- 1Botany, Institute of Biology, University of Kassel, Kassel, Germany
- 2Systematic Botany, Institute of Botany, Justus-Liebig-University Giessen, Giessen, Germany
- 3Bioinformatics and Systems Biology, Justus-Liebig-University Giessen, Giessen, Germany
- 4Evolutionary Developmental Biology of Plants, Institute of Botany, Justus-Liebig-University Giessen, Giessen, Germany
- 5Physical Institute, Justus-Liebig-University Giessen, Giessen, Germany
The important worldwide forage crop red clover (Trifolium pratense L.) is widely cultivated as cattle feed and for soil improvement. Wild populations and landraces have great natural diversity that could be used to improve cultivated red clover. However, to date, there is still insufficient knowledge about the natural genetic and phenotypic diversity of the species. Here, we developed a low-cost complexity reduced mRNA analysis (mRNA-GBS) and compared the results with population genetic (GBS) and previously published mRNA-Seq data, to assess whether analysis of intraspecific variation within and between populations and transcriptome responses is possible simultaneously. The mRNA-GBS approach was successful. SNP analyses from the mRNA-GBS approach revealed comparable patterns to the GBS results, but due to site-specific multifactorial influences of environmental responses as well as conceptual and methodological limitations of mRNA-GBS, it was not possible to link transcriptome analyses with reduced complexity and sequencing depth to previously published greenhouse and field expression studies. Nevertheless, the use of short sequences upstream of the poly(A) tail of mRNA to reduce complexity are promising approaches that combine population genetics and expression profiling to analyze many individuals with trait differences simultaneously and cost-effectively, even in non-model species. Nevertheless, our study design across different regions in Germany was also challenging. The use of reduced complexity differential expression analyses most likely overlays site-specific patterns due to highly complex plant responses under natural conditions.
Introduction
Red clover (Trifolium pratense) is an economically relevant crop in temperate agriculture, and also a major component of sustainable farming. Red clover has a high protein content and serves as livestock fodder, promotes soil fertility and is an important component of crop rotation systems. Red clover is well known for its high biomass production (with up to 2–4 tons dry matter/A) and good re-growth capability after mowing (Kleen et al., 2011; Dewhurst, 2013; Eriksen et al., 2014; Herbert et al., 2018). The species belongs to the Fabaceae (legumes) which encompasses several other agronomical important crops, like Glycine max (soy), Medicago truncatula (barrel clover), Phaseolus vulgaris (common bean), Vigna unguiculata (cowpea). Natural populations of T. pratense are diploid with a rather small genome, which is important for high throughput molecular and functional analyses.
Agriculture is faced with the challenge of continuously optimizing crops in order to adapt them to changing climatic and cultivation conditions and to meet the steadily increasing demand for domestic feed or more sustainable production. In red clover, there is still high potential for breeding optimization, as in wild populations as well as in germplasm collections there is a highly significant morphological and genetic variation (e.g., Smith et al., 1985; Kölliker et al., 2003; Dias et al., 2008; Zanotto et al., 2021). The natural variability of red clover, which is native to northwest Africa, throughout Europe, and much of Asia and has been introduced to North America, South America, Australia, and New Zealand, can be used in breeding programs to identify promising populations for improving agronomically important traits (e.g., plant size, growth habit, leaf area Herbert et al., 2018; Gross et al., 2021; Osterman et al., 2021), inflorescence size, number of inflorescences, flowering, disease susceptibility, and others (Isobe et al., 2009; Eriksen et al., 2014; Yates et al., 2014; Vega et al., 2015). This might especially be relevant in times of fast climatic and anthropogenic changes.
Today, rapidly evolving new next generation sequencing (NGS) techniques, tools and analytical methods of genome and transcriptome sequencing, their statistical analysis and related informatics offer new opportunities to support agricultural breeding programs with genomic information. This allows for fostering knowledge in complex biological systems at various organizational levels (from individuals to populations, e.g., Wisecaver et al., 2017; Li et al., 2020), in different dimensions of time and space (Joly and Faure, 2015; Gould et al., 2018; Mead et al., 2019; Marx et al., 2020) and under different treatments, greenhouse conditions, or in the field (Herbert et al., 2021). The development of the RNA-Seq method for quantitative next-generation sequencing of expressed genes has made expression studies for non-model species feasible. However, the method remains expensive and often requires a high number of replicates, so scalability is often not straightforward (Lohman et al., 2016). Genomic DNA fingerprinting [e.g., ddRadSeq (Hohenlohe et al., 2011) or genotyping by sequencing (GBS; Elshire et al., 2011)] is now widely used to perform association studies in many species, including those with complex genomes (Caballero et al., 2021), for revealing genetic diversity and population structure (Müller et al., 2020), for fingerprinting germplasm resources (Wang et al., 2021), or for the detection of candidate genes by fine mapping, especially for improving plant breeding strategies (e.g., Purugganan and Jackson, 2021).
Here, we tested whether we can bridge the gap between genomic DNA fingerprinting and reduced complexity functional genomics in such a way that the natural diversity of a species can be studied quickly and inexpensively, so that the data can be linked relatively easily to functional analyses suitable for improving breeding programs. To our knowledge, the only published study that has tackled a similar approach for non-model plant populations used a TagSeq approach (Marx et al., 2020) on two Poaceae, one Amaranthaceae and one Fabaceae species (Bouteloua aristidoides, Eragrostis lehmanniana, Tidestromia lanuginose, Parkinsonia florida) by investigating populations in geographic proximity and at short time intervals. Marx et al. (2020) recovered quantifiable loci, decisive for spatial differentiation in expression for all species. Another approach by Pallares et al. (2020), called TM3’seq results in a reduced-complexity 3′-enriched library RNA-seq preparation protocol designed for high-throughput processing of individual samples. However, to date it has only been tested on a number of human and Drosophila melanogaster RNA samples, but not on plants.
Here, we developed a complexity reduced mRNA analysis (mRNA-GBS) approach. We tested our mRNA-GBS approach on natural populations (wild type) of red clover in three regions of the Biodiversity Exploratory sites in Germany, and evaluated how it correlates with genomic diversity of populations (analyzed with GBS) over a geographic range and to an earlier published gene expression profiling approach (mRNA-Seq; Herbert et al., 2021). Herbert et al. (2021) examined the expression patterns of red clover in relation to species-specific responses to mowing at one of the Biodiversity Exploratories and in the greenhouse. They identified candidate genes whose annotation suggests potential importance for phenotype changes in response to mowing. However, these analyses are currently only possible for a limited number of sites and individuals due to high costs and immense amounts of data (Gould et al., 2018; Marx et al., 2020). By combining fingerprinting with transcriptome profiling techniques across many samples, treatments, and locations, we test here whether it is possible to detect multiple genetic variants found across taxa and genomes in wild populations of red clover. Furthermore, we test whether this approach is able to simultaneously identify genomic population differences and candidate gene-signals potentially indicative for adaptive genetic variation. Our goal was to assess whether mRNA-GBS provides results that are equitable and relatable to GBS and RNA-Seq, are biologically informative, and are more cost-effective due to the shallow sequencing depth.
Materials and methods
Study site and sampling
Sampling of plant material for mRNA-GBS and GBS was performed on the premises of the long-term open research platform Biodiversity Exploratory in June 2017 on the three Biodiversity Exploratories “Schorfheide-Chorin (S)” in the State of Brandenburg, “Hainich-Dün (H)” in Thuringia, and “Swabian Alb (A)” in the State of Baden-Württemberg, Germany (Fischer et al., 2010). The large-scale research landscapes are used for biodiversity and ecosystem research under real conditions with a long-term perspective. We sampled six agricultural sites per Biodiversity Exploratory, 18 populations in total (Table 1 and Figure 1). The sites each have different management intensities, landscape structures, and climatic conditions. One population (AEG9) deviated so much from the other populations in its values and patterns that it was excluded from further analyses in the mRNA-GBS as well as in the GBS analysis. The experimental plots were managed as normal agricultural land colonized with native, established red clover populations. The not-mown pastures and meadows were neither grazed nor mown in the year of sampling (Herbert et al., 2021). Red clover was abundant in all fields in the region, so it was impossible to estimate population size. The fields are grasslands which are mown once or twice a year or used for grazing. Collection permits from farmers and local authorities were obtained centrally by the Biodiversity Exploratory research platform. At least seven individuals per site (126 in total) were quick-frozen in liquid nitrogen in the field and stored at −80°C until further processing.
