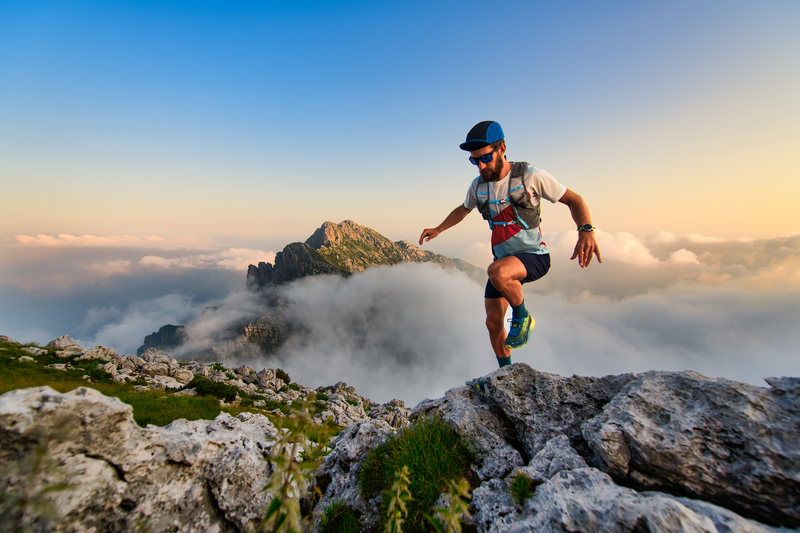
95% of researchers rate our articles as excellent or good
Learn more about the work of our research integrity team to safeguard the quality of each article we publish.
Find out more
ORIGINAL RESEARCH article
Front. Ecol. Evol. , 20 October 2021
Sec. Behavioral and Evolutionary Ecology
Volume 9 - 2021 | https://doi.org/10.3389/fevo.2021.732514
This article is part of the Research Topic Cognitive Movement Ecology View all 16 articles
Spatial partitioning between neighboring colonies is considered a widespread phenomenon in colonial species, reported mainly in marine birds. Partitioning is suspected to emerge due to various processes, such as competition, diet specialization, memory, information transfer, or even “foraging cultures.” Yet, empirical evidence from other taxa, and studies that tease apart the relative contribution of the processes underlying partitioning, remain scarce, mostly due to insufficiently detailed movement data. Here, we used high-resolution movement tracks (at 0.125 Hz) of 107 individuals belonging to two neighboring colonies of the Egyptian fruit bat (Rousettus aegyptiacus), a highly gregarious central-place forager, using the ATLAS reverse-GPS system in the Hula Valley, Israel. Based on comparisons between agent-based mechanistic models and observed spatial partitioning patterns, we found high levels of partitioning of both area and tree resources (<11% overlap) that were stable across different fruiting seasons. Importantly, partitioning could not have emerged if the bats’ movement was only limited by food availability and travel distances, as most commonly hypothesized. Rather than density-dependent or between-colony competition, memory, and, to a lesser extent, conformity in tree-use explain how partitioning develops. Elucidating the mechanisms that shape spatial partitioning among neighboring colonies in the wild under variable resource conditions is important for understanding the ecology and evolution of inter-group coexistence, space use patterns and sociality.
Foragers that congregate at a central place in large numbers (e.g., in roosts) face several conflicting demands. On the one hand, as the colony grows, direct and indirect competition forces individuals to spend energy exploring resources farther away from the colony (Ashmole, 1963; Gaston et al., 2007; Hinsch and Komdeur, 2010). On the other hand, congregations in roosts and around food patches offer ample opportunities to gather and transfer information about the location and status of unfamiliar resources (Dall et al., 2005; Van Moorter et al., 2009; Evans et al., 2016; Trapanese et al., 2019).
The tension between the risk of competition and information gain is even more acute when individuals from several conspecific colonies forage within the same landscape. In such cases, colonies often divide their territories to minimize conflict while sustaining intra-colony information flow (Adler and Gordon, 2003; Bolton et al., 2019). When resources and roosts are abundant, colonies are typically located far from each other, passively creating minimal resource mixing and competition risk (Wakefield et al., 2013; Aarts et al., 2021), a division termed the Hinterland Model (Cairns, 1989). Yet, accumulating evidence shows that spatial partitioning also occurs between neighboring colonies separated by a distance smaller than the individuals’ typical foraging distance. For example, Wakefield et al. (2013) showed that 12 colonies of northern gannets (Morus bassanus), distributed around the British shoreline, forage in mutually exclusive areas, including six colonies located very close to each other. Other studies reported spatial partitioning between neighboring colonies in a wide range of systems, most commonly in breeding colonies of marine birds (Ainley et al., 2004; Ceia et al., 2015; Corman et al., 2016; Mott et al., 2016; Sánchez et al., 2018; Bolton et al., 2019; Ito et al., 2020; Jessopp et al., 2020), but also in other birds (Cecere et al., 2018), ants (Gordon and Kulig, 1996; Adler and Gordon, 2003; Richardson et al., 2017) and a few other taxa (Ellis et al., 2009; Papastamatiou et al., 2018).
Although this line of evidence implies that spatial partitioning between neighboring colonies is widespread, three key elements are missing for understanding how and by which cognitive and social mechanisms partitioning is sustained. First, studies are biased toward marine birds while breeding, which cannot be readily generalized to other species that live within the same home range year-round or have different types of diet, such as non-mobile food (Bolton et al., 2019; Riotte-Lambert and Matthiopoulos, 2020). The degree of resource unpredictability, in particular, has been suggested as the key evolutionary driver of animal congregations, increasing information transfer and therefore reducing resource uncertainty (Ward and Zahavi, 1973; Egert-Berg et al., 2018; Gager, 2019; Riotte-Lambert and Matthiopoulos, 2020). Yet, most studies focus on a single season or average spatial partitioning across seasons and resource conditions, thereby neglecting the dynamic nature of resource availability (Grémillet et al., 2004; Aarts et al., 2021). Second, spatial partitioning is often reported based on the observation that colonies maintain minimal overlap between their foraging areas. However, to confirm that partitioning does not emerge through a random process or is the consequence of travel distance limitations alone, the observed overlap must be compared against those emerging from appropriate reference models that can isolate effects associated with these processes (Cecere et al., 2018; Bolton et al., 2019; Ito et al., 2020). Third, most studies did not systematically examine the mechanisms contributing to partitioning, namely competition, memory of resources, and information transfer within the colony (Grémillet et al., 2004; Corman et al., 2016; Bolton et al., 2019; Aarts et al., 2021). Furthermore, studies that investigated these mechanisms used population or agent-based simulations that were only partially derived from observed movement distributions, without comparing the results to the observed between-colony overlap (e.g., Barta and Szép, 1995; Adler and Gordon, 2003; Wakefield et al., 2013; Dallas et al., 2019; Aarts et al., 2021). Therefore, it remains unknown which mechanism, if any, explains observed spatial partitioning patterns under natural conditions. A major limitation in making these inferences has been the lack of movement data at a temporal resolution high enough to measure (and model) local spatial overlap. The emergence of new technologies, namely high-throughput reverse-GPS systems such as ATLAS (Toledo et al., 2020) and some acoustic telemetry systems (Baktoft et al., 2015), can now collect long-term, high-resolution movement data that can bridge these gaps.
The most hypothesized driver of spatial partitioning between neighboring colonies is density-dependent competition and its tradeoff with travel costs (Grémillet et al., 2004; Wakefield et al., 2013; Corman et al., 2016; Sheppard et al., 2018). According to this idea, coined the Density Dependent Hinterland Model by Wakefield et al. (2013), individuals of one colony expand their home range into areas overlapping an adjacent colony’s patches following local food depletion. As the number of individuals within the overlapping area increases, both colonies are pushed back to exploit more available resources in non-overlapping areas until an equilibrium is reached and the two colonies hold mutually exclusive ranges. However, this hypothesis assumes that density-dependent competition exists, despite little and very system-specific evidence (Lewis et al., 2001). Another possibility that has been given less attention is that individuals compete only with members of the other colony, that is, a between-colony competitive response that might affect spatial partitioning more directly than density-dependent competition. Yet, this idea assumes that conspecifics can differentiate between foreign and self-colony members and attribute the level of perceived competition accordingly. Some taxonomic groups, especially eusocial insect such as ants and bees, and highly social mammals such as mongoose, are known to recognize individuals from a rival group by processing chemical “signature mixtures.” Yet, chemical or similar recognition mechanisms are less likely to emerge in other groups, such as birds and less social mammals (Wyatt, 2005, 2010).
