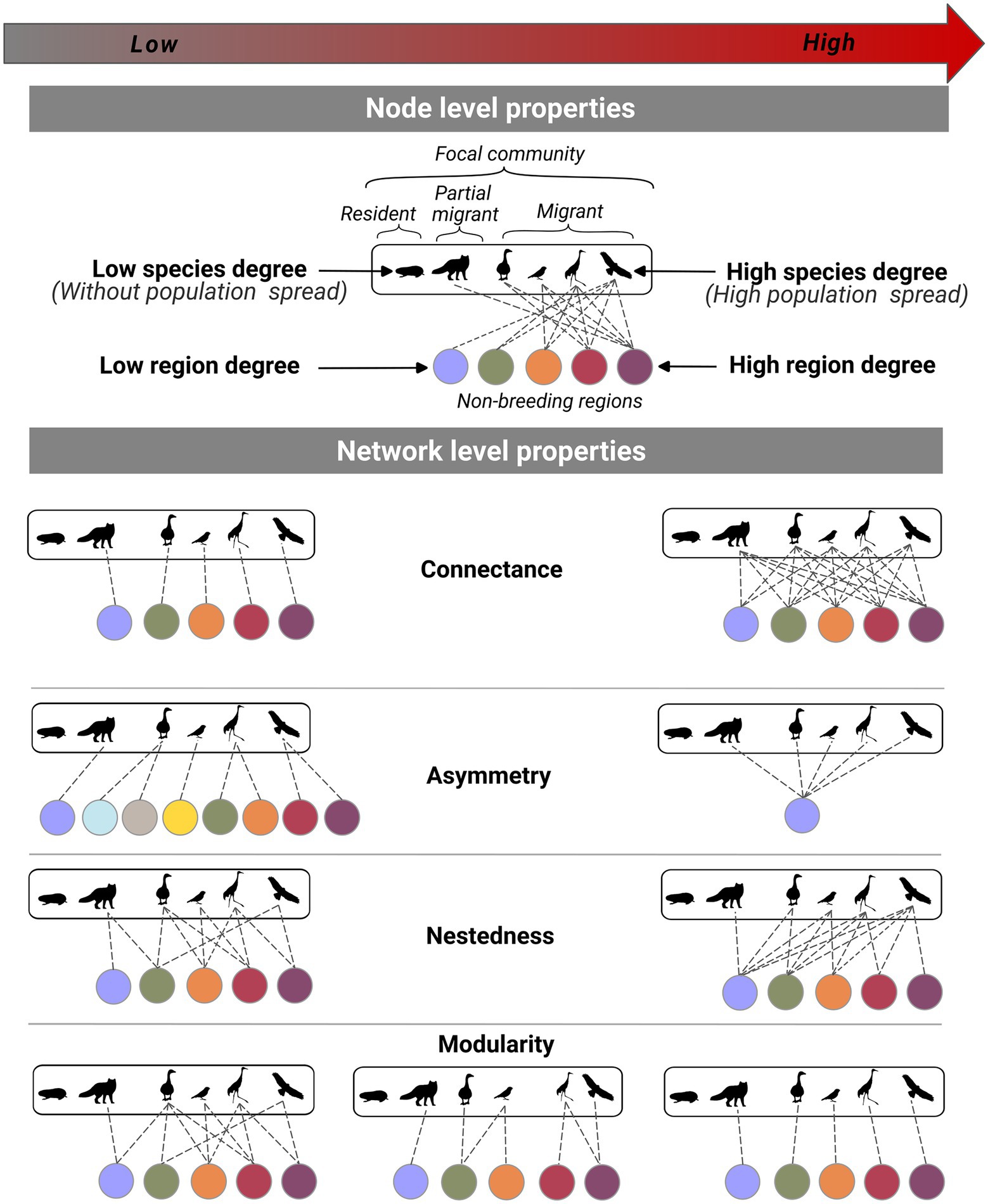
Figure 3. Schematic definitions and examples of low and high values of bipartite community migration network metrics. The white rectangle represents the focal community, and the colored circles represent the connected geographic regions through migrations. The dotted lines represent the movements of species between the focal community and the regions used during another period of their annual cycle. The movements of species between the focal community and the connected regions are presented as undirected to represent the use of the same pathways back and forth. Resident species are represented by the absence of edges.
At the network level, we suggest using connectance, asymmetry, nestedness and modularity to characterize community migration networks. Connectance represents the degree of spatial overlap between the species of a focal community during another period of the annual cycle. A high connectance would mean a large spatial overlap between the species of a focal community during another period of the annual cycle, suggesting perturbation of connected regions could potentially affect multiple species simultaneously. Asymmetry represents the proportion of species nodes versus region nodes in the network. It illustrates the relative concentration or spatial diffusion of species once they leave the focal community. A positive asymmetry value would represent more species than regions and a negative value would point toward the opposite. An extremely high asymmetry would represent the concentration of the species in a small number of regions and suggest that the focal community is exposed to highly localized perturbations occurring in distant but connected regions. Nestedness assesses if small-range species are found inside the boundaries of broad-range species during another period of the annual cycle. If the network is nested, some regions host multiple species of different range sizes. A nested structure of migratory pathways would mean that if a perturbation happens on a species with a small non-breeding range, it also has a high chance of propagating to the community through species with larger non-breeding ranges. The modules represent groups of species that have more spatial overlap with each other on their range outside the focal community than with other species of the community. Modularity highlights the degree of overlap between the modules, with little overlap between modules showing high modularity. A modular community migration network would mean that perturbations occurring on distant but connected regions would propagate to the focal community through subgroups of species. In this paper, we have decided to limit ourselves to a description of the network and to propose interpretations for some traditional network metrics. Still, we will discuss the use of traditional network metrics in community migration networks.
3. Materials and methods
3.1. Network definition
We studied the Bylot Island tundra community located at the northern tip of Baffin Island in Nunavut, Canada (73° N, 80° W). The study area of 425km2 (Giroux et al., 2012) is located in the southern part of the island inside a mosaic of upland mesic tundra habitat interspersed with lowland wetlands (Gauthier et al., 2013). Based on a long-term community monitoring program, we identified 35 vertebrate species in the food web (5 mammals and 30 birds; see Supplementary material section 1.1 for details and Supplementary Table S1 for the species list and scientific names). Among the 35 vertebrate species, 28 are long-distance migrants, two are partial migrants (Arctic fox and common raven), and five are residents (Gauthier et al., 2011). We define migrants as individuals performing seasonal and highly synchronous movements between a breeding and a non-breeding ground (Rubenstein and Hobson, 2004; Mueller and Fagan, 2008), residents as individuals performing movements within a community throughout the annual cycle, and partial migrants as the combination of resident and migratory and/or individuals performing long-distance foraging trips outside the community (Chapman et al., 2011; Gauthier et al., 2011).
The non-breeding range of each migratory species was defined by the most representative range data available (Supplementary Material section 1.2). We used non-breeding range maps (BirdLife International and Handbook of the Birds of the World, 2019; Fink et al., 2020) along with global avian migration flyways (geographical grouping of annual ranges of avian migratory species; Wetlands International, 2022) when tracking data was unavailable for long-distance migratory species (22 of 28 species). The association of flyway(s) to each species was based on known migratory pathways by eastern Canadian Arctic individuals. We retained only the portion of the non-breeding range within the boundaries of the flyway(s) assigned to each species, thereby removing portions of the non-breeding ranges where individuals from the focal community are absent. The seasonal migratory movement of long-tailed jaegers (Seyer et al., 2021), common-ringed plovers (Léandri-Breton et al., 2019), American golden-plovers (Lamarre et al., 2021), snowy owls (Therrien et al., 2012; Robillard et al., 2018), and snow geese (Legagneux et al., unpublished data) and the partial migratory movement of Arctic fox (Lai et al., 2017) have been documented with tracking devices deployed from the study site. Migratory movements of king eiders were monitored from another breeding site in the eastern Canadian Arctic (Gilchrist et al., 2004). The partially migratory species in the community (Arctic fox and common raven) perform short-distance movements within the Arctic. They are thus considered circumpolar residents at the species level, and it was not possible to use species non-breeding range maps for these species as we did for long-distance migrants. The non-breeding ranges of partially migratory species were defined with a buffer zone centered on the study site with a radius size corresponding to the maximum distance travelled during migratory or long-distance foraging movements observed in the literature.
