- Department of Integrative Biology, University of South Florida, Tampa, FL, United States
Soil microbial communities both reflect and influence biotic and abiotic processes occurring at or near the soil surface. Ecosystem engineers that physically alter the soil surface, such as burrowing ground squirrels, are expected to influence the distribution of soil microbial communities. Black-tailed prairie dogs (Cynomys ludovicianus) construct complex burrows in which activities such as nesting, defecating, and dying are partitioned spatially into different chambers. Prairie dogs also experience large-scale die-offs due to sylvatic plague, caused by the bacterium Yersinia pestis, which lead to mass mortality events with potential repercussions on microbial communities. We used 16S sequencing to examine microbial communities in soil that was excavated by prairie dogs from different burrow locations, and surface soil that was used in the construction of burrow entrances, in populations that experienced plague die-offs. Following the QIIME2 pipeline, we assessed microbial diversity at several taxonomic levels among burrow regions. To do so, we computed community similarity metrics (Bray–Curtis, Jaccard, and weighted and unweighted UniFrac) among samples and community diversity indexes (Shannon and Faith phylogenetic diversity indexes) within each sample. Microbial communities differed across burrow regions, and several taxa exhibited spatial variation in relative abundance. Microbial ecological diversity (Shannon index) was highest in soil recently excavated from within burrows and soils associated with dead animals, and was lowest in soils associated with scat. Phylogenetic diversity varied only marginally within burrows, but the trends paralleled those for Shannon diversity. Yersinia was detected in four samples from one colony, marking the first time the genus has been sampled from soil on prairie dog colonies. The presence of Yersinia was a significant predictor of five bacterial families and eight microbial genera, most of which were rare taxa found in higher abundance in the presence of Yersinia, and one of which, Dictyostelium, has been proposed as an enzootic reservoir of Y. pestis. This study demonstrates that mammalian modifications to soil structure by physical alterations and by mass mortality can influence the distribution and diversity of microbial communities.
Introduction
Microbial communities are diverse assemblages of microbiotic species that, through interactions with each other and with the physical and chemical components of their abiotic environments, have substantial impacts on global processes. Microbes play an important role in global nutrient cycling (Treseder et al., 2016; Heijboer et al., 2018) and energy flow through ecosystems (Konopka, 2009). In turn, microbial communities are structured by the physical and chemical properties (Leff et al., 2015; Garcia et al., 2020; Xia et al., 2020) of the soil substrate, including soil moisture, C:N ratio, pH, and total carbon content (Shen et al., 2013; Li et al., 2017).
In addition to their interactions with abiotic processes, soil microbiota structure biotic diversity and regulate the health of hosts that house the microbial communities (Ichinohe et al., 2011; Shen et al., 2018). Soil microbes influence plant and animal communities (Lau and Lennon, 2011; Seastedt et al., submitted1) through mechanisms such as increasing plant nutrient acquisition (Hestrin et al., 2019) and resistance to desiccation (Xi et al., 2018) and inhibiting or facilitating the establishment of pathogens (Perez et al., 2008; van Elsas et al., 2012). Soil microbes are in turn governed by the actions of plants (Zak et al., 2003; Prescott and Grayston, 2013; Lange et al., 2015) and animals (Kandeler et al., 1999; Cline et al., 2017; Bray et al., 2019), creating feedbacks between soil microbial and aboveground communities (Bartelt-Ryser et al., 2005).
Biotic and abiotic processes that influence soil characteristics may be predicted to govern microbial diversity. For instance, ecosystem engineers that influence sediment abiotic properties (e.g., bioturbating shrimp, Laverock et al., 2010; Populus, Ciadamidaro et al., 2013) or soil nutrients (e.g., prairie dogs, Anacker et al., 2021) should thus also determine the microbial communities present (Gutiérrez and Jones, 2006; Cregger et al., 2018; Zotti et al., 2020). Similarly, mass mortality events in animals supply nutrient pulses that should alter microbial communities and contribute to terrestrial nutrient cycling (Metcalf et al., 2016b). Mass mortality in ecosystem engineers or keystone species, which influence the abundance of other (typically plant and animal) taxa, could have an especially pronounced effect. Soil microbiota can regulate the microbial pathogens causing such mass mortality, for instance if soil microbial communities contain animal pathogens or reservoirs for animal pathogens (Markman et al., 2018) or, conversely, microbes that inhibit establishment of animal pathogens. Through facilitation or inhibition of pathogens (Perez et al., 2008; van Elsas et al., 2012), soil microbes thus contribute to the maintenance of biodiversity of plants and animals.
Black-tailed prairie dogs (Cynomys ludovicianus) are social, fossorial ground squirrels inhabiting North American grasslands that build extensive underground burrows. Burrows typically range in length from 5 to 10 m long and extend as deep as 3–4 m below ground (Wilcomb, 1954; Hoogland, 1995). Burrows maximize air and water flow through the burrow and minimize water retention within the burrow, thus creating a moist but not wet environment. Their burrows increase soil porosity (Gedeon et al., 2012, which can facilitate deeper penetration of precipitation (Munn, 1993). Prairie dogs also increase the total nitrogen content and productivity of soils inside or near their burrows, leading to higher plant growth and diversity (Whicker and Detling, 1988; Holland and Detling, 1990).
More than half of a prairie dog’s life is spent within its burrow: prairie dogs use their burrows for reproducing, storing food, and escaping from both predators and the environment (Hoogland, 1995). Therefore, burrows are complex and heterogeneous in structure, and include spatially segregated chambers with various purposes, including nesting, hibernating (in species that hibernate; Cooke and Swiecki, 1992), defecating, and burying or isolating dead kin (Burns et al., 1989). Prairie dogs can die in their burrows over winter as a result of insufficient resources, and at other times of year from causes such as infectious disease. The primary disease affecting prairie dogs is sylvatic plague, caused by the Gram-negative bacterium Yersinia pestis. Typically transmitted by fleas, the pathogen is extremely virulent to prairie dogs, with individual colonies undergoing severe population declines ranging between 85% and complete extinction (Cully et al., 2010). These die-offs can thus result in hundreds of kilograms of carcasses appearing over the course of several weeks. In between epizootics, the plague reservoir is unknown: Some have hypothesized the pathogen persists in an alternative mammalian (Salkeld et al., 2010) host or flea vector (Webb et al., 2006) while others have posited that the reservoir is telluric (Drancourt and earlier; Eisen et al., 2008), residing in an invertebrate such as a nematode or amoeba (Markman et al., 2018).
Prairie dogs regularly clean out their burrows, leaving piles of nesting material, scat, and bones near some entrances of burrows. This excavated soil provides an opportunity to non-invasively explore the microbial composition of various locations within prairie dog burrows. We hypothesize that prairie dogs structure soil microbial communities through their functional partitioning of burrows, and that this structure may be pronounced after mass mortality caused by the pathogen Y. pestis. This study is the first to characterize the fine-scale spatial variation in microbial communities in the complex structure of prairie dog burrows.
Materials and Methods
Soil Sampling and Processing
Seventy-nine soil samples were collected in 2009 from six prairie dog colonies (named 1A, 12A, 17A, 19A, 30A, and 47A after Bai et al., 2008; Sackett et al., 2013; Supplementary Figure 1) located in Boulder County, CO (United States). All six colonies experienced die-offs from plague in 2006, and recolonization had begun in 2007 (five colonies) or 2008 (one colony; Sackett et al., 2013). Samples were collected from several locations, targeting different regions of the inner burrow (Figure 1; designed after Wilcomb, 1954): (1) loose soil on or adjacent to the burrow mound that had been recently excavated from within the burrow, “adjacent”; (2) soil at the burrow entrance that had been excavated from within the burrow along with prairie dog bones, “bones”; (3) soil at the burrow entrance that had been excavated along with prairie dog bones and scat, “bones + scat”; (4) soil that had been excavated along with remnants of a dead prairie dog, or soil at the entrance of a burrow emitting the smell of a dead animal, “dead”; (5) soil collected from within the mouth/entrance of the burrow, “entrance”; (6) loose soil from burrows containing plague-exposed animals (Sackett et al., 2013) in previous years, “plague”; and (7) soil at the burrow entrance located next to prairie dog scat (usually scat had been excavated from within the burrow), “scat.” Whenever possible (in all but five cases, where dry soils were sampled from beneath bones), we sampled soil that was still moist (indicated by visible moisture). Soils were stored frozen in 15 mL vials or plastic ziploc bags until nutrient analysis and DNA extraction.
