- 1College of Earth, Ocean, and Atmospheric Sciences, Oregon State University, Corvallis, OR, United States
- 2Department of Biomedical Physics, Wayne State University, Detroit, MI, United States
- 3Public Policy Associates, Inc., Lansing, MI, United States
- 4Department of Biochemistry, Microbiology, and Immunology, Wayne State University, Detroit, MI, United States
- 5Department of Sciences and Mathematics, Mississippi University for Women, Columbus, MS, United States
- 6Department of Chemistry, Institute for Pheromone Research, Indiana University, Bloomington, IN, United States
- 7Department of Biological Sciences, California State University Stanislaus, Turlock, CA, United States
- 8Department of Biology, California State University Fresno, Fresno, CA, United States
Introduction: Avian preen oil, secreted by the uropygial gland, is an important source of volatile compounds that convey information about the sender’s identity and quality, making preen oil useful for the recognition and assessment of potential mates and rivals. Although intrinsic factors such as hormone levels, genetic background, and diet can affect preen oil volatile compound composition, many of these compounds are not the products of the animal’s own metabolic processes, but rather those of odor-producing symbiotic microbes. Social behavior affects the composition of uropygial microbial communities, as physical contact results in microbe sharing. We experimentally manipulated social interactions in captive dark-eyed juncos (Junco hyemalis) to assess the relative influence of social interactions, subspecies, and sex on uropygial gland microbial composition and the resulting preen oil odor profiles.
Methods: We captured 24 birds at Mountain Lake Biological Station in Virginia, USA, including birds from two seasonally sympatric subspecies – one resident, one migratory. We housed them in an outdoor aviary in three phases of social configurations: first in same-sex, same-subspecies flocks, then in male-female pairs, and finally in the original flocks. Using samples taken every four days of the experiment, we characterized their uropygial gland microbiome through 16S rRNA gene sequencing and their preen oil volatile compounds via GC-MS.
Results: We predicted that if social environment was the primary driver of uropygial gland microbiome composition, and if microbiome composition in turn affected preen oil volatile profiles, then birds housed together would become more similar over time. Our results did not support this hypothesis, instead showing that sex and subspecies were stronger predictors of microbiome composition. We observed changes in volatile compounds after the birds had been housed in pairs, which disappeared after they were moved back into flocks, suggesting that hormonal changes related to breeding condition were the most important factor in these patterns.
Discussion: Although early life social environment of nestlings and long-term social relationships have been shown to be important in shaping uropygial gland microbial communities, our study suggests that shorter-term changes in social environment do not have a strong effect on uropygial microbiomes and the resulting preen oil volatile compounds.
Introduction
The microbiome is widely acknowledged as a critical contributor to a host individual’s development, health, and survival (Bosch and McFall-Ngai, 2011). The hologenome theory of evolution thus urges incorporating the microbiome, and its associated evolutionary dynamics, into hypotheses about how host animals evolve (Rosenberg and Zilber-Rosenberg, 2011; Bordenstein and Theis, 2015; Theis et al., 2020). A holobiont is an emergent individual made up of an animal and their symbiotic microbial communities at any given point in time, and the resulting phenotype is subject to evolutionary forces (Bordenstein and Theis, 2015). Importantly, the composition of an animal’s microbiome is not stable throughout the host’s lifetime, shifting in response to changes in the host animal’s biology or exchanges with the physical or social environment. Understanding the evolution of traits affected by microbes requires exploration of how these microbial communities are acquired, maintained, and shared (Archie and Theis, 2011; Ezenwa and Williams, 2014).
Since symbiotic microbes have a different mode of transmission than an animal’s nuclear genes, the hologenome concept incorporates elements of Lamarckian evolutionary theory, defined as inheritance of acquired characteristics (Rosenberg et al., 2009). Bordenstein and Theis (2015) suggest that Lamarckian theory can be incorporated into our understanding of the evolution of the hologenome as follows: host individuals may initially acquire their symbionts through environmental acquisition, and holobionts can then pass these traits on to their offspring through vertical transmission. Over evolutionary time, the hosts’ physiology and behavior may evolve mechanisms for the effective transmission and housing of beneficial microbes through natural selection, stabilizing the relationship between host and microbiome.
Social environment has been shown to play an important role in microbiome composition, as skin and gut bacterial communities reflect group membership and social networks in several mammalian (Song et al., 2013; Theis et al., 2013; Leclaire et al., 2014; Tung et al., 2015; Moeller et al., 2016; Yarlagadda et al., 2021) and avian (Kulkarni and Heeb, 2007; Ruiz-Rodríguez et al., 2014; Whittaker et al., 2016; Goodenough et al., 2017; Engel, et al., 2020) species. Indeed, behaviors that promote the transmission of beneficial microbes have been documented in several animal species, such as overmarking or allomarking in scent-marking animals (Buesching et al., 2003; Theis et al., 2008) and coprophagy (Osawa et al., 1993; Kobayashi et al., 2019), though microbe transmission can occur through other social behaviors like grooming and play (Meadow et al., 2013; Perofsky et al., 2017). Some researchers have suggested that microbe sharing is a key benefit of sociality (Troyer, 1984; Lombardo, 2008). Host genetics and physiology also regulate the composition of the microbiome (Spor et al., 2011). Several studies have suggested that immune genes, in particular the major histocompatibility complex, play a key role in this regulation, as they influence whether the body tolerates specific microbes (Kubinak et al., 2015; Khan et al., 2019). Other experiments have found that environmental transmission can overwhelm the influence of genetic background on microbiome composition (Burns et al., 2017).
Skin and gland microbiota have been shown to produce odors used by host animals in communication (Ezenwa and Williams, 2014; Maraci et al., 2018). In mammalian scent glands and avian uropygial glands, the composition of microbial communities and volatile odor profiles reflect group membership, suggesting that social exchange of bacteria contributes to the derivation of group-specific scent (Theis et al., 2012; Leclaire et al., 2014; Whittaker et al., 2016). However, little is understood about the degree of social interactions required to affect the phenotype. If socially-mediated microbial composition affects the information content, effectiveness, or attractiveness of chemical signals, then social behavior must be considered when modeling how such signals evolve.
The dark-eyed junco (Junco hyemalis), often recognized as an ecological model species (Peterson et al., 2012), has been the subject of ongoing studies of avian chemical communication for over a decade. Chemical communication plays an important role in avian biology and behavior (reviewed in Caro et al., 2015; Whittaker and Hagelin, 2021). Bacteria associated with the uropygial gland, particularly bacteria in the phyla Actinobacteria, Bacteriodetes, Firmicutes, and Proteobacteria, produce volatile compounds present in junco preen oil (Whittaker et al., 2016, 2019). These volatile compounds reliably transmit information about a bird’s identity, including species (Soini et al., 2013) and sex (Whittaker et al., 2010), and also reflect the bird’s hormone levels and breeding condition (Whittaker et al., 2011, 2018). Furthermore, a study of mated juncos and their nestlings – some of them the product of extra-pair matings – showed that uropygial gland microbial community composition and preen oil volatile profiles were influenced by social group membership but not genetic relatedness (Whittaker et al., 2016), highlighting the need for studies on how these microbial communities are acquired, regulated, and maintained.
The aim of this study is to experimentally assess the effects of social contact on uropygial gland microbial communities, and of socially mediated changes in microbial communities on preen oil odors. We manipulated social group membership of captive dark-eyed juncos for short periods of time and sampled them at multiple time points through different social group configurations. To assess the relative importance of social behavior compared to genetic background, we included birds from two different subspecies of juncos.
Materials and methods
Study species and site
Our study included individuals from two seasonally sympatric subspecies of the dark-eyed junco (Junco hyemalis) near Mountain Lake Biological Station (MLBS) in Pembroke, VA. The Carolina junco (J. h. carolinensis, hereafter “resident”) is resident in this area year-round, establishing territories and forming socially monogamous pairs during the breeding season (April–July) and living in large flocks during the winter (Nolan et al., 2022). The Northern junco (J. h. hyemalis, hereafter “migrant”) breeds at higher latitudes in Alaska, Canada, and northern New England, but migrates south to overwinter in this area, resulting in seasonal sympatry of the two subspecies from October to April. Both subspecies are commonly referred to as “slate-colored juncos” (Nolan et al., 2022). Resident Carolina juncos are slightly larger (male average wing chord about 84 mm, females 79 mm) with lighter gray plumage and a dark gray beak, while migratory Northern juncos are smaller (male average wing chord 82 mm, females 77 mm) with darker gray plumage and a light pink beak (Ketterson and Nolan, 1976).
We captured birds for this study in late March 2018 (March 20–31) using baited mist nets. The use of mist nets has been found to be a safe practice with injuries and mortalities found to occur in under 1% of total captures (Spotswood et al., 2012). We monitored nets closely, checking them a minimum of every 15 min. All personnel were trained in proper bird handling techniques and all efforts were taken to minimize harm to the animals.
Migrants begin departing the area in mid-March, with none remaining by the end of April, while residents begin forming reproductive pairs during this period (Kimmitt et al., 2019). By April, when we conducted our experiment, both subspecies were expected to be reproductively viable (Fudickar et al., 2016). For this study, we captured a total of 24 adult juncos: 6 male and 6 female resident juncos, and 6 male and 6 female migratory juncos.
Upon capture, we banded each bird with a USFWS numbered metal band and a unique combination of colored plastic bands. We determined sex and subspecies using plumage, beak color, and wing length (Ketterson and Nolan, 1976; Nolan et al., 2022). We collected standard measurements including mass, tarsus length, wing length, tail length, and tail white score (proportion of the area of the outer tail feathers that is white). We also took initial uropygial gland and cloacal swab samples and preen oil samples (see details below).
Housing and social group manipulation
We housed all birds in an outdoor aviary at MLBS and followed long-established animal care protocols for this species (e.g., Enstrom et al., 1997). The aviary was rectangular in shape and comprised a central hallway with multiple enclosures on either side. Each enclosure had a door opening onto the hallway. The inner and outer walls of the aviary were constructed of hardware cloth attached to a wooden frame, which allowed light and air to flow through the aviary and between enclosures. We covered the walls between adjacent enclosures with clear plastic sheeting to limit cross-contamination of bacteria between enclosures. The front side of each enclosure, facing into the central hallway of the aviary, and the back side, facing the forest outside, were left uncovered. Birds could see and hear individuals in other enclosures. At the beginning of the experiment, we collected fresh pine branches to use as perches. All birds were fed the same diet ad libitum, which consisted of millet seeds and mealworms. Water was provided in trays with aquarium heaters to keep water from freezing.