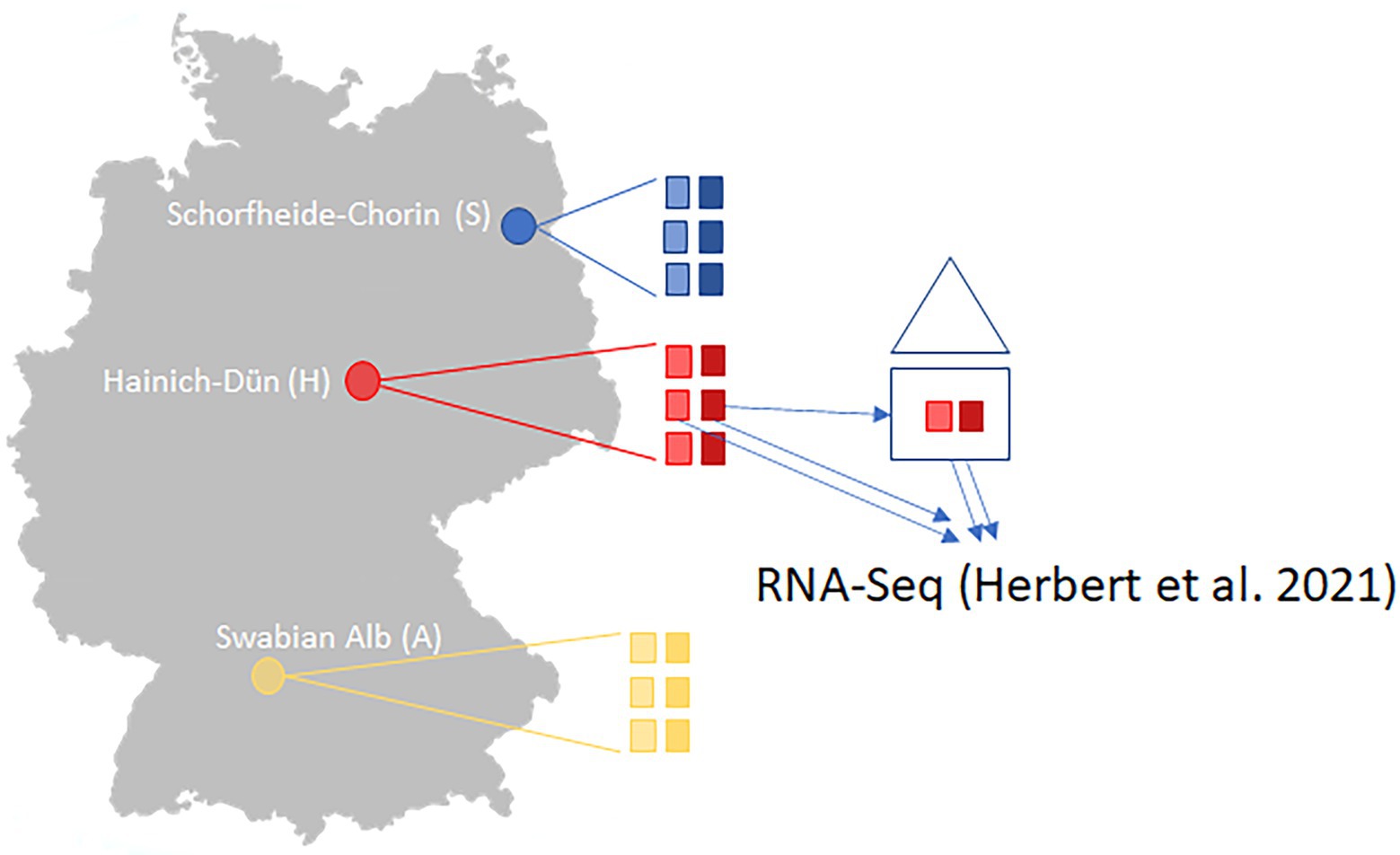
Figure 1. Study sites in Germany of the three Biodiversity Exploratory sites (S, Schorfheide-Chorin; H, Hainich-Dün; A, Swabian Alb) with 6 sampled populations per site (three mown (transparent colors) and three unmown (rich colors)) for the mRNA-GBS analysis and the GBS analysis and results were compared to the RNA-Seq-study of Herbert et al. (2021) where samples derived from the Hainich-Dün site directly and were cultivated in a greenhouse experiment.
Molecular techniques
Briefly, our mRNA-GBS library construction method involves 8 laboratory steps: (i) isolate total RNA, (ii) remove genomic DNA with DNase, (iii) convert mRNA into cDNA by using a reverse transcription kit (cDNA) using a BceA restriction sites containing PolyA primer with an anchor, (iv) digestion with BceA and MseI restriction enzymes, (v) NGS primer ligation with BceA adapter and index and MseI adapter, (vi) pooling, purification and PCR amplification, (vii) size selection, (viii) Illumina Next Seq 500 Vs sequencing (Figure 2).
For the mRNA-GBS analysis seven individuals per site were examined. For RNA extraction we used the NucleoSpin® RNA Plant kit (Macherey-Nagel, Germany) according to the manufacturer’s instructions. For the mRNA-GBS development the Maxima H Minus Double-Stranded cDNA Synthesis Kit (Thermo Fisher Scientific™, Germany) was used for double stranded cDNA-Synthesis, however, with a specially designed PolyT priming site, suitable to be cleaved by the BceAI restriction enzyme (gcBceAI-PolyA-TVN-Primer).
5’-CCGGCGCGACGGCTTTTTTTTTTTTTTTTVN-3′ following the user manual. Purification took place with the NucleoSpin® Gel and PCR Clean-up kit (Macherey-Nagel, Germany). Restriction was carried out, by digesting 200 ng double stranded cDNA with BceAI (2 U/μL) and MseI (10 U/μL) by 37°C in NEB 3.1 buffer (16 μL cDNA/H2O (200 ng cDNA), 2 μL buffer, 1 μL BceAI, 0.25 μL MseI and 0.75 μL H2O; 60 min incubation at 37°C and 20 min inactivation at 65°C). After preparing the samples 30 ng/μL of the digested material were transferred to LGC Genomics GmbH (Germany) for library preparation, pooling and sequencing (150 bp paired-end reads on an Illumina Next Seq 500 V2, Figure 2; Supplementary Figure S1).
For GBS analysis, DNA was extracted from five samples per site (Table 1). We used the Invisorb® Spin Plant Mini Kit from Stratec Molecular (Germany) according to the instructions for use. DNA quantity and quality were analysed using a NanoPhotometer™ (Implen GmBH, München, Germany). We sent 300 ng of DNA in 20 μL to LGC Genomics GmbH (Germany) where genomic DNA were digested with 1 Unit MslI (NEB) in 1 times NEB4 buffer in 30 μL volume for 1 h at 37°C. The restriction enzyme was heat inactivated by incubation at 80°C for 20 min. The indexed Illumina libraries were prepared by using the Encore Rapid Multiplex System (Nugen): 15 μL were transferred to a new 96 well PCR plate, mixed on ice first with 3 μL of one of the 192 L2 Ligation Adaptors and then with a 12 μL Mastermix (a combination of 4.6 μL D1 water/ 6 μL L1 Ligation Buffer Mix/ 1.5 μL L3 Ligation Enzyme Mix). Ligation reactions were incubated at 25°C for 15 min and heat inactivated at 65°C for 10 min. A 20 μL Final Repair Master Mix was added to each tube and the reaction was incubated at 72°C for 3 min. For purification, the reactions were diluted with 50 μL TE 10/50 (10 mM Tris/HCl, 50 mM EDTA, pH, 8.0) and mixed with 80 μL Agencourt XP beads, incubated for 10 min at RT and placed for 5 min on a magnet to collect the beads. The supernatant was discarded and the beads were washed two times with 200 μL 80% Ethanol. Beads were air dried for 10 min and libraries were eluted in 20 μL Tris Buffer (5 mM Tris/HCl pH 9) prior to sequencing on an Illumina NextSeq 500 V2, resulting in 150 bp paired-end reads.
Bioinformatics and genotyping
mRNA-GBS data SNP calling
The Illumina reads were mapped to the repeat-masked T. pratense reference genome (version GCA_900079335.1, ENSEMBL release 50) using the STAR short read mapper (Dobin and Gingeras, 2015). Duplicate reads were filtered using the Picard Toolkit (Broad Institute, 2019) and the MarkDuplicates algorithm (version 2.26.1). The samples of the same field site were pooled to get a higher resolution. Alleles were counted using bam-readcount (Khanna et al., 2022) with a minimum base quality of 20. Only loci with at least 10 reads in each pool were considered and alleles were called only when supported by at least three reads. Error rates with transcripts per million normalized read-counts (TPM) were calculated using the following pipeline.1
Genotyping by sequencing data analysis
After base calling and demultiplexing the quality of the sequenced reads were quality checked. SNP calling and genotyping was conducted with Freebayes (Garrison and Marth, 2012). We used adapter clipped data for further calculations in Stacks 1.48 (Catchen et al., 2011, 2013). UStacks and denovo_map were applied for analyses without a reference genome. The following (default) parameters for the formation of stacks and loci were used: minimum depth of coverage to create a stack –m = 3, maximum of distance allowed between stacks –M = 2, distance allowed between catalog loci –n = 0 (maximum distance allowed to align secondary reads –N = 4, maximum number of stacks allowed per de novo locus: 3) and –t to remove or break up highly repetitive RAD-Tags in UStacks. Next we ran CStacks (to build the catalog) and SStacks (match the samples against the catalog) pipelines without modifications. We applied the correction module rxstacks, filtering by locus log likelihood with the following options: -t 40 --conf_lim 0.25 --prune_haplo --model_type bounded --bound_high 0.1 --lnl_lim −8.0 --lnl_dist –verbose. Finally, we ran the population program in Stacks with following parameters for: -r 75 -p 75 -m 10 -t 20 --min_maf 0.04 --max_obs_het 0.5. PGDSPIDER v.2.1.0.0 (Lischer and Excoffier, 2012) was used to convert Stacks output files for further analyses.
Genetic diversity was estimated as percentage of polymorphic loci (PL) and as Nei’s gene diversity [He; Nei (1973)] using ARLEQUIN v.3.5.1. (Excoffier and Lischer, 2010) and the package „diveRsity “(Keenan et al., 2013) by using R 3.5.1 (R Core Team, 2013). To visualize the data STRUCTURE (Pritchard et al., 2000) was used, which shows the membership probabilities. For automation and parallelization of STRUCTURE (Pritchard et al., 2000) analysis we used the program StrAuto (Chhatre and Emerson, 2017). Genetic clusters were detected by applying the admixture model, with 1,000 Markov Chain Monte Carlo (MCMC) replicates, with a burn-in period of 1,000 and 10 repeats per run for each chosen cluster number (i.e., K = 1–20), Ploidy = 2. For all other settings, default options were used. To identify the most likely K modal distribution, delta K (Evanno et al., 2005) was determined by using STRUCTURE HARVESTER (Earl and von Holdt, 2012) wich is also integrated in StrAuto (Chhatre and Emerson, 2017). To verify the most probable cluster membership coefficient among the 10 runs of STRUCTURE and STRUCTURE HARVESTER we used CLUMPP v.1.1.2 (Jakobsson and Rosenberg, 2007). Corresponding graphs were constructed with DISTRUCT (Rosenberg, 2004). By using R 3.5.1 (R Core Team, 2013) and the R package “adegenet” v.1.4–2 (Jombart, 2008) a Principal Component Analysis (PCA) was performed to detect linearity or hidden patterns in the data set. With the R package ‘adegenet’ v.1.4–2 (Jombart, 2008) and ‘ape’ (Paradis et al., 2004) the dendrograms were created, euclidian distance was used. Genetic variation among groups of populations (FCT), among populations within groups (FSC) and within populations (FST) were partitioned with hierarchical analyses of molecular variance (AMOVA) by using ARLEQUIN v.3.5.1.2 (Excoffier and Lischer, 2010) with an allowed missing data level at 5%. Additionally, pairwise FST values were estimated among populations, with significance levels of 0.05 and 100 permutations.