Information about resources may operate on top (or independent) of competition to sustain spatial partitioning between colonies (Wakefield et al., 2013; Riotte-Lambert and Matthiopoulos, 2019). Different levels of home-range familiarity and the ability to memorize previous visits to resource patches have been shown to drive home-range formation for many species (Moorcroft, 2012; Fagan et al., 2013; Merkle et al., 2014; Bartumeus et al., 2016). In fact, memory on its own was shown to create non-overlapping individual home ranges in simulated free-ranging foragers, even when resources deplete relatively fast due to intense competition (Riotte-Lambert et al., 2015). At the colony level, theoretical simulations incorporating individual memory without competition were sufficient to form spatial partitioning in central-place gregarious foragers that show high fidelity to their colony roosts (Aarts et al., 2021). A practical challenge in modeling the effect of memory on resource and spatial partitioning is evaluating the true, or realistic, memory coefficient, such as the mean probability of the return to previously visited sites (Aarts et al., 2021). This is because metrics of revisits are highly dependent on the tracking and resource data resolution (Bracis et al., 2018). For example, low temporal resolution may cause short area-restricted-search events to be overlooked and therefore revisits to these areas might be underestimated. From a spatial perspective, low resolution may attribute false revisits if the selected grid size of the study area is too large, compared to the real size of foraging patches (Fagan et al., 2013).
When resources are temporally predictable (e.g., mobile prey, carcasses, or fruits of different plant species), group-living animals often share information to reduce uncertainty about resources (Ward and Zahavi, 1973; Kerth et al., 2001; Weimerskirch et al., 2010; Evans et al., 2016; Harel et al., 2017). One potential outcome of repeated exposure to information within a group, whether gained intentionally or inadvertently, is that individuals may conform to the same behavior (Herbert-Read et al., 2013; Somveille et al., 2018). Accumulating evidence shows that some species conform to the most abundant behavior, even without personal experience, regarding food preferences (Van De Waal et al., 2013), strategies for problem-solving (Dindo et al., 2009; Aplin et al., 2015), and group movement speeds and habitat use (Herbert-Read et al., 2013; Wilson et al., 2019). Although theoretical models show that information sharing minimizes overlap between neighboring colonies (most evidently in Wakefield et al., 2013), this mechanism has not yet been explicitly examined using empirical data (Aarts et al., 2021).
In this study, we used high-resolution movement data (collected at 0.125 Hz) of 107 Egyptian fruit bats (Rousettus aegyptiacus; hereafter abbreviated as EFB) from two large neighboring colonies in the Hula Valley, north Israel, along with a complete dataset of all fruit trees they visited within the study area. Preliminary exploration of these data revealed considerable spatial partitioning between bats from two large colonies, which motivated the investigation of three main hypotheses on the mechanisms that might be responsible for the observed resource and spatial partitioning between neighboring colonies. We first hypothesized that such partitioning could not have emerged due to travel distance limitation only, nor by chance. Second, we hypothesized that partitioning could have emerged due to one or more of three mechanisms commonly suggested in the literature, namely density-dependent competition, memory of resources, and information sharing within each colony. Third, we hypothesized that the observed partitioning could have occurred at some theoretical memory or conformity coefficients, which do not necessarily match the observed ones. To examine the first two hypotheses, we built competitive agent-based models derived from real bat tracks to simulate the tree-use patterns formed when using each of the abovementioned mechanisms, separately and in conjunction. We then compared the simulated partitioning indices of each model—representing the overlap of trees and home ranges between colonies—against the observed ones. Due to the ambiguity about how competition operates between colonies, we examined the empirical evidence for density-dependent and between-colony competition separately before including competition in our models. To address the third hypothesis, we repeated the same simulation models but replaced the observed memory and conformity coefficients with theoretical values and examined the thresholds above which partitioning develops.
The Egyptian fruit bat (Rousettus aegyptiacus) is a long-lived, widely distributed Old World fruit bat (Pteropodidae, Kwiecinski and Griffith, 1999). In Israel, EFBs are generalist foragers consuming a wide variety of fruits, most of which have a predictable seasonal fruiting phenology (Tsoar et al., 2011). However, some fruit trees, especially of the non-native Ficus genus (Moraceae), fruit in unpredictable cycles, ranging from 1 to 8 cycles a year (e.g., Galil et al., 1977). The study area in the Hula Valley, north Israel (Figure 1), covers a 19,000-ha area and is an agriculturally dominated landscape inhabited by ∼2,500 EFB fruit bats that roost mainly in two large caves—Gershom with ∼1,400 and Zemer with ∼500 individuals. Three other caves are occasionally inhabited for varying periods, from days to months (pers. obs.). The two main cave-roosts are located 3.5 km apart, a distance much shorter than the average 10.3 km an EFB covers a night, based on our long-term (4 years) tracking data. Two lines of evidence and observations guided our reasoning in testing the effect of memory and conformity on resource-use patterns in this species. First, similar to other fruit bats, individual EFBs tend to feed on a small subset of available trees and repeatedly revisit them for weeks and even months (Egert-Berg et al., 2018; Toledo et al., 2020), which affirms that EFBs rely heavily on individual memory. Additionally, we have recently shown that EFBs obtain a “cognitive map,” which encompasses information about a large number of tree locations, suggesting that memory expands beyond the trees used at a given time (Toledo et al., 2020). Second, EFBs are highly gregarious central place foragers, found in the hundreds in cave-roosts and dozens around fruit trees during foraging. High roost fidelity (Figure 1), together with repeated visits to the same trees, creates opportunities to exchange information on fruit locality and status, driving individuals to conform to the use of the same resources.
Figure 1. Tracks of Egyptian fruit bats of two neighboring colonies, Zemer in yellow and Gershom in purple, showing resource (trees) and spatial (area) partitioning of foraging areas within the Hula Valley, Israel. Bats of both colonies show high fidelity to their cave-roosts (red spheres), expressed by the proportion of nights individual bats roosted in each focal cave (pie-charts per colony on the left panel). Gray portions of the pie-chart are the percent of cave switching nights from the focal cave to other caves. Black spheres are individual fruit trees visited by bats. Each map shows foraging movements within the (A) lean-fruit months of November-December (147 bat-nights of Gershom and 155 from Zemer, left map), and (B) during peaks of fleshy fruits in June-July (82 bat-nights Gershom and 108 Zemer, right map), accumulated across 2018–2020.
EFBs were captured using mist nets, either around trees or at cave-exits. Bats were tracked by ATLAS (Advanced Tracking and Localization of Animals in real-life Systems), a reverse-GPS system that utilizes extremely lightweight, low-cost tags at a high temporal frequency with a 5 m mean localization error (Toledo et al., 2016; Weiser et al., 2016). ATLAS tags were either mounted using a shrink-coated cable-tie collar to allow tracking for up to 9 months or glued on their upper-backs with surgical cement (Perma- Type, Plainville, CT, United States) for bats that had not yet reached full adult size. Tag units weigh 7.4 g (5.2% of mean adult body mass) for collars or 3 g without. Captures and tagging procedures have been approved by the Ethics Committee of the Hebrew University (permit NS-15-14-14660-2) and the Israeli Nature & Parks Authority (permit NS-2020/42577).
This study analyzed data of 107 bats that regularly roosted in one of the two main caves during 2018–2020. Bats were tracked at a 0.125 Hz sampling rate for an average tracking period of 23.7 nights and up to 131 nights. We also mapped nearly all fruit trees in the study area (14,314 trees and 18,111 orchard trees), which enabled us to identify specific tree visits.