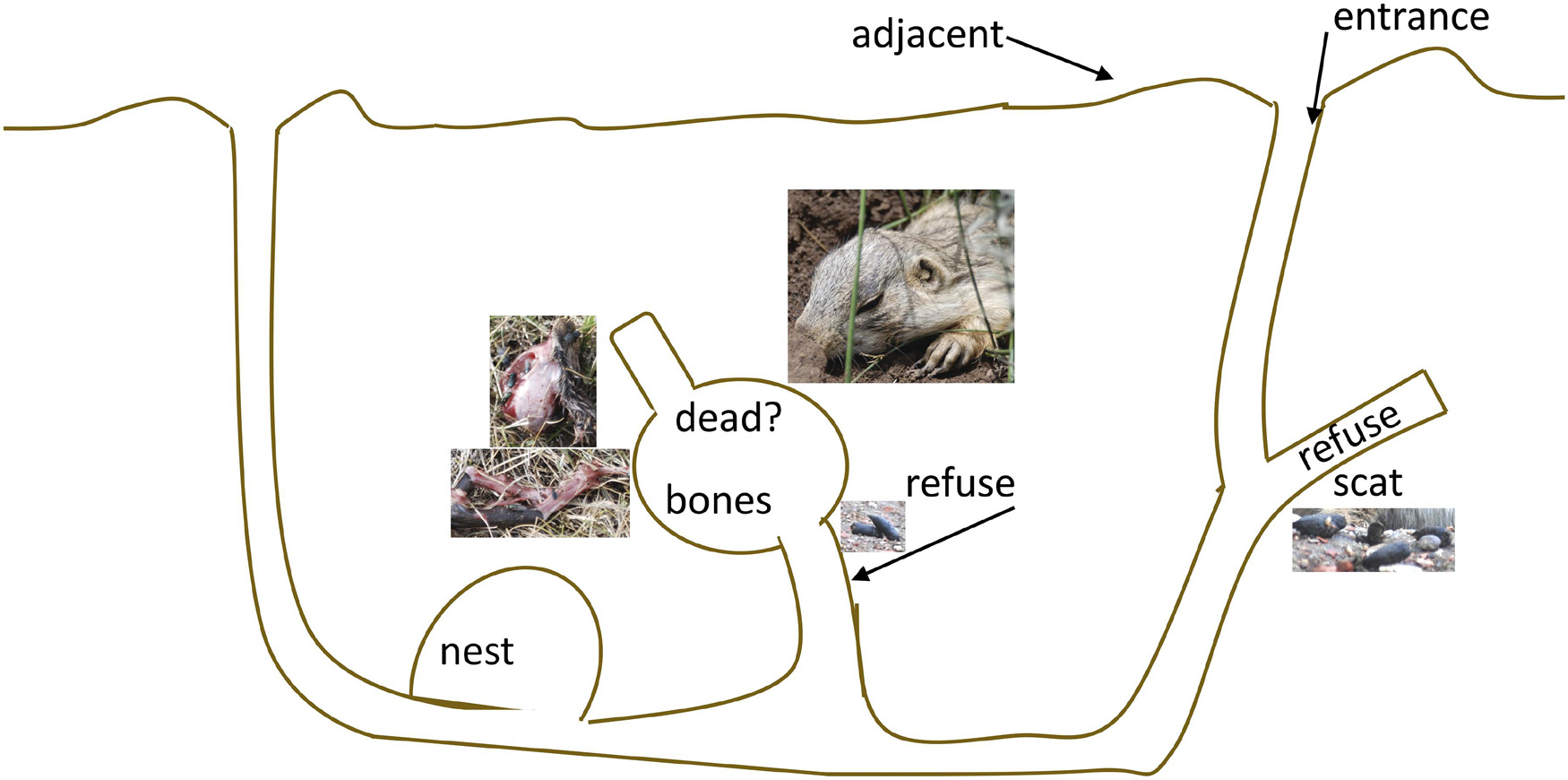
Figure 1. Schematic of the regions of a prairie dog burrow (not to scale), designed after Wilcomb (1954), showing chambers used for various purposes including nesting, defecating, and isolating dead individuals.
Nutrient analysis was performed at the Institute for Arctic and Alpine Research and at the Mountain Research Station at the University of Colorado. Total carbon, total nitrogen content, and C:N ratios were assessed on a CHN analyzer (LECO Corp., St. Joseph, MI, United States) with a standard run in between every 10 samples. Soil moisture was estimated by drying ∼1–2 g soil in an oven at 105°C for 5 days, weighing the samples before and after drying, and dividing the water weight by the wet soil weight. pH was measured using a ∼1:2 ratio of soil:water.
Variation in pH, water content, total nitrogen, total carbon, and carbon:nitrogen ratio among colonies and among regions within burrows were assessed using one-way ANOVA tests computed in R (R Core Team, 2018). A Tukey post hoc test was subsequently conducted for factors that varied significantly. These soil properties were included as covariates in the models below.
Sequencing and Quality Control
DNA was extracted from soil samples in duplicate using a PowerSoil extraction kit (MO Bio Laboratories Inc., Carlsbad, CA, United States) following manufacturer’s protocol. Sample processing, 16S sequencing, and core amplicon data analysis were performed by the Earth Microbiome Project2 (Thompson et al., 2017), and all amplicon sequence data and metadata have been made public through the EMP data portal3 (Qiita study 11519) and through the European Bioinformatics Institute (EBI) as project ERP106314.
The raw fastq files were compiled into a QIIME2 archive and all analyses were performed using Qiita (Gonzalez et al., 2018) and QIIME2 (RRID:SCR_021258, version 2017.8 or later). Sequences were demultiplexed using the demux plugin of QIIME2 and denoised using DADA2 (Callahan et al., 2016). The median Phred score of the sequences never dropped below 30; therefore, 3 bp were trimmed from the beginning and 5 bp from the end of each sequence to ensure all adapter sequences were removed. Both a feature table and its representative sequences were produced following denoising.
Analysis and Visualization
Taxonomic analysis of the soil samples was performed using a naive Bayesian classifier (Wang et al., 2007) trained using the Greengenes 13_8 99% OTUs (DeSantis et al., 2006; McDonald et al., 2012). This classifier was used along with the representative soil sequences in the q2-feature-classifier plugin (Bokulich et al., 2018) of QIIME2 (Bolyen et al., 2019) to assign taxonomies. Differences in the most abundant taxon in each burrow region were examined with a Chi-square test in R using different taxonomic levels.
Sequences were aligned and masked using mafft (Katoh and Standley, 2013), and an unrooted phylogenetic tree was generated using FastTree (Price et al., 2010). The tree was then rooted at its midpoint using the QIIME2’s phylogeny plugin. Using the rooted midpoint tree and the core-metrics plugin of QIIME2, the previously created feature table was rarefied with a sampling depth of 22,000 using the q2-diversity plugin to assess Bray–Curtis dissimilarity and Jaccard distance estimates and conduct a weighted (Lozupone et al., 2007) and unweighted UniFrac (Lozupone and Knight, 2005) diversity principal coordinates analysis (PCoA). All PCoA results were plotted using QIIME2’s Emperor plugin (Vázquez-Baeza et al., 2013) and visualized for clustering by burrow region.
We used a generalized linear modeling approach to determine the best predictors of ecological (Shannon) and phylogenetic (Faith) diversity. To do so, we modeled diversity as a function of burrow region, using pH, water content, and soil nutrients (C, N, and C:N) as covariates. We also tested models that included colony, excluded single nutrients, included relative abundance of Enterobacteriaceae, and included the presence of Yersinia, and we selected the best model using AIC. Next, we assessed whether Enterobacteriaceae was unique in its contribution to model fit (see section “Results”) by testing separately whether the addition of each of 565 microbial families also improved model fit. We evaluated model fit by comparing AIC values, irrespective of whether there was a significant relationship between a single taxon and diversity.
To assess the effect of burrow region on relative abundance of Enterobacteriaceae, we conducted a generalized linear model that included all predictors except colony. Next, to determine whether taxa in general varied in relative abundance at small spatial scales, we evaluated each taxon separately (565 families and 990 genera) in a generalized linear model with the same structure as the Enterobacteriaceae model. The significance of effects was determined using the Benjamini–Yekutieli false discovery rate correction (Benjamini and Yekutieli, 2001) for p-values returned from the glm.
Finally, we aimed to determine whether the presence of Yersinia (see section “Results”) was correlated with relative abundance of other taxa or the overall diversity of the sample. To do so, we first performed a non-parametric Kruskal–Wallis test on each taxon separately at the taxonomic levels of both family and genus and assessed significance using the Benjamini–Yekutieli false discovery rate correction (Benjamini and Yekutieli, 2001). Next, we assessed whether Yersinia presence was associated with levels of microbial diversity by performing a Kruskal–Wallis test on two measures of diversity at the genus level: the Shannon diversity index and the Faith phylogenetic diversity index (Faith, 1992). All R scripts are available on GitHub: https://github.com/CassinSackett/soilmicrobes/.
Results
We obtained 79 soil samples from 64 burrows in 6 colonies. Nitrogen content averaged 0.267% (range 0.062–0.685%) and carbon content averaged 3.56% (range 0.645–7.59%); mean C:N ratio was 14.8% (range 9.18–41.5%). Mean soil water content was 0.075 g/g (range 0.004–0.22) and mean pH was 7.89 (range 6.18–9.06). There was significant variation in soil properties among colonies (Supplementary Figure 1) and among burrow regions (Supplementary Figure 2). In particular, soil moisture was significantly higher in colony 30A and lower in colony 1A than other sites, and pH was significantly lower in colony 12A than several other sites (but sample sizes in 12A and 30A were small). Soil moisture was significantly higher in soil collected from the burrow entrance than in excavated soil containing prairie dog bones. The C:N ratio was significantly higher in soil sampled from excavated soil containing bones and scat than all other regions except those with scat. Total carbon, total nitrogen, and pH did not vary across sampling regions.
