- Faculty of Science, Queensland University of Technology, Brisbane, QLD, Australia
Many organizations are attempting to scale ecoacoustic monitoring for conservation but are hampered at the stages of data management and analysis. We reviewed current ecoacoustic hardware, software, and standards, and conducted workshops with 23 participants across 10 organizations in Australia to learn about their current practices, and to identify key trends and challenges in their use of ecoacoustics data. We found no existing metadata schemas that contain enough ecoacoustics terms for current practice, and no standard approaches to annotation. There was a strong need for free acoustics data storage, discoverable learning resources, and interoperability with other ecological modeling tools. In parallel, there were tensions regarding intellectual property management, and siloed approaches to studying species within organizations across different regions and between organizations doing similar work. This research contributes directly to the development of an open ecoacoustics platform to enable the sharing of data, analyses, and tools for environmental conservation.
Introduction
Australia has incredible fauna biodiversity, across a sparsely populated landscape, with many species under threat. Ecoacoustics offers a much-needed approach to large-scale threatened species and biodiversity monitoring. Yet there remain challenges to realizing this important vision both in Australia and globally. This paper presents an investigation into how ecoacoustics monitoring might be scaled-up to complement other ecological monitoring methods in the face of global biodiversity loss.
Passive acoustic monitoring offers the advantages of non-invasive, long-duration sampling of environmental sounds, including biodiversity, can detect cryptic species (Znidersic et al., 2020), estimate species richness (Xie et al., 2017), evaluate ecosystem health (Deichmann et al., 2018), and be used to model a species’ spatial distribution (Law et al., 2018). However, the ease with which large amounts of data can be collected complicates storage, analysis, and interpretation of results. Despite the development of new computing and visualization techniques (Eichinski and Roe, 2014; Phillips et al., 2017; Towsey et al., 2018; Truskinger et al., 2018), ecoacoustics research is currently hampered by bottlenecks in analysis and data management (Gibb et al., 2019). One challenge includes the amount of time, effort, and expertise needed to create labeled datasets with which to produce and evaluate automated call recognizers (McLoughlin et al., 2019). The use of automated methods of call identification to produce these datasets can greatly reduce the time compared to manual methods, but also increase the likelihood of false positives and negatives (Swiston and Mennill, 2009). The skillsets of computing science and ecology are also rarely held by the same person (Mac Aodha et al., 2014) leading to potential misalignments between data collection and data management protocols even in close collaborations (Vella et al., 2020). Scaling up ecoacoustics research will require a considerable effort to address the lack of standardization for acoustic data and metadata collection (Roch et al., 2016; Gibb et al., 2019), as well as the development of communities and platforms that enable the sharing of annotated datasets and tested species detection techniques. Enabling acoustic data categorization by citizen scientists also holds great promise (Jäckel et al., 2021). For example, citizen scientists engaging with Hoot Detective [a collaboration between the Australian Acoustic Observatory (A2O), the Australian Broadcasting Commission, Queensland University of Technology, and the University of New England, for National Science Week] have at the time of writing identified 2,624 native owl calls (Noonan, 2021). In the following, we focus on the Australian context, however, the challenges and opportunities that are identified are likely to be applicable in others.
A key initiative in ecoacoustics data collection, management and analysis has emerged in Australia, namely the Australian Acoustic Observatory (A20).1 The A2O (Roe et al., 2021), is a continent-wide acoustic sensor network collecting data from 360 continuously operating sensors. This and the Ecosounds platform,2 which manages the ecoacoustics data, visualization and analysis of negotiated research collaborations, are both using the open-source Acoustic Workbench software (Truskinger et al., 2021) available on GitHub. Other ecoacoustics data management is enabled by the Terrestrial Ecosystem Research Network (TERN3) project, which houses ecoacoustics data collected from TERN SuperSites: long-term research sites collecting a range of environmental data, including acoustic data. Another platform of note is the Atlas of Living Australia (ALA4), which aggregates a wide range of environmental data (i.e., observance records), but does not currently support the ingest of audio annotation observance records in a standardized form (Belbin and Williams, 2016). Finally, the Ecocommons5 platform promises to support a wide range of ecological modeling and a analysis needs, including those of ecoacoustics, through access to curated datasets, tools, and learning materials. While this suggests extensive support for ecoacoustics data management and analysis, in practice, ecoacoustics research continues to rely upon ad hoc approaches. For example, while many organizations in Australia, including universities, governments, non-government agencies such as Birdlife International, are collecting acoustic data, driven by the increasing availability of low-cost recorders, subsequent analysis tools are being developed on an individual/region basis. While recognizer development is a valid area of investigation, in this paper we focus instead on what is needed to effect smoother data interchange and improve the scale at which ecoacoustics analyses can be conducted. One means of addressing these aims is through the sharing of resources and expertise. Making ecological data open has obvious benefits, such as the re-use of datasets to answer new research questions, and the possibility of new discoveries through meta-analysis of disparate datasets (Chaudhary et al., 2010; Cadotte et al., 2012). Open data also enables local and specific data to address questions dealing with larger spatial and temporal scales (Hampton et al., 2013), and creates the impetus to make data findable, accessible, interoperable and reusable (FAIR, Wilkinson et al., 2016). One way data can be made findable is through linked data (structured data which is interlinked with other data, so it becomes more useful through semantic queries) (Bizer et al., 2011). Sharing data also opens the possibility of networking and collaboration with other researchers in and across fields. However, the movement toward open ecological data has been slow, due in part to concerns about time investment not being returned, lack of data standards, missing infrastructure, intellectual property issues, amongst others (Enke et al., 2012). Data also has a financial value, and can be withheld out of fear of losing research funding (Groom et al., 2015). The reluctance to share data for the reason of poor investment return is being slowly overcome through systems of reward and attribution (Heidorn, 2008), though these may be most effective within academic fields. While some infrastructure and standards have been developed for some forms of ecological practice, these are still in development for the management of ecoacoustics data and metadata.
Though there is also value in standards being applied to other natural or anthropogenic sounds, it should be noted that this paper focuses on biophony and ecoacoustics applications for biodiversity monitoring. Ecoacoustics standards development promises to promote understanding of long-term biodiversity trends by making acoustic data and metadata transfer across different platforms and software possible. Data and metadata standardization also goes hand-in-hand with the development of standardized approaches to ecoacoustic survey and study design. Currently, research on this topic has identified key applications (Sugai et al., 2019), approaches based on ecological research aim (Gibb et al., 2019), project specific guides (Roe et al., 2021) and some comprehensive guidelines, however, ones that do not yet provide guidance on best practice (Browning et al., 2017), or instead, focus on specific uses, such as the production of indices (Bradfer-Lawrence et al., 2019). Standardization would promote collaborative and collective efforts to collect verified call data for neglected taxa and regions (e.g., tropical terrestrial biomes). Centralized sound libraries containing consensus data and metadata standards (e.g., date/time of recording, geographic location, recording parameters, sensor position) (Roch et al., 2016), would also improve the accessibility and comparability of reference sound libraries (Mellinger and Clark, 2006). However, the movement toward standardization, as in most interdisciplinary endeavors is slow and full of friction (Edwards et al., 2011), making human-centered methods a useful approach (Vella et al., 2020).
An open science approach to ecoacoustics research should greatly increase the availability of biodiverse data annotations and call recognizers, and with standards, can maximize conservation outcomes through sharing limited resources for monitoring. However, the steps to achieving this are unclear. This study seeks to produce an overview of current practices, analytic techniques, and available metadata schemata, as well as produce a grounded understanding of how these are implemented (or not) across a range of organizations with a focus on conservation, land management, and research. Consequently, this research was largely driven by a human-centered approach to technology design with a series of online workshops conducted with a wide range of ecoacoustics practitioners. This paper concludes with a series of recommendations to guide the development of open ecoacoustics both within the Australian context and internationally.
Materials and Methods
The overall aim of this research was to inform the development of an open ecoacoustics platform and linked resources to scale-up ecoacoustics monitoring both nationally and internationally. As such, the study focused on current resources and practices utilizing exploratory review and online workshops.
Our choice to conduct workshops with a wide range of ecoacoustics practitioners was driven from a human-centered approach to technology design. We believe it necessary to do this kind of scoping with real users in a real-world context as the rich data provided by this approach provides insight into how to design for adoption and accessibility.
