- 1Institute of Evolutionary Ecology and Conservation Genomics, Ulm University, Ulm, Germany
- 2Programa de Conservación de Murciélagos de Costa Rica, San José, Costa Rica
- 3Smithsonian Tropical Research Institute, Panama City, Panamá
Habitat alteration for agriculture can negatively affect wildlife physiology and health by decreasing diet diversity and increasing exposure to agrochemicals for animals foraging in altered landscapes. Such negative effects may be mediated by the disruption of the gut microbiota (termed dysbiosis), yet evidence for associations between habitat alteration, wildlife health, and the gut microbiota remains scarce. We examine the association between management intensity of banana plantations and both the body condition and gut microbiota composition of nectar-feeding bats Glossophaga soricina, which commonly forage within banana plantations across Latin America. We captured and measured 196 bats across conventional monocultures, organic plantations, and natural forests in Costa Rica, and quantified gut microbiome bacterial phylogenetic diversity using 16S rRNA amplicon sequencing. We found that gut microbiota from bats foraging in conventional monocultures were overall less phylogenetically diverse than those from bats foraging in organic plantations or natural forests, both of which were characterized by diverse bacterial assemblages and individualized microbiota. Despite lower diversity, co-occurrence network complexity was higher in conventional monocultures, potentially indicating altered microbial interactions in agricultural landscapes. Bats from both organic and conventional plantations tended to be larger and heavier than their forest counterparts, reflecting the higher food supply. Overall, our study reveals that whilst both conventional monocultures and organic plantations provide a reliable food source for bats, conventional monocultures are associated with less diverse and potentially dysbiotic microbiota, whilst organic plantations promote diverse and individualized gut microbiota akin to their natural forest-foraging counterparts. Whilst the long-term negative effects of anthropogenically-altered microbiota are unclear, our study provides further evidence from a novel perspective that organic agricultural practices are beneficial for wildlife health.
Introduction
The extent to which agriculture affects the health and physiology of persisting species is currently poorly understood. With deforestation moving at alarming rates—10 million ha lost per year between 2015 and 2020—and agricultural expansion as the main cause behind native habitat destruction (FAO and UNEP, 2020), the effects of agriculture on biodiversity have been widely explored (Dudley and Alexander, 2017). However, species that manage to thrive in agricultural habitats are faced with new challenges, such as dietary changes and, sometimes, exposure to pesticides and hormones (Henriques et al., 1997), which may lead to non-lethal yet detrimental effects to their health and physiology (Mingo et al., 2017). However, recent years have encouraged mixed organic farming practices to increase the sustainability of food production (Eyhorn et al., 2019), which may mitigate the detrimental effects of habitat conversion on wildlife diversity and individual fitness (Stein-Bachinger et al., 2020).
Negative physiological effects of agricultural landscapes may be mediated not only by a general lack of resources or by agrochemicals, but also by the disruption of the gut microbiota, an integral part of an animal’s well-being providing numerous functional benefits to the host (Suzuki, 2017). Beyond essential nutritional services, the gut microbial community influences physiological processes and triggers the immune system, contributing to host health (Brestoff and Artis, 2013). Gut microbiome diversity is shaped to a large extent by the host’s diet (Ingala et al., 2019), in addition to biological (e.g., sex, age) and environmental factors (Amato et al., 2013), yet many beneficial microbes are passed from parents to offspring via vertical transmission, thereby ensuring that they are maintained across the host population (Moeller et al., 2018).
Increasing evidence suggests that anthropogenic changes to natural habitats and associated pollution exposure can reduce the abundance of beneficial microbes, leading to a state called microbial dysbiosis (Petersen and Round, 2014). Dysbiosis is characterized by increased pathogen susceptibility (Murray et al., 2020) and an impaired gut homeostasis that can lead to loss of body condition in adults and slowed development in juveniles (Videvall et al., 2019; Gillingham et al., 2020). This in turn may reduce host fitness (Suzuki, 2017), a mechanism by which human-altered landscapes may act as ecological traps (low quality habitats that are preferred over high-quality ones; Patten and Kelly, 2010). A well-studied example is the effect of industrialization on the human gut microbiome, whereby large-scale shifts in diet and antibiotic exposure over the past 100 years has led to a negative shift in gut microbial composition (dysbiosis; Petersen and Round, 2014) and is hypothesized to contribute to prevalent diseases in industrialized societies (Sonnenburg and Sonnenburg, 2019). The equivalent effects of management intensity on wildlife health are unclear, yet there is some evidence that it modifies gut microbiome composition in some avian species (San Juan et al., 2020) and that agricultural habitats lead to lower gut microbiome diversity (Fackelmann et al., 2021). However, the effects of land use change on the gut microbiota, how this is linked to wildlife health, and specifically the extent to which organic plantations buffer wildlife gut microbiota community changes compared to conventional monocultures, remains unknown.
Several bat species appear to adapt well to agricultural land use changes (Aziz et al., 2015). Pallas’s Long-tongued Bat (Glossophaga soricina, Glossophaginae: Phyllostomidae) is one of the most widespread species in the Caribbean lowland rainforests of Costa Rica, an area that has been, to a large extent, historically converted to banana monocultures. This nectar-feeding species is highly tolerant to anthropogenically modified habitats, inhabiting agricultural areas while maintaining stable populations in the remaining natural forests (Barquez et al., 2015), so it is an ideal choice for exploring the association between the intensity of agricultural habitat alteration, and the bats’ gut microbiota and associated health effects. High food availability and the presence of structures that act as roosts have allowed G. soricina to seemingly thrive in banana plantations, where it feeds mainly from banana nectar. Both conventional and organic plantations provide them with similar banana-dominated diets, yet bats foraging in organic plantations have higher protein intake than conventional monocultures (Alpízar et al., 2020), a diet slightly more like their typical one in natural forests—nectar-carbohydrates complemented with protein from pollen and insects (Alvarez et al., 1978).
In this study, we examine the effects of habitat conversion to conventional banana monocultures and organic banana plantations on the gut microbiota of G. soricina and investigate the relation between management intensity, gut microbiota composition, and body condition. Since diet (Ingala et al., 2019) and pesticides (Kittle et al., 2018) have been shown to affect microbiomes, we expected that individuals with homogeneous diets and exposed to agrochemicals would have altered gut microbiota. Accordingly, we expected: (1) lower alpha diversity indices in conventional monocultures; (2) more similar bacterial composition (beta diversity) between bats foraging in the same habitat; (3) high levels of microbiota homogenization in bats foraging in conventional monocultures compared to their natural forests or organic plantations-foraging counterparts; (4) co-occurrence networks with lower connectivity in bats from agricultural habitats; and (5) that exposure to pesticides in conventional monocultures would lead to lower body condition indicators (smaller, heavier bats) in individuals foraging in conventional monocultures.
Materials and Methods
Focal Species, Study Area, and Sample Collection
Our focal species, Palla’s Long-tongued Bat (Glossophaga soricina, Phyllostomidae: Glossophaginae), is the most common nectar-feeding bat in our study areas in the Costa Rican Caribbean lowlands. It is a rather tolerant species to anthropogenic habitat modifications that is easily found in gardens and some plantations, thus classified as least concern by the IUCN (Barquez et al., 2015). Their main dietary items are nectar and pollen, but they complement their diet with insects, fruits, and floral parts. They roosts in tunnels, caves, buildings, hollow trees, bridges, and sewers (Alvarez et al., 1978). The genus Glossophaga tends to have small foraging ranges (3.0 ± 1.0 ha) when food availability is high (Rothenwöhrer et al., 2011). The Costa Rican Caribbean lowlands are characterized by the presence of tropical moist and wet forests (Holdridge et al., 1966). Seasonality is low, with heavy annual rainfall patterns (average 2370–3710 mm) and warm temperatures (average 25–27°C) throughout the entire year and short dry periods (under a month, February and October) (Porras-Peñaranda et al., 2004; Solano and Villalobos, 2012).
We sampled bats in three foraging habitats with different degrees of alterations: organic banana plantations, conventional banana monocultures, and natural forests serving as controls, with two sites per habitat (Supplementary Figure 1). All sampling sites ranged between 50 and 350 ha and had a distance of at least 10 km between each other. The two banana plantation habitats differed in management intensity, agrochemical use, homogeneity level, and size. Organic plantations were mixed with other crops and included native plant species in edges or as corridors, relied on biological pest control methods (i.e., used no pesticides), and had an average size of 50–120 ha; while conventional banana monocultures comprised more than 200 ha of only banana plants and pesticides were applied regularly (27 active ingredients, 47.29 kg/ia/ha/year; Bravo et al., 2013).