We determined the biogeographic ecoregions associated with the defined non-breeding range of each species from the focal community (Supplementary Material section 1.3). Ecoregions represent relatively homogeneous species assemblages at the regional scale (Smith et al., 2018), and they consider ecological and geographical characteristics (e.g., Eastern Canadian forests), unlike biomes that focus only on ecological attributes (e.g., Boreal forests). We used worldwide classifications of terrestrial ecoregions from Olson et al. (2001) and marine ecoregions from Spalding et al. (2007). We derived a classification of coastal ecoregions based on Spalding et al. (2007). We extracted for each species a list of ecoregions overlapping the non-breeding range. We assigned main non-breeding habitat type(s) (terrestrial, coastal and/or marine) to each species based on the available literature to filter ecoregions from the extracted list that would be marginally used by the species (Supplementary material section 1.4). On average, the non-breeding range of each species was associated with 33 ± 46 ecoregions, including no connections for resident species. To validate our non-breeding ranges refinement and filtering methods, we performed a preliminary validation analysis based on the specific tracking programs available as reference (n = 6 species; American golden-plover, common-ringed plover, snowy owl, long-tailed jaeger, snow goose and king eider; Supplementary material section 1.5). Our method of refining and filtering non-breeding ranges removed a considerable number of irrelevant ecoregions associations. However, the use of refined species range maps still tend to overestimate the number of connected ecoregions in comparison with specific tracking data. We used the ecoregions associated with each species to create an incidence matrix of size, where I represents the total number of species in the focal community and J represents the total number of ecoregions connected with the community. Data manipulation and geoprocessing were done in R language and environment version 3.6.3 (R Core Team, 2020) with package sf (Pebesma, 2018) and dplyr (Wickham et al., 2021).
3.2. Network analysis
The community migration network was defined and analyzed as an unweighted and undirected bipartite network with species and biogeographic ecoregions. At the node level, we used the degree of each node (i.e., number of edges; Figure 3).
At the network level, we used connectance, asymmetry, nestedness (Dormann et al., 2009) and modularity (Farage et al., 2021). Connectance (C) is based on the proportion of realized edges in a network and is measured by in a bipartite network, where L represents the total number of edges. Network asymmetry (W) addresses the difference in the number of nodes of each type with respect to the total number of nodes, and it is measured by with values ranging from −1 to 1. A network is considered nested when low degree nodes share a subset of the connections of high degree nodes. Nestedness (T) is comprised between 0 (perfectly nested structure) and 100 (random structure), and it is commonly used to describe bipartite ecological networks (e.g., plant-pollinators, host-parasites, island biogeography; see Rodríguez-Gironés and Santamaría, 2006 for more details on the algorithm and the equation). We used the package bipartite (Dormann et al., 2008) to extract this network property. Finally, modularity (M) is a widely used network metric to assess the presence of sub-groups of nodes with more within-group edges than expected by random (see Farage et al., 2021 for more details on the algorithm and the equation). The modularity analysis was performed with the package infomapecology based on network flow (Farage et al., 2021), representing the flow of migratory individuals between the focal community and non-breeding regions (Supplementary material section 2.1). The modularity and nestedness values are influenced by other structural properties of the network (Fortuna et al., 2010). Therefore, we needed to compare the observed values with networks with the same degree distribution and connectance, but with randomly assigned edges. We used the quasi-swap algorithm (detailed in Miklós and Podani, 2004) from the package vegan (Oksanen et al., 2022) to simulate 1,000 null models. More specifically, the algorithm maintains the row and column sums of the original matrix (i.e., same degree distribution and connectance) and repeatedly shuffles the edges in 2×2 subsets of the original matrix (Miklós and Podani, 2004).
4. Results
The species of Bylot Island are distributed latitudinally during the non-breeding period from the High-Arctic to the southern tip of South America and longitudinally from the Pacific Northeast to West Africa (Figure 4). Geese and swans, passerines, cranes and gulls are the only functional groups from Bylot strictly found in North America during the non-breeding period, whereas jaegers are strictly found outside of North America. Shorebirds, jaegers and raptors are the only functional groups associated with ecoregions outside North America. The community of Bylot Island is connected with 393 ecoregions through the distribution of its migratory species during the non-breeding period (Figure 5). The peregrine falcon is the species with the highest degree in the network, associated with 257 ecoregions. On average, the non-breeding range of each species overlapped the boundaries of 33 ± 46 ecoregions, including no connections for resident species. The ecoregions are connected with one to 14 migratory species with a mean of 3.0 ± 2.4. For both species and ecoregions, a small number of nodes are highly connected, and many are weakly connected, resulting in both left-skewed distributions of the number of edges per node (Supplementary material Figure S5).
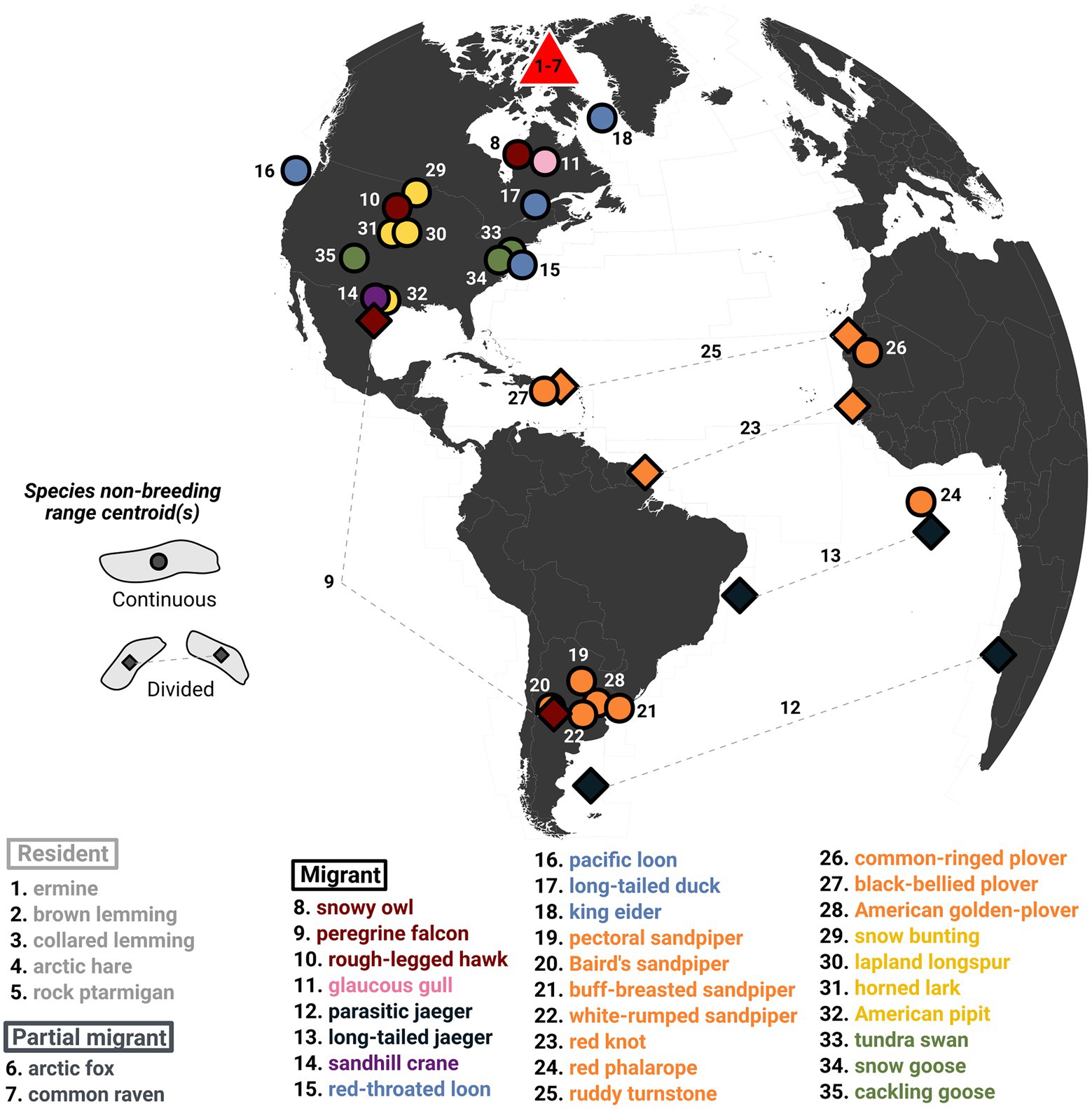
Figure 4. Non-breeding range centroids of vertebrate species from Bylot Island. The migratory species are colored by functional groups (red = raptors, pink = gulls, purple = cranes, dark blue = jaegers, light blue = ducks and loons, orange = shorebirds, yellow = passerines, green = geese and swans). The non-breeding centroid of residents and partial migrants are not represented since they are centered on Bylot Island. The centroids of migratory species with a continuous non-breeding range are represented by a circle, and squares are used to represent the centroids of divided non-breeding ranges (i.e., jaegers travel to both Atlantic coasts). The location of some centroids was slightly adjusted to better represent the species non-breeding habitat type, for instance, moving the centroid of a coastal species falling inland to the closest coastline.
