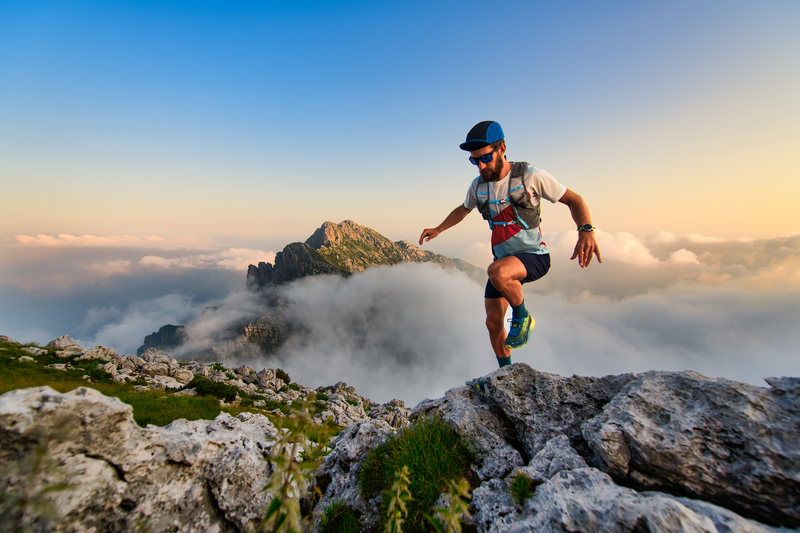
94% of researchers rate our articles as excellent or good
Learn more about the work of our research integrity team to safeguard the quality of each article we publish.
Find out more
ORIGINAL RESEARCH article
Front. Ecol. Evol. , 20 December 2021
Sec. Models in Ecology and Evolution
Volume 9 - 2021 | https://doi.org/10.3389/fevo.2021.802300
This article is part of the Research Topic Current Thoughts on the Brain-Computer Analogy - All Metaphors Are Wrong, But Some Are Useful View all 14 articles
Ordinary computing machines prohibit self-reference because it leads to logical inconsistencies and undecidability. In contrast, the human mind can understand self-referential statements without necessitating physically impossible brain states. Why can the brain make sense of self-reference? Here, we address this question by defining the Strange Loop Model, which features causal feedback between two brain modules, and circumvents the paradoxes of self-reference and negation by unfolding the inconsistency in time. We also argue that the metastable dynamics of the brain inhibit and terminate unhalting inferences. Finally, we show that the representation of logical inconsistencies in the Strange Loop Model leads to causal incongruence between brain subsystems in Integrated Information Theory.
Are brains like computers? Can technological metaphors provide satisfactory explanations for the complexity of human brains (and brains in general)? Before electronic computers became a reality, some versions of the previous questions had always been there. In the seventeenth century, the development of mechanical clocks and later on mechanical automata led to questions with far-reaching philosophical implications, such as the possibility of creating a mechanical human and an artificial mind (by René Descartes and others Wood, 2002). Later, brains and machines were compared to electric batteries (since it became clear that electricity was involved in brain processes), and early works by visionaries such as Alfred Smee represented brains and the activity of thinking in terms of networks of connected batteries (Smee, 1850). Other network-level metaphors of the brain such as telegraphs and telephone webs replaced the old ones, until the metaphor of the computer prevailed in the 1950s (Cobb, 2020).
The computer was apparently the right metaphor: It could store large amounts of data, manipulate them and perform complex input-output tasks that involved information processing. Additionally, the new wave of computing machines provided an appropriate technological context to simulate logical elements similar to those present in nervous systems. Theoretical developments within mathematical biology by McCulloch and Pitts (1943) revealed one first major result: The units of cognition—neurons—could be described with a formal framework. Formal neurons were described in terms of threshold units, largely inspired by the state-of-the-art knowledge of real neurons (Rashevsky, 1960). Over the last decades, major quantitative advances have been obtained by combining neuron-inspired models with multilayer architecture (LeCun et al., 2015) and physics of neuromorphic computing (Indiveri and Liu, 2015; Markovi et al., 2020). These developments are largely grounded in early theories (Rumelhart et al., 1986; Fukushima, 1988) with novel hardware improvements and a massive use of training data.
Despite the obvious success of computing and information technology, we are still far from the dream of building or simulating a truly intelligent system. To begin with, computers and their abstract representation in terms of Turing machines are highly modular, programmable and sequential (Arbib, 2012) (see Figure 1). Instead, neural systems are the result of evolutionary tinkering and selection that favored exploiting redundancy and parallelism (Allman, 1999; Martinez and Sprecher, 2020). That does not prohibit the existence of interesting links that help make sense of brain in terms of Turing machines: Many functional responses of brains are essentially sequential in nature, despite the highly parallel integration that feeds serial (and slow) cognitive task production (Zylberberg et al., 2011). Yet, the most remarkable departure of brains from computers is probably the presence of re-entrant circuits, i.e., the recursive exchange of signals across multiple, parallel and reciprocal connections (Edelman, 1992). Indeed, some authors have posited that closed feedback loops are crucial for conscious experience (Hofstadter, 1979; Oizumi et al., 2014). Are closed feedback loops the key for a formal differentiation between brains and computers? Closed feedback loops can allow for self-reference (Grim, 1993), and the human brain is capable of self-referential inference. So this begs the question: Why can the brain make sense of self-reference, whereas a computer can't?
Figure 1. Computer vs. brain architecture. A topological analysis of (A) computer chips and (B) brains (visual cortex organization) reveals fundamental dissimilarities. These include the strict modular organization of the former contrasted with the highly parallel, integrated architecture of the latter. The circuits responsible for higher-order cognitive brain tasks display re-entrant feedback loops that are absent on the in-silico counterparts (compare with Figure 2). Image adapted from Jonas and Kording (2017).
