- 1Collaborative Innovation Center for Biomedicine, Shanghai University of Medicine and Health Sciences, Shanghai, China
- 2School of Arts and Sciences, Shanghai University of Medicine and Health Sciences, Shanghai, China
- 3Jiading District Central Hospital Affiliated Shanghai University of Medicine and Health Sciences, Shanghai, China
Rapid global biodiversity loss and increasing emerging infectious diseases underscore the significance of identifying the diversity-disease relationship. Although experimental evidence supports the existence of dilution effects in several natural ecosystems, we still know very little about the conditions under which a dilution effect will occur. Using a multi-host Susceptible-Infected-Recovered model, we found when disease transmission was density-dependent, the diversity-disease relationship could exhibit an increasing, decreasing, or non-monotonic trend, which mainly depended on the patterns of community assembly. However, the combined effects of the host competence-abundance relationship and species extinction order may reverse or weaken this trend. In contrast, when disease transmission was frequency-dependent, the diversity-disease relationship only showed a decreasing trend, the host competence-abundance relationship and species extinction order did not alter this decreasing trend, but it could reduce the detectability of the dilution effect and affect disease prevalence. Overall, a combination of disease transmission mode, community assembly pattern, and host community composition determines the direction or strength of the diversity-disease relationship. Our work helps explain why previous studies came to different conclusions about the diversity-disease relationship and provides a deeper understanding of the pathogen transmission dynamics in actual communities.
Introduction
In recent decades, emerging infectious diseases (primarily zoonotic diseases) have been increasing at an unprecedented rate, posing a severe threat to human health and affecting agricultural production and wildlife conservation (Allen et al., 2017; Gibb et al., 2020). At the same time, the increasing disturbance of ecosystems by human activities has accelerated the rate of extinction for species (Estrada-Peña et al., 2014). The biodiversity-disease relationship is one of the fundamental challenges in studying infectious disease ecology (Huang et al., 2016). Accurate prediction of infectious disease risk in the context of declining biodiversity is important both in theory and in practice.
Although the link between host species richness and disease risk has long been identified in entomology, veterinary medicine, and epidemiology (Elton, 1958), this relationship attracted the attention of ecologists only since last two decades. The dilution effect hypothesis, whereby increased host biodiversity reduces disease risk, was formally proposed in studies of Lyme disease transmission dynamics (Keesing et al., 2006). The dilution effect shows a win-win situation in public health and nature conservation, reflecting the service function of ecosystems (Ostfeld and Keesing, 2012). Although many cross-taxa and cross-system empirical studies have confirmed the existence of the dilution effect in nature (Ostfeld and Keesing, 2000; Johnson et al., 2013; Liu et al., 2016), and ecologists have summarized the mechanisms by which the dilution effect occurs (Keesing et al., 2006), there are still some debates about the diversity-disease relationship. Several studies have discovered that the relationship between diversity and disease risk is highly context-dependent (Liu et al., 2020; Cortez and Duffy, 2021), with some studies confirming no effect (Salkeld et al., 2013; Vadell et al., 2020) or even an amplification effect (increased host biodiversity increases disease risk) in some ecosystems (Wood, 2014; Halliday et al., 2017). Clarifying the conditions under which the dilution effect occurs not only helps to gain insight into the dynamics of disease transmission in multi-host communities but also provides a theoretical basis for the prevention and control of infectious diseases in the real world.
Host community composition is essential in determining the relationship between biodiversity and disease risk (Johnson et al., 2015). Due to factors such as life history traits and evolutionary history, host competence (the host’s ability to maintain and transmit disease) varies significantly among different species (Downs et al., 2019). The number and proportion of high competent hosts in a community directly determine the disease prevalence of the community, so community composition can affect community disease risk. In fact, changes in community composition are primarily determined by species extinction orders under community disassembly (LoGiudice et al., 2003). Deterministic and stochastic extinctions reflect different host competence-extinction risk relationships. In the case of deterministic extinction, low competent hosts go extinct first. Therefore, it is not difficult to predict that as species richness decreases, the proportion of high competent hosts will increase, leading to an increase in disease risk in the community, i.e., a dilution effect occurs. Both field and theoretical studies have confirmed that deterministic extinction of host species is an important mechanism for the dilution effect (Lacroix et al., 2014; Johnson et al., 2019). However, when host species go extinct at random, it is unclear whether host richness promotes or inhibits disease risk.
Many empirical studies support a negative correlation between host competence and extinction risk, which is considered necessary to cause a dilution effect. Taking Lyme disease as an example, the less competent Virginia opossum and chipmunk are first to go extinct when host species richness decreases, while the most competent white-footed mouse can be abundant in communities with low species richness (Ostfeld and Keesing, 2000; Keesing et al., 2010). Similarly, Johnson et al. (2013) found a non-random nested structure of amphibian community composition in 345 wetlands in California. High competent hosts appeared in almost all wetlands, and low competent hosts usually appeared only in wetlands with higher species richness. This phenomenon also exists in plant communities (Lind et al., 2013; Liu et al., 2017). Although the above examples support a negative correlation between host competence and extinction risk, the generality of this finding remains to be confirmed due to limitations in field observations and experimental conditions (Rohr et al., 2019). For example, in a study of primates, no significant association was found between host competence and extinction risk (Young et al., 2013).
Since species richness and host abundance can influence pathogen transmission (Dobson, 2004), the total community abundance-species richness relationship should influence the diversity-disease relationship (Mihaljevic et al., 2014). The community assembly pattern reflects how community abundance scales with species richness. Compensatory assembly and additive assembly are two extreme assembly patterns often considered in theoretical studies, which yield completely different richness-abundance relationships. The former means that total community abundance does not vary with host richness, and the latter means that total community abundance increases linearly with host richness. It has been demonstrated that when disease transmission is density-dependent, the compensatory assembly will lead to a dilution effect, while the additive assembly will lead to an amplification effect (Rudolf and Antonovics, 2005; Chen and Zhou, 2015). However, some studies found that the saturated relationship between community abundance and species richness is more reasonable than additive and compensatory assemblies, and can better reflect the changes in the total community abundance during biodiversity loss (Lehman and Tilman, 2000; Rohr et al., 2019). Until now, few studies have considered the effect of saturated community assembly on the diversity-disease relationship [but see Mihaljevic et al. (2014)]. Furthermore, to our knowledge, no studies have explored the combined effects of community composition, community assembly patterns, and disease transmission modes on the diversity-disease relationship.