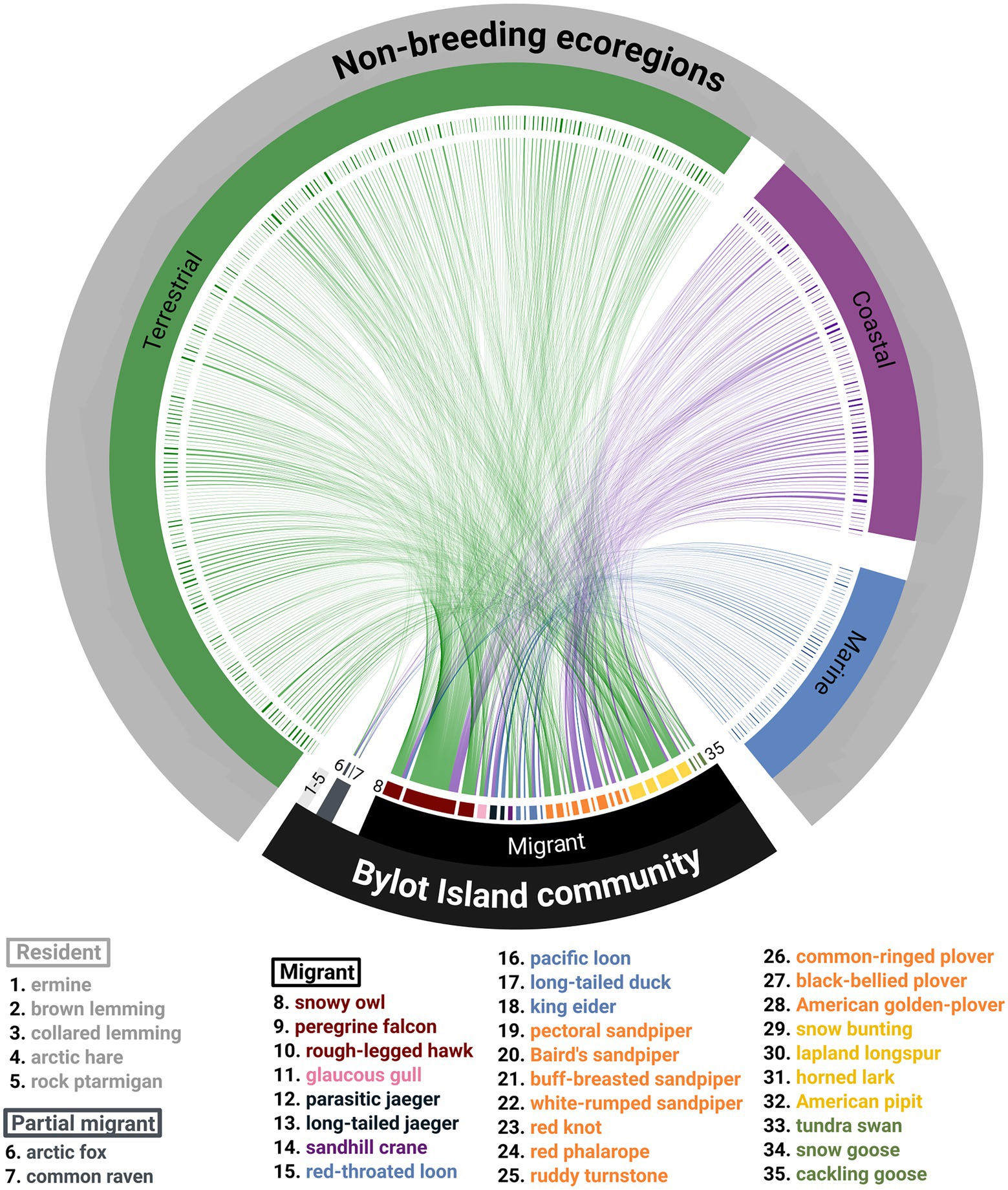
Figure 5. Community migration network of Bylot Island with the 35 species labeled in an anticlockwise way and the 393 biogeographic ecoregions colored by type (green = terrestrial, purple = coastal and blue = marine). Migratory species were colored based on their functional groups (red = raptors, pink = gulls, purple = cranes, dark blue = jaegers, light blue = ducks and loons, orange = shorebirds, yellow = passerines, green = geese and swans). The chord diagram representation was done with the R package circlize (Gu et al., 2014).
During the non-breeding period, the Bylot Island species are distributed in many ecoregions with a relatively low spatial overlap, which is further illustrated by the low connectance of the network (C = 0.09). The low asymmetry (W = −0.84) of the network is due to a large number of ecoregions (393) connected with the focal community compared to the number of vertebrate species present (35). The network presents a significantly lower nestedness than random networks (T = 8.36 p < 0.001).
The low spatial overlap among migratory species of Bylot Island during the non-breeding period is partly due to the presence of groups of species associated with common ecoregions (modules) during the non-breeding period (M = 6.32, p < 0.001; Figure 6 and Supplementary material Table S4 for the list of ecoregions and their assigned module). The modularity analysis revealed nine modules consisting of one to 13 species and six to 126 ecoregions. Among those modules, four are composed of a single species located: in the marine Pacific Northeast (pacific loon), marine and coastal North Atlantic (king eider), coastal and terrestrial Northwest Africa (common-ringed plover) and throughout the Americas (peregrine falcon). Long-tailed jaeger and red phalarope are associated with marine ecoregions along West Africa and parasitic jaeger, ruddy turnstone, red knot and black-bellied plover are associated with coastal ecoregions from both Atlantic coasts. A group of five shorebird species is associated with terrestrial and coastal ecoregions of the southern part of South America. The partially migratory species (common raven and Arctic fox) are identified as a distinct module. The module with the highest species and functional richness includes 13 migratory species associated with diverse environments (coastal, terrestrial and marine) across North America.