All clustering methods produced highly similar results, with the UniFrac unweighted method resulting in the highest proportion of variance explained by the first three axes. Samples collected from recently excavated soil adjacent to burrows clustered slightly on Axis 1, but samples from different regions were largely overlapping (Supplementary Figure 3).
The best initial model (excluding single taxa) of Shannon’s ecological diversity included the predictors: burrow region, pH, water content, and interactions between pH and water content and between carbon and nitrogen content (AIC 254.98). Colonies did not differ in ecological diversity, and inclusion of colony as a predictor worsened the model (AIC 263.65). Inclusion of Yersinia presence as a predictor worsened the model, but not significantly (AIC 256.97). All variables in the model significantly influenced diversity (Supplementary Table 1). Diversity was lowest in soil collected in the presence of scat, followed by soil with bones and scat, and was highest in soil recently excavated from burrows and from those with plague-positive animals (Figure 2). The best model of phylogenetic diversity included the same predictor variables (in this case, inclusion of colony as a predictor led to a worse, but statistically indistinguishable model: AIC with colony = 1125.9, AIC without colony = 1125.7). Inclusion of Yersinia presence as a predictor resulted in a statistically indistinguishable model (AIC 1125.8). All predictors significantly influenced phylogenetic diversity except for burrow location, which had a marginally significant effect (p = 0.074). Similar to the pattern observed for ecological diversity, phylogenetic diversity exhibited a trend toward lower diversity in soil collected in the presence of scat, followed by soil with bones and scat, and higher diversity in soil recently excavated from burrows, soil from burrows inferred to contain dead animals, and soil excavated from burrows with plague-positive animals (Figure 2).
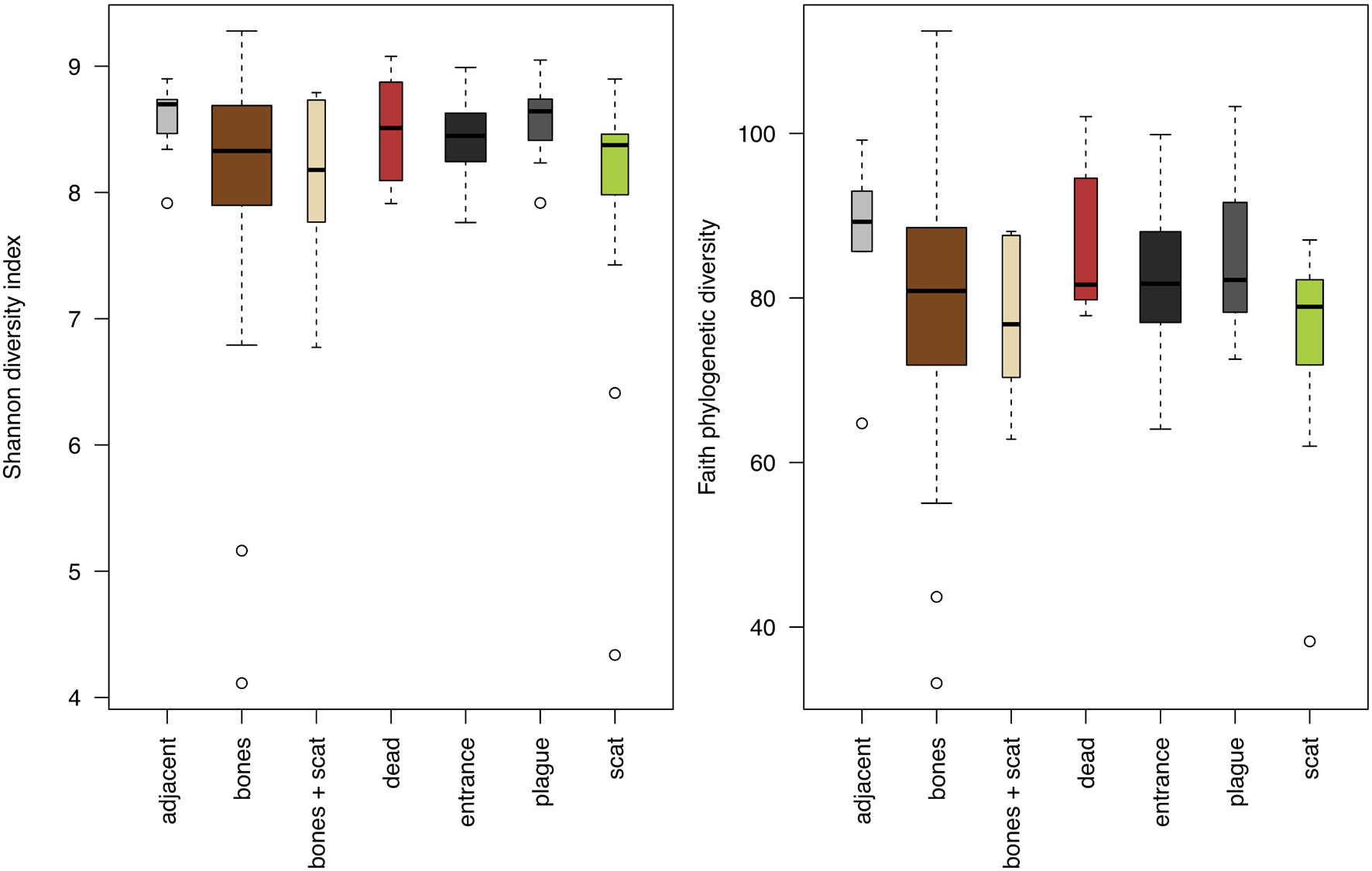
Figure 2. Fine-scale variation in microbial diversity among regions of the prairie dog burrow; width of boxes represents sample size. Left: ecological diversity measured by the Shannon index. Right: phylogenetic diversity measured by the Faith phylogenetic diversity index. Width of boxes represents relative sample sizes.
Adding the relative abundance of Enterobacteriaceae improved the AIC of both models (Shannon diversity AIC 252.05, significant improvement; Faith diversity AIC 1124.3, marginal improvement). The relative abundance of Enterobacteriaceae had a negative effect on both ecological and phylogenetic diversity, although this effect seemed to be driven by an outlier with a relatively high proportion of Enterobacteriaceae and low diversity. Removing the outlier changed the magnitude (and significance) of the relationship, but the trend toward an inverse relationship persisted. The improvement of model fit with the inclusion of relative abundance of Enterobacteriaceae was not unique to this family; in fact, the inclusion of 157 single taxa significantly improved model fit (reducing AIC by more than 2); 59 families (one at a time) reduced AIC values by >10. In particular, the best single-taxon model of Shannon’s diversity included relative abundance of Planococcaceae (AIC 188.53) in addition to the previous predictors, and the only other model within 10 AIC was a model including relative abundance of Micrococcaceae (AIC 194.10). Both of these taxa exhibited a strong negative relationship with Shannon’s diversity. Similarly, the inclusion of 157 single taxa significantly improved model fit (reducing AIC by more than 2) for phylogenetic diversity, and 67 families reduced AIC values by >10. The best single-taxon model of phylogenetic diversity included the relative abundance of an unknown family in order WD2101 (class Phycisphaerae, AIC 1064.83) in addition to the previous predictors, and the only other model within 10 AIC was a model including relative abundance of an unknown family in order iii1–15 (class Acidobacteria-6, AIC 1071.36). Both of these taxa exhibited a positive relationship with phylogenetic diversity.
Burrow regions differed significantly in the most abundant taxa at all taxonomic levels (phylum, class, order, family, and genus; Supplementary Figure 4). Across all samples, the dominant family averaged 12.5% of the total sequences per sample, and ranged from comprising 5.6–49.2% of the total sequences per sample. In soils collected from burrows inferred to currently contain dead animals, Firmicutes were more abundant than expected (Chi-square = 74.528, df = 30, p-value = 1.172e−05). Burrows with dead animals contained more Bacilli (and Bacillales) and Rubrobacteria (and Rubrobacterales) than expected, while soils containing bones were characterized by a lower abundance of Alphaproteobacteria than expected (Chi-square = 182.36, df = 72, p-value = 1.545e−11). Soils containing bones and scat possessed a lower abundance of Rhizobiales than expected (Chi-square = 234.44, df = 96, p-value = 1.382e−13).
Forty-eight bacterial families and 76 bacterial genera varied significantly in relative abundance across burrow regions (Supplementary Tables 2–5). Among the taxa most significantly varying across burrow regions were an unknown family and genus in the AKIW781 order of class Chloroflexi, which was an order of magnitude higher in soil with bones and scat (Figure 3A); Deinococcus (Deinococcaceae), which was an order of magnitude higher in soil with bones and scat and an order of magnitude lower in soil associated with dead animals (Figure 3B); an unknown genus in Planococcaceae, which was highest in soil associated with dead animals (Figure 3C); and Cellulosimicrobium (Promicromonosporaceae, Actinomycetales), which was highest in soils sampled with scat (Figure 3D).