In this study, using a multi-host Susceptible-Infected-Recovered (SIR) model, we hope to explore how host community composition, community assembly pattern, and disease transmission mode jointly affect community disease risk under community disassembly. We predict that different patterns of community assembly and disease transmission modes will result in different trends in diversity-disease relationships, and that shifts in community composition may alter such trends.
Materials and methods
The SIR model is the most classical and fundamental infectious disease model. It can characterize both density-dependent and frequency-dependent transmission of diseases. Similar to Dobson (2004), we adopt the classical multi-host SIR model to describe the state transition process of disease:
Here the subscript i represents the host species i (i = 1 …n), Si,Ii,Ri represent three different states of host i, namely susceptible, infected and recovered. represents the number of newly infected individuals of host i per unit time. di, μi, and γi represent natural death rate, disease-induced death rate, and recovery rate, respectively. Other parameters and their meanings are shown in Table 1.
The framework of the simulation was as follows. First, we constructed a global species pool containing 49 host species, the vital parameters of each species followed the parameter setting method established by Roche et al. (2012) and Mihaljevic et al. (2014). Second, we simulated the process of biodiversity loss under deterministic and stochastic extinction respectively, generating local communities with different species richness. Finally, for each local community, we calculated community R0 as a measure of community disease risk to explore how different combinations of community composition, disease transmission mode, and community assembly pattern affect community disease risk under community disassembly.
Generating a global species pool
In multi-host communities, different hosts have different epidemiological and ecological traits (Joseph et al., 2013). In order to make the constructed communities closer to the actual communities, we drew heavily on the existing community ecology rules and parameter setting methods to assign reasonable parameter values to each host species (Roche et al., 2012; Joseph et al., 2013). These parameters mainly include species abundance, demographic rate, and epidemiological traits.
Species abundance
Species abundance in the global species pool was assumed to follow Preston’s law. This law yields a species-abundance relationship that follows a log-normal distribution, in which a few species are common, and most species are rare (Preston, 1948). This distribution has been proved to describe the abundance distribution patterns in many ecosystems, such as vertebrates, alpine meadows, and fungi (Preston, 1962). It can be expressed as
where z is a constant, s0 is the number of species in the modal rank, and s(P) is the number of species in Pth rank from the modal rank. We assumed a total of eight ranks, including 49 species (Table 1). The abundance at each rank was assigned on alog2 base, which we considered to be the species’ equilibrium abundanceKi (Roche et al., 2012).
Demographic rate
Assuming that the community was in equilibrium, i.e., the birth rate of any host is equal to death rate. Empirical studies have shown that species’ birth and death rates tend to be allometrically related to body weight (Charnov, 1993). Similar to Roche et al. (2012), the relationship between birth (death) rate and body weight was expressed as
where Mi represented the average body weight of species i. We assumed the body weight is exponentially related to its abundance (Mihaljevic et al., 2014),
It can be seen from the above equation that the species with the smallest body weight is the most abundant.
Epidemiological traits
Field evidence suggests that host species vary significantly in their competence. Taking West Nile Virus as an example, hosts range from birds to horses to humans (Ezenwa et al., 2006). Birds are the most competent hosts, while horses are dead-end hosts, contributing little to the spread of the disease. In order to reflect the variations in host competence, we drew intraspecific values R0i from a truncated gamma distribution. The value of R0i reflects the competence of the host i. If R0i is greater than one, the pathogen can successfully invade the host population, and vice versa.
We considered three possible relationships between host competence R0i and abundance. First, R0i was positively related to abundance, so the most abundant species are the most competent. Then we relaxed this condition by assuming that there was no relationship between R0i and species abundance (neutral). Finally, we considered a negative relationship between R0i and host abundance, where the most abundant species were the least competent (Vinson and Park, 2019).
Similar to Mihaljevic et al. (2014), we calculated intraspecific transmission rate βii by
The interspecific transmission rate βij was proportional to the geometric mean of the intraspecific transmission rate βii and βjj, modified by a constant scaling factor ε, i.e., (Roberts and Heesterbeek, 2018). In this study, we assumed the interspecific transmission rate were symmetrical (i.e., βij = βji), and the transmission rates were the same for different individuals of the same species.
Till now, we have constructed a global species pool containing 49 hosts and described in detail how different demographic and epidemiological parameters were assigned to host species (Table 1). To get robust results, we repeated the above process 1,000 times to generate different global species pools.
Simulating the process of community disassembly
For each global species pool, we considered deterministic extinction and stochastic extinction of host species to simulate different biodiversity loss processes, generating local communities with species richness ranging from 2 to 49. According to the life history trade-off theory, the higher body weight, the lower number of species, and therefore the higher the risk of extinction (Huang et al., 2013). Thus, under deterministic extinction, we removed the highest body weight (the least abundant) host species from the community at a time until two host species remained in the community. While under stochastic extinction, we randomly selected one host species to remove at a time to simulate the decrease of species richness. Note that these two different species extinction ways correspond to different species extinction orders, thus reflecting different host community compositions.
Next, we separately considered the additive, compensatory, and saturated community assembly under each extinction pattern. In the additive assembly, the addition of new species increases the total community abundance, so the total community abundance was linearly accumulated with species richness. In contrast, in the compensatory assembly, the total community abundance was kept constant, and the abundance of remaining species in the community was compensated in proportion to their abundance when species richness decreased. In the saturated assembly, the community abundance (Kcom) increased asymptotically with species richness (R) by
This asymptotic function is close to the form of the relationship between plant cover and species richness (Tilman et al., 1996). If the total community abundance KT < Kcom, the abundance of each species was not adjusted, and the increase in community abundance was almost linearly with species richness. In contrast, if KT > Kcom, the adjusted abundance of each host was obtained by multiplying the abundance of each species by a scaling factor Kcom/KT, and then the increase in community abundance was almost a fully compensatory assembly.
In order to get rid of random effects and to get general results, we repeated the process of stochastic extinction 1,000 times for each of the above scenarios [all the nine possible combinations of community assembly patterns (i.e., additive, saturated, and compensatory assembly) and host competence-abundance relationships (i.e., positive, neutral, and negative correlations)].