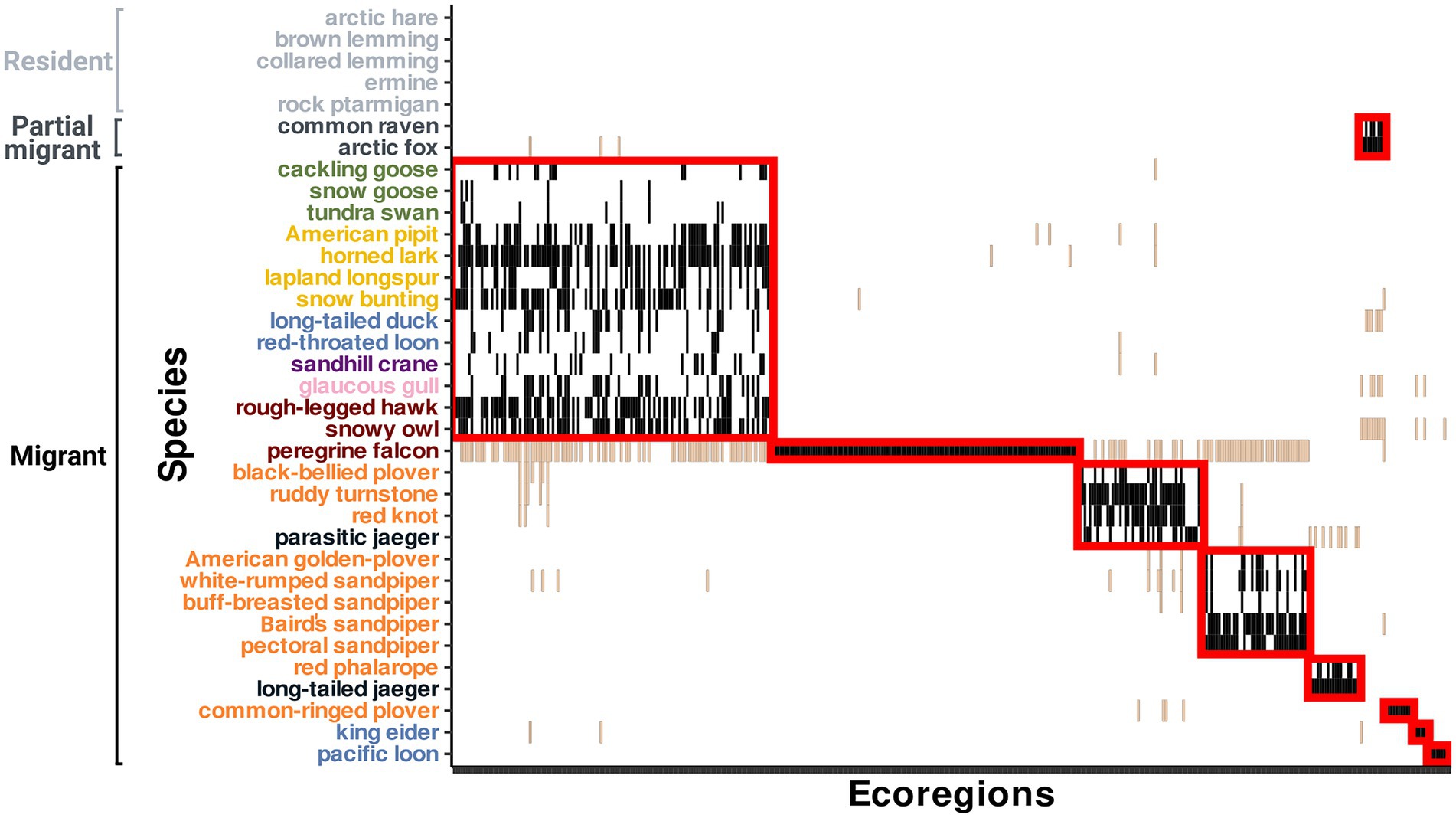
Figure 6. Partition of the Bylot Island community migration network with the highest modularity based on the infomapecology algorithm (M = 6.32, p < 0.001), with nine modules of species and ecoregions sharing more edges between them than with other species and ecoregions of the network. Modules are represented by red boxes and can include multiple ecoregions and species. Interactions non-associated with a module are represented in light brown and are not included in boxes. Species and ecoregions are ordered by modules and species labels are colored by functional groups (red = raptors, pink = gulls, purple = cranes, dark blue = jaegers, light blue = ducks and loons, orange = shorebirds, yellow = passerines, green = geese and swans).
The modularity analysis performed with the Bylot Island community migration network allows us to complement existing food web models by linking migratory pathways with trophic interactions in the community (Figure 7). We illustrate that the shorebirds functional group composed of 10 species in the Bylot Island food web is divided into four modules containing at least one species of shorebirds using distinct ecoregions during the non-breeding period. Therefore, the effect of high goose abundance driven by agricultural perturbations in temperate North America has the potential to propagate indirectly at the meta-community level to different sets of ecoregions through trophic interactions in the Arctic.
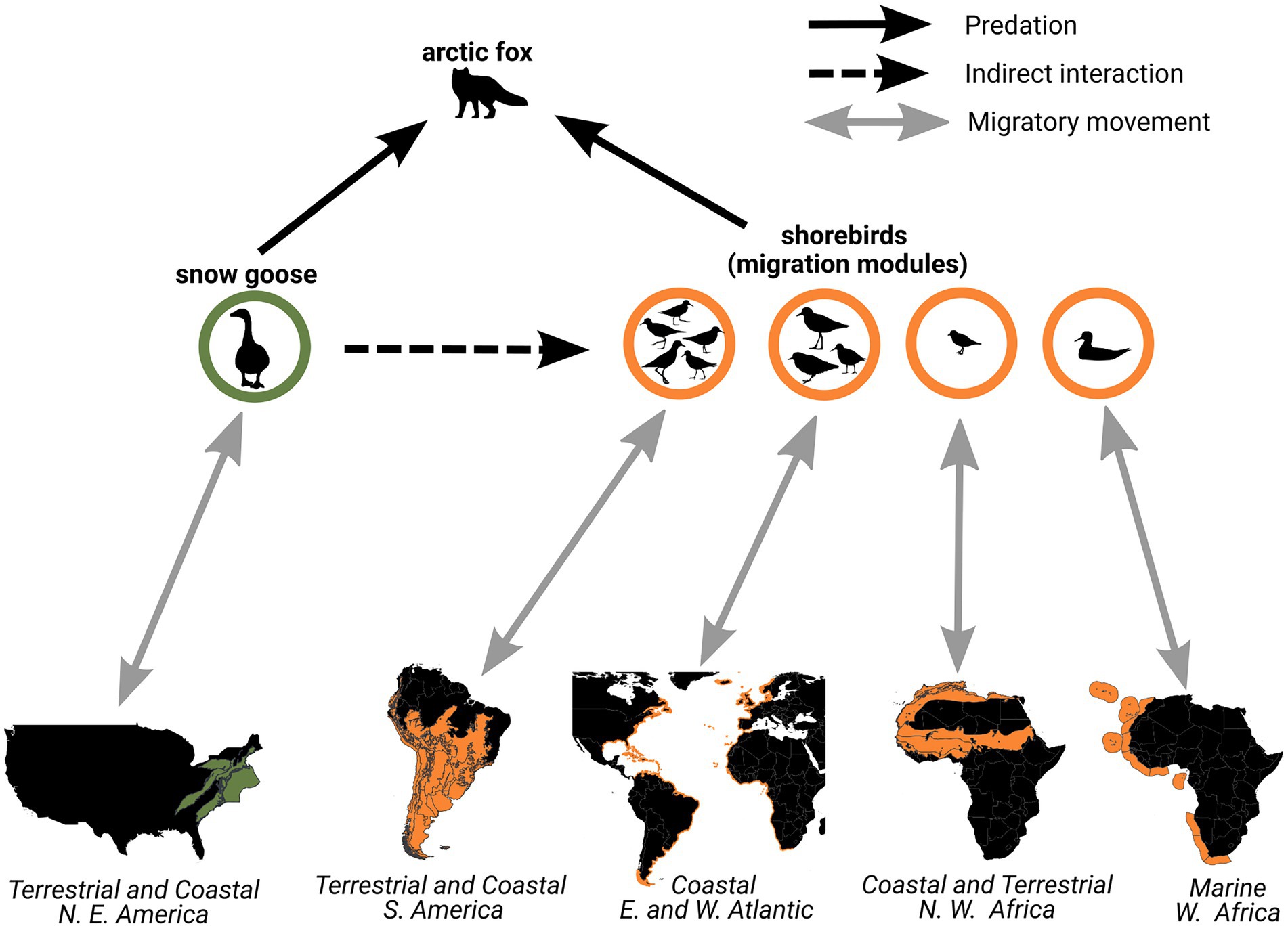
Figure 7. Snow geese and shorebirds breeding on Bylot Island share a predator (Arctic fox), which may result in the spatial propagation of agricultural changes occurring in temperate ecoregions used by geese during the non-breeding period (green) to ecoregions used by shorebirds during the non-breeding period (orange). The functional group of shorebirds is divided into four circles representing the modules in the community migration network and the extent of their associated ecoregions is shown on the maps. Negative indirect effects of geese on shorebirds could also pass-through overgrazing on the breeding grounds, which reduces nest site quality or availability.