Review
We conducted a review of available ecoacoustic hardware, software tools and field-wide standards to better our understanding of common practices, availability of techniques and tools, and limitations related to the management and analysis of ecoacoustic data. An additional aim of the review was to identify common data formats, metadata fields, and analysis related to ecoacoustic data to inform the development of a metadata schema. We conducted a search of the literature and included reviews of the field of bioacoustics and ecoacoustics, and searched literature on bioacoustic and ecoacoustic related software using the following search terms: “ecoacoustics,” “bioacoustics,” “review,” “automated processing,” “long duration recording,” “metadata,” “analysis software,” “call recognizers,” “detection algorithm,” “recognizer performance,” “repository,” “standards.” The search of the literature was conducted between May and August 2021 and involved searching Google Scholar and the Queensland University of Technology’s Library for the above keywords. Advanced search terms included articles from 2019 to present. These publications referred to other relevant literature, which was also included. We also accessed technical guides of the hardware and software tools identified and created summary tables of the information found. Existing standards for ecoacoustic analysis procedures and the ingesting of metadata were identified. This review also informed the development of materials for the following workshops with ecoacoustics researchers, and organizations that were incorporating this research method into their programs.
Workshops
Online workshops were conducted from 25 June to 1 September 2021, under QUT Human Ethics Clearance 2021000353. We recruited participation from partner organizations and end users. In this study, we are reporting upon the end user workshops only. These workshops were carried out across two sets:
1. Current Practices: The first user workshop aimed to understand how users work and interact with their current data, tools, and technologies; explore how current activities are performed with the support of current technologies; and identify issues faced within those current practices and potential solutions to those issues.
2. Requirements Gathering: The second user workshop aimed to map out an “ideal” open ecoacoustics platform; gather requirements that would increase accessibility and improve the user experience; produce a skeleton training plan; and list IP conditions.
Participants
End users (N = 23) were recruited from ten organizations within Australia who were largely responsible for or focused on conservation management, protecting endangered species, and conducting ecological and environmental research. They included universities, conservation advocacy groups, and State government departments. Wherever possible, participants were placed in workshops with others from the same organization.
The first set of workshops had twenty participants drawn from nine groups (Australian Wildlife Conservancy, Birdlife Australia, Charles Sturt University, Department of Biodiversity, Conservation and Attraction (Western Australia), Department of Primary Industries, Parks, Water and Environment (Tasmania), Griffith University, James Cook University, Museums Victoria, and University of Melbourne. In total, eight workshops were conducted, and each workshop ran for approximately 2 h.
The second set of workshops had eleven participants from six groups (Australian Wildlife Conservancy, Bush Heritage, James Cook University, Museums Victoria, University of Melbourne, and Birdlife Australia). In total, five workshops were conducted (each between 1 and 2 h in length) and included eight participants who had also participated in the first set.
Participants varied greatly in their familiarity with ecoacoustics data collection, management, and analysis. This was most likely due to the participants holding different roles within organizations (e.g., project manager vs. project officer), as well as these organizations having different sets of capacities, funding models, and priorities (e.g., universities vs. conservation advocates).
Procedure
Each workshop was 2 h in duration, was run via Zoom and Miro (an online, collaborative whiteboard tool), and was audio and video recorded. At least two members of the research team were present during the workshops, with one running the workshop and the other taking notes. At the conclusion of each workshop, screenshots and photos were taken of the mapping activities completed in Miro and the spreadsheet template.
Workshop 1—Current Practices
At the beginning of each workshop participants were asked to introduce themselves, their role, the organization they were from and the aims of that organization, and their interest in the project. We then explored their current practices around ecoacoustics research, data collection and analysis.
As part of this exploration, we created a spreadsheet template of some of the functional aspects of common software, tools and platforms used in the workflow of ecoacoustic data management and analysis. We asked participants to use this template to identify the tools they were using in their analysis process, as well as better understand how they use them and some of the pain points associated with them. Once this was complete, we then ran a brainstorming session which explored this workflow to highlight issues and potential solutions within that workflow.
Workshop 2—Requirements Gathering
At the beginning of each workshop, participants were asked to introduce themselves. They were then immediately shown a Miro board with a selection of ecoacoustics study designs. They were asked if there were any that we hadn’t captured, and which were more important to them.
Following this they were shown example ecoacoustics use cases (one of these is presented in Figure 1), and this used to prompt discussion of their own use cases. These were listed and then reproduced in a similar format to that of Figure 1, i.e., they captured the flow of ecoacoustics data from the field, survey through to data management, analysis, and use (see pink boxes in Figure 1). Discussion followed from the construction of this use case.
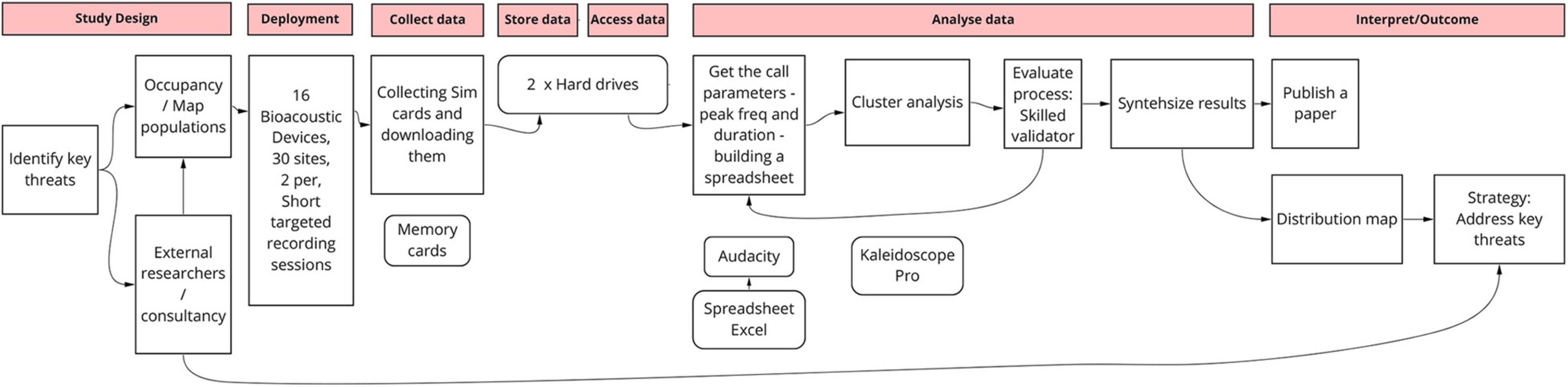
Figure 1. An example ecoacoustics use case showing the flow of data between multiple organizations. The research group QUT Ecoacoustics accesses data from an ecoacoustics portal the A2O which is then shared with the Hoot Detective citizen science project.
Once a use case was completed, it was duplicated. Participants were then asked to think about how they would like this process modified and where. Prompts included thinking about what platforms or tools would remain essential; what parts of the research process they would like to outsource; how they’d like to manage data storage. The duplicated use case was changed as necessary, and notes taken. These use cases were also used to frame discussion about training resources (what was needed, ideal delivery system, and formats); data sharing (data sensitivities, what conditions would be necessary to enable sharing). Lastly, participants were asked if there were any use cases that were worth considering in the future.
Workshop Data Analysis
The analyzed dataset includes audio transcripts, Miro board outputs, and facilitator notes taken during the workshop. Audio transcripts and facilitator notes were analyzed in Nvivo, Release 1.5. All data went through a deductive coding process driven by particular areas of research focus, which were used as deductive codes when analyzing data from both workshop 1 and 2. These codes included tools and technologies; current research practices and activities; data collection, management, and analysis; standards; education and training; relationships; needs and expectations; and challenges.
Authors 1, 2, and 3 each individually analyzed the Workshop 1 audio transcripts and facilitator notes. Following this, they met to discuss their initial analysis and examine the Miro board outputs. All insights from this process were captured in Miro, where they then conducted deductive coding to collate participant findings within codes created from predetermined research questions. These insights were used to inform Workshop 2. Author 1 conducted deductive coding of the outputs of Workshop 2 utilizing both the initial codes and the codes created from the data from Workshop 1, collecting insights from this process also in Miro. At the conclusion of both workshops, a final round of discussion amongst Authors 1, 2, 3, and 4, was conducted using data from both workshops to determine the contents of the final themes.