Community basic reproduction number R0
As in previous studies, the community basic reproduction number R0 was used as a proxy for disease risk, which measures the possibility of successful disease invasion in a multi-host community (Roberts and Heesterbeek, 2013; O’Regan et al., 2015). We used the next-generation matrix method to calculate community R0 (Dobson, 2004). For a local community containing m species, the expression of the next-generation matrix G based on the SIR model is
where i,j = 1…m. represents the lifespan of the infected host i. pji depends on whether the disease transmission mode is density-dependent or frequency-dependent. For density-dependent transmission, pji equals to the equilibrium abundance Ki of infected host species i. For frequency-dependent transmission, pji equals to the proportion of infected host species i, namely . By calculating the dominant eigenvalues of the next-generation matrix G, we can obtained the community basic reproduction number R0.
Results
Results in the case of density-dependent transmission
In the case of density-dependent transmission and stochastic species extinction, the relationship between species richness and community abundance determined how community R0 varied with species richness (Figure 1). Specifically, when community abundance increased linearly with species richness (i.e., the additive assembly), community R0 increased monotonically with species richness, i.e., the amplification effect. While when community abundance was kept constant with species richness (i.e., the compensatory assembly), the community R0 initially decreased monotonically with increasing species richness and then gradually became stable. That is, a dilution effect occurred at low species richness, while when species richness was high, the community R0 was almost unchanged with species richness. When community abundance was saturated with species richness (the saturated assembly), the diversity-disease relationship showed a non-monotonic hump-shaped trend, with a peak corresponding to species richness of about 15. Along the species richness gradient, the community R0 first increased (amplification effect) and then decreased (dilution effect).
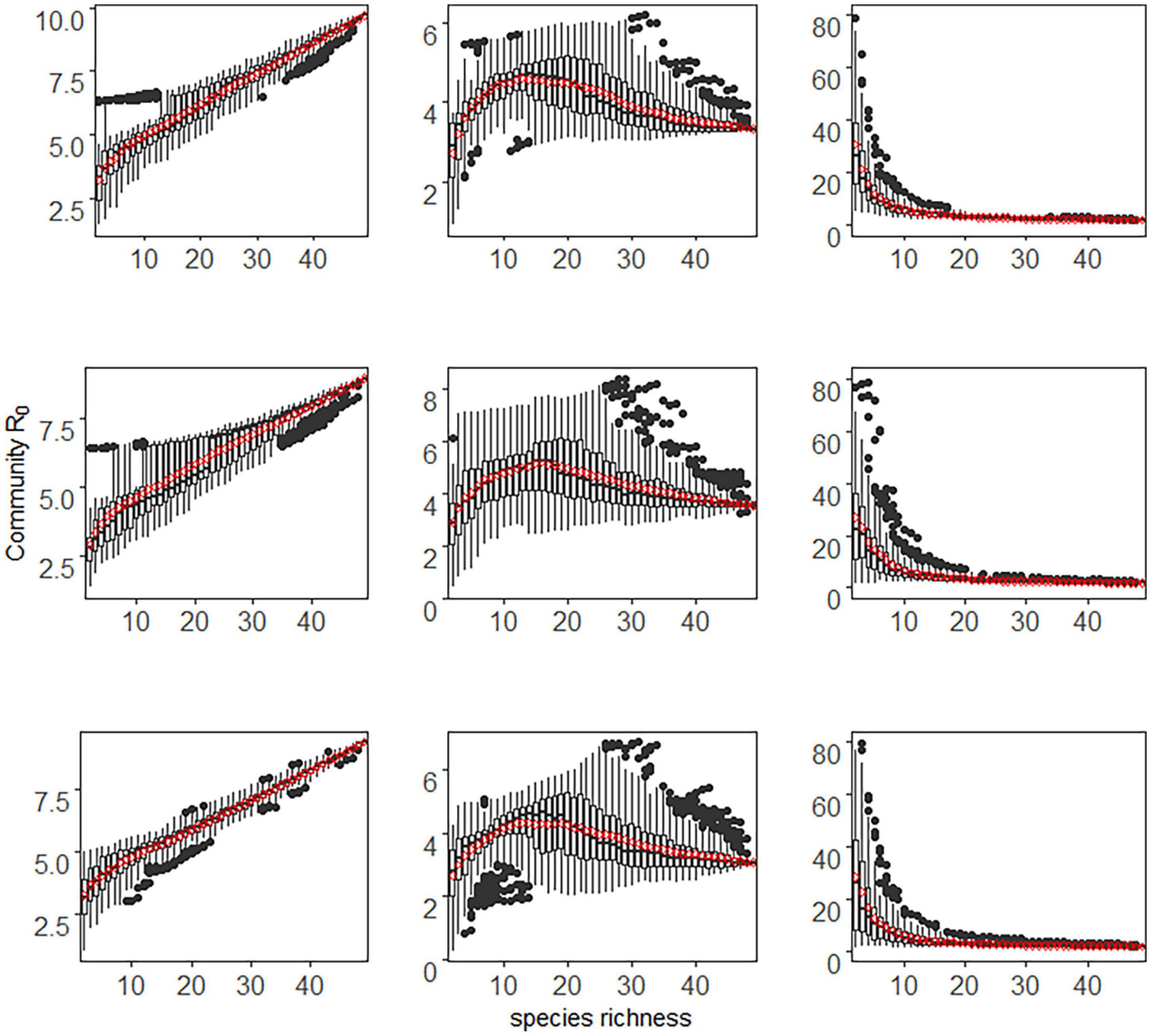
Figure 1. The relationship between host species richness and community R0 under density-dependent transmission and stochastic extinction scenario. Each column represents different community assembly pattern. From the first column to the third column are additive, saturated, and compensatory assembly, respectively. Each row represents the relationship between host competence and abundance. Positive, neutral, and negative correlations are from the first to the third row. The x-axis in each panel represents species richness, and the y-axis represents community R0. Each boxplot represents the values of 1,000 simulations for each species richness level, and the red dots represent the mean values of community R0.
As shown in Figure 1, the above conclusions still hold for positive, neutral, or negative species competence-richness relationships. These findings suggested that in this case, community assembly patterns qualitatively determined whether disease risk increases, decreases, or is non-monotonic under community disassembly. However, the relationship between species competence and extinction risk could only quantitatively but not qualitatively alter the diversity-disease trend.
