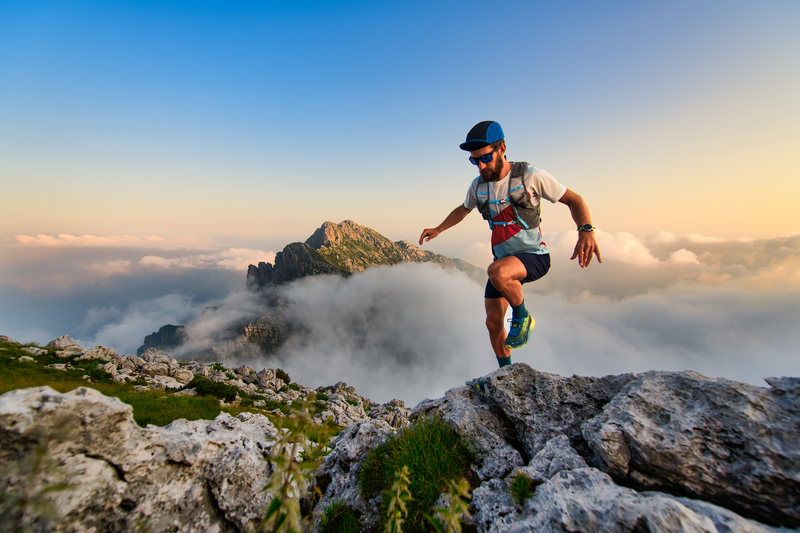
94% of researchers rate our articles as excellent or good
Learn more about the work of our research integrity team to safeguard the quality of each article we publish.
Find out more
ORIGINAL RESEARCH article
Front. Ecol. Evol. , 30 November 2021
Sec. Population, Community, and Ecosystem Dynamics
Volume 9 - 2021 | https://doi.org/10.3389/fevo.2021.783546
Accurate assessments of the patterns and drivers of livestock depredation by wild carnivores are vital for designing effective mitigation strategies to reduce human-wildlife conflict. Snow leopard’s (Panthera uncia) range extensively overlaps pastoralist land-use and livestock predation there is widely reported, but the ecological determinants of livestock consumption by snow leopards remain obscure. We investigated snow leopard dietary habits at seven sites across the Sanjiangyuan region of the Qinghai–Tibetan Plateau (QTP), an area central to the species’ global range. Snow leopard abundance, wild prey composition, and livestock density varied among those sites, thus allowing us to test the effects of various factors on snow leopard diet and livestock predation. Using DNA metabarcoding, we obtained highly resolved dietary data from 351 genetically verified snow leopard fecal samples. We then analyzed the prey preferences of snow leopards and examined ecological factors related to their livestock consumption. Across the sites, snow leopard prey was composed mainly of wild ungulates (mean = 81.5% of dietary sequences), particularly bharal (Pseudois nayaur), and supplemented with livestock (7.62%) and smaller mammals (marmots, pikas, mice; 10.7%). Snow leopards showed a strong preference for bharal, relative to livestock, based on their densities. Interestingly, both proportional and total livestock consumption by snow leopards increased linearly with local livestock biomass, but not with livestock density. That, together with a slight negative relationship with bharal density, supports apparent facilitation between wild and domestic prey. We also found a significant positive correlation between population densities of snow leopard and bharal, yet those densities showed slight negative relationships with livestock density. Our results highlight the importance of sufficient wild ungulate abundance to the conservation of viable snow leopard populations. Additionally, livestock protection is critically needed to reduce losses to snow leopard depredation, especially where local livestock abundances are high.
The survival of large carnivores is threatened globally due to conflicts with humans (Ripple et al., 2014; Wolf and Ripple, 2017). The high energy demands of those predators require large prey populations and home ranges that often unavoidably overlap with human-occupied land. In pastoral societies where humans and wild carnivores coexist, carnivore predation on domestic livestock is widespread (Mishra, 1997; Janeiro-Otero et al., 2020), and that can result in substantial economic costs that ultimately precipitate most human–carnivore conflicts. Such conflicts often lead to negative attitudes toward carnivores and even retaliatory killings that threaten carnivore species survival (Kissui, 2008). Effective mitigation of these conflicts relies on accurate identification of predation patterns and their underlying ecological mechanisms, which can then be used to devise informed management strategies that both resolve conflicts and promote sustainable coexistence between human and carnivores (van Eeden et al., 2018; Wilkinson et al., 2020).
The snow leopard (Panthera uncia) is an iconic large felid inhabiting high elevations in the mountains of Central Asia, the Qinghai–Tibetan Plateau (QTP), and the Himalayas. Decreasing snow leopard populations are due mainly to habitat loss and fragmentation, increasing human activities, and prey depletion (Jackson et al., 2010; Li et al., 2016; McCarthy et al., 2017). Wild ungulates (particularly caprines) typically constitute the main prey base for snow leopards, which are supplemented with smaller mammals (e.g., marmots, pikas, etc.) and birds. However, the snow leopard’s habitats largely overlap the grazing lands of pastoral communities in China, Mongolia, India, Pakistan, and Nepal, and snow leopard predation of livestock has occurred in almost all investigated regions (Li, 2012; Mishra et al., 2016). Across the snow leopard’s range, livestock consumption varies considerably from none (Jumabay-Uulu et al., 2014) to near 70% (Anwar et al., 2011; Bocci et al., 2017), but it generally accounts for 15–30% of their diets (Mallon et al., 2016). Consequently, widely reported retaliatory killings of snow leopards, triggered by livestock attacks, potentially threaten the species’ subsistence (Oli et al., 1994; Bagchi and Mishra, 2006; Aryal et al., 2014). For snow leopard conservation to succeed, the ecological determinants of livestock predation must be understood. Deprivation of wild prey in pastoral regions has been linked to livestock depredation by snow leopards (Lovari and Mishra, 2016). Hence, recovery of the snow leopard’s wild ungulate prey populations may help reduce livestock predation (Mishra et al., 2003) through the mechanism of apparent facilitation, whereby the increased availability of one prey reduces predation on the alternative prey by their shared predator (Colton, 1987). However, predator density can be positively related to wild prey availability. As wild prey populations increase, so may the coexisting predator abundance, which in turn could aggravate livestock predation, a phenomenon termed apparent competition (Holt and Lawton, 1994; Abrams and Matsuda, 1996). Several studies have shown higher livestock depredation by snow leopards in more wild-prey-abundant areas or seasons (Bagchi and Mishra, 2006; Suryawanshi et al., 2017; Shrestha et al., 2018), thus challenging whether boosting wild prey abundance can effectively mitigate human–snow leopard conflicts. Taken together, the mode and dynamics of snow leopard predation on both wild and domestic prey in relation to their availabilities may vary among regions, depending on specific combinations of local ecological and social factors.
Currently, 45% of the entire snow leopard range (by area) is on the QTP, and within that, the Sanjiangyuan region contains the largest continuous snow leopard habitat on the plateau (Figure 1; Xiao et al., 2019; Li et al., 2020). Of the approximately one million people (> 90% Tibetan ethnics) who live in that region, most lead a pastoral lifestyle (Liu et al., 2016). Records of early pastoralist habitation on the Plateau date back more than 8,000 years (Brantingham and Xing, 2006; Miehe et al., 2009), so human–wildlife coexistence is well established and has been the norm for much of the regions’ human occupation. However, rapid growth of both human and livestock populations over the last 50 years may have generated unprecedented pressure on both wild prey and predators (Harris, 2010). Despite Sanjiangyuan’s key position for regional and global snow leopard conservation, little data are available on the species’ feeding ecology in that region. The most well-known large-scale snow leopard diet analysis in the region was done more than 30 years ago by Schaller (1988), who identified relatively low dietary proportions of both wild ungulates (30–38%) and livestock (3–22%), along with curiously high proportions of small mammals (41–51%; marmots in particular). That Sanjiangyuan dataset differs considerably with the results from other regions of similar studies that invariably show snow leopard prey composition dominated by large, wild and domestic, ungulate species (Lyngdoh et al., 2014). Two later studies, both using morphological identification of fecal origin and food type, found incongruent results of snow leopard diets in Sanjiangyuan: Liu et al. (2003) reported prey composition similar to Schaller (1988), whereas Li (2012) found high proportions of ungulates (68% bharal [Pseudois nayaur] and 16% livestock, in biomass) and only 17% small mammals.