We sampled bats during 22 months between 2015 and 2018, covering all 12 months of the year to consider seasonal changes. We captured bats using three to eight ground-level mist nets of 6–12 m (Ecotone, Poland) that were set up 1 h after sunset to guarantee bats had fed before capture and the successful acquisition of fecal matter. We collected basic data from each individual (weight, forearm, sex, age, and reproductive state), and marked them using wing punches to avoid resampling. We captured and handled bats following the guidelines of the American Society of Mammalogists (Sikes and Gannon, 2011), with the compulsory Costa Rican permits (#SINAC-SE-CUS-PI-R-095-2016 and #R-003-2017-OT-CONAGEBIO). After capture, we placed bats in sterile cloth bags until they defecated or for a maximum of 1 h. Fecal material was stored in sterile vials with 96% ethanol in a 4°C cooler until transport to a −20°C freezer, where they were stored until processing. Each bat was sampled only once.
Bacterial DNA Extraction and 16S rRNA Gene Amplicon Sequencing
We followed the protocol detailed by Wasimuddin et al. (2018), to homogenize and extract bacterial DNA using a Nucleo7 Spin 96 Soil Kit (Macherey-Nagel, Germany). During extraction, we included seven extraction controls with only the extraction reagents. We amplified the hypervariable V4 region of the 16S rRNA gene (291 bp) using the primer pair 515F (5′-GTGCCAGCMGCCGCGGTAA-3′) and 806R (5′-GGACTACHVGGGTWTCTAAT-3′). We followed a standardized Fluidigm protocol (Access Array System for Illumina Sequencing Systems, ©Fluidigm Corporation), in which individual PCR reactions were tagged with a 10-base pair identifier. The PCR (15 μl of volume) was performed as described in detail by Menke et al. (2014). Barcoded samples were purified (NucleoMag bead-based size selection, Macherey-Nagel, Germany) and quantified (DropSense, Trinean, United States) prior to pooling a sample library that was paired-end sequenced in a single run on an Illumina MiSeq platform (2 × 250 bp). We sequenced a total of 269 samples (one per bat individual), seven extraction blanks, and 10 PCR blanks.
Bioinformatics
Initial sequencing read processing was done using QIIME2 (version 2020.6) (Bolyen et al., 2019). We removed low quality sequences and trimmed primers. We truncated our forward and reverse reads to 190 bp due to the decrease of the average quality scores at the end of our sequences. Clustering into amplicon sequence variants (ASVs) and denoising was performed with the DADA2 algorithm (Callahan et al., 2016). We generated and constructed a phylogenetic tree with MAFFT (Katoh and Standley, 2013) and FastTree (Price et al., 2009), and rooted it using an archaeal sequence. Taxonomy was assigned to the ASVs using a pre-trained classifier (McDonald et al., 2012; Bokulich et al., 2018; Robeson et al., 2020) for the Greengenes database version 13_8 as a reference (Second Genome, Inc.). We removed all sequences classified as chloroplast, mitochondria, archaea, Eukaryota, or unclassified at the phylum level. After filtering, 9,763,418 reads from 11,951 ASVs remained.
Data Analysis
We retained only samples that included over 5000 reads for the analysis, for a final sample size of 196 samples (conventional monocultures: 78, organic plantations: 70, and natural forests: 48). We carried out all analyses in R using the packages phyloseq 1.34.0 (McMurdie and Holmes, 2013), vegan 2.5-6 (Oksanen et al., 2019), and NetCoMi 1.0.2.9000 (Peschel et al., 2021).
Effects of Foraging Habitat on Microbiota Alpha and Beta Diversity
To estimate alpha diversity within individuals, we rarefied counts to 5,000 reads based on rarefaction curve examination (Supplementary Figure 2). We calculated three alpha diversity indices: number of observed ASVs, Shannon index (weights ASVs by their relative evenness across a community) (Shannon, 1948), and Faith’s Phylogenetic Diversity index (accounts for the ASVs’ phylogenetic diversity) (Faith, 1992). To test the effect of the foraging habitat type whilst controlling for other confounding variables, we built three generalized linear models (GLMMs) with a gamma distribution for each alpha diversity index, using the glmm 1.4.2 package (Knudson, 2017). Initial models included foraging habitat, sex, age, reproductive state, sampling year, and sequencing depth. Model comparison and selection based on AIC was performed using the dredge function from the MuMIn 1.43.17 package (Bartón, 2020).
We assessed beta diversity between individuals through a MDS ordination using rarefied counts and applying three distance measures: unweighted UniFrac (accounts for phylogenetic distance, but only considers presence-absence) (Lozupone et al., 2011), weighted UniFrac (accounts for phylogenetic distance and abundance and is more appropriate to show core microbiota) (Lozupone et al., 2011; Risely et al., 2020), and Bray-Curtis index (accounts for abundance, but not for phylogenetic distance) (Real and Vargas, 1996). The trend observed in the unweighted Unifrac ordination was similar to that of weighted Unifrac, therefore, we did not consider unweighted Unifrac further in our results. We tested for the effect of foraging habitat type, alongside other potential confounding variables (sex, age, reproductive state, sampling year, and sequencing depth) with permutational multivariate analyses of variance (PERMANOVA, adonis function); and for multivariate homogeneity of habitat dispersion (betadisper function), both from the vegan 2.5-6 package (Oksanen et al., 2019).
Differential Abundance Analysis of Bacterial Taxa Using TOPICS
We explored differential abundance using TOPICS, a family of analyses that models differential abundance for co-occurring clusters (“topics”) in sparse and compositional data (Woloszynek et al., 2019). First, ASVs were agglomerated using phyloseq’s tip_glom function (h = 0.2) in order to reduce dimensionality of the data, and only agglomerated taxa with over 20% prevalence were retained. We did not used rarefaction because the TOPICS function normalizes data internally. TOPICS analysis was performed using the themetagenomics 1.0.2 package (Woloszynek et al., 2019). Our model looked for structure according to the foraging habitat, with samples from natural forests used as a reference, and corrected according to sequencing depth.
Co-occurrence Networks
To construct co-occurrence networks, we applied a method similar to that of Calatayud et al. (2020) which compared bacterial taxa correlations with a null model distribution. Consistent with the TOPICS analysis, ASVs from the rarefied dataset were again agglomerated using phyloseq’s tip_glom function (h = 0.2), and only bacterial taxa with over 20% prevalence were retained to ensure statistical robustness (n = 47 taxa). We built co-occurrence networks for each foraging habitat type using the NetCoMi 1.0.2.9000 package (Peschel et al., 2021) and assessed significance of each association by comparing the strength of the association (Spearman’s r) to a null distribution based on 999 random permutations. Null distributions were generated by constructing 999 random adjacency matrices with the same row and column totals as the real data, using vegan’s nullmodel function with the “quasiswap_count” method to retain zero inflation. We calculated an association matrix based on Spearman’s correlation for each of the 999 random adjacency matrices and generated a null distribution of Spearman’s correlation coefficients for each potential edge in a fully connected network. Edges were considered significant if the original Spearman’s correlation fell outside three standard deviations of the null distribution mean. Edge weight represented the effect size of a one-tailed t-test of Spearman’s r against the null distribution. This method is superior to those based solely on correlations, because it can identify and exclude correlations that result from structure alone (i.e., common taxa are statistically more likely to have more significant associations than rarer taxa) than those generated by chance. As such, it also more sensitive to weak but real associations, which would otherwise be discarded if applying just correlations alone to identify associations. We tested the robustness of our results by repeating these analyses when data was normalized by Centered Log Ratio (CLR), and, because sample sizes were unequal across habitat types, we also randomly sub-sampled 40 samples per group and repeated the analysis. Results from both sensitivity analyses were almost identical to those presented.