5. Discussion
We proposed a framework with simple network metrics to characterize the structure of migratory pathways maintained by species of a focal community and provided the first empirical description for a vertebrate community. We highlighted that through the annual movement of migratory species, terrestrial Arctic communities could potentially be indirectly exposed to perturbations occurring in hundreds of ecoregions distributed across continents. The modular structure of migratory pathways observed at the community level suggests that distant perturbations could propagate to Arctic communities through subgroups of migratory species. Community migration networks can be used as a starting point to better study how distant perturbations can propagate at the community level through migratory species.
In our case study, the combination and centralization of migratory pathways knowledge in a community-based migration network allowed us to fill empirical gaps that could not previously be addressed on the spatial overlap of migratory species during the non-breeding period. The functional diversity observed in some of the species groups associated with distinct sets of ecoregions suggests that perturbations in certain regions of the globe are more likely to propagate to the focal community through multiple trophic levels. For example, a perturbation that would occur in a terrestrial ecoregion in temperate Northeastern America could simultaneously affect herbivorous, insectivorous and vertivorous species breeding on Bylot Island in the High Canadian Arctic. In contrast, a perturbation in a terrestrial ecoregion in northwest Africa could affect only one insectivore. On the other hand, spatial cascades that would spread through the community via migratory herbivores (geese and swans) could only originate from a single module composed of temperate ecoregions. As they are widely spread during the non-breeding period, the guild of avian predators would be expected to respond to perturbations occurring at a large (continental) scale. In some cases, species with similar trophic positions in the Bylot Island food web, like shorebirds, can face highly heterogeneous environmental conditions during the non-breeding period. Our results showed that high spatial overlap can occur for both functionally similar and functionally different species, but at the community level species have very little spatial overlap during the non-breeding period. These empirical advances will help to assess the vulnerability of the Bylot Island food web to global changes.
Spatial cascades via migratory species have been described in well-known cases of animal migration, highlighting the diversity of mechanisms by which migratory species can propagate perturbations. Perturbations at geese non-breeding and staging grounds can alter nutrient subsidies (Hessen et al., 2017), trophic subsidies and associated indirect species interactions (Lamarre et al., 2017; Duchesne et al., 2021), and even cause drastic shifts in communities and ecosystems used for breeding (Jefferies et al., 2004). Springer et al. (2018) showed that hatchery-induced increases in salmon populations led to a decrease in available food for short-tailed shearwaters (Ardenna tenuirostris) at their non-breeding grounds, leading to a reduction in population size at their breeding grounds. As a result, nutrient subsidies would be reduced and this could lead to cascading changes in the insular communities used for breeding. In the well-known Seregenti system, Holdo et al. (2009) showed that a change in the population size of migratory wildebeest (Connochaetes taurinus) can influence fire frequency and forest cover at the meta-ecosystem level. Perturbations do not act in isolation in communities and may interact with each other and cause additive, synergistic, or antagonistic effects at the community level (Beauchesne et al., 2021). The diverse effects of migratory species on communities and ecosystems (Bauer and Hoye, 2014) highlight the difficulty of assessing the net effect of perturbations, which currently limits the applications of our approach.
The modular (non-random) network structure observed in our case study suggests that evolutionary and geographic constraints as well as ecological factors potentially shape the structure of migratory pathways at the community level. The proportion of migratory species is strongly correlated with the magnitude of seasonality, at least for terrestrial bird communities (Hurlbert and Haskell, 2003; Somveille et al., 2013). Also, migratory birds tend to relocate from breeding to non-breeding grounds by minimizing migration distance to reach suitable grounds in terms of climate and resources, akin to a refuge from the harsher conditions of the breeding ground (Somveille et al., 2015, 2019, 2021; Bonnet-Lebrun et al., 2021). Unsurprisingly, most migratory species from Bylot Island reach non-breeding grounds in temperate environments (intermediate migration distance from the focal community), suggesting an influence of community geographical location on the structure of migratory pathways. Somveille et al. (2019) also observed that trans-hemispheric migrants did not seem to respond to the same ecological drivers as migrants travelling within the same hemisphere. Interestingly, in the community migration network of Bylot Island, we observed a clear distinction between the functional groups performing trans-hemispheric migrations (shorebirds, jaegers and some raptors) and those who do not (geese and swans, passerines, cranes, gulls and ducks and loons and some raptors). The presence of modules composed of functionally similar species associated with common habitats (e.g., shorebirds in South American ecoregions) suggests that adaptive constraints on flight efficiency, environmental tolerance and diet may influence network structure by increasing the modularity. We could address the influence of phylogeny on migrations at the community level by linking phylogenetic distance, geographic distance and location of migratory destinations and species degree in the network. Within modules of functionally similar species, ecological factors such as interspecific interactions (mutualism or competition) could potentially increase or decrease the spatial overlap between species (Gotelli et al., 2010). Linking community and species traits, geography, phylogeny and species interactions with community migration network metrics will allow a better understanding of the factors and constraints shaping migratory patterns at the community level.
Ideally, community migration networks would be defined for focal communities with well-known geographic ranges and movements over their entire annual cycle for each migratory population. In our study, we estimated the non-breeding range of migratory species with the most accurate data available for our focal community. Despite the use of some rough non-breeding range maps, we still observed a highly structured network. We avoided interpretations at the node level (e.g., degree of ecoregions and species) because we used coarse spatial data for several species. Our approach includes two sources of measurement uncertainty, the sampling of the community assemblage and the delineation of species ranges. We did not quantify either of these sources and therefore do not know the uncertainty of the observed metrics. A community surveyed with little effort would underestimate the number of migratory species, which could potentially influence network metrics in both directions (higher or lower). However, in our case, the surveyed community was heavily sampled. Despite the small sample size of our validation analysis, it suggests that using refined species range maps tends to overestimate the number of connected ecoregions, which could also influence network metrics in both directions. Developing an approach to measure these two sources of uncertainty, as well as their effects on community migration network metrics is a gap that needs to be filled in order to compare the structure of migratory pathways between communities. Moreover, the proposed framework focuses on a single community, which prevents encompassing the complete spatial patterns at the species level. For instance, in our case study, we focused on a single breeding ground, which prevents the integration of population spread from non-breeding to breeding grounds. We did not include stopovers and staging grounds because data were too scarce, despite their recognized importance in migratory species demography (Newton, 2006; Iwamura et al., 2013). The ongoing refinement of range maps for migratory species with consideration of the spatiotemporal patterns of population distribution (Smith et al., 2022; Smithsonian Migratory Bird Center, Georgetown University, National Audubon Society and the Cornell Lab of Ornithology, 2022) will reduce uncertainty and broaden opportunities to develop more accurate and precise community migration networks.
Community migration networks represent a simple conceptual framework that should be further developed by network ecologists to study migrations at the community level. The seasonal nature of migratory movements represents theoretical and methodological challenges to scale migrations at the community level. For instance, seasonal edges (temporally discontinuous) in a network of interacting local populations imply a time-delayed response of perturbations between nodes, which is often not considered in ecological models. As a result, we currently have limited knowledge of the effect of the ephemeral and predictable (seasonal) pulses of migratory organisms on community stability and resilience (Holdo et al., 2011; Jeltsch et al., 2013; Bauer et al., 2016; Furey et al., 2018). In our study system, it has been shown that the seasonal presence of migrants can substantially change the dynamic of the food web (Hutchison et al., 2020). It is important to note that the traditional network metrics we used have not been developed with networks comprising seasonal nodes and edges. We may have to develop specific metrics of community migration networks more relevant to the biology of migratory species. This could include centrality metrics that better represent the potential of species to spatially propagate perturbations and cause local cascading effects based on the population spread (species degree), the sensibility to perturbations, the degree of migratory connectivity between populations and the position in the food web.