We address this question by considering paradoxes of self-reference and negation (Prokopenko et al., 2019). Studies in logic, linguistics, and general philosophy for many centuries have illustrated that when statements negatively refer to their own features, contradictions follow in short order. This is made clear from sentences such as:
Taking this sentence at its word—supposing it to be true—we find out it is false. However, taking it to be false, we are forced to conclude that it is true. When we assign truth values to sentences, we classically assume that truth and falsity are mutually exclusive and exhaustive, yet self-referential sentences appear to have over-determined truth values (Priest, 2006, pp. 14–15): We are obliged to evaluate them simultaneously as true and false, a contradiction. This may compel the logician to use formal languages that block such self-referential constructions to preserve their consistency at the cost of limiting their expressiveness. Such a pursuit of consistency is perhaps well-motivated in purely formal settings such as mathematics, but self-reference is readily available within natural language, and human minds are capable of formulating and thinking about self-referential paradoxes and becoming aware of their inconsistency.
Computers are incapable of resolving a paradox such as sentence 1—they get caught in endless loops—, whereas the brain can “reason” about this paradox. Let us examine the latter statement by bringing forward some basic facts about the workings of the brain. In the ordinary course of experience, our state of mind may possess many subtle and composite features, but we only ever occupy one such mental state at a time: There are no “superpositions” of mental states. Furthermore, if we take mental states to be somehow derivative of brain states (by whatever account of the emergence of consciousness one prefers), the deterministic or unitary evolution of physical systems given by our best physical theories suggests that our brains only ever occupy a single physical state.1 Whatever the mechanism responsible for the emergence of mental states from brain states is, surely the brain state that grounds the awareness of some fact is different from the brain state that grounds the awareness of its negation. Thus, occupying a mental state corresponding to awareness of a contradiction would seem to be a physical impossibility par excellence insofar as it would necessitate one's brain to be in two distinct states at once. Yet, upon interpreting the sentence 1, the reader comes to think about a self-referential statement and understand its contradictory nature, and so the cognitive processing of self-referential statements is clearly not a physical impossibility (nor do they get stuck in an unhalting cycle of thoughts one might expect of a machine tasked with deciding the truth value of such a sentence). How is this possible?
In this paper, we address this question by constructing a high-level model of the brain, termed a Strange Loop Model (Section 2), from which we conclude that:
1. The brain makes sense of self-reference by spreading out inconsistent truth values in time, thereby avoiding physically impossible states (Section 3.1).
2. The representation of logical inconsistencies in the brain leads to causal incongruence between brain subsystems (Section 3.3).
3. The metastable dynamics of the brain and its interactions with external stimuli inhibit and terminate unhalting inferences (Section 3.4).
Statement 1 says that the brain represents and processes self-referential sentences by treating their truth values as dynamical quantities. It follows that the resulting contradictions are unfolded in time, and thus do not require physically impossible brain states. Statement 2 describes how this “unfolding” works: Different parts of the brain yield disagreeing predictions about the brain's future states, and this disagreement is made apparent by analyzing the causal feedback between these parts. This disagreement is known in Integrated Information Theory (IIT) as incongruence (Albantakis and Tononi, 2019). This causal feedback is not encountered in Turing machines because they are feed-forward systems. Statement 3 claims that the brain does not succumb to halting problems when processing statements whose truth values are undecidable, because the metastable nature of brain dynamics precludes falling into lock-in states (Tognoli and Kelso, 2014).
This paper is structured as follows. We present the Strange Loop Model (SLM) of the brain (Section 2), and we use it to represent self-referential inferences in the brain (Section 3). Finally we conclude and discuss further directions (Section 4).
Here we present a high-level model of the brain by describing it as a discrete dynamical system (Section 2.1), partitioning it into functionally distinct modules (Section 2.2), and investigating their causal structure (Section 2.3). The name originates from Hofstadter (1979, 2007): Strange loops arise when, by moving only upwards (or downwards) in a hierarchy, one encounters oneself at the same place where one started.
Here we describe the brain as a discrete dynamical network of connectomic units (Sporns et al., 2005). We consider that n such units (indexed i = 1, …, n), evolving in discrete time t ∈ ℤ, and denote the state of unit i at time t by , where Σi is a finite state space. The state of the “brain” in the SLM at time t is denoted
The dynamics of such a system are given by a transition function so that and we denote ith component of by .
We consider a probability distribution p on Σ. For any z ∈ Σi, the conditional probability (also denoted p) is defined as
We suppose that all units are conditionally independent at any given time t ∈ ℤ, so they satisfy:
Additionally, we suppose that the future state of the brain depends only on the immediately preceding state (Markovianity), so that if t1 < t2 < ⋯ < T, the joint probability distribution factors as
With this setup one may use the intervention calculus from probabilistic causal modeling (e.g., as elaborated by Pearl, 2009) to understand how connectomic units causally influence each other. Following the exposition in Krohn and Ostwald (2017), given any two subsystems X, Y ⊆ B, one defines the effect probability pe, the joint cause-effect probability pce, and the cause probability pc to be:
where q(Yt−1) is the uniform distribution over the state space of Y. The distribution indicates the extent to which the current state of Y is an effect caused the previous state of X. Likewise, indicates the extent to which the previous state of Y was a cause of the current state of X.
The brain carries out a wide array of distinct, though integrated processes. While it is difficult to list and classify all of them, they may be roughly partitioned into three general interconnected categories: (i) pre-conscious processes, (ii) conscious processes, and (iii) post-conscious processes.
Pre-conscious processes are those which occur independent of conscious experience. The activity of the autonomic nervous system is paradigmatic of this category. Though extremely important for sustaining life, these functions are somewhat irrelevant to our considerations and shall hence be ignored in what follows.
Conscious processes are those which directly give rise to conscious experience; that is, they govern the dynamics of the neural correlates of consciousness, and include those responsible for perception, the categorical discrimination thereof, awareness, and short-term memory recall, among other things. They are not to be conflated with the first-person subjective conscious experiences to which these correlates are thought to somehow give rise. At the physiological level, all we are concerned with are the neural correlates of conscious experience and awareness; we are agnostic as to how the mental states are determined by these correlates, and therefore do not commit to any view about the origins of consciousness as such.
Post-conscious processes are those which are not the primary basis for conscious experience, but still depend on the correlates of consciousness such as language processing and inference-making. This class of brain functions is roughly equivalent to cognitive processes2.
Each of these classes of brain processes has a reasonably well-defined collection of physiological regions in the brain which carry them out. Hence it is possible for us to conceptually partition the brain into three physical “modules.” The important feature of these modules is that they are deeply interconnected. While it is hard to cleanly demarcate their physiological boundaries, what is important for our purposes is not how to carve up the brain into these modules, but the causal relations between them.