Relationship Between Foraging Habitat Type, Body Condition, and the Microbiota
To determine if an individual bat’s body size and mass are associated with foraging habitat and changes in microbiota alpha diversity and beta dissimilarity, we created two Gaussian-distributed GLMMs predicting forearm size and scale body mass (SBM = mass/forearm), respectively, using the package lme4 1.1-25 (Bates et al., 2019). Initial models included foraging habitat, sex, age, reproductive state, sampling year, sequencing depth, alpha diversity (Shannon index), and beta diversity (first axis for Weighted Unifrac and Bray-Curtis indices). Model comparison and selection based on AIC was performed using the dredge function from the MuMIn 1.43.17 package (Bartón, 2020).
We also explored the relationship between bat body condition and common gut microbiota families using TOPICS analysis. For this, we first created a model predicting mass against foraging habitat, reproductive state, and size (forearm), and calculated a residual body mass (RBM) index. Using RBM instead of SBM allowed us to consider the variability from all variables that predicted body mass when comparing it to the gut microbiota composition. We divided the RBM values in three categories (terciles): low [−3.13, −0.423], normal [−0.423, 0.413], and high [0.413, 5.71]. The TOPICS analyses looked for structure according to RBM, and we used the normal category as a reference.
Results
Bacterial Composition
The top ten most abundant bacterial families made up over 65% of the relative abundance of all sampled individuals (Figure 1A). Enterobacteriaceae (phylum Proteobacteria) was the dominant family across combined samples and was especially abundant in bats from conventional monocultures, alongside Burkholderiaceae (phylum Proteobacteria). Samples from organic plantations and natural forests showed higher proportions of Mycoplasmataceae, Moraxellaceae, Streptococcaceae, and Pseudonocardiaceae (phylum Actinobacteria; Figure 1A) and were less dominated by Enterobacteriaceae (Supplementary Table 1).
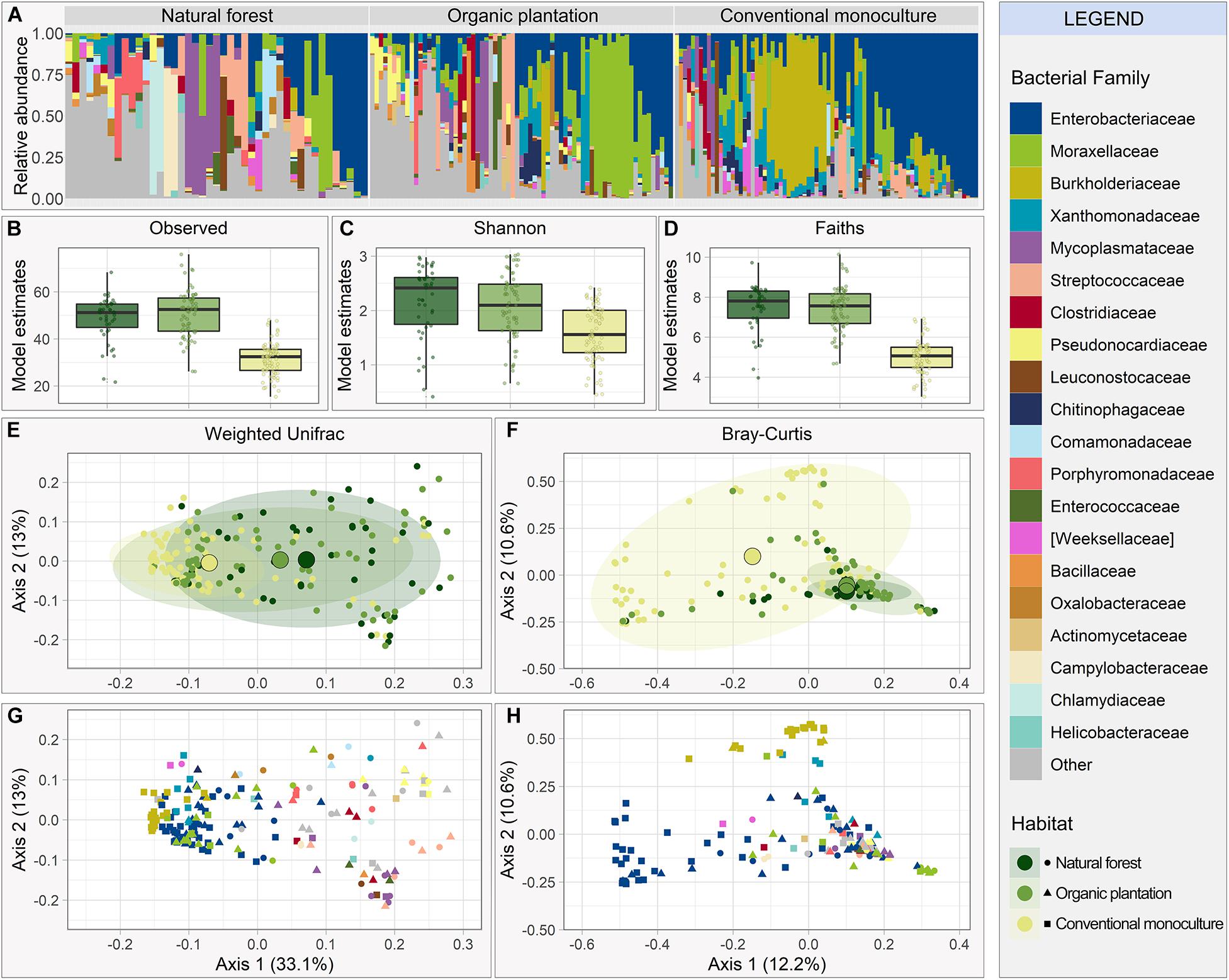
Figure 1. Gut microbiome composition and diversity of Glossophaga soricina individuals using different foraging habitats (natural forests, organic banana plantations or conventional banana monocultures). (A) Relative abundance of bacterial families. Bars show the top 20 bacterial families found in the overall microbiome; remaining families are lumped into “Other families.” Estimated alpha diversity accounting for sequencing depth, based on (B) number of observed ASVs, (C) Shannon index, and (D) Faith’s Phylogenetic Diversity. Multi-Dimensional Scaling to visualize beta diversity using (E) Weighted Unifrac index and (F) Bray-Curtis dissimilarity index colored by foraging habitat, and (G) Weighted Unifrac index and (H) Bray-Curtis dissimilarity index colored by dominant bacterial family (the most abundant bacterial family in each sample).
Relationship Between Foraging Habitat and Alpha and Beta Diversity
All alpha diversity indices were lower for bats foraging in conventional monocultures compared to other habitats (Figures 1B–D). Estimated mean observed ASV richness was 50.94 (95% CI [39.72, 65.33]), 53.12 (95% CI [32.80, 86.02]), and 32.76 (95% CI [20.32, 52.82]) for natural forests, organic plantations, and conventional monocultures, respectively, with significant differences in observed richness between conventional monocultures and the two other foraging habitats (Conventional-Organic: t = 4.50, p < 0.001; Conventional-Forest: t = 3.09, p < 0.001; Supplementary Table 2). Sequencing depth, sampling year, and reproductive state also explained a small amount of variation in some or all alpha diversity indices (Supplementary Table 2).
We next examined the relationship between foraging habitat and microbial beta diversity. Gut microbiota from individuals foraging in natural forests and organic plantations were more similar to each other in overall composition than those from conventional monocultures, when represented by the first two axes of an MDS ordination using both Weighted Unifrac (Figure 1E) and Bray-Curtis (Figure 1F). The foraging habitat explained approximately 7% of variation in beta diversity across all axes (Supplementary Table 2), yet the major differences between samples from different habitat types lay in their dispersion. Gut microbiota from bats inhabiting conventional monocultures had highly similar phylogenetic composition to each other (average distance to centroid = 0.16, F = 24.881, p < 0.0001), yet were highly dispersed when measured by Bray-Curtis distance (average distance to centroid = 0.58, F = 35.564, df = 2, p < 0.0001), which does not account for phylogenetic relationships between taxa. Thus, samples from conventional monocultures are heavily dominated by a small number of families (Figures 1G,H and Supplementary Table 1), but these families consist of a large number of disparate ASVs, potentially representing different bacterial species. The separate clusters in the bats from conventional monocultures represented by Bray Curtis (Figure 1F) represented microbiota dominated by either Enterobacteriaceae or Burkholderiaceae (Figure 1G), and because both belong to Proteobacteria, they cluster together when phylogeny is considered (Figure 1G), but separately when just ASV abundance is considered (Figure 1H). Sequencing depth and year also explained differences in beta diversity (Supplementary Table 2).