Results
Sampling and genotyping
The mRNA-GBS sequencing yielded a total of 183,747,290 reads for the 126 investigated samples, with 42 individuals per region (S, H, A; Table 2). Retrieved read numbers varied strongly between individuals with an average of 1.1 million raw reads per sample (range: 7,106,704 – 31,481). In total 91,870,548 adapter clipped read pairs were retrieved (Supplementary Figure S3; Supplementary Figure S4). To analyze error rates, we calculated TPM-normalized read counts for each sample (Figure 3) by testing our mRNA-GBS library against the RNA-Seq library of Herbert et al. (2021). Since TPM normalizes to sequencing depth, the value should be stable with respect to the actual read count if the sequencing depth was appropriate. When we reduced our samples from 90 to 60% sequencing depth (Figure 3), the changes in error rate indicated that our sequencing depth was insufficient to perform gene expression studies and to be matched against the T. pratense transcriptome (Herbert et al., 2021) for subsequent analysis, whereas the error rate in Herbert et al. (2021) was stable and in line with expectations.
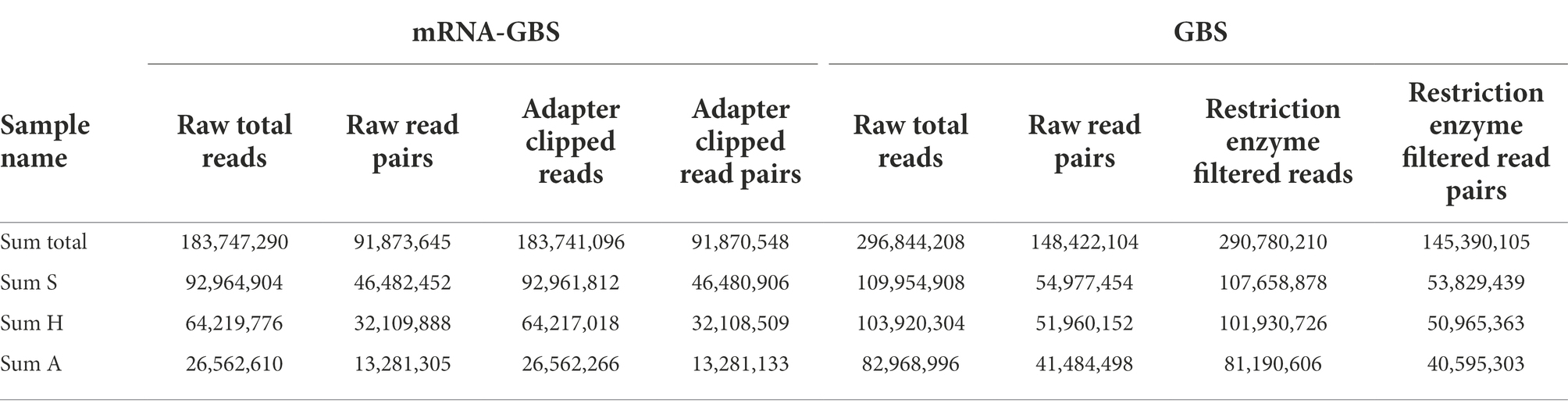
Table 2. Number of raw reads retained in mRNA-GBS and GBS analysis after each filtering step for Trifolium pratense samples from the three biodiversity exploratory sites in Germany (S, Schorfheide-Chorin; H, Hainich-Dün; A, Swabian Alb).
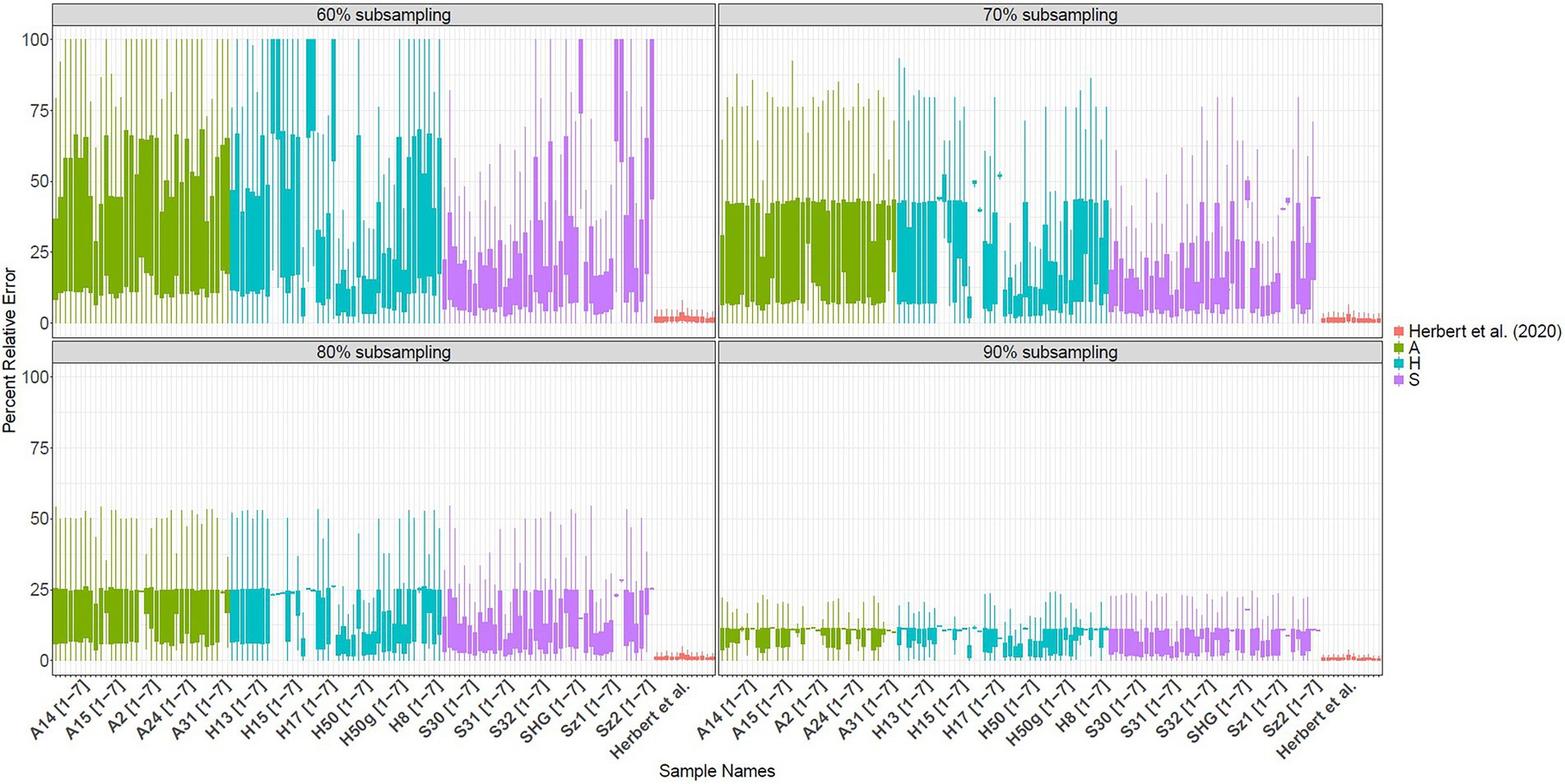
Figure 3. Error rates for the TPM normalized read counts for the samples of the mRNA-GBS analysis, depicted in light green (S), bluish green (H) and purple (A) and the RNA-Seq data of Herbert et al. (2021) in red, calculated with 90% coverage (upper left), 80% coverage (upper right), 70% (lower left), 60% (lower right) an revealing strong differences in the error rate detection in the mRNA-GBS samples, when coverage is reduced, with little differences in the RNA-Seq data, which is stable and thus usable for gene expression analysis.
To identify SNPs for population genetic studies, the sequencing depth for SNP analysis of individual samples was also too shallow. Therefore, individuals within sites of similar treatments (mown/not mown) were combined in bulk samples to obtain a site-specific pattern. In this way, a total of 15,111 SNPs were obtained for subsequent analysis.
The GBS sequencing yielded a total of 296,844,208 raw reads (range: 2,212,232 – 777,242) for the 90 investigated samples from each of the three regions (Tables 1, 2; Figure 1), on average 3.6 million reads per sample, resulting in a total of 1,719 SNPs for subsequent analyses.
Genetic diversities and differentiation
The mRNA-GBS analysis revealed a comparatively high mean genetic diversity of the investigated red clover bulk samples of ØHe = 0.76, ranging from He = 0.72 (S) to He = 0.82 (A, Table 3), if the regions are to be considered. The genetic diversity is higher, if sites with treatments (mown/not mown) are to be considered ØHe = 0.82, ranging from He = 0.79 (S mown) to He = 0.86 (A not mown, Table 3). Because the analysis included multiple combined individuals from three populations per site and only two sites per region, the population comparison was too low to calculate genetic diversity among patches. The GBS analysis revealed a significantly lower mean genetic diversity of the investigated red clover populations of ØHe = 0.17, ranging from He = 0.16 (AEG15, AEG31) to He = 0.19 (HEG50, HEG13, Table 3). The region specific mean genetic diversity is lowest in A (ØHe = 0.17) and S (ØHe = 0.17), highest in H (ØHe = 0.18). According to the Kruskal-Wallis-Test, genetic diversity among the three regions did not differ significantly (chi-squared = 45,076, df = 2, p = 0.105). The ANOVA with polymorphic loci only revealed no differences between A, H and S (F = 3,437, p = 0.0611). The AMOVA revealed moderate genetic differentiation among regions (FCT = 0.08) and within populations (FST = 0.09) which are highly significant. However, for among populations within regions the genetic differentiation is negligible (FSC = 0.005). Thus, differentiation within populations were greater than among regions. Pairwise population FST estimates for the entire study area indicates low to great genetic differentiation among populations (0.00 – 0.18, Supplementary Figure S4). Pairwise population differentiation within regions is low to negligible for all regions (Ø A FST = −0.00, Ø S FST = 0.007, Ø H FST = 0.006).