Results
Review Findings
This review was conducted with the aim of promoting standardization across ecoacoustics research. Standardizing data should reduce some of the frictions of interoperability between analysis programs and the harvesting of metadata from audio recordings. In turn, by making analyses and comparisons between analyses easier, data standardization should also encourage the development of standardized protocols. The following outlines the state of current guidelines and standards for acoustic recorders, data, analysis tools and techniques, and acoustic annotation. Following this is an overview of interoperability between tools; methods for evaluating recognizer performance; available acoustic repositories and registries; and finally, a summary of the key findings and gaps.
Standards for Acoustic Recorders
We reviewed the technical specifications and available metadata fields for acoustic sensor devices Song Meter 4, Song Meter SM4 Bat FS, Song Meter Mini, Song Meter Mini Bat, Song Meter Micro from Wildlife Acoustics, Inc.,6 BAR-LT from Frontier Labs,7 AudioMoth, μMoth from Open Acoustic Devices8 (Hill et al., 2018), Swift from Cornell Lab,9 and Bugg.10 Detailed information is supplied as Supplementary Material, but in brief we found that for all sensor devices except Bugg, technical specifications were easily accessible either on the website, in linked technical guides and user documents or related publications. A metadata standard for audio collected by BAR-LT sensor includes a comprehensive list of terms of key recorder and recording attributes, however this standard is not adopted by other sensor devices and is not in a format readily transferable to other devices. Whilst there are a wide variety of audio recorders available, there are no common standards between manufacturers, particularly those related to metadata standards that capture: timestamps including UTC offsets; location stamps; gain; serial numbers for sensors, microphones, and memory cards; microphone type; firmware version; temperature; battery level. The Wildlife Acoustic devices share a proprietary standard which has been reversed engineered (i.e., a program was written to interpret the information present in audio headers11), however, this method is less efficient and potentially less accurate than working from a common standard. In addition to technical specifications of the audio sensor, it is important that metadata relating to environmental and ecological factors is accounted for in statistical analysis (Browning et al., 2017). It is therefore recommended that any relevant environmental or ecological metadata (e.g., rainfall, temperature, phenological events) be collected in addition to audio.
Standards for Data
Biodiversity Information Standards (TDWG, originally called Taxonomic Database Working Group)12 have developed such as Darwin Core (Wieczorek et al., 2012) and Audubon Core (GBIF/TDWG Multimedia Resources Task Group, 2013) that have the overall aims of facilitating the sharing of information about biodiversity and representing metadata originating from multimedia resources and collections respectively. The intent of Audubon Core is to inform users of the suitability of the resource for biodiversity science application—a feature that would be desirable in an ecoacoustics standard. The Audubon Core standard contains several vocabulary terms relating specifically to audio resources and helps to promote the integration of existing standards by drawing vocabulary from other standards such as Darwin Core, Dublin Core (DCMI, 2020) and others. The standard, however, does not contain metadata terms that directly capture metadata pertaining to audio analysis and results and as such would need to be extended upon to capture all of the metadata fields that could be desired in an ecoacoustics standard. The metadata structuring rules named “Tethys,” developed by Roch et al. (2016) more adequately address metadata fields that are not currently captured by existing systems and that are specific to bioacoustic research design, analysis and quality control (Roch et al., 2016). These include terms relating to the fields of project, quality control, description of analysis method and algorithm used, and a description of annotation effort and annotation boundaries Whilst “Tethys” is a good example of a published standard of practice capturing metadata beyond just segments of audio in time, the schema is difficult to adopt outside of the Tethys Metadata Workbench. Drawing upon the example of “Tethys,” standards for ecoacoustic data should not only capture metadata about the recording itself, but metadata about the project, deployment (including survey design), recordings, objects annotated (including annotation effort and parameters) and analysis (including description of methods, algorithms, parameters, results and performance statistics). By applying standards to data in this way, comparisons and analyses of data would be easier and the development of standardized protocols would be encouraged (Roch et al., 2016).
Analysis Tools and Techniques
The entire analysis workflow of an ecoacoustics project can include various stages, such as collection of data, storage of data, manual analysis of data, automatic analysis of data, documentation of methods, and sub-sampling analysis effort. This review focused on common analysis software and does not represent a comprehensive list of analysis software, nor of the latest automated approaches to data processing. For example, the Practical AudioMoth Guide (Rhinehart, 2020) contains a list of software targeted at or used by bioacoustics researchers and that is either stable, currently in active development or recently released as of 2020.13 Darras et al. (2020) reviewed software tools built specifically for ecoacoustics and find limited software that can perform all of the data processing tasks required and Priyadarshani et al. (2018) reported 19 software tools, of which 3 were no longer in use as of 2020 (Darras et al., 2020). Browning et al. (2017) list 19 software packages and tools for analysis of acoustic recordings, including a brief summary of each software and status of availability. It is important to note that software is continually updating, with new methods of analysis emerging in the literature.
We reviewed a range of free and proprietary software (some requiring a license), consisting of cloud-based, locally run, server run, command line based or software with graphical user interfaces. The list of software reviewed includes: Audacity, Kaleidoscope Lite and Kaleidoscope Pro (Wildlife Acoustics, 2019), Raven Lite (K. Lisa Yang Center for Conservation Bioacoustics, 2016) and Raven Pro (K. Lisa Yang Center for Conservation Bioacoustics, 2011), AviaNZ (Marsland et al., 2019), Rainforest Connection (RFCx) Arbimon (Aide et al., 2013), Ecosounds, Biosounds (Darras et al., 2020), and BioAcoustica (Baker et al., 2015). The reviewed software also includes popular command line run packages such as bioacoustics (Marchal et al., 2021), monitoR (Hafner and Katz, 2018), and seewave (Sueur et al., 2008). All the software reviewed have some form of visual data inspection capacity and all software have analysis capabilities to varying degrees. Most have annotation capabilities, with approximately half having recognizer building capacity and only a few with recognizer tuning and testing capabilities. Few have detection review capabilities, and standards for evaluating recognizer performance are inconsistent across software. Furthermore, in the literature, there is a lack of consensus on the best approach to evaluating recognizer performance (see section “Evaluating Recognizer Performance”).
Of the above software, we highlight the following four software with qualities that promise to assist with realizing scalable ecoacoustics in being capable of the management and visualization of soundscape-level acoustic data, whilst also being able to support multiple kinds of analyses: Ecosounds, BioSounds (Darras et al., 2020), AviaNZ (Marsland et al., 2019), and RFCx Arbimon (Aide et al., 2013). The Ecosounds website is a key platform for the management, access, visualization and analysis of environmental acoustic data through the open-source and freely available Acoustic Workbench software—which the website hosts. In addition, the Ecosounds website acts as a repository of environmental recordings, and any annotations made are downloadable and available as.csv files making annotation outputs readable across multiple software. An advantage of the Ecosounds platform is that it is cloud based and supports the visualization and navigation of long duration and continuous recordings.
Similar to Ecosounds, BioSounds is an open-source, online platform for ecoacoustics which can manage both soundscape and reference recordings, be used to create and review annotations and also perform basic sound measurements in time and frequency (Darras et al., 2020). On the platform, recordings can be collaboratively analyzed and reference collections can be created and hosted (Darras et al., 2020). However, a major limitation of BioSounds is that at present, it does not have the capacity to develop species-specific recognizers and therefore lacks the capacity to develop efficient solutions for automatic analysis of long duration datasets—something which has been identified as one of the major barriers for the expansion of terrestrial acoustic monitoring (Sugai et al., 2019).
Another open-source and freely available software for automatic processing of long-duration acoustic recordings is AviaNZ (Marsland et al., 2019). This software facilitates the annotation of acoustic data, provides a preloaded list of species (based on New Zealand bats and birds for annotation IDs) and facilitates the building and testing of recognizers whilst providing performance metrics and statistics. AviaNZ can import annotations made with other software as well as export any lists of annotations or verified detections, therefore more readily interfacing with other software. In addition, pre-built detectors (for several New Zealand species of bats and birds) are available for use in AviaNZ and any filters (recognizers) created by users using AviaNZ can be uploaded for use on the platform, facilitating the sharing of resources among users.