Conversely, in the case of density-dependent transmission and deterministic species extinction, the community R0 increased monotonically with species richness when community assembly was additive (Figure 2). The host competence-richness relationship only affected the value of community R0, but did not change this increasing trend. These results were consistent with the stochastic extinction scenario. However, when community abundance was saturated with species richness (saturated), community R0 increased monotonically with the increase of species richness, which is different from the non-monotonic humped-shaped curve in the stochastic extinction scenario. When community abundance did not change with species richness (compensatory), the direction of the diversity-disease relationship depended on how host competence varied with abundance (The third column in Figure 2). If host competence was positively correlated with abundance, then community R0 decreased as the increase of species richness, i.e., a dilution effect occurred. In contrast, if host competence was negatively correlated with abundance, then community R0 increased with species richness, i.e., an amplification effect occurred. If host competence was neutral with abundance, community R0 first slightly decreased and then slightly increased with species richness. An interesting finding is that the results in the latter two cases (negative or neutral relationship between host competence and abundance) were quite different from those in stochastic extinction scenario (Figures 1, 2).
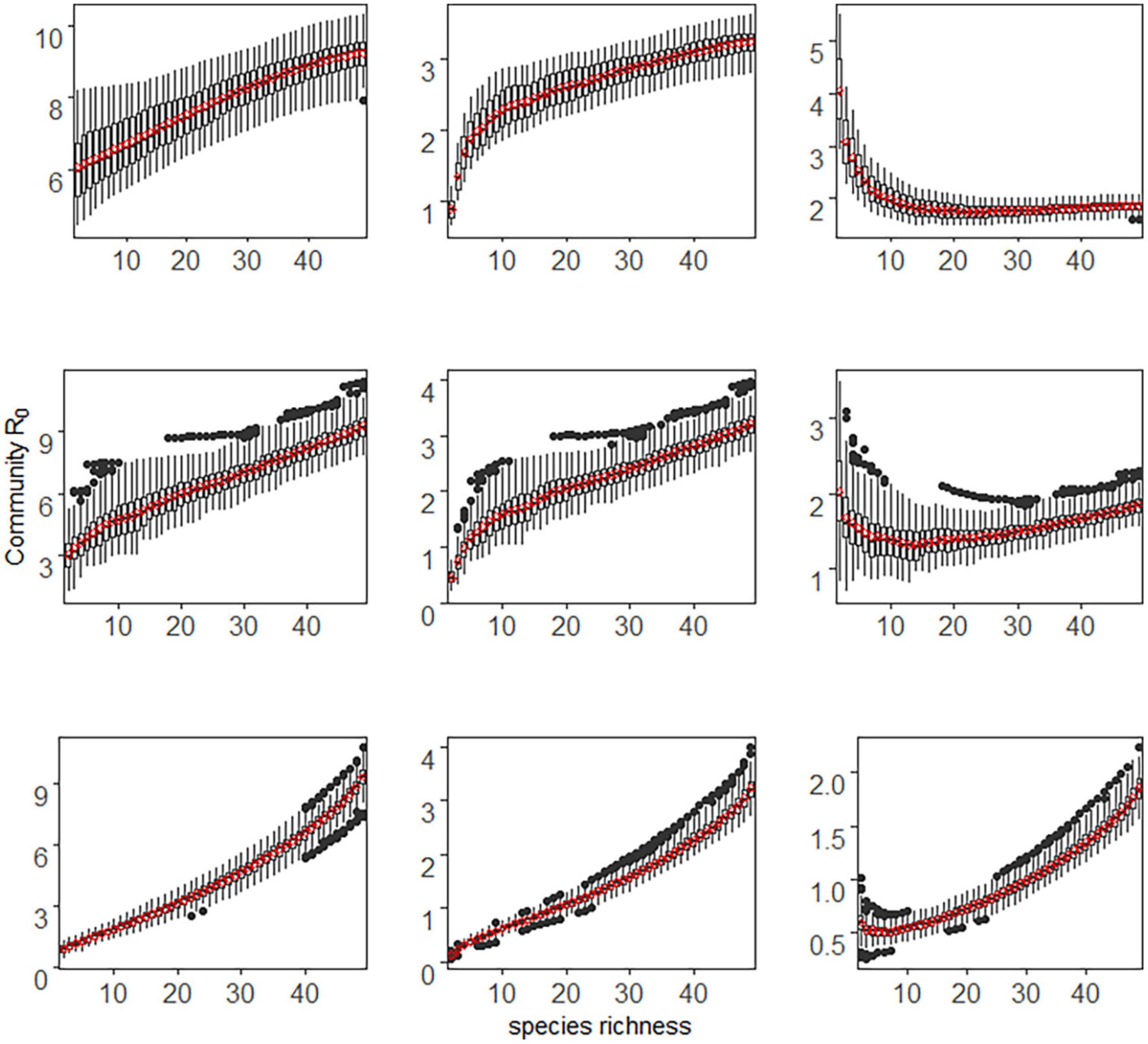
Figure 2. The relationship between host species richness and community R0 under density-dependent transmission and deterministic extinction scenario. Other details are the same as in Figure 1.
In summary, the direction and strength of the relationship between diversity and disease risk under density-dependent transmission were determined by a combination of community assembly pattern, host community composition, and host competence-abundance relationship.
Results in the case of frequency-dependent transmission
In the case of frequency-dependent transmission, disease transmission was determined by the proportion of host species, not by host abundance. Therefore, there was little difference in disease transmission whether the community assembly was additive, compensatory, or saturated. Thus, we used the additive method as an example to show how species extinction pattern and host competence-abundance relationship affect the relationship between diversity and disease risk (Figures 3, 4).