Figure 1. Map of the study region showing sampling locations and snow leopard dietary compositions at each of the seven sites that were analyzed. Pie charts show the relative read abundance of prey categories in the snow leopard diet at each site. The inset shows the locations of the Qinghai-Tibetan Plateau and Sanjiangyuan region, and of the study region (green box) within China. The current global distribution range of the snow leopard was retrieved from the International Union for Conservation of Nature’s Red List of Threatened Species (McCarthy et al., 2017).
Earlier snow leopard diet studies in Sanjiangyuan invariably relied on traditional morphology-based methods, which can suffer substantially from misidentification of predator and prey (Monterroso et al., 2019). Recently developed fecal DNA-based molecular tools provide an unprecedented level of highly accurate and efficient prey identification and greatly facilitate large-scale analysis of complex diets (Pompanon et al., 2012; Ando et al., 2020). When used to analyze snow leopard diets in a number of regions, this approach has revealed previously unknown prey diversity (Shehzad et al., 2012; Hacker et al., 2021). However, because those studies included few samples (n < 10) from the QTP, and none from Sanjiangyuan, updated dietary information for that core snow leopard stronghold fell short and thus failed to help our understanding of the snow leopard feeding ecology and trophic network in that region. Furthermore, wild and domestic prey availabilities may vary among different areas in Sanjiangyuan, and knowledge of how snow leopard food choice responds to changes in local prey composition is critically needed to predict the ability of this species to persist in human-dominated landscapes.
In this study, we used fecal DNA metabarcoding to investigate snow leopard diets at seven sites across Sanjiangyuan (Figure 1 and Supplementary Table 1), and thus uncovered highly resolved prey compositions in the region and revealed the underlying ecological factors that affect livestock predation by snow leopards. We tested spatial and seasonal variations in diet compositions and examined the correlations between the availabilities of the main wild prey and of domestic livestock with dietary patterns. Our findings elucidate the feeding ecology of the snow leopard in its central range in China, and that can be used to improve management interventions designed to preserve this species on the QTP.
Our study area in the Sanjiangyuan region (31°39′–36°12′N, 89°45′–102°23′E), also known as the Three-River Headwaters Region of the QTP, covers 363,000 km2, has an average elevation of > 4,000 m (3,335–6,564 m) ASL, and includes the headwaters of Asia’s three largest rivers: the Yangtze, Yellow, and Lancang (Mekong) (Li et al., 2013). It has a typical plateau, continental monsoon climate in which 80% of its annual rainfall (mean 262–773 mm) occurs from May to October and its mean annual temperature varies from −5.6 to 3.8°C (Bai et al., 2020). Vegetation in Sanjiangyuan is mainly grasslands (alpine meadow, steppe, and desert grassland) with scattered forests, shrubs, cushion plants, swamp vegetation, etc. (Li et al., 2012).
Based on the gradient of livestock grazing intensity (with an increasing trend from northwest to southeast) and on pilot studies, we selected seven sites for fecal sampling: Suojia, Zhaqing, Angsai, Baizha, Xialaxiu, Zhongda, and Haxiu. Each of these sites represents a township that was spaced at least 50 km from any of the others, and they were located in Zhiduo, Zaduo, Nangqian, and Yushu Counties (Supplementary Table 1).
From 2009 to 2017, we collected putative snow leopard feces along transect lines. Samples from most sites were collected in 2010–2014, except for Angsai, where most sampling was conducted in 2017. Field species identification was based on morphological characteristics (i.e., diameter, length, shape, and number of clumps) of the feces (Janečka et al., 2008). Geographic coordinates and other field information were then recorded. In the field, each fecal sample was stored either in a plastic ziplock bag or a sterile 50 mL centrifuge tube and all samples were placed in a cool, dry place. Once at the laboratory, all samples were transferred into sterile 50 mL centrifuge tubes containing silica gel and stored at −20°C.
We estimated snow leopard density at each study site by using independent capture rates provided by camera trapping data gathered in 2014–2015, following Rowcliffe et al. (2008). Briefly, each study site was divided into several small valleys (mean area = 16 km2/valley). To detect snow leopards, we used a total of 73 infrared cameras deployed across all valleys, with 1–2 cameras placed in each valley. The independent capture rate of each camera trap was then calculated as the number of independent captures divided by the total camera-days. We obtained 632 independent snow leopard captures over 13,143 camera-days.
We collected local bharal density data in 2014 by using double-observer surveys (Suryawanshi et al., 2012): Two observers walked together along transects within the study sites and independently scanned and counted bharal through binoculars, and then the total number of bharal counted for each survey area was divided by the area size to obtain the bharal density. More details of snow leopard and bharal density data collection are described in Xiao (2017).
Livestock biomass, which was measured in sheep units (SU), provided a standardized abundance index across different livestock species: 1 yak = 5 SU, 1 sheep or goat = 1 SU; similar to Miller, 2000). Livestock numbers, in natural individuals regardless of species, at the study sites were obtained from the 2011 official statistics of the Animal Husbandry Bureau of the Department of Agriculture, Yushu Prefecture, Qinghai Province. Total livestock counts in Qinghai remained at a highly consistent level throughout the study period (average yearly change = 0.83% between 2007 and 2017). Local livestock densities were calculated using the number of livestock divided by the township area of each site.
We used the 2CTAB/PCI method to extract fecal DNA from the samples (Vallet et al., 2007). This method had been used successfully for molecular dietary analysis of various carnivore species (Xiong et al., 2017; Shao et al., 2021). Briefly, we extracted DNA from each sample (100–200 mg from multiple parts) and processed in small batches (typically 23 samples/batch) together with one extraction blank per batch to monitor for cross-contamination. To remove potential PCR inhibitors, extracted DNA was purified with an EasyPure PCR Purification Kit (TransGen Biotech, Beijing, China) according to the manufacturer’s instructions.
We used molecular species identification to identify the specific origin of each fecal sample. To accomplish that we used the 16S rRNA primer pair (16S-F: GAGAAGACCCTATGGAGC; 16S-R: ATAGAAACCGACCTGGAT) to amplify an ∼350 bp (excluding the primers) mitochondrial 16S rRNA gene sequence that can unambiguously assign snow leopard and other coexisting carnivore species (Xiong et al., 2016). The PCR conditions and program, as well as the PCR product visualization and sequencing, were carried out following slightly modified (annealing temperature = 50°C) protocols described by Xiong et al. (2016). Each sample was assigned its origin species based on DNA amplicons matched to sequences in the GenBank database (98–100% identity). Samples identified as originating from snow leopards were included in the subsequent dietary analyses.
Prey DNA metabarcoding was done using the vertebrate primer pair 12SV5 (12SV5-F: TAGAACAGGCTCCTCTAG; 12S V5-R: TTAGATACCCCACTATGC), which targets a fragment (∼100 bp excluding the primers) of the V5 loop of the mitochondrial 12S rRNA gene (Riaz et al., 2011). Despite its short size, this amplicon region has demonstrated a high taxonomic resolution among vertebrate taxa (Riaz et al., 2011). To allow for multiplexing and sequencing PCR products within a single high-throughput sequencing run, each forward and reverse primer was tagged at its 5′ end with a unique 9-nucleotide sequence that differed by at least 3 nucleotides from any other tag. To specifically restrict the amplification of snow leopard DNA, a blocking oligonucleotide, UnciB (CTATGCTTAGCCCTAAACCTAGATAGTTAGCTCAAACAA AACTAT-C3; Shehzad et al., 2012) was included in the PCRs.
Each PCR was performed in 25 μL reactions composed of 12.5 μL of 2 × EasyTaq PCR SuperMix (TransGen Biotech), 0.5 μL of each 10 μM 12SV5 twin-tagged primers (F1-R1, F2-R2, etc.), 2.5 μL of 40 μM UnciB, 0.5 μL of 20 mg/mL bovine serum albumin, 6.5 μL of double-distilled water (ddH2O), and 2 μL of DNA extracts. The PCR profile included an initial denaturing step at 95°C for 5 min followed by 35 cycles at 95°C for 30 s, 60°C for 30 s, and 72°C for 30 s, and a final extension step at 72°C for 10 min. Extraction blank PCRs and negative PCR controls (substituting ddH2O for the DNA extract) were run alongside the sample PCRs.