To estimate resource and spatial partitioning between colonies, we first filtered raw EFB tracks for localization errors based on the covariance matrices attributed to each ATLAS fix (Gupte et al., 2021) and localization that exceeded the highest realistic speed threshold for this species of 20 m/s. Then, we defined visits to trees (location, tree ID, and duration) based on track segmentation utilizing the first-passage algorithm to determine the center of a “cloud of fixes” where the animal has spent a specified number of observations within a certain radius (source code and details at https://github.com/ATLAS-HUJI/R/tree/master/AdpFixedPoint). We then related the median coordinates of each cloud to the closest tree in the dataset. Colony membership was assigned based on the cave-roost a bat exited from each night. For this analysis, we took out foraging nights that followed cave switching events between the two main colonies because we could not choose the colony membership for those particular cases and since they were relatively rare (< 11% of bat-nights, Figure 1). Resource partitioning was measured as the percent of tree overlap (shared trees/all trees used, Table 1). Spatial partitioning was estimated by drawing the 75% Kernel utility density (KUD) contours for all bats of each colony and calculating the percent of area overlap (overlapping KUDs area/total area used, Table 1). We chose KUDs with the most common “href” bandwidth and selected the 75% contours to represent a compromise between falsely enlarging the area due to single events of longer bouts and underestimating the area due to the exclusion of short, but sometimes repetitive, visits to trees. For simplicity, we did not consider variation in tree quality when calculating overlap, for instance, by using the duration in time spent on a tree as a proxy of tree value. Both types of overlap are expected to vary according to fruit availability. For instance, some periods are characterized by a dense concentration of fruits around the line of equidistance between caves (e.g., Morus nigra and Ficus carica trees in May–July), while in other periods, bats rely on the emergence of less predictable fruits, e.g., invasive species of the Ficus genus (family Moraceae) in November-December. We, therefore, corrected for changes in fruit availability by estimating trees and area overlap for each month separately, including only months that contained at least five bats from each cave (range 20–33 total bats), aggregated across 2018–2020. Finally, the overlap estimation between tracked bats is more accurate for months in which we had more data. Hence, we weighted the percentage of overlap by the number of bat-nights in each month to create the weighted mean tree overlap (WTO) and the weighted mean area overlap (WAO) indices (Table 1).
Table 1. Observed movement parameters used in the agent-based models and indices of observed resource (trees) and spatial (areas) partitioning, for two neighboring Egyptian fruit bats colonies during 2018–2020.
The purpose of the agent-based models was to tease apart the contribution of memory, conformity, and competition on the observed resource and spatial partitioning patterns. To this end, we simulated the movement of bats with and without these different mechanisms and compared the emergent simulated partitioning indices against the ones observed in real EFBs (WTO and WAO, see section “Observed Metrics of Resource and Spatial Partitioning”). The first set of models uses movement data derived from observed bat tracks to identify the mechanism contributing to partitioning in our system. The second builds upon the same structure but uses theoretical values for memory and conformity to identify the value beyond which partitioning can (theoretically) develop. Here, we begin by detailing the empirical parameters used in the simulations (see Table 1 for a summary), and then we outline the principle simulation workflow (Figure 2). See supporting information for a more detailed model description, following the ODD (Overview, Design concepts, Details) protocol (Grimm et al., 2006, 2020).
Figure 2. Flowchart showing the decision-making process of a simulated bat at each step (tree visit) of the agent-based models. A step begins by sampling a bat-agent and ends with a tree selection. Colored frames represent the null model (gray) or the implementation of the different mechanisms that are hypothesized to affect resource and spatial partitioning—memory (red), conformity (blue) and competition (green). At the end of the simulation, each colony is attributed a set of trees from which the between-colony partitioning indices (WTO and WAO) are calculated.
Observed movement parameters were based on the tree-visit patterns from real bat tracks, the trees they visited, and the location of each colonys’ roost (Table 1). Movement parameters and the available tree dataset were collected separately for each month to account for seasonality (for reasoning, see section “Observed Metrics of Resource and Spatial Partitioning”). Movement parameters can be divided into three categories: (1) variables used to simulate the number of bats, nights tracked, and trees used per bat-night (steps). (2) Parameters to account for distance constraints—the distributions of flight distances undertaken by bats between the cave-roost and the first tree, as well as between consecutive trees (i.e., tree-to-tree distance). (3) Memory and conformity coefficients. Memory coefficients are the probabilities of visiting a tree used in previous nights (number of already-used trees/total trees, per bat-night). Since simulations have a time interval of 1 month, we assume that bats can remember all trees they used during this period, following our previous finding that EFBs memorize trees for at least 158 nights (Toledo et al., 2020). Conformity coefficients are the maximum proportion of shared trees among colony members (maximum number of shared trees/total trees, per colony). Conformity reflects information transfer indirectly because it assumes that if the information is shared (unrelated to how it is shared), it will push bats to conform and use the same trees. We used the maximum value of shared trees because the real proportion of trees shared (including non-tracked bats) is likely higher since EFBs almost always congregate around fruit trees (Kwiecinski and Griffith, 1999). Before incorporating competition into the model, we evaluated whether there is evidence for it in our data. Two types of competition were tested—density-dependent (bats are negatively affected by the presence of other bats on trees) and between-colony competition (bats are negatively affected by bats from the other colony). For density-dependent competition, we first estimated if the density of tracked bats increases around trees used by both colonies (i.e., “shared”) against trees used by a single colony. Then, we measured the probability to visit and the duration of time spent on trees that hosted increasing numbers of tracked bats to see if individuals changed their behavior in response to higher densities. Similarly, whether between-colony competition occurs was estimated based on the same dependent variables, but as a function of the number of bats from the other colony only. According to the competition results (Supplementary Figures 2–5), we implemented a simple and weak competition rule without considering different competition strengths in our models.
All simulations (illustrated in Figure 2) followed the same temporal hierarchy: First, the list of trees and the observed movement parameters were collected for each month (section “Observed Parameters” and Table 1). Then, the maximal number of observed tracked nights was sampled, and tree visits were simulated for each night separately. Since any of the studied mechanisms probably affected bats before our tracking began, we forced agents to select only trees originally attributed to their colony on the first night. Lastly, we derived the number of steps for each month and night to be twice the number of tracked bats and all the trees they visited (2 × n bats × n trees) as a compromise between sample size and computational load.
Each step began by randomly sampling a bat and ended with a tree visit (Figure 2). Bats were randomly sampled so that they could simultaneously forage and affect each other’s tree choices. The first time a bat was sampled, it was given a colony ID based on the observed probability of belonging to each colony (proportions of tracked bats from each roost). We assumed bats do not switch between colonies, owing to the high levels of roost-fidelity we observed (Figure 1). Additionally, although colony size changes seasonally (pers. obs.), there is no data to date on the duration, or even existence, of group cohesion of any size in this species. The selection of trees for the first night was initialized the same way in all models: for the first tree, a distance was randomly sampled from the observed distances from the roosts to the first tree that real bats visited (Table 1). Then the tree that was positioned the closest to that distance (± 100 m) was selected. The rest of the trees in the first night were selected in the same way, only based on a distance sampled from the observed tree-to-tree travel distance distribution (Table 1). The above first-night initiation resulted in a baseline of trees that bats of the given colony originally visited.
In the null model, bat-agents could choose among all seasonally available trees (of both colonies) and selected a tree based only on the tree-to-tree travel distances, as in the initiation phase (Figure 2). Simulating memory and conformity from observed coefficients (Table 1) followed the same decision sequence. For the memory model, a memory coefficient μ was randomly sampled from the probability distribution of visiting already-used trees. We then randomly sampled a probability r1, taken from a uniform distribution [0,1]. If μ > r1, the bat proceeded to select among known trees, i.e., made a memory step. The specific (known) tree was selected based on the relative proportion of the focal individual’s previous visits to each tree (a linear tendency to select highly used trees). For the conformity model, bats would select among unknown (new) trees that they did not memorize. Then, a conformity coefficient β was selected for each colony (a single value, see section “Observed Parameters”). We sampled another random number r2, and a conformity step was made if β > r2. The specific tree was then selected based on its “attractiveness,” i.e., the proportion of the same-colony members that used a tree. When neither the memory nor conformity models were applied, or when μ < r1 and β < r2, a tree was selected based on the tree-to-tree travel distance distribution, as in the null model. Competition in the simulation operated in retrospect after a tree was selected. When applied, a step was deleted if a probability θ that grows logarithmically with the density of simulated bats that used that tree was higher than a randomly sampled number r3 (Figure 2). Because removing visits results in an overall smaller number of steps, corresponding to a mean of 7 (± 3) percent of steps lost, we added 10% more steps when running the competition models.
In addition to the observed movement parameters (Table 1), the procedure above was repeated using theoretical and constant memory and conformity coefficients (range 0.2–0.97) to identify thresholds above which resource and spatial partitioning emerge. These resulted in one set of agent-based models derived from observed memory and conformity coefficients and additional sets for each theoretical memory and conformity coefficient, with and without their interaction with competition.
All models executed 100 iterations per month. Then WTO and WAO were calculated based on the trees and area overlap and the total number of simulated bat-nights per month (as for the observed indices, see section “Observed Metrics of Resource and Spatial Partitioning”). All models were constructed in R version 4.0.4 (R Core Team, 2021), with the rgeos package (Bivand and Rundel, 2020) for spatial object manipulations and amt package (Signer and Fieberg, 2019) for home range estimations and area overlap. Code will be made available upon request. p-values for testing the difference between each model against the observed tree or area overlap (WTO and WAO, respectively) were determined as the proportion of 100 simulated indices smaller than the observed index.