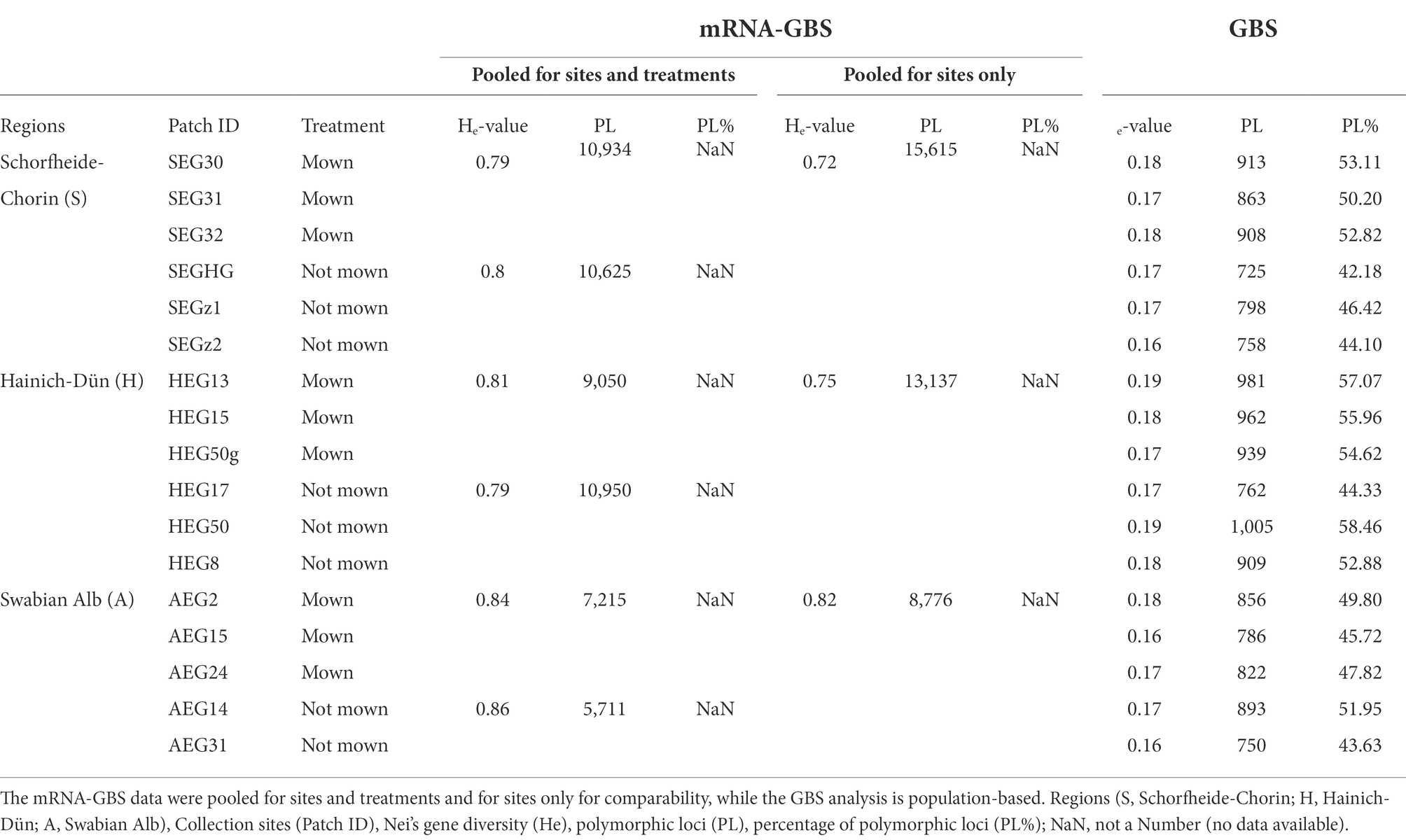
Table 3. Population genetic statistics of Trifolium pratense with mRNA-GBS and GBS data across the three biodiversity exploratories in Germany (S, H, A).
STRUCTURE analyses based on the BIC and Bayesian clustering approaches revealed two genetic clusters, the proportional cluster membership of each being almost region-specific in the GBS analysis (Figure 4A). The mRNA-GBS approach resulted in similar trends that were less prominent (Figure 4B). This is also confirmed by the PCA (Figure 5), which shows the respective site specificity of the centroids of all individuals (GBS) or bulk samples (mRNA-GBS) belonging to one sampling region, however, with much greater genetic similarity between individuals from S and H and the greater distance from A in the GBS analysis and more overlap in the mRNA-GBS data. This overlap is partly due to mowing treatment: the mown populations in the mRNA-GBS analysis showed a stronger pattern of site specificity, while the mRNA-GBS pattern of the unmown individuals was highly divergent. The GBS Neighbor Joining tree (Figure 6A) reflects the patterns of the AMOVA, PCA, and STRUCTURE analyses, with individuals from A distinctly different from those from H and S, with some minor overlap between H and S among the individuals considered. The mRNA-GBS tree (Figure 6B) also reflects the separate positions of the populations in A, but shows more mixing between H and S. The not mown populations A (AEG31, AEG14, Figure 6B), and two out of three of the not mown populations in S (SEGHG, SEGz1) are also clustered, but lack a clear pattern as several other not mown populations appear scattered in the tree (SEGz2, HEG17, HEG8, HEG50).
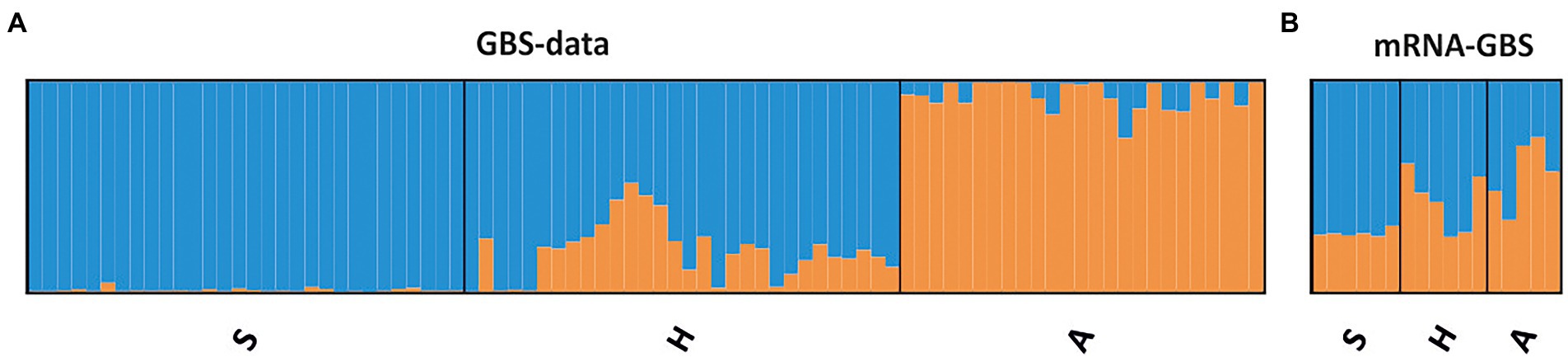
Figure 4. Population genetic structure of the investigated red clover individuals (GBS) or site specific bulk samples (mRNA-GBS) across the different Biodiversity Exploratories (S, Schorfheide-Chorin; H, Hainich-Dün; A, Swabian Alb) as revealed by the STRUCTURE analyses and ΔK (Evanno et al., 2005). (A) The GBS data where each column represents individuals within one region. (B) mRNA-GBS data, where each column represents the bulk samples within one population.