In the SLM (cf. Section 2.1), we shall denote the “consciousness” module by XCon ⊆ B and the individual connectomic units that compose it by {xi}. Likewise, we shall denote the “cognition” module by YCog ⊆ B and the connectomic units that compose it by {yi}. The region of the brain that is relevant for our purposes is the joint system XCon ∪ YCog.
We now argue that the brain modules XCon and YCog mutually exhibit causal feedback.
To see that XCon causally influences YCog, note that cognitive tasks are like computational tasks (broadly construed) which take as their inputs the correlates of consciousness. For instance, learning is a cognitive process that is informed by sensory stimuli. Likewise, language processing is a cognitive process that begins with a more abstract input of which the cognizing subject is usually consciously aware. More generally, changing what a person perceives or is conscious of affects how they make sense of their perceptions and what sorts of inferences they will draw.
What does the causal relation from XCon to YCog look like? It is known that a single neuron may participate in bringing about many sorts of perceptions and experiences, and many different neuronal states may correspond to one and the same perceptual experience (as there is great degeneracy). Hence, one cannot easily reduce a correlate of consciousness to an arrangement of neurons. That is, the correlates of consciousness are not identical to the state of XCon—they are only determined by XCon. More specifically, the intrinsic network of causal influences within XCon determines these neural correlates (see Tononi and Edelman, 1998; Edelman, 2005; Park and Friston, 2013 for discussion).3 In order for cognition to take the correlates of consciousness as inputs, the system YCog must be connected to system XCon in such a way that the internal causal structure of XCon is “read off” of its state and encoded directly into the states of the neurons of YCog, which must encode features of the probability distributions pe, pce, and pc of the subsystem XCon. Since we shall establish that there are causal relations in both directions, to prevent circularity, we suppose that YCog represents the intrinsic causal structure of XCon as it appears when marginalized to XCon (i.e., ignoring correlations with YCog). Determining exactly how this translation could be carried out would require a full account of the emergence of conscious experience from the relevant causal information which we do not have. However, one may view the units of YCog as “simulating” the intrinsic causal structure of XCon, and then carrying out an effective computing procedure on this simulation—this simulation could be modeled with ideas from hierarchical predictive processing which adopts a similar organizational structuring of the brain (cf. Friston, 2005; Friston and Kiebel, 2009; Clark, 2013). In summary, the causal relation XCon → YCog is highly non-trivial.
What does the causal relation from YCog to XCon look like? On its own, the system XCon gives rise to the moment-by-moment passive perceptions present in the thinking subject's conscious experience. However, the content of conscious experience—at least for humans—is not merely a passive stream of perception; there is further underlying semantic content within these perceptions of which we come to be aware by carrying out cognitive tasks. While our perceptual apparatus may be capable of carrying out discrimination tasks to categorize our perceptions (e.g., such that we may become aware of the presence of “pain” or “blue” and so on within a given experience), we also come to be consciously aware of much richer structural and abstract features as well. Deprived of all sensory input, the mathematician may still prove complex theorems structured by a sophisticated underlying mathematical grammar and logic, but only if they are consciously aware that they are doing so. To the extent that the thinking subject may be conscious of the outcomes of their cognition—which they certainly are in many cases—we see that there must exist some non-trivial causal relation between YCog and XCon in which the former causally influences the latter.
More specifically, acts of cognition may change the content of conscious experience such that we may acquire understanding of our perceptions, for instance by giving them grammatical structure (over and above merely discriminating qualia), or by carrying out introspection or higher inference-making. It is through this process that one may go from a state of mind of the form “it is the case that ϕ” to the state of mind “I know that it is the case that ϕ.” Likewise, it is through this process that one may go from the state of mind that “it is the case that ϕ and ϕ → ψ” to the state of mind “it is the case that ψ” (via inference by modus ponens). In short, the outcomes of cognitive processes are re-integrated back into the correlates of consciousness. This causal feedback via simulation and re-integration between modules in illustrated in Figure 2.
Figure 2. The causal relations XCon → YCog needed to simulate perceptions for inference-making, and YCog → XCon manifest in the awareness of the outcome of cognitive processing.
We have established that cognition causally influences the content of conscious experience, and vice versa. This is not to say, however, that cognition is itself “perceived.” In everyday life, the content of our experience forms the basis of some cognitive inference we may make and we become aware of the outcome of this inference, but we never perceive the inference itself. Indeed, even when one is proving mathematical theorems, at most one is aware of what cognitive rules they are applying when carrying out a deduction: they do not, however, experience the application of these rules as such. This illustrates that, while we argue that cognition causally influences the course of conscious experience in a very strong way, it is not itself directly responsible for conscious experience; the neuronal basis for cognition is not itself populated with correlates of consciousness, it merely interacts with these correlates in a reentrant manner. In this sense, we may faithfully view the cognitive module YCog as implementing feed-forward computing procedures, e.g., through a neural network that is reintegrated with XCon (such that inference making in its entirety is not merely a computing procedure).
Formally, since both XCon and YCog causally influence one another in a highly non-trivial manner, we expect that
Thus, the simulation of XCon encoded in YCog will generally not be a faithful predictor of the future behavior of XCon, since it ignores its own causal influence on this behavior. This is the reason we suppose that YCog simulates the causal structure of XCon as marginalized to XCon. In Box 1 we provide a concrete realization of the SLM presented above model, as well as its application to self-reference.