We analyzed 107 bats that were tracked for a total of 1,271 foraging nights. Out of which, 764 bat-nights originated from Gershom roost and 507 from Zemer. Bats visited < 10% of fruit trees in the study area (1,874 trees overall, mean per bat-night = 7.1). The data includes only tracks of months for which we had a sufficient number of bats from each roost simultaneously (>5 bats, 6 months in total), pooled across 2018–2020, and representing varying levels of fruit availability (see section “Observed Metrics of Resource and Spatial Partitioning”). The age and sex structures of tracked bats from each colony were similar: from Gershom, 51% were adults and 66% from Zemer, with the remaining individuals being < 1-year-old juveniles. Sex structure was also similar between colonies, with 62% males in Gershom and 54% in Zemer. The movement parameters of individual bats per colony were statistically the same (e.g., maximal distance, time spent foraging, mean duration foraging on a tree, p > 0.05, ArtAnova, Wobbrock et al., 2011) but differed in the total area covered, showing varying differences between colonies depending on the month (Table 1), and slightly in the number of trees used per bat (mean trees difference = 6 trees per bat-month, Table 1). Regardless of the relative fruit tree distribution, bats of both colonies showed markedly different foraging ranges, with very little overlap between them (Figure 1). As represented by the WTO index, resource partitioning was substantial, with only 5.4% of trees used by both colonies (ranging from 2 to 10% between months, Table 1). Similarly, spatial partitioning, represented by the WAO index, showed that 10% of the total area was shared between colonies (ranging from 3 to 19%), which is 11.8 km2. High levels of colony fidelity further reinforce observed partitioning as bats of both caves continued to roost in the same cave between 62 and 73% of foraging nights. For the remainder of the nights, bats mostly switched to temporary and smaller caves, spending only 8–10% of the nights at the other main cave (Figure 1).
We found support for the first hypothesis postulating that partitioning between EFB colonies is not the outcome of distance limitations nor chance, based on significantly lower observed WTO and WAO relative to the null model (null models indices = 48 and 25%, respectively, p < 0.01, Figure 3). Among the three drivers of partitioning in EFB, outlined in our second hypothesis, the memory model created the strongest effect with WTO < 20% and WAO < 5% (Figure 3). The observed conformity model had a smaller effect, with WTO < 35% and WAO < 15% and the competition model did not result in WTO or WAO distributions that were meaningfully different than those of the null model (Figure 3). Additionally, the combined effect of memory with conformity and competition did not reduce WTO or WAO substantially more than each mechanism in isolation (Figure 3). These results are directly linked to the observed memory and conformity coefficients (Table 1): memory was high, with > 83% of trees already visited in previous nights. The mean of the maximal conformity coefficient for each month was 25% (range 10–60%, Table 1), suggesting that only a small proportion of trees are shared between same colony members. We did not find evidence for competition because higher densities did not affect the duration bats spent on trees nor their revisit probabilities. This held both in the case of density-dependent competition (i.e., when considering total bat density, Supplementary Figures 2, 3) and between-colony competition (i.e., when considering only the densities of bats from the other colony, Supplementary Figures 4, 5). In the simulations, we nevertheless included competition, using only the density-dependent form, since it is more commonly considered the main driver of partitioning in the literature, to reference the effects of the two other tested mechanisms and all possible interactions between them. The effect of memory and conformity was stronger for WAO than WTO (Figure 3). Importantly, none of the models for resource partitioning derived from real tracks, and none of their interactions, significantly reached the observed level of WTO (Figure 3A). For area partitioning, however, all models incorporating memory (alone or associated interactions) showed a lower level of WAO compared to the observed ones, while those incorporating conformity (alone or with competition) nearly matched the observed level (Figure 3B).
Figure 3. Resource and spatial partitioning between two neighboring Egyptian fruit bat colonies. Violin plots represent the frequency distribution of (A) the weighted tree overlap (WTO, range: 9–51%) and (B) weighted area overlap (WAO, 75% KUDs, range: 0–31%) after 100 iterations of agent-based models for observed coefficients of memory and conformity, density-dependent competition, and their interactions. The dashed line represents the observed values of tree and area overlap. ∗p-value ≥ 0.05 comparing simulated and observed.
To address the third hypothesis and identify the thresholds above which resource and area partitioning can theoretically be reached, we used controlled and constant coefficients of memory, conformity, and the interaction between them and with density-dependent competition (Figure 4). We found that relatively high values of memory (= 0.97) and conformity (= 0.8), without any interactions, result in the observed WTO. These results suggest that if memory is the only mechanism and more than 97% of trees are repeatedly visited night after night, the observed resource partitioning is achieved. Similarly, the WTO is reached for conformity when 80% of the trees are shared among colony-members. Unlike partitioning of trees, the observed WAO was achieved at smaller coefficients (memory = 0.6, conformity = 0.4). Furthermore, the threshold values did not change meaningfully for both resource and area overlaps when each mechanism interacts with density-dependent competition. Surprisingly, the combined effect of conformity with memory at lower values (< 0.6 for WTO and < 0.4 for WAO) did not reach partitioning (heatmaps on Figure 4). A lack of interaction effects highlights that partitioning of both types is achieved by one of these mechanisms (at relatively high values), suggesting they act in a non-additive, independent manner.
Figure 4. Resource and spatial partitioning between two neighboring Egyptian fruit bat colonies. Each violin plot (A–D) represents the frequency distribution of the weighted tree overlap (WTO, A,C) and weighted area (75% KUDs) overlap (WAO, B,D) after 100 iterations of the agent-based models. (A–B) represent increasing values of theoretical memory coefficients on the x-axis in red (range 3–42% for WTO and 2–23% for WAO), and memory with competition in dark red (range 3–42% for WTO and 2–23% for WAO). (C,D) Represent increasing values of theoretical conformity coefficients on the x-axis in blue (range 3–39% for WTO and 0–19% for WAO), and conformity with competition in dark blue (range 3–40% for WTO and 0–23% for WAO). All dashed lines represent the observed WTO or WAO. Heatmaps (E,F) show the combined effect conformity and memory coefficients on the WTO (E) and WAO (F) indices, with darker red colors indicating smaller overlap. ∗p-value ≥ 0.05 comparing simulated and observed.
Spatial partitioning between neighboring colonies is considered a widespread phenomenon in colonial species, especially marine birds (Bolton et al., 2019). Although several plausible mechanisms have been proposed to explain what drives wild populations to segregate, they have rarely been systematically examined using competing models. Comparisons based on real movement data collected under varying resource availabilities are even more scarce (but see Wakefield et al., 2013; Cecere et al., 2018; Ito et al., 2020). Here, we analyzed high-throughput movement tracks from two neighboring colonies of Egyptian fruit bats, tracked during different fruit seasons. We found that the partitioning of both resources and areas was high, with < 11% of fruit trees and area being shared between colonies (Figures 3, 4). Importantly, these values could not have emerged if colonies’ foraging ranges were limited strictly by food availability and travel distances, as represented in our null-model (Figure 3) and hypothesized by the Hinterland model (Cairns, 1989). As such, this forms the first account of strong within-species spatial partitioning in a terrestrial mammal. We also found that memory was the strongest and most significant driver of resource and spatial partitioning (Figure 3). The role of memory suggests that individually acquired information, with or without information-sharing and competition, most likely sustains segregation between the two neighboring EFB colonies. This result is consistent with the high memory probabilities observed in this study (Table 1) and with known tendencies of these bats revisiting and thus memorizing the state of particular trees (Tsoar et al., 2011; Toledo et al., 2020).
However, when replacing the observed memory and conformity coefficients with theoretical ones, both memory and conformity resulted in the observed partitioning in isolation, at relatively high values (Figure 4). It is thus evident that information sharing can also drive resource and spatial partitioning (as suggested by, e.g., Wakefield et al., 2013; Corman et al., 2016). But conformity was less important in our study system characterized by animals that are highly familiar with their home-range and use mostly spatially predictable food resources (Gager, 2019; Harten et al., 2020; Toledo et al., 2020). Although the mechanisms that we tested explain the observed partitioning of colony areas, the weighted overlap of trees was statistically smaller than the one that emerged from all the models (Figures 3, 4). This may be due to tree characteristics, other than the fruiting season, which may have biased the bats’ tree selection more than we considered, resulting in fewer trees revisited overall and less of them shared between colonies.