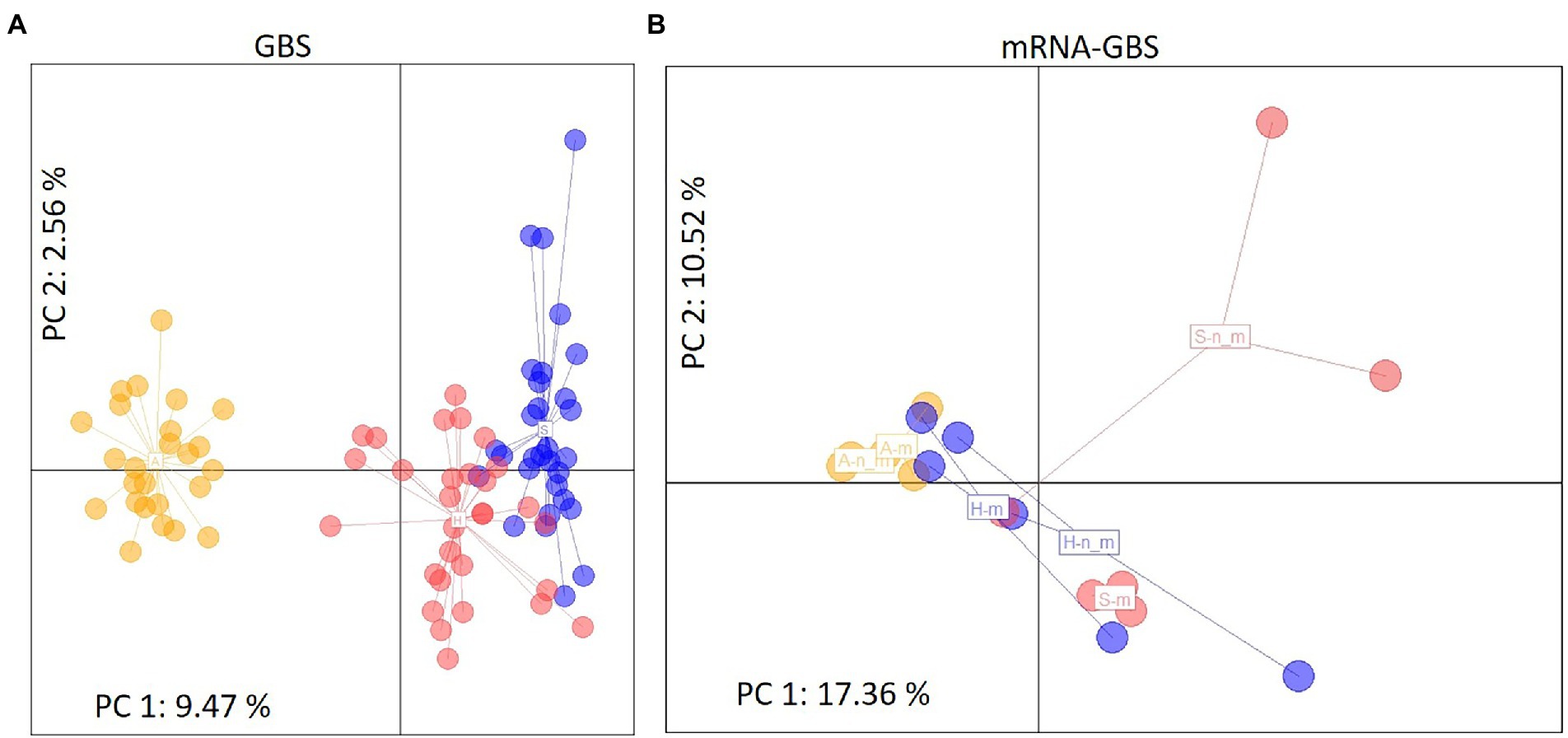
Figure 5. Principal Component Analysis (PCA) of genetic distances between individuals (GBS) or site specific bulk samples (mRNA-GBS) of Trifolium pratense across the different Biodiversity Exploratories (S, Schorfheide-Chorin; H, Hainich-Dün; A, Swabian Alb). Colored label positions represent the centroids of all individuals belonging to one sampling region for (A) The GBS analysis, depicting color coded individuals within each region, where the third axis is representing 2.47% of genetic variation (Σ 14.50%) and (B) the mRNA-GBS analysis, depicting color coded populations of bulk samples within each region (S, H, A where n_m is not mown, m is mown). The third axis is representing 8.72% genetic variation (Σ 36.60%).
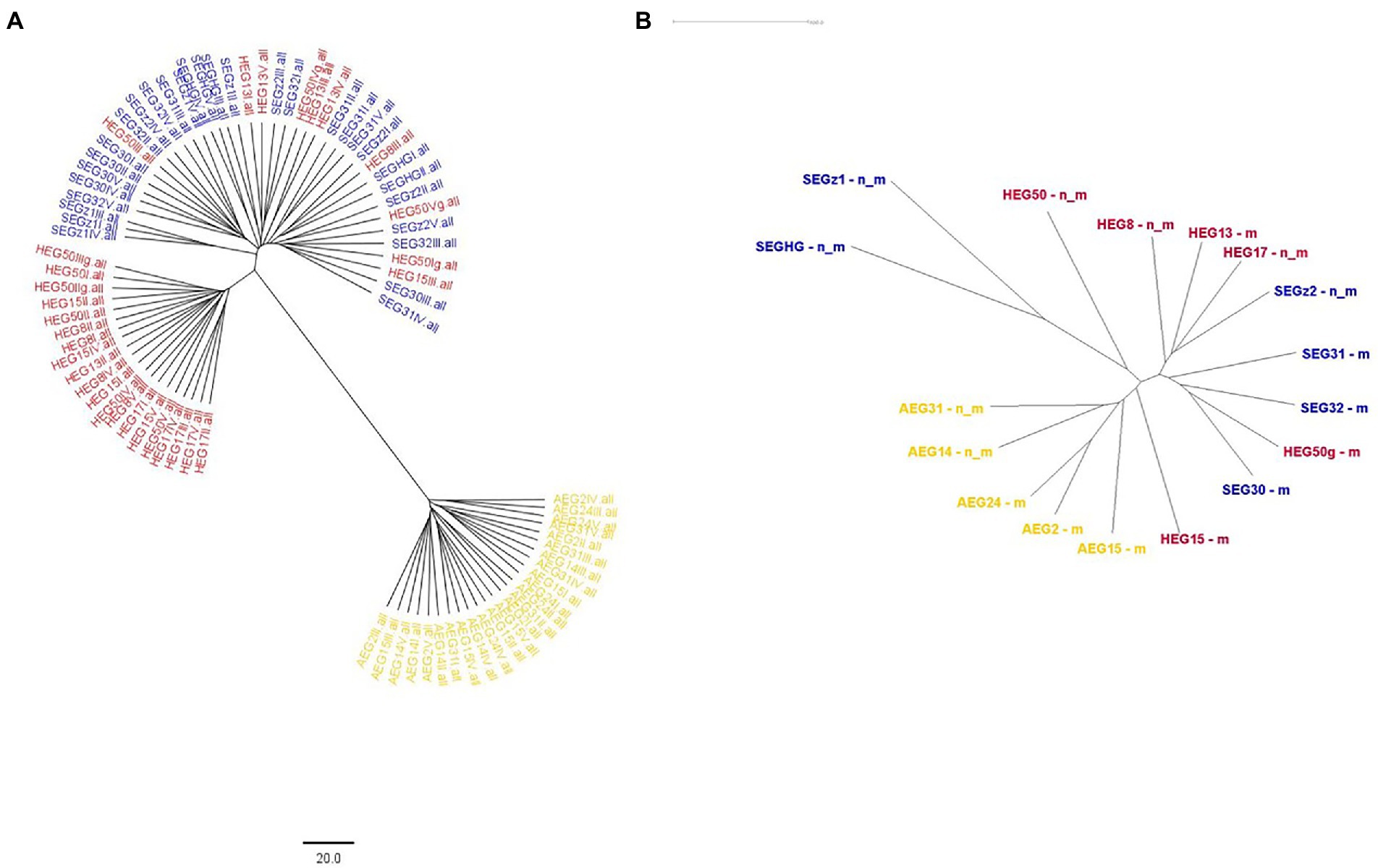
Figure 6. Neighbor Joining tree for the individuals and populations of Trifolium pratense across the different Biodiversity Exploratories (yellow: Schorfheide-Chorin; red: Hainich-Dün; blue: Swabian Alb). (A) The GBS analysis. (B) The mRNA-GBS analysis (n_m, not mown, m, mown).
Discussion
The ability to link population genetic and genome information in the form of transcriptome analyses in a rapid, cost-effective, and technically relatively simple manner would be of great importance for a better understanding of naturally occurring variability and for breeding studies. This approach would allow for the simultaneous screening of diversity while identifying expression of specific candidate genes involved in the response to certain species-specific environmental interactions. Currently this is very time consuming and costly (Bhat et al., 2016). Therefore, we developed and tested a new mRNA-GBS approach to fill the gap by offering a low-cost reduced complexity transcriptome analysis (mRNA-GBS).
Further, this is the first approach to link a mRNA-GBS with an in depth RNA-Seq analysis (Herbert et al., 2021) and a GBS approach on natural occurring plant populations and across a broader geographic scale. We tested the mRNA-GBS approach on several individuals of red clover from 11 populations and three regions in Germany. We hereby evaluate whether the analysis of intraspecific variation within and between populations and transcriptome responses is possible simultaneously. The mRNA-GBS approach revealed population genetic patterns, but linkage with mRNA-Seq data was not possible. The drawbacks and needed optimization steps are discussed in the following.
mRNA-GBS and comparison with RNA-Seq and GBS
Herbert et al. (2021) conducted an in-depth transcriptome analysis on populations of red clover from Hainich-Dün (H) to compare the global transcriptional response to mowing under greenhouse conditions and in agricultural fields. This population was also analyzed in the present study. They simulated mowing and compared the transcriptome response in mown and not mown T. pratense individuals, as in our analysis. Herbert et al. (2021) obtained a total number of short reads ranging from 44.7 to 58.1 million for each library, which on average is a 10-times higher read depth per individual than in our study. Their sequencing approach comprised 608,041,012 raw reads for the analysis of only six different sites/treatments, of eight pooled samples while in the presented mRNA-GBS approach we investigated 13 plants on five to six fields in three regions in Germany. With this approach, they identified 119–142 differentially expressed genes (DEGs, with a log2fold-change >2) that are up- or down-regulated when mown plants were compared with non-mown plants. The mRNA-GBS library was highly variable in terms of read length per individual (80 bp on average), and pooling of samples did not allow us to correlate site-specific multifactorial influences of environmental responses in a statistically robust way. Only 50–86% of the retrieved short sequences are located within the 100 bp region upstream of the poly(A) tail, and only 0.9–3.2% are located within the last 25 bp, which hampered mRNA mapping and prevented the screening for differentially expressed genes. SNP calling and expression studies were thus not possible.
However, also Herbert et al. (2021) discovered that plants grown in the field exhibited more and different stress responses than plants grown in greenhouses, leading them to conclude that field grown plants respond to multiple environmental stresses that are of site specific, abiotic, and biotic in origin. For example, they found some genes upregulated in mown plants being chitinase homologs suggesting that these plants are stressed by insects and/or fungi and that this stress may be more gene expression differences than the loss of biomass due to mowing at the time of sample extraction. Duke (1981) described that more than 65 different fungi and nematodes and more than 20 viruses, insects, and bacteria can infect red clover. Accordingly, our pilot study of mRNA-GBS in such a broad geographic and ecologically diverse range was overly ambitious.
Our sequencing depth with an average of 1.1 million raw reads per sample for mRNA-GBS was too shallow to quantify gene expression differences. Hou et al. (2013) suggested sequencing of 15–50 million reads to cover the majority of transcripts in human tissues with an average of 19,116 protein-coding genes (Piovesan et al., 2019). Thus, for red clover with approximately 47,398 protein-coding genes (Ištvánek et al., 2014), we must aim for at least a 30-fold higher read depth than in this project. However, this does not meet our requirements that the method be inexpensive and easy to perform on multiple individuals.