Differential Abundance Analysis Using TOPICS
To understand which bacterial taxa may be driving differences in beta diversity between foraging habitats, we performed a differential abundance analysis using TOPICS analysis, which identifies groups of co-occurring bacteria associated with different treatment groups (Woloszynek et al., 2019). Co-occurring taxa were grouped to form 15 topics, of which seven were significantly associated with foraging habitat. The largest effect sizes represented the inflation of Enterobacteriaceae, Burkholderiaceae, Xanthomonadaceae, and Chitinophagaceae in conventional monocultures (Figure 2). Whilst the latter three families tended to co-occur together, the abundance of Enterobacteriaceae (the most common family across habitats) was independent of other taxa and formed its own topic.
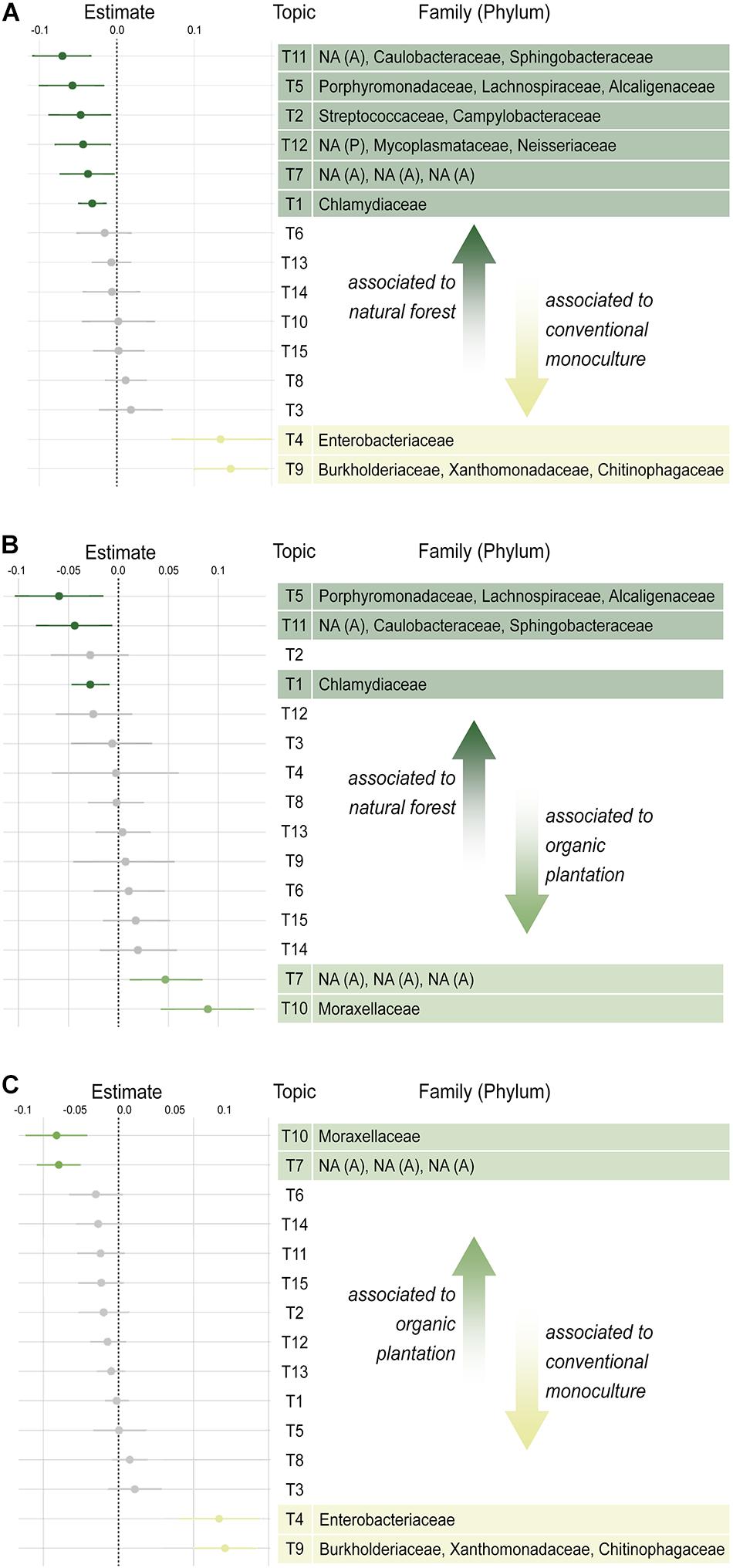
Figure 2. Pairwise differential abundance of co-occurring groups of bacterial taxa (TOPICS); (A) natural forests vs. conventional banana monocultures; (B) natural forests vs. organic banana plantations; (C) organic banana plantations vs. conventional banana monocultures. Unidentified taxa are marked as NA (Phylum), in which A, Actinobacteria and P, Proteobacteria.
The microbiota of bats foraging in natural forests, however, were characterized by a far more diverse suite of rare taxa, including Sphingobacteriaceae, Caulobacteriaceae, Actinomycetales (topic 11); Alcaligenaceae, Lachnospiraeceae, Porphyromonadaceae (topic 5); and Chlamydiaceae (topic 1) all of which tended to be found at low abundances. Organic plantations were characterized by inflation of Moraxellaceae. Overall, differences between natural forests and organic plantation microbiota were much smaller in their effect sizes than those between conventional monocultures and either natural forests or organic plantations, supporting the beta diversity finding that the gut microbiota of bats from natural forest and organic plantation cluster apart from those of bats foraging in conventional monocultures.
Relationship Between Foraging Habitat and Co-occurrence Network Structure
Co-occurrence network analyses were used to assess the effect of foraging habitat on the gut microbiota ecological interactions. According to the number of edges and the average degree, network complexity increased with management intensity (Figure 3), and networks from conventional monocultures also have more negative interactions. The most highly connected taxa were different between each network, with no single node being important across networks, suggesting that whilst microbiota from natural forests and organic plantations are similar in phylogenetic composition, their taxa interactions differ. For the natural forest network, not one taxon was disproportionately connected, with Ruminococcaceae (Firmicutes), Porphyromonadaceae (Bacteroidetes), and Rhizobiales (Proteobacteria) taxa representing the best-connected nodes. In the organic plantation, Actinomycetales (Actinobacteria), Solirubrobacterales (Actinobacteria), and Lachnospiraceae (Firmicutes) were disproportionately important. For the conventional monoculture network, negative interactions were largely mediated by Chitinophagaceae and Streptococcaceae (both Bacteroidetes), and positive interactions by Lactobacilliales, Leuconostocaceae, Ruminococcaceae, and Lachnospiraceae (all Firmicutes). Other than Chitinophagaceae, taxa that were highly abundant in conventional monocultures, such as Enterobacteriaceae, Burkholdiceae, and Xanthomonadaceae, did not appear to be important in networks.
