- 1School of Management, University of Science and Technology of China, Hefei, China
- 2Economics and Management School, Nantong University, Nantong, China
- 3School of Economics and Management, Nanjing Forestry University, Nanjing, China
Urban agglomeration has become a unique form of cities during the rapid development of emerging economies. With the increasing attention on global energy and environmental efficiency, air quality evaluation and pollution control have become important standards to measure the health and orderly development of such agglomerations. Based on panel data of 60 cities in the three major urban agglomerations of Beijing-Tianjin-Hebei (BTH), Yangtze River Delta (YRD), and Pearl River Delta (PRD), this study uses the Modified MetaFrontier Dynamic SBM model to evaluate their air quality over the 5-year period of 2013–2017. The results present that the development level of air pollution prevention and control in China’s three major urban agglomerations is relatively low, and YRD as the most developed area has the worst effect of air pollution prevention and control. The MetaFrontier and Group Frontier Efficiency analysis confirms the conclusion of the cluster analysis that a significant two-level differentiation exists in China’s three urban agglomerations. Moreover, China’s three major urban agglomerations are still in the stage of high energy consumption and high development. Lastly, we point out different recommendations for industrial structure and governance foci of the three major urban agglomerations. Dust prevention technology should be improved to reduce PM2.5 in BTH, desulfurization technology should be enhanced to cut industrial SO2 emissions in YRD, and better emission reduction targets and other targeted measures should be formulated in PRD.
Introduction
With the development of economic globalization and regional integration, the degree of agglomeration between cities has significantly improved, and urban agglomerations are emerging with one or more large cities or mega-cities as their core. For example, the Northeast Megalopolis1 in the northeast of the United States, the Great Lakes Megalopolis2 in North America, the Pacific Coastal Urban Agglomeration3 in Japan, the London Metropolitan Area,4 the Northwest European Urban Agglomeration, and the Yangtze River Delta (YRD) in China have become universally recognized world-class urban agglomerations. Gottmann (1957) believes that urban agglomeration as the core of a region has a great impact on the region’s political, economic, cultural, and other fields. Different from the past, urban agglomeration has gradually replaced the single city as an independent unit of development and research subject. However, environmental pollution and air quality problems in urban agglomerations have become more and more serious, thus attracting broader scholarly research and attention, such as the famous Great Smog of London (Polivka, 2018), the Donora Smog (Jacobs et al., 2018), and the dead lakes caused by industrial acid rain in North America. Governments and international organizations around the world have issued corresponding agreements to combat air pollution, such as the ASEAN Agreement on Transnational Haze Pollution (2002), Montreal Protocol (1987), etc. The research results on air quality evaluation of urban agglomerations conform to the direction and focus of global environmental protection.
China’s own urban agglomerations have been developing rapidly in recent years. City cluster areas driven by urban agglomerations are now a huge influence space for attracting domestic and international businesses. Located respectively in North China, East China, and South China and accounting for about 1/5 of China’s total population and 34.2% of its gross domestic product (GDP) (2017),5 Beijing-Tianjin-Hebei (BTH), YRD, and PRD are the three most representative urban agglomerations in the country and cover Beijing (BTH), Shanghai (YRD), Guangzhou (PRD), Shenzhen (PRD), and other dominant political, cultural, economic, financial, and first-tier cities. YRD is especially one of the premier world-class urban agglomerations6 and has had important economic and social impacts on the world.
The China government has paid attention to the air pollution caused by economic development and approved the Action Plan For the Prevention and Control of Air Pollution in 2013, which shows it is attaching great importance to environmental governance, energy conservation, and emission reduction, but the actual outcomes are not optimistic. In 2019, among 337 prefecture-level and above cities in China, only 157 met the standard of ambient air quality, accounting for 46.6% of the total number of cities, while the ambient air quality of 180 cities exceeded the standard, accounting for 53.4%.7 Therefore, it is of great practical significance to evaluate the air quality efficiency of typical urban agglomerations in China.