To construct sequencing libraries, PCR products (n = 116–128 in each library) were analyzed using 1.5% agarose gel electrophoresis, and then pooled in equal volumes (20 μL each) and purified using the EasyPure PCR Purification Kit. Using a PCR-free protocol with the KAPA Hyper Prep Kit (KAPA Biosystems, Wilmington, Massachusetts, United States), four sequencing libraries were prepared and then sequenced (2 × 150 bp paired-end reads) on a HiSeq X Ten platform (Illumina, San Diego, California, United States) by the Beijing Genomics Institute (Wuhan, China).
We processed the raw sequence data through a bioinformatics pipeline using the OBITools package (Boyer et al., 2016). Briefly, forward and corresponding reverse sequences were aligned and assembled using illuminapairedend, and sequences with low alignment quality (< 40) were removed using obigrep. Demultiplexing was done using ngsfilter to assign sequences to their original samples based on perfect matches to tags and ≤ 2 mismatches on primers. Identical sequences were dereplicated using obiuniq and then sequences with lengths shorter than 80 bp, or having a count less than 100, were removed using obigrep. Sequences putatively resulting from PCR amplification or sequencing errors (i.e., ‘‘internal’’ sequences) were detected and discarded using obiclean (r = 0.5). The remaining sequences were compared to a taxonomic reference library using ecotag as follows. First, the reference library was generated using ecoPCR with the EMBL1 (release 133) and the NCBI Taxonomy2 databases. Any of our sample sequences having a percentage of identity less than 95% to its closest match in the reference library was considered a putative contaminant (e.g., chimera or highly degraded sequence) and excluded. To obtain more precise identification, the taxonomy of the remaining unique sequences was reassigned after BLAST searching (blastn with default parameters) each sequence through the GenBank database.3
We used the following criteria for making taxonomic assignments: the query sequence was assigned (i) to species-level when it matched a single locally occurring species in the database with ≥ 98% identity or (ii) to the lowest taxonomic level including all the species when it matched more than one locally occurring species with ≥ 98% identity. We then clustered the unique sequences that were assigned to the same species. A list of wild mammals that occur locally in Sanjiangyuan was compiled from literature, surveys, and camera trapping records from that region (Wang et al., 2009; Cai et al., 2019; Gao et al., 2019; Jia et al., 2020).
To exclude PCR artifacts, tag jumps, and cross-contamination in each sample, we removed low-frequency sequences, i.e., sequences that made up < 2% of a PCR product, or were fewer than 50 reads or the corresponding reads in negative control PCRs (Schnell et al., 2015; Yang et al., 2021). Obvious human contaminants and predator reads were also excluded.
To quantify the dietary composition, we converted our taxa-by-samples matrix of sequence data into occurrence and read abundance and summarized the data using four metrics. Percent frequency of occurrence (FOO) is the percent of fecal samples containing a given prey taxon. Percent of occurrence (POO) is the number of given taxon occurrences divided by the total number of taxa occurrences. Weighted percent of occurrence (wPOO) weights each sample equally by calculating the number of weighted occurrences (e.g., if a sample contains two taxa, each will be given one-half the weight) divided by the total number of samples. Relative read abundance (RRA) is the sum of read proportion of a given sequence across samples divided by the total number of samples. The RRA metric has been used widely in dietary metabarcoding studies (Ando et al., 2020), and it is both less sensitive to rare taxa with low-abundance reads and acceptably accurate at the population-level (Deagle et al., 2019). We used Spearman’s correlation analyses with the “cor” function in the R package stats to examine possible correlations between the different metrics. Then based on those results (see section “Results”), we decided to use RRA data in most of the dietary analyses.
We constructed a predator–prey trophic network using the R package bipartite v.2.11 (Dormann et al., 2008) to visualize the regional dietary composition of snow leopards.
Then we estimated the prey diversity in the snow leopards’ diets at each study site by calculating Shannon’s diversity index H (where q = 1, the exponential of the classic Shannon index) in the R package iNEXT v.2.0.19 (Hsieh et al., 2016), and then constructing rarefaction and extrapolation curves for each site to assess whether the sample sizes were adequate.
We also tested year, season, and site dietary differences using permutational multivariate analysis of variance (PERMANOVA) tests that were run using the “Adonis” function with 999 permutations in the R package vegan v.2.5-6 (Oksanen et al., 2019). We used non-parametric Wilcoxon rank-sum tests with the “wilcox.test” function in R to evaluate how snow leopard consumption of each main prey category (i.e., wild ungulates, livestock, and small mammals) varied between warm and cold seasons. Warm (May–October) and cold (November–April) seasons were based on average monthly temperatures above or below 0°C.
To calculate the percent contribution of each prey taxon to the dietary dissimilarity among sites, a similarity percentage analysis (SIMPER) based on Bray–Curtis dissimilarities was conducted in PAST v.4.05 software (Hammer et al., 2001). We also performed principal component analysis (PCA) in PAST, using a correlation matrix and scatter biplot to detect differences in the dietary compositions among sites. Then, using the R package UpsetR v.1.4.0 (Conway et al., 2017), we visualized both shared and unique prey taxa among sites. Next, we analyzed prey preference using Jacobs’ D index, which measures the utilization of prey taxa relative to their availability (Jacobs, 1974). That index value ranges from −1 (complete avoidance) to 1 (complete preference), with 0 indicating utilization in proportion to availability.
To evaluate factors that may influence the snow leopard’s dietary composition, we performed univariate general linear model analysis by using the “lm” function in the R package stats. To begin, we constructed linear models using the proportions of bharal (the main wild prey species) and livestock in the diets (RRA) and snow leopard density (independent captures/camera day) as the response variables and bharal density (individuals/km2), total livestock consumption (estimated by the product of livestock RRA and snow leopard density), livestock biomass (measured in SU), livestock density (individuals/km2), and density ratio of livestock to total ungulates (livestock and bharal) as the explanatory variables. Correlations with the above variables were also assessed for total livestock consumption as measured by the product of livestock RRA and local snow leopard density. In addition, we constructed linear models between snow leopard density and bharal or livestock density, and between bharal density and livestock density. Ordinary least squares regression was used to determine correlations between variables, and a t-test was used to test the significance of linear regression slopes.
Statistical analyses were performed in R v.3.5.0 (R Core Team, 2020) or using PAST v.4.05 (Hammer et al., 2001). All statistical tests were two-tailed with the significance level set at 0.05.
Of the 1,043 fecal samples we collected from the seven study sites during 2009–2017, 419 (40.2%) were genetically verified to be snow leopard feces (see section “Materials and Methods”). The number of snow leopard fecal samples varied among sites, from 10 to 203 (Supplementary Table 1). Samples that came from other sympatric carnivores [n = 307; mainly including gray wolf (Canis lupus), brown bear (Ursus arctos), leopard (Panthera pardus), Eurasian lynx (Lynx lynx), red fox (Vulpes vulpes), Tibetan fox (V. ferrilata), and Pallas’ cat (Otocolobus manul)] were not analyzed in this study.
We used the mtDNA 12S-V5 region for the DNA metabarcoding that identified the vertebrate prey in the snow leopard fecal samples (see section “Materials and Methods”). A total of 10–20 million paired-end reads were generated from each sequencing library, and following sequence filtering steps, we obtained 26,789–41,982 reads per PCR (Supplementary Table 2). Dietary sequence data were obtained for 351 samples, with 9–165 samples from each site (Supplementary Table 1). Based on sequence similarity and local fauna inventories, we identified 20 unique vertebrate taxa comprising 9 families and 18 genera (Table 1 and Figure 2). The 12S-V5 barcode identified snow leopard prey at a high taxonomic resolution—19 (95%) of the taxa were assigned to the species level. The only exception was the genus Buteo (Buteonine hawks), for which two potential species (B. hemilasius and B. buteo) that co-occur in the study region have identical sequences. The most species-rich prey category was the ungulates (6 wild and 4 domestic species) and that was followed by small mammals (lagomorphs and rodents; 8 species) and birds (2 species/genus). The number of dietary species per sample varied from 1 to 3 (mean = 1.12, SD = 0.38), with 90.03% of the samples containing only one prey species, 7.98% having two species, and 1.99% with three.