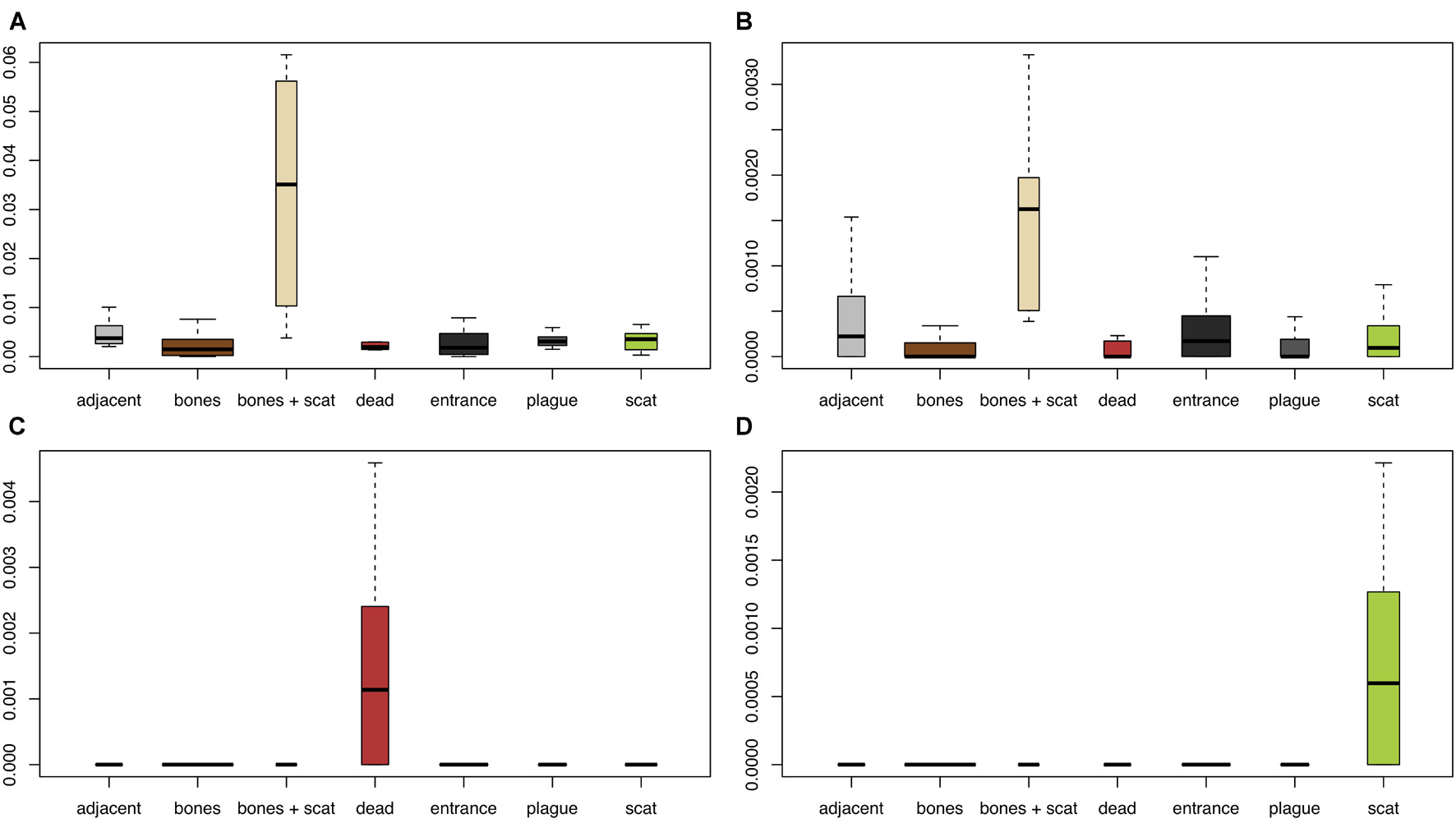
Figure 3. Variation in relative abundance of four representative microbial taxa among regions of the prairie dog burrow. (A) An unknown family and genus in the AKIW781 order of class Chloroflexi, (B) Deinococcus (Deinococcaceae), (C) an unknown genus in Planococcaceae, and (D) Cellulosimicrobium (Promicromonosporaceae, Actinomycetales). Y-axes are the percentage of total microbial sequences that comprise represented taxa. Width of boxes represents relative sample sizes.
Enterobacteriaceae, the family containing Y. pestis, was found in all soil samples, but at low proportions (never exceeding 3%). The proportion of Enterobacteriaceae sequences was significantly higher in samples with higher C:N (p = 0.0002) and in burrow regions associated with dead animals than in other regions (p = 0.013). Although we ran this model first due to our particular interest in the family, we also aimed to determine the extent to which spatial variation in abundance was characteristic shared by many microbial taxa. When we ran separate models for all 565 families and 990 genera, the false discovery rate correction led to a loss of statistical significance for spatial variation in Enterobacteriaceae (data not shown). Yersinia was identified in four samples from two burrows in one colony (19A). All Yersinia-containing samples were collected from waste chambers. Presence of this genus was a significant predictor of the relative abundance of five bacterial families and eight microbial genera (Figure 4 and Tables 1, 2). All of these taxa were found in significantly higher abundance in samples where Yersinia was present. Many of these genera (e.g., 9 out of the 10 strongest associations) were extremely rare taxa that appeared only or primarily in the samples containing Yersinia. The presence of Yersinia in a sample was associated with slightly, but not significantly, lower microbial diversity within samples (Shannon without Yersinia 8.303, Shannon with Yersinia 8.059, p = 0.14; Faith PD without Yersinia 81.807, Faith PD with Yersinia 75.111, p = 0.17; Figure 5).
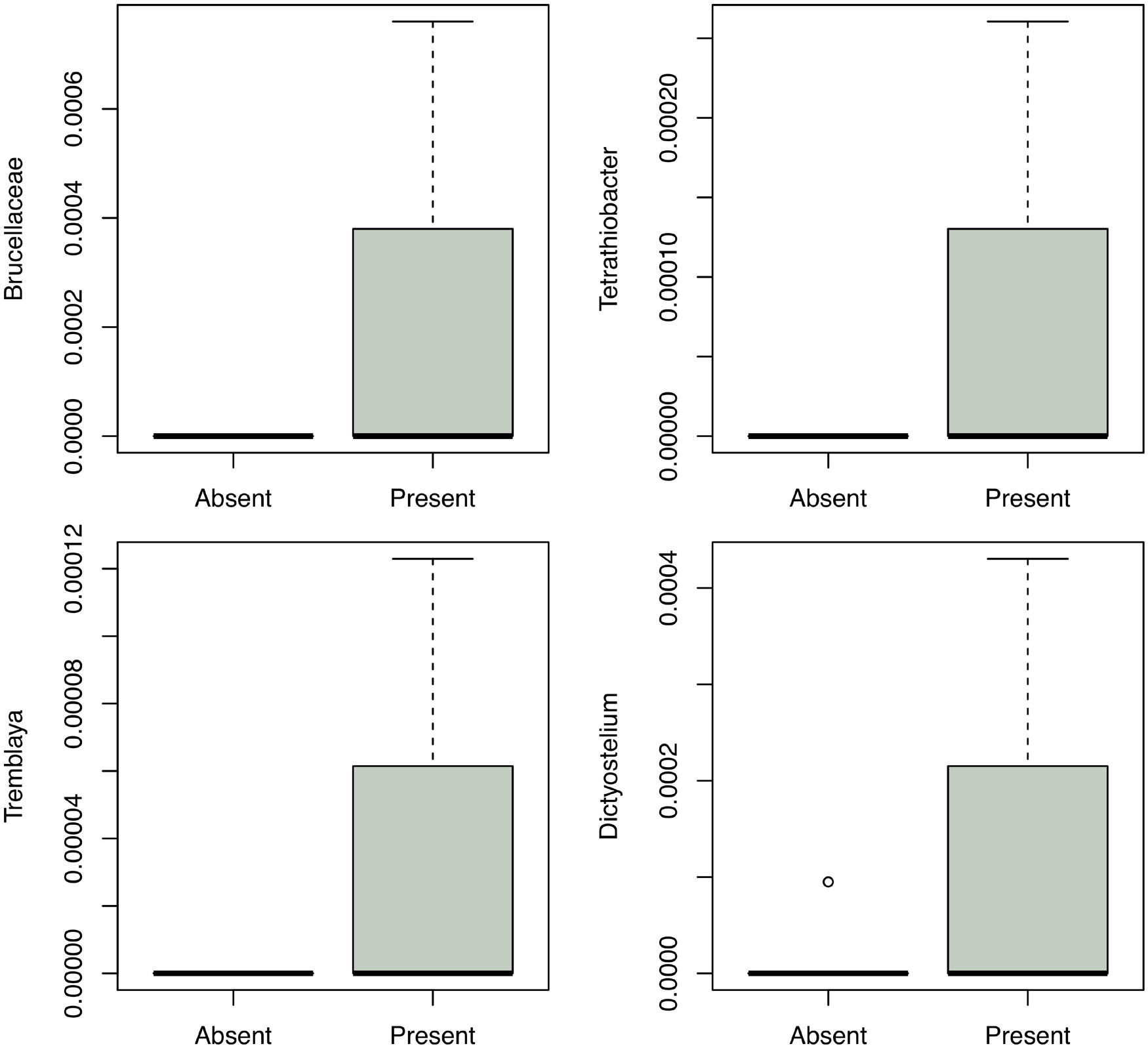
Figure 4. Representative genera that varied in relative abundance in soils with Yersinia relative to soils without Yersinia.