However, the high error rates, possibly due to PCR bias, insufficient sample number, or most likely the low sequencing depth, though, are due to conceptual and methodological limitations of mRNA-GBS, resulting in artifacts and a relatively high false positive rate of variants such as SNPs and InDels. These not only affect the mRNA-GBS approach but also estimates of population genetic parameters (Davey et al., 2011; Andrews et al., 2016; Cariou et al., 2016; Dorant et al., 2019). This became apparent when we pooled the reads of different individuals from mown and not mown populations from each region, mapped them against a reference genome, and analyzed SNPs and compared them to the GBS analysis. The genetic diversity indices revealed significant inconsistencies between He-GBS (ØHe = 0.17) and He-mRNA-GBS (ØHe = 0.76) values. Our inconsistencies are based on the fact that different evolutionary mechanisms exert both neutral processes such as drift and immigration and adaptive processes such as selection, so that the different evolutionary origins of SNPs, being of coding (mRNA-GBS and GBS) and also non-coding origin (only GBS), limit significance and and the noncoding SNPs dominate in the GBS analysis (Vellend and Geber, 2005; Lamy et al., 2013). Moreover, these inconsistencies are most likely also due to the fact that the mRNA-GBS analysis produced an insufficient number of reads that could be unambiguously mapped annotated regions of the genome. Furthermore, Dorant et al. (2019) previously pointed out problems associated with GBS involving mutations at restriction sites that lead to allelic dropouts and PCR biases such that correct genetic diversity is not reflected and significant misinterpretation of commonly used statistics in population genetics studies leads to incorrect conclusions (Arnold et al., 2013; Gautier, 2015; Cariou et al., 2016). Several studies investigated the genetic diversity of red clover populations and germplasm collections, e.g., using RAPD (He = 0.12–0.18; Campos-de Quiroz and Ortega-Klose, 2001; Ulloa et al., 2003), AFLP (Kölliker et al., 2003; Herrmann et al., 2005), and SSR (He = 0.32–0.38) (Gupta et al., 2017), and several of them found relatively high values for genetic diversity estimates similar to or slightly lower than those of our mRNA-GBS analysis. Pfeiffer et al. (2018) compared GBS and AFLP data in an herbaceous perennial sedge species (Carex gayana) and found slightly higher estimates of genetic diversity with SNPs than with AFLP data, but also discovered some populations where this trend was reversed. SNP mutation rates are relatively low (10 × 10−8 to 10 × 10−9; Nachman and Crowell, 2000; Pfeiffer et al., 2018), lower than those of microsatellites (0.001 to 0.005, Pinto et al., 2013; Fischer et al., 2017), whereas AFLP mutation rates can exceed those of microsatellites (Kuchma et al., 2011).
STRUCTURE analyses revealed two genetic clusters for the GBS and pooled mRNA-GBS results, and the patterns were nearly region-specific in both analyses. In the mRNA-GBS, they were even treatment-specific (mown/not mown), which is weakly supported by PCA (Figure 5) but no longer evident in the Neighbor Joining analysis. Deeper sequencing would potentially lead to the detection of transcribed genes with low expression, resulting in stronger site-specific pattern recognition. GBS analysis revealed greater genetic similarity between individuals from S and H and a greater distance from A, while mRNA GBS data showed no differences among the three regions. Little to no differentiation was also found in other population genetic comparisons of plants in the Biodiversity Exploratories, e.g., Veronica chamaedrys (Kloss et al., 2011) in an AFLP study. While Kloss et al. (2011) found very little difference within and between populations, suggesting that the effects of genetic drift are counterbalanced by gene flow between populations, we found some differences. Both red clover and V. chamaedrys are commonly outcrossing perennials for which high gene flow is known to counteract the effects of genetic drift, either through high natural or human-induced dispersal of seeds and pollen or through large effective population sizes (Nybom, 2004; Musche et al., 2008).
mRNA-GBS and other marker assisted approaches
The advantage of mRNA-GBS is that it provides SNPs of transcripts from housekeeping genes as well as of very specific biological processes at a specific time point and under the conditions prevailing there that characterize the phenotype, even if we mainly target the far 3′ end. However, to identify differentially expressed transcripts involved in specific processes like growth and development or response to biotic and abiotic stresses requires a much higher sequencing depth, because transcription factors expressed at extremely low levels regulate these processes. In contrast, highly expressed genes are mostly involved in primary metabolism, such as ATP synthesis, amino acid-, nucleic acid-, and c1 metabolism and they are expressed constitutively (Das and Bansal, 2019).
In contrast, the GBS approach and similar molecular techniques used for NGS-based population genomic analyses (e.g., Hy-Rad, ddRAD-Seq, Pool-Seq, Hy-Rad, restriction site-associated DNA capture (Rapture), bulk and low-coverage NGS, and others, e.g., discussed in Dorant et al. e.g., discussed in Dorant et al., 2019), SNPs from genomic regions and reflect only genotype, whereas phenotype is influenced by both its genotype and environment. RNA-Seq experiments targeting phenotype, as in Herbert et al. (2021) in our study, can currently only be performed for a limited number of individuals and replicates due to the high cost of library preparation and deep sequencing, and assignment to a reference genome is required (Pallares et al., 2020). For marker-assisted breeding and for a better understanding of natural variability in populations, the mRNA-GBS approach developed here aims to identify specific genes by direct or indirect analysis of genomes to replace standard comparative deep transcriptomics (Collard and Mackill, 2008).
Currently one approach is published, investigating gene expression in non-model plant populations with reduced complexities (Marx et al., 2020) and in comparison with RNA-Seq by using a TagSeq approach. Marx et al. (2020) performed RNA-Seq analysis on four non-model species at their natural habitats. They then mapped TagSeq data from individuals at weekly intervals over three weeks and were able to align the short sequences with the reference transcriptome. However, they did not analyze these findings in a population genetic context. The TM3’seq approach (Pallares et al., 2020) also targets 3′ ends of transcripts while preserving sample identity at each step and enables simultaneous high-throughput processing of individual samples, but this approach has not been explored on plant samples, yet.
Conclusion
In summary, we found that mRNA-GBS is a promising tool for population genetic analysis, but greater sequencing depth is required and fewer divergent populations need to be compared. The mRNA-GBS analysis described here resulted in too many divergent short sequence reads impeding assignment to transcripts. We thus recommend to focus more on generating reads from upstream of the poly(a) tail of the mRNA. As expected, we found experimental bias in our analysis due to the use of NGS and GBS tools, corroborating previous work by other groups. Our comparative analysis of T. pratense has contributed to knowledge enhancement at a time when intensive research on genomic fingerprinting analyses and reduced RNA-Seq approaches is underway, particularly for non-model species.
Data availability statement
The datasets presented in this study can be found in online repositories. The names of the repository/repositories and accession number(s) can be found at: https://www.ebi.ac.uk/ena, PRJEB40235, PRJEB40236, https://www.ncbi.nlm.nih.gov/genbank/, SRX6746841 – SRX6746852.
Author contributions
BG planned and designed the mRNA-GBS approach and the research. CM and BG conducted fieldwork. CM conducted the lab work. CM, OR, and MS analyzed the data. BG, CM, AB, and OR wrote the manuscript. All authors contributed to the article and approved the submitted version.
Funding
This work has been funded through the DFG Priority Program 1374 “Biodiversity Exploratories” to BG (GE1242/14–1/14–2) and AB (BE 2547/12–1/12–2). We used the de.NBI infrastructure German Network for Bioinformatics Infrastructure, the de.NBI project was funded by the BMBF (FKZ 031A532–031A540).
Acknowledgments
We thank the managers of the three Exploratories and all former managers, for their work in maintaining the plot and project infrastructure, Christiane Fischer and Jule Mangels for their support through the central office, Andreas Ostrowski and Michael Owonibi for managing the central database, and Markus Fischer, Eduard Linsenmair, Dominik Hessenmöller, Daniel Prati, Ingo Schöning, Francois Buscot, Ernst-Detlef Schulze, Wolfgang Weisser and the late Elisabeth Kalko for their role in setting up the Biodiversity Exploratories project. Fieldwork permits were issued by the responsible state environmental offices of Baden-Württemberg, Thüringen, and Brandenburg (according to § 72 331 BbgNatSchG). We are grateful to Volker Wissemann, Annalena Kurzweil and Thomas Groß for lab and administrative support. We are thankful to Andrea Weisert and Sabine Mutz for carrying out RNA extractions and cDNA synthesis steps.
Conflict of interest
The authors declare that the research was conducted in the absence of any commercial or financial relationships that could be construed as a potential conflict of interest.
Publisher’s note
All claims expressed in this article are solely those of the authors and do not necessarily represent those of their affiliated organizations, or those of the publisher, the editors and the reviewers. Any product that may be evaluated in this article, or claim that may be made by its manufacturer, is not guaranteed or endorsed by the publisher.
Supplementary material
The supplementary material for this article can be found online at: https://www.frontiersin.org/articles/10.3389/fevo.2022.1003057/full#supplementary-material
SUPPLEMENTARY FIGURE S1 | Overview of the mRNA-GBS approach.
SUPPLEMENTARY FIGURE S2 | Distribution of tag lengths of the mRNA-GBS analysis.
SUPPLEMENTARY FIGURE S3 | Summary of mean read counts and mRNA fragment length for the mRNA-GBS analysis (green: counts TR) and the RNA-Seq analysis (red: counts RS) by Herbert et al. (2021).
SUPPLEMENTARY FIGURE S4 | Matrix of Pairwise Fst for each population analysed with GBS. Genetic differentiation from little (0 – 0,05), moderate (0,05 – 0,15) to great (0,15-0,25) (Wright, 1984).