Box 1. A concrete realization of the Strange Loop Model
To instantiate the SLM, suppose first that a mental state amounts to the awareness of some sentence in a formal language L. Such sentences carry an internal time index τ: at physical time t, one may occupy a mental state of remembering some sentence ϕ at an earlier time (i.e., τ < t), they may anticipate being aware of ϕ in the future (i.e., τ > t), or they may be aware of ϕ as a feature of the present experience (i.e., τ = t). We suppose that every pair (ϕ, τ) is represented by a unit of YCog. The mental state determined by the state of XCon is simulated by the elements of YCog via an injective map S : ΣX → {(ϕ, τ)|ϕ ∈ L, τ ∈ ℤ} where ΣX is the state space of XCon. That is, S takes the state of XCon to the unit of YCog that represents the corresponding mental state. The state of each unit y = (ϕ, τ) ∈ YCog at time t is given by yt = (at(y), st(y)) ∈ {0, 1} × {0, 1}, where at(y) = 1 if the thinking subject is consciously aware of y at time t, and it is 0 otherwise, and st(y) = 1 if the thinking subject assigns truth to ϕ at time τ (i.e., if they think ϕ was/is/will be true at time τ), and it is 0 otherwise. The state of YCog at time t is determined by:
That is, to be aware of (ϕ, τ) at time t is to think it to be true, and to think ϕ is false is to be aware of the truth of ¬ϕ. The state of YCog at time t + 1 is determined by the application of some inferential mechanism by YCog. If the thinking subject applies a rule of inference of the form {σ1, …, σk}⊢ψ, at(y) is updated so that one is only presently aware of ψ, namely at+1(ψ, t + 1) = 1, and at+1(ϕ, τ) = 0 for ϕ ≠ ψ and any τ. The transition rule for st is
and st+1(ϕ, τ) = st(ϕ, τ) for all τ when ϕ is independent of ψ, and st+1(ξ, τ) = st(ξ, τ) for all τ ≠ t + 1 and any ξ. Sentences containing ψ have their truth values adjusted according with the change in the truth value of ψ, for example st+1(¬ψ, t + 1) = 1 − st+1(ψ, t + 1) and st+1(ϕ ∧ ψ, t + 1) = st+1(ϕ, t + 1) · st+1(ψ, t + 1) and so on. We do not fully specify the transition rule for XCon, but we require that it be such that after such an inference, .
The self-referential paradox arises when one may assert that for t1 ≠ t2. But, as shown in Section 3.1, this scenario is not challenging to understand; these are two different nodes of , and there is no consistency requirement preventing this as a value assignment. Even if one imposes consistency conditions at equal times, since these are unequal-time units, such conditions need not prohibit this behavior.
Here we use the SLM to investigate how to make sense of self-reference by unfolding the inconsistency in time (Section 3.1) and provide some clarifying remarks (Section 3.2). Then we show how logical inconsistency is transformed to incongruence (Section 3.3), and argue that the brain does not get caught in endless loops (Section 3.4).
We now analyze how the intrinsic thought process of an agent carrying out a self-referential deduction as given by the Inclosure Schema (Box 2) would appear in the dynamical behavior of the joint system XCon ∪ YCog. In formal logic, a deduction in a given formal system is a sequence of grammatically well-formed strings of symbols such that each string is either an instance of an assumed axiom or premise, or is the result of the application of a permitted rule of inference to previous lines in the sequence. If one views a deduction as a dynamical time-dependent thought process in which each line in the deduction corresponds to some fact about which the thinking subject is aware, the sequential ordering of the lines of the deduction may be interpreted as the time ordering of a series of mental states (and thus, a constraint of the compatible dynamics of the underlying brain states).
Box 2. Diagonalization, self-reference and paradoxes
While self-reference and its paradoxical consequences arise in a wide range of settings, the construction of the self-referential statement leading toward contradiction typically has a standard form, termed the Inclosure Schema; cf. Priest, 2002, Chapter 9.4). At a higher level of abstraction, this may be viewed as an instance of Lawvere's Theorem (Yanofsky, 2003; Lawvere, 2006; Roberts, 2021).
In plain language, the relevant actresses of the Inclosure Schema are the following. A predicate is a property that elements of a set may possess, and we identify the predicate with its extension, i.e., with the set of elements that instantiate it. For example, the predicate “odd” of the set of natural numbers is the set {1, 3, 5, 7, 9, …}. If a set x has property P, we write P(x), meaning that P(x) is true, i.e., x is in the extension of P. We will consider the collection of all sets V, and a function Δ : V → V.
More formally, let φ and ψ denote two predicates that may apply to arbitrary sets (where “set” is meant in the sense of natural language, which is more expressive than formal set theory at the cost of being inconsistent), and let Δ be a function on sets. Then self-reference occurs when:
1. Eφ = {y|φ(y)} is a set, and ψ(Eφ)
2. If x ⊆ Eφ such that ψ(x), then Δ(x) ∉ x and Δ(x) ∈ Eφ
Statement 1 says that the extension of the predicate φ is a set and is called Eφ, and that Eφ has property ψ. Statement 2 defines the features of Δ, namely Δ takes sets with property ψ whose elements all have property φ to sets whose elements have property φ but are not contained in the original set. The contradiction associated with self-reference appears when one applies condition 2 to the maximal subset, namely, Eφ itself, from which it follows that Δ(Eφ) ∈ Eφ and Δ(Eφ) ∉ Eφ; a contradiction.
Let us see this argument in action by considering Russell's paradox. In naïve set theory, the extension of any predicate is a set. Russell's paradox is as follows: suppose X is the set of all sets that do not contain themselves. Then if X ∈ X, by definition it follows that X ∉ X. However, if X ∉ X, then since X is the set of all sets that do not contain themselves, we find X ∈ X; a contradiction. On the Inclosure Schema this paradox may be recast as follows. First, ψ is the predicate “is a set,” φ is the predicate “does not contain itself,” and Δ is defined by Δ(x) = {y ∈ x|y ∉ y}, i.e., it's image is the set of all sets in x that do not contain themselves. Since ψ is a predicate in naïve set theory, 1 is true and asserts that Eφ exists and is the notorious “set of all sets that do not contain themselves.” Then if x is a set, clearly Δ(x) ∈ Eφ. Likewise, if Δ(x) ∈ Δ(x), then by definition of Δ, Δ(x) ∉ Δ(x) and so we must conclude Δ(x) ∉ x. Thus 2 is also satisfied. But then setting x = Eφ, this implies simultaneously that Δ(Eφ) ∈ Eφ and Δ(Eφ) ∉ Eφ; a contradiction. This contradiction historically called for the reformulation set theory and was one of the many factors leading to modern-day ZF axiomatic set theory. All other famous self-reference paradoxes may be articulated using this Inclosure Schema.