We sampled all birds on their capture day (Time 0, see Figure 1). For the first phase of the experiment (“Initial Flocks”), birds were housed in four same-sex, same-subspecies flocks (six birds in each flock), each in a separate aviary compartment. Birds were held in this phase until four days after the last birds were caught (a minimum of four days for the last birds caught, up to fifteen days for the birds caught at the very beginning of the field season). At the end of this phase (Time 1), we sampled the birds again before moving into the second phase of the experiment (“Pairs”). In the Pairs phase, we housed birds in twelve randomly assigned opposite-sex pairs, each in their own compartment. Eight pairs were comprised of birds from the same subspecies (four resident pairs, four migrant pairs), and four pairs were mixed subspecies (two pairs with a male migrant and a female resident, two pairs with a female migrant and a male resident). The pairs were held in these compartments for eight days, after which they were moved back into their original same-sex, same-subspecies flocks for the third and final phase of the experiment (“Final Flocks”), which lasted another eight days. We sampled all birds every four days after Time 1 (see Figure 1), with the last sample collected at Time 5.
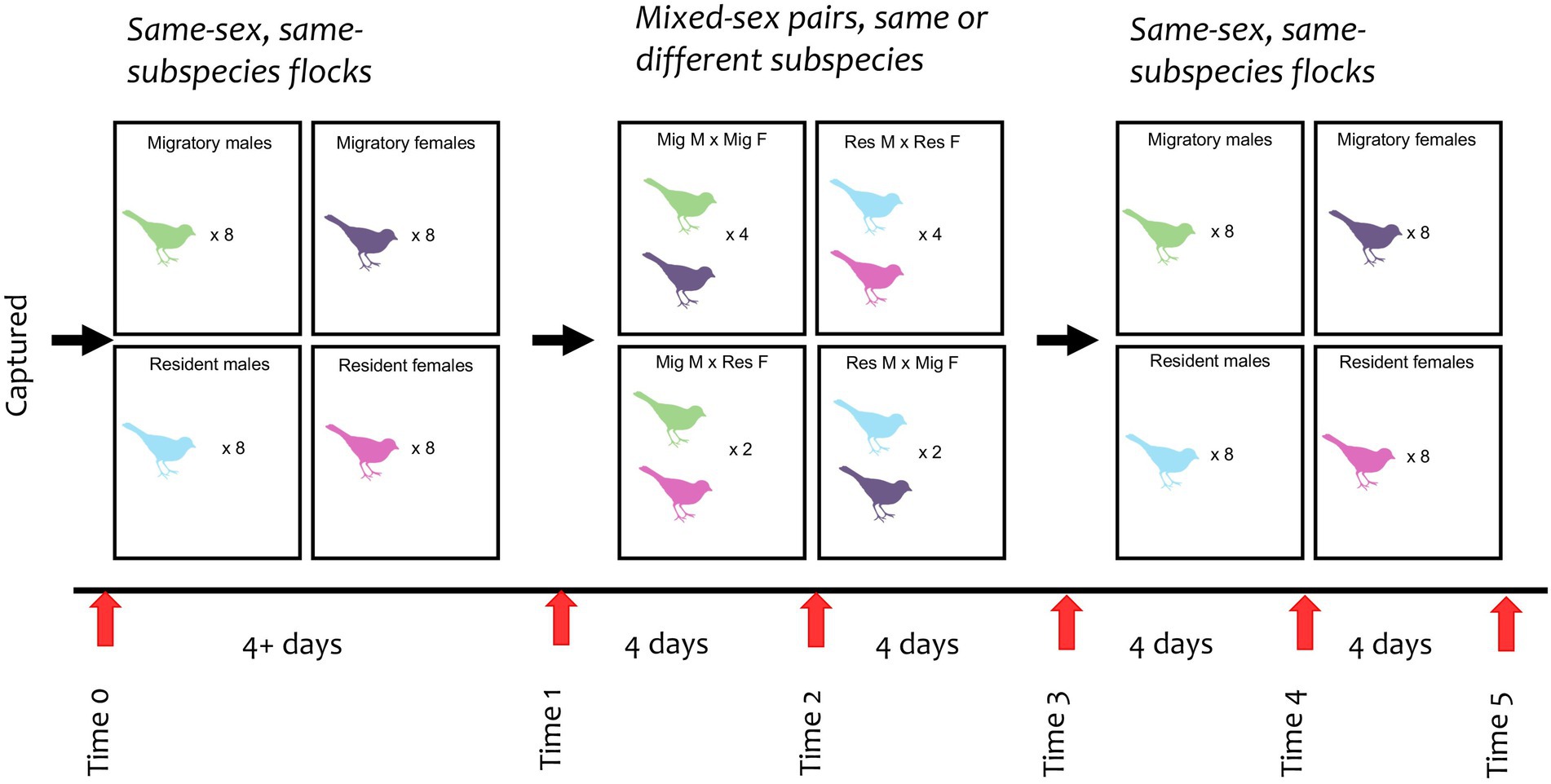
Figure 1. Experimental design of junco flocks and pairs from time 0 to 5. Each bird color represents a sex and subspecies (green = migratory male; purple = migratory female; blue = resident male; dark pink = resident female). Numbers within each box indicates the quantity of birds per flock or pair type. All pairs were housed separately.
At the end of the experiment, the birds were transported to the Kent Farm Bird Observatory at Indiana University for long-term housing and use in additional behavioral and physiological experiments. This study was conducted in compliance with the Indiana University Bloomington Institutional Animal Care and Use Committee guidelines (IACUC protocol 15–026), US Fish and Wildlife Service permit MB093279-1, and Virginia Department of Game and Inland Fisheries permit 058772.
Sample collection
We sampled preen oil at each time point by gently rubbing the uropygial gland with a 100 μl glass capillary tube (Drummond Scientific, Broomall, PA, United States), which stimulates the gland to secrete 1–3 mg of preen oil (Whittaker et al., 2010). We stored preen oil at -20°C within 10 min of collection until we could analyze it using gas chromatography–mass spectrometry (GC–MS, see below). At each time point we also sampled cloacal and uropygial microbial communities with a cotton swab. We first wetted the swab with a sterile enzymatic lysis buffer solution (20 mM Tris pH 8; 2 mM EDTA; 1.2% Triton X-100). We rubbed each sample site (uropygial gland) for at least 5 s to ensure collection of a representative sample. The rubbing motion mimics the birds’ own preen oil collection behavior when preening, and ensured that our samples include a small amount of preen oil and microbes from both inside and outside the gland. Such a mixture represents the same microbial communities that would be present on the birds’ bills when preening. Swab samples were stored at -80° C until analysis.
DNA extraction and microbiome sequencing
We extracted genomic DNA from the swabs using the QIAGEN DNeasy Powerlyzer PowerSoil® DNA Isolation kit (QIAGEN, Germantown, MD, United States), with minor modifications to the manufacturer’s protocol (Whittaker et al., 2019). Due to the low microbial biomass and high concentration of background host DNA in these swab samples, we amplified and sequenced the bacterial DNA using a nested PCR approach (Fan et al., 2009; Yu et al., 2015). The first round of amplifications included 15 cycles, with each reaction containing 1 μl each of the universal 16S rRNA gene primers 27f-CM (5′-AGA GTT TGA TCM TGG CTC AG-3′) and 1492R (5′-ACG GCT ACC TTG TTA CGA CTT-3′), 12.5 μl of 2× GoTaq Green Master Mix (Promega, Madison, WI, United States), and 3.0 μl purified DNA. Following an initial 5 min incubation at 95°C, the cycling parameters were 94°C for 30 s, 50°C for 30 s and 72°C for 120 s. Amplification products were then diluted by 1:15 using nuclease-free water. The primers targeting the V4 region of the 16S rRNA gene were 515F (5′-GTG CCA GCM GCC GCG GTA A-3′) and 806R (5′-GGA CTA CHV GGG TWT CTA AT-3′). PCR was performed under the following conditions: 95°C for 2 min, followed by 30 cycles at 95°C for 20 s, 55°C for 30 s and 72°C for 5 min, with an additional elongation at 72°C for 10 min. We sequenced the V4 region of the 16S rRNA gene on the Illumina MiSeq platform at Michigan State University Research Technology Support Facility’s Genomics Core, using a V2 500 cycle MiSeq Reagent Kit (Illumina MS102-2003), and the dual indexing sequencing strategy developed by Kozich et al. (2013). MiSeq sequence files for all samples have been deposited on the NCBI Sequence Read Archive (BioProject ID PRJNA858485, Accession Numbers SAMN29721623–SAMN29721766).
Preen oil volatile analysis
We used stir bar sorptive extraction (Soini et al., 2005) to extract volatile compounds from preen oil samples. Following our previously successful protocols (e.g., Whittaker et al., 2019), we placed the capillary tube containing the sample into a vial of 2 ml high-purity water, 100 mg of ammonium sulfate, and a Twister® (Gerstel GmbH, Mülheim an der Ruhr, Germany) stir bar. We added an internal standard (8 ng of 7-tridecanone in 5 μl of methanol) to each sample, which was then stirred at ≥ 800 rpm for a 60-min extraction time.
We performed quantitative analysis with an Agilent 6890N gas chromatograph connected to a 5973i MSD mass spectrometer (Agilent Technologies, Inc., Wilmington, DE, United States) with a Thermal Desorption Autosampler and Cooled Injection System (TSDA-CIS 4 from Gerstel) at Indiana University’s Institute for Pheromone Research. We identified all major compounds by comparing mass spectra and retention times to standards (Sigma-Aldrich). We normalized peak areas of the compounds of interest by dividing each peak area by that of the internal standard in corresponding runs, yielding relative concentrations (i.e., relative amounts per 1.0 mg of preen oil).