Footnotes
References
Andrews, K. R., Good, J. M., Miller, M. R., Luikart, G., and Hohenlohe, P. A. (2016). Harnessing the power of RADseq for ecological and evolutionary genomics. Nat. Rev. Genet. 17, 81–92. doi: 10.1038/nrg.2015.28
Arnold, B., Corbett-Detig, R. B., Hartl, D., and Bomblies, K. (2013). RADseq underestimates diversity and introduces genealogical biases due to nonrandom haplotype sampling. Mol. Ecol. 22, 3179–3190. doi: 10.1111/mec.12276
Bhat, J. A., Ali, S., Salgotra, R. K., Mir, Z. A., Dutta, S., Jadon, V., et al. (2016). Genomic selection in the era of next generation sequencing for complex traits in plant breeding. Front. Genet. 7:221. doi: 10.3389/fgene.2016.00221
Broad Institute (2019). Picard toolkit. GitHub Repository. Available online at: https://broadinstitute.github.io/picard/
Caballero, A., Villanueva, B., and Druet, T. (2021). On the estimation of inbreeding depression using different measures of inbreeding from molecular markers. Evol. Appl. 14, 416–428. doi: 10.1111/eva.13126
Campos-de Quiroz, H., and Ortega-Klose, F. (2001). Genetic variability among elite red clover (Trifolium pratense L.) parents used in Chile as revealed by RAPD markers. Euphytica 122, 61–67. doi: 10.1023/A:1012617504493
Cariou, M., Duret, L., and Charlat, S. (2016). How and how much does RAD-seq bias genetic diversity estimates? BMC Evol. Biol. 16:240. doi: 10.1186/s12862-016-0791-0
Catchen, J., Hohenlohe, P. A., Bassham, S., Amores, A., and Cresko, W. A. (2013). Stacks: an analysis tool set for population genomics. Mol. Ecol. 22, 3124–3140. doi: 10.1111/mec.12354
Catchen, J. M., Amores, A., Hohenlohe, P., Cresko, W., Postlethwait, J. H., and De, K. D.-J. (2011). Stacks: building and genotyping loci De novo from short-read sequences. G3 1, 171–182. doi: 10.1534/g3.111.000240
Chhatre, V. E., and Emerson, K. J. (2017). StrAuto: automation and parallelization of STRUCTURE analysis. BMC Bioinform. 18:192. doi: 10.1186/s12859-017-1593-0
Collard, B. C. Y., and Mackill, D. J. (2008). Marker-assisted selection: an approach for precision plant breeding in the twenty-first century. Philosophical transactions of the Royal Society of London. Series B Biol. Sci. 363, 557–572. doi: 10.1098/rstb.2007.2170
Das, S., and Bansal, M. (2019). Variation of gene expression in plants is influenced by gene architecture and structural properties of promoters. PLoS One 14:e0212678. doi: 10.1371/journal.pone.0212678
Davey, J. W., Hohenlohe, P. A., Etter, P. D., Boone, J. Q., Catchen, J. M., and Blaxter, M. L. (2011). Genome-wide genetic marker discovery and genotyping using next-generation sequencing. Nat. Rev. Genet. 12, 499–510. doi: 10.1038/nrg3012
Dewhurst, R. (2013). Milk production from silage: comparison of grass, legume and maize silages and their mixtures. AFSci 22, 57–69. doi: 10.23986/afsci.6673
Dias, P. M. B., Julier, B., Sampoux, J.-P., Barre, P., and Dall’Agnol, M. (2008). Genetic diversity in red clover (Trifolium pratense L.) revealed by morphological and microsatellite (SSR) markers. Euphytica 160, 189–205. doi: 10.1007/s10681-007-9534-z
Dobin, A., and Gingeras, T. R. (2015). Mapping RNA-seq reads with STAR. Curr. Protoc. Bioinformatics 51, 11.14.1–11.14.19. doi: 10.1002/0471250953.bi1114s51
Dorant, Y., Benestan, L., Rougemont, Q., Normandeau, E., Boyle, B., Rochette, R., et al. (2019). Comparing Pool-seq, rapture, and GBS genotyping for inferring weak population structure: the American lobster (Homarus americanus) as a case study. Ecol. Evol. 9, 6606–6623. doi: 10.1002/ece3.5240
Earl, D. A., and von Holdt, B. M. (2012). STRUCTURE HARVESTER: a website and program for visualizing STRUCTURE output and implementing the Evanno method. Conserv. Genet. Resour. 4, 359–361. doi: 10.1007/s12686-011-9548-7
Elshire, R. J., Glaubitz, J. C., Sun, Q., Poland, J. A., Kawamoto, K., Buckler, E. S., et al. (2011). A robust, simple genotyping-by-sequencing (GBS) approach for high diversity species. PLoS One 6:e19379. doi: 10.1371/journal.pone.0019379
Eriksen, J., Askegaard, M., and Søegaard, K. (2014). Complementary effects of red clover inclusion in ryegrass-white clover swards for grazing and cutting. Grass Forage Sci. 69, 241–250. doi: 10.1111/gfs.12025
Evanno, G., Regnaut, S., and Goudet, J. (2005). Detecting the number of clusters of individuals using the software STRUCTURE: a simulation study. Mol. Ecol. 14, 2611–2620. doi: 10.1111/j.1365-294X.2005.02553.x
Excoffier, L., and Lischer, H. E. L. (2010). Arlequin suite ver 3.5: a new series of programs to perform population genetics analyses under Linux and windows. Mol. Ecol. Resour. 10, 564–567. doi: 10.1111/j.1755-0998.2010.02847.x
Fischer, M., Bossdorf, O., Gockel, S., Hänsel, F., Hemp, A., Hessenmöller, D., et al. (2010). Implementing large-scale and long-term functional biodiversity research: the biodiversity Exploratories. Basic Appl. Ecol. 11, 473–485. doi: 10.1016/j.baae.2010.07.009
Fischer, M. C., Rellstab, C., Leuzinger, M., Roumet, M., Gugerli, F., Shimizu, K. K., et al. (2017). Estimating genomic diversity and population differentiation - an empirical comparison of microsatellite and SNP variation in Arabidopsis halleri. BMC Genomics 18:69. doi: 10.1186/s12864-016-3459-7
Garrison, E., and Marth, G. (2012). Haplotype-based variant detection from short-read sequencing. doi: 10.48550/arXiv.1207.3907
Gautier, M. (2015). Genome-wide scan for adaptive divergence and association with population-specific covariates. Genetics 201, 1555–1579. doi: 10.1534/genetics.115.181453
Gould, B. A., Chen, Y., and Lowry, D. B. (2018). Gene regulatory divergence between locally adapted ecotypes in their native habitats. Mol. Ecol. 27, 4174–4188. doi: 10.1111/mec.14852
Gross, T., Müller, C. M., Becker, A., Gemeinholzer, B., and Wissemann, V. (2021). Common garden versus common practice – phenotypic changes in Trifolium pratense L. in response to repeated mowing. J. Appl. Bot. Food Qual. 94, 1–6. doi: 10.5073/JABFQ.2021.094.001
Gupta, M., Sharma, V., Singh, S. K., Chahota, R. K., and Sharma, T. R. (2017). Analysis of genetic diversity and structure in a genebank collection of red clover (Trifolium pratense L.) using SSR markers. Plant genet. Resource 15, 376–379. doi: 10.1017/S1479262116000034
Herbert, D. B., Ekschmitt, K., Wissemann, V., and Becker, A. (2018). Cutting reduces variation in biomass production of forage crops and allows low-performers to catch up: a case study of Trifolium pratense L. (red clover). Plant Biol. 20, 465–473. doi: 10.1111/plb.12695
Herbert, D. B., Gross, T., Rupp, O., and Becker, A. (2021). Transcriptome analysis reveals major transcriptional changes during regrowth after mowing of red clover (Trifolium pratense). BMC Plant Biol. 21:95. doi: 10.1186/s12870-021-02867-0
Herrmann, D., Boller, B., Widmer, F., and Kölliker, R. (2005). Optimization of bulked AFLP analysis and its application for exploring diversity of natural and cultivated populations of red clover. Genome 48, 474–486. doi: 10.1139/g05-011
Hohenlohe, P. A., Amish, S. J., Catchen, J. M., Allendorf, F. W., and Luikart, G. (2011). Next-generation RAD sequencing identifies thousands of SNPs for assessing hybridization between rainbow and westslope cutthroat trout. Mol. Ecol. Resour. 11, 117–122. doi: 10.1111/j.1755-0998.2010.02967.x
Hou, R., Yang, Z., Li, M., and Xiao, H. (2013). Impact of the next-generation sequencing data depth on various biological result inferences. Sci. China Life Sci. 56:104–109. doi: 10.1007/s11427-013-4441-0
Isobe, S., Kölliker, R., Hisano, H., Sasamoto, S., Wada, T., Klimenko, I., et al. (2009). Construction of a consensus linkage map for red clover (Trifolium pratense L.). BMC Plant Biol. 9:57. doi: 10.1186/1471-2229-9-57
Ištvánek, J., Jaroš, M., Křenek, A., and Řepková, J. (2014). Genome assembly and annotation for red clover (Trifolium pratense; Fabaceae). Am. J. Bot. 101, 327–337. doi: 10.3732/ajb.1300340
Jakobsson, M., and Rosenberg, N. A. (2007). CLUMPP: a cluster matching and permutation program for dealing with label switching and multimodality in analysis of population structure. Bioinformatics 23, 1801–1806. doi: 10.1093/bioinformatics/btm233
Joly, D., and Faure, D. (2015). Next-generation sequencing propels environmental genomics to the front line of research. Heredity 114, 429–430. doi: 10.1038/hdy.2015.23
Jombart, T. (2008). Adegenet: a R package for the multivariate analysis of genetic markers. Bioinformatics 24, 1403–1405. doi: 10.1093/bioinformatics/btn129
Keenan, K., McGinnity, P., Cross, T. F., Crozier, W. W., and Prodöhl, P. A. (2013). Diversity: an R package for the estimation and exploration of population genetics parameters and their associated errors. Methods Ecol. Evol. 4, 782–788. doi: 10.1111/2041-210X.12067