Table 1. Classification of bacterial families found at significantly higher abundance in soil samples containing Yersinia.
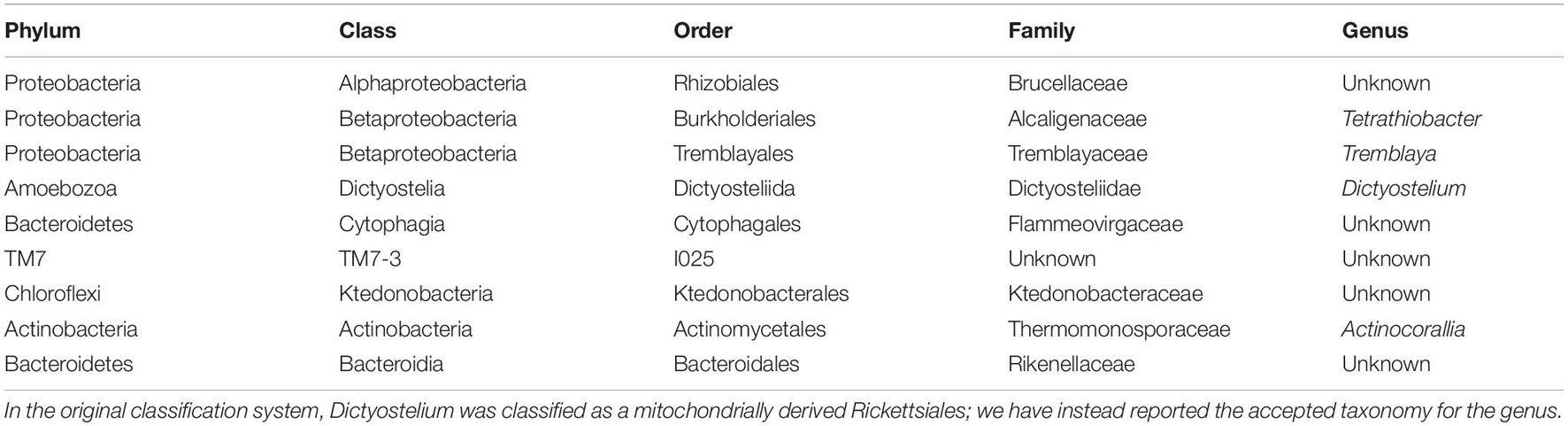
Table 2. Classification of microbial genera found at significantly higher abundance in soil samples containing Yersinia.
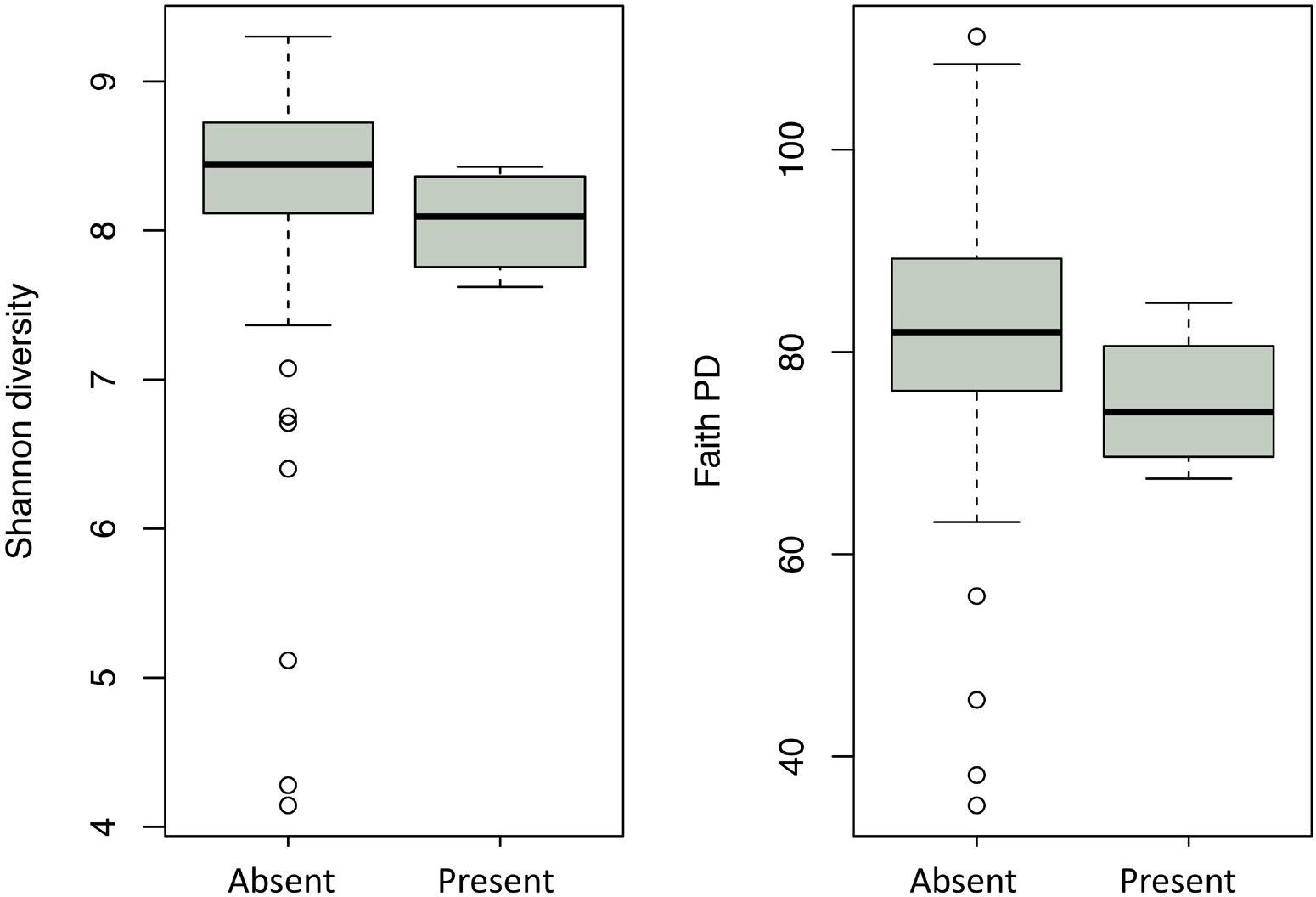
Figure 5. Microbial diversity in soils with and without Yersinia. Left: ecological diversity measured by the Shannon index. Right: phylogenetic diversity measured by the Faith phylogenetic diversity index.
Discussion
Microbial communities as a whole varied – and many specific taxa differed in relative abundance – at small spatial scales among regions of a prairie dog burrow following a mass mortality event. Dominant taxa were consistent with predictions of microbial succession following the nutrient pulse that occurs during decomposition of mammalian corpses (Metcalf et al., 2016a, b). In addition, several taxa were significantly associated with the presence of Yersinia in soil samples, primarily as a result of taxa of low abundance found at higher abundance when Yersinia was present. Both ecological and phylogenetic diversity resulted from the combined influences of soil properties and burrow region.
Other studies have shown similar degrees of fine-scale spatial structure in microbial communities resulting from niche differentiation (Zhuang et al., 2020), particularly in microbial communities associated with plant roots (Aas et al., 2019) and other plant tissues (Cregger et al., 2018). Niche diversification may be particularly likely when niches are divergent even at small spatial scales, when specific microbes present in high abundance in certain environments exert selection on other microbial taxa (e.g., predatory microbes) or when microenvironments are less hospitable (e.g., very dry). In this system, microbial communities associated with scat may be specialized for living in the mammalian gut, metabolizing plant tissues, or both. Soil collected with bones were the driest soils we sampled, thereby potentially exerting strong selection on microbial communities in these soils.
Fine-scale spatial structure could also arise from community assembly (Nemergut et al., 2013) and succession processes such as colonization of a deceased animal from soil microbiota (Metcalf et al., 2016b), particularly if animals died in a spatially structured way or were moved to specific locations after death – scenarios that are consistent with the few existing observations of deceased prairie dogs within burrows (Burns et al., 1989). For instance, Enterobacteriaceae are abundant in the early stages of corpse decay, while Planococcaceae become more abundant as corpse decay progresses (Metcalf et al., 2016a). This is consistent with our observation of significantly higher abundance of both taxa in soils collected near dead animals.