Given some statement ϕ, to say that an agent is aware of ϕ at time t is to say that the physical state of grounds the mental state of being aware of ϕ. One can actively perceive ϕ by occupying such a mental state, or one can remember having perceived ϕ at a previous time. Thus, there is an internal time index τ ≤ t that tracks the time at which ϕ was perceived that may differ from the time index of the state of XCon. If we denote that class of all brain states that give rise to this mental state by [ϕ], and index the time at which ϕ is thought to be (or have been) perceived by [ϕτ], we thus have if the thinking subject is actively thinking about ϕ, and for τ < t if they are recalling having thought about ϕ previously.
If ϕ and ψ are two formulas that are not logically equivalent to one another, one might suppose that [ϕτ] ∩ [ψτ] = ∅. This very general claim may be objected to in principle by noting, for instance, that if ϕ and ψ are sufficiently complex, the thinking subject may not always be immediately aware of their logical (in)equivalence4. Nevertheless, it should be agreeable that there are no brain states that are simultaneously neural correlates of the awareness of ϕ and also neural correlates of the awareness of ¬ϕ. This weaker hypothesis is all we shall require. Then, if the thinking agent carries out a deductive inference whose sequential lines are denoted {ϕn}, this corresponds to their brain undergoing a dynamical evolution of the form:
where τ : ℤ → ℤ satisfies τ(t) ≤ t. While the individual lines of a deduction correspond to mental states (and thus restricted classes of brain states), the axioms and rules of inference from which subsequent lines are produced do not reflect processes of which one is consciously aware during such a thought process. Rather, they reflect the cognitive rules that the thinking agent's brain may apply to the content of their experience in order to bring about their subsequent mental states. In this way, the axioms and rules of inference that enable one to formalize a given deduction correspond in the underlying thought process to processes implementations of cognitive processes via YCog (see Box 1 for a concrete realization thereof).
To illustrate this, let us consider a simple example. Suppose one sees a green apple before them. This perception, and the discrimination of various features of this perception are grounded in neural correlates that reside physiologically in the brain module XCon at the present time t. Suppose, subsequently (say, at time t + 1), that one remembers from their past experiences that essentially all green apples have a sour taste. (Of course, the inductive formation of such a generalized belief from past memories is non-trivial, but it nevertheless happens.) This association, then, of sour flavor with green apples in general is something about which the thinking subject becomes consciously aware, and hence forms part of their conscious experience. Therefore, it is likewise encoded in the neural correlates of consciousness present in XCon at time t + 1. From these two perceptions, the thinking subject may apply modus ponens to conclude that the apple they saw at time t would likely have had a sour taste were they to eat it. The general rule of modus ponens, however, is not something of which one has direct perception when it is being implemented; making such inferences is a higher cognitive process. The implementation of modus ponens, therefore, is a process carried out by the brain module YCog. Importantly, once this inference has been carried out, the subject becomes aware of its outcome. Namely, at a subsequent time (say, t + 2), they become consciously aware that, had they eaten the apple, it would likely have tasted sour. This is the general manner in which deduction may be realized as thought processes implemented within our brain model.
We now apply this perspective to the linguistic processing of self-referential statements via the Inclosure Schema (see Box 2). The idea is to distinguish between the abstract logical results and the thought processes obtained when a thinking subject confronts an instance of self-reference and thinks about it over a finite period of time. Logically speaking, the contradiction arising from a diagonalization argument is absolute; we do not contest this. However, when we infer this contradiction—i.e., when the dynamical behavior of a subject's brain implements the thought process that yields this contradiction—using diagonalization, we do so in two temporally separate parts; first, we prove that Δ(x) ∉ x and conclude that Δ(Eφ) ∉ Eφ. Then, at a later time, we conclude that Δ(Eφ) ∈ Eφ. The contradiction arises when we remember at a third time that we had proven both of these two facts separately.
Let us look at Tarski's paradox to see this play out concretely, following the exposition by Priest (2002). To begin, let T be a “truth” predicate on sentences, i.e., for any sentence x, T(x) is true if and only if x is true (this is called Tarski's T-schema). Let ψ denote definability such that ψ(X) is true for any set of sentences X just in case there exists a sentence x which defines X as a set (of sentences). If X is any definable set of sentences, let Δ(X) = α where α = 〈α ∉ X〉 (here 〈·〉 is used to denote the proper name of a sentence). That is, Δ(X) is the sentence α which expresses that α is not an element of the set of sentences X. Clearly, α is self-referential. If an agent thinks about the T-schema, their thought process might look like the following. First, one supposes that the totality of all true sentences exists and is definable, that is, that Tr : = {x∣T(x)} is a set that may be defined by some sentence. If X is definable (whence ψ(X) is true) and if X ⊆ Tr, we have in the temporal framework described:
Let us now look at the brain states that could in principle produce the mental states associated with each line of this deduction. We may rewrite the above inference as follows:
To prove a contradiction in time in a manner that could require a physically impossible brain state, one would need to show that and for a single t. This does not happen. In this way, if we want to model deductive inferences as processes carried out by a physical systems such as the brain which evolves in time, we see that the contradictions appear not directly, but spread out in time and then recalled, and so they may be implemented by a machine such as the brain that operates in time (Figure 3). In particular, we do not encounter the fractal picture given in Grim et al. (1993).
Figure 3. Unfolding self-reference in time can be imagined as unfolding a circle many-times packed into a corkscrew, where the time dimension corresponds to the long dimension of the corkscrew. Equivalently, it can be imagined as the evolution of circularly polarised light.
Moreover, because it is possible to have and at different times t ≠ t′, we see that the brain has on this model sufficient expressive power to treat truth values as dynamically changing quantities. This may be contrasted with Turing machines tasked with deciding truth values; the state of such a machine may evolve in time, but the truth value it aims to decide is static.
Let us make a few remarks on the conclusions reached so far. We are not denying the logical contradiction that appears in the above deduction. Indeed, what we have done here amounts to a temporal version of what (Priest, 2002) calls parameterization; it is a standard approach to avoid paradoxes, and in general, any contradiction that is avoided by parameterization will reappear at a higher level again when one analyzes the parameterized formalism. However, this is irrelevant to our aims: what we have shown is that an inference-making device that has a register that expresses its state of deduction in time (while some auxiliary system carries out further inference-making tasks leading to eventual update of the register) can effectively model contradictory scenarios without existing in a contradictory state itself. That is, there is never an instant where such a system need occupy two different physical states simultaneously.