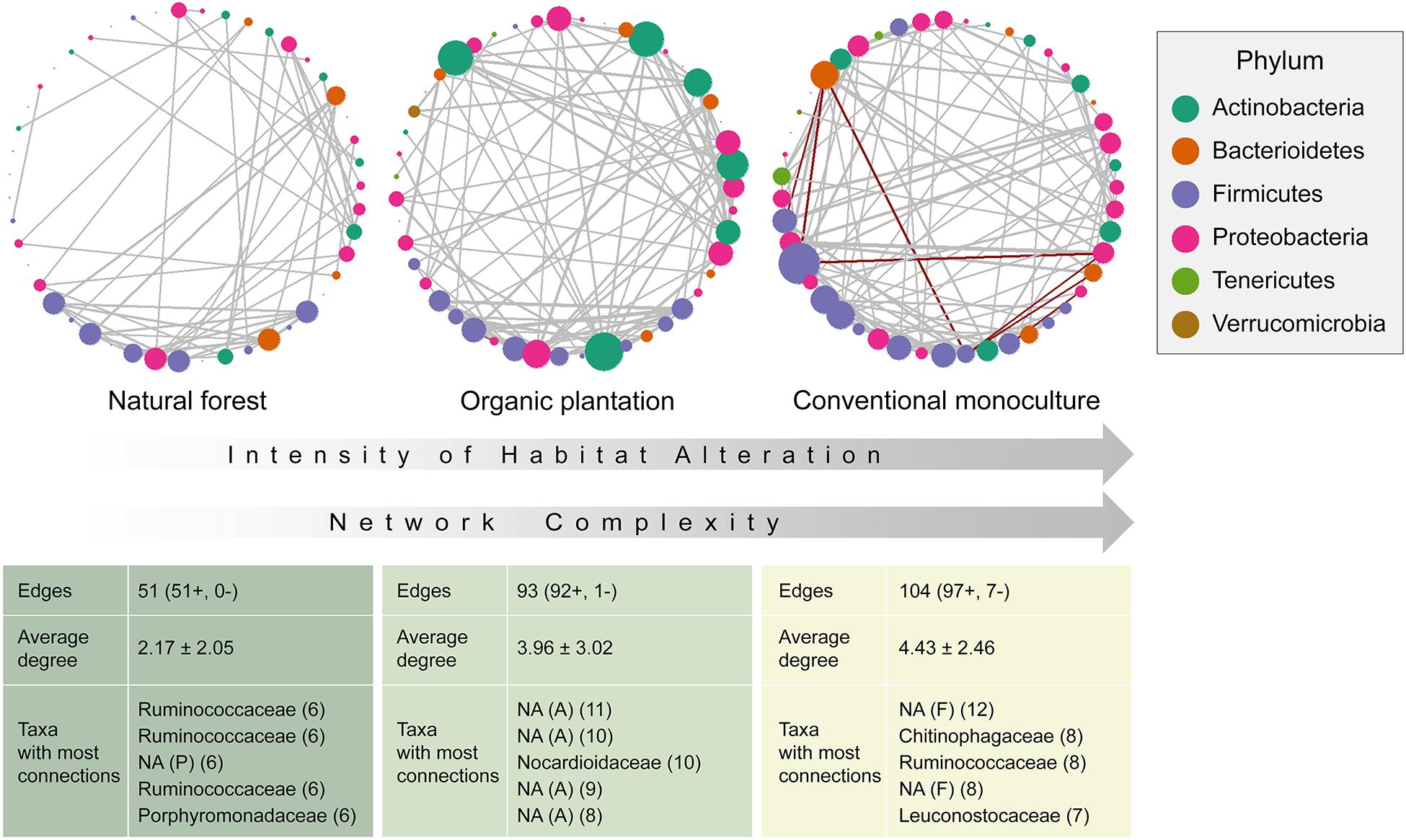
Figure 3. Networks and network complexity indicators for the microbiome of Glossophaga soricina individuals according to their foraging habitat. Positive interactions colored gray, and negative colored red. Unidentified taxa are marked as NA.
Relations Between Foraging Habitat, Body Condition, and the Microbiota
Bats foraging in banana plantations had higher SBM and were larger than those foraging in natural forests, with organic plantations supporting particularly large individuals (Figures 4A,B and Supplementary Table 3). To examine associations between body mass and the microbiota, we accounted for foraging habitat by calculating RBM in a model predicting mass against habitat, reproductive state, and size (forearm), and then examined whether any taxa were associated with high or low RBM using TOPICS analysis. From the 15 topics created, only three were significantly related to the bats’ RBM (Figure 4C). We found no taxa associated to bats with low RBM (Supplementary Figure 3), whilst Chlamydiaceae, Xanthomonadaceae, Chitinophagaceae, and Weeksellaceae were associated with normal RBM. Bats with high RBM were associated with Mycoplasmataceae, Lactobacillales, and Campylobacterales. Interestingly, these taxa were more abundant in bats foraging in natural forest than in either banana plantation, even though the first tended to be smaller and lighter.
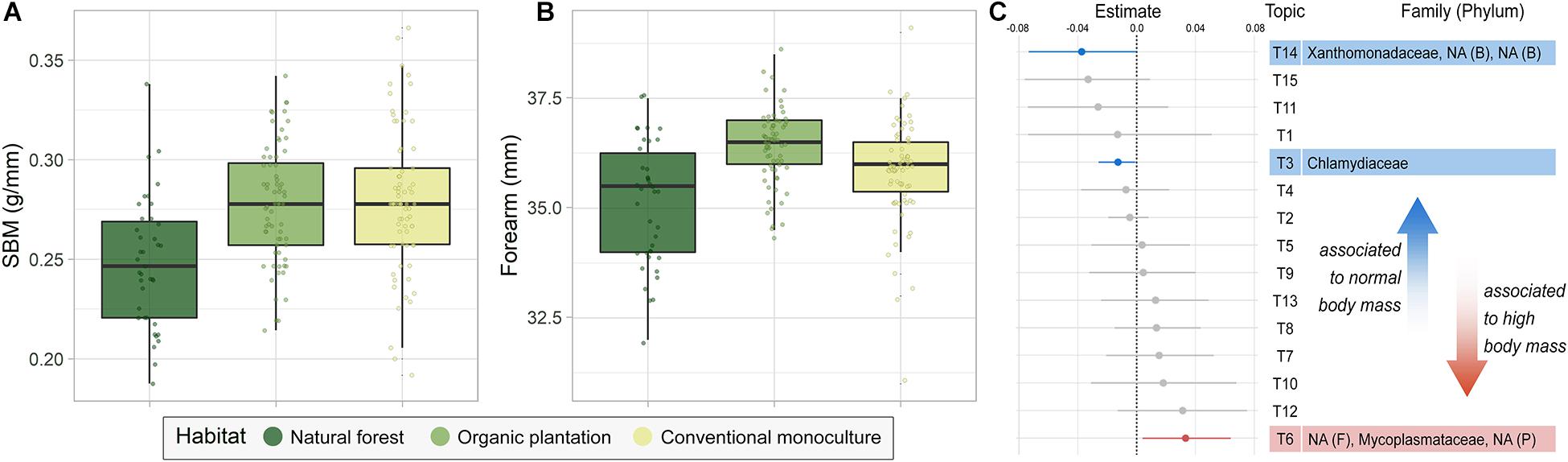
Figure 4. (A,B) Body condition of bats using different foraging habitats. (A) Scaled body mass (SBM) and (B) forearm length. (C) Pairwise microbiome comparison between bats with normal and high residual body mass (RBM), assessed using groups of co-occurring taxa (TOPICS). Unidentified taxa are marked as NA.
Discussion
Our study explores differences in gut microbiota diversity, composition, and network complexity between three foraging habitats of the nectar-feeding bat G. soricina differing in the management intensity and examines their effects on gut microbiota composition and body condition. We found that management intensity had strong effects on microbiota diversity. Gut microbiota from bats foraging in conventional monocultures were highly homogenous both across and within samples and differed markedly from bats foraging in natural forest or organic plantation habitats, including in co-occurrence networks, potentially indicating microbial dysbiosis. Interestingly, bats foraging in both types of plantations were heavier and larger than those from natural forests, reflecting the reliable food supply provided by both, therefore bats from monocultures did not demonstrate lower condition indices, as we predicted. Nevertheless, less diverse gut microbiota in bats foraging in conventional monocultures may suggest that these habitats potentially have negative physiological consequences for the animals (e.g., gut inflammation and metabolic disease), and may act as ecological traps. Organic plantations, on the other hand, supported heavier bats that retained more diverse microbiota that were closer to their natural forest-foraging counterparts, indicating that organic practices may maintain high diversity of commensal microbiota.