RFCx Arbimon14 ‘s free, cloud based analytical tool can be used to upload audio (and bulk upload.csv files), visualize, store, annotate, aggregate, analyze, and organize audio recordings (Aide et al., 2013). RFCx Arbimon’s analysis capabilities includes automated species identification and soundscape analyses—functions that support the analysis of both bioacoustic and ecoacoustic audio data.
Our review findings support that, of the software available for data processing, none support the entire workflow or can perform all data processing tasks required by ecologists when analyzing large acoustic data sets (Darras et al., 2020).
Approaches to Annotations
There are many reasons why practitioners may choose to annotate sound data collected from the monitoring program, and these may depend on the aims of the study or program and range from being taxa dependent to purpose specific. Whilst there is no single approach to annotation, common approaches usually include start and stop times of the sound event in either time, frequency, or both, such as by the drawing of a box on a spectrogram around the event. In addition to the creation of annotations, some software can compute an array of acoustic parameters of signals of interest, which can be exported for use in statistical analyses (Rountree et al., 2020). Raven Pro software, for example, has over 70 different measurements available for rectangular time-frequency selections around signals of interest. As such, there is no standard approach to annotations. Depending on what the annotation was trying to capture/measure and what software was used to complete the annotation, variable metadata about the annotation may be available. Uniquely, the “Tethys” metadata schema introduced by Roch et al. (2016) provides an example of a structure that aims to capture annotation effort—that is, what proportion of detections were made systematically “OnEffort” and which detections were made opportunistically “OffEffort.” Specifying the analysis effort with “Tethys” includes denoting which portions of the recording were examined, as well as the target signals were being detected (“Effort”) (Roch et al., 2016). Considering the analysis effort is especially relevant when considering the number and frequency of annotations made. Considering this effort can prompt questions such as: what portion of the data were analyzed?; were all calls found, or were only one call per site/per day identified?; given the analysis effort, can the species truly be declared absent from the recordings? (Roch et al., 2016). Given the varying approaches to annotation, it would be useful to understand the level of effort and a description of the protocols used to create annotations. This could be done through the creation of a standard that offers different levels of certification of the annotation made, ranging from: (0) unknown or unstructured; (1) a protocol was followed, (2) a protocol was followed and verified and finally, (3) a protocol was followed and verified, but by multiple people.
Interoperability
With no single, unified approach to analysis of ecoacoustic data, ideally, components of analysis tools will be able to interoperate. Within the reviewed software, aspects of interoperability have been identified due to the capacity of software to ingest data of a certain format, as well as export data in a certain format. For example, Kaleidoscope Pro can create CSV format output files of detections and verified detections, and AviaNZ are RFCx Arbimon can import annotations from software in CSV or Excel format. Ecosounds can export upon request: annotations, acoustic indices and recognizer events detected CSV files. Raven Pro can export.txt format files of annotations and detection measurements, and the MonitoR packages can export sound data as text files—all of which can be imported by Audacity. The Seewave package can also import audio markers exported by Audacity. Whilst there exists some degree of interoperability due to common data formats of underlying audio data, translational issues are likely to arise when file formats are not supported; the exact structure and semantics of annotations varies between tools and additional scripts are needed; or when there is difficulty accessing and sharing resources and tools.
Evaluating Recognizer Performance
Without consistent metrics to quantify performance, it can be difficult to compare performance of analysis techniques across studies and across techniques. In addition, Browning et al. (2017) report that classification errors for proprietary software are often inadequately reported. It is desirable that recognizer performance metrics are included with any reporting of use of a recognizer, and that details of the construction of the recognizer are included (Teixeira et al., 2019). Whilst there are a range of metrics that can be used to evaluate classification performance and compare performance across studies, four agreed upon key metrics recommended by Knight et al. (2017) and Priyadarshani et al. (2018) are: precision, recall, accuracy, and F1 score (the harmonic mean of precision and recall). Calculating these four metrics for each automatic classification task allows users to contrast performance results of analyses and studies of potentially vastly different designs by using common metrics of assessment. One promising tool in development, egret (Truskinger, 2021), can be used to report efficacy of recognizers and publish the results in a standard format by evaluating precision, recall, accuracy metrics. By promoting such standards in future, comparison of studies that previously would not have been possible due to different approaches in assessing and reporting classification metrics, will become possible.
Available Archives
The need for more extensive and detailed collections of labeled ecoacoustic data to support automated call recognition has been identified (Gibb et al., 2019). These can take the form of registries (that register the various locations of data), repositories, and reference libraries We define a repository as software capable of storing annotations for large acoustic datasets—the annotations of which are particularly important for supervised machine learning tasks (McLoughlin et al., 2019). Of the above reviewed software, Ecosounds, BioSounds, AviaNZ, and RFCx’s Arbimon have the capacity to also act as a repository. Separate to repositories are reference libraries, for example Macauly sound library,15 Xeno-Canto16 (mainly oriented for birds) and BioAcoustica (Baker et al., 2015) and Zenodo17 Whilst reference libraries and repositories offer potentially high quality reference material, often certain taxonomic groups, habitats and regions are data deficient (Browning et al., 2017). Further limitations of reference libraries and repositories is that currently recordings of single species prevail over soundscape recordings (Gibb et al., 2019; Abrahams et al., 2021). If these potentially data rich repositories and reference libraries are to be used in the development, testing, comparison and validation of machine learning methods for ecoacoustic applications, then standardized methods of describing these datasets will ensure that they are both findable to researchers and assessable for fitness and inclusion into studies.
Key Findings and Identification of Gaps
This review finds that there is no one best approach or best choice of analysis techniques or best software that can be used in the processing of ecoacoustic data. Few freely available, open-source analysis tools available unify the multiple steps of the ecoacoustics workflow. Whilst there is some evidence of interoperability among software, few software have the capacity to share analysis and annotations. Some key reference libraries and repositories exist, however there is a lack of availability of strongly labeled datasets (in both publications and repositories) due to the absence of clear standards and the effort required to create such datasets. Few software that have the capacity to manage soundscape level data by visualizing large amounts of acoustic data also have the capacity to develop and test species-specific recognizers. Of the metadata schemas reviewed, none contain enough ecoacoustics-specific terms to capture the level of data that practitioners, moving forward, may wish to track. Finally, whilst conventions for annotations exist, there is yet to be a standard approach to annotations which is likely to continue to impact upon the reusability of labeled training datasets using in machine learning classification tasks.
Workshop Findings
Our workshop revealed tensions around data management and identified a range of analytic pain points. Participants reported a need for help locating learning resources that describe the most appropriate software, analytic techniques, and processes. We also identified a range of considerations for encouraging ecoacoustics data openness, and interoperability challenges.
Data Management and Analysis
Common practices around data storage included the collection of data on hard drives and memory cards, and their subsequent storage on the same. Backup of data could include duplication onto more hard drives, and very occasionally, cloud storage. Cloud storage costs were mitigated by making use of free services such as Arbimon.
Effort was expended creating consistent file structures when data was collected from different recorders (with different associated metadata); as well as when porting data between software with different data fields (see Figure 2 for an example workflow from Workshop 2). Participants would make use of multiple software and platforms to access the functionality they needed. While simple scripts could address these, less computer science savvy users were not always able to easily produce these. Ad hoc workarounds were developed that might negatively impact reuse of data, for example the division of large sound files into 1-min segments to expedite analysis. This practice destroys associated metadata necessary for archiving and produces a set of files that might negatively impact the performance of any hosting platform (decreasing the likelihood that a platform might accept this data at a later date).
Recognizer development was similarly hampered, both in terms of learning the best techniques to apply, applying these techniques, annotation of datasets, and verification. Users from conservation advocacy groups reported, in some cases, that analyses being outsourced to contractors to overcome some of these issues. Many participants identified that expert validation was particularly important, especially when monitoring critically endangered species. Most users trained recognizers with datasets specific to regions and because of regional variation in species’ calls, recognizer re-use in other regions may be limited or challenging. Anascheme (Gibson and Lumsden, 2003) was identified as a potential solution because it had developed “regional keys,” a signature for a species across different regions. Participants reported a strong desire for a “toolkit” of recognizers from many regions, however there was also a need for these recognizers to be associated with metadata or “notes” describing the process through which the recognizer was developed.