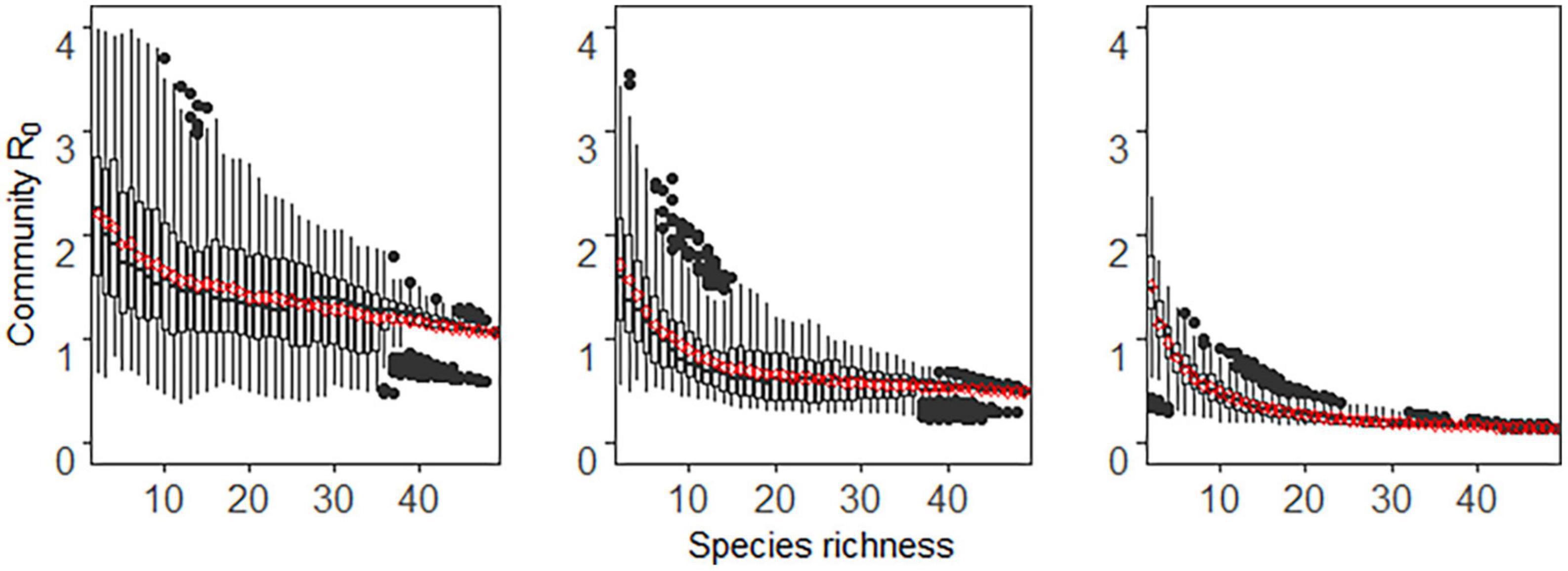
Figure 3. The relationship between host species richness and community R0 under frequency-dependent transmission and stochastic extinction scenario. Each column represents different relationship between host competence and abundance. Positive, neutral, and negative correlations are from the first to the third column. Each boxplot represents the values of 1,000 simulations for each species richness level, and the red dots represent the mean values of community R0.
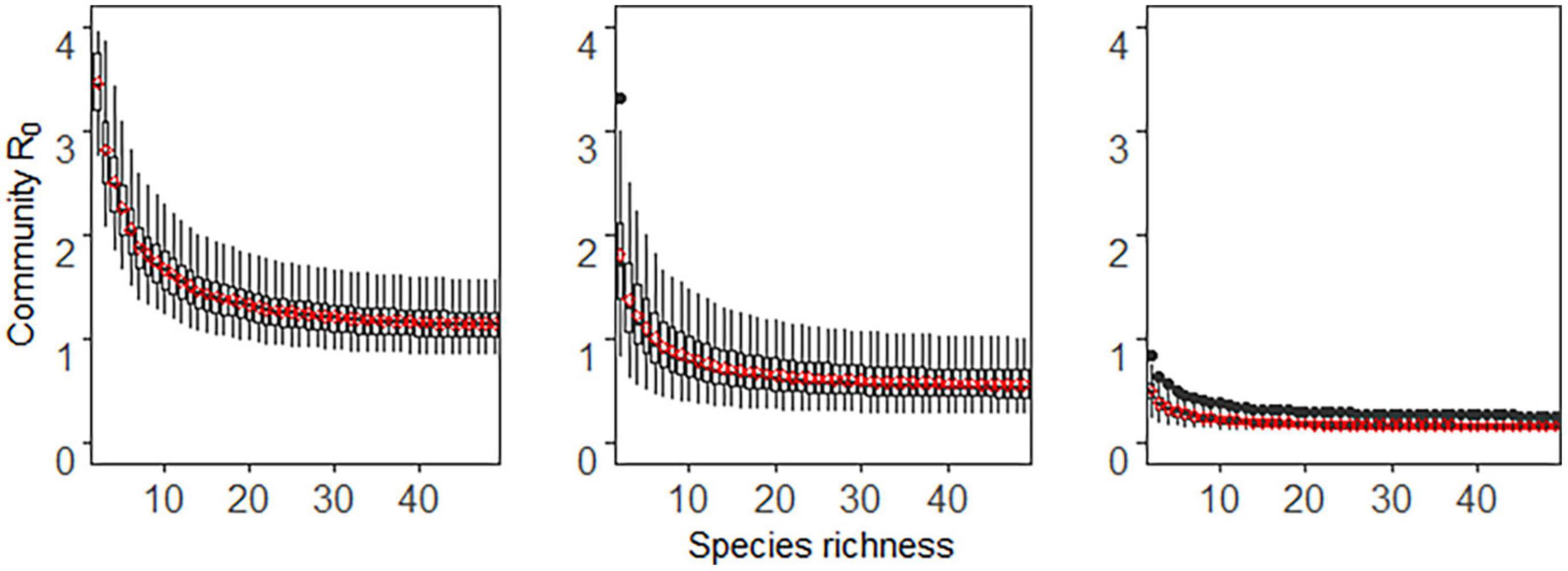
Figure 4. The relationship between host species richness and community R0 under frequency-dependent transmission and deterministic extinction scenario. Other details are the same as in Figure 3.
As seen in Figures 3, 4, regardless of whether species extinction was deterministic or stochastic, community R0 decreased monotonically with the increase of host richness, i.e., only a dilution effect occurred. The host competence-abundance relationship only changed the value of community R0, but did not alter diversity-disease trends. For a fixed species richness level, the community R0 was highest when host competence and abundance were positively correlated and lowest when there was a negative correlation between them. In particular, a negative host competence-abundance relationship exhibited a weak dilution effect when the species richness was low, while when species richness was high, community R0 did not change with species richness, that is species richness had no effect on disease risk (third column in Figures 3, 4).
Discussion
The relationship between host diversity and disease risk is one of the central concerns in disease ecology. In the context of rapid global biodiversity loss and increasing emerging infectious diseases, a deeper understanding of the diversity-disease relationship is important for public health and wildlife conservation. Although extensive experimental evidence supports the existence of dilution effects in natural ecosystems, we still know very little about the conditions under which the dilution effect occurs (Keesing and Ostfeld, 2021). By building a multi-host SIR model, we found when disease transmission was density-dependent, the diversity-disease relationship could exhibit an increasing, decreasing, or non-monotonic trend, which mainly depended on the patterns of community assembly. However, the combined effects of the host competence-abundance relationship and species extinction order may reverse or weaken this trend. In contrast, when disease transmission was frequency-dependent, the diversity-disease relationship only showed a decreasing trend (i.e., only a dilution effect occurred). The host competence-abundance relationship and species extinction order did not alter this decreasing trend in this case, but it could reduce the detectability of the dilution effect and affect the value of the community R0. Overall, a combination of disease transmission mode, community assembly pattern, and host community composition can alter the direction or strength of the diversity-disease relationship.