Following typical community responses in human-modified landscapes (Teyssier et al., 2020) and agricultural habitats (Stein-Bachinger et al., 2020), the gut microbiota of bats foraging in conventional monocultures had lower alpha diversity and was mostly dominated by only few taxa, mainly Enterobacteriaceae and Burkholderiaceae (57.3% vs. 21.5% in organic and 19.06% in forests), we suggest this is caused by dietary changes and pesticide use. Glossophaga soricina showed a less diverse diet in both types of banana plantations in comparison to natural forests (Alpízar et al., 2020), and such a simplified diet may result in a reduced microbiome diversity (Amato et al., 2013). However, no significant differences were found in the diet diversity between both banana plantation types (Alpízar et al., 2020), indicating that altered gut microbiotas in bats from conventional monocultures might be caused by other sources. Since our focal species typically complements its diet with insects (Clare et al., 2014), an important protein source, lower insect availability in conventional monocultures (Matlock and de La Cruz, 2002; Markó et al., 2017) certainly leads to strong dietary changes in G. soricina, potentially modifying their gut microbiota composition. In addition, pesticides, especially glyphosate, inhibit several of the bacterial microbiome taxa distinctive for bats from natural forests (Campylobacteraceae, Streptococcaceae, and Neisseriaceae) (Shehata et al., 2013; Mao et al., 2018; Blot et al., 2019). It reduces microbiome diversity and taxonomic richness of Bacteroidetes and Proteobacteria phyla (Yang et al., 2019), causes inflamed gut microenvironments, and induces blooms of Enterobacteriaceae in mice (Stecher et al., 2012). Generally, glyphosate decreases commensal bacterial species, but not pathogenic ones (Shehata et al., 2013), supporting our hypothesis that microbial communities from monocultures may be dysbiotic. However, further studies are required to assess health markers such as gut inflammation, as well as any effects on reproduction and survival, to prove any negative consequences.
We found that microbial co-occurrence networks became more complex with increased management intensity, which contrasts with previous work showing that smaller forest patches (Speer et al., 2020) and increased land use (Gámez-Virués et al., 2015) lead to fewer biotic interactions. Rare taxa were promoting important positive interactions, which is in line with our understanding of ecological networks (Calatayud et al., 2020). Enterobactericeae and Burkholdiceae, the two major families found in bats foraging in conventional plantations, were surprisingly not important in networks, which suggests they are not out competing other taxa but rather inhabiting their own niche. The simplified networks in natural forest and organic plantations may be explained with how extremely simplified the microbiota of G. soricina were in these habitats, with each bat harboring a distinct community, thereby limiting interactions between taxa. The influence of pesticides, combined with the simplified diet of G. soricina individuals foraging in conventional monocultures may promote an expansion of co-occurring generalist bacteria, explaining the increase in positive interactions in gut microbiota networks from these highly modified habitats.
Bats from both types of banana plantations were heavier and larger than those from natural forest, suggesting that these crops provide a reliable food source (quantity-wise) for G. soricina. However, our results also unveiled an interesting interaction between foraging habitat, body condition, and the gut microbiota: the bacterial taxa associated with high RBM were more common in bats foraging in natural forests. This suggests that the gut microbiota plays an important role in promoting fat deposition in these bats when food resources are patchy and more unreliable but are of less functional importance to bats in plantations with a constant access to food. The nectar produced in banana plantations provides enough food for five G. soricina individuals per hectare throughout the year (Alpízar et al., 2020), and colonies roost usually inside the plantations (Alpízar, unpublished data). High nectar availability in banana plantations translates to shorter foraging flights and less fat-storage needs, as well as the means to sustain heavier builds in bats. In comparison, G. soricina foraging in natural forests must deal with changes in food availability, seasonality, and patchiness (Tschapka, 2004), which involves longer food-locating flights and explains why fat deposits might be more important. Since studies about bat fitness and survival rates in agricultural landscapes are non-existent (Meyer et al., 2016), we are not able to comment on whether a reliable homogeneous food supply with high pesticide inputs translates into positive fitness for G. soricina foraging in banana plantations or if indeed heavier bats are healthier bats, but future research that disentangles these complex interactions is vital to understand the mechanisms by which industrial agriculture may affect persisting wildlife populations.
The gut microbiota is likely to be influenced by several factors that we were unable to control for. For example, many animals demonstrate seasonal variations in their gut microbiotas as a response to diet switching between dry and rainy seasons, and due to our sampling design and sample size, we were unable to control for any seasonal shifts in composition. In bats, changes to flower and insect availability across the year may influence the gut microbiota. Even if G. soricina has been observed to shift diets between rainy and dry seasons (Willig et al., 1993), the Costa Rican Caribbean lowlands have reduced seasonality compared to temperate regions and even to more seasonal areas inside the tropics. Therefore, we believe that seasonal effects in this species would be small. Another factor that may affect our results are farming practices such as harvesting and levels of pesticide use, for which we did not have information. Variation in plantation harvesting schedules across farms and over the year may generate effects on bat foraging ecology that we cannot detect; for example, this may explain the large variation in individual microbiomes between bats (Figure 1F). In particular, measuring pesticide use and flower availability at sampling sites would help disentangle the mechanisms (diet vs. pesticide use) leading to simplified gut microbiotas in banana plantations.
Our study provides a first insight into the effects of organic and conventional agriculture on animal body condition and gut microbiota. Our results suggest that conventional banana plantations provide a reliable food supply for nectar-feeding bats, yet monocultures simplify the gut microbiota, which may have negative physiological consequences for the animals. In contrast, organic banana plantation management also provides reliable food sources while maintaining diversity within the gut microbiota of nectar-feeding bats. Our results suggest that organic practices represent a sustainable agricultural land use that maintain not only higher levels of biodiversity than monocultures but also microbial health for the bats.
Data Availability Statement
The datasets presented in this study can be found in the OSF online repository under doi: 10.17605/OSF.IO/CMN5K.
Ethics Statement
The animal study was reviewed and approved by MINAE, Costa Rica.
Author Contributions
PA and MT conceived the objectives and study design with input from SS. PA coordinated the fieldwork, collected all samples, and carried out laboratory analyses in SS’ lab. PA with the supervision of AR, performed the bioinformatic and statistical analyses. PA took the lead in writing the manuscript in consultation with AR, and edited by AR, MT, and SS. All authors agreed on the final version of the manuscript, and different countries collaborated during this study, including a scientist from the country where the study was carried out.
Funding
PA was supported by grants from the Rufford Foundation (Grant Nos. 16703-1 and 20385-2), Bat Conservation International, the German Academic Exchange Program (DAAD), and the Science, Technology, and Telecommunications Ministry of Costa Rica (MICITT).
Conflict of Interest
The authors declare that the research was conducted in the absence of any commercial or financial relationships that could be construed as a potential conflict of interest.
Publisher’s Note
All claims expressed in this article are solely those of the authors and do not necessarily represent those of their affiliated organizations, or those of the publisher, the editors and the reviewers. Any product that may be evaluated in this article, or claim that may be made by its manufacturer, is not guaranteed or endorsed by the publisher.
Acknowledgments
We thank K. Wilhelm, R. Jiménez, Wasimuddin, L.R. Víquez-Rodríguez, and U. Stehle for their support with laboratory work and bioinformatics. Two referees provided very helpful comments. A special thanks to J. Schneider for his constant encouragement during the entire project, mainly for his help during fieldwork and with image composition. We thank the staff at Fincas Rebusca, Super Amigos, and Guarumo Platanera Río Sixaola, Tirimbina Biological Reserve, Dave and Dave’s Costa Rica Nature Park, Lapa Verde Refuge, and La Ceiba Natural Reserve. We acknowledge the work from our field assistants: A. Segura, G. Arias, R. Sánchez, F. Bonnet, A. Duarte, F. Mora, J. Sandoval, L. Ehrmanntraut, and B. Honner.
Supplementary Material
The Supplementary Material for this article can be found online at: https://www.frontiersin.org/articles/10.3389/fevo.2021.746783/full#supplementary-material
References
Alpízar, P., Schneider, J., and Tschapka, M. (2020). Bats and bananas: Simplified diet of the nectar-feeding bat Glossophaga soricina (Phyllostomidae: Glossophaginae) foraging in Costa Rican banana plantations. Glob. Ecol. Conserv. 24:e01254. doi: 10.1016/j.gecco.2020.e01254
Alvarez, J., Willig, M. R., Jones, J. K., and Webster, W. D. (1978). Glossophaga soricina. Mamm. Species 379, 1–7.
Amato, K. R., Yeoman, C. J., Kent, A., Righini, N., Carbonero, F., Estrada, A., et al. (2013). Habitat degradation impacts black howler monkey (Alouatta pigra) gastrointestinal microbiomes. ISME J. 7, 1344–1353. doi: 10.1038/ismej.2013.16
Aziz, S. A., Olival, K. J., Bumrugnsri, S., Richards, G. C., and Racey, P. A. (2015). “The conflict between pteropodid bats and fruit growers: species, legislation, and mitigation,” in Bats in the Anthropocene: Conservation of bats in a changing world, eds C. C. Voigt and T. Kingston (New York, NY: Springer International Publishing), 559–575.
