- 1Alfred Wegener Institute Helmholtz Centre for Polar and Marine Research, Polar Terrestrial Environmental Systems, Potsdam, Germany
- 2Institute of Biochemistry and Biology, University of Potsdam, Potsdam, Germany
- 3Institute of Environmental Sciences and Geography, University of Potsdam, Potsdam, Germany
- 4Institute of Natural Sciences, North-Eastern Federal University of Yakutsk, Yakutsk, Russia
Woody plants are expanding into the Arctic in response to the warming climate. The impact on arctic plant communities is not well understood due to the limited knowledge about plant assembly rules. Records of past plant diversity over long time series are rare. Here, we applied sedimentary ancient DNA metabarcoding targeting the P6 loop of the chloroplast trnL gene to a sediment record from Lake Ilirney (central Chukotka, Far Eastern Russia) covering the last 28 thousand years. Our results show that forb-rich steppe-tundra and dwarf-shrub tundra dominated during the cold climate before 14 ka, while deciduous erect-shrub tundra was abundant during the warm period since 14 ka. Larix invasion during the late Holocene substantially lagged behind the likely warmest period between 10 and 6 ka, where the vegetation biomass could be highest. We reveal highest richness during 28–23 ka and a second richness peak during 13–9 ka, with both periods being accompanied by low relative abundance of shrubs. During the cold period before 14 ka, rich plant assemblages were phylogenetically clustered, suggesting low genetic divergence in the assemblages despite the great number of species. This probably originates from environmental filtering along with niche differentiation due to limited resources under harsh environmental conditions. In contrast, during the warmer period after 14 ka, rich plant assemblages were phylogenetically overdispersed. This results from a high number of species which were found to harbor high genetic divergence, likely originating from an erratic recruitment process in the course of warming. Some of our evidence may be of relevance for inferring future arctic plant assembly rules and diversity changes. By analogy to the past, we expect a lagged response of tree invasion. Plant richness might overshoot in the short term; in the long-term, however, the ongoing expansion of deciduous shrubs will eventually result in a phylogenetically more diverse community.
Introduction
Recent warming has triggered vegetation changes in the Arctic (Wilson and Nilsson, 2009). Woody taxa have expanded their range further north (Kharuk et al., 2006; Pearson et al., 2013) albeit at a rather low rate compared to the corresponding increase in temperature (Kruse et al., 2018). Patterns of taxonomic richness are one of the indicators for biodiversity monitoring of arctic plants (Khitun et al., 2016). Richness reflects the extinction and colonization of all taxa in a community, and thus shows a more general signal compared to the response of individual taxa. Some modern observations suggest an increased richness in the arctic tundra related to tree expansion under a warming climate (Rupp et al., 2001; Danby et al., 2011), whereas other studies suggest a decline (Walker et al., 2006).
The increase in richness could result from ecological facilitation, which brings mutual beneficial interactions between trees or supporting the establishment of other plants (Carcaillet et al., 2012), whereas the decline of richness would result from competition or inhibition from trees to herbs and forbs (Mod et al., 2016). Patterns of phylogenetic diversity are of interest as they can indicate changes in assembly rules under warmer and colder climate conditions. Low phylogenetic diversity reflects the co-occurrence of genetically close species. In contrast, a community with high phylogenetic diversity hosts an assembly of genetically distant spe cies (Webb, 2000; Webb and Pitman, 2002). On the one hand, phylogenetically more varied plant species coexist when more soil nutrients are available as a result of warming (Chu and Grogan, 2010; Zhu et al., 2020). On the other hand, climate warming may decrease phylogenetic diversity (Thuiller et al., 2011) through environmental filtering due to drought (Dorji et al., 2012). Accordingly, the major rules of the assembly process under the ongoing warming are not well understood, which makes it difficult to predict the future vegetation composition as well as taxonomic and phylogenetic diversity.
Conventional studies of vegetation history are mostly based on pollen analysis (Moser and MacDonald, 1990; Anderson and Brubaker, 1994; Seppä et al., 2002; Andreev et al., 2011). Many Eurasian pollen records show lower palynological richness during the cold and dry Late Glacial (transition from the Last Glacial to the Holocene, approximately 14–10 ka) compared with the warm and wet Holocene (Feurdean et al., 2011; Chytrý et al., 2017). However, some studies indicate a relatively high pollen-taxa diversity during the Late Glacial (Blarquez et al., 2014; Reitalu et al., 2015) and a decreasing diversity during the Holocene (Blarquez et al., 2014). Sedimentary pollen records from north-eastern Europe show that climate warming led to an increase in overall plant richness, but a decrease in phylogenetic diversity during the post-glacial period (Reitalu et al., 2015). Our knowledge about past changes in phylogenetic diversity is still very scarce, especially for Siberia, and thus our understanding of plant assembly rules in terms of warming is insufficient.
Knowledge gaps about millennial-scale composition and diversity changes may, to some extent, arise from the limited taxonomic resolution and taphonomic peculiarities of pollen records. For example, Larix, the treeline-forming genus in most of Siberia is poorly reflected in pollen records (Sjögren et al., 2008; Binney et al., 2011; Niemeyer et al., 2017). Another restriction of pollen records is that they reflect regional vegetation signals instead of local signals. Pollen records from a European subalpine lake reported increased richness in vegetation which disperses over long distances, while macroremain assemblages analyzed from the same core displayed decreased richness, probably resulting from the reduced tree-cover (Blarquez et al., 2013). Plant DNA preserved in lake sediments can be a reliable proxy for vegetation composition and diversity (Bálint et al., 2018). Modern studies have confirmed that sedimentary DNA from high-latitude lakes reliably reflect the vegetation surrounding the lake (Niemeyer et al., 2017; Alsos et al., 2018) and can be used to trace temporal changes in plant diversity (Zimmermann et al., 2017; Parducci et al., 2019; Liu et al., 2020; Rijal et al., 2021). The universal, plant-specific and short barcode marker of the P6 loop of the chloroplast is used for sedimentary ancient DNA (sedaDNA) studies (Taberlet et al., 2007).
Plant DNA metabarcoding has revealed that Siberian plant diversity was highest during pre-Last Glacial Maximum (pre-LGM, 50–25 ka) and lowest during the Last Glacial Maximum (LGM, 25–15 ka) (Willerslev et al., 2014; Epp et al., 2018; Clarke et al., 2019). This was speculatively related to changes in forb abundance and the impact of megafauna (Willerslev et al., 2014). However, Clarke et al. (2019) hypothesize that the growth of shrubs during the Late Glacial led to competition between arctic taxa, resulting in reduced taxon diversity. Our study investigates the plant assembly rules by assessing changes in vegetation composition as well as taxonomic and phylogenetic diversity as inferred from sedaDNA from Lake Ilirney (Chukotka, Far East Russia) since 28 ka. We aim (1) to reconstruct vegetation composition changes; (2) to investigate changes in taxonomic alpha diversity and its relationship with vegetation compositional changes, particularly during woody taxa invasion; and (3) to explore changes in phylogenetic diversity and how this reflects vegetation composition and taxonomic diversity.
Materials and Methods
Study Area
Lake Ilirney (67°21′N, 168°19′E, 421 m a.s.l.) is a glacially formed lake located in the autonomous region of Chukotka (Far East Russia, Figure 1). The lake is bounded by the Anadyr Mountains (up to 1,790 m a.s.l.) to the north. According to the meteorological station at Ilirney, mean annual temperature is −13.5°C, and mean January and July temperatures are −33.4 and 12.1°C, respectively. Annual precipitation is ∼200 mm (Menne et al., 2012). As an area with unique vegetation composition that harbors many endemic species (Kuzmina et al., 2011), Chukotka is a hotspot for biodiversity in the Russian Arctic. It is a key region for understanding both Quaternary glaciation history and transcontinental species migrations as it lies at the far eastern edge of Asia bordering the Bering Sea (Kuzmina et al., 2011), which is known for being a glacial refugium during the LGM (Brubaker et al., 2005). Chukotka is characterized by a comparatively pristine environment including wild herbivore mammals which have survived human impact.
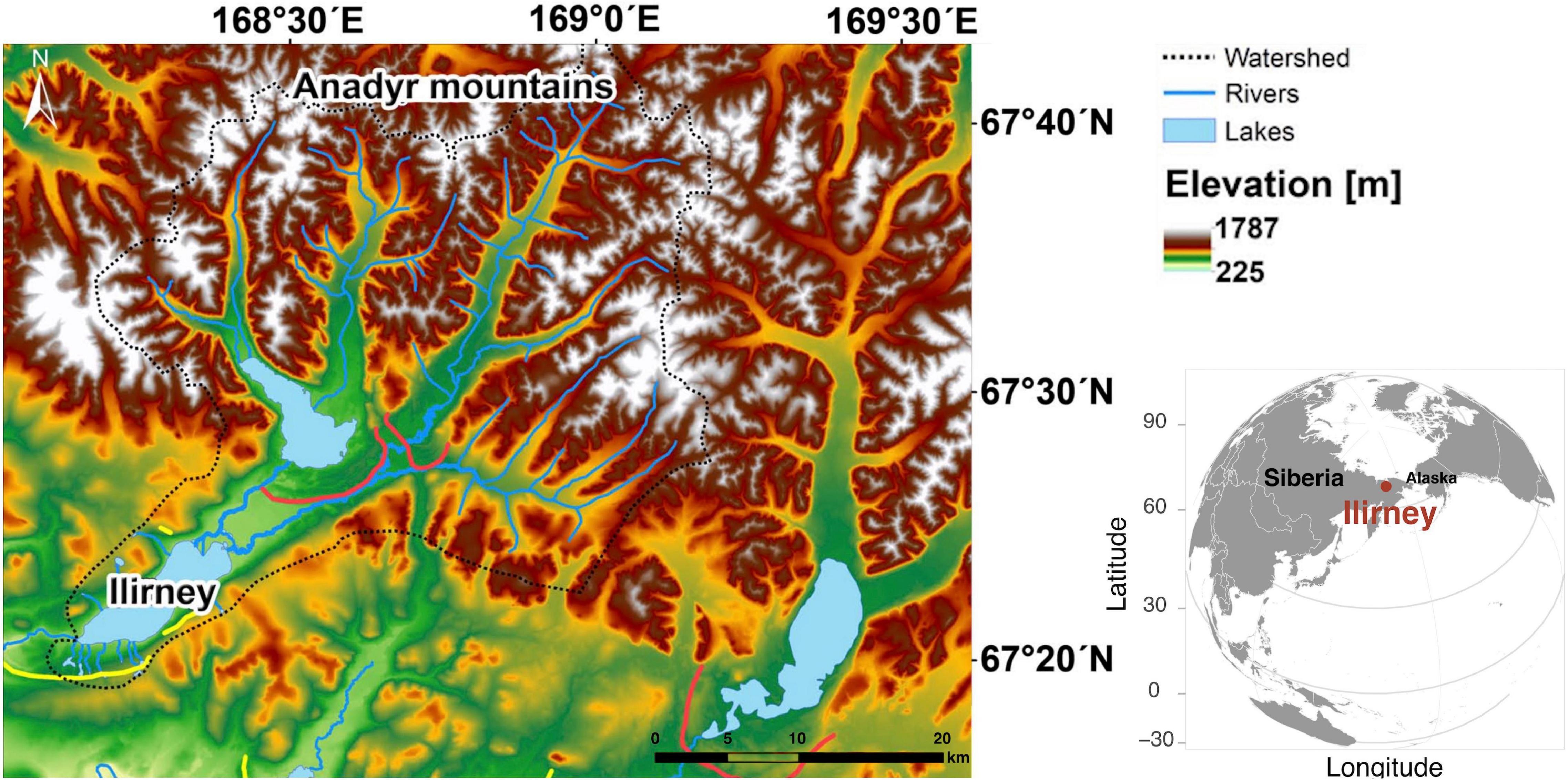
Figure 1. Overview of Ilirney area in the Russian Arctic and the study location. The figure is adapted from Vyse et al. (2020).
Lake Ilirney is situated at the tundra-taiga ecotone, which is characterized by a mixture of forest tundra, shrub tundra, and open tundra. Stands of Larix cajanderi are common in the lake vicinity as well as low and dwarf shrubs such as Salix spp., Betula nana, Ledum palustre, Vaccinium spp., and Empetrum nigrum. Elevational transitions between forest tundra and open tundra are often covered by patchy dwarf pine (Pinus pumila). Open tundra on the gentle slopes (540–700 m a.s.l) is graminoid-rich including hummock (Eriophorum vaginatum) and non-hummock (Carex spp.) tundra with dense moss cover in the ground layer. Intermediate elevations (700–900 m a.s.l.) are dominated by Dryas octopetala and lichens, accompanied by herbs from the Fabaceae, Orobanchaceae, Poaceae, Rosaceae, and Saxifragaceae. The vegetation at higher elevations becomes barren.