16S rRNA gene sequence analysis
The paired-end, demultiplexed sequences were imported using QIIME2 v2022.2 (Bolyen et al., 2019). We used the DADA2 plugin to denoise the sequences and to remove phiX and chimeric sequences (Callahan et al., 2016). Based on the quality plot generated, reads were truncated at 220 bp. We used the pre-trained Greengenes full-length database to assign taxonomy (McDonald et al., 2012; Bokulich et al., 2018; Robeson et al., 2021). The R package qiime2R v0.99.6 (Bisanz, 2018) was used to import QIIME2 artifacts into R. We used phyloseq v1.40.0 (McMurdie and Holmes, 2013) to remove rare taxa (relative abundance < 0.005%), generate figures, and perform some statistical analyses. We used the microbiome v1.18.0 (Lati and Shetty, 2017) package to determine the prevalence of ASVs across all samples. The MicEco package v0.9.17 (Russel, 2021) was used to determine shared amplicon sequence variants (ASVs) found in at least 50% of samples in a group. To determine whether identity of the bird contributes to any of our metrics, we performed a repeated measures test using lme4 (Bates et al., 2015) package in R with identity of bird as a random variable. We found that the variance explained by bird random effects was 0.022 for time point, and 0.000 for all other variables and interactions of variables. For alpha diversity metrics, we calculated observed features, Shannon diversity, and Simpson’s diversity index using the estimate_richness feature of phyloseq. Data were tested for normality using the Shapiro–Wilk normality test. Shannon diversity was normally distributed, but observed ASVs and Simpson’s diversity were not. Pairwise comparisons were done using the Wilcoxon rank sum exact test with Holm correction. When a category had three or more levels (flock and time point), we first performed a Kruskal–Wallis rank sum test, followed by the pairwise comparisons as described above. We determined Bray–Curtis distance using the distance feature in phyloseq and visualized them using non-parametric multidimensional scaling (NMDS). The analysis of similarity (ANOSIM) test with 999 permutations was used to determine any differences between communities using the R package vegan v2.6-2 (Oksanen et al., 2022). To determine if preen oil composition was correlated with microbial community composition we performed a Mantel test with vegan using Spearman correlation and 999 permutations. The R package ggplot2 v3.3.6 (Wickham, 2016) was used for figure generation. Finally, we used a Brown–Forsythe test in GraphPad Prism 9.0 to test for significant changes in the spread of microbial diversity for each diversity measure.
Statistical analysis of volatile profiles
Using the GC–MS data, we identified 18 volatile compounds in the preen oil samples, similar to the results of previous studies (Whittaker et al., 2010, 2019). To assess the overall volatile profile of each preen oil sample, we converted the relative abundance of each compound to a proportion of total peak area (Whittaker et al., 2013). Since several values of 0% were present in the dataset, we applied an arcsine transformation to the proportions (Sokal and Rohlf, 2011). We then used a Principal Components Analysis (PCA) with varimax rotation on the arcsine-transformed proportions to reduce the number of variables using JMP 16. We retained principal components (PCs) with eigenvalues greater than 1, allowing us to focus on PC axes that captured more variation than an average original variable. The PCA resulted in 5 PCs, which together explain 72.7% of the variance (Table 1).
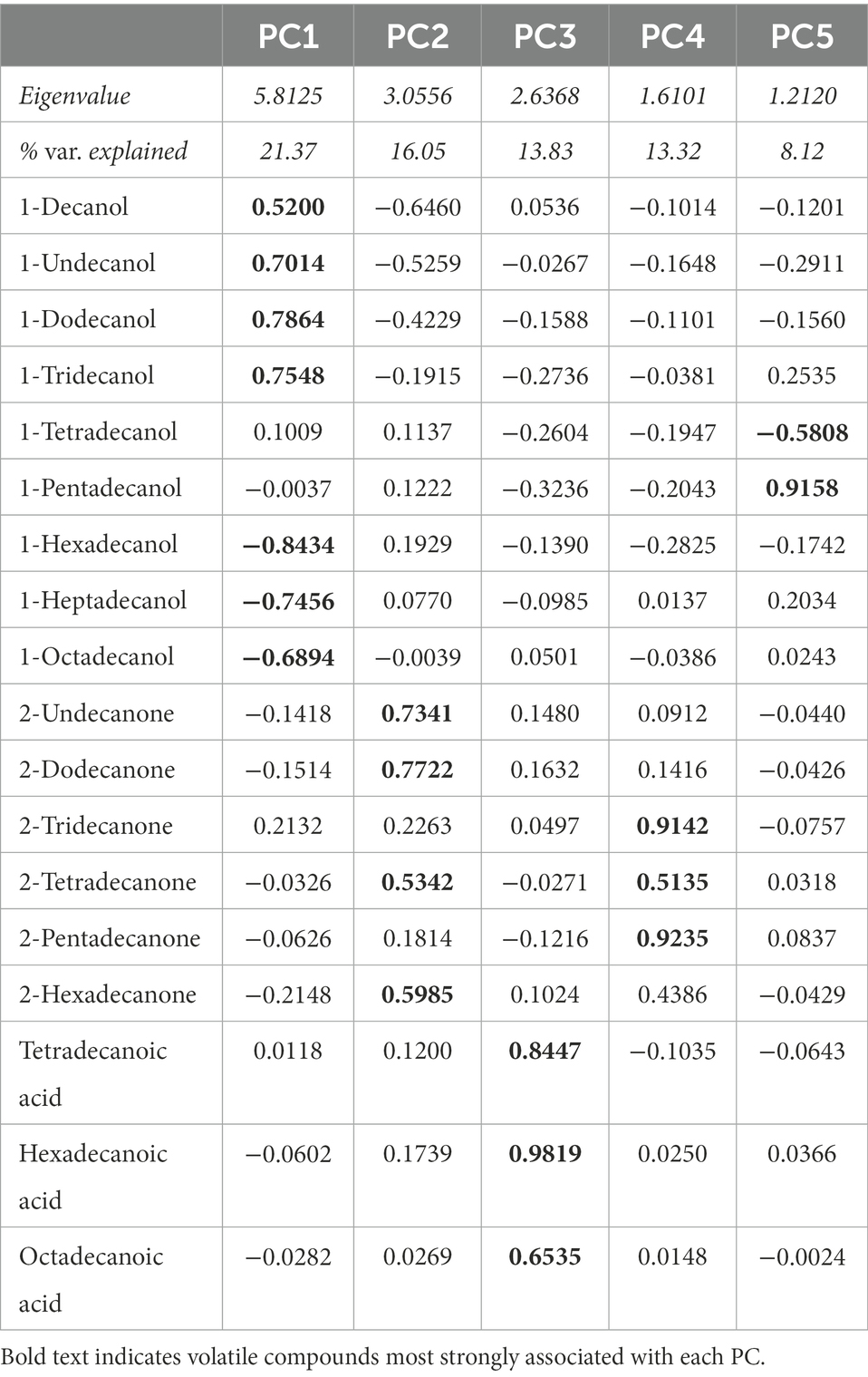
Table 1. Eigenvalues, percentage of variation explained, and variable loading matrix for the principal components analysis (PCA) of volatile compounds present in preen oil samples.
To assess the influence of Flock housing on volatile profile variation, we ran a one-way non-parametric multivariate analysis of variance (NPMANOVA), using the five PCs as the dependent variables and Flock as the independent variable. We used the function adonis in the R package vegan (Oksanen et al., 2022) to run these analyses at each time point, with 10,000 permutations. We used the function pairwise.adonis to run additional posthoc tests for sex and population on any time points that had significant NPMANOVA results.
To examine the influence of Pairs housing on cohabitated male and female pairs, we first calculated the difference in volatile compound composition (as measured by the five principal components) as Euclidean distance measures using the following formula:
where p represents female volatile compound composition and q represents male volatile compound composition; qi and pi represent Euclidean vectors, and n represents the number of principal components. To estimate whether there was a statistically significant difference in the distance between assigned pairmates and that between all possible male–female pairs, we calculated the mean Euclidean distance between all pairmates, and then calculated the mean Euclidean distance for every possible combination of males and females in our sample except for those that were housed together. Due to the relatively small sample size, Euclidean distance for all male and female pairs was directly estimated, rather than using a random sample or simulation. We estimated results at each time point and then compared the principal component scores for the two groups (assigned pairs vs. all other possible pairs) using t-tests in Stata. Due to missing data, sample sizes are not the same at each time point. As a robustness test, the data were re-analyzed using the 18 transformed measures of volatile compounds rather than the principal components. The results were very similar, with only slight, statistically insignificant differences in volatile compounds between assigned and unassigned pairs.
Results
Uropygial gland microbial diversity
Following quality control and removal of rare taxa (relative abundance < 0.005%), we retained 144 samples and 1,671 amplicon sequence variants (ASVs). More than 90% of the ASVs were found in fewer than 20% of our samples. The most abundant phyla were Proteobacteria, Bacteriodetes, Firmicutes, Actinobacteria, and Acidobacteria (Figure 2). When we considered the flocks that our birds were organized into at the beginning and end of the experiment (resident males, migratory males, resident females, and migratory females), we identified only 10 ASVs that were found in at least 50% of individuals in all groups. When we grouped all the samples by their time points, we found four ASVs present in at least 50% of individuals. There were 12 ASVs shared at this level between resident and migratory birds and 12 between male and female birds.