Finally, citizen science was seen as a possible solution to the bottleneck in the generation of annotated datasets, but existing platforms have not been optimized for audio tasks. For example, Zooniverse (Simpson et al., 2014) and Arbimon (Aide et al., 2013) were being used for annotation and verification of identifications but were not well suited to audio analysis or segmentation.
Training and Education
Finding training for new techniques was described as a “black box,” or opaque, by one participant. Participants sought training information and help from hardware manufacturers (e.g., Wildlife Acoustics, 2019), software forums, authors of academic papers on ecoacoustics, and through their networks. Knowledge varied across groups, with the university groups reporting greater confidence in their ability to choose and carry out the most appropriate analysis technique for their research question and data. Conservation advocacy groups were interested in being able to enable community groups to work on their own projects, as well as to upskill land managers, but had limited capacity (time, money, staff) to learn ecoacoustics analysis techniques. Choice of tool was driven by cost, familiarity, recommendation, and any additional benefits (e.g., free data storage, such as with Arbimon).
Participants identified a need for advice on best practice, across a range of areas including:
• Guidelines on data collection procedures including how to design monitoring programs to best use acoustics with other techniques in the field
• Choice of monitoring tools
• Ideal recording parameters for the target species or habitat
• How to prepare and analyze data, including the best techniques for developing recognizers
• The kinds of metadata that should be collected
• How to compare different types of data in a meaningful way
Specific examples were preferred that showed how people have tackled problems. Some mechanism for triaging analytic techniques based on data type and research question was also seen as desirable. Participants also demonstrated varied levels of confidence and capacity for learning the programming skills necessary for some analytic and data management tasks, with universities being better equipped than conservation advocacy groups. Developing user interfaces and tools that account for users with low levels of programming experience or the time to learn these may supplant the need for some forms of technical training.
Workshops were the preferred format for the delivery of ecoacoustics training and education, though it was noted that attending live events was challenged by fieldwork commitments. Workshops were seen as a way to collaborate across disciplines (ecology, computer science) to solve current problems. Having materials online and always available was considered necessary, particularly for organizations operating with limited resources and those engaging in seasonal fieldwork. Forums were desirable as this would allow researchers to ask questions and seek expert advice.
Interoperability
Participants reported using analysis tools including RFCx Arbimon, Audacity, Kaleidoscope Lite and Pro, Raven Lite and Pro, various R scripts including MonitoR, Seewave and more. It was recognized that being able to relate acoustics data to other forms of data provided much needed context. Consequently, there was a strong desire to be able to input ecoacoustics data into other ecological modeling tools, for example to relate acoustic data to environmental variables such as water level, habitat structure, or vegetation, or to spatially and temporally model species occupancy. Figure 3 shows a current practice in which indices and verified annotations are compared with other types of data using an analysis process with multiple streams. Interoperation with other platforms such as Atlas of Living Australia (Belbin and Williams, 2016) was also seen as desirable.
Hardware outputs, e.g., file formats, were not always well suited to analytic software requirements and the need for common data interchange formats was recognized. Issues were identified with the upload and download of audio from analytic platforms.
Intellectual Property and Sharing
Participants reported a lack of awareness regarding other groups’ ecoacoustics projects, with siloing of information sometimes experienced within groups operating across multiple regions. There was a strong desire to discover and use acoustic data and/or recognizers that others had developed as this would minimize the labor costs of monitoring and increase their overall ability to monitor environments.
Various barriers to sharing this data were identified. Participants universally stated a reluctance to share data on sensitive species (e.g., critically endangered), and noted that partnership agreements with academics, landowners, commercial companies, and Indigenous communities may also impact sharing. Less anticipated was the disclosed reluctance to share data that might be leveraged for philanthropic funding by another organization. In the space of not-for-profit conservation advocacy, this funding is directly tied to being able to show novel or innovative outcomes that might engage the public, and organizations were in competition for these funds. Another barrier to sharing large acoustic datasets was the possibility of it containing human voices and an inability to automatically detect them.
Methods to encourage sharing included producing clear guidelines and licensing agreements for sharing and copyright of data. Levels of access were also explored that might give users more control over who could access the data, and when. Associated location data would in some cases need to be obscured (e.g., private land, sensitive species). Intellectual property agreements would need to account for relationships between universities, other not-for-profit organizations, community groups, Indigenous communities, and commercial enterprises.
Discussion
Realizing scalable ecoacoustics monitoring is hampered by some of the problems common to emerging fields. These include a lack of consensus regarding the best techniques to apply to a given research problem, and a lack of infrastructure to accommodate the specificities of data use and management. This study combined a review and workshops with ecoacoustics practitioners, to identify key issues for scaling up ecoacoustics monitoring programs. Contributing factors were identified by both the review and the workshop methods. These include a lack of standardization in methods, poor software and platform interoperability, difficulty finding training resources and best practice examples, and a lack of ecoacoustics data storage infrastructure available to a wide range of ecoacoustics practitioners. Of these, some practitioners were impacted more than others, with university-led users having greater access to expertise, as well as high-performance computing and data storage. Specific data sensitivities were also identified that impact how data sharing would be negotiated. The next section discusses these points and offers recommendations across the areas of enabling the sharing of data and metadata; standardization and other improvements to ecoacoustics data analysis workflows; and knowledge and skill acquisition for a range of actors. Finally, we make recommendations to ensure that ecoacoustics research becomes increasingly open and FAIR (Wilkinson et al., 2016).
Registries and Repositories
Making datasets and call recognizers searchable through either a registry or repository would enable the field of ecoacoustics to greatly expand its analytic capacity, by allowing recognizers to be re-used, and by increasing the number of cross-region and longitudinal comparisons that can be made (Hampton et al., 2013). Repositories also contribute to the wider ecoacoustics landscape by reorienting research toward openness, as they represent successful negotiations around intellectual property rights, and the fields’ long-term aspirations. The movement toward open data requires a critical mass, where the most common and basic operations are documented, open, and standard.
We propose a method for sharing recognizers and evaluation datasets. Each recognizer made should be published to a source code repository, like a GitHub repository. This model allows researchers to publish recognizers openly by default while also allowing for private repositories (useful for embargo situations). Users can also maintain sovereignty for their recognizers (as they are version controlled), group recognizers together by project or organization, and generate DOIs automatically through services like Zenodo. Training and test datasets, depending on their size, can be published with the recognizers, or linked from the repository by using tools like Git LFS18 or the increasingly popular DVC toolset, which is used to track datasets for experiments (Kuprieiev et al., 2021). Tools like egret (Truskinger, 2021) can be used to report efficacy of recognizers and publish the results in a standard format. Template recognizer repositories can be set up and published along with guides to make this process easier for beginners.
Standardization and Interoperability
Standards would also greatly support interoperability between software and platforms. Our review of metadata standards reveals there are few dedicated environmental audio standards, and none that are open and accessible. Whilst Tethys is the best example of a metadata schema containing fields that go beyond just technical specifications of acoustic sensor devices and ecological and environmental data accompanying recordings, we suggest that the most sustainable and responsive model for standards development is open—something that Audubon Core excels at. Further, we believe that linked data and formal ontologies, while important, aren’t useful in day-to-day scientific work. We propose that linked data standard (such as Dublin Core, and the extensions relevant to us, like Biodiversity Information Standards, Audubon Core, and the annotations interest groups) are most important to technical implementers, like archives, software, device manufactures and other actors that need to share data or otherwise interoperate. Not one of our workshop participants mentioned linked data, ontologies, or other technical minutiae, however, this is explained by the lack of participants with a background focused on structuring information so that it is searchable, persistent, and linked to other data.
Currently ecoacoustics practitioners are using a wide range of acoustic editing and analytic tools to complete analysis. Whilst some software may focus on targeting one or a few analysis functions within the ecoacoustic workflow, key challenges remain in managing soundscape level data. In particular, the capacity to develop or test species-specific recognizers, and to upload and visualize large amounts of acoustic data challenges practitioners. Issues also emerge when porting data between software to access additional functionality. Ideally, there needs to be more integration of a suite of analysis functions tools into software to perform a greater proportion of the acoustic analysis workflow. Alternatively, translational software that assists with software interoperability could address some of these issues. There is also a need to compare ecoacoustics data with other forms of data (e.g., to conduct spatial modeling). Although there are ad hoc approaches to achieve this (see Law et al., 2018), these analyses would be greatly aided by free, non-proprietary, cloud-based tools. While there are some software that meet one or more of these requirements, few can capture all three criteria in a way that can be scaled-up.