The most commonly proposed mechanism for explaining inter-colony spatial partitioning is density-dependent competition (Grémillet et al., 2004; Wakefield et al., 2013; Bolton et al., 2019). However, competition is typically inferred based solely on the indirect measure of a positive relationship between colony size and foraging distances (Ashmole, 1963; Corman et al., 2016; Lamb et al., 2017). Here, we measured competition directly by examining changes to bat behavior as the local density on a tree increases. Based on this analysis, we did not find evidence for density-dependent competition (Supplementary Figures 2, 3) nor between-colony competition (Supplementary Figures 4, 5). Congruently, competition had no apparent effect on partitioning (Figure 4).
However, we cannot fully dismiss density effects, given that the number of tracked bats might not represent the true bat densities. Importantly, we assume that the relationship between the number of tracked and true bat densities is the same for both colonies. To resolve the ambiguity about the role of competition in spatial and resource partitioning, we hypothesize that density dependence might have driven foraging site selection strongly, following the Density Dependent Hinterland model (see section “Introduction” and Wakefield et al., 2013), but only during the initial stages of the colonies’ establishment. After the colonies formed, memory maintained partitioning by channeling individuals to use the same (non-overlapping) resources, and competition was reduced to act only as a “ceiling effect” by negatively influencing the experience of individuals that seldom invaded the neighboring colony’s range. In addition to memory, information transfer between same-colony members reinforce partitioning further because newly detected resources are more likely to be discovered within the colonys’ range, making exploration into the neighboring colonys’ territory less profitable unless the colony reached its carrying capacity (Grémillet et al., 2004; Wakefield et al., 2013). Because our models did not simulate the long-term dynamics of colony establishment across generations, this hypothesis could not be tested. Future studies aiming to untangle the links between density-dependence and inter-group partitioning could benefit from incorporating data on in situ aggressive interactions, for instance, recording social calls to estimate the intensity of agonistic interactions at sites with different densities (Prat et al., 2016).
The tendency of individuals to repeatedly visit the same locations is typical in many species and was given many synonyms, e.g., foraging site fidelity, recursive movement, memory-based, and reinforcement-learning movements (Grémillet et al., 2004; Fagan et al., 2013; Merkle et al., 2014; Berger-Tal and Bar-David, 2015; Wakefield et al., 2015; Bracis et al., 2018; Riotte-Lambert and Matthiopoulos, 2019; Goldshtein et al., 2020). While environmental cues could potentially trigger these behaviors, two characteristics of EFB movement imply that their recurrent visits to trees are driven by individual memory, following Fagan et al. (2013)’s classification. First, EFBs revisit specific trees, not patches with multiple foraging options, which must be beyond the individual’s perceptual range. Second, EFBs fly in very straight routes without using landmarks or beacons (Harten et al., 2020; Toledo et al., 2020), suggesting prior knowledge of their target location.
Several studies, mostly based on data-inspired simulations, point to the possibility that memory-based movement drives individual spatial and resource partitioning (Van Moorter et al., 2009; Merkle et al., 2014; Riotte-Lambert et al., 2015). For example, Goldshtein et al. (2020) tracked the movements of lactating females of the lesser long-nosed bat (Leptonycteris yerbabuenae), which travel immense distances to forage on isolated patches of flowering cacti each night. Once at the location, individuals partition into consistent foraging “cores,” with little overlap between them. Based on competitive agent-based models, the authors found that the best explanation for this division into cores was reinforcement-learning, a similar algorithm to the one used in our memory model. Recently, Aarts et al. (2021) showed that similar memory-based rules that create partitioning between individuals also drive between-colony segregation of central-place foragers. In their simulations, inspired by tracked movements of harbor seals (Phoca vitulina), they show that for species with spatial cognitive abilities and high levels of colony fidelity, memorizing a sub-set of available resources is sufficient to minimize overlap and conflict. Our results lend empirical support to these findings, showing that spatial partitioning in wild bats’ is primarily maintained by high memory levels (Figure 3 and Table 1).
Whether, how, and where information is shared in congregating species is species-specific and a matter of continuous debate (Barta and Giraldeau, 2001; Danchin et al., 2004; Evans et al., 2016; Jones et al., 2017). However, it appears plausible that fruit bats, which roost and forage in groups, use social information, irrespective of whether it was gained inadvertently or intentionally. For example, controlled captive experiments on Peter’s tent-making bats (Uroderma bilobatum) show that individuals prefer to interact with an unknown conspecific carrying a novel and palatable food item over an interaction with a familiar roost-mate carrying no food (Ramakers et al., 2016). Moreover, interactions were stronger if the odor originated from the information givers’ mouth than if it was only present on the surface of its fur, suggesting that bats also evaluate the quality of social information (O’Mara et al., 2014). Specifically for EFBs, it has been shown that roost-mates form long-lasting bonds, fitting to the producer-scrounger game where a sub-set of individuals actively collect food while others scrounge for it from their mouths (Harten et al., 2018).
However, the specific way information on food resources is used remains unknown in this species.
For example, EFBs were not observed foraging in pairs or groups (Harten et al., 2020; Toledo et al., 2020), rendering following behavior—a prerequisite for the information center hypothesis—highly unlikely (Barta and Giraldeau, 2001; Harel et al., 2017; Egert-Berg et al., 2018). Some bat species, mainly echolocating insectivores, gather local information by foraging in groups and eavesdropping on each other’s feeding buzzes (Fenton, 2003). Yet this pathway of local enhancement is less likely for EFBs (and fruit bats in general) because they rarely use echolocation or are found foraging in groups, and other types of local enhancement were not yet verified (Prat et al., 2016; Gager, 2019). Given this level of inquiry, we did not attempt to model complex information pathways. Rather, we used the term “conformity” and assumed that if information about fruit trees is shared and used by colony members, it will result in individuals conforming to use similar trees. We found that the observed conformity level of this EFB population was low (Table 1). Yet, conformity could, on its own, reach the observed resource and area partitioning at high (theoretical) coefficient values (Figure 4). The discrepancy between observed and theoretical conformity values (Figure 3 vs. Figure 4), as well as the independent effects of memory and conformity (Figure 4), point to a potential trade-off between the use of personal and social information, which is likely affected by the level of resource predictability, as elaborated in the next section.
The level of resource predictability in space and time determines whether individuals rely more on self-acquired (memory) or socially transferred information (Janmaat et al., 2016; Egert-Berg et al., 2018; Riotte-Lambert and Matthiopoulos, 2020). For example, when resources are spatially predictable, as are fruit trees, personal memory would be advantageous, as knowledge about the location of trees is not expected to vary. However, some introduced tree species, mainly of tropical origin, are temporally unpredictable in their fruiting phenology, producing abrupt and large fruit crops that are especially important in times of fruit scarcity (Galil et al., 1977; Marshall, 1983; Bleher et al., 2003; Fahr et al., 2015; Crestani et al., 2019). Accordingly, foragers highly familiar with their home range may adjust the frequency of using memory or information transfer based on the reliability of the information. At the group-level, one would expect an advantage of confining information transfer to the limits of a single colony. This is because separation into smaller units ensures naïve individuals are more rapidly exposed to the resources (e.g., in the roost). Moreover, density-dependent competition over the newly discovered resources is guaranteed to be limited to a single colony, at least until exploratory individuals of the other colony discover the same site.
While information affects movement decisions, individual space use patterns themselves alter resource predictability (Spiegel and Crofoot, 2016). For example, memory affects partitioning, which minimizes competition, reduces uncertainty about the accessibility to food resources, and feeds back to promote even higher reliance on memory (Lee et al., 2016; Riotte-Lambert and Matthiopoulos, 2020). More research is needed to understand how animals maneuver between using personal and social information under different predictability scenarios and how their behaviors affect the same considerations of conspecifics. Such endeavors might be achieved by field experiments that manipulate resource predictability, such as preventing access to highly used (memorized) resources or creating completely novel feeding opportunities in conjunction with long-term movement data.
Our simulations revealed differences in the level of partitioning depending on the resolution of the resources examined. Specifically, the simulations based on observed coefficients of memory and, to a lesser extent, conformity, were able to reconstruct the observed values for partitioning of areas (colony range) but not of the finer-grained level of trees (Figure 3). Technically, this discrepancy is unavoidable as multiple areas can be estimated between the same set of visited trees, similar to the “clique problem” for the distribution of suitable resources (Donovan et al., 2012). Thus, whereas estimating overlap among trees visited by members of each colony is straightforward and has a single solution, estimating area overlap requires subjective decisions on how to measure area coverage (e.g., choosing different kernel bandwidths or home range calculation methods) and has multiple solutions, implying a higher level of uncertainty. Furthermore, careful step-by-step observations of how tree and area overlap measures changed when simulated bats visited more trees revealed that the vast majority of newly visited trees were placed within the colony area at some relatively high number of steps. This finding implies that sufficiently long simulations lead to a higher level of tree overlap without increasing area overlap. Therefore, area and tree overlap estimates are likely to be under- and over-estimated, respectively, as reflected in our results.