Extending this to our model of the brain, the “inference” column label could be replaced with “the thought of which the conscious agent is aware” at each given time, while the “rule” column label could just as well be interpreted as “the cognitive process being carried out in the intermediate time window.” In this way, we have a rough picture for how the brain could physically model the contradictions that arise from self-reference paradoxes (noting that the above proof for the contradiction in Tarski's paradox is of the generic diagonalization form) without itself being in any strange superposition of disagreeing physical configurations.
What makes this temporal parameterization technique useful is that while in a purely logical setting, the relation between subsequent lines in a deduction is strictly a logical one (with no temporality and so forth), when represented on a physical system, is no longer an abstractly logic relation, but is instead a causal relation indicating an interaction between these two brain modules we have discussed. In particular, it is a causal relation which requires an intermediate physical process to commence and terminate. Hence, there is an intermittent time, and so the contradiction may be “stretched out” in time in the appropriate sense. (This is analogous to the Kantian view of time as a means for the thinking subject to experience contradictory perceptions without an actual contradiction obtaining Kant, 1998, A32/B48).
We now apply the IIT formalism (see Box 3) to the SLM, and show how the logical inconsistency of self-referential paradoxes is transformed to incongruence.
Box 3. Integrated Information Theory
Integrated Information Theory (IIT; see Oizumi et al., 2014; Tononi and Koch, 2015; Tononi et al., 2016) is a framework that seeks to provide a constructive account of the origins of conscious experience by describing it as an emergent feature of causally integrated dynamical systems such as the brain. IIT begins by articulating those features of conscious experience that one might take to be constitutive, and then identifies features of the causal structure of a dynamical system that qualitatively realize these features (in a manner that can be made quantifiably precise via informational measures). A model of the IIT formalism is a dynamical system X (such as the SLM) together with all of the probabilities of the form . From the causal probabilities defined in Equation (4), the IIT formalism defines measures to quantify the extent to which a subsystem S ⊆ X cannot be causally reduced, e.g., to a pair of subsystems G and H with S = G ∪ H and the extent to which every such S is causally integrated.
A state of a subsystem at some time is irreducible if and only if the probabilities that characterize its intrinsic causal structure cannot be exactly recovered by partitioning it into subsystems. Irreducibility is quantified using an informational measure; those subsystems of X that realize the maximum of this measure for the system X are called maximally irreducible. There are generally many different maximally irreducible subsystems.
According to IIT, only those subsystems that are maximally irreducible at a given moment contribute to consciousness at that moment, forming the instantaneous correlates of consciousness. The manner in which a maximally irreducible subsystem contributes to consciousness is dictated by its causal probabilities which populate points in a supposed space of qualia. The conscious experience realized by a physical substrate (a human brain or otherwise) is a byproduct of that substrate's maximally irreducible intrinsic causal structure. Here we do not assess the plausibility of IIT as a theory of consciousness; rather, we note that our SLM can be recast within the IIT formalism straightforwardly.
First observe that since the correlates of consciousness were taken to reside in XCon, it is reasonable to suppose that for any subsystem of the brain Z ⊆ B, if Z is maximally irreducible while in some state Zt, it must be the case that Z ∩ XCon ≠ ∅. In most cases, Z will simply be a subsystem of XCon. However, from Equation (5), there will be some irreducible subsystems that overlap with YCog as well. In particular, XCon ∪ YCog is expected to be maximally irreducible.
Incongruence in IIT is defined as follows. For any system S, given a pair of subsystems G, H ⊆ S, G and H are incongruent if they make differing predictions about the past or future behavior of some particular node z ∈ S (see Haun and Tononi, 2019; Albantakis and Tononi, 2019, p. 5). This occurs, for instance, if p(zt+1|Gt) ≠ p(zt+1|Ht). When self-referential inferences are made, if we suppose ϕ is thought about at time t, ¬ϕ is thought about at t + 1, and ϕt ∧ ¬ϕt+1 is thought about at time t + 2, then it is because of the cognitive processes in YCog implemented at time t and t + 1 that this is the case. In particular, if we presently think some sentence is true, we expect that it will be true still at the next instant, so that
is large, while
is small. However, YCog implements a rule of inference in this transition, which causes to occur. During self-referential inferences, not only do two different subsystems disagree about the probabilities assigned to a particular node's future state (cf. Equation 5); rather, they assign essentially opposite probabilities to the future behavior of the subsystem spanned by all maximally irreducible subsystems. Hence, incongruence arises in a strong way.
Put differently, causal incongruence in IIT offers a precise sense in which the parts of a system fail to describe the whole of the system, namely, taken separately, the parts may disagree with one another about the descriptions they provide. In the SLM framework, this is exploited as a feature: it is this disagreement that enables the brain to represent contradictions in the requisite manner needed to make sense of self-referential statements.
We now argue that the cyclic behavior of the SLM, as described in Section 3.1, does not persist indefinitely (as it would for an unhalting Turing machine). When the thinking subject gets caught in a cognitive cycle of the same form, if their attention is drawn away from the cyclic inference at hand, the cycle will end. This is so because, as a thinking subject learns by repeating a task many times, they devote less and less attention and focus toward the task being learned (Kandel et al., 2013, Chapter 64). In the present context, this means that if the thinking subject cycles through the thought process associated with deriving disagreeing truth values for a self-referential statement, they will not get caught in a loop, but rather will pay less attention to the inference upon subsequent iterations. Since the brain actively monitors a large class of sensory stimuli and implements many cognitive processes in parallel, as this attention diminishes, the thinking subject is increasingly likely to refocus their attention elsewhere. In short, if attention is a resource, the architecture of the brain is such that the re-allocation of this resource inhibits the ensuing feedback and makes infinite inferential loops unstable.
This is analogous to binocular rivalry, where the subject's visual field is eventually changed, whence their visual sensations escape from flowing toward lock-in states (Hohwy et al., 2008; Clark, 2013), and to visual paradoxes, like the Necker cube (where two alternative possible attractors are present) or the recognition of ambiguous images (Inoue and Nakamoto, 1994; Kelso, 1995). This metastable behavior due to self-reference can also be found in gene networks, where the causal feedback associated with cross-regulatory interactions can be spread in time or space leading to interesting phenomena (Isalan, 2009).