Opening up ecoacoustics data necessarily requires consideration of interoperability with existing data platforms, and for this to include publishing to or allowing access to audio data and derived data (e.g., annotations or calculated statistics from audio data). These are opportunities where translational tools can help scientists transfer their data between platforms using formal data standards, for example, to enable annotations of acoustic events to be uploaded as observance records to the Atlas of Living Australia. Similarly, existing citizen science platforms (e.g., Zooniverse) that are not currently well suited to acoustics data categorization and annotation could benefit from generalized tools for working with audio. Working with these platforms to enhance their capacity to utilize acoustic data will greatly aid the development of automated methods of detection and raise the profile of ecoacoustics more broadly.
Best Practice and Training
Relatedly, ecoacoustics training resources aimed at undergraduate ecology and land management courses would greatly aid the development and the standardization of methods in the field. As an emerging field, best practice is a work-in-progress. To date, no comprehensive guide to ecoacoustic survey and study design exists, though promising directions are indicated (Browning et al., 2017; Bradfer-Lawrence et al., 2019; Gibb et al., 2019; Sugai et al., 2019). However, there is currently enough collective knowledge to provide worked examples of how to approach a number of research questions with ecoacoustics methods. File organization, sensor deployment, sensor settings, relevant field data needed for collection, dealing with audio files, recommendations for storage, and many other topics are all worthwhile publishing. The goal should be broad adoption of easy-to-use best practices that are easy to understand. With this platform of practices set up, the goal will be to have these de facto standards coalesce into true standards. Suggested data formats and layouts, become de facto standards through adoption, and critical mass will lead to tools that interoperate with these formats, which will realize gains for the community. Similarly, to the recognizer registry proposed above, there will be need for public contribution, version tracking, citable, and transparency when developing these standards of practice. A wiki platform or a source code repository are ideal choices if consumers see a website first, and editors can be onboarded in a friendly manner.
In addition to best practices, there is value in creating more formal training resources. Based on our participants’ responses we can suggest that training resources need to be free for not-for-profits, on-demand, modular, facilitate interactive learning (questioning/answering), and tied to research questions. Ideally, any educational material recommends the use of software. Currently, with a plethora of analysis software, tools, and techniques available, there is no clear guide to what technique/software to apply when, or to which ecological problem. Of the software reviewed, categories of analysis software ranged from being locally installed, run from the cloud or a server, with either command line or Graphic User Interface interaction. Some software is paid and requires a license, whereas others are freely available. The choice of analysis software and technique will largely depend on requirements such as whether the software is free, whether it can handle large amounts of acoustic data, whether it can perform analysis to the desired level and whether it is accessible to the user. We recommend that formal training resources recommend software that is open-source and caters for a range of technical experience—allowing for simple and effective analyses with little to no code, scaling up to resources supporting advanced programming (like deep learning research).
Open Ecoacoustics
Addressing these components—registries and repositories, standardization and interoperability, best practices, and training—will allow ecoacoustics monitoring to practically scale up. Collectively, these improvements will also make ecoacoustics methods more accessible for less well-resourced actors such as not-for-profit conservation organizations. These groups are well-versed in the promotion of conservation initiatives as well as community engagement and—together with improvements in ecoacoustics citizen science methods—these groups have the potential to greatly influence the public imagination. This in turn, can support the movement toward gaining widely accessible open ecoacoustics data repositories. However, the changes suggested above do not entirely address the challenges of open ecoacoustics. As such, we make the following recommendations for promoting open ecoacoustics research:
• Publication of ecoacoustics research should require submission of data to an appropriate repository.
○ Recognizers or other classification tools should be published as per the recognizer repository concept.
○ Original audio recording data should be placed in a suitable archive, such as an Acoustic Workbench instance or RFCx’s Arbimon.
• Opportunities for decentralized community collaboration should be produced, particularly for best practices and guides.
• Ecoacoustics leaders and organizations should place an emphasis on open by default research and data. Options for sensitive data or proprietary intellectual property agreements need to continue to exist but should be communicated as the exception to the rule.
○ Particularly for vulnerable species, archives of data must be transparent in their dissemination of said data and build trust with stakeholders when storing data. Options for embargos and fine-grained access control are paramount. These levels of access are to be reviewed periodically.
○ Some forms of metadata must remain open regardless of data sensitivities, e.g., project name, what type of data was collected, who collected it, and how it was collected may be negotiated stay open so that data remains searchable, but users may wish for greater levels of control regarding the where and when.
• Ecoacoustic platforms (software, archives, and hardware) must work cooperatively on formal data standards and interoperation.
• Ecoacoustic archives must invest persistent identifiers for their data collections. Datasets need to be associated with Digital Object Identifiers (DOIs), other research related persistent identifiers, and citing data must become commonplace.
Limitations
We did not review every available tool, technique, or software relating to ecoacoustics. Further review of existing survey and study designs that synthesize commonalities would also be valuable. Obvious next steps include systematically reviewing the literature and supplementing this with a broad survey of common practices, worldwide. However, we are confident, based upon similarities with the workshop findings, that the current review does provide a representative illustration of the types of techniques currently used in the ecoacoustics field.
The workshop participants from organizations conducting conservation research and/or land management. We did not recruit participants from industry (e.g., forestry, agriculture, mining), or commercial environmental consultants. It seems likely that these groups may have different needs for intellectual property protection that would impact open data agreements. Further research in this direction could consider how commercial and conservation-focused ecoacoustics interact and how data openness and protections might be achieved. Additionally, participation in the workshops was limited to Australian ecoacoustics practitioners. Australia is unique in its environmental conditions and species, and some of the problems our participants face may not be faced by ecoacoustics practitioners elsewhere. Though the opposite is also true, and the specificities of what is needed to realize scalable ecoacoustic monitoring in other parts of the globe requires further investigation.
While the workshop participants mentioned complexities with the development of call recognizers with training data from species of different regions to their target species, we did not follow up with an in-depth account of how they tackled this technically. Clearly, there is a need for tools and techniques that can address regional variation in calls, and this remains a design challenge that could be further explored through a canvasing of current techniques.
Conclusion
This study of current ecoacoustics practices, tools, and standards highlights the key obstacles for realizing scalable ecoacoustic monitoring for conservation and suggests ways to move forward. Strategies have been identified that address the challenges identified by workshop participants, and the gaps established by the review. Amongst these are the continued development of formal standards by platforms and the establishment of open-source best practices for scientists and related stakeholders (e.g., land managers in charge of deployment). Additionally, the development and production of training and learning materials is needed to guide the next generation of ecoacoustics researchers. Recognizer repositories and registries should be established with a focus on open-by-default methods and practices. This will be supported by the publication of data along with research being strongly encouraged by organizations and journals, and a focus on FAIR data—that is findable, accessible, interoperable, and reusable (Wilkinson et al., 2016)—and persistent identifiers for said data.
There is massive potential for the ecoacoustics field to influence biodiversity research and conservation, as well as computing techniques in related fields (e.g., bioinformatics). With the aforementioned suggestions implemented, the field of ecoacoustics can continue to grow into an established science.
Data Availability Statement
The datasets presented in this article are not readily available because we do not have ethical permission to share raw, anonymized human data (interview transcripts) publicly. Requests to access the datasets should be directed to corresponding author.
Ethics Statement
The studies involving human participants were reviewed and approved by the University Human Research Ethics Committee (UHREC), Queensland University of Technology. The patients/participants provided their written informed consent to participate in this study.
Author Contributions
KV, TC, and AG contributed to the method, data collection, analysis, first draft, and final edits. AT contributed to the analysis, first draft, and final edits. SF and PR contributed to the research design, final edits, and project funding. All authors contributed to the article and approved the submitted version.
Funding
This research was supported by the Australian Research Data Commons (ARDC). The ARDC was funded by NCRIS.
Conflict of Interest
The authors declare that the research was conducted in the absence of any commercial or financial relationships that could be construed as a potential conflict of interest.
Publisher’s Note
All claims expressed in this article are solely those of the authors and do not necessarily represent those of their affiliated organizations, or those of the publisher, the editors and the reviewers. Any product that may be evaluated in this article, or claim that may be made by its manufacturer, is not guaranteed or endorsed by the publisher.