Keystone microbial taxa (Banerjee et al., 2018) can influence the abundance of other community members based on ecological interactions (Herren and McMahon, 2018) including the prevention of pathogen establishment (Trivedi et al., 2017). We found >50 taxa that significantly influenced ecological or phylogenetic diversity among samples, with some having particularly strong effects. Four single taxa [Planococcaceae, Micrococcaceae, unknown family in WD2101 (Planctomycetes), and unknown family in iii1–15 (Acidobacteria)] were statistically separated as predictors of diversity (in conjunction with abiotic soil properties) from other taxa, indicating their potential role as keystone taxa. A negative relationship between Planococcaceae and ecological diversity supports previous findings of this family becoming more abundant after disturbance of an ecological community (Aanderud et al., 2019). Micrococcaceae have been associated with increased plant growth (Hong et al., 2016), which could cause feedbacks with microbial diversity, although the mechanism underlying this potential relationship is not clear. Both WD2101 and iii1–15 are among the most abundant soil bacteria globally (Delgado-Baquerizo et al., 2018). The lack of taxon sharing within WD2101 (<2% shared OTUs) even in similar environments (Dedysh et al., 2020) and the low degree of genomic match to characterized sequences (Delgado-Baquerizo et al., 2018) suggest a large amount of cryptic diversity in the group that could be a driving force behind the high phylogenetic diversity we found here. The abundance of iii1–15 responds to soil moisture (Barnard et al., 2013), which could provide a mechanism for its relationship with phylogenetic diversity (Brockett et al., 2012).
Among the taxa that varied spatially within prairie dog burrows was an unknown member of the AKIW781 class (order Chloroflexi), found here with bones and scat, which has previously been described in soils from deserts in North and South America (Mogul et al., 2017; Lucas et al., 2020) and is likely adapted to dry conditions. Similarly, we found Deinococcus to be higher in soils with bones and scat, which may be not only drier but more exposed to sunlight than soils excavated from other parts of the burrow. Deinococcus is resistant to solar radiation and increases in relative abundance in irradiated soils (Ogwu et al., 2019). An unknown genus in Planococcaceae was highest in soils associated with dead animals, consistent with previous description of the abundance of this family in later stages of the decomposition process (Metcalf et al., 2016a). Finally, Cellulosimicrobium was found at highest relative abundance in soils containing scat, which supports the role of this genus in breaking down plant material (Bakalidou et al., 2002; Schumann and Stackebrandt, 2015).
In line with other studies of pathogens and soil microbial diversity (van Elsas et al., 2012), the presence of Yersinia in a sample was negatively associated with microbial diversity (although the relationship here was not significant). The most notable microbial association with Yersinia was with Dictyostelium, an amoeba that consumes bacteria. Previous experimental work has shown that Y. pestis can escape phagocytosis by and replicate within D. discoideum for at least 48 h (in comparison with control bacteria, which were consumed within 1 h; Markman et al., 2018). The prevalence of Dictyostelium (present in 2 of 158 samples) and another amoeba, Acanthamoeba (10 of 158 samples), in our soils was lower than that recovered in Markman et al. (2018), although the methods of recovery differed. To our knowledge, this is the first study to detect Yersinia in soil samples collected from prairie dog colonies. Although we were unable to classify the sequences at the species level due to read length constraints, this suggestive finding adds to the collective evidence that Y. pestis is present in prairie dog colonies in the absence of epizootics (3 years after the prairie-dog population die-off) and that soil amoebae may be a potential reservoir for plague in inter-epizootic intervals.
Our results show that variation in soil microbial communities occurs at fine spatial scales in relation to functional partitioning of below-ground space by a social mammalian herbivore. This fine-scale structure likely interacts with mass mortality events, for example by sudden drastic increases in input to certain physical burrow regions (e.g., chambers used for quarantining dead individuals). The existence of fine-scale spatial structure in community diversity in this and other studies suggests that estimates of beta-diversity should account for fine-scale structure in order to accurately estimate the true degree of diversity. Collectively, our results demonstrate how soil microbial communities can interact with animal pathogens (van Elsas et al., 2012; Trivedi et al., 2017) to shape above- and below-ground biodiversity in grasslands.
Data Availability Statement
The datasets presented in this study can be found in online repositories. The names of the repository/repositories and accession number(s) can be found below: https://qiita.microbio.me/emp, 11519; https://www.ebi.ac.uk/ena/browser/view/ERP106314.
Author Contributions
LC-S conceived, designed, and oversaw this study. CK and LC-S analyzed the data and wrote the manuscript. Both authors contributed to the article and approved the submitted version.
Funding
This project was funded through a crowdfunding initiative hosted by RocketHub. Open Access charges were funded through a startup award to LC-S by the University of Louisiana.
Conflict of Interest
The authors declare that the research was conducted in the absence of any commercial or financial relationships that could be construed as a potential conflict of interest.
Publisher’s Note
All claims expressed in this article are solely those of the authors and do not necessarily represent those of their affiliated organizations, or those of the publisher, the editors and the reviewers. Any product that may be evaluated in this article, or claim that may be made by its manufacturer, is not guaranteed or endorsed by the publisher.
Acknowledgments
We are grateful to Se Jin Song for guidance and discussions regarding methodology, to Holly Archer for extracting DNA from the soils, and to Tim Seastedt, Stower Beals, Robin Reibold, and Shivani Ehrenfeucht for measuring soil moisture, pH, and CHN. Sample processing, 16S sequencing, and core amplicon data analysis were performed by the Earth Microbiome Project (www.earthmicrobiome.org). This project was funded through a crowdfunding initiative and we are grateful to each funder for making it possible.
Supplementary Material
The Supplementary Material for this article can be found online at: https://www.frontiersin.org/articles/10.3389/fevo.2021.758348/full#supplementary-material
Footnotes
- ^ Seastedt, T. R., Porazinska, D. L., Gendron, E. M. S., and Schmidt, S. K. (submitted). An annual grass restructures the soil food web and alters soil carbon sequestration of a perennial grassland. Plant Soil
- ^ www.earthmicrobiome.org
- ^ qiita.microbio.me/emp
References
Aanderud, Z. T., Bahr, J., Robinson, D. M., Belnap, J., Campbell, T. P., Gill, R. A., et al. (2019). The burning of biocrusts facilitates the emergence of a bare soil community of poorly-connected chemoheterotrophic bacteria with depressed ecosystem services. Front. Ecol. Evol. 7:467. doi: 10.3389/fevo.2019.00467
Aas, A. B., Andrew, C. J., Blaalid, R., Vik, U., Kauserud, H., and Davey, M. L. (2019). Fine-scale diversity patterns in belowground microbial communities are consistent across kingdoms. Fems Microbiol. Ecol. 95:fiz058. doi: 10.1093/femsec/fiz058
Anacker, B., Seastedt, T. R., Halward, T. M., and Lezberg, A. L. (2021). Soil carbon and plant richness relationships differ among grassland types, disturbance history and plant functional groups. Oecologia 196, 1153–1166. doi: 10.1007/s00442-021-04992-x
Bai, Y., Kosoy, M. Y., Ray, C., Brinkerhoff, R. J., and Collinge, S. K. (2008). Temporal and spatial patterns of Bartonella infection in black-tailed prairie dogs (Cynomys ludovicianus). Microb. Ecol. 56, 373–382. doi: 10.1007/s00248-007-9355-6
Bakalidou, A., Kämpfer, P., Berchtold, M., Kuhnigk, T., Wenzel, M., and König, H. (2002). Cellulosimicrobium variabile sp. nov., a cellulolytic bacterium from the hindgut of the termite Mastotermes darwiniensis. Int. J. Syst. Evol. Microbiol. 52, 1185–1192. doi: 10.1099/00207713-52-4-1185
Banerjee, S., Schlaeppi, K., and van der Heijden, M. G. (2018). Keystone taxa as drivers of microbiome structure and functioning. Nat. Rev. Microbiol. 16, 567–576. doi: 10.1038/s41579-018-0024-1
Barnard, R. L., Osborne, C. A., and Firestone, M. K. (2013). Responses of soil bacterial and fungal communities to extreme desiccation and rewetting. ISME J. 7, 2229–2241. doi: 10.1038/ismej.2013.104
Bartelt-Ryser, J., Joshi, J., Schmid, B., Brandl, H., and Balser, T. (2005). Soil feedbacks of plant diversity on soil microbial communities and subsequent plant growth. Perspect. Plant Ecol. Evol. Syst. 7, 27–49. doi: 10.1016/j.ppees.2004.11.002
Benjamini, Y., and Yekutieli, D. (2001). The control of the false discovery rate in multiple testing under dependency. Ann. Stat. 29, 1165–1188. doi: 10.1214/aos/1013699998
Bokulich, N. A., Kaehler, B. D., Rideout, J. R., Dillon, M., Bolyen, E., Knight, R., et al. (2018). Optimizing taxonomic classification of marker-gene amplicon sequences with QIIME 2’s q2-feature-classifier plugin. Microbiome 6, 1–17. doi: 10.1186/s40168-018-0470-z
Bolyen, E., Rideout, J. R., Dillon, M. R., Bokulich, N. A., Abnet, C. C., Al-Ghalith, G. A., et al. (2019). Reproducible, interactive, scalable and extensible microbiome data science using QIIME 2. Nat. Biotechnol. 37, 852–857. doi: 10.1038/s41587-019-0209-9
Bray, N., Kao-Kniffin, J., Frey, S. D., Fahey, T., and Wickings, K. (2019). Soil macroinvertebrate presence alters microbial community composition and activity in the rhizosphere. Front. Microbiol. 10:256. doi: 10.3389/fmicb.2019.00256
Brockett, B. F., Prescott, C. E., and Grayston, S. J. (2012). Soil moisture is the major factor influencing microbial community structure and enzyme activities across seven biogeoclimatic zones in western Canada. Soil Biol. Biochem. 44, 9–20. doi: 10.1016/j.soilbio.2011.09.003
Burns, J. A., Flath, D. L., and Clark, T. W. (1989). On the structure and function of white-tailed prairie dog burrows. Great Basin Nat. 49, 517–524.