Table 1. Snow leopard dietary compositions, presented as mean values of relative read abundance (RRA) of individual prey species, at seven sampling sites.
Figure 2. Dietary composition of the snow leopard in Sanjiangyuan on the Qinhai-Tibetan Plateau. Proportional representation of relative read abundance (RRA) of vertebrate prey in (A) all snow leopard samples and (B) in the diets at each sampling site.
Dietary data summarized using occurrence-based and sequence abundance-based metrics (POO, wPOO, and RRA; see section “Materials and Methods”) showed highly consistent quantitative profiles (POO and RRA: ρ = 0.836, p < 0.0001; wPOO and RRA: ρ = 0.915, p < 0.0001; Supplementary Figure 1 and Supplementary Data 1). Therefore, we used RRA data exclusively for subsequent dietary analyses. Across all samples, wild ungulates accounted for the largest proportions of the snow leopard diets, and that was followed by domestic livestock, small mammals, and birds (Figure 2A). The bharal was the most dominant species consumed (mean RRA = 82.1%; Supplementary Data 1), while the yak (Bos grunniens) was the most common livestock species consumed (3.89%). Among the small mammals, the Himalayan marmot (Marmota himalayana) was the most dominant prey species (5.16%).
Snow leopard diets varied substantially among sites as prey species richness (2–12) and Levins’ standardized niche breadths (0.03–0.48) demonstrate (Table 1). Although prey richness can be greatly affected by sample size, and insufficient sampling may lead to underestimated dietary diversity, sample-based rarefaction curves showed that prey species diversity (Shannon’s diversity index) approached the asymptote for the least sampled Zhongda and Xialaxiu sites, thus indicating that low prey richnesses at those sites cannot be solely attributed to their small sample sizes. However, rarefaction curves showed that prey species diversity approached the asymptote for all sites (estimated sample coverage 0.90–0.99) except Baizha (coverage 0.83) and Haxiu (coverage 0.82), which needed larger sample sizes to fully capture their snow leopards’ dietary diversities (Supplementary Figure 2).
Since samples were collected over extended time periods at several sites (Supplementary Table 1), we first assessed the effect of sampling year on dietary composition. Because sampling spanned the longest period (6 years) in Suojia and its sample size was considerably larger across years compared to those of other sites, we tested dietary difference by year using the Suojia dietary data. PERMANOVA results indicated a lack of variation in relative proportions (RRA) of prey species from different years [Pseudo-F(5, 159) = 0.919, p = 0.460]. So, we combined dietary data across years for each site for subsequent analyses. Next, PERMANOVA of snow leopard diets at Suojia, Zhaqing, and Angsai, the sites with the largest number of samples collected during both the warm (May–October) and cold (November–April) seasons, showed no seasonal variations in prey species compositions at each site [Suojia: Pseudo-F(1, 163) = 0.681, p = 0.574; Zhaqing: Pseudo-F(1, 68) = 2.019, p = 0.114; Angsai: Pseudo-F(1, 63) = 0.751, p = 0.573]. We further compared consumptions of each main prey category (i.e., wild ungulates, livestock, and small mammals) between the warm and cold seasons and found no seasonal variation in the per-sample RRA at each site except for Zhaqing, where significantly more small mammals (mainly marmots) were consumed in the warm than in the cold seasons (Wilcoxon rank-sum test: W = 360, p = 0.028).
Snow leopard dietary compositions (in terms of RRA of individual prey species; Figure 2B) varied considerably by site [PERMANOVA: Pseudo-F(6, 344) = 3.370, p = 0.001]. Variations in the proportion of bharal (SIMPER contribution = 27.4%) in the diets accounted for most of the dissimilarity across sites, and that was followed by proportions of marmot (SIMPER contribution = 16.4%) and goat (Capra hircus; SIMPER contribution = 13.3%) (Supplementary Table 3). Differences in prey composition were also clearly shown by PCA (Figure 3), in which bharal, goat, and marmot accounted for most of the variation in the first two principal components, which together explained 85.0% of the variance. Bharal, the only prey species found in the diets at all seven sites, was followed by marmot at six sites, but 15 prey species were found only at one or two sites (Supplementary Figure 3). By prey category, wild ungulates predominated in the diets at all sites (sum RRA = 74.3–91.9; mean = 81.5%; Figure 1), with bharal consistently being the main prey species (RRA = 59.4–91.9%; mean = 76.0%) (Table 1). Livestock constituted 0–23.1% (mean = 7.6%) of snow leopard diets across sites (Figure 1), but the species consumed the most varied. For instance, goat was the most abundant livestock prey species at Xialaxiu, but domestic yak was the most consumed livestock species at Zhaqing. Proportions of small mammals also varied considerably among sites (0–18.4%; mean = 10.7%) (Table 1).
Figure 3. Principal component analysis of snow leopard prey composition at seven sampling sites. Site positions are shown in relation to the relative read abundance of the select prey species that contributed the most to areal dietary differences.
As wild ungulates (bharal in particular) and domestic livestock constituted the main prey base for snow leopards, we assessed prey preference for bharal and livestock by using their estimated densities that were based on field observation and official statistics, respectively (see section “Materials and Methods”), and on their dietary representation in snow leopard diets. The results showed that snow leopards strongly preferred bharal over livestock across the sites (Figure 4), and that relatively high livestock consumption occurred only where livestock density was substantially greater than bharal density (see also Figure 5).
Figure 4. Snow leopard selectivity of livestock and bharal at six sampling sites. Jacobs’ prey selectivity index (D), which can vary from −1 to 1, was calculated based on relative read abundances (shown in the circles) in the snow leopard diets and the population densities of livestock and bharal at six sampling sites. There were no bharal density data from Angsai, so that site was excluded from this analysis. Circle color and size indicate the value of D for the two prey types at each site.
Figure 5. Correlations between the proportion of livestock in snow leopard diets and (A–D) prey (livestock and bharal) abundance and (E) predator density, and (F) between total livestock consumption and livestock biomass at the study sites. Livestock biomass is measured in sheep units (SU). Livestock densities (in individuals/km2) are based on the total number of livestock and the township area of each site. Bharal densities (in individuals/km2) were estimated using double-observer surveys. Snow leopard densities (in independent captures/camera day) were estimated using camera-trapping data (see section “Materials and Methods” for details). Total livestock consumption was estimated as the product of livestock relative read abundance (RRA) and snow leopard density. Solid lines indicate statistically significant (at p < 0.05) linear regressions with the 95% confidence intervals shaded. Dotted lines indicate non-significant linear fits of the corresponding variables. SJ, Suojia; ZQ, Zhaqing; AS, Angsai; BZ, Baizha; XLX, Xialaxiu; ZD, Zhongda; HX, Haxiu. Bharal and snow leopard density data for Angsai were not available, so only the data from the remaining six sites are shown in (C–F). **p < 0.01.
To investigate the factors that may influence snow leopards to consume livestock, we analyzed the proportion of livestock in snow leopard diets in relation to predator and prey availability at the study sites (Figure 5). Snow leopard and bharal density data were not available for Angsai, so that site was excluded from the following analyses. Livestock RRA was significantly, positively correlated in a linear fashion with total local livestock biomass (see section “Materials and Methods”) (Figure 5A), but it had no apparent relationship with local livestock densities across the sites (Figure 5B). Likewise, livestock RRAs were not correlated with either bharal densities, the relative densities of livestock out of all ungulates, or snow leopard densities (Figures 5C–E) at six sites. As snow leopard density varied among sites, we also estimated total livestock consumption as the product of livestock RRA in the diet and snow leopard density at each site. This metric gave a measure of total livestock consumption by snow leopards across sites. Again, we found a significant linear relationship between total livestock consumption and livestock biomass (Figure 5F). Also, total livestock consumption was not correlated with prey or predator densities (Supplementary Figure 4). The pattern remained the same whether livestock density was measured in SU or individuals per area (data not shown). These results indicate that total livestock biomass was the most important factor contributing to proportional and total livestock consumption by snow leopards. We also analyzed the relationships of bharal RRA in snow leopard diets with prey and predator availabilities and found no significant correlations (Supplementary Figure 5).