Acknowledgments
We wish to thank our participants and participating organizations for their time and input, including: the Australian Wildlife Conservancy, Birdlife Australia, Bush Heritage, Charles Sturt University, Department of Biodiversity, Conservation and Attraction (Western Australia), Department of Primary Industries, Parks, Water and Environment (Tasmania), Griffith University, James Cook University, Museums Victoria, and University of Melbourne.
Supplementary Material
The Supplementary Material for this article can be found online at: https://www.frontiersin.org/articles/10.3389/fevo.2021.809576/full#supplementary-material
Footnotes
- ^ https://acousticobservatory.org
- ^ https://www.ecosounds.org/
- ^ https://www.tern.org.au/
- ^ https://www.ala.org.au/
- ^ https://www.ecocommons.org.au/
- ^ https://www.wildlifeacoustics.com
- ^ https://frontierlabs.com.au
- ^ https://www.openacousticdevices.info
- ^ https://www.birds.cornell.edu/ccb/swift/
- ^ https://www.bugg.xyz/
- ^ https://github.com/riggsd/guano-spec
- ^ http://www.tdwg.org
- ^ https://github.com/rhine3/audiomoth-guide/blob/master/resources/analysis-software.md
- ^ https://arbimon.rfcx.org/
- ^ www.macaulaylibrary.org
- ^ www.xeno-canto.org
- ^ https://zenodo.org
- ^ https://git-lfs.github.com/
References
Abrahams, C., Desjonquères, C., and Greenhalgh, J. (2021). Pond acoustic sampling scheme: a draft protocol for rapid acoustic data collection in small waterbodies. Ecol. Evol. 11, 7532–7543. doi: 10.1002/ece3.7585
Aide, T. M., Corrada Bravo, C., Campos Cerqueira, M., Milan, C., Vega, G., and Alvarez, R. (2013). Real-time bioacoustics monitoring and automated species identification. PeerJ 1:e103. doi: 10.7717/peerj.103
Baker, E., Price, B. W., Rycroft, S. D., Hill, J., and Smith, V. S. (2015). BioAcoustica: a free and open repository and analysis platform for bioacoustics. Database 2015:bav054. doi: 10.1093/database/bav054
Belbin, L., and Williams, K. J. (2016). Towards a national bio-environmental data facility: experiences from the atlas of living Australia. Int. J. Geogr. Inf. Sci. 30, 108–125. doi: 10.1080/13658816.2015.1077962
Bizer, C., Heath, T., and Berners-Lee, T. (2011). “Linked data: the story so far,” in Semantic Services, Interoperability and Web Applications: Emerging Concepts, ed. A. Sheth (Hershey, PA: IGI Global), 205–227.
Bradfer-Lawrence, T., Gardner, N., Bunnefeld, L., Bunnefeld, N., Willis, S. G., and Dent, D. H. (2019). Guidelines for the use of acoustic indices in environmental research. Methods Ecol. Evol. 10, 1796–1807. doi: 10.1111/2041-210X.13254
Browning, E., Gibb, R., Glover-Kapfer, P., and Jones, K. E. (2017). Passive Acoustic Monitoring in Ecology and Conservation. Woking: WWF-UK.
Cadotte, M., Mehrkens, L., and Menge, D. (2012). Gauging the impact of meta-analysis on ecology. Evol. Ecol. 26, 1153–1167. doi: 10.1007/s10682-012-9585-z
Chaudhary, B., Walters, L., Bever, J., Hoeksema, J., and Wilson, G. (2010). Advancing synthetic ecology: a database system to facilitate complex ecological meta-analyses. Bull. Ecol. Soc. Am. 91, 235–243. doi: 10.1890/0012-9623-91.2.235
Darras, K., Pérez, N., and Mauladi, and Hanf-Dressler, T. (2020). BioSounds: an open-source, online platform for ecoacoustics. F1000Research 9:1224. doi: 10.12688/f1000research.26369.1
DCMI (2020). DCMI Metadata Terms. Available online at: https://www.dublincore.org/specifications/dublin-core/dcmi-terms/ (accessed October 30, 2021).
Deichmann, J. L., Acevedo-Charry, O., Barclay, L., Burivalova, Z., Campos-Cerqueira, M., d’Horta, F., et al. (2018). It’s time to listen: there is much to be learned from the sounds of tropical ecosystems. Biotropica 50, 713–718. doi: 10.1111/btp.12593
Edwards, P. N., Mayernik, M. S., Batcheller, A. L., Bowker, G. C., and Borgman, C. L. (2011). Science friction: data, metadata, and collaboration. Soc. Stud. Sci. 41, 667–690. doi: 10.1177/0306312711413314
Eichinski, P., and Roe, P. (2014). “Heat maps for aggregating bioacoustic annotations,” in Proceedings of the 2014 18th International Conference on Information Visualisation, Paris.
Enke, N., Thessen, A., Bach, K., Bendix, J., Seeger, B., and Gemeinholzer, B. (2012). The user’s view on biodiversity data sharing — investigating facts of acceptance and requirements to realize a sustainable use of research data. Ecol. Inf. 11, 25–33. doi: 10.1016/j.ecoinf.2012.03.004
GBIF/TDWG Multimedia Resources Task Group (2013). Audubon Core Multimedia Resources Metadata Schema. Biodiversity Information Standards (TDWG). Available online at: http://www.tdwg.org/standards/638 (accessed October 30, 2021).
Gibb, R., Browning, E., Glover-Kapfer, P., and Jones, K. E. (2019). Emerging opportunities and challenges for passive acoustics in ecological assessment and monitoring. Methods Ecol. Evol. 10, 169–185. doi: 10.1111/2041-210X.13101
Gibson, M., and Lumsden, L. (2003). The AnaScheme automated bat call identification system. Austr. Bat Soc. Newslett. 20, 24–26.
Groom, Q., Desmet, P., Vanderhoeven, S., and Adriaens, T. (2015). The importance of open data for invasive alien species research, policy and management. Manag. Biol. Invas. 6, 119–125. doi: 10.3391/mbi.2015.6.2.02
Hafner, S. D., and Katz, J. (2018). monitoR: Acoustic Template Detection in R [Computer software] R package Version 1.0.7. Available online at: http://www.uvm.edu/rsenr/vtcfwru/R/?Page=monitoR/monitoR.htm (accessed October 30, 2021).
Hampton, S. E., Strasser, C. A., Tewksbury, J. J., Gram, W. K., Budden, A. E., Batcheller, A. L., et al. (2013). Big data and the future of ecology. Front. Ecol. Environ. 11:156–162. doi: 10.1890/120103
Heidorn, P. (2008). Shedding light on the dark data in the long tail of science. Libr. Trends 57, 280–299. doi: 10.1353/lib.0.0036
Hill, A. P., Prince, P., Piña Covarrubias, E., Doncaster, C. P., Snaddon, J. L., and Rogers, A. (2018). AudioMoth: evaluation of a smart open acoustic device for monitoring biodiversity and the environment. Methods Ecol. Evol. 9, 1199–1211. doi: 10.1111/2041-210X.12955
Jäckel, D., Mortega, K. G., Sturm, U., Brockmeyer, U., Khorramshahi, O., and Voigt-Heucke, S. L. (2021). Opportunities and limitations: a comparative analysis of citizen science and expert recordings for bioacoustic research. PLoS One 16:e0253763. doi: 10.1371/journal.pone.0253763
K. Lisa Yang Center for Conservation Bioacoustics (2011). Raven Pro: Interactive Sound Analysis Software [Computer software]. Available online at: http://ravensoundsoftware.com/ (accessed October 30, 2021).
K. Lisa Yang Center for Conservation Bioacoustics (2016). Raven Lite: Interactive Sound Analysis Software [Computer software]. Available online at: http://ravensoundsoftware.com/ (accessed October 30, 2021).
Knight, E. C., Hannah, K. C., Foley, G. J., Scott, C. D., Brigham, R. M., and Bayne, E. (2017). Recommendations for acoustic recognizer performance assessment with application to five common automated signal recognition programs. Avian Conserv. Ecol. 12:14. doi: 10.5751/ACE-01114-120214
Kuprieiev, R., Pachhai, S., Petrov, D., Redzyñski, P., da Costa-Luis, C., Schepanovski, A., et al. (2021). DVC: Data Version Control - Git for Data & Models. Zenodo. Available online at: https://zenodo.org/record/5654595 (accessed October 30, 2021).