We restricted our study to a descriptive case of a community migration network with a single breeding site. However, the approach could be replicated in other communities and the framework could be adapted and further developed to better address various ecological questions. Replicating our approach with several communities and comparing their metrics may reveal global biogeographic patterns of migratory pathways at the community level. Migratory pathways of avian species tend to converge towards the poles and migratory species tend to minimize migration distance (Somveille et al., 2015, 2019, 2021; Bonnet-Lebrun et al., 2021), so the structure of community migration networks could possibly vary with latitude. Quantifying the flow of migratory organisms in community migration networks would allow for a better representation of connections at the meta-level, providing a more direct way to link migrations to ecosystem and community processes. This could be done by weighting edges with biomass to address energy, nutrient, and contaminant exchanges or by weighting edges with abundance to address parasite and disease exchanges and trophic effects. Since migratory species are important vectors of parasites and diseases (Tian et al., 2015; Viana et al., 2016; Varpe and Bauer, 2022), there may be interest in using community migration networks to address how these spread between distant regions of the globe through a common focal site used by multiple migratory species. Combining ecological (food webs, plant-pollinators and host-parasites) and migration networks within a single framework would allow a better representation of the net effects of perturbations in meta-communities maintained by migrations. This could involve integrating species traits and trophic position into community migration networks to identify distant regions and species more likely to generate spatial cascades. Scaling migrations at the community level is a first step towards assessing community vulnerability to changes occurring in distant locations connected through animal movement. To practically use our approach in a perturbation propagation context (e.g., conservation purposes), we will need to examine the relationship between community migration network metrics and community sensitivity, resilience, and vulnerability to distant changes. The development of community migration networks should thus improve our ability to anticipate the effects of global changes on ecosystems.
Data availability statement
Publicly available datasets were analyzed in this study. The data can be found here: eBird range maps: Long-tailed Jaegers: https://www.movebank.org Study ID = 1978212368.
Common-ringed plovers: https://www.movebank.org, Study ID = 849807214.
American Golden-Plovers: https://www.movebank.org, Study ID = 565443493.
Snowy owls: https://www.movebank.org, Study ID = 12112706.
King eiders: https://www.movebank.org, Study ID = 43747715.
Terrestrial Ecoregions of the World: https://www.worldwildlife.org/publications/terrestrial-ecoregions-of-the-world.
Freshwater Ecoregions of the World: https://www.feow.org/download.
Marine and Coastal Ecoregions of the World: https://www.worldwildlife.org/publications/marine-ecoregions-of-the-world-a-bioregionalization-of-coastal-and-shelf-areas.
shelf-areas Major Flyways of the World: https://wpp.wetlands.org/downloads/downloads.
Scripts for data manipulation, analysis, and visualization can be found at: https://github.com/Louis-Moisan/Community_Migration_Networks. All other data are available on request from the corresponding author.
Ethics statement
Ethical review and approval was not required for the animal study because the study is based on published datasets approved by animal care committee.
Author contributions
JB, DG, and LM: designed the study, developed the conceptual approach, and analyzed the data. GG, JB, and PL: secured the funding for the long-term monitoring program. J-FL, J-FT, D-JL-B, GG, JB, and PL: collected the tracking data. J-FL, J-FT, and D-JL-B: process the raw tracking data. LM: manipulated the data. LM, JB, DG, and MS contributed to the interpretation of the results. LM wrote the first draft of the manuscript with contributions from all authors. All authors contributed and approved the submitted version.
Funding
This project was funded by (alphabetical order): Arctic Goose Joint Venture, Arctic Net, BIOS2 (NSERC CREATE Training Programs), Canadian Wildlife Service, EnviroNord (NSERC CREATE Training Program in Northern Environmental Sciences), Fonds de recherche du Québec - Nature et technologies (FRQNT), Indigenous and Northern Affairs Canada, Natural Resources Canada (PCSP), Natural Science and Engineering Research Council of Canada (NSERC), Nunavut Wildlife Management Board, Polar Knowledge Canada, Université Laval, Université du Québec à Rimouski and Centre d’Études Nordiques.
Acknowledgments
We are sincerely grateful for the data collected with a lot of effort from multiple researchers across years of fieldwork at the Bylot Island research station that made this project possible, especially Y. Seyer and A. Robillard that provided tracking data. We are thankful to eBird and BirdLife International for providing us the access to those valuable datasets. We would also like to thank the Center of Northern Studies for their research installations and the Polar Continental Shelf Program and the Sirmilik National Park (Parks Canada) for the logistic help throughout this project. We would also like to especially thank the Mittimatalik community and the Mittimatalik Hunters & Trappers Organization for their long-term support of the Bylot Island ecological monitoring and for allowing us to pursue research on their land. Finally, we thanked the reviewers for their constructive comments that contributed to the quality of this manuscript.
Conflict of interest
The authors declare that the research was conducted in the absence of any commercial or financial relationships that could be construed as a potential conflict of interest.
The handling editor M-JF declared a past co-authorship with the author DG.
Publisher’s note
All claims expressed in this article are solely those of the authors and do not necessarily represent those of their affiliated organizations, or those of the publisher, the editors and the reviewers. Any product that may be evaluated in this article, or claim that may be made by its manufacturer, is not guaranteed or endorsed by the publisher.
Supplementary material
The Supplementary material for this article can be found online at: https://www.frontiersin.org/articles/10.3389/fevo.2022.1077260/full#supplementary-material
References
Bauer, S., and Hoye, B. J. (2014). Migratory animals couple biodiversity and ecosystem functioning worldwide. Science 344:1242552. doi: 10.1126/science.1242552
Bauer, S., Lisovski, S., and Hahn, S. (2016). Timing is crucial for consequences of migratory connectivity. Oikos 125, 605–612. doi: 10.1111/oik.02706
Beauchesne, D., Cazelles, K., Archambault, P., Dee, L. E., and Gravel, D. (2021). On the sensitivity of food webs to multiple stressors. Ecol. Lett. 24, 2219–2237. doi: 10.1111/ele.13841
BirdLife International and Handbook of the Birds of the World. (2019). Data from: bird species distribution maps of the world version 2019.1. (Available at: http://datazone.birdlife.org/species/requestdis (Accessed May 1, 2020).
Bonnet-Lebrun, A. S., Somveille, M., Rodrigues, A. S., and Manica, A. (2021). Exploring intraspecific variation in migratory destinations to investigate the drivers of migration. Oikos 130, 187–196. doi: 10.1111/oik.07689
Chapman, B. B., Brönmark, C., Nilsson, J. Å., and Hansson, L. A. (2011). The ecology and evolution of partial migration. Oikos 120, 1764–1775. doi: 10.1111/j.1600-0706.2011.20131.x
Clermont, J., Grenier-Potvin, A., Duchesne, É., Couchoux, C., Dulude-de Broin, F., Beardsell, A., et al. (2021). The predator activity landscape predicts the anti-predator behavior and distribution of prey in a tundra community. Ecosphere 12:e03858. doi: 10.1002/ecs2.3858
Dale, M., and Fortin, M. J. (2010). From graphs to spatial graphs. Annu. Rev. Ecol. Evol. Syst. 41, 21–38. doi: 10.1146/annurev-ecolsys-102209-144718
Dormann, C. F., Fründ, J., Blüthgen, N., and Gruber, B. (2009). Indices, graphs and null models: analyzing bipartite ecological networks. Open Ecol. J. 2, 7–24. doi: 10.2174/1874213000902010007
Dormann, C. F., Gruber, B., and Fründ, J. (2008). Introducing the bipartite package: analysing ecological networks. R News 8, 8–11.
Duchesne, É., Lamarre, J.-F., Gauthier, G., Berteaux, D., Gravel, D., and Bêty, J. (2021). Variable strength of predator-mediated effects on species occurrence in an arctic terrestrial vertebrate community. Ecography 44, 1236–1248. doi: 10.1111/ecog.05760
Dunne, J. A., Williams, R. J., and Martinez, N. D. (2002). Food-web structure and network theory: the role of connectance and size. Proc. Natl. Acad. Biol. Sci. U. S. A. 99, 12917–12922. doi: 10.1073/pnas.192407699
Farage, C., Edler, D., Eklöf, A., Rosvall, M., and Pilosof, S. (2021). Identifying flow modules in ecological networks using infomap. Methods Ecol. Evol. 12, 778–786. doi: 10.1111/2041-210X.13569
Finch, T., Butler, S. J., Franco, A. M., and Cresswell, W. (2017). Low migratory connectivity is common in long-distance migrant birds. J. Anim. Ecol. 86, 662–673. doi: 10.1111/1365-2656.12635
Fink, D., Auer, T., Johnston, A., Strimas-Mackey, M., Robinson, O., Ligocki, S., et al. (2020). Data from: eBird Status and Trends, Data Version: 2019; Released: 2020. Ithaca, New York: Cornell Lab of Ornithology.