Khanna, A., Larson, D., Srivatsan, S., Mosior, M., Abbott, T., Kiwala, S., et al. (2022). Bam-readcount - rapid generation of basepair-resolution sequence metrics. J. Open Source Softw. 7:3722. doi: 10.21105/joss.03722
Kleen, J., Taube, F., and Gierus, M. (2011). Agronomic performance and nutritive value of forage legumes in binary mixtures with perennial ryegrass under different defoliation systems. J. Agric. Sci. 149, 73–84. doi: 10.1017/S0021859610000456
Kloss, L., Fischer, M., and Durka, W. (2011). Land-use effects on genetic structure of a common grassland herb: a matter of scale. Basic Appl. Ecol. 12, 440–448. doi: 10.1016/j.baae.2011.06.001
Kölliker, R., Herrmann, D., Boller, B., and Widmer, F. (2003). Swiss Mattenklee landraces, a distinct and diverse genetic resource of red clover (Trifolium pratense L.). TAG. Theoretical and applied genetics. Theoretische und angewandte Genetik 107, 306–315. doi: 10.1007/s00122-003-1248-6
Kuchma, O., Vornam, B., and Finkeldey, R. (2011). Mutation rates in scots pine (Pinus sylvestris L.) from the Chernobyl exclusion zone evaluated with amplified fragment-length polymorphisms (AFLPs) and microsatellite markers. Mutat. Res. 725, 29–35. doi: 10.1016/j.mrgentox.2011.07.003
Lamy, T., Jarne, P., Laroche, F., Pointier, J.-P., Huth, G., Segard, A., et al. (2013). Variation in habitat connectivity generates positive correlations between species and genetic diversity in a metacommunity. Mol. Ecol. 22, 4445–4456. doi: 10.1111/mec.12399
Li, Z., Wang, P., You, C., Yu, J., Zhang, X., Yan, F., et al. (2020). Combined GWAS and eQTL analysis uncovers a genetic regulatory network orchestrating the initiation of secondary cell wall development in cotton. New Phytol. 226, 1738–1752. doi: 10.1111/nph.16468
Lischer, H. E. L., and Excoffier, L. (2012). PGDSpider: an automated data conversion tool for connecting population genetics and genomics programs. Bioinformatics (Oxford, England) 28, 298–299. doi: 10.1093/bioinformatics/btr642
Lohman, B. K., Weber, J. N., and Bolnick, D. I. (2016). Evaluation of TagSeq, a reliable low-cost alternative for RNAseq. Mol. Ecol. Resour. 16, 1315–1321. doi: 10.1111/1755-0998.12529
Marx, H. E., Scheidt, S., Barker, M. S., and Dlugosch, K. M. (2020). TagSeq for gene expression in non-model plants: a pilot study at the Santa Rita experimental range NEON core site. Applic. Plant Sci. 8:e11398. doi: 10.1002/aps3.11398
Mead, A., Peñaloza Ramirez, J., Bartlett, M. K., Wright, J. W., Sack, L., and Sork, V. L. (2019). Seedling response to water stress in valley oak (Quercus lobata) is shaped by different gene networks across populations. Mol. Ecol. 28, 5248–5264. doi: 10.1111/mec.15289
Müller, C. M., Linke, B., Strickert, M., Ziv, Y., Giladi, I., and Gemeinholzer, B. (2020). Comparative genomic analysis of three co-occurring annual Asteraceae along micro-geographic fragmentation scenarios. Persp. Plant Ecol. Evol. Syst. 42:125486. doi: 10.1016/j.ppees.2019.125486
Musche, M., Settele, J., and Durka, W. (2008). Genetic population structure and reproductive fitness in the plant Sanguisorba officinalis in populations supporting colonies of an endangered Maculinea butterfly. Int. J. Plant Sci. 169, 253–262. doi: 10.1086/524112
Nachman, M. W., and Crowell, S. L. (2000). Estimate of the mutation rate per nucleotide in humans. Genetics 156, 297–304. doi: 10.1093/genetics/156.1.297
Nei, M. (1973). Analysis of gene diversity in subdivided populations. Proc. Natl. Acad. Sci. U. S. A. 70, 3321–3323. doi: 10.1073/pnas.70.12.3321
Nybom, H. (2004). Comparison of different nuclear DNA markers for estimating intraspecific genetic diversity in plants. Mol. Ecol. 13, 1143–1155. doi: 10.1111/j.1365-294X.2004.02141.x
Osterman, J., Hammenhag, C., Ortiz, R., and Geleta, M. (2021). Insights into the genetic diversity of Nordic red clover (Trifolium pratense) revealed by SeqSNP-based genic markers. Front. Plant Sci. 12:748750. doi: 10.3389/fpls.2021.748750
Pallares, L. F., Picard, S., and Ayroles, J. F. (2020). TM3'seq: a Tagmentation-mediated 3' sequencing approach for improving scalability of RNAseq experiments. G3 10, 143–150. doi: 10.1534/g3.119.400821
Paradis, E., Claude, J., and Strimmer, K. (2004). APE: analyses of phylogenetics and evolution in R language. Bioinformatics 20, 289–290. doi: 10.1093/bioinformatics/btg412
Pfeiffer, V. W., Ford, B. M., Housset, J., McCombs, A., Blanco-Pastor, J. L., Gouin, N., et al. (2018). Partitioning genetic and species diversity refines our understanding of species-genetic diversity relationships. Ecol. Evol. 8, 12351–12364. doi: 10.1002/ece3.4530
Pinto, N., Magalhães, M., Conde-Sousa, E., Gomes, C., Pereira, R., Alves, C., et al. (2013). Assessing paternities with inconclusive STR results: the suitability of bi-allelic markers. Forensic Sci. Int. Genet. 7, 16–21. doi: 10.1016/j.fsigen.2012.05.002
Piovesan, A., Antonaros, F., Vitale, L., Strippoli, P., Pelleri, M. C., and Caracausi, M. (2019). Human protein-coding genes and gene feature statistics in 2019. BMC. Res. Notes 12:315. doi: 10.1186/s13104-019-4343-8
Pritchard, J. K., Stephens, M., and Donnelly, P. (2000). Inference of population structure using multilocus genotype data. Genetics 155, 945–959. doi: 10.1093/genetics/155.2.945
Purugganan, M. D., and Jackson, S. A. (2021). Advancing crop genomics from lab to field. Nat. Genet. 53, 595–601. doi: 10.1038/s41588-021-00866-3
R Core Team (2013) R: A language and environment for statistical computing. R Foundation for Statistical Computing: Vienna, Austria. Available online at: https://www.R-project.org/
Rosenberg, N. A. (2004). Distruct: a program for the graphical display of population structure. Mol. Ecol. Notes 4, 137–138. doi: 10.1046/j.1471-8286.2003.00566.x
Smith, R. R., Taylor, N. L., and Bowley, S. R. (1985). “Red clover” in Clover science and technology. eds. N. L. Taylor and N. L. Taylor (Madison, WI, United States: American Society of Agronomy), 457–470.
Ulloa, O., Ortega, F., and Campos, H. (2003). Analysis of genetic diversity in red clover (Trifolium pratense L.) breeding populations as revealed by RAPD genetic markers. Genome 46, 529–535. doi: 10.1139/g03-030
Vega, D. J. J., Ayling, S., Hegarty, M., Kudrna, D., Goicoechea, J. L., Ergon, Å., et al. (2015). Red clover (Trifolium pratense L.) draft genome provides a platform for trait improvement. Sci. Rep. 5:17394. doi: 10.1038/srep17394
Vellend, M., and Geber, M. A. (2005). Connections between species diversity and genetic diversity. Ecol. Lett. 8, 767–781. doi: 10.1111/j.1461-0248.2005.00775.x
Wang, Y., Lv, H., Xiang, X., Yang, A., Feng, Q., Dai, P., et al. (2021). Construction of a SNP fingerprinting database and population genetic analysis of cigar tobacco germplasm resources in China. Front. Plant Sci. 12:618133. doi: 10.3389/fpls.2021.618133
Wisecaver, J. H., Borowsky, A. T., Tzin, V., Jander, G., Kliebenstein, D. J., and Rokas, A. (2017). A global coexpression network approach for connecting genes to specialized metabolic pathways in plants. Plant Cell 29, 944–959. doi: 10.1105/tpc.17.00009
Wright, S. (1984). Variability within and among natural populations. Univ. of Chicago Press, Chicago, Ill.
Yates, S. A., Swain, M. T., Hegarty, M. J., Chernukin, I., Lowe, M., Allison, G. G., et al. (2014). De novo assembly of red clover transcriptome based on RNA-Seq data provides insight into drought response, gene discovery and marker identification. BMC Genomics 15:453. doi: 10.1186/1471-2164-15-453
Keywords: Trifolium pratense, red clover, GBS, mRNA-GBS, field conditions
Citation: Gemeinholzer B, Rupp O, Becker A, Strickert M and Müller CM (2023) Genotyping by sequencing and a newly developed mRNA-GBS approach to link population genetic and transcriptome analyses reveal pattern differences between sites and treatments in red clover (Trifolium pratense L.). Front. Ecol. Evol. 10:1003057. doi: 10.3389/fevo.2022.1003057
Edited by:
Ferhat Matur, Dokuz Eylül University, TurkeyReviewed by:
Fatih Kahrıman, Çanakkale Onsekiz Mart University, TurkeyWang Minxiao, Institute of Oceanology (CAS), China
Copyright © 2023 Gemeinholzer, Rupp, Becker, Strickert and Müller. This is an open-access article distributed under the terms of the Creative Commons Attribution License (CC BY). The use, distribution or reproduction in other forums is permitted, provided the original author(s) and the copyright owner(s) are credited and that the original publication in this journal is cited, in accordance with accepted academic practice. No use, distribution or reproduction is permitted which does not comply with these terms.
*Correspondence: Birgit Gemeinholzer, Yi5nZW1laW5ob2x6ZXJAdW5pLWthc3NlbC5kZQ==