Fieldwork and Sampling
Sediment core “16-KP-01-L02” was obtained from Lake Ilirney (67.34148, 168.30443) in summer 2016. The maximum water depth is 44 m in the southwestern basin and 25 m in the northeastern basin (Vyse et al., 2020). The coring was accomplished using a modified UWITEC gravity coring device deployed from a catamaran. We used a cable winch fixed on the catamaran to support a precise position of the coring (Andreev et al., 2021). The 235 cm-long sediment core was cut into 1-m-long pieces and transferred to the Alfred Wegener Institute for Polar and Marine Research (AWI) in Potsdam, Germany where it was stored at 4°C prior to sub-sampling.
The core segments were cut into two halves. One was stored as an archive at 4°C and the other was opened for subsampling. The core subsampling was performed in the climate chamber of the Helmholtz Centre Potsdam - German Research Centre for Geosciences (GFZ). The subsampling environment was under strict hygienic rules to prevent contamination with modern DNA. The surface in the climate chamber was cleaned with DNA Exitus Plus™ (VWR, Germany) and purified water. All sampling tools were cleaned regularly when taking each sample (Champlot et al., 2010). Sediment at either end of each core section was removed as they were in contact with the plastic tube and considered unsterile (Parducci et al., 2017). DNA samples were taken every 2 cm with 5 mL disposable syringes, in which the anterior caps were previously cut off with sterile scalpels. In total, 58 DNA samples were collected into 8 mL sterile tubes (Sarstedt) across the entire core. The collected samples were then stored at −20°C.
Dating and Chronology
The age-depth model of core 16-KP-01-L02 has been published in Andreev et al. (2021). It is based on Accelerator Mass Spectrometry (AMS) radiocarbon dating of seven bulk total organic carbon samples (Supplementary Table 1). The samples were 14C dated in Mini Carbon Dating System (MICADAS) laboratory at AWI Bremerhaven (Germany). Alternative dating techniques were applied to constrain the old carbon reservoir effect (Andreev et al., 2021). The ages were modeled using the package “Rbacon” (Blaauw and Christen, 2011) in R 2.4.11 using the IntCal13 calibration curve (Reimer et al., 2013). To have a robust chronology of Lake Ilirney, the age model of our core was compared with the age model of another sediment core from the same lake. This core is named EN18208 and its age model has been published by Vyse et al. (2020) with a higher resolution. The two cores have a distance of approximately 250 m and have highly homogenous sediment patterns based on acoustic profiles (Andreev et al., 2021). Thus the modeled calibrated ages from core EN18208 were transferred to core 16-KP-01-L02 (Vyse et al., 2020). The age-depth plot, calibrated and modeled ages, and correlation between two cores based on the data derived from X-ray fluorescence analysis are presented in Supplementary Figure 1.
Genetic Laboratory Works
DNA isolation and polymerase chain reaction (PCR) setup were performed in the paleogenetic laboratory of AWI in Potsdam, Germany. This lab is dedicated to ancient DNA environment and is located in a building devoid of any modern molecular genetic work. For hygiene maintenance, the lab is equipped with an antechamber and separate rooms and individual UV-working station for DNA extraction and PCR set-up. Generally, the lab is cleaned by nightly UV-irradiation with ceiling UV-lamps.
DNA Extraction
The 58 samples selected for DNA extraction range from 1 to 235 cm depth. DNA isolation was performed using the DNeasy PowerMax Soil Kit (Qiagen, Germany). The DNA extraction was divided into 10 batches. To check for chemical contamination, one extraction blank was included for each extraction batch. Approximately 3 g of sediment sample, 15 mL bead solution, 1.2 mL C1 buffer, 400 μL of 2 mg/L proteinase K (VWR International) and 100 μL of 5 M dithiothreitol were prepared in a bead tube for each sample. The samples were incubated at 56°C in a rocking shaker overnight. The subsequent steps were carried out according to the instructions of the manufacturer by Qiagen, and the final volume of the isolated DNA was 2 mL. DNA concentration was measured with Qubit 4.0 fluorometer (Invitrogen, Carlsbad, CA, United States) using the Qubit dsDNA BR Assay Kit (Invitrogen, Carlsbad, CA, United States). Depending on the initial DNA concentrations we purified a volume of 600 or 1,000 μL using the GeneJET PCR Purification KIT (Thermo Scientific, Carlsbad, CA, United States) and eluted twice with 15 or 25 μL elution buffer, respectively. Then we repeated DNA concentration measurement with Qubit. Finally, DNA was diluted to 3 ng/μL. The DNA extracts and aliquots were stored at −20°C.
Polymerase Chain Reaction
Polymerase chain reaction protocols were set up with plant-specific g and h primers of the trnL gene (Taberlet et al., 2007). To distinguish the samples after sequencing, both forward and reverse primers were modified on the 5′ end by adding eight unique randomized nucleotides (Binladen et al., 2007). The primers were elongated by three additional unidentified bases (NNN) for improving cluster detection on Illumina sequencing platforms (Barba et al., 2014). The PCR setup contained 1.25U Platinum ® Taq High Fidelity DNA Polymerase (Invitrogen, United States), 1 × HiFi buffer (Invitrogen, United States), 2 mM MgSO4, 0.25 mM mixed dNTPs, 0.8 mg Bovine Serum Albumin (VWR, Germany) 0.2 mM of each primer and 3 μL of DNA template (with a concentration of 3 ng/μL).
All Post-PCR steps were conducted in a dedicated Post-PCR area located in a different building and strictly separated from the paleogenetic laboratories. PCRs were run in the Post-PCR area and were performed in a TProfessional Basic Thermocycler (Biometra, Germany). The PCRs were carried out in three replicates using different primer tag combinations. Twenty-one PCR reactions were performed, and 9–11 sediment samples were included in each reaction. PCR no template control (NTC) and the DNA extraction blank (blank) were run alongside each reaction. In total there were 216 PCR products including replicates, NTCs, and extraction blanks. The expected amplification products were validated by 2% agarose (Carl Roth GmbH and Co. KG, Germany) gel electrophoresis.
Purification, Pooling, and Sequencing
The PCR products were purified using the MinElute PCR Purification Kit (Qiagen, Germany), following the kit manufacturer’s instructions. The purified PCR products were eluted to a final volume of 20 μL. The DNA concentrations were quantified with the Qubit ® dsDNA BR Assay Kit (Invitrogen, United States) using 1 μL of the purified DNA. All samples were then pooled equimolarly to a final concentration of approximately 60 ng in 1,711.80 μL. NTCs and extractions blanks were standardized to a volume of 10 μL and were also included in the pool. Pools were sent to by Fasteris SA sequencing service (Switzerland), who prepared the DNA library following the Metafast PCR-free protocol and performed parallel high-throughput paired-end (2 × 150 bp) amplicon sequencing on the Illumina NextSeq 500 platform (Illumina Inc.). The sequencing at Fasteris was done in parallel with other sequencing projects on the same flow cell, but none of which targeted plant DNA amplifications.
Processing the Sequence Data
Sequence data were processed using the OBITools package (Boyer et al., 2016). The raw forward and reverse reads were assembled to a single file using illuminapairedend. Based on an exact match to the tag combinations, the sequences were assigned to samples using ngsfilter. Identical sequences were then deduplicated using obiuniq. Sequences with less than 10 read counts were removed using obigrep to reduce possible artifacts. As a final denoising step, obiclean was used to exclude further sequence variants that likely originate from PCR and sequencing errors (Boyer et al., 2016). The reference used for taxonomic assignment is based on the curated Arctic and Boreal vascular plant and bryophytes database. It contains 1,664 vascular plants and 486 bryophyte species (Willerslev et al., 2014; Soininen et al., 2015). The processed sequences were assigned using ecotag by searching for possible matches within the reference library.
Further Sequence Data Filtering
To have a reliable taxonomic assignment of the sequences but not to overestimate the diversity, the data were further filtered (Supplementary Table 2). Blanks and NTCs accounted for 1,115,522 reads (2.5% of the data before filtering). More than 80% of those reads were assigned to Dryas and Saliceae. They occurred in the blanks of three PCR batches (PCR batch 01, 02, and 21 in Supplementary Table 3), with the reads two to three levels of magnitude lower than the reads in the samples. The affected samples are between 28 and 23 ka. However, we kept the data from the two contaminated batches, as we identified the counts in the rest of the samples from these batches that have similar patterns as their corresponding replicates. After excluding extraction blanks and NTCs, the PCR replicates were summed up. Finally, we excluded sequences that were assigned to submerged aquatics and bryophytes. To obtain normalized count data, the true counts were rarefied 100 times based on the minimum number of counts (84,343) across the samples2. The true and rarefied data can be found in Supplementary Data 1.
Statistical Analyses
Statistical analyses were computed in R 3.6.03. Relative count proportions of taxa within a sample were calculated to visualize taxa abundance for all ages. We applied a double-square root transformation on the relative proportions of the sedaDNA dataset to mitigate the effect of overrepresented and rare sequences (Zimmermann et al., 2017), followed by a Chord transformation using method = “norm” in decostand (Legendre and Borcard, 2018). Analyses were performed in the “vegan” and “rioja” packages (Juggins, 2009). The transformed proportions were converted to Euclidean dissimilarity distances by vegdist. Hierarchical clustering was CONISS constrained using the function chclust. Zonation was evaluated by the broken-stick model using bstick. Detrended correspondence analysis (DCA) was applied to the dataset using decorana. Results showed that a length of the first DCA axis < 3, suggesting linear relationships among variables (ter Braak and Šmilauer, 2002). The principal component analysis (PCA) was then calculated based on covariance matrix (scale = FALSE). We used rda and restricted to those taxa that occur at a minimum proportion of 0.2% and in at least three samples.
Richness was calculated for each of the rarefied datasets and then averaged. However, richness can depend on how rare taxa are. It is highly sensitive to sampling effort and relative abundance (Roswell et al., 2021). Therefore, Hill’s N1 and N2 (Hill, 1973) have been considered, as they both explicitly include the relative abundance in the calculation (Roswell et al., 2021). They were calculated for each rarefied dataset using the function diversity in “vegan” and then averaged. The classic Shannon and Simpson indexes do not measure the same quantities (Roswell et al., 2021). They also have different scales in terms of species gain and loss (Tuomisto, 2010). One solution to overcome this is to use the diversity metric developed by Hill (1973), which calculates the exponential of Shannon’s index as Hill’s N1 and the inverse of Simpson’s index as Hill’s N2. They depend on the exponent, which can vary from counting all species equally to emphasizing the species that are most common (Roswell et al., 2021).
In the above formulars for Hill’s N1 and N2 (Hill, 1973), S = number of species in the sample S equals to the number of species in the sample and
Evenness was calculated as Hill’s N1 divided by log(richness). Error bars are the minimum and maximum values of the rarefied data.
The taxonomic uniqueness and the taxa that contribute the most to the compositional differences were evaluated by local contribution to beta diversity (LCBD) and species contribution to beta diversity (SCBD). They were calculated using beta.div and the Euclidean method in the “adespatial” package (Dray et al., 2017) in both abundance- and occurrence-based data.
In the above formulars for LCBD and SCBD (Legendre and Cáceres, 2013), s represents an n × p rectangular matrix. We use index i for sampling units and index j for species. yij means an individual value in a data table containing the presence-absence or the abundance values of p species (column vectors y1, y2,…, yp) observed in n sampling units (row vectors x1, x2, … xn), and sij is the square of the difference between the yij value and the mean value of the corresponding jth species:
The phylogenetic distances were retrieved from the implemented mega-trees of vascular plants in the “V.Phylomaker” package (Jin and Qian, 2019). Extracted genetic distances were used to calculate the phylogenetic alpha diversity and beta diversity. For visualization of phylogenetic relatedness, we calculated a phylogenetic tree including plant taxa involved in our study using the function phylo.maker and the “scenario 1” approach (Jin and Qian, 2019). The following phylogenetic analyses were performed in the “picante” package (Kembel et al., 2010). We supplied the phylogenetic tree with the plant proportion data using match.phylo.comm. The cophenetic distance was calculated using cophenetic. The net relatedness index (NRI) and nearest taxon index (NTI) were used to quantify the degree of phylogenetic relatedness (Webb, 2000). We created 999 null communities by randomly assembling communities using an “independent swap” algorithm. The null communities were used to compare the standardized effect sizes of mean phylogenetic distance (MPD) and mean nearest phylogenetic taxon distance (MNTD) to their observed communities implemented with ses.mpd and ses.mntd, respectively. We obtained the NRI by multiplying the estimates of MPD (mpd.obs.z) by −1 and NTI by multiplying the estimate of MNTD (mntd.obs.z) by −1. A significant and positive NTI or NRI indicates the phylogenetic clustering of the taxa occurs. In contrast, a significant and negative NTI or NRI indicates the phylogenetic overdispersion in a community (Webb, 2000).