Callahan, B. J., McMurdie, P. J., Rosen, M. J., Han, A. W., Johnson, A. J. A., and Holmes, S. P. (2016). DADA2: high-resolution sample inference from Illumina amplicon data. Nat. Methods 13, 581–583. doi: 10.1038/nmeth.3869
Ciadamidaro, L., Madejón, E., Puschenreiter, M., and Madejón, P. (2013). Growth of Populus alba and its influence on soil trace element availability. Sci. Tot. Envviron. 454, 337–347. doi: 10.1016/j.scitotenv.2013.03.032
Cline, L. C., Zak, D. R., Upchurch, R. A., Freedman, Z. B., and Peschel, A. R. (2017). Soil microbial communities and elk foraging intensity: implications for soil biogeochemical cycling in the sagebrush steppe. Ecol. Lett. 20, 202–211. doi: 10.1111/ele.12722
Cooke, L. A., and Swiecki, S. R. (1992). Structure of a white-tailed prairie dog burrow. Great Basin. Natural. 52:12.
Cregger, M. A., Veach, A. M., Yang, Z. K., Crouch, M. J., Vilgalys, R., Tuskan, G. A., et al. (2018). The Populus holobiont: dissecting the effects of plant niches and genotype on the microbiome. Microbiome 6, 1–14. doi: 10.1186/s40168-018-0413-8
Cully, J. F. Jr., Johnson, T. L., Collinge, S. K., and Ray, C. (2010). Disease limits populations: plague and black-tailed prairie dogs. Vector Borne Zoonotic Dis. 10, 7–15. doi: 10.1089/vbz.2009.0045
Dedysh, S. N., Beletsky, A. V., Ivanova, A. A., Kulichevskaya, I. S., Suzina, N. E., Philippov, D. A., et al. (2020). Wide distribution of Phycisphaera-like planctomycetes from WD2101 soil group in peatlands and genome analysis of the first cultivated representative. Environ. Microbiol. 23, 1510–1526. doi: 10.1111/1462-2920.15360
Delgado-Baquerizo, M., Oliverio, A. M., Brewer, T. E., Benavent-González, A., Eldridge, D. J., Bardgett, R. D., et al. (2018). A global atlas of the dominant bacteria found in soil. Science 359, 320–325. doi: 10.1126/science.aap9516
DeSantis, T. Z., Hugenholtz, P., Larsen, N., Rojas, M., Brodie, E. L., Keller, K., et al. (2006). Greengenes, a chimera-checked 16S rRNA gene database and workbench compatible with ARB. Appl. Environ. Microbiol. 72, 5069–5072. doi: 10.1128/AEM.03006-05
Eisen, R. J., Petersen, J. M., Higgins, C. L., Wong, D., Levy, C. E., Mead, P. S., et al. (2008). Persistence of Yersinia pestis in soil under natural conditions. Emerg. Infect. Dis. 14, 941–943. doi: 10.3201/eid1406.080029
Faith, D. P. (1992). Conservation evaluation and phylogenetic diversity. Biol. Conserv. 61, 1–10. doi: 10.1016/0006-3207(92)91201-3
Garcia, M. O., Templer, P. H., Sorensen, P. O., Sanders-DeMott, R., Groffman, P. M., and Bhatnagar, J. M. (2020). Soil microbes trade-off biogeochemical cycling for stress tolerance traits in response to year-round climate change. Front. Microbiol. 11:616. doi: 10.3389/fmicb.2020.00616
Gedeon, C. I., Drickamer, L. C., and Sanchez-Meador, A. J. (2012). Importance of burrow-entrance mounds of Gunnison’s prairie dogs (Cynomys gunnisoni) for vigilance and mixing of soil. Southwest. Nat. 57, 100–104.
Gonzalez, A., Navas-Molina, J. A., Kosciolek, T., McDonald, D., Vázquez-Baeza, Y., Ackermann, G., et al. (2018). QIITA: rapid, web-enabled microbiome meta-analysis. Nat. Methods 15, 796–798. doi: 10.1038/s41592-018-0141-9
Gutiérrez, J. L., and Jones, C. G. (2006). Physical ecosystem engineers as agents of biogeochemical heterogeneity. BioScience 56, 227–236. doi: 10.1641/0006-3568(2006)056[0227:PEEAAO]2.0.CO;2
Heijboer, A., de Ruiter, P. C., Bodelier, P. L., and Kowalchuk, G. A. (2018). Modulation of litter decomposition by the soil microbial food web under influence of land use change. Front. Microbiol. 9:2860. doi: 10.3389/fmicb.2018.02860
Herren, C. M., and McMahon, K. D. (2018). Keystone taxa predict compositional change in microbial communities. Envviron. Microbiol. 20, 2207–2217. doi: 10.1111/1462-2920.14257
Hestrin, R., Hammer, E. C., Mueller, C. W., and Lehmann, J. (2019). Synergies between mycorrhizal fungi and soil microbial communities increase plant nitrogen acquisition. Commun. Biol. 2, 1–9. doi: 10.1038/s42003-019-0481-8
Holland, E. A., and Detling, J. K. (1990). Plant response to herbivory and belowground nitrogen cycling. Ecology 71, 1040–1049. doi: 10.2307/1937372
Hong, S. H., Ham, S. Y., Kim, J. S., Kim, I. S., and Lee, E. Y. (2016). Application of sodium polyacrylate and plant growth-promoting bacterium, Micrococcaceae HW-2, on the growth of plants cultivated in the rooftop. Int. Biodeterioration Biodegradation 113, 297–303. doi: 10.1016/j.ibiod.2016.04.018
Hoogland, J. L. (1995). The Black-Tailed Prairie Dog: Social Life of a Burrowing Mammal. Chicago, IL: University of Chicago Press.
Ichinohe, T., Pang, I. K., Kumamoto, Y., Peaper, D. R., Ho, J. H., Murray, T. S., et al. (2011). Microbiota regulates immune defense against respiratory tract influenza A virus infection. Proc. Natl. Acad. Sci. U.S.A. 108, 5354–5359. doi: 10.1073/pnas.1019378108
Kandeler, E., Kampichler, C., Joergensen, R. G., and Mölter, K. (1999). Effects of mesofauna in a spruce forest on soil microbial communities and N cycling in field mesocosms. Soil Biol. Biochem. 31, 1783–1792. doi: 10.1016/S0038-0717(99)00096-6
Katoh, K., and Standley, D. M. (2013). MAFFT multiple sequence alignment software version 7: improvements in performance and usability. Mol. Biol. Evol. 30, 772–780. doi: 10.1093/molbev/mst010
Konopka, A. (2009). What is microbial community ecology? ISME J. 3, 1223–1230. doi: 10.1038/ismej.2009.88
Lange, M., Eisenhauer, N., Sierra, C. A., Bessler, H., Engels, C., Griffiths, R. I., et al. (2015). Plant diversity increases soil microbial activity and soil carbon storage. Nat. Commun. 6, 1–8. doi: 10.1038/ncomms7707
Lau, J. A., and Lennon, J. T. (2011). Evolutionary ecology of plant–microbe interactions: soil microbial structure alters selection on plant traits. New Phytol. 192, 215–224. doi: 10.1111/j.1469-8137.2011.03790.x
Laverock, B., Smith, C. J., Tait, K., Osborn, A. M., Widdicombe, S., and Gilbert, J. A. (2010). Bioturbating shrimp alter the structure and diversity of bacterial communities in coastal marine sediments. ISME J. 4, 1531–1544. doi: 10.1038/ismej.2010.86
Leff, J. W., Jones, S. E., Prober, S. M., Barberán, A., Borer, E. T., Firn, J. L., et al. (2015). Consistent responses of soil microbial communities to elevated nutrient inputs in grasslands across the globe. Proc. Natl. Acad. Sci. U.S.A. 112, 10967–10972. doi: 10.1073/pnas.1508382112
Li, Y., Adams, J., Shi, Y., Wang, H., He, J. S., and Chu, H. (2017). Distinct soil microbial communities in habitats of differing soil water balance on the Tibetan Plateau. Sci. Rep. 7:46407.