Law, B. S., Brassil, T., Gonsalves, L., Roe, P., Truskinger, A., and McConville, A. (2018). Passive acoustics and sound recognition provide new insights on status and resilience of an iconic endangered marsupial (koala Phascolarctos cinereus) to timber harvesting. PLoS One 13:e0205075. doi: 10.1371/journal.pone.0205075
Mac Aodha, O., Stathopoulos, V., Brostow, G. J., Terry, M., Girolami, M., and Jones, K. E. (2014). “Putting the scientist in the loop – accelerating scientific progress with interactive machine learning,” in Proceedings of the 22nd International Conference on Pattern Recognition, Stockholm, 24–28.
Marchal, J., Fabianek, F., Scott, C., Corben, C., Riggs, D., Wilson, P., et al. (2021). bioacoustics: Analyse Audio Recordings and Automatically Extract Animal Vocalizations [Computer software]. Available online at: https://github.com/wavx/bioacoustics/ (accessed October 30, 2021).
Marsland, S., Priyadarshani, N., Juodakis, J., and Castro, I. (2019). AviaNZ: a future-proofed program for annotation and recognition of animal sounds in long-time field recordings. Methods Ecol. Evol. 10, 1189–1195. doi: 10.1111/2041-210x.13213
McLoughlin, M., Stewart, R., and McElligott, A. (2019). Automated bioacoustics: methods in ecology and conservation and their potential for animal welfare monitoring. J. R. Soc. Interface 16:20190225. doi: 10.1098/rsif.2019.0225
Mellinger, D. K., and Clark, C. W. (2006). MobySound: a reference archive for studying automatic recognition of marine mammal sounds. Appl. Acoust. 67, 1226–1242. doi: 10.1016/j.apacoust.2006.06.002
Noonan, C. (2021). Hoot Detective. National Science Week. Available online at: https://www.scienceweek.net.au/hoot-detective/ (accessed October 31, 2021)
Phillips, Y. F., Towsey, M., and Roe, P. (2017). “Visualization of environmental audio using ribbon plots and acoustic state sequences,” in Proceedings of the 2017 International Symposium on Big Data Visual Analytics (BDVA), Adelaide, SA.
Priyadarshani, N., Marsland, S., and Castro, I. (2018). Automated birdsong recognition in complex acoustic environments: a review. J. Avian Biol. 49:jav–01447. doi: 10.1111/jav.01447
Rhinehart, T. A. (2020). AudioMoth: A Practical Guide to the Open-Source ARU. GitHub repository. Available online at: https://github.com/rhine3/audiomoth-guide (accessed October 30, 2021).
Roch, M. A., Batchelor, H., Baumann-Pickering, S., Berchok, C. L., Cholewiak, D., Fujioka, E., et al. (2016). Management of acoustic metadata for bioacoustics. Ecol. Inf. 31, 122–136. doi: 10.1016/j.ecoinf.2015.12.002
Roe, P., Eichinski, P., Fuller, R. A., McDonald, P. G., Schwarzkopf, L., Towsey, M., et al. (2021). The Australian acoustic observatory. Methods Ecol. Evol. 12, 1802–1808. doi: 10.1111/2041-210X.13660
Rountree, R. A., Juanes, F., and Bolgan, M. (2020). Temperate freshwater soundscapes: a cacophony of undescribed biological sounds now threatened by anthropogenic noise. PLoS One 15:e0221842. doi: 10.1371/journal.pone.0221842
Simpson, R., Page, K. R., and De Roure, D. (2014). “Zooniverse: observing the world’s largest citizen science platform,” in Proceedings of the 23rd International Conference on World Wide Web, Seoul.
Sueur, J., Aubin, T., and Simonis, C. (2008). Seewave: a free modular tool for sound analysis and synthesis. Bioacoustics 18, 213–226. doi: 10.1080/09524622.2008.9753600
Sugai, L. S. M., Desjonquères, C., Silva, T. S. F., and Llusia, D. (2019). A roadmap for survey designs in terrestrial acoustic monitoring. Remote Sens. Ecol. Conserv. 6, 220–235.
Swiston, K. A., and Mennill, D. J. (2009). Comparison of manual and automated methods for identifying target sounds in audio recordings of pileated, pale-billed, and putative Ivory-billed woodpeckers. J. Field Ornithol. 80, 42–50. doi: 10.1111/j.1557-9263.2009.00204.x
Teixeira, D., Maron, M., and van Rensburg, B. J. (2019). Bioacoustic monitoring of animal vocal behavior for conservation. Conserv. Sci. Pract. 1:e72. doi: 10.1111/csp2.72
Towsey, M., Znidersic, E., Broken-Brow, J., Indraswari, K., Watson, D. M., Phillips, Y., et al. (2018). Long-duration, false-colour spectrograms for detecting species in large audio data-sets. J. Ecoacoust. 2, 1–13. doi: 10.22261/JEA.IUSWUI
Truskinger, A. (2021). QutEcoacoustics/egret: Version 1.0.0 (1.0.0) [Computer software]. Brisbane, QLD: Zenodo, doi: 10.5281/zenodo.5644413
Truskinger, A., Brereton, M., and Roe, P. (2018). “Visualizing five decades of environmental acoustic data,” in Proceedings of the 2018 IEEE 14th International Conference on e-Science (e-Science), Brisbane, QLD: Amsterdam.
Truskinger, A., Cottman-Fields, M., Eichinski, P., Alleman, C., and Roe, P. (2021). QutEcoacoustics/baw-Server: Zenodo chore release (3.0.4) [Computer software]. Zenodo, doi: 10.5281/zenodo.4748041
Vella, K., Oliver, J. L., Dema, T., Brereton, M., and Roe, P. (2020). “Ecology meets computer science: designing tools to reconcile people, data, and practices,” in Proceedings of the 2020 CHI Conference on Human Factors in Computing Systems, (New York, NY: Association for Computing Machinery).
Wieczorek, J., Bloom, D., Guralnick, R., Blum, S., Döring, M., Giovanni, R., et al. (2012). Darwin core: an evolving community-developed biodiversity data standard. PLoS One 7:e29715. doi: 10.1371/journal.pone.0029715
Wildlife Acoustics (2019). Kaleidoscope Pro Analysis Software [Computer Software]. Maynard, MA: Wildlife Acoustics.
Wilkinson, M. D., Dumontier, M., Aalbersberg, I. J., Appleton, G., Axton, M., Baak, A., et al. (2016). The FAIR guiding principles for scientific data management and stewardship. Sci. Data 3:160018. doi: 10.1038/sdata.2016.18
Xie, J., Towsey, M., Zhu, M., Zhang, J., and Roe, P. (2017). An intelligent system for estimating frog community calling activity and species richness. Ecol. Indic. 82, 13–22. doi: 10.1016/j.ecolind.2017.06.015
Keywords: ecoacoustics, open data, open science, monitoring, conservation, standards
Citation: Vella K, Capel T, Gonzalez A, Truskinger A, Fuller S and Roe P (2022) Key Issues for Realizing Open Ecoacoustic Monitoring in Australia. Front. Ecol. Evol. 9:809576. doi: 10.3389/fevo.2021.809576
Received: 05 November 2021; Accepted: 20 December 2021;
Published: 14 January 2022.
Edited by:
Brett K. Sandercock, Norwegian Institute for Nature Research (NINA), NorwayReviewed by:
Jake L. Snaddon, University of Southampton, United KingdomGuillaume Dutilleux, Norwegian University of Science and Technology, Norway
Copyright © 2022 Vella, Capel, Gonzalez, Truskinger, Fuller and Roe. This is an open-access article distributed under the terms of the Creative Commons Attribution License (CC BY). The use, distribution or reproduction in other forums is permitted, provided the original author(s) and the copyright owner(s) are credited and that the original publication in this journal is cited, in accordance with accepted academic practice. No use, distribution or reproduction is permitted which does not comply with these terms.
*Correspondence: Kellie Vella, a2VsbGllLnZlbGxhQHF1dC5lZHUuYXU=