We calculated the inter-community mean pairwise distance using the function comdist. The phylogenetic local contribution to beta diversity (pLCBD) was estimated using the function LCBD.comp in the “adespatial” package (Dray et al., 2017). A high pLCBD value corresponds to the phylogenetic uniqueness of the taxa in an assemblage compared to other assemblages. The Pearson’s correlation between species composition, taxonomic, and phylogenetic diversity was calculated using corr.test in the “psych” package (Revelle, 2018).
Results
Overview of the Sequencing Data and Taxonomic Composition
The final dataset of 58 samples contains 21,697,725 reads and 158 amplicon sequence variants (ASVs) with 100% identify to reference taxa. Twelve ASVs are identified to the family including subfamily level, 79 ASVs are identified to genus or tribe and subtribe level, and 67 to species level. Aside from a few outliers, the number of reads per sample is of the same order of magnitude. The first, second and third quartiles of the reads are 261,465, 319,778, and 467,465, respectively. 79.5% of the total counts are from shrubs and dwarf shrubs and 20.4% are from herbaceous plants. Picea, Larix, Populus, and Ulmus are the only tree taxa in our dataset and occur with low counts.
According to the clustering and zonation results, the plant assemblage referred by aDNA can be divided into three zones (Figure 2). There are 19 unique taxa in Zone 1 (28–19 ka) and 21 in Zone 3 (14–0 ka) (Supplementary Table 4). No unique taxa are detected in Zone 2 (18–14 ka). Zone 1 features prevalent and diverse dwarf shrubs and herbaceous plants, including Cassiope tetragona, Dryas sp., Papaver sp., Anthemideae 1, and Saxifraga sp. 2. Saxifragaceae and Asteraceae are the most diverse families in Zone 1. Saliceae and Betulaceae show strong between-zone variation by increasing greatly from Zone 1 to Zone 2. There is an overall reduction of plant taxa in Zone 2. Despite harboring 76% of the taxa seen in the LGM, Zone 3 is dominated by woody taxa from the Salicaceae, Betulaceae, and Ericaceae families. Shrubs such as Alnus alnobetula, Populus, and Ribes start to become dominant at 11 ka, with Alnus alnobetula showing a high relative abundance between 9 and 6 ka. There is a noticeable rise in the diversity of dwarf shrubs in Zone 3. In total, 87% (137 taxa) are detected during 28–14 ka, of which 73% (100 taxa) persist through the expansion of the coverage of dwarf and erect shrubs during 14–0 ka, and only 56% (88 taxa) remain during the maximum expansion of shrubs and trees (9–6 ka).
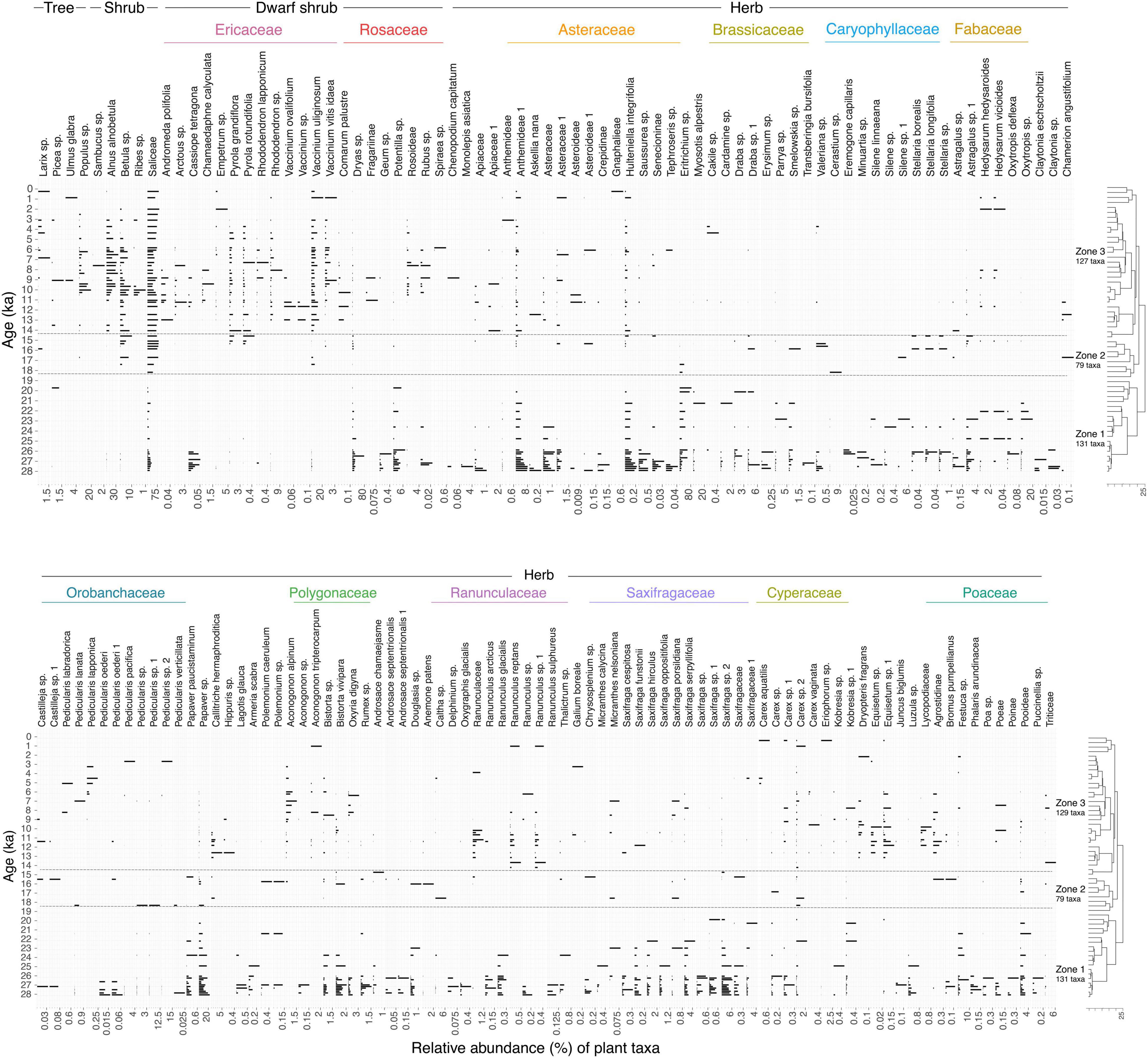
Figure 2. Stratigram of taxa recovered from sedimentary ancient DNA samples showing the relative proportions as a percentage of the plant taxa in each sample (horizontal bars). The plot is split into the upper and lower parts. Zones are derived based on a depth-constrained CONISS cluster analysis. Numbers of plant taxa occurring in each zone are indicated.
The PCA biplot (Figure 3) of the first and second PC axes explain, respectively, 39.7% and 8.0% of the variance in the dataset. The ordination shows a clear separation of samples older and younger than 14 ka. Samples from 28 to 14 ka are mostly dispersed toward the positive end of the PC1 axis, with the highest loading on PC1 given by Eritrichium sp. and Papaver sp. Along the positive PC1 axis, samples since 14 ka form a cluster, characterized by high values of Alnus alnobetula and Vaccinium uliginosum.
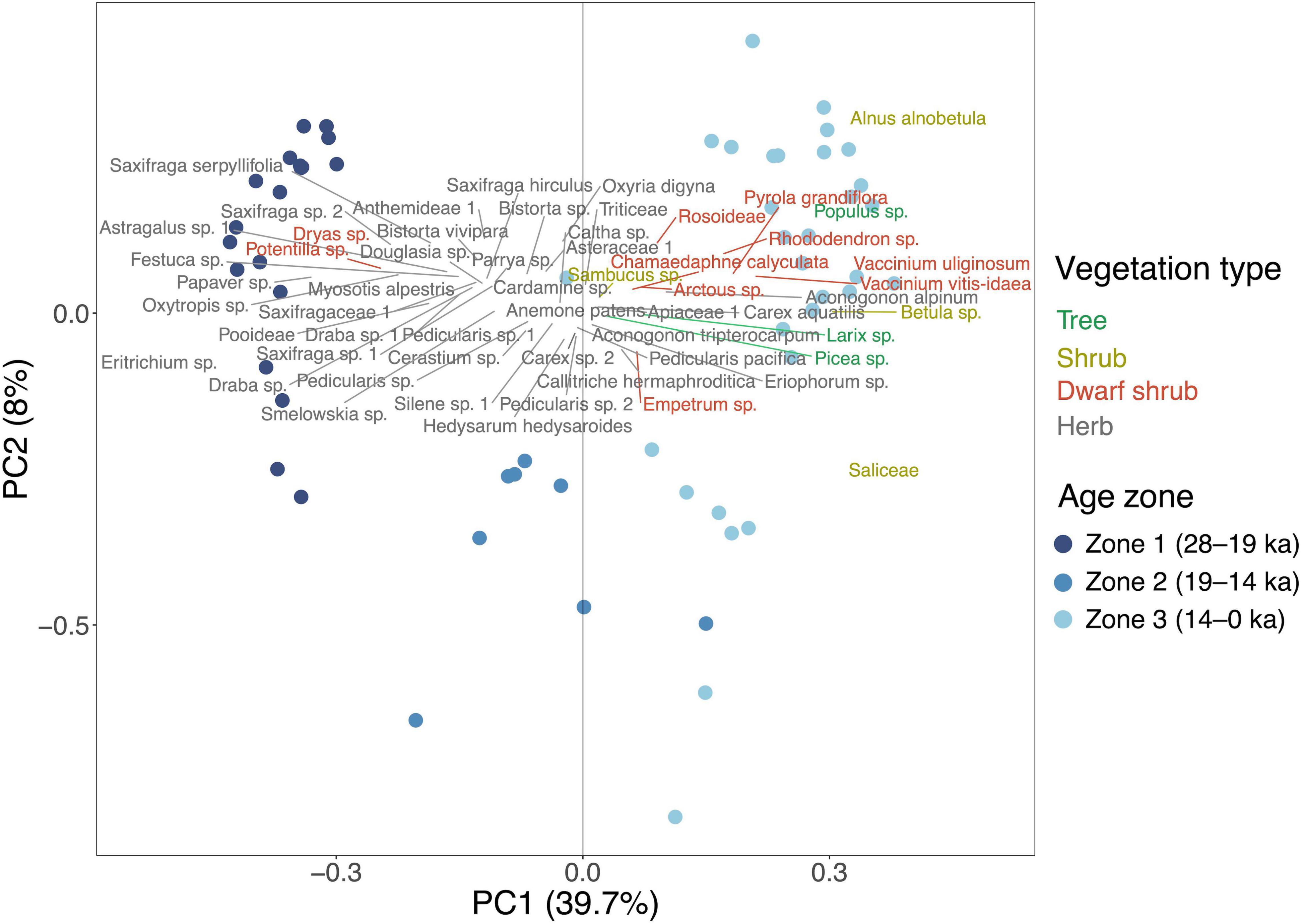
Figure 3. Principal component analysis (PCA) biplot of plant taxa from sedimentary ancient DNA, showing a subset of the dominant taxa (maximum relative abundance > 20% and occurrence > 3) which explain most of the variance in the dataset. Sample ages are color-graded based on zones, which are estimated by a depth-constrained CONISS cluster analysis.
Taxonomic Alpha and Beta Diversity
We infer the highest species richness in the plant assemblages from 28 to 23 ka and a second richness peak during 13–9 ka (Figure 4). The richness of every single repetition of the experiment is similar and has a significant positive correlation to the one using summed repeats (Supplementary Figure 3 and Supplementary Table 5), indicating that the difference in the PCR replicates has minor influence on the diversity in our data. Overall, we find almost no relationship between the rarefied richness and the number of reads based on the un-rarefied data (r = −0.26, p = 0.05), although the lowest read numbers during the post-LGM co-occur with the lowest richness. Similar to richness, the diversity indices and evenness have high values before 21 ka (Figure 4), but the second peaks of diversity and evenness lag the second richness peak by 3,000 years (9–6 ka).