Fortuna, M. A., Stouffer, D. B., Olesen, J. M., Jordano, P., Mouillot, D., Krasnov, B. R., et al. (2010). Nestedness versus modularity in ecological networks: two sides of the same coin? J. Anim. Ecol. 79, 811–817. doi: 10.1111/j.1365-2656.2010.01688.x
Furey, N. B., Armstrong, J. B., Beauchamp, D. A., and Hinch, S. G. (2018). Migratory coupling between predators and prey. Nat. Ecol. Evol. 2, 1846–1853. doi: 10.1038/s41559-018-0711-3
García-Callejas, D., Molowny-Horas, R., Araújo, M. B., and Gravel, D. (2019). Spatial trophic cascades in communities connected by dispersal and foraging. Ecology 100:e02820. doi: 10.1002/ecy.2820
Gauthier, G., Berteaux, D., Bêty, J., Tarroux, A., Therrien, J.-F., McKinnon, L., et al. (2011). The tundra food web of bylot island in a changing climate and the role of exchanges between ecosystems. Écoscience 18, 223–235. doi: 10.2980/18-3-3453
Gauthier, G., Bêty, J., Cadieux, M. C., Legagneux, P., Doiron, M., Chevallier, C., et al. (2013). Long-term monitoring at multiple trophic levels suggests heterogeneity in responses to climate change in the Canadian Arctic tundra. Philos. Trans. R. Soc. B Biol. Sci. 368:20120482. doi: 10.1098/rstb.2012.0482
Gauthier, G., Giroux, J.-F., Reed, A., Béchet, A., and Bélanger, L. (2005). Interactions between land use, habitat use, and population increase in greater snow geese: what are the consequences for natural wetlands? Glob. Change Biol. 11, 856–868. doi: 10.1111/j.1365-2486.2005.00944.x
Gauthier, G., Hughes, R. J., Reed, A., Beaulieu, J., and Rochefort, L. (1995). Effect of grazing by greater snow geese on the production of graminoids at an arctic site (Bylot Island, NWT, Canada). J. Ecol. 83, 653–664. doi: 10.2307/2261633
Gilchrist, G., Mosbech, A., and Sonne, C. (2004). Data available on movebank.Org, study name common/king eiders; East Bay island, Nunavut; Gilchrist/Mosbech/Sonne 2001 and 2003, study ID 43747715.
Giroux, M.-A., Berteaux, D., Lecomte, N., Gauthier, G., Szor, G., and Bêty, J. (2012). Benefiting from a migratory prey: spatio-temporal patterns in allochthonous subsidization of an arctic predator. J. Anim. Ecol. 81, 533–542. doi: 10.1111/j.1365-2656.2011.01944.x
Gotelli, N. J., Graves, G. R., and Rahbek, C. (2010). Macroecological signals of species interactions in the Danish avifauna. Proc. Natl. Acad. Sci. U. S. A. 107, 5030–5035. doi: 10.1073/pnas.0914089107
Gounand, I., Harvey, E., Little, C. J., and Altermatt, F. (2018). Meta-ecosystems 2.0: rooting the theory into the field. Trends Ecol. Evol. 33, 36–46. doi: 10.1016/j.tree.2017.10.006
Gu, Z., Gu, L., Eils, R., Schlesner, M., and Brors, B. (2014). Circlize implements and enhances circular visualization in r. Bioinformatics 30, 2811–2812. doi: 10.1093/bioinformatics/btu393
Hessen, D. O., Tombre, I. M., van Geest, G., and Alfsnes, K. (2017). Global change and ecosystem connectivity: how geese link fields of Central Europe to eutrophication of Arctic freshwaters. Ambio 46, 40–47. doi: 10.1007/s13280-016-0802-9
Holdo, R. M., Holt, R. D., Sinclair, A. R., Godley, B. J., and Thirgood, S. (2011). “Migration impacts on communities and ecosystems: empirical evidence and theoretical insights” in Animal Migration: A Synthesis. eds. E. Milner-Gulland, J. M. Fryxell, and A. R. Sinclair (Oxford: Oxford University Press)
Holdo, R. M., Sinclair, A. R., Dobson, A. P., Metzger, K. L., Bolker, B. M., Ritchie, M. E., et al. (2009). A disease-mediated trophic cascade in the Serengeti and its implications for ecosystem C. PLoS Biol. 7:e1000210. doi: 10.1371/journal.pbio.1000210
Hurlbert, A. H., and Haskell, J. P. (2003). The effect of energy and seasonality on avian species richness and community composition. Am. Nat. 161, 83–97. doi: 10.1086/345459
Hutchison, C., Guichard, F., Legagneux, P., Gauthier, G., Bêty, J., Berteaux, D., et al. (2020). Seasonal food webs with migrations: multi-season models reveal indirect species interactions in the Canadian Arctic tundra. Philos. Trans. Royal Soc. A 378:20190354. doi: 10.1098/rsta.2019.0354
Iwamura, T., Possingham, H. P., Chadès, I., Minton, C., Murray, N. J., Rogers, D. I., et al. (2013). Migratory connectivity magnifies the consequences of habitat loss from sea-level rise for shorebird populations. Philos. Trans. R. Soc. B Biol. Sci. 280:20130325. doi: 10.1098/rspb.2013.0325
Jefferies, R., Rockwell, R., and Abraham, K. (2004). Agricultural food subsidies, migratory connectivity and large-scale disturbance in arctic coastal systems: a case study. Integr. Comp. Biol. 44, 130–139. doi: 10.1093/icb/44.2.130
Jeltsch, F., Bonte, D., Pe'er, G., Reineking, B., Leimgruber, P., Balkenhol, N., et al. (2013). Integrating movement ecology with biodiversity research-exploring new avenues to address spatiotemporal biodiversity dynamics. Mov. Ecol. 1, 1–13. doi: 10.1186/2051-3933-1-6
Knight, S. M., Bradley, D. W., Clark, R. G., Gow, E. A., Bélisle, M., Berzins, L. L., et al. (2018). Constructing and evaluating a continent-wide migratory songbird network across the annual cycle. Ecol. Monogr. 88, 445–460. doi: 10.1002/ecm.1298
Lai, S., Bêty, J., and Berteaux, D. (2017). Movement tactics of a mobile predator in a meta-ecosystem with fluctuating resources: the Arctic fox in the high Arctic. Oikos 126, 937–947. doi: 10.1111/oik.03948
Lamarre, J. F., Gauthier, G., Lanctot, R. B., Saalfeld, S. T., Love, O. P., Reed, E., et al. (2021). Timing of breeding site availability across the north American Arctic partly determines spring migration schedule in a long-distance Neotropical migrant. Front. Ecol. Evol. 9:710007. doi: 10.3389/fevo.2021.710007
Lamarre, J. F., Legagneux, P., Gauthier, G., Reed, E. T., and Bêty, J. (2017). Predator-mediated ne- gative effects of overabundant snow geese on arctic-nesting shorebirds. Ecosphere 8:e01788. doi: 10.1002/ecs2.1788
Léandri-Breton, D.-J., Lamarre, J.-F., and Bêty, J. (2019). Seasonal variation in migration strategies used to cross ecological barriers in a nearctic migrant wintering in Africa. J. Avian Biol. 50:e02101. doi: 10.1111/jav.02101
Legagneux, P., Gauthier, G., Berteaux, D., Bêty, J., Cadieux, M.-C., Bilodeau, F., et al. (2012). Disentangling trophic relationships in a High Arctic tundra ecosystem through food web modeling. Ecology 93, 1707–1716. doi: 10.1890/11-1973.1
McCann, K. S., Rasmussen, J. B., and Umbanhowar, J. (2005). The dynamics of spatially coupled food webs. Ecol. Lett. 8, 513–523. doi: 10.1111/j.1461-0248.2005.00742.x
Miklós, I., and Podani, J. (2004). Randomization of presence–absence matrices: comments and new algorithms. Ecology 85, 86–92. doi: 10.1890/03-0101
Mueller, T., and Fagan, W. F. (2008). Search and navigation in dynamic environments–from individual behaviors to population distributions. Oikos 117, 654–664. doi: 10.1111/j.0030-1299.2008.16291.x
Newton, I. (2006). Can conditions experienced during migration limit the population levels of birds? Journal of Ornithology 147, 146–166. doi: 10.1007/s10336-006-0058-4
Newton, I., and Dale, L. C. (1996). Bird migration at different latitudes in eastern North America. Auk 113, 626–635. doi: 10.2307/4088983
Norris, D. R. (2005). Carry-over effects and habitat quality in migratory populations. Oikos 109, 178–186. doi: 10.1111/j.0030-1299.2005.13671.x
Oksanen, J., Simpson, G., Blanchet, F., Kindt, R., Legendre, P., Minchin, P., et al. (2022). Vegan: community ecology package version 2.6-2. Available online at: https://CRAN.R-project.org/package=vegan
Olson, D. M., Dinerstein, E., Wikramanayake, E. D., Burgess, N. D., Powell, G. V., Underwood, E. C., et al. (2001). Terrestrial ecoregions of the world: a new map of life on earth: new global map of terrestrial ecoregions provides an innovative tool for conserving biodiversity. Bioscience 51, 933–938. doi: 10.1641/0006-3568(2001)051[0933:TEOTWA]2.0.CO;2
Pebesma, E. (2018). Simple features for R: standardized support for spatial vector data. R J. 10, 439–446. doi: 10.32614/RJ-2018-009
R Core Team (2020). R: A Language and Environment for Statistical Computing version 4.2.1. R Foundation for Statistical Computing. Vienna, Austria: R Core Team.