Exploring the general consequences of the host competence-abundance relationship on disease risk is one of the main goals of this study. An interesting finding is that under density-dependent and deterministic extinction scenario (Figure 2), different host competence-abundance relationships (positive, neutral, or negative) may lead to opposite results in the case of compensatory assembly. The reason may be that a positive relationship between host competence and abundance means that low competent hosts are the first to be extirpated from the community. Therefore, community disease risk increases with decreasing species richness, i.e., a dilution effect occurs (Rosenthal et al., 2021). Conversely, if host competence is negatively related to abundance, then the most competent hosts go extinct first, resulting in community contains a large number of less competent hosts. Hence, disease risk decreases when community disassembly, i.e., an amplification effect occurs. If host competence is neutral with abundance, then host species are randomly removed as species richness decreases, therefore the relationship between diversity and disease risk is weak.
Our results have been confirmed by some empirical studies. For example, a survey of amphibian communities in 147 wetland areas in California found that the relationship between diversity and trematodes disease risk showed a dilution effect when low competent host species went extinct first, but showed no effect when host species went extinct at random (Johnson et al., 2019). Similar results were found for plant communities. For example, for alpine meadow plant communities, the low competent host plants were the first to go extinct after nitrogen addition, and the relationship between plant richness and leaf fungal disease risk showed a dilution effect. However, when plants were randomly removed in controlled experiments, plant richness had almost no effect on fungal disease risk (Liu et al., 2018). It is worth noting that traditional biodiversity-ecosystem function controlled experiments tend to assemble communities by randomly sampling species from the species pool. This may ignore the importance of species loss order and may underestimate disease risk (Wolfi and Zavaleta, 2015).
In the case of deterministic extinction, our results were consistent with previous findings that an amplification effect occurred when community assembly was additive, and a dilution effect occurred when community assembly was compensatory under density-dependent transmission (Rudolf and Antonovics, 2005; Mihaljevic et al., 2014). While under frequency-dependent transmission, the diversity-disease relationship did not change with different patterns of community assembly. However, as mentioned in the Introduction, neither additive nor compensatory community assembly patterns reflect species richness-abundance relationship in actual communities. In a community, both additive and compensatory assembly can occur (Rohr et al., 2019). In general, additive assembly happens in the early stages of a community (Chen and Zhou, 2015). As competition gets tougher and resources are limited, it will switch to compensatory assembly. Therefore, the saturated assembly is more reasonable, and can better reflect the changes in the total community abundance under community disassembly. For example, using three different theoretical models, Lehman and Tilman (2000) found a saturated relationship between total community biomass and species richness due to competition. Similarly, Guo et al. (2006) showed a non-monotonic relationship between grassland biomass and plant richness within a certain range of species richness.
Saturated community assembly resulted in a non-monotonic hump-shaped diversity-disease relationship under density-dependent transmission and stochastic extinction scenario, which emphasized the importance of host richness ranges for identifying diversity-disease relationships in field studies. The occurrence of a dilution or amplification effect depends on whether species richness falls to the left or right side of the peak (Halliday et al., 2019; Rohr et al., 2019). If host species richness falls to the left side of the peak, then an amplification effect can occur when community disassembly. On the contrary, there will be a dilution effect. This finding may explain the divergent results in field studies and the controlled experiments. In most field studies, species richness often falls to the right side of the peak, making it easy to conclude a dilution effect (Johnson et al., 2013; Kilpatrick et al., 2017). However, in controlled experiments, given the difficulty of manipulating species richness, species richness tends to fall to the left side of the peak, so it is easier to conclude an amplification effect (Halliday et al., 2017). In future research, we must focus on evaluating the shape of the diversity-disease relationship and determining where species richness lies on this curve.
Due to the complexity of the multi-host-pathogen system, exploring the general diversity-disease relationship is challenging, for which we made some simplifications. For example, in natural communities, the presence of keystone species may have a significant impact on diversity-disease relationships (Levi et al., 2016), which we did not take into account. Furthermore, we only considered the saturated community abundance-species richness relationship due to competition. However, in many natural systems where predation has a more substantial effect on disease dynamics than competition (Chen et al., 2022), all of which needs to be addressed in future studies. Despite these limitations, this study demonstrated for the first time that the trends of the diversity-disease relationship did not only depend on community assembly pattern and disease transmission mode. The relationship between host competence and extinction risk could alter the direction and strength of the diversity-disease relationship. This study not only explains the current debate about the dilution effect, but also contributes to a deeper understanding of the pathogen transmission dynamics in actual communities.
Data availability statement
The original contributions presented in this study are included in the article/supplementary material, further inquiries can be directed to the corresponding author.
Author contributions
LC and PK designed the study and analyzed the model. LC, LH, and YZ performed the software. LC, PK, and LZ drafted the original manuscript. LC and LZ reviewed and edited the manuscript. All authors have read and agreed to the published version of the manuscript.
Funding
This work was supported by the National Natural Science Foundation of China (31700466 and 82072228), the Foundation of National Key R&D Program of China (2020YFC2008700), the Foundation of Shanghai Municipal Commission of Economy and Informatization (202001007), and the Special Project of Health Shanghai Action (2022–2024).
Conflict of interest
The authors declare that the research was conducted in the absence of any commercial or financial relationships that could be construed as a potential conflict of interest.
Publisher’s note
All claims expressed in this article are solely those of the authors and do not necessarily represent those of their affiliated organizations, or those of the publisher, the editors and the reviewers. Any product that may be evaluated in this article, or claim that may be made by its manufacturer, is not guaranteed or endorsed by the publisher.
References
Allen, T., Murray, K. A., Zambrana-Torrelio, C., Morse, S. S., Rondinini, C., Di Marco, M., et al. (2017). Global hotspots and correlates of emerging zoonotic diseases. Nat. Commun. 8:1124. doi: 10.1038/s41467-017-00923-8
Charnov, E. L. (1993). Life History Invariants: Some Explorations of Symmetry in Evolutionary Ecology. Oxford: Oxford University Press.