Most international research studies on air quality efficiency evaluation are based on single cities (Kassomenos et al., 2012; Yotova et al., 2016) and the whole country (Aleksandropoulou et al., 2012; Wang and Wang, 2019; Zhou et al., 2020) as research objects, or they do not focus on efficiency, but rather other kinds of evaluations of air or environmental quality in urban agglomerations (Chen et al., 2017; Wang Y. et al., 2017; Xu L. et al., 2019; Yu et al., 2020; Zhang et al., 2020). Few researchers have actually studied the efficiency evaluation and improvement of air quality in important urban agglomerations around the world such as China’s three major urban agglomerations in this study at present. In terms of economic level or geographical location, urban agglomerations offer great research value. While they are economically developed and densely populated areas, their air quality has significant impacts on people’s lives such as giving rise to traffic accidents and air pollution diseases. Therefore, this article not only offers great practical significance, but also can fill the existing research gaps. Our study also presents suggestions for the three urban agglomerations on air quality improvement and economic coordinated development.
Based on the efficiency evaluation perspective of the input–output method, this article constructs the Modified MetaFrontier Dynamic SBM model, using Employment Population and Energy Consumption per Unit of GDP as input indicators and GDP, Industrial SO2 Emissions, PM2.5, and Industrial Smoke Dust Emissions as output indicators to calculate the MetaFrontier Efficiency (MFE), Group Frontier Efficiency (GFE), and TGR and to evaluate the air quality of 60 cities in these three urban agglomerations from 2013 to 2017. We also include the Fixed Asset Investment indicator as a carry-over variable to subdivide the change and impact of air quality over the time series. The results of this article offer forward-looking significance and innovative value for environmental evaluation and air governance of world-class urban agglomerations.
Literature Review
Air quality evaluations are presently divided into three directions as follows.
Air Quality Efficiency Evaluation Based on Data Envelopment Analysis Methods
The data envelopment analysis (DEA) approach is widely employed for evaluating air pollution by composing environmental efficiency of different entities in public or private sectors (Sueyoshi et al., 2017). Some advanced DEA models are constructed to measure the environmental efficiency of CO2 emissions by generating multiple variables into one index, such as the slack-based measure (SBM; Tone, 2003), directional distance function (Song and Wang, 2018), two-stage DEA model (Hu et al., 2018), and meta-frontier DEA model (Ding et al., 2020). Additional emissions of atmospheric particles are also taken into consideration to scale the environmental efficiency of decision making units (DMUs). Under the DEA framework, Zaim (2004) consider NO, SO2, and CO as the major indices to reflect the negative influence upon air quality, while Sueyoshi and Yuan (2015) use PM2.5 and PM10 to measure environmental efficiency. Wang et al. (2013) select CO2 and SO2 as the major air pollution emissions and use DEA window analysis to estimate regional total-factor energy and environmental efficiency of different regions in China. Zhou et al. (2018) apply the AQI to calculate China’s air quality, which includes NO, SO2, CO, PM2.5, and PM10. Halkos and Polemis (2018) construct a hybrid window DEA model to calculate the environmental efficiency of the 50 states in the United States, taking CO2, Nox, and SO2 into consideration as undesirable outputs. These studies show that the DEA model has good applicability to air quality analysis, especially on a spatial scale.
Many comprehensive methods such as the DEA model are combined with other models or indices and used in studies, like the SBM model, GML productivity index, and panel Tobit model being combined to study pollution emission efficiency. One work finds that pollution emission efficiency in China has improved, but it still needs to be further strengthened (Wang K.-L. et al., 2019). The Non-radial Direction Distance Function (DDFC) and its dual model are able to evaluate air environmental efficiency, with findings presenting that the efficiency tends to improve, but supervision needs to be strengthened (Zhang et al., 2020). DEA windows analysis and AHP are also combined for various analyses. Some results show that the most serious air pollution areas in China are concentrated in the central region, which is a continuous pollution area with Shanxi Province as its center (Zhou et al., 2020). One paper combines the interval evidence reasoning (IER) model and interval data envelopment analysis (IDEA) to evaluate the interval efficiency of air pollution management and finds, compared to some existing efficiency evaluation models, that the IER-IDEA model effectively distinguishes the differences of regional air pollution management efficiency (Ye et al., 2020).
Fractional dimension and scenario analysis are also used on the collaborative development of the low-carbon economy. Results show that the transfer of low-carbon economic development capacity from high gradient area to low gradient area will be delayed, but it will not affect the coordinated development of urban agglomerations (Xu W. B. et al., 2019). Super-efficiency DEA and the Malmquist index have been combined to evaluate dynamic environmental efficiency (Chen et al., 2017) and findings note that YRD has higher environment efficiency than that in the Chengyu Urban Agglomeration and Urban Agglomeration in the Middle Reaches of the Yangtze River areas. This indicates that the scale and location of urban agglomerations have a certain correlation with environmental efficiency, and it is necessary to strengthen the hypothesis and uncertainty outcome of the DEA model under different spatial scales of various urban agglomerations.