To assess relationships of snow leopard population size with prey availability, we first tested possible correlations between snow leopard density and bharal and livestock density and biomass (Figure 6). Snow leopard density showed a positive but borderline significant (p = 0.07) linear correlation with bharal density across six study sites (Figure 6A), but after adding data from another QTP site where we had conducted field surveys of snow leopard and bharal populations (Xueshan Township; Supplementary Tables 1, 4), the resulting correlation was significant (p = 0.04; Figure 6B). While snow leopard density exhibited no clear relationship with livestock biomass (Figure 6C), there was a slight decreasing trend with increasing livestock density (Figure 6D). In addition, bharal density showed slight negative relationships with both livestock biomass and density (Figures 6E,F). Therefore, snow leopard population size most likely hinges on the availability of its main wild prey, bharal.
Figure 6. Correlations between snow leopard density and prey (bharal and livestock) abundance (A–D), and between bharal density and livestock abundance (E,F) at the study sites. (A) Includes data from six study sites and (B) includes the same data plus field survey data from an additional site on the Qinghai-Tibetan Plateau: Xueshan (XS). See Figure 5 for explanations of data sources, sampling sites, and graphical elements and interpretations. *p < 0.05.
Molecular dietary analysis has revolutionized the field of food web ecology and has enabled highly resolved, accurate, and reproducible diet reconstruction for diverse organisms (Symondson, 2002; Pringle and Hutchinson, 2020). Using this method, we obtained a large-scale snow leopard dietary dataset from Sanjiangyuan for the first time since Schaller’s (1988) early study. Although we found variations in dietary diversity and composition among sites, which was likely primarily because prey diversity and availability differed among localities, wild ungulates were invariably the predominant prey category at all sites (mean RRA = 81.5% across sites), and small mammals were generally low in snow leopard diets (mean RRA = 10.7%; < 20% at the highest site). These results are in stark contrast to Schaller’s data but are consistent with snow leopard diets reported for most other regions (Lyngdoh et al., 2014; Hacker et al., 2021). It is possible that some of Schaller’s feces samples were misidentified as snow leopard while actually belonging to other coexisting carnivores. A recent review of over 500 carnivore diet analyses revealed considerably high error rates in fecal morphology-based predator species identification (Monterroso et al., 2019). In particular, the chances of feces from other carnivores (e.g., foxes, wolves, dogs, etc.) being misidentified as snow leopard feces are high (33–79%; Anwar et al., 2011; Jumabay-Uulu et al., 2014; Weiskopf et al., 2016), and those sympatric carnivores generally have broader dietary ranges and consume more small prey than snow leopards do. Therefore, such misidentifications lead to both overestimating snow leopard consumption of small mammals and underestimating ungulates in their diets. Additionally, traditional morphological dietary analysis relies on undigested remains (bones, hairs, claws, etc.) for prey identification, and that can lead to occurrence counts biased toward smaller prey species because they may leave more remains per consumed biomass than do large prey. Based on our updated and precise dataset, the regional dietary specificity of snow leopards on the QTP in relation to other regions (such as in Lyngdoh et al., 2014) should be re-evaluated. However, it should be noted that because our fecal sample sizes for several study sites were small (n < 20), snow leopard dietary diversity may not have been fully captured at those sites (see rarefaction curves in Supplementary Figure 2). Further sample collection at those sites would provide more comprehensive dietary profiles and accurate areal comparative analysis.
Wild ungulates, particularly bharal, are the snow leopards’ preferred prey across Sanjiangyuan, and bharal remained the dominant prey choice even where livestock density was about 10-times higher than bharal density (Supplementary Figure 5D). This is consistent with studies in India and Mongolia that showed that snow leopards preferred wild ungulates over livestock, even when the latter was much more abundant than the former (Johansson et al., 2015; Suryawanshi et al., 2017). Although livestock is generally not the main food source for snow leopards in Sanjiangyuan (mean RRA = 7.6% across sites), it did reach 23% at one site, and that is a serious predation problem that causes considerable financial loss to pastoralists. We found that livestock consumption by snow leopards (both per sample RRA and total amount) was strongly correlated with local livestock biomass, but not with livestock density (Figure 5). Livestock density is a common index used to assess wild predator depredation response to domestic prey availability (Chetri et al., 2017; Pimenta et al., 2018; Khanal et al., 2020). However, we argue that spatial distribution of livestock, compared with wild prey, is more heterogeneous and often clustered near resources and shelters, thus making average density across large areas an inappropriate measure of livestock availability. Townships with greater total numbers of livestock tend to have livestock in larger herds that may be more conspicuous and attractive to carnivores, and chances for successful attacks on such aggregations are likely to be high. Similarly, studies from Nepal (Chetri et al., 2019; Tiwari et al., 2020) and Mongolia (Mijiddorj et al., 2018) reported that livestock losses attributed to snow leopards intensified with increasing herd size, thus suggesting that attacking larger livestock herds may be a common snow leopard predation tactic.
Could increasing wild prey populations for snow leopard conservation also attenuate livestock depredation? The contradictory answers from field evidence (see section “Introduction”) underscore the complexity of snow leopard–wild prey–livestock interactions and the importance of the ecological and social contexts in designing human–carnivore conflict mitigation strategies. In line with the prediction of apparent facilitation, our results show a slight negative trend between bharal density and livestock consumption by snow leopards (Figure 5C). Data from most sites appear to support this trend, with greater bharal density associated with less livestock in snow leopard diets. However, Zhongda, where bharal density was the lowest of all sites, showed low livestock consumption that was similar to that of Suojia and Haxiu, two of our most bharal-abundant sites. Those three sites had similar livestock biomass (Figure 5A), thus suggesting that livestock depredation at Zhongda was determined mainly by local livestock biomass rather than by bharal density. Our results do not seem to support the apparent competition mechanism, since the site with the highest bharal and snow leopard densities, Suojia, showed low livestock consumption (Figures 5C,F). Apparent facilitation between wild and domestic prey is expected to occur when wild prey availability is sufficiently high (Suryawanshi et al., 2017). A comparative study across the snow leopard range indicated considerably higher overall wild ungulate densities in Sanjiangyuan than in Mongolia, Nepal, and India (Xiao, 2017). This difference in wild prey abundance may be an important mechanism underlying the discrepancy in the functional response of the snow leopards’ livestock intake with respect to wild prey availability between our sites and in those regions where increasing wild prey is often associated with heightened livestock predation by snow leopards (Bagchi and Mishra, 2006; Suryawanshi et al., 2017; Shrestha et al., 2018). Using the DNA metabarcoding method with additional samples from more sites, we could more fully determine how the effects and interactions of different ecological variables modulate livestock predation by snow leopards. The slight negative relationships between livestock and bharal densities across sites may result from competitive exclusion between domestic and wild ungulates that occupy similar ecological niches, as shown in other snow leopard range regions (Mishra et al., 2004; Karimov et al., 2018). Alternatively, disparate habitat preferences of livestock and bharal may also lead to negative associations between their local population abundances (Xiao, 2017).
Our study provides several important insights into snow leopard feeding ecology and conflicts with humans in Sanjiangyuan. First, snow leopards strongly preferred bharal relative to livestock and only increased predation of the latter when its availability far exceeded bharal. Unlike the snow leopards in several other regions where livestock dominates their diets (e.g., India, Bagchi and Mishra, 2006 and Pakistan, Bocci et al., 2017), the snow leopards in Sanjiangyuan are not the main perpetrators of livestock depredation. This agrees with the local pastoralist views that livestock losses to snow leopards are considerably less than to other carnivores, particularly wolves (Li, 2012). Second, livestock loss to snow leopards rose significantly as local livestock abundance (biomass) increased. Finally, congruent with previous studies of snow leopards (Suryawanshi et al., 2017) and other large carnivores (Carbone and Gittleman, 2002; Karanth et al., 2004), our results support the critical dependency of snow leopard population size on wild prey abundance.