Chen, L., Chen, S., Kong, P., and Zhou, L. (2022). Host competence, interspecific competition and vector preference interact to determine the vector-borne infection ecology. Front. Ecol. Evol. 10:993844. doi: 10.3389/fevo.2022.993844
Chen, L., and Zhou, S. (2015). A combination of species evenness and functional diversity is the best predictor of disease risk in multihost communities. Am. Nat. 186, 755–765. doi: 10.1086/683774
Cortez, M. H., and Duffy, M. A. (2021). The context-dependent effects of host competence. competition, and pathogen transmission mode on disease prevalence. Am. Nat. 198, 179–194. doi: 10.1086/715110
Dobson, A. (2004). Population dynamics of pathogens with multiple host species. Am. Nat. 164, S64–S78. doi: 10.1086/424681
Downs, C. J., Schoenle, L. A., Han, B. A., Harrison, J. F., and Martin, L. B. (2019). Scaling of host competence. Trends Parasitol. 35, 182–192. doi: 10.1016/j.pt.2018.12.002
Elton, C. S. (1958). The Ecology of Invasions by Animals and Plants. London: Methuen & Co. Ltd., 181. doi: 10.1007/978-1-4899-7214-9
Estrada-Peña, A., Ostfeld, R. S., Peterson, A. T., Poulin, R., and de la Fuente, J. (2014). Effects of environmental change on zoonotic disease risk: an ecological primer. Trends Parasitol. 30, 205–214. doi: 10.1016/j.pt.2014.02.003
Ezenwa, V. O., Godsey, M. S., King, R. J., and Guptill, S. C. (2006). Avian diversity and West Nile virus: testing associations between biodiversity and infectious disease risk. Proc. R. Sci. 273, 109–117. doi: 10.1098/rspb.2005.3284
Gibb, R. J., Redding, D. W., Chin, K. Q., Donnelly, C. A., Blackburn, T. M., Newbold, T., et al. (2020). Zoonotic host diversity increases in human-dominated ecosystems. Nature 584, 398–402. doi: 10.1038/s41586-020-2562-8
Guo, Q., Shaffer, T. L., and Buhl, T. K. (2006). Community maturity, species saturation and the variant diversity-productivity relationships in grasslands. Ecol. Lett. 9, 1284–1292. doi: 10.1111/j.1461-0248.2006.00980.x
Halliday, F. W., Heckman, R. W., Wilfahrt, P. A., and Mitchell, C. E. (2017). A multivariate test of disease risk reveals conditions leading to disease amplification. Proc. R. Soc. B Biol. Sci. 284:20171340. doi: 10.1098/rspb.2017.1340
Halliday, F. W., Heckman, R. W., Wilfahrt, P. A., and Mitchell, C. E. (2019). Past is prologue: host community assembly and the risk of infectious disease over time. Ecol. Lett. 22, 138–148. doi: 10.1111/ele.13176
Huang, Z. Y., de Boer, W. F., van Langevelde, F., Olson, V., Blackburn, T. M., and Prins, H. H. (2013). Species’ life-history traits explain interspecific variation in reservoir competence: a possible mechanism underlying the dilution effect. PLoS One 8:e54341. doi: 10.1371/journal.pone.0054341
Huang, Z. Y. X., van Langevelde, F., Estrada-Peña, A., Suzán, G., and de Boer, W. F. (2016). The diversity–disease relationship: evidence for and criticisms of the dilution effect. Parasitology 143, 1075–1086. doi: 10.1017/S0031182016000536
Johnson, P. T., Calhoun, D. M., Riepe, T., McDevitt-Galles, T., and Koprivnikar, J. (2019). Community disassembly and disease: realistic—but not randomized—biodiversity losses enhance parasite transmission. Proc. R. Sci. 286:20190260. doi: 10.1098/rspb.2019.0260
Johnson, P. T., Ostfeld, R. S., and Keesing, F. (2015). Frontiers in research on biodiversity and disease. Ecol. Lett. 18, 1119–1133. doi: 10.1111/ele.12479
Johnson, P. T., Preston, D. L., Hoverman, J. T., and Richgels, K. L. (2013). Biodiversity decreases disease through predictable changes in host community competence. Nature 494, 230–233. doi: 10.1038/nature11883
Joseph, M. B., Mihaljevic, J. R., Orlofske, S. A., and Paull, S. H. (2013). Does life history mediate changing disease risk when communities disassemble? Ecol. Lett. 16, 1405–1412. doi: 10.1111/ele.12180
Keesing, F., Belden, L. K., Daszak, P., Dobson, A., Harvell, C. D., Holt, R. D., et al. (2010). Impacts of biodiversity on the emergence and transmission of infectious diseases. Nature 468, 647–652. doi: 10.1038/nature09575
Keesing, F., Holt, R. D., and Ostfeld, R. S. (2006). Effects of species diversity on disease risk. Ecol. Lett. 9, 485–498. doi: 10.1111/j.1461-0248.2006.00885.x
Keesing, F., and Ostfeld, R. S. (2021). Dilution effects in disease ecology. Ecol. Lett. 24, 2490–2505. doi: 10.1111/ele.13875
Kilpatrick, A. M., Salkeld, D. J., Titcomb, G., and Hahn, M. B. (2017). Conservation of biodiversity as a strategy for improving human health and well-being. Philos. Trans. R. Soc. Lond. B Biol. Sci. 372:20160131. doi: 10.1098/rstb.2016.0131
Lacroix, C., Jolles, A., Seabloom, E. W., Power, A. G., Mitchell, C. E., and Borer, E. T. (2014). Non-random biodiversity loss underlies predictable increases in viral disease prevalence. J. R. Soc. Interface 11:20130947. doi: 10.1098/rsif.2013.0947
Lehman, C. L., and Tilman, D. (2000). Biodiversity, stability, and productivity in competitive communities. Am. Nat. 156, 534–552. doi: 10.1086/303402
Levi, T., Keesing, F., Holt, R. D., Barfield, M., and Ostfeld, R. S. (2016). Quantifying dilution and amplification in a community of hosts for tick-borne pathogens. Ecol. Appl. 26, 484–498. doi: 10.1890/15-0122
Lind, E. M., Borer, E., Seabloom, E., Adler, P., Bakker, J. D., Blumenthal, D. M., et al. (2013). Life-history constraints in grassland plant species: a growth-defence trade-off is the norm. Ecol. Lett. 16, 513–521. doi: 10.1111/ele.12078
Liu, X., Chen, F., Lyu, S., Sun, D., and Zhou, S. (2018). Random species loss underestimates dilution effects of host diversity on foliar fungal diseases under fertilization. Ecol. Evol. 8, 1705–1713. doi: 10.1002/ece3.3749