Conceptually, this discrepancy raises the question of whether partitioning should be estimated at the finest grain of a single discrete resource, the larger grain of a resource patch, or perhaps the whole foraging range. In the case of EFBs, mounting evidence suggests high familiarity and fidelity to specific fruit trees (Tsoar et al., 2011; Harten et al., 2020; Toledo et al., 2020), indicating that a single tree is an appropriate resolution for this species. However, resources available to seabirds and probably many other animal species are unlikely to be fine-tuned to particular point locations. The relevant resolution is presumably at least as large as the typical size of a foraging patch (Vilk et al., 2021). Looking forward, the expansion of high-throughput tracking systems provide the means to examine further if, how, and why patterns of partitioning vary across spatial resolutions.
This study assessed the role of different mechanisms which underly resource and spatial portioning, one of the most fundamental processes enabling inter-colony coexistence. Methodologically, our study highlights the merits of high-throughput tracking systems like ATLAS that generate large high-resolution movement datasets. These datasets provide the means to reliably estimate the observed patterns and construct highly realistic models to unravel alternative underlying mechanisms, namely memory, information sharing, and competition, in free-ranging animals under various environmental conditions. Major questions for further research highlighted in this study are the lack of support for the role of the density-dependent competition in sustaining partitioning patterns continuously, the possibility for independent roles of personal memory or socially transferred information, and their potential association with variation in resource predictability.
The raw data supporting the conclusions of this article will be made available by the authors, without undue reservation.
The animal study was reviewed and approved by Ethics Committee of the Hebrew University (permit NS-15-14-14660-2) and the Israeli Nature and Parks Authority (permit NS-2020/42577).
EL and RN conceived the study. RN conceived. ST developed the ATLAS system. EL carried out fieldwork and analyzed the data together with IS. EL and RN wrote the manuscript with input from all other co-authors. All authors contributed to the article and approved the submitted version.
ATLAS development, maintenance, and studies have been supported by the Minerva Center for Movement Ecology, the Minerva Foundation, and ISF grant ISF-965/15; bat research in the movement ecology lab was supported also by grants from ISF-1316/05, ISF-1259/09, and GIF 1316/15. We also acknowledge support from Adelina and Massimo Della Pergola Chair of Life Sciences to RN.
The authors declare that the research was conducted in the absence of any commercial or financial relationships that could be construed as a potential conflict of interest.
All claims expressed in this article are solely those of the authors and do not necessarily represent those of their affiliated organizations, or those of the publisher, the editors and the reviewers. Any product that may be evaluated in this article, or claim that may be made by its manufacturer, is not guaranteed or endorsed by the publisher.
We thank Anat Levi and Ran Lotan for their valuable assistance with the fieldwork, Sivan Margalit for her support in the analysis, Yoav Bartan and Yotam Orchan for support with ATLAS, and all Movement Ecology Lab and the Minerva Center for Movement Ecology members.
The Supplementary Material for this article can be found online at: https://www.frontiersin.org/articles/10.3389/fevo.2021.732514/full#supplementary-material
Aarts, G., Mul, E., Fieberg, J., Brasseur, S., van Gils, J., Matthiopoulos, J., et al. (2021). Individual-level memory is sufficient to create spatial segregation among neighboring colonies of central-place foragers. Am. Nat. 198, E37–E52. doi: 10.1086/715014
Adler, F. R., and Gordon, D. M. (2003). Optimization, conflict, and non-overlapping foraging ranges in ants. Am. Nat. 162, 529–543.
Ainley, D. G., Ribic, C. A., Ballard, G., Heath, S., Gaffney, I., Karl, B. J., et al. (2004). Geographic structure of Adélie penguin populations: overlap in colony-specific foraging areas. Ecol. Monogr. 74, 159–178. doi: 10.1890/02-4073
Aplin, L. M., Farine, D. R., Morand-Ferron, J., Cockburn, A., Thornton, A., and Sheldon, B. C. (2015). Experimentally induced innovations lead to persistent culture via conformity in wild birds. Nature 518, 538–541.
Ashmole, N. P. (1963). The regulation of numbers of tropical oceanic birds. IBIS 103b, 458–473. doi: 10.1111/j.1474-919X.1963.tb06766.x
Baktoft, H., Petr, Z., Thomas, K., Jon, C. S, Lene, J., Martin, W. P., et al. (2015). Performance assessment of two whole-lake acoustic positional telemetry systems-is reality mining of free-ranging aquatic animals technologically possible? PLoS One 10:e0126534.
Barta, Z., and Giraldeau, L. A. (2001). Breeding colonies as information centers: a reappraisal of information-based hypotheses using the producer-scrounger game. Behav. Ecol. 12, 121–127. doi: 10.1093/beheco/12.2.121
Barta, Z., and Szép, T. (1995). Frequency-dependent selection on information-transfer strategies at breeding colonies: a simulation study. Behav. Ecol. 6, 308–310. doi: 10.1093/beheco/6.3.308
Bartumeus, F., Campos, D., Ryu, W. S., Lloret-Cabot, R., Méndez, V., and Catalan, J. (2016). Foraging success under uncertainty: search tradeoffs and optimal space use. Ecol. Lett. 19, 1299–1313. doi: 10.1111/ele.12660
Berger-Tal, O., and Bar-David, S. (2015). Recursive movement patterns: Review and synthesis across species. Ecosphere 6:149. doi: 10.1890/ES15-00106.1
Bivand, R., and Rundel, C. (2020). rgeos: Interface to Geometry Engine – Open Source (GEOS). Available online at: https://cran.r-project.org/package=rgeos (accessed August 2020).
Bleher, B., Potgieter, C. J., Johnson, D. N., and Böhning-Gaese, K. (2003). The importance of figs for frugivores in a South African coastal forest. J. Trop. Ecol. 19, 375–386. doi: 10.1017/S0266467403003420
Bolton, M., Conolly, G., Carroll, M., Wakefield, E. D., and Caldow, R. (2019). A review of the occurrence of inter-colony segregation of seabird foraging areas and the implications for marine environmental impact assessment. IBIS 161, 241–259. doi: 10.1111/ibi.12677
Bracis, C., Bildstein, K. L., and Mueller, T. (2018). Revisitation analysis uncovers spatio-temporal patterns in animal movement data. Ecography 41, 1801–1811. doi: 10.1111/ecog.03618
Cairns, D. K. (1989). The regulation of seabird colony size: a hinterland model. Am. Nat. 134, 141–146. doi: 10.1086/284970
Cecere, J. G., Bondì, S., Podofillini, S., Imperio, S., Griggio, M., Fulco, E., et al. (2018). Spatial segregation of home ranges between neighbouring colonies in a diurnal raptor. Sci. Rep. 8:11762. doi: 10.1038/s41598-018-29933-2
Ceia, F. R., Paiva, V. H., Ceia, R. S., Hervías, S., Garthe, S., Marques, J. C., et al. (2015). Spatial foraging segregation by close neighbours in a wide-ranging seabird. Oecologia 177, 431–440. doi: 10.1007/s00442-014-3109-1
Corman, A. M., Mendel, B., Voigt, C. C., and Garthe, S. (2016). Varying foraging patterns in response to competition? A multicolony approach in a generalist seabird. Ecol. Evol. 6, 974–986. doi: 10.1002/ece3.1884
Crestani, A. C., Mello, M. A. R., and Cazetta, E. (2019). Interindividual variations in plant and fruit traits affect the structure of a plant-frugivore network. Acta Oecol. 95, 120–127. doi: 10.1016/j.actao.2018.11.003
Dall, S. R. X., Giraldeau, L. A., Olsson, O., McNamara, J. M., and Stephens, D. W. (2005). Information and its use by animals in evolutionary ecology. Trends Ecol. Evol. 20, 187–193. doi: 10.1016/j.tree.2005.01.010
Dallas, T., Melbourne, B. A., and Hastings, A. (2019). When can competition and dispersal lead to checkerboard distributions? J. Anim. Ecol. 88, 269–276.