Our results support fostering wild ungulate population recovery as a measure to both boost snow leopard populations and, consequently, reduce their predation of livestock. Although current snow leopard predation on livestock is comparatively low in Sanjiangyuan, continuous human population growth and expansion of livestock husbandry could exacerbate resource competition between domestic and wild ungulates and drive declines in wild prey abundances, thus leading to intensified livestock depredation and escalating conflicts between pastoralists and wild carnivores. Conservation management should focus on controlling livestock abundance and devising alternative income sources to alleviate economic reliance on livestock grazing. Such efforts have been implemented across the QTP in the last decade and they have resulted in recovery of damaged grassland (Li et al., 2021). Additionally, improved livestock husbandry techniques and guarding practices, such as attended herding and nighttime use of reinforced corrals, can be highly effective at minimizing losses to wild carnivores (Jackson and Wangchuk, 2001; Johansson et al., 2015). Those practices would be particularly helpful for communities with large herds that have greater predation risks.
The datasets presented in this study can be found in online repositories. The names of the repository/repositories and accession number(s) can be found below: NCBI (accession: PRJNA767363).
Ethical review and approval was not required for the animal study because fecal samples were collected non-invasively.
MY and ZL designed the research. LX and CC contributed to fecal sample collection. QL conducted all experiments and dietary data analysis and generated the figures. LX collected field data. MY was the primary author of the text with contributions from all authors. All authors approved the final manuscript.
This project was funded by the National Natural Science Foundation of China (Grant No. 31970431), the Second Tibetan Plateau Scientific Expedition and Research Program (STEP) (Grant Nos. 2019QZKK0304 and 2019QZKK0503), Panthera, and the Snow Leopard Trust.
The authors declare that the research was conducted in the absence of any commercial or financial relationships that could be construed as a potential conflict of interest.
All claims expressed in this article are solely those of the authors and do not necessarily represent those of their affiliated organizations, or those of the publisher, the editors and the reviewers. Any product that may be evaluated in this article, or claim that may be made by its manufacturer, is not guaranteed or endorsed by the publisher.
We are grateful to Juan Li, Yanlin Liu, Hang Yin, and Gongbao Zhala for their assistance with sample collection.
The Supplementary Material for this article can be found online at: https://www.frontiersin.org/articles/10.3389/fevo.2021.783546/full#supplementary-material
Supplementary Data 1 | Dietary compositions: relative read abundance (RRA), percent of occurrence (POO), weighted POO (wPOO), percent frequency of occurrence (FOO).
Abrams, P. A., and Matsuda, H. (1996). Positive indirect effects between prey species that share predators. Ecology 77, 610–616. doi: 10.1111/1365-2656.12305
Ando, H., Mukai, H., Komura, T., Dewi, T., Ando, M., and Isagi, Y. (2020). Methodological trends and perspectives of animal dietary studies by noninvasive fecal DNA metabarcoding. Environ. DNA 2, 391–406. doi: 10.1002/edn3.117
Anwar, M. B., Jackson, R., Nadeem, M. S., Janečka, J. E., Hussain, S., Beg, M. A., et al. (2011). Food habits of the snow leopard Panthera uncia (Schreber, 1775) in Baltistan, Northern Pakistan. Eur. J. Wildl. Res. 57, 1077– 1083.
Aryal, A., Brunton, D., Ji, W., Barraclough, R. K., and Raubenheimer, D. (2014). Human-carnivore conflict: ecological and economical sustainability of predation on livestock by snow leopard and other carnivores in the Himalaya. Sustain. Sci. 9, 321–329.
Bagchi, S., and Mishra, C. (2006). Living with large carnivores: predation on livestock by the snow leopard (Uncia uncia). J. Zool. 268, 217–224. doi: 10.1111/j.1469-7998.2005.00030.x
Bai, Y., Guo, C., Degen, A. A., Ahmad, A. A., Wang, W., Zhang, T., et al. (2020). Climate warming benefits alpine vegetation growth in three-river headwater region. China. Sci. Total Environ. 742:140574. doi: 10.1016/j.scitotenv.2020.140574
Bocci, A., Lovari, S., Khan, M. Z., and Mori, E. (2017). Sympatric snow leopards and Tibetan wolves: coexistence of large carnivores with human-driven potential competition. Eur. J. Wildl. Res. 63:92.
Boyer, F., Mercier, C., Bonin, A., Le Bras, Y., Taberlet, P., and Coissac, E. (2016). OBITOOLS: a UNIX-inspired software package for DNA metabarcoding. Mol. Ecol. Resour. 16, 176–182. doi: 10.1111/1755-0998.12428
Brantingham, P. J., and Xing, G. (2006). Peopling of the northern Tibetan Plateau. World Archaeol. 38, 387–414.
Cai, Z. Y., Qin, W., Gao, H. M., Wu, T., Chi, X. W., Yang, J. D., et al. (2019). Species diversity and fauna of mammals in Sangjingyuan National Park. Acta Theriol. Sin. 39, 410–420.
Carbone, C., and Gittleman, J. L. (2002). A common rule for scaling carnivore density. Science 295, 2273–2276. doi: 10.1126/science.1067994
Chetri, M., Odden, M., and Wegge, P. (2017). Snow leopard and Himalayan wolf: food habits and prey selection in the Central Himalayas, Nepal. PLoS One 12:e0170549. doi: 10.1371/journal.pone.0170549
Chetri, M., Odden, M., Devineau, O., and Wegge, P. (2019). Patterns of livestock depredation by snow leopards and other large carnivores in the Central Himalayas, Nepal. Glob. Ecol. Conserv. 17:e00526.
Colton, T. F. (1987). Extending functional response models to include a second prey type: an experimental test. Ecology 68, 900–912. doi: 10.2307/1938361
Conway, J. R., Lex, A., and Gehlenborg, N. (2017). UpSetR: an R package for the visualization of intersecting sets and their properties. Bioinformatics 33, 2938–2940. doi: 10.1093/bioinformatics/btx364
Deagle, B. E., Thomas, A. C., McInnes, J. C., Clarke, L. J., Vesterinen, E. J., Clare, E. L., et al. (2019). Counting with DNA in metabarcoding studies: how should we convert sequence reads to dietary data? Mol. Ecol. 28, 391–406. doi: 10.1111/mec.14734
Dormann, C., Gruber, B., and Früend, J. (2008). Introducing the bipartite package: analysing ecological networks. R News 8, 8–11.
Gao, H. M., Cai, Z. Y., Qin, W., Huang, Y. G., Wu, T., Chi, X. W., et al. (2019). Species diversity of birds in the three-river-source national park. Acta Ecol. Sin. 39, 8254–8270.
Hacker, C. E., Jevit, M., Hussain, S., Muhammad, G., Munkhtsog, B., Munkhtsog, B., et al. (2021). Regional comparison of snow leopard (Panthera uncia) diet using DNA metabarcoding. Biodivers. Conserv. 30, 797–817.
Hammer, O., Harper, D. A. T., and Ryan, P. D. (2001). PAST: paleontological statistics software package for education and data analysis. Palaeontol. Electronica 4, 1–9.
Harris, R. B. (2010). Rangeland degradation on the Qinghai-Tibetan plateau: a review of the evidence of its magnitude and causes. J. Arid Environ. 74, 1–12. doi: 10.1016/j.jaridenv.2009.06.014
Holt, R. D., and Lawton, J. H. (1994). The ecological consequences of shared natural enemies. Annu. Rev. Ecol. Syst. 25, 495–520. doi: 10.1002/ecy.1714
Hsieh, T. C., Ma, K. H., and Chao, A. (2016). iNEXT: an R package for rarefaction and extrapolation of species diversity (Hill numbers). Meth. Ecol. Evol. 7, 1451–1456.
Jackson, R. M., and Wangchuk, R. (2001). Linking snow leopard conservation and people-wildlife conflict resolution: grassroots measures to protect the endangered snow leopard from herder retribution. Endangered Species Update 18, 138–141.
Jackson, R. M., Mishra, C., McCarthy, T. M., and Ale, S. B. (2010). “Snow leopards: conflicts and conservation,” in The Biology and Conservation of Wild Felids, eds D. W. MacDonald and A. J. Loveridge (Oxford, UK: Oxford University Press), 417–430.