In this work, we have constructed a high-level discrete dynamical model of the brain, termed the Strange Loop Model (SLM; Section 2), in order to describe inference-making, which uses causal feedback between conscious and cognitive processes. We have used the SLM to model self-reference and shown that logical inconsistencies unfold in time (Section 3.1), and hence the contradictions dissolve, as one never encounters inconsistent truth values simultaneously. Rather, one deduces at different times that a sentence has different truth values and then remembers having carried out both such deductions. This flexibility enables the human brain to model self-reference in a manner that is inaccessible to usual computing devices by construction. We have also applied the SLM within the context of IIT and shown that logical inconsistencies are transformed into incongruences (Section 3.3). Finally we have argued that, because the brain is receptive to a wide range of different stimuli, and because one devotes less attention to repetitive cognitive tasks as time passes, these cyclic inferences are unstable are thus terminated (Section 3.4).
The interaction between XCon and YCog via the described causal feedback enables the human mind to be aware of the outcomes of cognitive inferences, and likewise further cognize about such an awareness. Put differently, the causal feedback here described enables the thinking subject to be aware of their own cognitive processes, and to then make inferences about their own cognition. This situation is reminiscent of universality encountered in Turing machines, spin models and neural networks (De las Cuevas, 2020).
Finally, we may compare the SLM with a Turing machine or any other standard computing machine. Unlike an algorithm running in a Turing machine, the processing carried out by the SLM is not a deciding process, because it need not reach a static truth value of a variable. Moreover, the only relevant features of a Turing machine are its input–output functionality (that is, the formal language it recognizes Kozen, 1997), whereas the intrinsic causal structure of the brain is crucial. In this way, we conclude that the process carried out by the brain and the computer is different.
The original contributions presented in the study are included in the article/supplementary material, further inquiries can be directed to the corresponding author.
All authors listed have made a substantial, direct, and intellectual contribution to the work and approved it for publication.
PF is supported in part by funding from the Social Sciences and Humanities Research Council. RS thanks the Spanish Ministry of Economy and Competitiveness, grant PID2019-111680GB-I00, an AGAUR FI 2018 grant, and the Santa Fe Institute. GD acknowledges funding from the Austrian Science Fund (FWF) (START Prize Y-1261-N).
The authors declare that the research was conducted in the absence of any commercial or financial relationships that could be construed as a potential conflict of interest.
All claims expressed in this article are solely those of the authors and do not necessarily represent those of their affiliated organizations, or those of the publisher, the editors and the reviewers. Any product that may be evaluated in this article, or claim that may be made by its manufacturer, is not guaranteed or endorsed by the publisher.
We wish to thank Larissa Albantakis and Wolfram Hinzen for insightful discussions.
1. ^Since the brain is fundamentally a quantum system, this physical state could be in a superposition, but as Tegmark (2000) has shown, even neurons are sufficiently macroscopic systems that decoherence would likely prevent quantum effects from being relevant.
2. ^It is worth mentioning that there may be some cognitive processes which may be independent of conscious experience. We consider such processes to be pre-consicous ones, and thus irrelevant to our analysis.
3. ^While the dynamical evolution of the brain may be reduced to a description of its individual neurons, and while its intrinsic causal structure is grounded in the interactions of these neurons, the intrinsic causal structure is not robust against small changes to the network architecture. It is in this way that neural correlates of consciousness are not “reducible” to individual neurons.
4. ^One might consider, for instance, the classic example due to (Descartes, 1993, Meditation VI) of the chiliagon (a 1000-sided polygon). The thinking subject, it may be argued, uses an identical mental representation to depict a polygon with 1000 sides as one with only 999 sides. Such mental representations, then, would be given the same class of corresponding brain states, even if it is a logically different object. Hence, logical inequivalence is inadequate for individuating mental states (or brain states).
Albantakis, L., and Tononi, G. (2019). Causal composition: structural differences among dynamically equivalent systems. Entropy 21, 989. doi: 10.3390/e21100989
Clark, A. (2013). Whatever next? Predictive brains, situated agents, and the future of cognitive science. Behav. Brain Sci. 36, 181–204. doi: 10.1017/S0140525X12000477
Edelman, G. (1992). Bright Air, Brilliant Fire: On the Matter of the Mind. New York, NY: Basic Books.
Edelman, G. M. (2005). Wider Than the Sky: The Phenomenal Gift of Consciousness. New Haven, CT: Yale University Press.
Friston, K. (2005). A theory of cortical responses. Philos. Trans. R. Soc. B 360, 815–836. doi: 10.1098/rstb.2005.1622
Friston, K., and Kiebel, S. (2009). Predictive coding under the free-energy principle. Philos. Trans. R. Soc. B 364, 1211–1221. doi: 10.1098/rstb.2008.0300
Fukushima, K. (1988). Neocognitron: a hierarchical neural network capable of visual pattern recognition. Neural Netw. 1, 119–130. doi: 10.1016/0893-6080(88)90014-7
Grim, P. (1993). “Self-reference, chaos, and fuzzy logic,” in Integration of Fuzzy Logic and Chaos Theory. (Berlin; Heidelberg; New York, NY: Springer), 317–359.
Grim, P., Mar, G., Neiger, M., and St. Denis, P. (1993). Self-reference and paradox in two and three dimensions. Comput. Graphics 17, 609–612. doi: 10.1016/0097-8493(93)90013-Y
Haun, A., and Tononi, G. (2019). Why does space feel the way it does? Towards a principled account of spatial experience. Entropy 21, 1160. doi: 10.3390/e21121160
Hohwy, J., Roepstorff, A., and Friston, K. (2008). Predictive coding explains binocular rivalry: an epistemological review. Cognition 108, 687–701. doi: 10.1016/j.cognition.2008.05.010
Indiveri, G., and Liu, S. (2015). Memory and information processing in neuromorphic systems. Proc. IEEE 103:1379–1397. doi: 10.1109/JPROC.2015.2444094
Inoue, M., and Nakamoto, K. (1994). Dynamics of cognitive interpretations of a necker cube in a chaos neural network. Progr. Theor. Phys. 92, 501–508. doi: 10.1143/PTP.92.501
Isalan, M. (2009). Gene networks and liar paradoxes. Bioessays 31, 1110–1115. doi: 10.1002/bies.200900072
Jonas, E., and Kording, K. (2017). Could a neuroscientist understand a microprocessor? PLoS Comput. Biol. 13:e1005268. doi: 10.1371/journal.pcbi.1005268
Kandel, E. R., Schwartz, J. H., Jessell, T. M., Siegelbaum, S. A., and Hudspeth, A. J. (Eds.). (2013). Principles of Neural Science, 5th Edn. New York, NY: McGraw Hill Medical.