Danchin, É, Giraldeau, L. A., Valone, T. J., and Wagner, R. H. (2004). Public information: from noisy neighbors to cultural evolution. Science 305, 487–491. doi: 10.1126/science.1098254
Dindo, M., Whiten, A., and de Waal, F. B. M. (2009). Intra-group conformity sustains different foraging traditions in capuchin monkeys (Cebus apella). PLoS One 4:e7858. doi: 10.1371/journal.pone.0007858
Donovan, T. M., Warrington, G. S., Schwenk, W. S., and Dinitz, J. H. (2012). Estimating landscape carrying capacity through maximum clique analysis. Ecol. App. 22, 2265–2276.
Egert-Berg, K., Hurme, E. R., Greif, S., Goldstein, A., Harten, L., Herrera, L. G. M., et al. (2018). Resource ephemerality drives social foraging in bats. Curr. Biol. 28, 3667.e–3673.e. doi: 10.1016/j.cub.2018.09.064
Ellis, W. A. H., Melzer, A., and Bercovitch, F. B. (2009). Spatiotemporal dynamics of habitat use by koalas: the checkerboard model. Behav. Ecol. Sociobiol. 63, 1181–1188. doi: 10.1007/s00265-009-0761-2
Evans, J. C., Votier, S. C., and Dall, S. R. X. (2016). Information use in colonial living. Biol. Rev. Camb. Philos. Soc. 91, 658–672. doi: 10.1111/brv.12188
Fagan, W. F., Lewis, M. A., Auger-Méthé, M., Avgar, T., Benhamou, S., Breed, G., et al. (2013). Spatial memory and animal movement. Ecol. Lett. 16, 1316–1329. doi: 10.1111/ele.12165
Fahr, J., Abedi-Lartey, M., Esch, T., Machwitz, M., Suu-Ire, R., Wikelski, M., et al. (2015). Pronounced seasonal changes in the movement ecology of a highly gregarious central-place forager, the African straw-coloured fruit bat (Eidolon helvum). PLoS One 10:e0138985. doi: 10.1371/journal.pone.0138985
Fenton, M. B. (2003). Eavesdropping on the echolocation and social calls of bats. Mamm. Rev. 33, 193–204.
Gager, Y. (2019). Information transfer about food as a reason for sociality in bats. Mamm. Rev. 49, 113–120. doi: 10.1111/mam.12146
Galil, J., Stein, M., and Horovitz, A. (1977). On the origin of the sycamore fig Ficus sycomorus in the middle east. Gard. Bull. 29, 191–206.
Gaston, A. J., Ydenberg, R. C., and Smith, G. E. J. (2007). Ashmole’s halo and population regulation in seabirds. Mar. Ornithol. 35, 119–126.
Goldshtein, A., Handel, M., Eitan, O., Bonstein, A., Shaler, T., Collet, S., et al. (2020). Reinforcement learning enables resource partitioning in foraging bats. Curr. Biol. 30, 4096–4102.e6. doi: 10.1016/j.cub.2020.07.079
Gordon, D. M., and Kulig, A. W. (1996). Founding, foraging, and fighting: colony size and the spatial distribution of harvester ant nests. Ecology 77, 2393–2409. doi: 10.2307/2265741
Grémillet, D., Dell’Omo, G., Ryan, P. G., Peters, G., Ropert-Coudert, Y., and Weeks, S. J. (2004). Offshore diplomacy, or how seabirds mitigate intra-specific competition: a case study based on GPS tracking of Cape gannets from neighbouring colonies. Mar. Ecol. Prog. Ser. 268, 265–279. doi: 10.3354/meps268265
Grimm, V., Berger, U., Bastiansen, F., Eliassen, S., Ginot, V., Giske, J., et al. (2006). A standard protocol for describing individual-based and agent-based models. Ecol. Modell. 198, 115–126. doi: 10.1016/j.ecolmodel.2006.04.023
Grimm, V., Railsback, S. F., Vincenot, C. E., Berger, U., Gallagher, C., Deangelis, D. L., et al. (2020). The ODD protocol for describing agent-based and other simulation models: a second update to improve clarity, replication, and structural realism. J. Artif. Soc. Soc. Simul. 23:7. doi: 10.18564/jasss.4259
Gupte, P. R., Beardsworth, C. E., Spiegel, O., Lourie, E., Toledo, S., Nathan, R., et al. (2021). A guide to pre-processing high-throughput animal tracking data. bioRxiv [Preprint] doi: 10.1101/2020.12.15.422876
Harel, R., Spiegel, O., Getz, W. M., and Nathan, R. (2017). Social foraging and individual consistency in following behaviour: testing the information centre hypothesis in free-ranging vultures. Proc. R. Soc. B Biol. Sci. 284:20162654. doi: 10.1098/rspb.2016.2654
Harten, L., Katz, A., Goldshtein, A., Handel, M., and Yovel, Y. (2020). The ontogeny of a mammalian cognitive map in the real world. Science 369, 194–197. doi: 10.1126/science.aay3354
Harten, L., Matalon, Y., Galli, N., Navon, H., Dor, R., and Yovel, Y. (2018). Persistent producer-scrounger relationships in bats. Sci. Adv. 4:e1603293. doi: 10.1126/sciadv.1603293
Herbert-Read, J. E., Krause, S., Morrell, L. J., Schaerf, T. M., Krause, J., and Ward, A. J. W. (2013). The role of individuality in collective group movement. Proc. R. Soc. B Biol. Sci. 280:20122564. doi: 10.1098/rspb.2012.2564
Hinsch, M., and Komdeur, J. (2010). Defence, intrusion and the evolutionary stability of territoriality. J. Theor. Biol. 266, 606–613. doi: 10.1016/j.jtbi.2010.07.033
Ito, K., Watanabe, Y. Y., Kokubun, N., and Takahashi, A. (2020). Inter-colony foraging area segregation quantified in small colonies of Adélie Penguins. IBIS 163:12837. doi: 10.1111/ibi.12837
Janmaat, K. R. L., Boesch, C., Byrne, R., Chapman, C. A., Goné Bi, Z. B., Head, J. S., et al. (2016). Spatio-temporal complexity of chimpanzee food: how cognitive adaptations can counteract the ephemeral nature of ripe fruit. Am. J. Primatol. 78, 626–645. doi: 10.1002/ajp.22527
Jessopp, M., Arneill, G. E., Nykänen, M., Bennison, A., and Rogan, E. (2020). Central place foraging drives niche partitioning in seabirds. Oikos 129, 1704–1713. doi: 10.1111/oik.07509
Jones, T. B., Aplin, L. M., Devost, I., and Morand-Ferron, J. (2017). Individual and ecological determinants of social information transmission in the wild. Anim. Behav. 129, 93–101. doi: 10.1016/j.anbehav.2017.05.011
Kerth, G., Wagner, M., and König, B. (2001). Roosting together, foraging apart: Information transfer about food is unlikely to explain sociality in female Bechstein’s bats (Myotis bechsteinii). Behav. Ecol. Sociobiol. 50, 283–291. doi: 10.1007/s002650100352
Lamb, J. S., Satgé, Y. G., and Jodice, P. G. R. (2017). Influence of density-dependent competition on foraging and migratory behavior of a subtropical colonial seabird. Ecol. Evol. 7, 6469–6481. doi: 10.1002/ece3.3216
Lee, A. E. G., Ounsley, J. P., Coulson, T., Marcus Rowcliffe, J., and Cowlishaw, G. (2016). Information use and resource competition: an integrative framework. Proc. R. Soc. B Biol. Sci. 283:20152550. doi: 10.1098/rspb.2015.2550
Lewis, S., Sherratt, T. N., Hamer, K. C., and Wanless, S. (2001). Evidence of intra-specific competition for food in a pelagic seabird. Nature 412, 816–819.