Barquez, R., Perez, S., Miller, B., and Diaz, M. (2015). Glossophaga soricina. United Kingdom: The IUCN Red List of Threatened Species.
Bates, D., Machler, M., Bolker, B., Walker, S., Bojesen Christensen, R. H., Singmann, H., et al. (2019). Package ‘lme4’. R package version 1.1.-21.
Blot, N., Veillat, L., Rouzé, R., and Delatte, H. (2019). Glyphosate, but not its metabolite AMPA, alters the honeybee gut microbiota. PLoS One 14:e0215466. doi: 10.1371/journal.pone.0215466
Bokulich, N. A., Kaehler, B. D., Rideout, J. R., Dillon, M., Bolyen, E., Knight, R., et al. (2018). Optimizing taxonomic classification of marker-gene amplicon sequences with QIIME 2’s q2-feature-classifier plugin. Microbiome 6, 90. doi: 10.1186/s40168-018-0470-z
Bolyen, E., Rideout, J. R., Dillon, M. R., Bokulich, N. A., Abnet, C. C., Al-Ghalith, G. A., et al. (2019). Reproducible, interactive, scalable and extensible microbiome data science using QIIME 2. Nat. Biotechnol. 37, 848–857. doi: 10.1038/s41587-019-0209-9
Bravo, V., de la Cruz, E., Herrera, G., and Ramírez, F. (2013). Uso de plaguicidas en cultivos agrícolas como herramienta para el monitoreo de peligros en salud. Uniciencia 27, 351–376.
Brestoff, J. R., and Artis, D. (2013). Commensal bacteria at the interface of host metabolism and the immune system. Nat. Immunol. 14, 676–684. doi: 10.1038/ni.2640
Calatayud, J., Andivia, E., Escudero, A., Melián, C. J., Bernardo-Madrid, R., Stoffel, M., et al. (2020). Positive associations among rare species and their persistence in ecological assemblages. Nat. Ecol. Evol. 4, 40–45. doi: 10.1038/s41559-019-1053-5
Callahan, B. J., McMurdie, P. J., Rosen, M. J., Han, A. W., Johson, A. J. A., and Holmes, S. P. (2016). DADA2: High resolution sample inference from Illumina amplicon data. Nat. Methods 13, 581–583. doi: 10.1038/nmeth.3869
Clare, E. L., Drapeau, H. R., Holderied, V. A., Adams, M. W., and Nagel, A. M. (2014). Trophic niche flexibility in Glossophaga soricina: how a nectar seeker sneaks an insect snack. Funt. Ecol. 28:632e641. doi: 10.1111/1365-2435.12192
Dudley, N., and Alexander, S. (2017). Agriculture and biodiversity: a review. Biodiversity 18, 45–49. doi: 10.1080/14888386.2017.1351892
Eyhorn, F., Muller, A., Reganold, J. P., Frison, E., Herren, H. R., Luttikholt, L., et al. (2019). Sustainability in global agriculture driven by organic farming. Nat. Sustainab. 2, 253–255. doi: 10.1038/s41893-019-0266-6
Fackelmann, F., Gillingham, M. A. F., Schmid, J., Heni, A. C., Wilhelm, K., and Sommer, S. (2021). Human encroachment into wildlife gut microbiomes. Comm. Biol. 4:800. doi: 10.1038/s42003-021-02315-7
Faith, D. P. (1992). Conservation evaluation and phylogenetic diversity. Biolog. Conserv. 61, 1–10. doi: 10.1016/0006-3207(92)91201-3
Gámez-Virués, S., Gámez-Virués, S., Perović, D. J., Gossner, M. M., Börschig, C., Blüthgen, N., et al. (2015). Landscape simplification filters species traits and drives biotic homogenization. Nat. Comm. 6:8568. doi: 10.1038/ncomms9568
Gillingham, M. A. F., Borghesi, F., Montero, B. K., Migani, F., Béchet, A., Rendón-Martos, M., et al. (2020). Bioaccumulation of trace elements affects chick body condition and gut microbiome in greater flamingos. Sci. Total Env. 2020:143250. doi: 10.1016/j.scitotenv.2020.143250
Henriques, W., Jeffers, R. D., Lacher, T. E., and Kendall, R. J. (1997). Agrochemical use on banana plantations in Latin America: Perspectives on ecological risk. Env. Toxicol. Chem. 16, 91–99. doi: 10.1002/etc.5620160110
Ingala, M. R., Becker, D. J., Bak Holm, J., Kristiansen, K., and Simmons, N. B. (2019). Habitat fragmentation is associated with dietary shifts and microbiota variability in common vampire bats. Ecol. Evol. 9, 6508–6523. doi: 10.1002/ece3.5228
Katoh, K., and Standley, D. M. (2013). MAFFT Multiple Sequence Alignment Software Version 7: Improvements in Performance and Usability Article Fast Track. Mole. Biol. Evol. 30, 772–780. doi: 10.1093/molbev/mst010
Kittle, R. P., McDermid, K. J., Muehlstein, L., and Balazs, G. H. (2018). Effects of glyphosate herbicide on the gastrointestinal microflora of Hawaiian green turtles (Chelonia mydas) Linnaeus. Marine Pollution Bulletin 127, 170–174. doi: 10.1016/j.marpolbul.2017.11.030
Lozupone, C., Lladser, M. E., Knights, D., Stombaugh, J., and Knight, R. (2011). UniFrac: an effective distance metric for microbial community comparison. ISME J. 5, 169–172. doi: 10.1038/ismej.2010.133
Mao, Q., Manservisi, F., Panzacchi, S., Mandrioli, D., Menghetti, I., Vornoli, A., et al. (2018). The Ramazzini Institute 13-week pilot study on glyphosate and Roundup administered at human-equivalent dose to Sprague Dawley rats: Effects on the microbiome. Env. Health 17, 1–12. doi: 10.1186/s12940-018-0394-x
Markó, V., Elek, Z., Kovács-Hostyánszki, A., Kõrösi, Á, Somay, L., Földesi, R., et al. (2017). Landscapes, orchards, pesticides–Abundance of beetles (Coleoptera) in apple orchards along pesticide toxicity and landscape complexity gradients. Agricult. Ecosyst. Env. 247, 246–254. doi: 10.1016/j.agee.2017.06.038
Matlock, R. B., and de La Cruz, R. (2002). An inventory of parasitic Hymenoptera in banana plantations under two pesticide regimes. Agric. Ecosyst. Environ. 93, 147e164. doi: 10.1016/S0167-8809(02)00002-6
McDonald, D., Price, M. N., Goodrich, J., Nawrocki, E. P., DeSantis, T. Z., Probst, A., et al. (2012). An improved Greengenes taxonomy with explicit ranks for ecological and evolutionary analyses of bacteria and archaea. Evol. Genet. 6, 610–618. doi: 10.1038/ismej.2011.139
McMurdie, P. J., and Holmes, S. (2013). phyloseq: An R Package for Reproducible Interactive Analysis and Graphics of Microbiome Census Data. PLoS One 8:e61217. doi: 10.1371/journal.pone.0061217
Menke, S., Wasimuddin, Meier, M., Melzheimer, J., Mfune, J. K. E., Heinrich, S., et al. (2014). Oligotyping reveals differenes between gut microbiomes of free-ranging sympatric Namibian carnivores (Acinonyx jubatus, Canis mesomelas) on a bacterial species-like level. Frontiers in Microbiology 5:1–12. doi: 10.3389/fmicb.2014.00526
Meyer, C. F. J., Struebig, M. J., and Willig, M. R. (2016). “Responses of tropical bats to habitat fragmentation, logging, and deforestation,” in Bats in the Anthropocene: Conservation of bats in a changing world, eds C. C. Voigt and T. Kingston (New York, NY: Springer International Publishing), 63–103.