Robillard, A., Gauthier, G., Therrien, J.-F., and Bêty, J. (2018). Wintering space use and site fidelity in a nomadic species, the snowy owl. J. Avian Biol. 49:01707. doi: 10.1111/jav.01707
Rodríguez-Gironés, M. A., and Santamaría, L. (2006). A new algorithm to calculate the nestedness temperature of presence–absence matrices. J. Biogeogr. 33, 924–935. doi: 10.1111/j.1365-2699.2006.01444.x
Rubenstein, D. R., and Hobson, K. A. (2004). From birds to butterflies: animal movement patterns and stable isotopes. Trends Ecol. Evol. 19, 256–263. doi: 10.1016/j.tree.2004.03.017
Seyer, Y., Gauthier, G., Bêty, J., Therrien, J.-F., and Lecomte, N. (2021). Seasonal variations in migration strategy of a long-distance Arctic-breeding seabird. Mar. Ecol. Prog. Ser. 677, 1–16. doi: 10.3354/meps13905
Smith, J. R., Letten, A. D., Ke, P. J., Anderson, C. B., Hendershot, J. N., Dhami, M. K., et al. (2018). A global test of ecoregions. Nat. Ecol. Evol. 2, 1889–1896. doi: 10.1038/s41559-018-0709-x
Smith, M. A., Mahoney, J., Knight, E. J., Taylor, L., Seavy, N. E., Bailey, O. H., et al. (2022). Bird migration explorer. National Audubon Society Available at: Birdmigrationexplorer.org (Accessed September 20, 2022).
Smithsonian Migratory Bird Center, Georgetown University, National Audubon Society and the Cornell Lab of Ornithology (2022). The migratory connectivity project - the atlas of migratory connectivity for the birds of North America. Available at: http://migratoryconnectivityproject.org/atlas-of-migratory-connectivity/ (Accessed August 29, 2022).
Somveille, M., Bay, R. A., Smith, T. B., Marra, P. P., and Ruegg, K. C. (2021). A general theory of avian migratory connectivity. Ecol. Lett. 24, 1848–1858. doi: 10.1111/ele.13817
Somveille, M., Manica, A., Butchart, S. H. M., and Rodrigues, A. S. L. (2013). Mapping global diversity patterns for migratory birds. PLoS One 8:e70907. doi: 10.1371/journal.pone.0070907
Somveille, M., Manica, A., and Rodrigues, A. S. L. (2019). Where the wild birds go: explaining the differences in migratory destinations across terrestrial bird species. Ecography 42, 225–236. doi: 10.1111/ecog.03531
Somveille, M., Rodrigues, A. S. L., and Manica, A. (2015). Why do birds migrate? A macroecological perspective. Glob. Ecol. Biogeogr. 24, 664–674. doi: 10.1111/geb.12298
Spalding, M. D., Fox, H. E., Allen, G. R., Davidson, N., Ferdaña, Z. A., Finlayson, M., et al. (2007). Marine ecoregions of the world: a bioregionalization of coastal and shelf areas. Bioscience 57, 573–583. doi: 10.1641/B570707
Springer, A. M., van Vliet, G. B., Bool, N., Crowley, M., Fullagar, P., Lea, M. A., et al. (2018). Transhemispheric ecosystem disservices of pink salmon in a pacific ocean macrosystem. Proc. Natl. Acad. Sci. U. S. A. 115, E5038–E5045. doi: 10.1073/pnas.1720577115
Taylor, C. M. (2019). Effects of natal dispersal and density-dependence on connectivity patterns and population dynamics in a migratory network. Front. Ecol. Evol. 7:354. doi: 10.3389/fevo.2019.00354
Taylor, C. M., and Norris, D. R. (2010). Population dynamics in migratory networks. Theor. Ecol. 3, 65–73. doi: 10.1007/s12080-009-0054-4
Therrien, J.-F., Gauthier, G., and Bêty, J. (2012). Survival and reproduction of adult snowy owls tracked by satellite. J. Wildl. Manag. 76, 1562–1567. doi: 10.1002/jwmg.414
Tian, H., Zhou, S., Dong, L., Van Boeckel, T. P., Cui, Y., Newman,, et al. (2015). Avian influenza H5N1 viral and bird migration networks in Asia. Proc. Natl. Acad. Biol. Sci. U. S. A. 112, 172–177. doi: 10.1073/pnas.1405216112
Varpe, Ø., and Bauer, S. (2022). “Seasonal animal migrations and the Arctic: ecology, diversity, and spread of infectious agents,” in Arctic One Health. ed. M. Tryland (Cham: Springer).
Viana, D. S., Santamaría, L., and Figuerola, J. (2016). Migratory birds as global dispersal vectors. Trends Ecol. Evol. 31, 763–775. doi: 10.1016/j.tree.2016.07.005
Webster, M. S., Marra, P. P., Haig, S. M., Bensch, S., and Holmes, R. T. (2002). Links between worlds: unraveling migratory connectivity. Trends Ecol. Evol. 17, 76–83. doi: 10.1016/S0169-5347(01)02380-1
Wetlands International (2022). What are flyways. Available at: https://wpp.wetlands.org/background/WAF; https://wpp.wetlands.org/downloads/downloads (Accessed September 6, 2022).
Wickham, H., François, R., Henry, L., and Müller, K. (2021). Dplyr: a grammar of data manipulation version 1.0.9 Available at: https://CRAN.R-project.org/package=dplyr
Keywords: seasonal migration, meta-community, meta-ecosystem, migratory pathways, community migration network, ecological network, bipartite network, Arctic
Citation: Moisan L, Gravel D, Legagneux P, Gauthier G, Léandri-Breton D-J, Somveille M, Therrien J-F, Lamarre J-F and Bêty J (2023) Scaling migrations to communities: An empirical case of migration network in the Arctic. Front. Ecol. Evol. 10:1077260. doi: 10.3389/fevo.2022.1077260
Edited by:
Marie-Josee Fortin, University of Toronto, CanadaReviewed by:
Marie Auger-Methe, University of British Columbia, CanadaNigel Yoccoz, UiT The Arctic University of Norway, Norway
Courtney R. Shuert, Freshwater Institute, Fisheries and Oceans Canada (DFO), Canada
Copyright © 2023 Moisan, Gravel, Legagneux, Gauthier, Léandri-Breton, Somveille, Therrien, Lamarre and Bêty. This is an open-access article distributed under the terms of the Creative Commons Attribution License (CC BY). The use, distribution or reproduction in other forums is permitted, provided the original author(s) and the copyright owner(s) are credited and that the original publication in this journal is cited, in accordance with accepted academic practice. No use, distribution or reproduction is permitted which does not comply with these terms.
*Correspondence: Louis Moisan, ✉ Louis.Moisan@uqar.ca; Joël Bêty, ✉ joel_bety@uqar.ca