Marshall, A. G. (1983). Bats, flowers and fruit: evolutionary relationships in the Old World. Biol. J. Linn. Soc. 20, 115–135. doi: 10.1111/j.1095-8312.1983.tb01593.x
Merkle, J. A., Fortin, D., and Morales, J. M. (2014). A memory-based foraging tactic reveals an adaptive mechanism for restricted space use. Ecol. Lett. 17, 924–931. doi: 10.1111/ele.12294
Moorcroft, P. R. (2012). Mechanistic approaches to understanding and predicting mammalian space use: recent advances, future directions. J. Mammal. 93, 903–916. doi: 10.1644/11-MAMM-S-254.1
Mott, R., Herrod, A., and Clarke, R. H. (2016). Interpopulation resource partitioning of Lesser Frigatebirds and the influence of environmental context. Ecol. Evol. 6, 8583–8594. doi: 10.1002/ece3.2565
O’Mara, T., Dechmann, D. K. N., and Page, R. A. (2014). Frugivorous bats evaluate the quality of social information when choosing novel foods. Behav. Ecol. 25, 1233–1239. doi: 10.1093/beheco/aru120
Papastamatiou, Y. P., Bodey, T. W., Friedlander, A. M., Lowe, C. G., Bradley, D., Weng, K., et al. (2018). Spatial separation without territoriality in shark communities. Oikos 127, 767–779. doi: 10.1111/oik.04289
Prat, Y., Taub, M., and Yovel, Y. (2016). Everyday bat vocalizations contain information about emitter, addressee, context, and behavior. Sci. Rep. 6:39419. doi: 10.1038/srep39419
Ramakers, J. J. C., Dechmann, D. K. N., Page, R. A., and Mara, M. T. O. (2016). Frugivorous bats prefer information from novel social partners. Anim. Behav. 116, 83–87. doi: 10.1016/j.anbehav.2016.03.021
Richardson, T. O., Giuggioli, L., Franks, N. R., and Sendova-Franks, A. B. (2017). Measuring site fidelity and spatial segregation within animal societies. Methods Ecol. Evol. 8, 965–975. doi: 10.1111/2041-210X.12751
Riotte-Lambert, L., and Matthiopoulos, J. (2019). Communal and efficient movement routines can develop spontaneously through public information use. Behav. Ecol. 30, 408–416. doi: 10.1093/beheco/ary180
Riotte-Lambert, L., and Matthiopoulos, J. (2020). Environmental predictability as a cause and consequence of animal movement. Trends Ecol. Evol. 35, 163–174. doi: 10.1016/j.tree.2019.09.009
Riotte-Lambert, L., Benhamou, S., and Chamaillé-Jammes, S. (2015). How memory-based movement leads to nonterritorial spatial segregation. Am. Nat. 185, E103–E116. doi: 10.1086/680009
Sánchez, S., Reina, R. D., Kato, A., Ropert-Coudert, Y., Cavallo, C., Hays, G. C., et al. (2018). Within-colony spatial segregation leads to foraging behaviour variation in a seabird. Mar. Ecol. Prog. Ser. 606, 215–230. doi: 10.3354/meps12764
Sheppard, C. E., Inger, R., McDonald, R. A., Barker, S., Jackson, A. L., Thompson, F. J., et al. (2018). Intragroup competition predicts individual foraging specialisation in a group-living mammal. Ecol. Lett. 21, 665–673. doi: 10.1111/ele.12933
Signer, J., and Fieberg, J. (2019). Animal movement tools (amt): R package for managing tracking data and conducting habitat selection analyses. Ecol. Evol. 2, 880–890. doi: 10.1002/ece3.4823
Somveille, M., Firth, J. A., Aplin, L. M., Farine, D. R., Sheldon, B. C., and Thompson, R. N. (2018). Movement and conformity interact to establish local behavioural traditions in animal populations. PLoS Comput. Biol. 14:e1006647. doi: 10.1371/journal.pcbi.1006647
Spiegel, O., and Crofoot, M. C. (2016). The feedback between where we go and what we know – information shapes movement, but movement also impacts information acquisition. Curr. Opin. Behav. Sci. 12, 90–96. doi: 10.1016/j.cobeha.2016.09.009
Toledo, S., Kishon, O., Orchan, Y., Shohat, A., and Nathan, R. (2016). “Lessons and experiences from the design, implementation, and deployment of a wildlife tracking system,” in Proceedings of the 2016 IEEE International Conference on Software Science, Technology and Engineering (SWSTE), (Piscataway, NJ: IEEE), 51–60.
Toledo, S., Shohami, D., Schiffner, I., Lourie, E., Orchan, Y., Bartan, Y., et al. (2020). Cognitive map-based navigation in wild bats revealed by a new high-throughput wildlife tracking system. Science 193, 188–193.
Trapanese, C., Meunier, H., and Masi, S. (2019). What, where and when: spatial foraging decisions in primates. Biol. Rev. 94, 483–502. doi: 10.1111/brv.12462
Tsoar, A., Nathan, R., Bartan, Y., Vyssotski, A., Dell’Omo, G., and Ulanovsky, N. (2011). Large-scale navigational map in a mammal. Proc. R. Soc. B Biol. Sci. 108, E718–E724.
Van De Waal, E., Borgeaud, C., and Whiten, A. (2013). Potent social learning and conformity shape a wild primate’s foraging decisions. Science 340, 483–485. doi: 10.1126/science.1232769
Van Moorter, B., Visscher, D., Benhamou, S., Börger, L., Boyce, M. S., and Gaillard, J. M. (2009). Memory keeps you at home: a mechanistic model for home range emergence. Oikos 118, 641–652. doi: 10.1111/j.1600-0706.2008.17003.x
Vilk, O., Orchan, Y., Charter, M., Ganot, N., Toledo, S., Nathan, R., et al. (2021). Ergodicity breaking and lack of a typical waiting time in area-restricted search of avian predators. arXiv [Preprint] 2101.11527,
Wakefield, E. D., Bodey, T. W., Bearhop, S., Blackburn, J., Colhoun, K., Davies, R., et al. (2013). Space partitioning without territoriality in gannets. Science 341, 68–70. doi: 10.1126/science.1236077
Wakefield, E. D., Cleasby, I. R., Bearhop, S., Bodey, T. W., Davies, R. D., Miller, P. I., et al. (2015). Long-term individual foraging site fidelity-why some gannets don’t change their spots. Ecology 96, 3058–3074. doi: 10.1890/14-1300.1
Ward, P., and Zahavi, A. (1973). The importance of certain assemblages of birds as “information-centres” for food-finding. IBIS 115, 517–534.
Weimerskirch, H., Bertrand, S., Silva, J., Marques, J. C., and Goya, E. (2010). Use of social information in seabirds: compass rafts indicate the heading of food patches. PLoS One 5:e9928. doi: 10.1371/journal.pone.0009928
Weiser, A. W., Orchan, Y., Nathan, R., Charter, M., Weiss, A. J., and Toledo, S. (2016). “Characterizing the accuracy of a self-synchronized reverse-GPS wildlife localization system,” in Proceedings of the 2016 15th ACM/IEEE International Conference Information Processing Sensor Networks, IPSN 2016, (Vienna). doi: 10.1109/IPSN.2016.7460662
Wilson, A. D. M., Burns, A. L. J., Crosato, E., Lizier, J., Prokopenko, M., Schaerf, T. M., et al. (2019). Conformity in the collective: differences in hunger affect individual and group behavior in a shoaling fish. Behav. Ecol. 30, 968–974. doi: 10.1093/beheco/arz036
Wobbrock, J. O., Findlater, L., Gergle, D., and Higgins, J. J. (2011). “The aligned rank transform for nonparametric factorial analyses using only ANOVA procedures,” in Proceedings of the SIGCHI Conference on Human Factors in Computing Systems, (New York, NY: ACM), 143–146. doi: 10.1145/1978942.1978963
Wyatt, T. D. (2005). Pheromones: convergence and contrasts in insects and vertebrates. Chem. Signal. Verteb. Spri. 10, 7–19.
Keywords: partitioning, memory, conformity, competition, Rousettus aegyptiacus, animal movement, coloniality
Citation: Lourie E, Schiffner I, Toledo S and Nathan R (2021) Memory and Conformity, but Not Competition, Explain Spatial Partitioning Between Two Neighboring Fruit Bat Colonies. Front. Ecol. Evol. 9:732514. doi: 10.3389/fevo.2021.732514
Received: 29 June 2021; Accepted: 23 September 2021;
Published: 20 October 2021.
Edited by:
Eliezer Gurarie, University of Maryland, College Park, United StatesReviewed by:
Edward Hurme, Max Planck Institute of Animal Behaviour, GermanyCopyright © 2021 Lourie, Schiffner, Toledo and Nathan. This is an open-access article distributed under the terms of the Creative Commons Attribution License (CC BY). The use, distribution or reproduction in other forums is permitted, provided the original author(s) and the copyright owner(s) are credited and that the original publication in this journal is cited, in accordance with accepted academic practice. No use, distribution or reproduction is permitted which does not comply with these terms.
*Correspondence: Emmanuel Lourie, ZW1tLmxvdXJpZUBnbWFpbC5jb20=
Disclaimer: All claims expressed in this article are solely those of the authors and do not necessarily represent those of their affiliated organizations, or those of the publisher, the editors and the reviewers. Any product that may be evaluated in this article or claim that may be made by its manufacturer is not guaranteed or endorsed by the publisher.
Research integrity at Frontiers
Learn more about the work of our research integrity team to safeguard the quality of each article we publish.