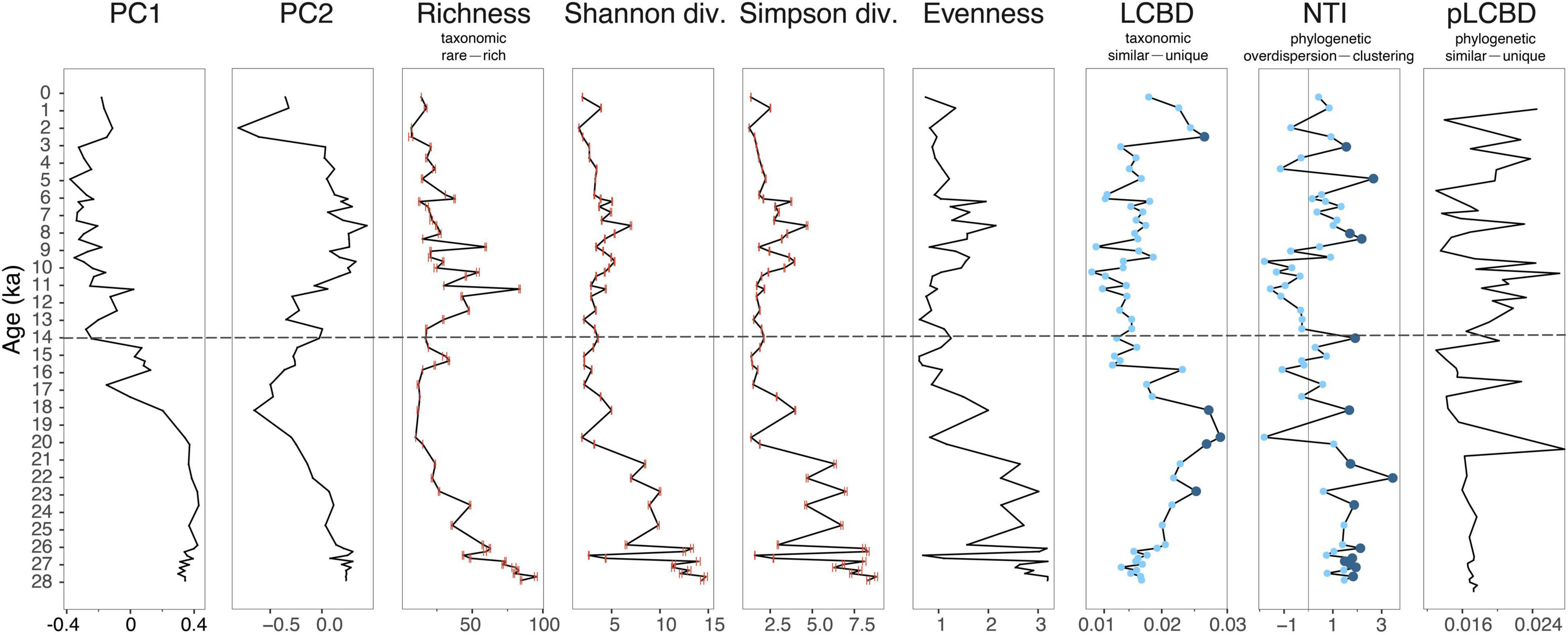
Figure 4. Plant assemblage composition and taxonomic and phylogenetic diversity derived from sedimentary ancient DNA. The measurements from left to right are first and second axes of a principal component analysis (PC1 and PC2), taxonomic richness, Hill’s N1 (exponential of Shannon diversity), Hill’s N2 (inverse Simpson diversity) and evenness, abundance-weighted local contributions to beta diversity (LCBD), abundance-weighted nearest taxon index (NTI), and phylogenetic abundance-weighted local contributions to beta diversity (pLCBD). Maximum and minimum values of the 100 resampling runs are indicated as error bars in red. Significance of NTI and LCBD are colored in dark blue when the values are significant (p < 0.05) and light blue when the values are not significant (p > 0.05).
The abundance-weighted LCBD (Figure 4) indicate that plant populations are taxonomically unique during 24–18 ka as well as from 3 to 2 ka. Eritrichium sp., Saliceae, and Alnus alnobetula contribute most to beta diversity (Supplementary Figure 4A). In contrast, the occurrence-based LCBD (Supplementary Figure 2) indicate significant uniqueness during 28–23 and 13–9 ka, that is, indicating times of high richness.
Phylogenetic Alpha and Beta Diversity
The phylogenetic tree (Figure 5) enables an estimation of phylogenetic diversity indices. The abundance- (Figure 4) and occurrence-based NTI, as well as NRI (Supplementary Figure 2), show overall similar patterns. Most of the NTI values are statistically significant and positive (p < 0.05) between 28 and 21 ka and between 9 and 5 ka in both abundance- and occurrence-based data, indicating a phylogenetic clustering in the plant assemblages during these periods. Although not significant, the NTI values are lowest and negative during 14–10 ka, suggesting a tendency of phylogenetic dispersion during that period.
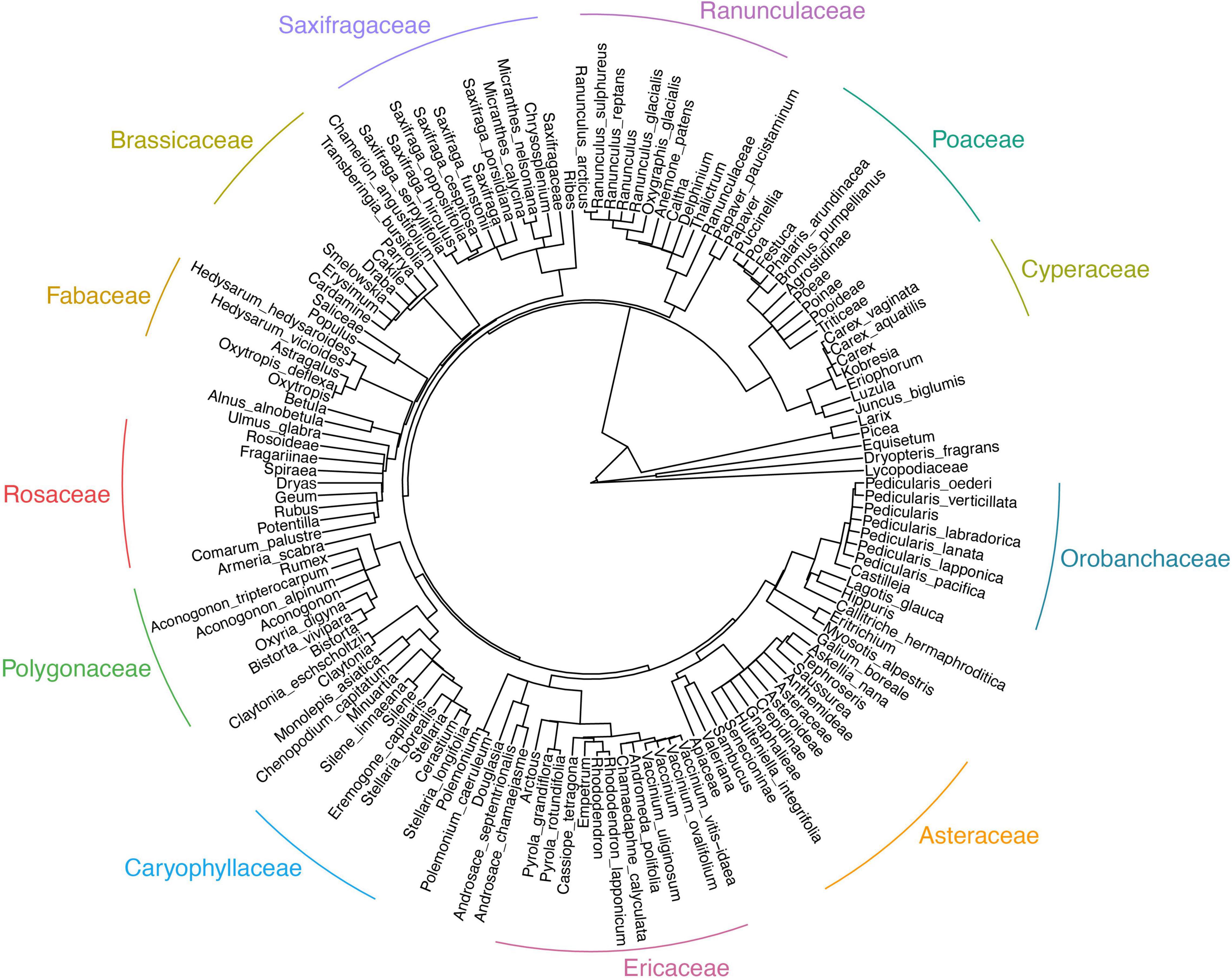
Figure 5. Phylogenetic tree of plant taxa from sedimentary ancient DNA, demonstrating the evolutionary relationship of the presented plant taxa from subarctic Far East Russia covering the last 28 thousand years.
Both abundance- (Figure 4) and occurrence-based (Supplementary Figure 2) pLCBD have in general higher values during 14–0 than 28–0 ka (0.016 ± 0.0027 during 28–14 ka and 0.018 ± 0.0037 during 14–0 ka in abundance-based pLCBD; 0.015 ± 0.0036 during 28–14 ka and 0.019 ± 0.0058 during 14–0 ka in occurrence-based pLCBD). This suggests that the 14–0 ka period tend to contribute more strongly to the phylogenetic beta diversity and the plant assemblages have higher phylogenetic uniqueness at that time.
Relationship Between Taxonomic Composition and Phylogenetic Diversity
The correlation of indices for phylogenetic alpha diversity, taxonomic composition, and phylogenetic beta diversity of the abundance- and occurrence-based data are summarized in Table 1. In addition to the correlation over the last 28 k years, we report the results for the 28–14 and 14–0 ka periods.
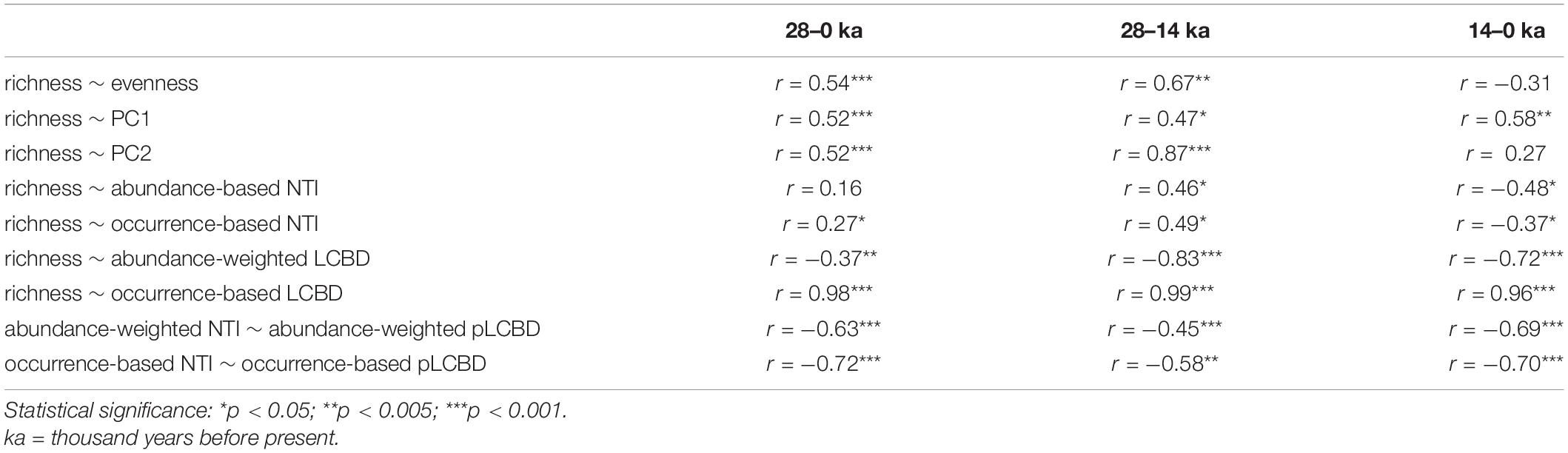
Table 1. Pearson’s correlation between plant assemblage composition and taxonomic and phylogenetic diversity in the records inferred from sedimentary ancient DNA (sedaDNA) from subarctic Far East Russia.