Lozupone, C., and Knight, R. (2005). UniFrac: a new phylogenetic method for comparing microbial communities. Appl. Environ. Microbiol. 71, 8228–8235. doi: 10.1128/AEM.71.12.8228-8235.2005
Lozupone, C. A., Hamady, M., Kelley, S. T., and Knight, R. (2007). Quantitative and qualitative β diversity measures lead to different insights into factors that structure microbial communities. Appl. Environ. Microbiol. 73, 1576–1585. doi: 10.1128/AEM.01996-06
Lucas, D. A., Cahill, T. M., and Marshall, P. A. (2020). A microbiome analysis of soil samples from three abandoned lead-silver mines in the arizona sonoran desert. J. Arizona-Nevada Acad. Sci. 49, 1–15. doi: 10.2181/036.049.0101
Markman, D. W., Antolin, M. F., Bowen, R. A., Wheat, W. H., Woods, M., Gonzalez-Juarrero, M., et al. (2018). Yersinia pestis survival and replication in potential ameba reservoir. Emerg. Infect. Dis. 24:294. doi: 10.3201/eid2402.171065
McDonald, D., Price, M. N., Goodrich, J., Nawrocki, E. P., DeSantis, T. Z., Probst, A., et al. (2012). An improved Greengenes taxonomy with explicit ranks for ecological and evolutionary analyses of bacteria and archaea. ISME J. 6, 610–618. doi: 10.1038/ismej.2011.139
Metcalf, J. L., Xu, Z. Z., Weiss, S., Lax, S., Van Treuren, W., Hyde, E. R., et al. (2016b). Microbial community assembly and metabolic function during mammalian corpse decomposition. Science 351, 158–162. doi: 10.1126/science.aad2646
Metcalf, J. L., Carter, D. O., and Knight, R. (2016a). Microbiology of death. Curr. Biol. 26, R561–R563. doi: 10.1016/j.cub.2016.03.042
Mogul, R., Vaishampayan, P., Bashir, M., McKay, C. P., Schubert, K., Bornaccorsi, R., et al. (2017). Microbial community and biochemical dynamics of biological soil crusts across a gradient of surface coverage in the Central Mojave Desert. Front. Microbiol. 8:1974. doi: 10.3389/fmicb.2017.01974
Munn, L. C. (1993). “Effects of prairie dogs on physical and chemical properties of soils,” in Management of Prairie Dog Complexes for the Reintroduction of the Black-Footed Ferret, ed. J. L. Oldemeyer (Washington, DC: US Department of the Interior), 11–17.
Nemergut, D. R., Schmidt, S. K., Fukami, T., O’Neill, S. P., Bilinski, T. M., Stanish, L. F., et al. (2013). Patterns and processes of microbial community assembly. Microbiol. Mol. Biol. Rev. 77, 342–356. doi: 10.1128/MMBR.00051-12
Ogwu, M. C., Srinivasan, S., Dong, K., Ramasamy, D., Waldman, B., and Adams, J. M. (2019). Community ecology of Deinococcus in irradiated soil. Microb. Ecol. 78, 855–872. doi: 10.1007/s00248-019-01343-5
Perez, C., Dill-Macky, R., and Kinkel, L. L. (2008). Management of soil microbial communities to enhance populations of Fusarium graminearum-antagonists in soil. Plant Soil 302, 53–69. doi: 10.1007/s11104-007-9455-6
Prescott, C. E., and Grayston, S. J. (2013). Tree species influence on microbial communities in litter and soil: current knowledge and research needs. For. Ecol. Manage. 309, 19–27. doi: 10.1016/j.foreco.2013.02.034
Price, M. N., Dehal, P. S., and Arkin, A. P. (2010). FastTree 2–approximately maximum-likelihood trees for large alignments. PLoS One 5:e9490. doi: 10.1371/journal.pone.0009490
R Core Team, (2018). R: A Language and Environment for Statistical Computing. R Foundation for Statistical Computing, Vienna, Austria. Vienna: R Core Team.
Sackett, L. C., Collinge, S. K., and Martin, A. P. (2013). Do pathogens reduce genetic diversity of their hosts? Variable effects of sylvatic plague in black-tailed prairie dogs. Mol. Ecol. 22, 2441–2455.
Salkeld, D. J., Salathé, M., Stapp, P., and Jones, J. H. (2010). Plague outbreaks in prairie dog populations explained by percolation thresholds of alternate host abundance. Proc. Natl. Acad. Sci. U.S.A. 107, 14247–14250. doi: 10.1073/pnas.1002826107
Schumann, P., and Stackebrandt, E. (2015). Cellulosimicrobium. Bergey’s Manual of Systematics of Archaea and Bacteria. 1–9. doi: 10.1002/9781118960608.gbm00127
Shen, C., Xiong, J., Zhang, H., Feng, Y., Lin, X., Li, X., et al. (2013). Soil pH drives the spatial distribution of bacterial communities along elevation on Changbai Mountain. Soil. Biol. Biochem. 57, 204–211. doi: 10.1016/j.soilbio.2012.07.013
Shen, Z., Penton, C. R., Lv, N., Xue, C., Yuan, X., Ruan, Y., et al. (2018). Banana Fusarium wilt disease incidence is influenced by shifts of soil microbial communities under different monoculture spans. Microb. Ecol. 75, 739–750. doi: 10.1007/s00248-017-1052-5
Thompson, L. R., Sanders, J. G., McDonald, D., Amir, A., Jansson, J. K., Gilbert, J. A., et al. (2017). A communal catalogue reveals Earth’s multiscale microbial diversity. Nature 551, 457–463. doi: 10.1038/nature24621
Treseder, K. K., Marusenko, Y., Romero-Olivares, A. L., and Maltz, M. R. (2016). Experimental warming alters potential function of the fungal community in boreal forest. Glob. Change Biol. 22, 3395–3404. doi: 10.1111/gcb.13238
Trivedi, P., Delgado-Baquerizo, M., Trivedi, C., Hamonts, K., Anderson, I. C., and Singh, B. K. (2017). Keystone microbial taxa regulate the invasion of a fungal pathogen in agro-ecosystems. Soil. Biol. Biochem. 111, 10–14. doi: 10.1016/j.soilbio.2017.03.013
van Elsas, J. D., Chiurazzi, M., Mallon, C. A., Elhottovā, D., Krištůfek, V., and Salles, J. F. (2012). Microbial diversity determines the invasion of soil by a bacterial pathogen. Proc. Natl. Acad. Sci. U.S.A. 109, 1159–1164. doi: 10.1073/pnas.1109326109
Vázquez-Baeza, Y., Pirrung, M., Gonzalez, A., and Knight, R. (2013). EMPeror: a tool for visualizing high-throughput microbial community data. Gigascience 2:16. doi: 10.1186/2047-217X-2-16
Wang, Q., Garrity, G. M., Tiedje, J. M., and Cole, J. R. (2007). Naive Bayesian classifier for rapid assignment of rRNA sequences into the new bacterial taxonomy. Appl. Environ. Microb. 73, 5261–5267. doi: 10.1128/AEM.00062-07
Webb, C. T., Brooks, C. P., Gage, K. L., and Antolin, M. F. (2006). Classic flea-borne transmission does not drive plague epizootics in prairie dogs. Proc. Natl. Acad. Sci. U.S.A. 103, 6236–6241. doi: 10.1073/pnas.0510090103
Whicker, A. D., and Detling, J. K. (1988). Ecological consequences of prairie dog disturbances. BioScience 38, 778–785. doi: 10.2307/1310787
Wilcomb, M. J. (1954). A Study of Prairie Dog Burrow Systems and the Ecology of Their Arthropod Inhabitants in Central Oklahoma. Doctoral dissertation. Oklahoma, OK: University of Oklahoma.
Xi, N., Chu, C., and Bloor, J. M. (2018). Plant drought resistance is mediated by soil microbial community structure and soil-plant feedbacks in a savanna tree species. Environ. Exp. Bot. 155, 695–701. doi: 10.1016/j.envexpbot.2018.08.013
Xia, Z., Yang, J., Sang, C., Wang, X., Sun, L., Jiang, P., et al. (2020). Phosphorus reduces negative effects of nitrogen addition on soil microbial communities and functions. Microorganisms 8:1828. doi: 10.3390/microorganisms8111828
Zak, D. R., Holmes, W. E., White, D. C., Peacock, A. D., and Tilman, D. (2003). Plant diversity, soil microbial communities, and ecosystem function: are there any links? Ecology 84, 2042–2050. doi: 10.1890/02-0433
Zhuang, W., Yu, X., Hu, R., Luo, Z., Liu, X., Zheng, X., et al. (2020). Diversity, function and assembly of mangrove root-associated microbial communities at a continuous fine-scale. NPJ Biofilms Microbiomes 6, 1–10. doi: 10.1038/s41522-020-00164-6
Keywords: environmental microbiome project, nutrient pulse, grasslands, pathogens, extirpation, spatial partitioning
Citation: Kaufmann C and Cassin-Sackett L (2021) Fine-Scale Spatial Structure of Soil Microbial Communities in Burrows of a Keystone Rodent Following Mass Mortality. Front. Ecol. Evol. 9:758348. doi: 10.3389/fevo.2021.758348
Received: 13 August 2021; Accepted: 24 September 2021;
Published: 15 October 2021.
Edited by:
Susana Caballero, University of Los Andes, ColombiaCopyright © 2021 Kaufmann and Cassin-Sackett. This is an open-access article distributed under the terms of the Creative Commons Attribution License (CC BY). The use, distribution or reproduction in other forums is permitted, provided the original author(s) and the copyright owner(s) are credited and that the original publication in this journal is cited, in accordance with accepted academic practice. No use, distribution or reproduction is permitted which does not comply with these terms.
*Correspondence: Loren Cassin-Sackett, Y2Fzc2luLnNhY2tldHRAZ21haWwuY29t
†Present address: Loren Cassin-Sackett, Department of Biology, University of Louisiana, Lafayette, LA, United States