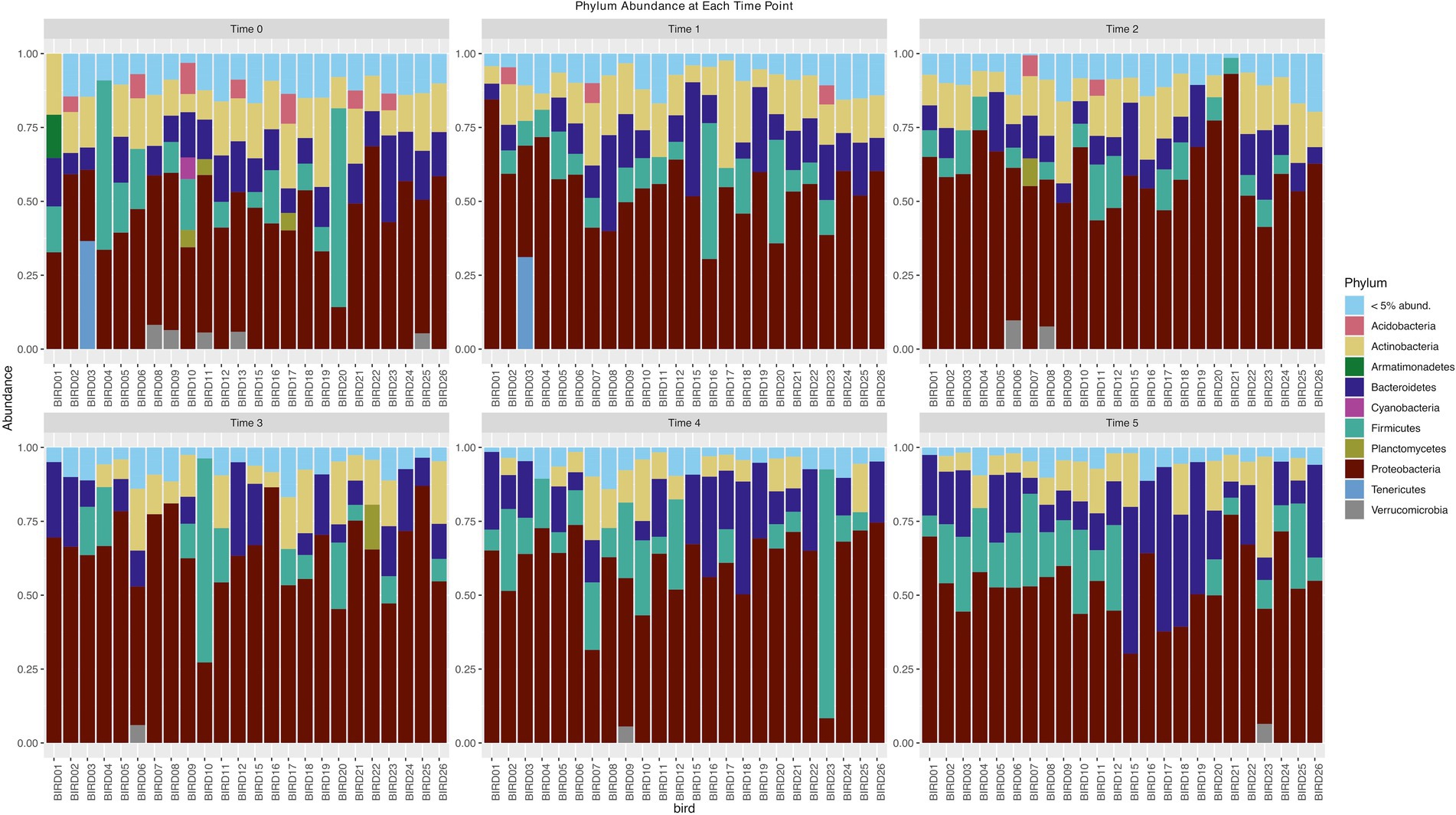
Figure 2. Phylum abundance of bacteria phyla for each time point of the experiment. Each bar represents an individual junco.
We used Observed ASVs, Shannon, and Simpsons indices as measures of diversity. Statistical significance was determined using the Wilcoxon rank sum test with Holm correction for multiple comparisons. We found no significant differences between the sexes (Observed ASVs W = 2133, p = 0.067; Shannon index W = 2148, p = 0.076; Simpsons index W = 2132, p = 0.066; Figure 3A). When comparing the various flocks, we found significant differences with all three measures of diversity using the Kruskal–Wallis rank sum test (Observed ASVs χ2 = 10.306, p = 0.016; Shannon index χ2 = 10.641, p = 0.014; Simpson index χ2 = 14.215, p = 0.003). Pairwise comparisons with the Wilcoxon rank sum test with Holm correction found the diversity to be significantly lower in migratory females compared to resident males with all three metrics (Observed ASVs p = 0.006, Shannon index p = 0.011, Simpsons index p = 0.010; Figure 3B). Migratory females also had significantly lower diversity than resident females based on Simpsons index (p = 0.008). Overall, migratory birds had significantly lower diversity than residents (Observed ASVs W = 1970.5, p = 0.013; Shannon index W = 1978, p = 0.014; Simpsons index W = 1984, p = 0.015; Figure 3C). There were no significant differences between the other groups. Finally, when comparing the different time points, we found significant differences with all three measures of diversity using the Kruskal–Wallis rank sum test (Observed ASVs χ2 = 20.126, p = 0.001; Shannon index χ2 = 21.692, p < 0.001; Simpson index χ2 = 15.164, p = 0.010; Figure 4). Pairwise comparisons with the Wilcoxon rank sum test with Holm correction found the diversity to be significantly lower at time 3 when compared to time 0 using Shannon and Simpsons indices (p = 0.024 and p = 0.030, respectively). Time 3 was significantly lower than time 1 with Observed ASVs (p = 0.016) and Shannon index (p = 0.036). Time 4 was significantly lower than time 0 with the Shannon index (p = 0.044) and lower than time 1 with Observed ASVs (p = 0.024). All other comparisons were not significant. When comparing the data for each diversity index across the time points, there is a significant decrease in observed ASVs (r = −0.41, p < 0.0001; Figure 4A) and Shannon index (r = −0.32, p < 0.0001; Figure 4B), but not for the Simpsons index (r = −0.041, p = 0.62; Figure 4C). In addition to the overall alpha diversity decreasing over the course of the experiment, we also see a significant reduction in the spread of data at each progressive time point (Figure 4) for observed ASVs (Brown–Forsythe F = 12.01, p < 0.0001), and Shannon index (Brown–Forsythe F = 4.80, p = 0.0004), but not for the Simpson index (Brown–Forsythe F = 0.531, p = 0.753).
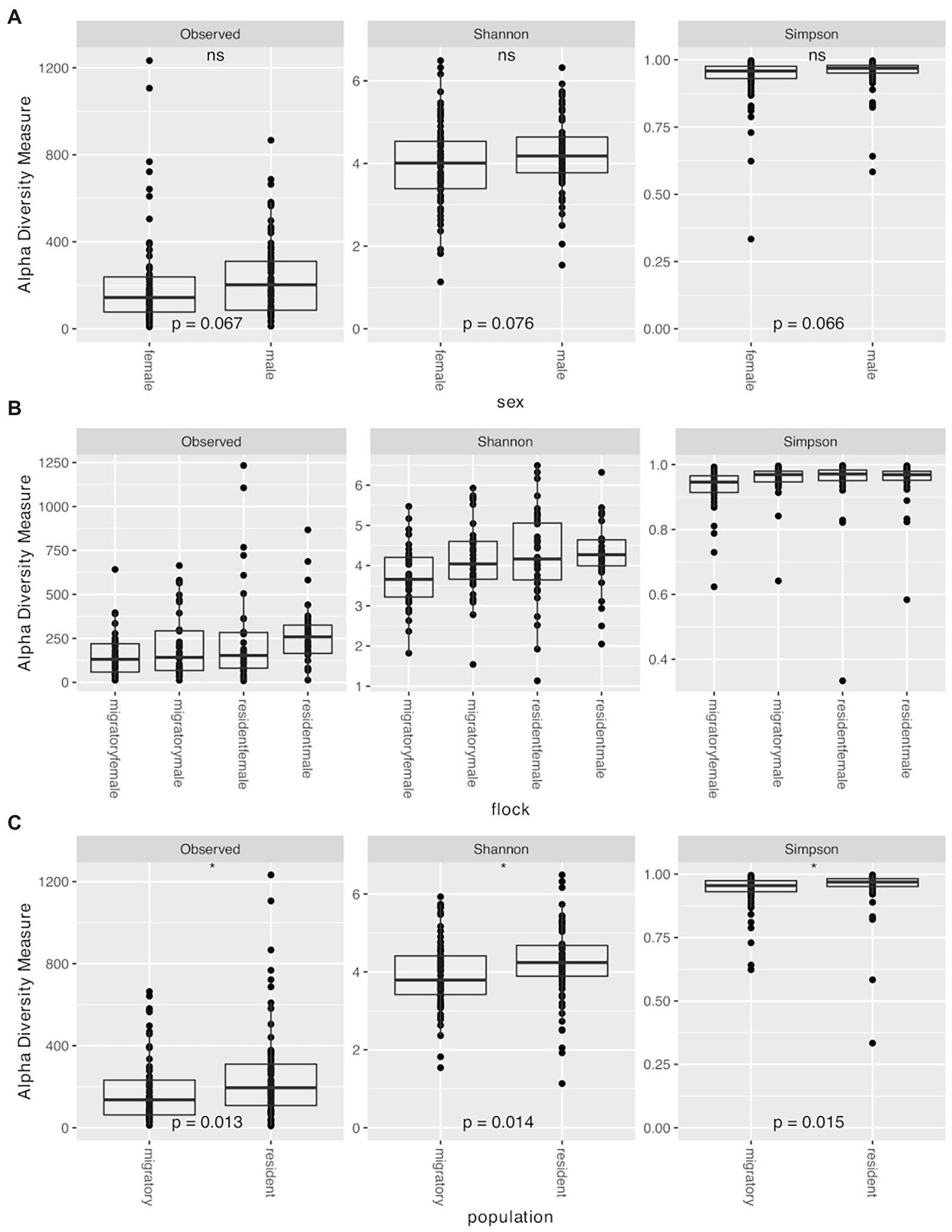
Figure 3. Alpha diversity measures (Observed ASVs, Shannon, and Simpson) for the juncos sampled in the experiment based on (A) sex, (B) flock group for the entire experiment (time 1 through time 5), and (C) population (sub-species). Asterisks denote significance (p < 0.05).
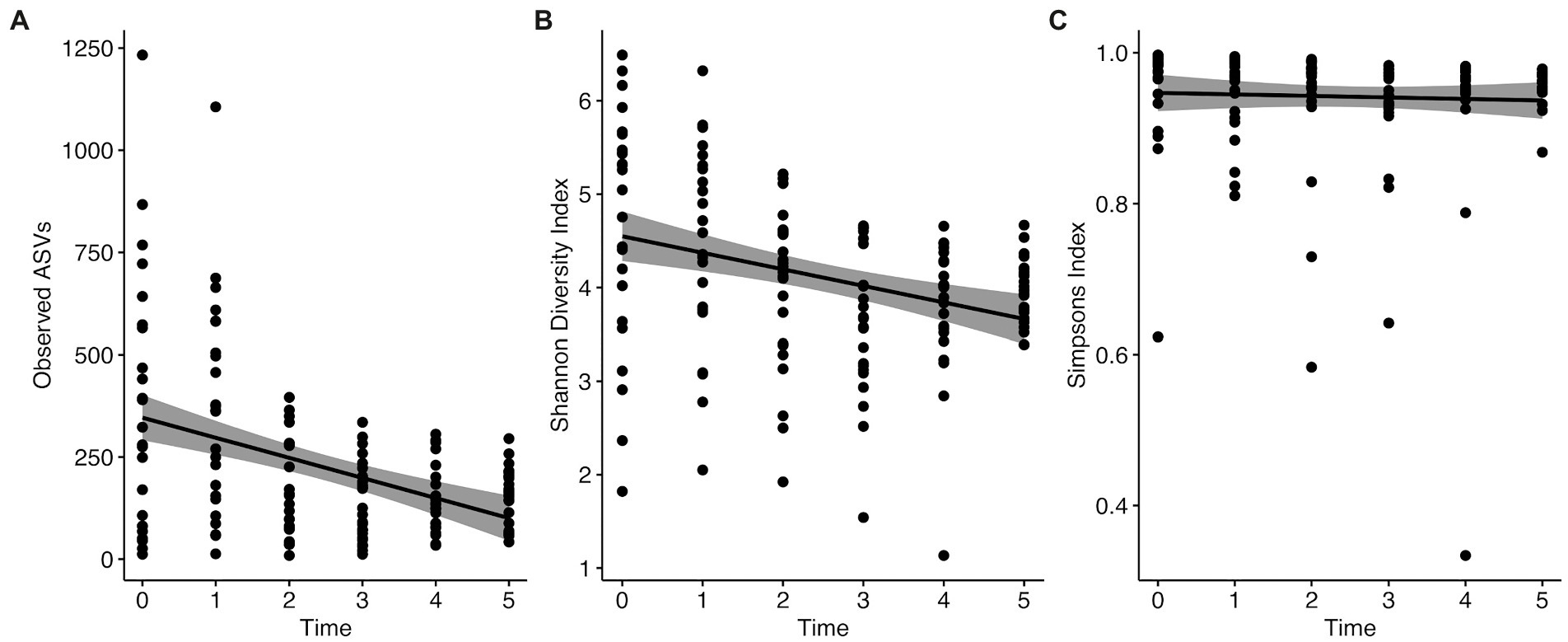
Figure 4. Change in alpha diversity measures from time 0 to time 5 of the experiment. (A) Observed ASVs, (B) Shannon Index, and (C) Simpson index.