We merged the 28–19 and 19–14 ka zones as the cold and dry climate during 19–14 ka is more closely aligned to the climate during 28–19 ka (Andreev et al., 2021). Such a division makes the sample numbers between the two periods are more even (27 samples for 28–14 ka; 32 samples for 14–0 ka). There are 137 taxa in the records of 28–14 ka, where 40.9% (56 taxa) are identified to species level and 92% (126 taxa) are at genus including below genus levels. Similarly, there are 129 taxa in the records of 14–0 ka, where 39.5% (51 taxa) are identified to species level and 90.7% (117 taxa) are at genus including below genus levels.
NTI values are positively correlated with richness during 28–14 ka (r = 0.46, p < 0.05) but are negatively correlated with richness during 14–0 ka (r = −0.48, p < 0.05). This indicates that rich plant assemblages are associated with closely related species during the cool period of 28–14 ka, but with more distantly related species afterward. Species richness is negatively related to the abundance-weighted LCBD (r = −0.37, p < 0.005) for the whole record, whereas a strong positive relationship is found between species richness and occurrence-based LCBD (r = 0.98, p < 0.001). This suggests that the rich plant assemblages are taxonomically unique only in the incidence data. When the abundance-weight of the species is considered, rich assemblages are taxonomically even.
Nearest taxon index is negatively associated with pLCBD in both abundance- and occurrence-based data since 28 ka (p < 0.001), suggesting that the phylogenetically unique assemblages are more common within genetically divergent species.
Discussion
Vegetation History Revealed by Sedimentary Ancient DNA
Our results indicate that forb-dominated steppe tundra occurred from 28 to 19 ka and was followed by Saliceae-shrub tundra during 19–14 ka. Since 14 ka, Ericaceae dwarf-shrubs expanded in the area, and the lowlands of the Lake Ilirney catchment were covered by deciduous erect shrubs including Betula and Alnus. By mapping the above-ground biomass of recent and historical times in central Chukotka, regions with more erect shrubs are found with the highest above-ground biomass (Shevtsova et al., 2020, 2021). Based on this correlation, the erect shrubs in our records likely reached a maximum density during 10–6 ka. Since the mid-Holocene, open Larix forest expanded, several thousand years later than the maximum vegetation density and warming.
Zone 1 (28–19 ka) is characterized by forb-dominated steppe. Forbs such as Asteraceae, Saxifragaceae, Dryas, and Eritrichium (Boraginaceae) are common in the lake’s vicinity. This agrees with other ancient DNA-based studies in south-eastern Siberia (Courtin et al., 2021) and west Beringia of similar age (Willerslev et al., 2014). Our results suggest a dominant role for Eritrichium during the glacial period. As an alpine cushion nurse plant, Eritrichium may have facilitated diversity under harsh environments through interactions with other species (Cavieres and Badano, 2009). Shrubs such as Betula, Alnus alnobetula, Saliceae, and Ericaceae are absent or occur only at very low relative abundances. This contrasts with pollen records that show a relatively high percentage of shrubs from the same core (Andreev et al., 2021), which likely reflect a long-distant rather than a catchment-scale signal. As with previous sedaDNA evidence (Courtin et al., 2021), we do not find any indication of a grass-dominated landscape as suggested by pollen records (Guthrie, 2013), also because grasses such as Poaceae and Cyperaceae can be underrepresented in the trnL metabarcoding data (Alsos et al., 2018). Despite the evidence of mammoth steppe in other plant metabarcoding records from northeastern Siberia during Last Glacial (Courtin et al., 2021), the taxonomic composition in our data does not match the description of the mammoth steppe, which are diverse and vastly covered by grasses, forbs, and sedges (Johnson, 2009). The formation of the forb-dominated assemblages in our records was thus likely not shaped by mammals.
Zone 2 (18–14 ka) is significantly different from Zone 1 in its assemblage composition. Compared to Zone 1, forb taxa such as Asteraceae, Brassicaceae, and Cyperaceae greatly reduce their relative abundances, whereas dwarf birch and dwarf willow increase. This is probably related to climate amelioration during the Late Glacial period (Lozhkin et al., 2007). An increased representation of shrubs may also be related to a local signal of meltwater along the glacial channels (Whittaker, 1993). The presence of Betula in the wider region is also confirmed from 18.7 ka by pollen records from the same core, though at low abundance (Andreev et al., 2021).
Zone 3 (14–0 ka) witnesses a shift from dwarf-shrub tundra to erect-shrub tundra and open forest with an Ericaceae understory. High relative abundance of deciduous erect shrubs (Alnus, Betula, and Ribes) and deciduous trees (Populus) occurred between 10 and 6 ka. This is also reported by other Siberian and Beringian pollen records (Szeicz and MacDonald, 2001; Velichko et al., 2002; Anderson and Lozhkin, 2015) and likely relates to increased summer warmth and moisture (Mann et al., 2002). The period from 13 to 9 ka shows slightly reduced erect shrub values which might be related to cold events (Lozhkin, 1993; Kokorowski et al., 2008). Interestingly, the late Holocene is characterized by an invasion of Larix, although sporadically and at low relative abundance. This implies Larix invaded the area after the Holocene Climatic Optimum; however, this needs further investigation.
It appears that there is an overrepresentation of certain plant taxa in our sedaDNA record, for example, Saliceae, which is not dominant in the pollen records from Ilirney (Andreev et al., 2021). Willows preferentially grow along rivers and channels. Hence they have a higher chance of their vegetative remains being transported to the center of a lake compared with upland taxa (Lozhkin et al., 2001). This invests massively in their roots and creeping stems, which increases their DNA preservation potential (Pedersen et al., 2013).
By analogy to the past, future warming will likely result in increased summer-green shrub growth for upland areas replacing forb communities, which has already been observed via remote-sensing data of the last 20 years in the catchment (Shevtsova et al., 2020). Furthermore, future changes in catchment hydrology may have a strong impact on the vegetation grow along the waters, such as willows. Our finding of delayed forest response to warming implies that tree invasion strongly lags ongoing and future warming.
Patterns of Taxonomic Alpha Diversity and Their Relationship to Assemblage Composition
The change in species richness shows that the greatest diversity of plant taxa occurred about 28 thousand years ago (Figure 4) and was even higher than the maximum post-LGM richness, which occurred during 13–9 ka, and not, as expected, during the time of the Holocene vegetation “optimum” (as defined by the highest PC1 values) during 10–6 ka.
The specific geographical setting of our study area in central Chukotka probably favored higher biodiversity during the pre-LGM. The north-eastern Siberia was widely believed to be largely ice-free during most of the Pleistocene (Svendsen et al., 2004; Brubaker et al., 2005; Jakobsson et al., 2016). Meanwhile, the species-area relationship suggests a positive association between area and species richness (Connor and McCoy, 1979). Hence the enormous land area could have allowed more taxa to thrive during the pre-LGM and provided a glacial refugium for a variety of vegetation types during the LGM (Brubaker et al., 2005). We find no DNA evidence for the proposed shrub and herb vegetation during the pre-LGM, which is a presumed characteristic of the mammoth steppe (Johnson, 2009; Jørgensen et al., 2012). Hence, pre-LGM plant richness might not be related to the mammalian influence on vegetation (Sandom et al., 2014).
The LGM flora is largely a subset of pre-LGM flora. We find a decrease in richness compared to the pre-LGM, which is mainly led by the dramatic reduction of forb diversity, in particular for Asteraceae, Brassicaceae, and Cyperaceae. This probably results from the local extinctions of thermophilic species in response to the cooling into LGM (Stuart and Lister, 2007), or due to the potential breakdown of herbivore-vegetation interactions by declining mammalian populations during the LGM (Willerslev et al., 2014).
Interestingly, the pre-LGM forb diversity did not re-establish during the early Late Glacial, which suggests a delayed taxa invasion. The shift from herbaceous to woody plants during 18–14 ka may hinder or slow the establishment of a species-rich forb assemblage via an increase in shrub height and density. But could also be due to the overrepresentation of woody plants like Salicaceae as discussed in section “Vegetation History Revealed by sedaDNA,” which might out-compete with forbs, leading to the lower detection of forbs. The second richness peak between 13 and 9 ka also coincided with a slight reduction in the relative abundance of erect deciduous shrubs, while the relative abundance of dwarf shrubs (e.g., Ericaceae and Rosaceae) and herbs (e.g., Asteraceae and Ranunculaceae) increased.
Evenness, indicating a uniform distribution of taxa, and richness are positively correlated for the 28–14 ka interval in our record. In contrast, the high evenness during 10–6 ka is accompanied by relatively low richness resulting in a weak negative correlation between evenness and richness during the last 14 kyr (Table 1). We assume that the densification of deciduous woodlands formed by Betula, Alnus alnobetula, and Populus during the mid-Holocene may have resulted in a disconnect between richness and evenness by inhibiting the establishment of a diverse herb and dwarf-shrub flora, causing the dominance of a rather species-poor undergrowth.
Unlike the pollen data, the results derived from DNA metabarcoding are only semi-quantitative. The biases are usually caused by coverage and resolution of the PCR primer, by the completeness of the reference databases, and by DNA taphonomy. Several sources of bias may influence the diversity of the inferred vegetation. We used a short DNA marker in this study, which may limit the detection of taxa with relatively longer sequences, as shorter barcode sequences are more likely to be preserved and thus amplified in PCR. For example, Carex and Eriophorum have sequences longer than 80 bp (Alsos et al., 2018), causing detection to only genus or family level (Epp et al., 2015; Sjögren et al., 2016). Homopolymer versions of the species may be present within genera such as Vaccinium, Pyrola, Ranunculus, and Pedicularis. The restricted resolution of the marker may also have weakened the reliability of assessment in some families such as Asteraceae and Poaceae (Sønstebø et al., 2010). Family-specific markers such as ITS primers (Willerslev et al., 2014) are needed for reducing the biases in chloroplast primers. Further alternatives to resolve the PCR biases are to use metagenomic approaches such as shotgun sequencing or capture approaches of enriching barcode regions (Gauthier et al., 2019). Nevertheless, the current richness is very similar to the richness at genus and family levels (Supplementary Figure 5A). Despite potential problems such as homopolymers and marker resolution, our pattern of richness is not affected as demonstrated by the highly significant correlations between richness at the different taxonomic ranks (Supplementary Table 6).
Furthermore, sedaDNA diversity signals from Lake Ilirney may be impacted by changes in taphonomy (Giguet-Covex et al., 2019). For example, large fluvial input and high sedimentation rate from glacier meltwater during the Late Glacial as a result of increased erosion of organic-poor sediments may have led to a dilution of DNA concentration and thus an apparent richness decline (Pawłowska et al., 2016). While the reference database we used for taxonomic assignment contains plants from the circum-arctic (Willerslev et al., 2014), the establishment of a reference database for Beringia is therefore a future task.
Our record indicates that low relative abundance of shrubs may have enabled a rich forb-dominated steppe-tundra vegetation assemblage during the pre-LGM while the dominance of deciduous erect shrubs during 10–6 ka coincides with low richness, but by an evenly distributed assemblage. Our data imply that, at the catchment scale, the widespread expansion of shrubs in the currently warming Arctic (Chapin et al., 2005; Walker et al., 2006) may represent a regime shift for local plant diversity. By analogy to the past, the ongoing “Arctic greening” might result in a reduction in plant richness in the long term. The dominant taxa as identified from the sedaDNA are also common in the landscape of the study area according to modern observations (Shevtsova et al., 2020).
Relationship Between Richness and Phylogenetic Alpha and Beta Diversity
Our exploration of the changes in phylogenetic diversity using plant sedaDNA data reveals that species richness and phylogenetic clustering are positively related during the dry and cold 28–14 ka period but negatively during the wet and warm 14–0 ka period, indicating that rules of species assembly differed substantially during these periods.
The statistically significant and positive NTI values for 28–14 ka suggest phylogenetic clustering (Figure 4 and Table 1). Closely related taxa have more similar ecological preferences and often share similar environmental tolerances (Cavender-Bares et al., 2009; Mayfield and Levine, 2010), although some ubiquitous taxa such as Carex can inhabit a wide range of ecosystems. Plant assembly during the pre-LGM consists of closely related sequences assigned to forb families such as Asteraceae and Saxifragaceae (Figure 2). Phylogenetic clustering likely happened through a selection of climatically pre-adapted species or even adaptation to cope with severe glacial conditions (Marx et al., 2017; Kubota et al., 2018).