Kant, I. (1998). Critique of Pure Reason. The Cambridge Edition of the Works of Immanuel Kant. Cambridge: Cambridge University Press.
Kelso, J. S. (1995). Dynamic Patterns: The Self-Organization of Brain and Behavior. Cambridge, MA: MIT Press.
Krohn, S., and Ostwald, D. (2017). Computing integrated information. Neurosci. Consciousness 2017:nix017. doi: 10.1093/nc/nix017
Lawvere, F. W. (2006). “Diagonal arguments and cartesian closed categories,” in Reprints in Theory and Applications of Categories, Reprints Theory Appl. Categ. 1–13.
LeCun, Y., Bengio, Y., and Hinton, G. (2015). Deep learning. Nature 521, 436–444. doi: 10.1038/nature14539
Markovi, D., Mizrahi, A., Querlioz, D., and Grollier, J. (2020). Physics for neuromorphic computing. Nat. Rev. Phys. 2, 499–510. doi: 10.1038/s42254-020-0208-2
Martinez, P., and Sprecher, S. (2020). Of circuits and brains: the origin and diversification of neural architectures. Front. Ecol. Evolut. 8:82. doi: 10.3389/fevo.2020.00082
McCulloch, W., and Pitts, W. (1943). A logical calculus of the ideas immanent in nervous activity. Bull. Math. Biophys. 5, 115–133. doi: 10.1007/BF02478259
Oizumi, M., Albantakis, L., and Tononi, G. (2014). From the phenomenology to the mechanisms of consciousness: integrated information theory 3.0. PLoS Comput. Biol. 10:e1003588. doi: 10.1371/journal.pcbi.1003588
Park, H.-J., and Friston, K. (2013). Structural and functional brain networks: from connections to cognition. Science 342:1238411. doi: 10.1126/science.1238411
Pearl, J. (2009). Causality: Models, Reasoning, and Inference, 2nd Edn. Cambridge: Cambridge University Press.
Prokopenko, M., Harre, M., Lizier, J., Boschetti, F., Peppas, P., and Kauffman, S. (2019). Self-referential basis of undecidable dynamics: from the liar paradox and the halting problem to the edge of chaos. Phys. Life Rev. 31, 134–156. doi: 10.1016/j.plrev.2018.12.003
Rashevsky, N. (1960). Mathematical Biophysics: Physico-Mathematical Foundations of Biology, 3rd Edn. New York City, NY: Dover P. Inc.,.
Roberts, D. M. (2021). Substructural fixed-point theorems and the diagonal argument: theme and variations. arXiv:2110.00239.
Rumelhart, D., Hinton, G., and Williams, R. (1986). Learning representations by back-propagating errors. Nature 323, 533–536. doi: 10.1038/323533a0
Sporns, O., Tononi, G., and Ktter, R. (2005). The human connectome: a structural description of the human brain. PLoS Comput. Biol. 1:e42. doi: 10.1371/journal.pcbi.0010042
Tegmark, M. (2000). Importance of quantum decoherence in brain processes. Phys. Rev. E 61, 4194–4206. doi: 10.1103/PhysRevE.61.4194
Tognoli, E., and Kelso, J. A. S. (2014). The metastable brain. Neuron 81, 35–48. doi: 10.1016/j.neuron.2013.12.022
Tononi, G., Boly, M., Massimini, M., and Koch, C. (2016). Integrated information theory: from consciousness to its physical substrate. Nature Rev. Neurosci. 17, 450–461. doi: 10.1038/nrn.2016.44
Tononi, G., and Edelman, G. M. (1998). Consciousness and complexity. Science 282, 1846–1851. doi: 10.1126/science.282.5395.1846
Tononi, G., and Koch, C. (2015). Consciousness: here, there and everywhere? Philos. Trans. R. Soc. B 370:20140167. doi: 10.1098/rstb.2014.0167
Wood, G. (2002). Living Dolls: A Magical History of the Quest for Mechanical Life. London: Faber & Faber.
Yanofsky, N. S. (2003). A universal approach to self-referential paradoxes, incompleteness and fixed points. Bull. Symbolic Logic 9, 362–386. doi: 10.2178/bsl/1058448677
Keywords: self-reference, cognition, consciousness, computation, causal structure, integrated information theory
Citation: Fraser P, Solé R and De las Cuevas G (2021) Why Can the Brain (and Not a Computer) Make Sense of the Liar Paradox? Front. Ecol. Evol. 9:802300. doi: 10.3389/fevo.2021.802300
Received: 26 October 2021; Accepted: 25 November 2021;
Published: 20 December 2021.
Edited by:
Pedro Martinez, University of Barcelona, SpainReviewed by:
Eric Smith, The University of Texas at El Paso, United StatesCopyright © 2021 Fraser, Solé and De las Cuevas. This is an open-access article distributed under the terms of the Creative Commons Attribution License (CC BY). The use, distribution or reproduction in other forums is permitted, provided the original author(s) and the copyright owner(s) are credited and that the original publication in this journal is cited, in accordance with accepted academic practice. No use, distribution or reproduction is permitted which does not comply with these terms.
*Correspondence: Patrick Fraser, cC5mcmFzZXJAbWFpbC51dG9yb250by5jYQ==
Disclaimer: All claims expressed in this article are solely those of the authors and do not necessarily represent those of their affiliated organizations, or those of the publisher, the editors and the reviewers. Any product that may be evaluated in this article or claim that may be made by its manufacturer is not guaranteed or endorsed by the publisher.
Research integrity at Frontiers
Learn more about the work of our research integrity team to safeguard the quality of each article we publish.