Jacobs, J. (1974). Quantitative measurement of food selection - a modification of the forage ratio and Ivlev’s electivity index. Oecologia 14, 413–417. doi: 10.1007/BF00384581
Janečka, J. E., Jackson, R., Yuquang, Z., Diqiang, L., Munkhtsog, B., Buckley-Beason, V., et al. (2008). Population monitoring of snow leopards using noninvasive collection of scat samples: a pilot study. Anim. Conserv. 11, 401–411. doi: 10.1111/j.1469-1795.2008.00195.x
Janeiro-Otero, A., Newsome, T. M., Van Eeden, L. M., Ripple, W. J., and Dormann, C. F. (2020). Grey wolf (Canis lupus) predation on livestock in relation to prey availability. Biol. Conserv. 243:108433.
Jia, D., Li, P. Y., Zhao, X., Cheng, C., Xiao, L. Y., and Lu, Z. (2020). Overview of sanjiangyuan community-based camera-trapping monitoring platform. Biodivers. Sci. 28, 1104–1109.
Johansson, Ö, McCarthy, T., Samelius, G., Andrén, H., Tumursukh, L., and Mishra, C. (2015). Snow leopard predation in a livestock dominated landscape in Mongolia. Biol. Conserv. 184, 251–258.
Jumabay-Uulu, K., Wegge, P., Mishra, C., and Sharma, K. (2014). Large carnivores and low diversity of optimal prey: a comparison of the diets of snow leopards Panthera uncia and wolves Canis lupus in sarychat-ertash reserve in kyrgyzstan. Oryx 48, 529–535. doi: 10.1017/s0030605313000306
Karanth, K. U., Nichols, J. D., Kumar, N. S., Link, W. A., and Hines, J. E. (2004). Tigers and their prey: predicting carnivore densities from prey abundance. Proc. Natl. Acad. Sci. USA 101, 4854–4858. doi: 10.1073/pnas.0306210101
Karimov, K., Kachel, S. M., and Hacklaender, K. (2018). Responses of snow leopards, wolves and wild ungulates to livestock grazing in the Zorkul Strictly Protected Area, Tajikistan. PLoS One 13:e0208329. doi: 10.1371/journal.pone.0208329
Khanal, G., Mishra, C., and Ramesh Suryawanshi, K. (2020). Relative influence of wild prey and livestock abundance on carnivore-caused livestock predation. Ecol. Evol. 10, 11787–11797. doi: 10.1002/ece3.6815
Kissui, B. M. (2008). Livestock predation by lions, leopards, spotted hyenas, and their vulnerability to retaliatory killing in the Maasai steppe. Tanzania. Anim. Conserv. 11, 422–432.
Li, J. (2012). Ecology and Conservation Strategy of Snow Leopard (Panthera unica) in Sanjiangyuan Area on the Tibetan Plateau. Ph.D. thesis. Beijing: Peking University.
Li, J., Weckworth, B. V., McCarthy, T. M., Liang, X., Liu, Y., Xing, R., et al. (2020). Defining priorities for global snow leopard conservation landscapes. Biol. Conserv. 241:108387.
Li, J., Xiao, L., and Lu, Z. (2016). Challenges of snow leopard conservation in China. Sci. China Life Sci. 59, 637–639. doi: 10.1007/s11427-016-5067-9
Li, J., Yin, H., Wang, D., Jiagong, Z., and Lu, Z. (2013). Human-snow leopard conflicts in the sanjiangyuan region of the Tibetan Plateau. Biol. Conserv. 166, 118–123.
Li, M., Zhang, X., Wu, J., Ding, Q., Niu, B., and He, Y. (2021). Declining human activity intensity on alpine grasslands of the Tibetan Plateau. J. Environ. Manag. 296:113198. doi: 10.1016/j.jenvman.2021.113198
Li, X.-L., Brierley, G., Shi, D.-J., Xie, Y.-L., and Sun, H.-Q. (2012). “Ecological protection and restoration in sanjiangyuan national nature reserve, qinghai province, China,” in Perspectives on Environmental Management and Technology in Asian River Basins, ed. D. Higgitt (Netherlands: Springer).
Liu, C., Zheng, S., and Ren, J. (2003). Research foods and foods source about snow leopard. J. Shaanxi Normal Univer. 31, 154–159. doi: 10.7589/0090-3558-43.3.512
Liu, Y., Weckworth, B., Li, J., Xiao, L., Zhao, X., and Lu, Z. (2016). “China: the Tibetan Plateau, sanjiangyuan region,” in Snow Leopards, eds T. McCarthy and D. Mallon (Amsterdam: Elsevier).
Lovari, S., and Mishra, C. (2016). “Liveing on the edge: depletion of wild prey and survival of the snow leopard,” in Snow Leopards: Biodiversity of the World: Conservation from Genes to Landscapes, eds T. McCarthy and D. Mallon (Cambridge: Academic Press), 69–76.
Lyngdoh, S., Shrotriya, S., Goyal, S. P., Clements, H., Hayward, M. W., and Habib, B. (2014). Prey preferences of the snow leopard (Panthera uncia): regional diet specificity holds global significance for conservation. PLoS One 9:e88349. doi: 10.1371/journal.pone.0088349
Mallon, D., Harris, R. B., and Wegge, P. (2016). “Snow leopard prey and diet,” in Snow Leopards. Biodiversity of the Wold: Conservation from Genes to Landscapes, eds T. McCarthy and D. Mallon (Cambridge: Academic Press).
McCarthy, T., Mallon, D., Jackson, R., Zahler, P., and McCarthy, K. (2017). The IUCN Red List of Threatened Species 2017: e.T22732A50664030. Available Online at: https://dx.doi.org/10.2305/IUCN.UK.2017-2.RLTS.T22732A50664030.en (accessed June 9, 2021).
Miehe, G., Miehe, S., Kaiser, K., Reudenbach, C., Behrendes, L., Duo, L., et al. (2009). How old is pastoralism in Tibet? an ecological approach to the making of a Tibetan landscape. Palaeogeogr. Palaeoclimatol. Palaeoecol. 276, 130–147.
Mijiddorj, T. N., Alexander, J. S., and Samelius, G. (2018). Livestock depredation by large carnivores in the South Gobi, Mongolia. Wildl. Res. 45, 237–246. doi: 10.1071/wr18009
Miller, D. J. (2000). Tough times for Tibetan nomads in Western China: snowstorms, settling down, fences and the demise of traditional nomadic pastoralism. Nomadic Peoples 4, 83–109.
Mishra, C. (1997). Livestock depredation by large carnivores in the Indian trans-himalaya: conflict perceptions and conservation prospects. Environ. Conserv. 24, 338–343.
Mishra, C., Allen, P., McCarthy, T., Madhusudan, M. D., Bayarjargal, A., and Prins, H. H. T. (2003). The role of incentive programs in conserving the snow leopard. Conserv. Biol. 17, 1512–1520. doi: 10.1111/j.1523-1739.2003.00092.x
Mishra, C., Redpath, S. R., and Suryawanshi, K. R. (2016). “Livestock predation by snow leopards: conflicts and the search for solutions,” in Snow Leopards: Biodiversity of the World: Conservation from Genes to Landscapes, eds T. McCarthy and D. Mallon (Cambridge: Academic Press), 59–67.
Mishra, C., Van Wieren, S. E., Ketner, P., Heitkonig, I. M. A., and Prins, H. H. T. (2004). Competition between domestic livestock and wild bharal Pseudois nayaur in the Indian Trans-Himalaya. J. Appl. Ecol. 41, 344–354.
Monterroso, P., Godinho, R., Oliveira, T., Ferreras, P., Kelly, M. J., Morin, D. J., et al. (2019). Feeding ecological knowledge: the underutilised power of faecal DNA approaches for carnivore diet analysis. Mammal Rev. 49, 97–112.
Oksanen, J., Guillaume Blanchet, F., Friendly, M., Kindt, R., Legendre, P., McGlinn, D., et al. (2019). vegan: Community Ecology Package. R package version 2.5-5.
Oli, M. K., Taylor, I. R., and Rogers, M. E. (1994). Snow leopard Panthera uncia predation of livestock: an assessment of local perceptions in the annapurna conservation area. Nepal. Biol. Conserv. 68, 63–68.