A positive correlation between NTI and richness indicates that rich plant assemblages were composed of closely related species during 28–14 ka. A common assumption is that genetically closely related species tend to compete more intensely than their distantly related peers, resulting in reduced coexistence (Webb, 2000; Cahill et al., 2008). However, competition also increases the opportunities for ecological facilitation through niche differentiation (Gioria and Osborne, 2014). Some closely related arctic forbs have been found to interact positively (Kolář et al., 2013). For example, species of Asteraceae can coexist with their sister clades through stabilizing niche differences (Godoy et al., 2014).
There is a tendency toward an overall increase in the phylogenetic distance between 14 and 10 ka compared with the 28–14 ka interval, indicating a shift from phylogenetic clustering before 14 ka to phylogenetic overdispersion during 14–10 ka (Figure 4). Climate amelioration after 14 ka supported the invasion of many taxa in the catchment of Lake Ilirney. Species recruitment was therefore likely to be rather erratic and neither environmental nor biological filtering was shaping the assemblage, resulting in a phylogenetically overdispersed assemblage between 14 and 10 ka. Though species arrival depends not only on climate but also on the distance to the glacial refugia (Alsos et al., 2015) and on the presence of migration barriers (Crump et al., 2019), which could result in a delayed arrival. Non-angiosperms such as conifers and ferns were infrequent before 14 ka but became more common after 14 ka. Their influence on the NTI is, however, minor (Supplementary Figure 6 and Supplementary Table 7). Despite the potential problems such as homopolymers and marker resolution, the patterns of NTI are not affected as we find highly similar NTI results at both the genus and family levels (Supplementary Figures 5B,C and Supplementary Table 6).
A negative correlation between NTI and richness, as found after 14 ka, indicates a pattern that rich plant assemblages are distantly related. Following the stress gradient hypothesis (Bertness and Callaway, 1994), less stressful environments during 14–10 ka were characterized by erect-shrub tundra and open forest composed of distantly related species. These species interact positively while biotic filtering is of minor importance. Such beneficial ecological facilitation may have supported the maintenance of high productivity between 14 and 10 ka (Zhang et al., 2016). Increased NTI values during 10–6 ka are accompanied by reduced richness. This is probably due to an unstable co-existence within closely related shrubs. After the massive expansion of shrubs and trees during the early Holocene, co-occurring shrub species are more distantly related due to competitive exclusion. Alternatively, the closely related shrubs can have negative effects on the diversity of the understory by competition for light, water, and nutrients (Barbier et al., 2008). Further experiments are needed to validate whether competition or facilitation dominates when close relatives of arctic shrubs coexist.
Our results indicate that ecologically unique plant assemblages are rich when considering only presence and absence in the data (Figure 4 and Table 1). Species of intermediate abundance contribute most to the occurrence-based beta diversity (Supplementary Figure 4B) as they show strong abundance variations (Heino and Grönroos, 2016; Silva et al., 2018). A pattern of negative correlation between NTI and pLCBD during 28–0 ka is not surprising. Phylogenetically close species tend to share similar ecological requirements and are therefore often found in the same community (Mayfield and Levine, 2010; Kamilar et al., 2014). These assemblages then exhibit genetic clustering (Shooner et al., 2018) and become phylogenetically even less unique.
The changes in phylogenetic diversity before and after 14 ka differ from those shown by taxonomic diversity, suggesting that inferred assembly rules can be hampered if the analyses are based solely on taxonomic or solely on phylogenetic diversity (Chai et al., 2016). Using a combination of taxonomic data and phylogenetic data gives a more complete representation of assemblage structure. With respect to ongoing warming, our results imply, that, in the short term, many species might invade the area leading to rich phylogenetic overdispersed assemblages. However, expansion of shrubs might cause a reduction in plant taxonomic richness in the long term.
Data Availability Statement
Depths and the corresponding ages of the samples for this study can be found in Pangaea (https://doi.pangaea.de/10.1594/PANGAEA.925767). The raw sequencing data, related scripts for analysing and final sequence dataset with relative abundances and taxonomic assignments, can be found in Dryad (https://doi.org/10.5061/dryad.sbcc2fr4k).
Author Contributions
SH: conceptualization (lead), formal analysis (lead), and writing – original draft and review and editing (lead). KS-L: conceptualization (supporting), formal analysis (supporting), resources (supporting), and writing – review and editing (supporting). SL, JC, and AA: writing – review and editing (supporting). LP: resources (supporting) and writing – review and editing (supporting). UH: conceptualization (lead), resources (lead), writing – review and editing (lead), and supervision (lead). All authors contributed to the article and approved the submitted version.
Funding
This research has received funding from the European Research Council (ERC) under the European Union’s Horizon 2020 Research and Innovation Programme (Grant No. 772852, GlacialLegacy to UH), the Chinese Scholarship Council (CSC) (Grant No. 201708080102 to SH), and Deutsche Forschungsgemeinschaft (DFG) (Grant No. EP 98/3-1 to UH). The expedition is part of a joint Russian-German Expedition.
Conflict of Interest
The authors declare that the research was conducted in the absence of any commercial or financial relationships that could be construed as a potential conflict of interest.
Publisher’s Note
All claims expressed in this article are solely those of the authors and do not necessarily represent those of their affiliated organizations, or those of the publisher, the editors and the reviewers. Any product that may be evaluated in this article, or claim that may be made by its manufacturer, is not guaranteed or endorsed by the publisher.
Supplementary Material
The Supplementary Material for this article can be found online at: https://www.frontiersin.org/articles/10.3389/fevo.2021.763747/full#supplementary-material
Footnotes
- ^ https://www.r-project.org
- ^ https://github.com/StefanKruse/R_Rarefaction
- ^ https://www.r-project.org
References
Alsos, I. G., Ehrich, D., Eidesen, P. B., Solstad, H., Westergaard, K. B., Schönswetter, P., et al. (2015). Long-distance plant dispersal to North Atlantic islands: colonization routes and founder effect. AoB Plants 7:lv036. doi: 10.1093/aobpla/plv036
Alsos, I. G., Lammers, Y., Yoccoz, N. G., Jørgensen, T., Sjögren, P., Gielly, L., et al. (2018). Plant DNA metabarcoding of lake sediments: how does it represent the contemporary vegetation. PLoS One 13:e0195403. doi: 10.1371/journal.pone.0195403
Anderson, P. M., and Brubaker, L. B. (1994). Vegetation history of northcentral Alaska: a mapped summary of late-quaternary pollen data. Quat. Sci. Rev. 13, 71–92. doi: 10.1016/0277-3791(94)90125-2
Anderson, P. M., and Lozhkin, A. V. (2015). Late quaternary vegetation of chukotka (Northeast Russia), implications for Glacial and Holocene environments of Beringia. Quat. Sci. Rev. 107, 112–128. doi: 10.1016/j.quascirev.2014.10.016
Andreev, A. A., Raschke, E., Biskaborn, B. K., Vyse, S. A., Courtin, J., Böhmer, T., et al. (2021). Late Pleistocene to Holocene vegetation and climate changes in northwestern Chukotka (Far East Russia) deduced from lakes Ilirney and Rauchuagytgyn pollen records. Boreas 50, 652–670. doi: 10.1111/bor.12521
Andreev, A. A., Schirrmeister, L., Tarasov, P. E., Ganopolski, A., Brovkin, V., Siegert, C., et al. (2011). Vegetation and climate history in the Laptev Sea region (Arctic Siberia) during late Quaternary inferred from pollen records. Quat. Sci. Rev. 30, 2182–2199. doi: 10.1016/j.quascirev.2010.12.026
Bálint, M., Pfenninger, M., Grossart, H.-P., Taberlet, P., Vellend, M., Leibold, M. A., et al. (2018). Environmental DNA time series in ecology. Trends Ecol. Evol. 33, 945–957. doi: 10.1016/j.tree.2018.09.003
Barba, M. D., Miquel, C., Boyer, F., Mercier, C., Rioux, D., Coissac, E., et al. (2014). DNA metabarcoding multiplexing and validation of data accuracy for diet assessment: application to omnivorous diet. Mol. Ecol. Resour. 14, 306–323. doi: 10.1111/1755-0998.12188
Barbier, S., Gosselin, F., and Balandier, P. (2008). Influence of tree species on understory vegetation diversity and mechanisms involved—A critical review for temperate and boreal forests. Forest Ecol. Manag. 254, 1–15. doi: 10.1016/j.foreco.2007.09.038
Bertness, M. D., and Callaway, R. (1994). Positive interactions in communities. Trends Ecol. Evol. 9, 191–193. doi: 10.1016/0169-5347(94)90088-4
Binladen, J., Gilbert, M. T. P., Bollback, J. P., Panitz, F., Bendixen, C., Nielsen, R., et al. (2007). The use of coded PCR primers enables high-throughput sequencing of multiple homolog amplification products by 454 parallel sequencing. PLoS One 2:e197. doi: 10.1371/journal.pone.0000197
Binney, H. A., Gething, P. W., Nield, J. M., Sugita, S., and Edwards, M. E. (2011). Tree line identification from pollen data: beyond the limit?: Tree line identification from pollen data. J. Biogeogr. 38, 1792–1806. doi: 10.1111/j.1365-2699.2011.02507.x
Blaauw, M., and Christen, J. A. (2011). Flexible paleoclimate age-depth models using an autoregressive gamma process. Bayesian Anal. 6, 457–474. doi: 10.1214/11-ba618
Blarquez, O., Carcaillet, C., Frejaville, T., and Bergeron, Y. (2014). Disentangling the trajectories of alpha, beta and gamma plant diversity of North American boreal ecoregions since 15,500 years. Front. Ecol. Evol. 2:6. doi: 10.3389/fevo.2014.00006
Blarquez, O., Finsinger, W., and Carcaillet, C. (2013). Assessing paleo-biodiversity using low proxy influx. PLoS One 8:e65852. doi: 10.1371/journal.pone.0065852
Boyer, F., Mercier, C., Bonin, A., Bras, Y. L., Taberlet, P., and Coissac, E. (2016). obitools: a unix−inspired software package for DNA metabarcoding. Mol. Ecol. Resour. 16, 176–182. doi: 10.1111/1755-0998.12428
Brubaker, L. B., Anderson, P. M., Edwards, M. E., and Lozhkin, A. V. (2005). Beringia as a glacial refugium for boreal trees and shrubs: new perspectives from mapped pollen data. J. Biogeogr. 32, 833–848. doi: 10.1111/j.1365-2699.2004.01203.x
Cahill, J. F., Kembel, S. W., Lamb, E. G., and Keddy, P. A. (2008). Does phylogenetic relatedness influence the strength of competition among vascular plants? Perspect. Plant Ecol. Evol. Syst. 10, 41–50. doi: 10.1016/j.ppees.2007.10.001
Carcaillet, C., Hörnberg, G., and Zackrisson, O. (2012). Woody vegetation, fuel and fire track the melting of the Scandinavian ice-sheet before 9500calyrBP. Quat. Res. 78, 540–548. doi: 10.1016/j.yqres.2012.08.001
Cavender-Bares, J., Kozak, K. H., Fine, P. V. A., and Kembel, S. W. (2009). The merging of community ecology and phylogenetic biology. Ecol. Lett. 12, 693–715. doi: 10.1111/j.1461-0248.2009.01314.x
Cavieres, L. A., and Badano, E. I. (2009). Do facilitative interactions increase species richness at the entire community level? J. Ecol. 97, 1181–1191. doi: 10.1111/j.1365-2745.2009.01579.x
Chai, Y., Yue, M., Liu, X., Guo, Y., Wang, M., Xu, J., et al. (2016). Patterns of taxonomic, phylogenetic diversity during a long-term succession of forest on the Loess Plateau, China: insights into assembly process. Sci. Rep. 6:27087. doi: 10.1038/srep27087
Champlot, S., Berthelot, C., Pruvost, M., Bennett, E. A., Grange, T., and Geigl, E.-M. (2010). An efficient multistrategy DNA decontamination procedure of PCR reagents for hypersensitive PCR applications. PLoS One 5:e13042. doi: 10.1371/journal.pone.0013042
Chapin, F. S., Sturm, M., Serreze, M. C., McFadden, J. P., Key, J. R., Lloyd, A. H., et al. (2005). Role of Land-Surface changes in arctic summer warming. Science 310, 657–660. doi: 10.1126/science.1117368
Chu, H., and Grogan, P. (2010). Soil microbial biomass, nutrient availability and nitrogen mineralization potential among vegetation-types in a low arctic tundra landscape. Plant Soil 329, 411–420. doi: 10.1007/s11104-009-0167-y
Chytrý, M., Horsák, M., Syrovátka, V., Danihelka, J., Ermakov, N., German, D. A., et al. (2017). Refugial ecosystems in central Asia as indicators of biodiversity change during the Pleistocene–Holocene transition. Ecol. Indic. 77, 357–367. doi: 10.1016/j.ecolind.2016.12.033
Clarke, C. L., Edwards, M. E., Gielly, L., Ehrich, D., Hughes, P. D. M., Morozova, L. M., et al. (2019). Persistence of arctic-alpine flora during 24,000 years of environmental change in the Polar Urals. Sci. Rep. 9:19613. doi: 10.1038/s41598-019-55989-9
Connor, E. F., and McCoy, E. D. (1979). The statistics and biology of the species-area relationship. Am. Nat. 113, 791–833. doi: 10.1086/283438
Courtin, J., Andreev, A. A., Raschke, E., Bala, S., Biskaborn, B. K., Liu, S., et al. (2021). Vegetation changes in southeastern siberia during the late pleistocene and the Holocene. Front. Ecol. Evol. 09:625096. doi: 10.3389/fevo.2021.625096
Crump, S. E., Miller, G. H., Power, M., Sepúlveda, J., Dildar, N., Coghlan, M., et al. (2019). Arctic shrub colonization lagged peak postglacial warmth: molecular evidence in lake sediment from Arctic Canada. Glob. Change Biol. 25, 4244–4256. doi: 10.1111/gcb.14836
Danby, R. K., Koh, S., Hik, D. S., and Price, L. W. (2011). Four decades of plant community change in the Alpine Tundra of Southwest Yukon, Canada. Ambio 40, 660–671. doi: 10.1007/s13280-011-0172-2
Dorji, T., Totland, O., Moe, S. R., Hopping, K. A., Pan, J., and Klein, J. A. (2012). Plant functional traits mediate reproductive phenology and success in response to experimental warming and snow addition in Tibet. Global Change Biol. 19, 459–472. doi: 10.1111/gcb.12059
Dray, S., Blanchet, G., Borcard, D., Clappe, S., Guenard, G., Jombart, T., et al. (2017). Adespatial: Multivariate Multiscale Spatial Analysis. R Package Version 0.0-9.