Bray–Curtis dissimilarity was used to determine any differences in community composition between various sample groups. We used Analysis of Similarity (ANOSIM) to determine if there were significant differences (Table 2) and plotted Bray-Curtis distances using non-metric multidimensional scaling plots (Figure 5). Comparing the sexes showed no significant difference (p = 0.12) and a low r value (r < 0.01). While all other group comparisons showed significant differences (all comparisons, p < 0.0001), only the comparison between the various time points had an r value above 0.1 (r = 0.2547), indicating a slightly higher dissimilarity between the time points, though this value is still low. We found that microbial community composition was weakly correlated with overall preen oil abundance (r = 0.148; p = 0.0011), but when we considered only samples collected at the time of capture (t = 0), the correlation was stronger (r = 0.504; p = 0.0019) according to Mantel tests.
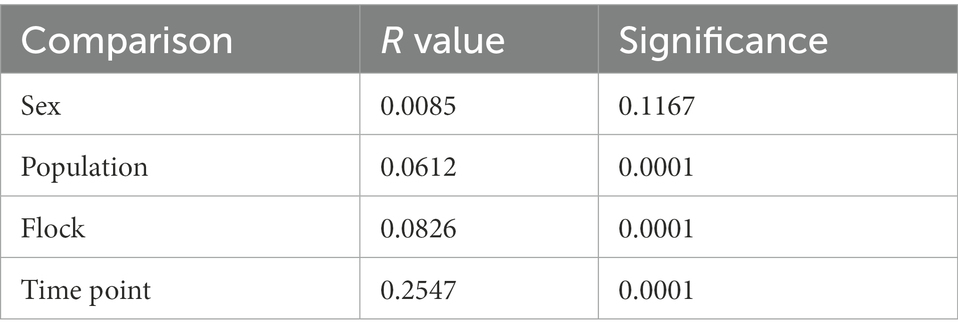
Table 2. Analysis of similarity (ANOSIM) results of junco preen gland microbial communities comparing sex, populations (sub-species), flock, and time point.
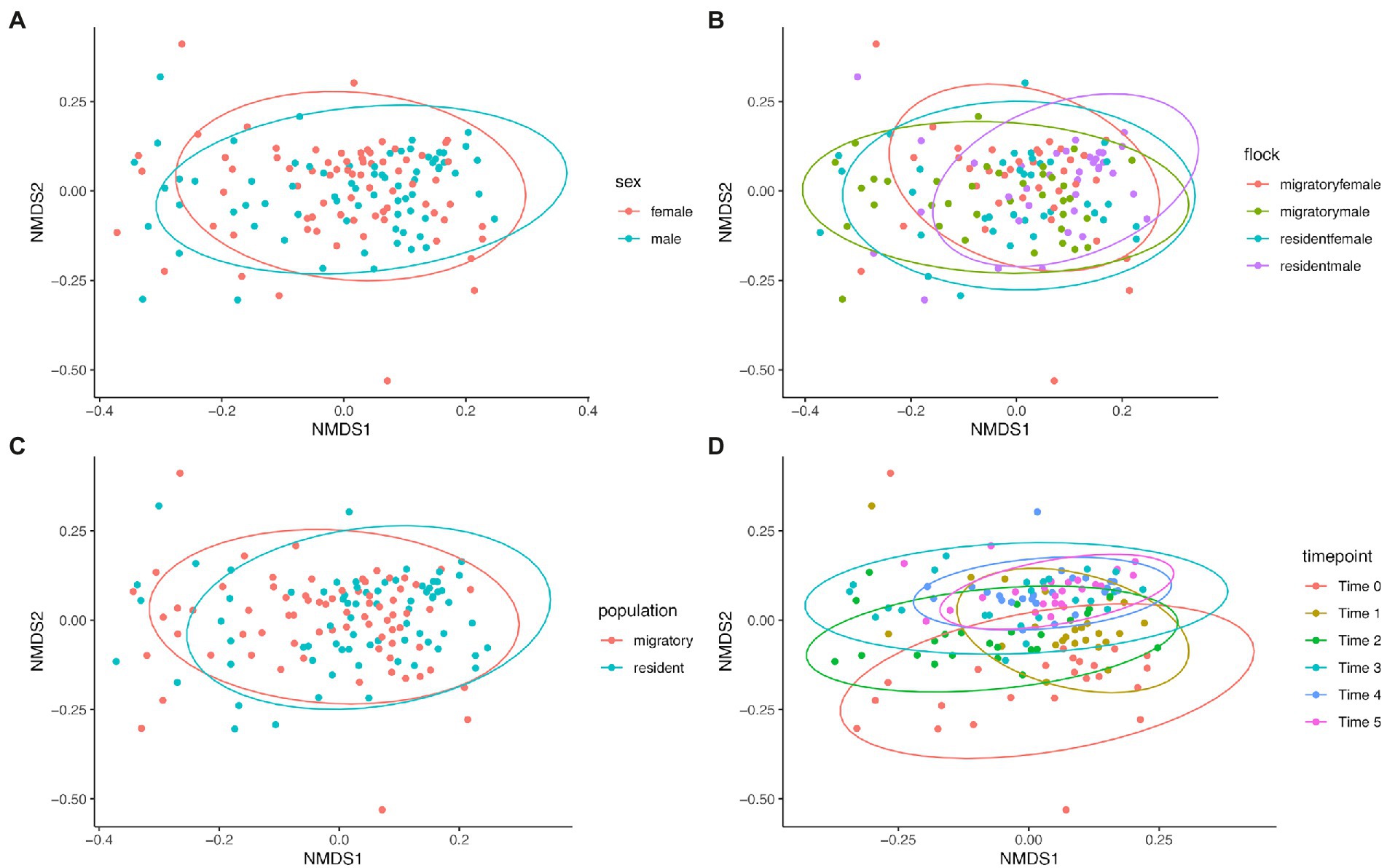
Figure 5. NMDS plots of Bray-Curtis dissimilarities between (A) sexes, (B) flock group, (C) population (sub-species), and (D) sampling time points.
Volatile profiles analysis
We used a one-way NPMANOVA to test for differences in volatile profiles between the assigned flocks at each time point (Table 3). At time point 3 (end of Pairs treatment/beginning of Final Flocks treatment), the four flocks were significantly different (F = 0.601, df = 23, p = 0.0003). Posthoc tests showed that the sexes differed significantly at this time point (F = 2.62, p = 0.036), as did the two populations (F = 2.40, p = 0.043). The four flocks were not significantly different at any other time point (Table 3, all comparisons: p > 0.1).
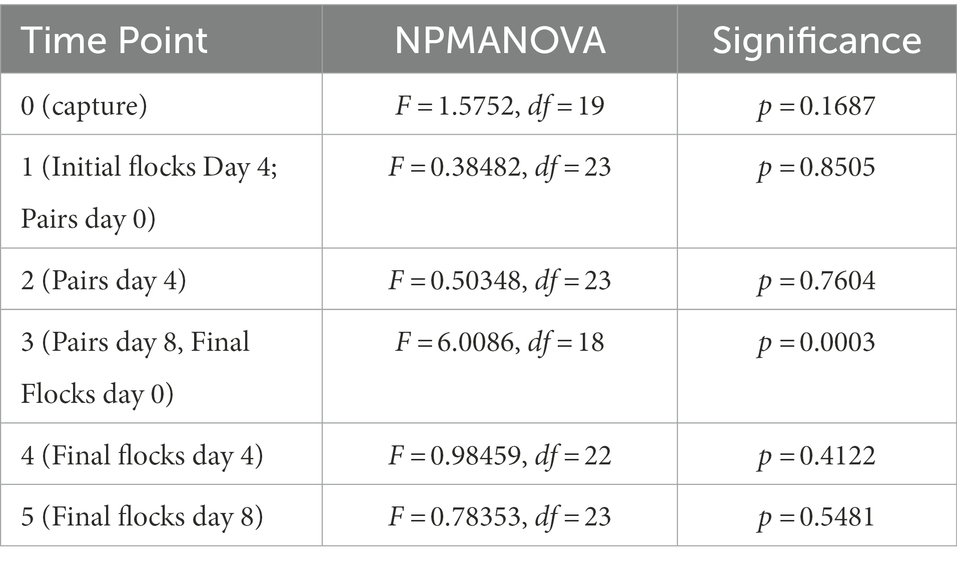
Table 3. Non-parametric multivariate of variance (NPMANOVA) analysis of volatile profile differences between flocks at each time point of the study.
To test whether the assigned male–female pairs had volatile profiles that were more like each other than like other birds in the study, we compared the Euclidean distance between assigned pairs and all possible unassigned male–female pairs at each time point using t-tests. Distance between assigned and unassigned pairs did not differ significantly at any time point in the study (Table 4).