The meta-frontier DEA method used in this article is also widely employed in calculating the meta-frontier and group-frontier efficiencies of energy consumption and air quality, thus providing strong inspiration for the effectiveness of this method upon urban agglomeration air quality. Chang and Hu (2018) utilize a long-term meta-frontier to analyze energy and emission efficiencies between G7 and BRICS. The results conclude that the United Kingdom, Italy, and France have the smallest overall technology gap, while the largest overall technology gap appears in South Africa, Russia, and the United States Chang et al. (2019) use a two-stage metafrontier DEA approach to evaluate pollution efficiency in Taiwan’s administrative regions. The empirical research shows that New Taipei City and Taipei City have the best performances in terms of MetaFrontier Efficiency and the technology gap ratio. Li et al. (2020) apply a modified two-stage Undesirable Meta Dynamic Network model to jointly analyze energy consumption, economic growth, air pollution, and health treatment data in 31 Chinese cities. Their results show that high-income cities have higher average efficiencies than upper-middle income cities.
Cheng et al. (2019) estimate the environmental efficiency of PM2.5 by the epsilon-based measure (EBM) meta-frontier model. Their findings offer large differences in PM2.5 environmental efficiency between cities and city groups. Wang X. et al. (2019) construct non-parametric evaluation and decomposition models using hybrid measures and meta-frontier techniques. Empirical analyses shows the worst performance is in the BRICS economic group, whereas the developed and developing economies of the G20 group show positive trajectories of performance. Lu et al. (2018) study environmental conservation and sustainable development between Japan’s and Taiwan’s high-tech industries via environmental efficiency by utilizing a one-stage model to measure the performances of decision-making units. Their result presents that Japan’s performance is better than Taiwan’s. Yu et al. (2019) examine the evolution and convergence of China’s ecological efficiency by a metafrontier approach, and their result shows significant regional disparity of eco-efficiency in China. Li et al. (2019) use a MetaFrontier Dynamic SBM model to analyze pollution efficiency in Chinese cities, finding large differences in the energy consumption and air pollution emission efficiency scores and much-needed improvements.
We see from the above literature review that applications of the DEA method in the efficiency evaluation of air quality are relatively mature, but a single DEA model will lead to estimation deviation in the final efficiency value. Although some efficiency evaluation studies combine the DEA model with other methods, the use of other methods is relatively scant, and they tend to predict and infer into the future, and so they are unable to standardize the influencing factors. Therefore, the DEA model of air quality efficiency evaluation needs to be modified and improved.
Many research studies use metafrontier approaches, but they are not new. Different from the common DEA, the metafrontier approach reflects the MFE, GFE, and TGR. However, the metafrontier approach can be better combined with other methods for a more comprehensive empirical result. Furthermore, compared to existing research, due to the different settings of variables and different research purposes, this article chooses the Modified MetaFrontier Dynamic SBM model for air quality efficiency evaluation. Most of the above studies do not distinguish between desirable and undesirable variables in the efficiency evaluation, and DMUs cannot be classified and analyzed, however, the Modified MetaFrontier Dynamic SBM model can solve this problem.
Different Indicators in Air Quality Evaluation
The existing indicators for air quality evaluation, especially efficiency evaluation, can be divided into three categories: Resource Indicators, Pollution Indicators, and Economic Indicators. In this article, Resource Indicators refer to those whose changes in amount may impact air quality, such as labor, economic investment, and energy consumption. Pollution Indicators refer to pollutants related to air quality, such as AQI. Economic Indicators refer to those related to the development of the economy such as GDP and other variables. Most existing research studies select the same Resource Indicators and Economic Variables, but different collocations of Pollution Indicators and other variables appear in such research. Most studies select Pollution Indicators such as CO2 emissions, industrial SO2 emissions, and other variables included in AQI (Aleksandropoulou et al., 2012; Sun et al., 2014, 2018; Feng et al., 2019; Zhao et al., 2019; Yu et al., 2020; Zhang et al., 2020; Zhou et al., 2020; Alemdar et al., 2021). Other scholars set up the selection of indicators according to their own research directions, such as including industrial pollutants produced by industrial agglomeration (Chen et al., 2017; Shen J. et al., 2019; Xu W. B. et al., 2019; Ye et al., 2020), considering the impact of soot and dust produced by energy consumption on air quality (Wang Z.-B. et al., 2017; Shen N. et al., 2019; Wang X. et al., 2019), and taking into account marine spray, mineral dust and human impacts (agricultural activities, combustion processes, and industrial sources), and aerosol components in the evaluation index (Yotova et al., 2016). Due to the different content needs of the respective studies, many researchers re-select and combine the relevant air quality indicators to obtain innovative indicators. Their techniques are different from this article, where we focus on the selection of traditional air quality indicators.