Epp, L. S., Gussarova, G., Boessenkool, S., Olsen, J., Haile, J., Schrøder-Nielsen, A., et al. (2015). Lake sediment multi-taxon DNA from North Greenland records early post-glacial appearance of vascular plants and accurately tracks environmental changes. Quat. Sci. Rev. 117, 152–163. doi: 10.1016/j.quascirev.2015.03.027
Epp, L. S., Kruse, S., Kath, N. J., Stoof-Leichsenring, K. R., Tiedemann, R., Pestryakova, L. A., et al. (2018). Temporal and spatial patterns of mitochondrial haplotype and species distributions in Siberian larches inferred from ancient environmental DNA and modeling. Sci. Rep. 8:17436. doi: 10.1038/s41598-018-35550-w
Feurdean, A., Tămaş, T., Tanţău, I., and Fărcaş, S. (2011). Elevational variation in regional vegetation responses to late-glacial climate changes in the Carpathians. J. Biogeogr. 39, 258–271. doi: 10.1111/j.1365-2699.2011.02605.x
Gauthier, M., Konecny−Dupré, L., Nguyen, A., Elbrecht, V., Datry, T., Douady, C., et al. (2019). Enhancing DNA metabarcoding performance and applicability with bait capture enrichment and DNA from conservative ethanol. Mol. Ecol. Resour. 20, 79–96. doi: 10.1111/1755-0998.13088
Giguet-Covex, C., Ficetola, G. F., Walsh, K., Poulenard, J., Bajard, M., Fouinat, L., et al. (2019). New insights on lake sediment DNA from the catchment: importance of taphonomic and analytical issues on the record quality. Sci. Rep. 9:14676. doi: 10.1038/s41598-019-50339-1
Gioria, M., and Osborne, B. A. (2014). Resource competition in plant invasions: emerging patterns and research needs. Front. Plant Sci. 5:501. doi: 10.3389/fpls.2014.00501
Godoy, O., Kraft, N. J. B., and Levine, J. M. (2014). Phylogenetic relatedness and the determinants of competitive outcomes. Ecol. Lett. 17, 836–844. doi: 10.1111/ele.12289
Guthrie, R. D. (2013). Frozen Fauna of the Mammoth Steppe: The Story of Blue Babe. Chicago, IL: University of Chicago Press.
Heino, J., and Grönroos, M. (2016). Exploring species and site contributions to beta diversity in stream insect assemblages. Oecologia 183, 151–160. doi: 10.1007/s00442-016-3754-7
Hill, M. O. (1973). Diversity and evenness: a unifying notation and its consequences. Ecology 54, 427–432. doi: 10.2307/1934352
Jakobsson, M., Nilsson, J., Anderson, L., Backman, J., Björk, G., Cronin, T. M., et al. (2016). Evidence for an ice shelf covering the central Arctic Ocean during the penultimate glaciation. Nat. Commun. 7:10365. doi: 10.1038/ncomms10365
Jin, Y., and Qian, H. (2019). V.PhyloMaker: an R package that can generate very large phylogenies for vascular plants. Ecography 42, 1353–1359. doi: 10.1111/ecog.04434
Johnson, C. N. (2009). Ecological consequences of Late Quaternary extinctions of megafauna. Proc. R. Soc. B Biol. Sci. 276, 2509–2519. doi: 10.1098/rspb.2008.1921
Jørgensen, T., Haile, J., Möller, P., Andreev, A., Boessenkool, S., Rasmussen, M., et al. (2012). A comparative study of ancient sedimentary DNA, pollen and macrofossils from permafrost sediments of northern Siberia reveals long−term vegetational stability. Mol. Ecol. 21, 1989–2003.
Juggins, S. (2009). ). Rioja: An R Package for the Analysis of Quaternary Science Data. Newcastle: Newcastle University Library.
Kamilar, J. M., Beaudrot, L., and Reed, K. E. (2014). The influences of species richness and climate on the phylogenetic structure of African Haplorhine and strepsirrhine primate communities. Int. J. Primatol. 35, 1105–1121. doi: 10.1007/s10764-014-9784-2
Kembel, S. W., Cowan, P. D., Helmus, M. R., Cornwell, W. K., Morlon, H., Ackerly, D. D., et al. (2010). Picante: R tools for integrating phylogenies and ecology. Bioinformatics 26, 1463–1464. doi: 10.1093/bioinformatics/btq166
Kharuk, V. I., Ranson, K. J., Im, S. T., and Naurzbaev, M. M. (2006). Forest-tundra larch forests and climatic trends. Russ. J. Ecol. 37, 291–298. doi: 10.1134/s1067413606050018
Khitun, O. V., Koroleva, T. M., Chinenko, S. V., Petrovsky, V. V., Pospelova, E. B., Pospelov, I. N., et al. (2016). Applications of local floras for floristic subdivision and monitoring vascular plant diversity in the Russian Arctic. Arct. Sci. 2, 103–126. doi: 10.1139/as-2015-0010
Kokorowski, H. D., Anderson, P. M., Mock, C. J., and Lozhkin, A. V. (2008). A re-evaluation and spatial analysis of evidence for a Younger Dryas climatic reversal in Beringia. Quat. Sci. Rev. 27, 1710–1722. doi: 10.1016/j.quascirev.2008.06.010
Kolář, F., Lučanová, M., Vít, P., Urfus, T., Chrtek, J., Fér, T., et al. (2013). Diversity and endemism in deglaciated areas: ploidy, relative genome size and niche differentiation in the Galium pusillum complex (Rubiaceae) in Northern and Central Europe. Ann. Bot. 111, 1095–1108. doi: 10.1093/aob/mct074
Kruse, S., Gerdes, A., Kath, N. J., Epp, L. S., Stoof-Leichsenring, K. R., Pestryakova, L. A., et al. (2018). Dispersal distances and migration rates at the arctic treeline in Siberia – a genetic and simulation based study. Biogeosciences 16, 1211–1224. doi: 10.5194/bg-2018-267
Kubota, Y., Kusumoto, B., Shiono, T., and Ulrich, W. (2018). Environmental filters shaping angiosperm tree assembly along climatic and geographic gradients. J. Veg. Sci. 29, 607–618. doi: 10.1111/jvs.12648
Kuzmina, S. A., Sher, A. V., Edwards, M. E., Haile, J., Yan, E. V., Kotov, A. V., et al. (2011). The late Pleistocene environment of the Eastern West Beringia based on the principal section at the Main River, Chukotka. Quat. Sci. Rev. 30, 2091–2106. doi: 10.1016/j.quascirev.2010.03.019
Legendre, P., and Borcard, D. (2018). Box–Cox−chord transformations for community composition data prior to beta diversity analysis. Ecography 41, 1820–1824. doi: 10.1111/ecog.03498
Legendre, P.Cáceres, M. D. (2013). Beta diversity as the variance of community data: dissimilarity coefficients and partitioning. Ecol. Lett. 16, 951–963. doi: 10.1111/ele.12141
Liu, S. Stoof-Leichsenring, K. R., Kruse, S., Pestryakova, L. A., and Herzschuh, U. (2020). Holocene vegetation and plant diversity changes in the north-eastern Siberian treeline region from pollen and sedimentary ancient DNA. Front. Ecol. Evol. 8:560243. doi: 10.3389/fevo.2020.560243
Lozhkin, A. V. (1993). Geochronology of late quaternary events in Northeastern Russia. Radiocarbon 35, 429–433. doi: 10.1017/s0033822200060446
Lozhkin, A. V., Anderson, P. M., Matrosova, T. V., and Minyuk, P. S. (2007). The pollen record from El’gygytgyn Lake: implications for vegetation and climate histories of northern Chukotka since the late middle Pleistocene. J. Paleolimnol. 37, 135–153. doi: 10.1007/s10933-006-9018-5
Lozhkin, A. V., Anderson, P. M., Vartanyan, S. L., Brown, T. A., Belaya, B. V., and Kotov, A. N. (2001). Late quaternary paleoenvironments and modern pollen data from Wrangel Island (Northern Chukotka). Quat. Sci. Rev. 20, 217–233. doi: 10.1016/s0277-3791(00)00121-9
Mann, D. H., Peteet, D. M., Reanier, R. E., and Kunz, M. L. (2002). Responses of an arctic landscape to Lateglacial and early Holocene climatic changes: the importance of moisture. Quat. Sci. Rev. 21, 997–1021. doi: 10.1016/s0277-3791(01)00116-0
Marx, H. E., Dentant, C., Renaud, J., Delunel, R., Tank, D. C., and Lavergne, S. (2017). Riders in the sky (islands): using a mega-phylogenetic approach to understand plant species distribution and coexistence at the altitudinal limits of angiosperm plant life. J. Biogeogr. 44, 2618–2630. doi: 10.1111/jbi.13073
Mayfield, M. M., and Levine, J. M. (2010). Opposing effects of competitive exclusion on the phylogenetic structure of communities: phylogeny and coexistence. Ecol. Lett. 13, 1085–1093. doi: 10.1111/j.1461-0248.2010.01509.x
Menne, M. J., Durre, I., Vose, R. S., Gleason, B. E., and Houston, T. G. (2012). An overview of the global historical climatology network-daily database. J. Atmos. Ocean. Technol. 29, 897–910.