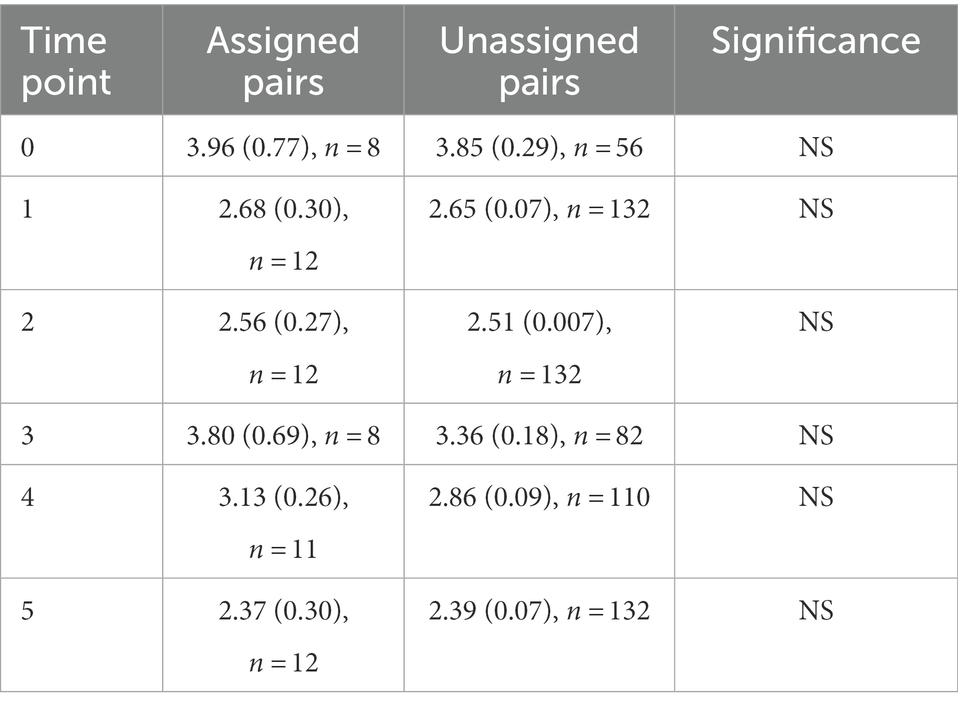
Table 4. Mean Euclidean distances of assigned and unassigned pairs, Differences between the two groups were assessed using a t-test, which showed no significance for any time point.
Discussion
Our experiment investigated whether social contact affects uropygial gland microbial communities and preen oil volatile compounds in dark-eyed juncos. We manipulated social contact in wild-caught birds by first housing them in same-sex, same-subspecies flocks, then moving them into male–female pairs, and finally returning them to their original flocks. We sampled uropygial gland communities and preen oil at several time points throughout the experiment and tested for differences between flocks, pairs, sexes, and subspecies.
We hypothesized that short-term changes in social environment would affect microbial community diversity and structure, with birds that were housed together becoming more like each other over the course of their time housed together. We also hypothesized that if the birds’ microbial communities became more similar, then their volatile profiles would change in the same direction. Our results do not show any clear trends that could be explained by social contact for all time points across the experiment. However, we did find several differences in microbial diversity between sexes, subspecies, and specific flocks, as well as a significant difference among flocks in preen oil volatiles at one time point midway through the experiment. These results suggest that background genetic differences between the subspecies, as well as hormonal differences between the sexes when in breeding condition, may have a stronger influence on symbiotic microbial communities and volatile profiles than social environment.
Uropygial gland microbiome
There were no significant differences in microbial diversity measures between groups housed together across the experimental time points, whether they were housed in flocks or pairs. Differences in diversity measures did appear between sexes, migrants and residents: females had lower diversity than males, migrants had lower diversity than residents, and migratory females had the lowest diversity of the four flocks. When comparing microbial composition between groups, we found significant differences between subspecies and flocks, but no difference between sexes. These patterns suggest that genetic background has a greater influence on uropygial gland microbial community diversity and composition than sex or social contact. These findings are also consistent with a previous study on the effects of social group membership on uropygial gland microbial communities and preen oil volatile profiles in free-living dark-eyed juncos, in which no differences were found between the sexes (Whittaker et al., 2016). We also found a negative correlation between microbial alpha and beta diversity, and sampling time point, whereby at the end of the experiment, the juncos’ uropygial gland microbiome had a drop in observed ASVs and Shannon diversity index, but showed no change in Simpson diversity index. Since the birds were captured and brought into captivity at the beginning of the experiment, this result suggests that time spent in captivity causes microbial diversity to decrease. Other captive bird experiments also show a drop in alpha diversity (Xenoulis et al., 2010; Wienemann et al., 2011; Ushida et al., 2016; Kelly et al., 2022). For example, captivity, not neophobia phenotype, in house sparrows (Passer domesticus) best explained a drop in alpha diversity (Kelly et al., 2022). A decrease in alpha diversity over time is likely reflected by similar environmental housing conditions (Rothschild et al., 2018), as the variation in observed ASVs and Shannon index between individuals from Time Point 0 to Time Point 5 decreases.
Several studies of social networks in mammals have shown that social group membership, social networks, and physical contact are predictors of composition of gut microbiomes, [e.g., chimpanzees (Pan troglodytes; Moeller et al., 2016); baboons (Papio cynocephalus; Tung et al., 2015); Verreaux’s sifaka (Propithecus verreauxi; Perofsky et al., 2017)], skin microbiomes, [e.g., humans, (Meadow et al., 2013; Song et al., 2013)], and scent gland microbiomes [e.g., spotted hyenas (Crocuta crocuta; Theis et al., 2012); meerkats (Suricata suricatta; Leclaire et al., 2014)]. Horizontal transmission of microbes is thought to play a key role in determining an animal’s microbiome composition, and such transmission can be promoted by behaviors such as allogrooming (Tung et al., 2015), coprophagy (Kobayashi et al., 2019) and, in the case of scent gland bacterial communities, overmarking (Theis et al., 2008) and allomarking (Buesching et al., 2003).
In birds, evidence for the role of social behavior in microbiome composition has been mixed. In dark-eyed juncos and Eurasian hoopoes (Upupa epops), group membership was the most important driver of uropygial gland microbiome similarity, with parents and nestlings from the same nest all having high similarity (Ruiz-Rodríguez et al., 2014; Whittaker et al., 2016). This finding was upheld even when the nestlings were unrelated to one or both parents, either as the result of extra-pair fertilizations (dark-eyed juncos) or cross-fostering (Eurasian hoopoes). Similarly, members of the same zebra finch families had similar skin microbiomes, and spatial proximity of nest sites correlated with similarity between families (Engel et al., 2020). However, a study of Leach’s storm petrels found that mated males and females were not more similar to each other at their uropygial and skin microbiomes than randomly selected individuals from the population (Pearce et al., 2017). Junco and hoopoe parents interact frequently while raising their offspring, but petrel parents spend much less time together, as they alternate spending days at sea foraging. These observations, together with the results of this study, suggest that repeated social interactions over an extended period can result in similar microbiome composition, but that short-term changes in the social environment are not sufficient to have this effect.
Preen oil volatile profiles
We found a significant difference in preen oil volatile compounds between the flocks only at time point 3. However, this time point was not at the end of a period when flocks had been housed together, but instead when the male–female pairs had been housed together for 8 days. A confounding factor when interpreting this result is that the flocks are also separated by sex and subspecies. Differences among the four flocks (migratory females, migratory males, resident females, and resident males) could be explained by seasonal hormonal changes that were not the result of housing manipulation. Our experiment took place in the early spring, which meant that we moved the birds into mixed-sex pairs at a time when they typically experience seasonal hormonal changes associated with breeding condition. Notably, migratory and resident juncos are also known to exhibit differences in the timing of these hormonal changes (Fudickar et al., 2016; Kimmitt et al., 2019), which could explain why we observed differences in volatile profiles between the subspecies as well as between the sexes. The observed differences in volatile compound composition at time point 3 disappeared after the birds were moved back into their same-sex, same-subspecies flocks, suggesting that the stimulation of being housed with an opposite-sex bird may have been responsible for the changes in volatile profiles. Furthermore, at no time point were the birds assigned to the same pairs more like each other than any other random male–female pair from the experiment. In other words, housing a male and a female together for 8 days did not make them smell more like each other.
Another confounding factor in interpreting our results is our choice to create same-sex, same-subspecies flocks for the first and last phases of the experiment. This study design makes it difficult to be certain whether differences observed between the flocks were due to subspecies and sex or due to housing configuration. However, if the housing configuration had caused the birds housed together to have more similar microbial compositions and preen oil volatile profiles, we would expect them to become more similar over the course of the experiment, which we did not observe. Additionally, the birds were brought into captivity at the beginning of the experiment, and any changes in their microbiomes related to experimental conditions cannot be disentangled from the effects of captivity.
Conclusion
In this study, we tested hypotheses about the role of social environment on the composition of microbial communities associated with the uropygial gland, and the resulting effects on the composition of preen oil volatile compounds. Specifically, we investigated whether short-term changes in social group composition in captivity led to related changes in microbiome and volatile profiles. Although the birds’ uropygial microbial communities and preen oil volatile profiles did change over the course of the experiment, these changes could not be attributed to the social group manipulations. Instead, we found that differences in microbiome composition were related to sex and subspecies, suggesting that genetic background was a more important determinant than social environment. The two subspecies of dark-eyed juncos in this experiment also have different migratory patterns—the Carolina subspecies is sedentary, while the Northern subspecies is a long-distance migrant—and migratory history could play a bigger role in shaping a bird’s microbiome than comparatively short periods of time spent housed with another individual. Changes in the birds’ volatile profiles differed by sex and subspecies, and did so only after they had been housed with an opposite-sex partner. Furthermore, we conducted this experiment in the early spring, when birds are entering breeding condition. This pattern suggests that these volatile compound changes were due to hormonal shifts related to reproduction, and while social environment (being housed with an opposite sex bird) may have triggered these shifts, the change cannot be attributed to microbe sharing. At least in the case of short-term changes in the social group composition of adults, individual identity and hormonal status appear to be much more important drivers of both uropygial gland microbial community composition and preen oil volatile compound profiles.
Data availability statement
The data presented in the study are deposited in the NCBI Short Read Archive (SRA) repository (https://www.ncbi.nlm.nih.gov/sra), BioProject PRJNA858485, accession number SRR20173167-SRR20173310.
Ethics statement
The animal study was reviewed and approved by Indiana University Bloomington Institutional Animal Care and Use Committee guidelines (IACUC protocol 15-026), US Fish and Wildlife Service permit MB093279-1, and Virginia Department of Game and Inland Fisheries permit 058772.