Two problems appear in these selections of current research variables. The first problem is in Pollution Indicators in that the air evaluation indicator form is too simplistic, and a combination of related indicators is lacking. Although AQI is a near-perfect indicator system, most research indicators only focus on AQI. As a comprehensive air quality indicator, AQI can intuitively show the overall air quality of a certain region, but at the same time it has the limitation of not being able to explore the influencing factors of internal pollution. If researchers only study the efficiency evaluation of AQI, then air pollution will not become a target to control.
The second problem is that most studies only evaluate the air pollution variables separately and do not combine the Resource Indicators, Pollution Indicators, and Economic Indicators. If the Pollution Indicators are not combined with the Resource Indicators, then the cause of air pollution cannot be accurately revealed. If the Pollution Indicators are not combined with the Economic Indicators, then the relationship between air pollution and economic growth is not easily found. As a result, most results only skim the surface of air quality, and it becomes difficult to explore the fundamental factors affecting it. Moreover, most studies do not distinguish desirable indicators from undesirable indicators, thus increasing the possibility of confusion over an evaluation system. Therefore, this article combines all three kinds of indicators in the hope of a comprehensive result.
Different Research Objects in Air-Related Evaluation
The existing research targets of air evaluation can be divided into the following four categories: urban agglomeration, single city or area, the whole country, and other independent sample. Many scholars around the world have taken urban agglomerations as research objects to conduct air-related evaluations (Chen et al., 2017; Wang Z.-B. et al., 2017; Czechowski et al., 2019; Shen N. et al., 2019; Xu W. B. et al., 2019; Zhao et al., 2019; Yu et al., 2020; Zhang et al., 2020). Some scholars only take a single city as the research object [Kassomenos et al., 2012 (Thessaloniki); Yotova et al., 2016 (Thessaloniki); Mohammed et al., 2018 (Kut City); Dang et al., 2021 (Beijing and Chengdu)], while others study small areas, such as the Estarreja area (Figueiredo et al., 2013), Rithala areas in India (Mishra et al., 2016), Thessaloniki area (Poupkou et al., 2011; Aleksandropoulou et al., 2012), Taiwan and Japan (Lu et al., 2018), and Taiwan Province (Chang et al., 2019). Other studies take the whole country as the object (Wang and Wang, 2019; Yu et al., 2019; Wang et al., 2020; Zhou et al., 2020). Furthermore, Chang and Hu (2018) set up the G7 and BRICS as research objects, while Qian et al. (2021) target major industrial sectors in China as research objects.
The coordinated development of cities in the form of urban agglomeration is a trend, and the development of cities should no longer be treated in isolation. In addition, air pollution has a certain fluidity, and the air quality between cities affects each other. Differences do exist in the results of air quality problems for various research objects. If only a single city is studied, then the field of vision is narrow. A single city or area as the research object can be observed more carefully, but it is difficult to consider the impact of its surrounding environment. Taking the whole country as the research object incurs the problem of too large a scope. Moreover, it is difficult to focus on micro-level factors while taking the whole country as the research object. Facing the problem of air quality (pollution) of cities in different levels, research on urban agglomerations and city clusters of similar scale needs to be further strengthened. At present, urban agglomeration seems to be a more appropriate research scope.