Mod, H. K., Heikkinen, R. K., Roux, P. C., Väre, H., and Luoto, M. (2016). Contrasting effects of biotic interactions on richness and distribution of vascular plants, bryophytes and lichens in an arctic–alpine landscape. Polar Biol. 39, 649–657. doi: 10.1007/s00300-015-1820-y
Moser, K. A., and MacDonald, G. M. (1990). Holocene vegetation change at Treeline North of Yellowknife, Northwest Territories, Canada. Quat. Res. 34, 227–239. doi: 10.1016/0033-5894(90)90033-h
Niemeyer, B., Epp, L. S., Stoof−Leichsenring, K. R., Pestryakova, L. A., and Herzschuh, U. (2017). A comparison of sedimentary DNA and pollen from lake sediments in recording vegetation composition at the Siberian treeline. Mol. Ecol. Resour. 17, e46–e62. doi: 10.1111/1755-0998.12689
Parducci, L., Alsos, I. G., Unneberg, P., Pedersen, M. W., Han, L., Lammers, Y., et al. (2019). Shotgun environmental DNA, pollen, and macrofossil analysis of lateglacial lake sediments from Southern Sweden. Front. Ecol. Evol. 7:189. doi: 10.3389/fevo.2019.00189
Parducci, L., Bennett, K. D., Ficetola, G. F., Alsos, I. G., Suyama, Y., Wood, J. R., et al. (2017). Ancient plant DNA in lake sediments. New Phytol. 214, 924–942. doi: 10.1111/nph.14470
Pawłowska, J., Zaja̧czkowski, M., Ła̧cka, M., Lejzerowicz, F., Esling, P., and Pawlowski, J. (2016). Palaeoceanographic changes in Hornsund Fjord (Spitsbergen, Svalbard) over the last millennium: new insights from ancient DNA. Clim. Past 12, 1459–1472. doi: 10.5194/cp-12-1459-2016
Pearson, R. G., Phillips, S. J., Loranty, M. M., Beck, P. S. A., Damoulas, T., Knight, S. J., et al. (2013). Shifts in Arctic vegetation and associated feedbacks under climate change. Nat. Clim. Change 3, 673–677. doi: 10.1038/nclimate1858
Pedersen, M. W., Ginolhac, A., Orlando, L., Olsen, J., Andersen, K., Holm, J., et al. (2013). A comparative study of ancient environmental DNA to pollen and macrofossils from lake sediments reveals taxonomic overlap and additional plant taxa. Quat. Sci. Rev. 75, 161–168. doi: 10.1016/j.quascirev.2013.06.006
Reimer, P. J., Bard, E., Bayliss, A., Beck, J. W., Blackwell, P. G., Ramsey, C. B., et al. (2013). IntCal13 and Marine13 radiocarbon age calibration curves 0–50,000 years cal BP. Radiocarbon 55, 1869–1887. doi: 10.2458/azu_js_rc.55.16947
Reitalu, T., Gerhold, P., Poska, A., Pärtel, M., Väli, V., and Veski, S. (2015). Novel insights into post-glacial vegetation change: functional and phylogenetic diversity in pollen records. J. Veg. Sci. 26, 911–922. doi: 10.1111/jvs.12300
Revelle, W. R. (2018). Psych: Procedures for Personality and Psychological Research. Evanston, IL: Northwestern University.
Rijal, D. P., Heintzman, P. D., Lammers, Y., Yoccoz, N. G., Lorberau, K. E., Pitelkova, I., et al. (2021). Sedimentary ancient DNA shows terrestrial plant richness continuously increased over the Holocene in northern Fennoscandia. Sci. Adv. 7:eabf9557. doi: 10.1126/sciadv.abf9557
Roswell, M., Dushoff, J., and Winfree, R. (2021). A conceptual guide to measuring species diversity. Oikos 130, 321–338. doi: 10.1111/oik.07202
Rupp, T. S., Chapin, F. S. III, and Starfield, A. M. (2001). Modeling the influence of topographic barriers on treeline advance at the forest-Tundra ecotone in Northwestern Alaska. Clim. Change 48, 399–416. doi: 10.1023/a:1010738502596
Sandom, C. J., Ejrnaes, R., Hansen, M. D. D., and Svenning, J.-C. (2014). High herbivore density associated with vegetation diversity in interglacial ecosystems. Proc. Natl. Acad. Sci. U.S.A. 111, 4162–4167. doi: 10.1073/pnas.1311014111
Seppä, H., Nyman, M., Korhola, A., and Weckström, J. (2002). Changes of treelines and alpine vegetation in relation to post-glacial climate dynamics in northern Fennoscandia based on pollen and chironomid records. J. Quat. Sci. 17, 287–301. doi: 10.1002/jqs.678
Shevtsova, I., Heim, B., Kruse, S., Schrder, J., Troeva, E. I., Pestryakova, L. A., et al. (2020). Strong shrub expansion in tundra-taiga, tree infilling in taiga and stable tundra in central Chukotka (north-eastern Siberia) between 2000 and 2017. Environ. Res. Lett. 15:085006. doi: 10.1088/1748-9326/ab9059
Shevtsova, I., Herzschuh, U., Heim, B., Schulte, L., Stünzi, S., Pestryakova, L. A., et al. (2021). Recent above-ground biomass changes in central Chukotka (Russian Far East) using field sampling and Landsat satellite data. Biogeosciences 18, 3343–3366. doi: 10.5194/bg-18-3343-2021
Shooner, S., Davies, T. J., Saikia, P., Deka, J., Bharali, S., Tripathi, O. P., et al. (2018). Phylogenetic diversity patterns in Himalayan forests reveal evidence for environmental filtering of distinct lineages. Ecosphere 9:e02157. doi: 10.1002/ecs2.2157
Silva, P. G., Hernández, M. I. M., and Heino, J. (2018). Disentangling the correlates of species and site contributions to beta diversity in dung beetle assemblages. Divers. Distrib. 24, 1674–1686. doi: 10.1111/ddi.12785
Sjögren, P., Edwards, M. E., Gielly, L., Langdon, C. T., Croudace, I. W., Merkel, M. K. F., et al. (2016). Lake sedimentary DNA accurately records 20th Century introductions of exotic conifers in Scotland. New Phytol. 213, 929–941. doi: 10.1111/nph.14199
Sjögren, P., van der Knaap, W. O., Huusko, A., and van Leeuwen, J. F. N. (2008). Pollen productivity, dispersal, and correction factors for major tree taxa in the Swiss Alps based on pollen-trap results. Rev. Palaeobot. Palynol. 152, 200–210. doi: 10.1016/j.revpalbo.2008.05.003
Soininen, E. M., Gauthier, G., Bilodeau, F., Berteaux, D., Gielly, L., Taberlet, P., et al. (2015). Highly overlapping winter diet in two sympatric lemming species revealed by DNA metabarcoding. PLoS One 10:e0115335. doi: 10.1371/journal.pone.0115335
Sønstebø, J. H., Gielly, L., Brysting, A. K., Elven, R., Edwards, M., Haile, J., et al. (2010). Using next-generation sequencing for molecular reconstruction of past Arctic vegetation and climate. Mol. Ecol. Resour. 10, 1009–1018. doi: 10.1111/j.1755-0998.2010.02855.x
Stuart, A. J., and Lister, A. M. (2007). Patterns of late quaternary megafaunal extinctions in Europe and northern Asia. Cour. Forschung. Senckenb. 259:287.
Svendsen, J. I., Alexanderson, H., Astakhov, V. I., Demidov, I., Dowdeswell, J. A., Funder, S., et al. (2004). Late Quaternary ice sheet history of northern Eurasia. Quat. Sci. Rev. 23, 1229–1271. doi: 10.1016/j.quascirev.2003.12.008
Szeicz, J. M., and MacDonald, G. M. (2001). Montane climate and vegetation dynamics in easternmost Beringia during the Late Quaternary. Quat. Sci. Rev. 20, 247–257. doi: 10.1016/s0277-3791(00)00119-0
Taberlet, P., Coissac, E., Pompanon, F., Gielly, L., Miquel, C., Valentini, A., et al. (2007). Power and limitations of the chloroplast trnL (UAA) intron for plant DNA barcoding. Nucleic Acids Res. 35:e14. doi: 10.1093/nar/gkl938
ter Braak, C. J. F., and Šmilauer, P. (2002). CANOCO Reference Manual and CanoDraw for Windows User’s Guide: Software for Canonical Community Ordination (version 4.5). Ithaca, NY: Microcomputer power.
Thuiller, W., Lavergne, S., Roquet, C., Boulangeat, I., Lafourcade, B., and Araujo, M. B. (2011). Consequences of climate change on the tree of life in Europe. Nature 470, 531–534. doi: 10.1038/nature09705
Tuomisto, H. (2010). A diversity of beta diversities: straightening up a concept gone awry. Part 2. Quantifying beta diversity and related phenomena. Ecography 33, 23–45. doi: 10.1111/j.1600-0587.2009.06148.x
Velichko, A. A., Catto, N., Drenova, A. N., Klimanov, V. A., Kremenetski, K. V., and Nechaev, V. P. (2002). Climate changes in East Europe and Siberia at the Late glacial–holocene transition. Quat. Int. 91, 75–99. doi: 10.1016/s1040-6182(01)00104-5
Vyse, S. A., Herzschuh, U., Andreev, A. A., Pestryakova, L. A., Diekmann, B., Armitage, S. J., et al. (2020). Geochemical and sedimentological responses of arctic glacial Lake Ilirney, Chukotka (Far East Russia) to palaeoenvironmental change since ∼51.8 ka BP. Quat. Sci. Rev. 247:106607. doi: 10.1016/j.quascirev.2020.106607
Walker, M. D., Wahren, C. H., Hollister, R. D., Henry, G. H. R., Ahlquist, L. E., Alatalo, J. M., et al. (2006). Plant community responses to experimental warming across the tundra biome. Proc. Natl. Acad. Sci. U.S.A. 103, 1342–1346. doi: 10.1073/pnas.0503198103
Webb, C. O. (2000). Exploring the phylogenetic structure of ecological communities: an example for rain forest trees. Am. Nat. 146, 145–155. doi: 10.1086/303378
Webb, C. O., and Pitman, N. C. A. (2002). Phylogenetic balance and ecological evenness. Syst. Biol. 51, 898–907. doi: 10.1080/10635150290102609
Whittaker, R. J. (1993). Plant population patterns in a glacier foreland succession: pioneer herbs and later-colonizing shrubs. Ecography 16, 117–136. doi: 10.1111/j.1600-0587.1993.tb00064.x
Willerslev, E., Davison, J., Moora, M., Zobel, M., Coissac, E., Edwards, M. E., et al. (2014). Fifty thousand years of Arctic vegetation and megafaunal diet. Nature 506, 47–51. doi: 10.1038/nature12921
Wilson, S. D., and Nilsson, C. (2009). Arctic alpine vegetation change over 20 years. Global Change Biol. 15, 1676–1684. doi: 10.1111/j.1365-2486.2009.01896.x
Zhang, L., Mi, X., and Shao, H. (2016). Phylogenetic relatedness influences plant interspecific interactions across stress levels in coastal ecosystems: a meta-analysis. Estuar. Coasts 39, 1669–1678. doi: 10.1007/s12237-016-0104-2
Zhu, J., Zhang, Y., Yang, X., Chen, N., Li, S., Wang, P., et al. (2020). Warming alters plant phylogenetic and functional community structure. J. Ecol. 108, 2406–2415. doi: 10.1111/1365-2745.13448
Zimmermann, H. H., Raschke, E., Epp, L. S., Stoof-Leichsenring, K. R., Schwamborn, G., Schirrmeister, L., et al. (2017). Sedimentary ancient DNA and pollen reveal the composition of plant organic matter in Late Quaternary permafrost sediments of the Buor Khaya Peninsula (north-eastern Siberia). Biogeosciences 14, 575–596. doi: 10.5194/bg-14-575-2017
Keywords: sedimentary ancient DNA (sedaDNA), metabarcoding, phylogenetic and taxonomic plant diversity, Arctic Russia, Siberia, holocene, glacial, treeline
Citation: Huang S, Stoof-Leichsenring KR, Liu S, Courtin J, Andreev AA, Pestryakova LA and Herzschuh U (2021) Plant Sedimentary Ancient DNA From Far East Russia Covering the Last 28,000 Years Reveals Different Assembly Rules in Cold and Warm Climates. Front. Ecol. Evol. 9:763747. doi: 10.3389/fevo.2021.763747
Received: 24 August 2021; Accepted: 11 November 2021;
Published: 01 December 2021.
Edited by:
Christopher Carcaillet, PSL Research University, FranceReviewed by:
Sarah Crump, University of California, Santa Cruz, United StatesJohn Birks, University of Bergen, Norway
Copyright © 2021 Huang, Stoof-Leichsenring, Liu, Courtin, Andreev, Pestryakova and Herzschuh. This is an open-access article distributed under the terms of the Creative Commons Attribution License (CC BY). The use, distribution or reproduction in other forums is permitted, provided the original author(s) and the copyright owner(s) are credited and that the original publication in this journal is cited, in accordance with accepted academic practice. No use, distribution or reproduction is permitted which does not comply with these terms.
*Correspondence: Ulrike Herzschuh, dWxyaWtlLmhlcnpzY2h1aEBhd2kuZGU=