Author contributions
DW and JS designed the experiment. DW, NB, and JS drafted the manuscript. DW, JS, and TH performed the field collection of the juncos and collected the preen oil and microbial samples throughout the experiment. MN and HS processed the preen oil using GC–MS. DW and NB statistically analyzed the preen oil data. AA, JG, and KT extracted the microbial DNA and prepared them for sequencing. TV analyzed the microbial data. All authors contributed to the article and approved the submitted version.
Funding
This work was supported by the BEACON Center for the Study of Evolution in Action (National Science Foundation DBI-0939454).
Acknowledgments
We thank the University of Virginia, Mountain Lake Biological Station, and the Mountain Lake Lodge. We are grateful to Ellen Ketterson for providing facilities and intellectual support for this project. We would like to thank Adam Fudickar and Devraj Singh for helping to sample birds in March 2018.
Conflict of interest
NB was employed by Public Policy Associates, Inc.
The remaining authors declare that the research was conducted in the absence of any commercial or financial relationships that could be construed as a potential conflict of interest.
Publisher’s note
All claims expressed in this article are solely those of the authors and do not necessarily represent those of their affiliated organizations, or those of the publisher, the editors and the reviewers. Any product that may be evaluated in this article, or claim that may be made by its manufacturer, is not guaranteed or endorsed by the publisher.
References
Archie, E. A., and Theis, K. R. (2011). Animal behaviour meets microbial ecology. Anim. Behav. 82, 425–436. doi: 10.1016/j.anbehav.2011.05.029
Bates, D., Mächler, M., Bolker, B., and Walker, S. (2015). Fitting linear mixed-effects models using lme4. J. Stat. Soft. 67, 1–48. doi: 10.18637/jss.v067.i01
Bisanz, J. E. (2018). qiime2R: importing QIIME2 artifacts and associated data into R sessions. Available at: https://github.com/jbisanz/qiime2R (Accessed May 30, 2022).
Bokulich, N. A., Kaehler, B. D., Rideout, J. R., Dillon, M., Bolyen, E., Knight, R., et al. (2018). Optimizing taxonomic classification of marker-gene amplicon sequences with QIIME 2’s q2-feature-classifier plugin. Microbiome 6:90. doi: 10.1186/s40168-018-0470-z
Bolyen, E., Rideout, J. R., Dillon, M. R., Bokulich, N. A., Abnet, C. C., Al-Ghalith, G. A., et al. (2019). Reproducible, interactive, scalable and extensible microbiome data science using QIIME 2. Nat. Biotechnol. 37, 852–857. doi: 10.1038/s41587-019-0209-9
Bordenstein, S. R., and Theis, K. R. (2015). Host biology in light of the microbiome: ten principles of holobionts and hologenomes. PLoS Biol. 13:e1002226. doi: 10.1371/journal.pbio.1002226
Bosch, T. C. G., and McFall-Ngai, M. J. (2011). Metaorganisms as the new frontier. Zoology (Jena) 114, 185–190. doi: 10.1016/j.zool.2011.04.001
Buesching, C. D., Stopka, P., and Macdonald, D. W. (2003). The social function of Allo-marking in the European badger (Meles meles). Behaviour 140, 965–980. doi: 10.1163/156853903322589597
Burns, A. R., Miller, E., Agarwal, M., Rolig, A. S., Milligan-Myhre, K., Seredick, S., et al. (2017). Interhost dispersal alters microbiome assembly and can overwhelm host innate immunity in an experimental zebrafish model. Proc. Natl. Acad. Sci. 114, 11181–11186. doi: 10.1073/pnas.1702511114
Callahan, B. J., McMurdie, P. J., Rosen, M. J., Han, A. W., Johnson, A. J. A., and Holmes, S. P. (2016). DADA2: high-resolution sample inference from Illumina amplicon data. Nat. Methods 13, 581–583. doi: 10.1038/nmeth.3869
Caro, S. P., Balthazart, J., and Bonadonna, F. (2015). The perfume of reproduction in birds: chemosignalling in avian social life. Horm. Behav. 68, 25–42. doi: 10.1016/j.yhbeh.2014.06.001
Engel, K., Pankoke, H., Jünemann, S., Brandl, H. B., Sauer, J., Griffith, S. C., et al. (2020). Family matters: skin microbiome reflects the social group and spatial proximity in wild zebra finches. BMC Ecol. 20:58. doi: 10.1186/s12898-020-00326-2
Enstrom, D. A., Ketterson, E. D., and Nolan, J. V. (1997). Testosterone and mate choice in the dark-eyed junco. Anim. Behav. 54, 1135–1146. doi: 10.1006/anbe.1997.0555
Ezenwa, V. O., and Williams, A. E. (2014). Microbes and animal olfactory communication: where do we go from here? BioEssays 36, 847–854. doi: 10.1002/bies.201400016
Fan, Z.-Y., Li, X.-R., Mao, D.-P., Zhu, G.-F., Wang, S.-Y., and Quan, Z.-X. (2009). Could nested PCR be applicable for the study of microbial diversity? World J. Microbiol. Biotechnol. 25, 1447–1452. doi: 10.1007/s11274-009-0033-3
Fudickar, A. M., Greives, T. J., Atwell, J. W., Stricker, C. A., and Ketterson, E. D. (2016). Reproductive allochrony in seasonally sympatric populations maintained by differential response to photoperiod: implications for population divergence and response to climate change. Am. Nat. 187, 436–446. doi: 10.1086/685296
Goodenough, A. E., Stallwood, B., Dandy, S., Nicholson, T. E., Stubbs, H., and Coker, D. G. (2017). Like mother like nest: similarity in microbial communities of adult female pied flycatchers and their nests. J. Ornithol. 158, 233–244. doi: 10.1007/s10336-016-1371-1
Kelly, T. R., Vinson, A. E., King, G. M., and Lattin, C. R. (2022). No guts about it: captivity, but not neophobia phenotype, influences the cloacal microbiome of house sparrows (Passer domesticus). Integr. Org. Biol. 4:obac010. doi: 10.1093/iob/obac010
Ketterson, E. D., and Nolan, V. (1976). Geographic variation and its climatic correlates in the sex ratio of eastern-wintering dark-eyed juncos (junco Hyemalis Hyemalis). Ecology 57, 679–693. doi: 10.2307/1936182
Khan, M. A. W., Stephens, W. Z., Mohammed, A. D., Round, J. L., and Kubinak, J. L. (2019). Does MHC heterozygosity influence microbiota form and function? PLoS One 14:e0215946. doi: 10.1371/journal.pone.0215946
Kimmitt, A. A., Hardman, J. W., Stricker, C. A., and Ketterson, E. D. (2019). Migratory strategy explains differences in timing of female reproductive development in seasonally sympatric songbirds. Funct. Ecol. 33, 1651–1662. doi: 10.1111/1365-2435.13386
Kobayashi, A., Tsuchida, S., Ueda, A., Yamada, T., Murata, K., Nakamura, H., et al. (2019). Role of coprophagy in the cecal microbiome development of an herbivorous bird Japanese rock ptarmigan. J. Vet. Med. Sci. 81, 1389–1399. doi: 10.1292/jvms.19-0014
Kozich, J. J., Westcott, S. L., Baxter, N. T., Highlander, S. K., and Schloss, P. D. (2013). Development of a dual-index sequencing strategy and curation pipeline for analyzing amplicon sequence data on the MiSeq Illumina sequencing platform. Appl. Environ. Microbiol. 79, 5112–5120. doi: 10.1128/AEM.01043-13
Kubinak, J. L., Stephens, W. Z., Soto, R., Petersen, C., Chiaro, T., Gogokhia, L., et al. (2015). MHC variation sculpts individualized microbial communities that control susceptibility to enteric infection. Nat. Commun. 6:8642. doi: 10.1038/ncomms9642
Kulkarni, S., and Heeb, P. (2007). Social and sexual behaviours aid transmission of bacteria in birds. Behav. Process. 74, 88–92. doi: 10.1016/j.beproc.2006.10.005
Lati, L., and Shetty, S. (2017). Tools for microbiome analysis in R. Version 1.18.0. Available at: https://microbiome.github.com/microbiome (Accessed May 30, 2022).
Leclaire, S., Nielsen, J. F., and Drea, C. M. (2014). Bacterial communities in meerkat anal scent secretions vary with host sex, age, and group membership. Behav. Ecol. 25, 996–1004. doi: 10.1093/beheco/aru074
Lombardo, M. P. (2008). Access to mutualistic endosymbiotic microbes: an underappreciated benefit of group living. Behav. Ecol. Sociobiol. 62, 479–497. doi: 10.1007/s00265-007-0428-9
Maraci, Ö., Engel, K., and Caspers, B. A. (2018). Olfactory communication via microbiota: what is known in birds? Genes 9:387. doi: 10.3390/genes9080387
McDonald, D., Price, M. N., Goodrich, J., Nawrocki, E. P., DeSantis, T. Z., Probst, A., et al. (2012). An improved Greengenes taxonomy with explicit ranks for ecological and evolutionary analyses of bacteria and archaea. ISME J. 6, 610–618. doi: 10.1038/ismej.2011.139
McMurdie, P. J., and Holmes, S. (2013). Phyloseq: an R package for reproducible interactive analysis and graphics of microbiome census data. PLoS One 8, –e61217. doi: 10.1371/journal.pone.0061217
Meadow, J. F., Bateman, A. C., Herkert, K. M., O’Connor, T. K., and Green, J. L. (2013). Significant changes in the skin microbiome mediated by the sport of roller derby. PeerJ 1:e53. doi: 10.7717/peerj.53
Moeller, A. H., Foerster, S., Wilson, M. L., Pusey, A. E., Hahn, B. H., and Ochman, H. (2016). Social behavior shapes the chimpanzee pan-microbiome. Sci. Adv. 2:e1500997. doi: 10.1126/sciadv.1500997
Nolan, J. V., Ketterson, E. D., Cristol, D. A., Rogers, C. M., Clotfelter, E. D., Titus, R. C., et al. (2022). “Dark-eyed junco (Junco hyemalis)” in Birds of Thet World. eds. A. F. Poole and F. B. Gill (Ithaca, NY: Cornell Lab of Ornithology)
Oksanen, J., Simpson, G. L., Blanchet, F. G., Kindt, R., Legendre, P., Minchin, P. R., et al. (2022). Vegan: Community ecology package. Available at: https://CRAN.R-project.org/package=vegan (Accessed August 16, 2022).