Liu, X., Chen, L., Liu, M., García-Guzmán, G., Gilbert, G. S., and Zhou, S. (2020). Dilution effect of plant diversity on infectious diseases: latitudinal trend and biological context dependence. Oikos 129, 457–465. doi: 10.1111/oik.07027
Liu, X., Lyu, S., Sun, D., Bradshaw, C. J., and Zhou, S. (2017). Species decline under nitrogen fertilization increases community-level competence of fungal diseases. Proc. R. Sci. 284:20162621. doi: 10.1098/rspb.2016.2621
Liu, X., Lyu, S., Zhou, S., and Bradshaw, C. J. (2016). Warming and fertilization alter the dilution effect of host diversity on disease severity. Ecology 97, 1680–1689. doi: 10.1890/15-1784.1
LoGiudice, K., Ostfeld, R. S., Schmidt, K. A., and Keesing, F. (2003). The ecology of infectious disease: effects of host diversity and community composition on Lyme disease risk. Proc. Natl. Acad. Sci. U.S.A. 100, 567–571. doi: 10.1073/pnas.0233733100
Mihaljevic, J. R., Joseph, M. B., Orlofske, S. A., and Paull, S. H. (2014). The scaling of host density with richness affects the direction, shape, and detectability of diversity-disease relationships. PLoS One 9:e97812. doi: 10.1371/journal.pone.0097812
O’Regan, S. M., Vinson, J. E., and Park, A. W. (2015). Interspecific contact and competition may affect the strength and direction of disease-diversity relationships for directly transmitted microparasites. Am. Nat. 186, 480–494. doi: 10.1086/682721
Ostfeld, R. S., and Keesing, F. (2000). Biodiversity series: the function of biodiversity in the ecology of vector-borne zoonotic diseases. Can. J. Zool. 78, 2061–2078. doi: 10.1139/z00-172
Ostfeld, R. S., and Keesing, F. (2012). Effects of host diversity on infectious disease. Annu. Rev. Ecol. Syst. 43, 157–182. doi: 10.1146/annurev-ecolsys-102710-145022
Preston, F. W. (1948). The commonness, and rarity, of species. Ecology 29, 254–283. doi: 10.2307/1930989
Preston, F. W. (1962). The canonical distribution of commonness and rarity: part I. Ecology 43, 185–215. doi: 10.2307/1931976
Roberts, M. G., and Heesterbeek, J. (2013). Characterizing the next-generation matrix and basic reproduction number in ecological epidemiology. J. Math. Biol. 66, 1045–1064. doi: 10.1007/s00285-012-0602-1
Roberts, M. G., and Heesterbeek, J. A. P. (2018). Quantifying the dilution effect for models in ecological epidemiology. J. R. Soc. Interface 15:20170791. doi: 10.1098/rsif.2017.0791
Roche, B., Dobson, A. P., Guégan, J.-F., and Rohani, P. (2012). Linking community and disease ecology: the impact of biodiversity on pathogen transmission. Philos. Trans. R. Soc. Lond. B Biol. Sci. 367, 2807–2813. doi: 10.1098/rstb.2011.0364
Rohr, J. R., Civitello, D. J., Halliday, F. W., Hudson, P. J., Lafferty, K. D., Wood, C. L., et al. (2019). Towards common ground in the biodiversity–disease debate. Nat. Ecol. Evol. 4, 24–33. doi: 10.1038/s41559-019-1060-6
Rosenthal, L. M., Brooks, W., and Rizzo, D. M. (2021). Species densities, assembly order, and competence jointly determine the diversity-disease relationship. Ecology 103:e3622. doi: 10.1002/ecy.3622
Rudolf, V. H., and Antonovics, J. (2005). Species coexistence and pathogens with frequency-dependent transmission. Am. Nat. 166, 112–118. doi: 10.1086/430674
Salkeld, D. J., Padgett, K. A., and Jones, J. H. (2013). A meta-analysis suggesting that the relationship between biodiversity and risk of zoonotic pathogen transmission is idiosyncratic. Ecol. Lett. 16, 679–686. doi: 10.1111/ele.12101
Tilman, D., Wedin, D. A., and Knops, J. M. H. (1996). Productivity and sustainability influenced by biodiversity in grassland ecosystems. Nature 379, 718–720. doi: 10.1038/379718a0
Vadell, M. V., Villafañe, I. E. G., and Carbajo, A. E. (2020). Hantavirus infection and biodiversity in the Americas. Oecologia 192, 169–177. doi: 10.1007/s00442-019-04564-0
Vinson, J. E., and Park, A. W. (2019). Vector-borne parasite invasion in communities across space and time. Proc. R. Soc. B Biol. Sci. 286:20192614. doi: 10.1098/rspb.2019.2614
Wolfi, A. A., and Zavaleta, E. S. (2015). Species traits outweigh nested structure in driving the effects of realistic biodiversity loss on productivity. Ecology 96, 90–98. doi: 10.1890/14-0131.1
Wood, C. L. (2014). Environmental change and the ecology of infectious disease. Science 346, 1192–1192. doi: 10.1126/science.aaa1810
Keywords: disease risk, dilution effect, amplification effect, community disassembly, host competence, stochastic extinction, deterministic extinction
Citation: Chen L, Kong P, Hou L, Zhou Y and Zhou L (2022) Host community composition, community assembly pattern, and disease transmission mode jointly determine the direction and strength of the diversity-disease relationship. Front. Ecol. Evol. 10:1032931. doi: 10.3389/fevo.2022.1032931
Received: 31 August 2022; Accepted: 20 September 2022;
Published: 04 October 2022.
Edited by:
Xiang Liu, Lanzhou University, ChinaCopyright © 2022 Chen, Kong, Hou, Zhou and Zhou. This is an open-access article distributed under the terms of the Creative Commons Attribution License (CC BY). The use, distribution or reproduction in other forums is permitted, provided the original author(s) and the copyright owner(s) are credited and that the original publication in this journal is cited, in accordance with accepted academic practice. No use, distribution or reproduction is permitted which does not comply with these terms.
*Correspondence: Lifan Chen, Y2hlbmxmQHN1bWhzLmVkdS5jbg==