Pimenta, V., Barroso, I., Boitani, L., and Beja, P. (2018). Risks a la carte: modelling the occurrence and intensity of wolf predation on multiple livestock species. Biol. Conserv. 228, 331–342. doi: 10.1016/j.biocon.2018.11.008
Pompanon, F., Deagle, B. E., Symondson, W. O. C., Brown, D. S., Jarman, S. N., and Taberlet, P. (2012). Who is eating what: diet assessment using next generation sequencing. Mol. Ecol. 21, 1931–1950. doi: 10.1111/j.1365-294X.2011.05403.x
Pringle, R. M., and Hutchinson, M. C. (2020). Resolving food-web structure. Annu. Rev. Ecol. Evol. Syst. 51, 55–80.
R Core Team (2020). R: a Language and Environment for Statistical Computing. Vienna: R Foundation for Statistical Computing.
Riaz, T., Shehzad, W., Viari, A., Pompanon, F., Taberlet, P., and Coissac, E. (2011). ecoPrimers: inference of new DNA barcode markers from whole genome sequence analysis. Nucleic Acids Res. 39;e145. doi: 10.1093/nar/gkr732
Ripple, W. J., Estes, J. A., Beschta, R. L., Wilmers, C. C., Ritchie, E. G., Hebblewhite, M., et al. (2014). Status and ecological effects of the world’s largest carnivores. Science 343:1241484. doi: 10.1126/science.1241484
Rowcliffe, J. M., Field, J., Turvey, S. T., and Carbone, C. (2008). Estimating animal density using camera traps without the need for individual recognition. J. Appl. Ecol. 45, 1228–1236. doi: 10.1002/jwmg.902
Schaller, G. B. (1988). Status of the snow leopard Panthera uncia in qinghai and gansu provinces. China. Biol. Conserv. 45, 179–194.
Schnell, I. B., Bohmann, K., and Gilbert, M. T. P. (2015). Tag jumps illuminated-reducing sequence-to-sample misidentifications in metabarcoding studies. Mol. Ecol. Resour. 15, 1289–1303. doi: 10.1111/1755-0998.12402
Shao, X., Lu, Q., Xiong, M., Bu, H., Shi, X., Wang, D., et al. (2021). Prey partitioning and livestock consumption in the world’s richest large carnivore assemblage. Curr. Biol. 31, 1203–1203. doi: 10.1016/j.cub.2021.08.067
Shehzad, W., McCarthy, T. M., Pompanon, F., Purevjav, L., Coissac, E., Riaz, T., et al. (2012). Prey preference of snow leopard (Panthera uncia) in South Gobi, Mongolia. PLoS One 7:e32104. doi: 10.1371/journal.pone.0032104
Shrestha, B., Aihartza, J., and Kindlmann, P. (2018). Diet and prey selection by snow leopards in the Nepalese Himalayas. PLoS One 13:e0206310. doi: 10.1371/journal.pone.0206310
Suryawanshi, K. R., Bhatnagar, Y. V., and Mishra, C. (2012). Standardizing the double-observer survey method for estimating mountain ungulate prey of the endangered snow leopard. Oecologia 169, 581–590. doi: 10.1007/s00442-011-2237-0
Suryawanshi, K. R., Redpath, S. M., Bhatnagar, Y. V., Ramakrishnan, U., Chaturvedi, V., Smout, S. C., et al. (2017). Impact of wild prey availability on livestock predation by snow leopards. R. Soc. Open Sci. 4:170026. doi: 10.1098/rsos.170026
Symondson, W. O. C. (2002). Molecular identification of prey in predator diets. Mol. Ecol. 11, 627–641. doi: 10.1046/j.1365-294x.2002.01471.x
Tiwari, M. P., Devkota, B. P., Jackson, R. M., Chhetri, B. B. K., and Bagale, S. (2020). What factors predispose households in trans-Himalaya (Central Nepal) to livestock predation by snow leopards? Animals 10:2187. doi: 10.3390/ani10112187
Vallet, D., Petit, E. J., Gatti, S., Levréro, F., and Ménard, N. (2007). A new 2CTAB/PCI method improves DNA amplification success from faeces of Mediterranean (Barbary macaques) and tropical (lowland gorillas) primates. Conserv. Genet. 9, 677–680. doi: 10.1007/s10592-007-9361-8
van Eeden, L. M., Crowther, M. S., Dickman, C. R., Macdonald, D. W., Ripple, W. J., Ritchie, E. G., et al. (2018). Managing conflict between large carnivores and livestock. Conserv. Biol. 32, 26–34. doi: 10.1111/cobi.12959
Wang, Y. Z., Li, C., Wu, K. M., Wei, Y. W., and Guo, W. T. (2009). Species and geographical distribution of carnivores and small mammals in Sanjiangyuan region. Chines J. Vector Biol. Control 20, 589–590.
Weiskopf, S. R., Kachel, S. M., and McCarthy, K. P. (2016). What are snow leopards really eating? identifying bias in food-habit studies. Wildl. Soc. Bull. 40, 233–240.
Wilkinson, C. E., McInturff, A., Miller, J. R. B., Yovovich, V., Gaynor, K. M., Calhoun, K., et al. (2020). An ecological framework for contextualizing carnivore-livestock conflict. Conserv. Biol. 34, 854–867. doi: 10.1111/cobi.13469
Wolf, C., and Ripple, W. J. (2017). Range contractions of the world’s large carnivores. R. Soc. Open Sci. 4:170052.
Xiao, L. (2017). The Interaction Among Snow Leopards (Panthera uncia), Blue Sheep (Pseudois nayaur) and Livestock in Sanjiangyuan Region. Ph.D. thesis. Beijing: Peking University.
Xiao, L., Cheng, C., Wan, H., Zhang, D., Wang, Y., and Tsedan et al. (2019). Defining conservation priority areas of snow leopard habitat in the Sanjiangyuan Region. Biodivers. Sci. 27, 943–950.
Xiong, M., Shao, X., Long, Y., Bu, H., Zhang, D., Wang, D., et al. (2016). Molecular analysis of vertebrates and plants in scats of leopard cats (Prionailurus bengalensis) in southwest China. J. Mammal. 97, 1054–1064.
Xiong, M., Wang, D., Bu, H., Shao, X., Zhang, D., Li, S., et al. (2017). Next-generation sequencing-based dietary analysis of two sympatric felids in the temperate mountain forests of Southwest China. Sci. Rep. 7:41909.
Keywords: apparent mutualism, blue sheep, feeding habits, molecular dietary analysis, human-carnivore conflict, prey selection, Sanjiangyuan
Citation: Lu Q, Xiao L, Cheng C, Lu Z, Zhao J and Yao M (2021) Snow Leopard Dietary Preferences and Livestock Predation Revealed by Fecal DNA Metabarcoding: No Evidence for Apparent Competition Between Wild and Domestic Prey. Front. Ecol. Evol. 9:783546. doi: 10.3389/fevo.2021.783546
Received: 26 September 2021; Accepted: 09 November 2021;
Published: 30 November 2021.
Edited by:
Pavel Kindlmann, Charles University, CzechiaReviewed by:
Sona Varachova, Czech Academy of Sciences, CzechiaCopyright © 2021 Lu, Xiao, Cheng, Lu, Zhao and Yao. This is an open-access article distributed under the terms of the Creative Commons Attribution License (CC BY). The use, distribution or reproduction in other forums is permitted, provided the original author(s) and the copyright owner(s) are credited and that the original publication in this journal is cited, in accordance with accepted academic practice. No use, distribution or reproduction is permitted which does not comply with these terms.
*Correspondence: Meng Yao, eWFvbUBwa3UuZWR1LmNu; orcid.org/0000-0002-8906-1461
Disclaimer: All claims expressed in this article are solely those of the authors and do not necessarily represent those of their affiliated organizations, or those of the publisher, the editors and the reviewers. Any product that may be evaluated in this article or claim that may be made by its manufacturer is not guaranteed or endorsed by the publisher.
Research integrity at Frontiers
Learn more about the work of our research integrity team to safeguard the quality of each article we publish.