Osawa, R., Blanshard, W., and Ocallaghan, P. (1993). Microbiological studies of the intestinal microflora of the koala, Phascolarctos-Cinereus. 2. Pap, a special maternal feces consumed by juvenile koalas. Aust. J. Zool. 41:611. doi: 10.1071/ZO9930611
Pearce, D. S., Hoover, B. A., Jennings, S., Nevitt, G. A., and Docherty, K. M. (2017). Morphological and genetic factors shape the microbiome of a seabird species (Oceanodroma leucorhoa) more than environmental and social factors. Microbiome 5:146. doi: 10.1186/s40168-017-0365-4
Perofsky, A. C., Lewis, R. J., Abondano, L. A., Di Fiore, A., and Meyers, L. A. (2017). Hierarchical social networks shape gut microbial composition in wild Verreaux’s sifaka. Proc. R. Soc. B Biol. Sci. 284:20172274. doi: 10.1098/rspb.2017.2274
Peterson, M. P., Whittaker, D. J., Ambreth, S., Sureshchandra, S., Buechlein, A., Podicheti, R., et al. (2012). De novo transcriptome sequencing in a songbird, the dark-eyed junco (Junco hyemalis): genomic tools for an ecological model system. BMC Genom. 13:305. doi: 10.1186/1471-2164-13-305
Robeson, M. S. II, O’Rourke, D. R., Kaehler, B. D., Ziemski, M., Dillon, M. R., Foster, J. T., et al. (2021). RESCRIPt: Reproducible sequence taxonomy reference database management. PLoS Comput. Biol. 17:e1009581. doi: 10.1371/journal.pcbi.1009581
Rosenberg, E., Sharon, G., and Zilber-Rosenberg, I. (2009). The hologenome theory of evolution contains Lamarckian aspects within a Darwinian framework. Environ. Microbiol. 11, 2959–2962. doi: 10.1111/j.1462-2920.2009.01995.x
Rosenberg, E., and Zilber-Rosenberg, I. (2011). Symbiosis and development: the hologenome concept. Birth Defects Res. C Embryo Today 93, 56–66. doi: 10.1002/bdrc.20196
Rothschild, D., Weissbrod, O., Barkan, E., Kurilshikov, A., Korem, T., Zeevi, D., et al. (2018). Environment dominates over host genetics in shaping human gut microbiota. Nature 555, 210–215. doi: 10.1038/nature25973
Ruiz-Rodríguez, M., Soler, J. J., Martín-Vivaldi, M., Martín-Platero, A. M., Méndez, M., Peralta-Sánchez, J. M., et al. (2014). Environmental factors shape the community of symbionts in the hoopoe uropygial gland more than genetic factors. Appl. Environ. Microbiol. 80, 6714–6723. doi: 10.1128/AEM.02242-14
Soini, H. A., Bruce, K. E., Wiesler, D., David, F., Sandra, P., and Novotny, M. V. (2005). Stir bar sorptive extraction: a new quantitative and comprehensive sampling technique for determination of chemical signal profiles from biological media. J. Chem. Ecol. 31, 377–392. doi: 10.1007/s10886-005-1347-8
Soini, H. A., Whittaker, D. J., Wiesler, D., Ketterson, E. D., and Novotny, M. V. (2013). Chemosignaling diversity in songbirds: chromatographic profiling of preen oil volatiles in different species. J. Chromatogr. A 1317, 186–192. doi: 10.1016/j.chroma.2013.08.006
Song, S. J., Lauber, C., Costello, E. K., Lozupone, C. A., Humphrey, G., Berg-Lyons, D., et al. (2013). Cohabiting family members share microbiota with one another and with their dogs. eLife 2:e00458. doi: 10.7554/eLife.00458
Spor, A., Koren, O., and Ley, R. (2011). Unravelling the effects of the environment and host genotype on the gut microbiome. Nat. Rev. Microbiol. 9, 279–290. doi: 10.1038/nrmicro2540
Spotswood, E. N., Goodman, K. R., Carlisle, J., Cormier, R. L., Humple, D. L., Rousseau, J., et al. (2012). How safe is mist netting? Evaluating the risk of injury and mortality to birds. Methods Ecol. Evol. 3, 29–38. doi: 10.1111/j.2041-210X.2011.00123.x
Theis, K. R., Heckla, A. L., Verge, J. R., and Holekamp, K. E. (2008). “The ontogeny of pasting behavior in free-living spotted hyenas, Crocuta crocuta” in Chemical Signals in Vertebrates 11. eds. J. L. Hurst, R. J. Beynon, S. C. Roberts, and T. D. Wyatt (New York, NY: Springer), 179–187.
Theis, K. R., Schmidt, T. M., and Holekamp, K. E. (2012). Evidence for a bacterial mechanism for group-specific social odors among hyenas. Sci. Rep. 2:615. doi: 10.1038/srep00615
Theis, K. R., Venkataraman, A., Dycus, J. A., Koonter, K. D., Schmitt-Matzen, E. N., Wagner, A. P., et al. (2013). Symbiotic bacteria appear to mediate hyena social odors. Proc. Natl. Acad. Sci. U. S. A. 110, 19832–19837. doi: 10.1073/pnas.1306477110
Theis, K. R., Whittaker, D. J., and Rojas, C. A. (2020). “A hologenomic approach to animal behavior” in Evolution in Action: Past, Present and Future: A Festschrift in Honor of Erik D. Goodman Genetic and Evolutionary Computation. eds. W. Banzhaf, B. H. C. Cheng, K. Deb, K. E. Holekamp, R. E. Lenski, and C. Ofria, et al. (Cham: Springer International Publishing), 247–263.
Troyer, K. (1984). Microbes, herbivory and the evolution of social behavior. J. Theor. Biol. 106, 157–169. doi: 10.1016/0022-5193(84)90016-X
Tung, J., Barreiro, L. B., Burns, M. B., Grenier, J.-C., Lynch, J., Grieneisen, L. E., et al. (2015). Social networks predict gut microbiome composition in wild baboons. eLife 4:e05224. doi: 10.7554/eLife.05224
Ushida, K., Segawa, T., Tsuchida, S., and Murata, K. (2016). Cecal bacterial communities in wild Japanese rock ptarmigans and captive Svalbard rock ptarmigans. J. Vet. Med. Sci. 78, 251–257. doi: 10.1292/jvms.15-0313
Whittaker, D. J., Gerlach, N. M., Slowinski, S. P., Corcoran, K. P., Winters, A. D., Soini, H. A., et al. (2016). Social environment has a primary influence on the microbial and odor profiles of a chemically signaling songbird. Front. Ecol. Evol. 4:90. doi: 10.3389/fevo.2016.00090
Whittaker, D. J., Gerlach, N. M., Soini, H. A., Novotny, M. V., and Ketterson, E. D. (2013). Bird odour predicts reproductive success. Anim. Behav. 86, 697–703. doi: 10.1016/j.anbehav.2013.07.025
Whittaker, D. J., and Hagelin, J. C. (2021). Female-based patterns and social function in avian chemical communication. J. Chem. Ecol. 47, 43–62. doi: 10.1007/s10886-020-01230-1
Whittaker, D. J., Rosvall, K. A., Slowinski, S. P., Soini, H. A., Novotny, M. V., and Ketterson, E. D. (2018). Songbird chemical signals reflect uropygial gland androgen sensitivity and predict aggression: implications for the role of the periphery in chemosignaling. J. Comp. Physiol. A 204, 5–15. doi: 10.1007/s00359-017-1221-5
Whittaker, D. J., Slowinski, S. P., Greenberg, J. M., Alian, O., Winters, A. D., Ahmad, M. M., et al. (2019). Experimental evidence that symbiotic bacteria produce chemical cues in a songbird. J. Exp. Biol. 222:jeb202978. doi: 10.1242/jeb.202978
Whittaker, D. J., Soini, H. A., Atwell, J. W., Hollars, C., Novotny, M. V., and Ketterson, E. D. (2010). Songbird chemosignals: volatile compounds in preen gland secretions vary among individuals, sexes, and populations. Behav. Ecol. 21, 608–614. doi: 10.1093/beheco/arq033
Whittaker, D. J., Soini, H. A., Gerlach, N. M., Posto, A. L., Novotny, M. V., and Ketterson, E. D. (2011). Role of testosterone in stimulating seasonal changes in a potential avian chemosignal. J. Chem. Ecol. 37, 1349–1357. doi: 10.1007/s10886-011-0050-1
Wienemann, T., Schmitt-Wagner, D., Meuser, K., Segelbacher, G., Schink, B., Brune, A., et al. (2011). The bacterial microbiota in the ceca of Capercaillie (Tetrao urogallus) differs between wild and captive birds. Syst. Appl. Microbiol. 34, 542–551. doi: 10.1016/j.syapm.2011.06.003
Xenoulis, P. G., Gray, P. L., Brightsmith, D., Palculict, B., Hoppes, S., Steiner, J. M., et al. (2010). Molecular characterization of the cloacal microbiota of wild and captive parrots. Vet. Microbiol. 146, 320–325. doi: 10.1016/j.vetmic.2010.05.024
Yarlagadda, K., Razik, I., Malhi, R. S., and Carter, G. G. (2021). Social convergence of gut microbiomes in vampire bats. Biol. Lett. 17:20210389. doi: 10.1098/rsbl.2021.0389
Keywords: Uropygial gland, microbiome, preen oil volatiles, dark-eyed junco, social experiment
Citation: Whittaker DJ, Atyam A, Burroughs NA, Greenberg JM, Hagey TJ, Novotny MV, Soini HA, Theis KR, Van Laar TA and Slade JWG (2023) Effects of short-term experimental manipulation of captive social environment on uropygial gland microbiome and preen oil volatile composition. Front. Ecol. Evol. 10:1027399. doi: 10.3389/fevo.2022.1027399
Edited by:
Magdalena Ruiz Rodriguez, University of Granada, SpainReviewed by:
David Martín-Gálvez, Complutense University of Madrid, SpainPaul Rose, University of Exeter, United Kingdom
Copyright © 2023 Whittaker, Atyam, Burroughs, Greenberg, Hagey, Novotny, Soini, Theis, Van Laar and Slade. This is an open-access article distributed under the terms of the Creative Commons Attribution License (CC BY). The use, distribution or reproduction in other forums is permitted, provided the original author(s) and the copyright owner(s) are credited and that the original publication in this journal is cited, in accordance with accepted academic practice. No use, distribution or reproduction is permitted which does not comply with these terms.
*Correspondence: Joel W. G. Slade, am9lbHNsYWRlQGNzdWZyZXNuby5lZHU=