Mingo, V., Lötters, S., and Wagner, N. (2017). The impact of land use intensity and associated pesticide applications on fitness and enzymatic activity in reptiles - A field study. Sci. Total Env. 590–591, 114–124. doi: 10.1016/j.scitotenv.2017.02.178
Moeller, A. H., Suzuki, T. A., Phifer-Rixey, M., and Nachman, M. W. (2018). Transmission modes of the mammalian gut microbiota. Science 362, 453–457. doi: 10.1126/science.aat7164
Murray, M. H., Lankau, E. W., Kidd, A. D., Welch, C. N., Ellison, T., Adams, H. C., et al. (2020). Gut microbiome shifts with urbanization and potentially facilitates a zoonotic pathogen in a wading bird. PLoS One 15:1–16. doi: 10.1371/journal.pone.0220926
Oksanen, A. J., Blanchet, F. G., Friendly, M., Kindt, R., Legendre, P., McGlinn, D., et al. (2019). Package ‘vegan’. R package version 2.5-6.
Patten, M. A., and Kelly, J. F. (2010). Habitat selection and the perceptual trap. Ecol. Applicat. 20, 2148–2156. doi: 10.1890/09-2370.1
Peschel, S., Müller, C. L., von Mutius, E., Boulesteix, A., and Depner, M. (2021). NetCoMi: Network Construction and Comparison for Microbiome Data in R. Brief Bioinform. 22:bbaa290
Petersen, C., and Round, J. L. (2014). Defining dysbiosis and its influence on host immunity and disease. Cell. Microbiol. 16, 1024–1033. doi: 10.1111/cmi.12308
Porras-Peñaranda, P., Robichaud, L., and Branch, F. (2004). New full-season count sites for raptor migration in Talamanca, Costa Rica. Ornitolog. Neotrop. 15, 267–278.
Price, M. N., Dehal, P. S., and Arkin, A. P. (2009). Fasttree: Computing large minimum evolution trees with profiles instead of a distance matrix. Mole. Biol. Evol. 26, 1641–1650. doi: 10.1093/molbev/msp077
Real, R., and Vargas, J. M. (1996). The probabilistic basis of Jaccard’s Index of Similarity. Syst. Biol. 45, 380–385. doi: 10.2307/2413572
Risely, A., Gillingham, M. A. F., Béchet, A., Brändel, S., Heni, A. C., Heurich, M., et al. (2020). Phylogeny – and abundance-based metrics allow for the consistent comparison of core gut microbiome diversity indices across host species. Front. Microb. 12:629918. doi: 10.3389/fmicb.2021.659918
Robeson, M. S. II, O’Rourke, D. R., Kaehler, B. D., Ziemski, M., Dillon, M. R., Foster, J. T., et al. (2020). RESCRIPt: Reproducible sequence taxonomy reference database management for the masses. bioRxiv 2020:326504. doi: 10.1101/2020.10.05.326504
Rothenwöhrer, C., Becker, N. I., and Tschapka, M. (2011). Resource landscape and spatio-temporal activity patterns of a plant-visiting bat in a Costa Rican lowland rainforest. J. Zool. 283, 108–116. doi: 10.1111/j.1469-7998.2010.00748.x
San Juan, P. A., Hendershot, J. N., Daily, G. C., and Fukami, T. (2020). Land-use change has host-specific influences on avian gut microbiomes. ISME J. 14, 318–321. doi: 10.1038/s41396-019-0535-4
Shehata, A. A., Schrödl, W., Aldin, A. A., Hafez, H. M., and Krüger, M. (2013). The effect of glyphosate on potential pathogens and beneficial members of poultry microbiota in vitro. Curr. Microbiol. 66, 350–358. doi: 10.1007/s00284-012-0277-2
Sikes, R. S., and Gannon, W. L. (2011). Guidelines of the American Society of Mammalogists for the use of wild mammals in research. J. Mamm. 92, 235–253. doi: 10.1644/10-MAMM-F-355.1
Solano, J., and Villalobos, R. (2012). Regiones y sub-regiones climáticas de Costa Rica. Costa Rica: Instituto Meteorológico Nacional.
Sonnenburg, E. D., and Sonnenburg, J. L. (2019). The ancestral and industrialized gut microbiota and implications for human health. Nat. Rev. Microbiol. 17, 383–390. doi: 10.1038/s41579-019-0191-8
Speer, K. A., Teixeira, T. S. M., Brown, A. M., Perkins, S. L., Dittmar, K., Ingala, M. R., et al. (2020). Parasite species identity and local community diversity mediate effects of habitat fragmentation on bacterial microbiomes. Authorea. Preprint 2020:35915032. doi: 10.22541/au.159200503.35915032
Stecher, B., Denzler, R., Maier, L., Bernet, F., Sanders, M. J., Pickard, D. J., et al. (2012). Gut inflammation can boost horizontal gene transfer between pathogenic and commensal Enterobacteriaceae. PNAS 109, 1269–1274. doi: 10.1073/pnas.1113246109
Stein-Bachinger, K., Gottwald, F., Haub, A., and Schmidt, E. (2020). To what extent does organic farming promote species richness and abundance in temperate climates? A review. Org. Agr. 2020:279. doi: 10.1007/s13165-020-00279-2
Suzuki, T. A. (2017). Links between natural variation in the microbiome and host fitness in wild mammals. Integr. Compar. Biol. 57, 756–769. doi: 10.1093/icb/icx104
Teyssier, A., Matthysen, E., Hudin, N. S., de Neve, L., White, J., and Lens, L. (2020). Diet contributes to urban-induced alterations in gut microbiota: experimental evidence from a wild passerine. Proc. R. Soc. B 287:20192182. doi: 10.1098/rspb.2019.2182
Tschapka, M. (2004). Energy density patterns of nectar resources permit coexistence within a guild of Neotropical flower-visiting bats. J. Zool. 263, 7–21. doi: 10.1017/S0952836903004734
Videvall, E., Son, S. J., Bensch, H. M., Strandh, M., Engelbrecht, A., Serfontein, N., et al. (2019). Major shifts in gut microbiota during development and its relationship to growth in ostriches. Mole. Ecol. 28, 2653–2667. doi: 10.1111/mec.15087
Wasimuddin Brändel, S. D., Tschapka, M., Page, R., Rasche, A., Corman, V. M., et al. (2018). Astrovirus infections induce age-dependent dysbiosis in gut microbiomes of bats. ISME J. 12, 2883–2893. doi: 10.1038/s41396-018-0239-1
Willig, M. R., Camilo, G. R., and Noble, S. J. (1993). Dietary overlap in frugivorous and insectivorous bats from edaphic cerrado habitats of Brazil. J. Mamm. 74, 117–128. doi: 10.2307/1381910
Woloszynek, S., Mell, J. C., Zhao, Z., Simpson, G., O’Connor, M. P., and Rosen, G. L. (2019). Exploring thematic structure and predicted functionality of 16S rRNA amplicon data. PLoS One 14:e0219235. doi: 10.1371/journal.pone.0219235
Yang, X., Song, Y., Zhang, C., Pang, Y., Song, X., Wu, M., et al. (2019). Effects of the glyphosate-based herbicide roundup on the survival, immune response, digestive activities and gut microbiota of the Chinese mitten crab, Eriocheir sinensis. Aqu. Toxicol. 214:105243. doi: 10.1016/j.aquatox.2019.105243
Keywords: co-occurrence networks, Enterobacteriaceae, Glossophaga soricina, habitat alteration, management intensity, organic agriculture, pesticide use
Citation: Alpízar P, Risely A, Tschapka M and Sommer S (2021) Agricultural Fast Food: Bats Feeding in Banana Monocultures Are Heavier but Have Less Diverse Gut Microbiota. Front. Ecol. Evol. 9:746783. doi: 10.3389/fevo.2021.746783
Received: 24 July 2021; Accepted: 26 August 2021;
Published: 23 September 2021.
Edited by:
Joel White, Université Toulouse III Paul Sabatier, FranceReviewed by:
Melissa R. Ingala, American Museum of Natural History, United StatesPriscilla San Juan, Stanford University, United States
Copyright © 2021 Alpízar, Risely, Tschapka and Sommer. This is an open-access article distributed under the terms of the Creative Commons Attribution License (CC BY). The use, distribution or reproduction in other forums is permitted, provided the original author(s) and the copyright owner(s) are credited and that the original publication in this journal is cited, in accordance with accepted academic practice. No use, distribution or reproduction is permitted which does not comply with these terms.
*Correspondence: Priscilla Alpízar, priscilla.alpizar@alumni.uni-ulm.de
†These authors have contributed equally to this work and share first authorship
‡These authors have contributed equally to this work and share senior authorship