The main contributions and innovations of this study are as follows. (1) Using the Modified MetaFrontier Dynamic SBM model to study the dynamic changes of urban agglomerations’ air quality evaluation presents an important modification and improvement of the DEA model. (2) Through the distinction between expected and unexpected outputs, the specific classification of evaluation indicators has greatly improved the diversity of index selection. Taking the Employment Population and Energy Consumption per Unit of GDP as input indicators, this study distinguishes GDP as a desirable output as well as Industrial SO2 Emissions, PM2.5, and Industrial Smoke Dust Emissions as undesirable outputs and innovatively introduces Fixed Asset Investment as a carry-over period variable. (3) The Modified MetaFrontier Dynamic SBM model is used to set a common benchmark for the efficiency evaluation of China’s three major urban agglomerations, so that they can be compared with each other, and the efficiency of each city in its own urban agglomerations is further studied by calculating the Group Frontier Efficiency. (4) The evaluation of air quality efficiency for three typical urban agglomerations in China not only extends the existing research, but also has great reference significance for the improvement of air quality, air pollution prevention, and control of world-class urban agglomerations.
Research Methods
Index Model
The Dynamic Data Envelopment Analysis Model
Klopp (1985) first proposes window analysis to analyze efficiency changes between two periods. Färe et al. (1994) then present the Malmquist index (UPI) to analyze a firm’s technical change and efficiency change. However, those researchers do not analyze the impact of carry-over activities during two periods. Subsequently, several DEA dynamic studies appear by Färe and Grosskopf (1996); Bogetoft et al. (2008), Chen (2009); Park and Park (2009). In order to employ carry-over activities as a form of connectivity, Tone and Tsutsui (2010) propose the SBM dynamic DEA model.
Our study designs the methodology based on Tone and Tsutsui’s (2010) assumption and can be described as follows. Suppose there are n DMUs over T terms; each DMU has different input and output during period t, which are then carried over (linked) to the next period (t + 1). The existence of activities is divided into a four-model analysis: (1) desirable (Zgood); (2) undesirable (Zbad); (3) discretionary (Zfree); and (4) non-discretionary (Zfix). The carry-over variable from period t to period t + 1 is guaranteed by:
Equation 2 is the non-oriented model:
The relevant constraints are as follows in Equations 3–12.
The most efficient solution is ρ* in Equation 13.
The Modified MetaFrontier Dynamic Slack-Based Measures Model
Most DEA models usually assume that all DMUs have the same level of technology, but the assessed DMUs are often in different geographical locations or under different national policies, which result in different technology levels. Since this study considers undesirable output and regional differences of the three major urban agglomerations in the dynamic SBM model, we can modify Tone and Tsutsui’s (2010) dynamic SBM model to be the MetaFrontier Dynamic SBM model with undesirable output. The input variables are Employed Population and Energy Consumption per Unit of GDP. The output variables are GDP (desirable), SO2 (undesirable), PM2.5 (undesirable), and Industrial Smoke and Soot (undesirable). The carry-over variable is Fixed Assets. Figure 1 presents the structure of the Modified MetaFrontier Dynamic SBM model used in this study.
MetaFrontier
We assume all units (N) are composed of DMUs in g groups (N = N1 + N2 + ….+ NG); yrj and xij indicate output item r (r = 1, 2, …, s) of item j (j = 1, 2, …, N) and input item i (i = 1, 2, …, m) of item j (j = 1, 2, …, N) under the MetaFrontier, respectively. The MetaFrontier k of DMU efficiency can be solved by the following linear programming (LP):
The relevant constraints are as follows in Equations 15–20.
Using Equations 13, 14, 20, we are able to find the overall technical efficiency (OTE) value of all DMUs under the MetaFrontier model.
Group Frontier
Each DMU under the group frontier chooses the most favorable final output weighted, so that the efficiency of the DMUs under the Group Frontier can be solved by the following Equation 21.
Technology Gap Ratio
Since the MetaFrontier model contains g groups, the technical efficiency of the MFE will be less than the technical efficiency of the GFE. The ratio value, called the TGR, is:
PM2.5, SO2, and Industrial Smoke Dust Emission Efficiency Indices
Labor and energy consumption per GDP are set as inputs in this study. Output variables are GDP, Inhalable Fine Particle Emissions (PM2.5), Sulfur Oxide Emissions (SO2), and Industrial Smoke Dust Emissions. Here, PM2.5, SO2, and Industrial Smoke Dust Emissions are all undesired outputs. Fixed assets are a carry-over variable.
To overcome any possible bias in the traditional energy and environmental efficiency indicators, Hu and Wang (2006) propose the total-factor efficiency index for each specific evaluated object. Referring to this method, we calculate PM2.5, SO2, and Industrial Smoke Dust Emission efficiencies by the following Equations 30–32:
Data Sources and Description
Table 1 lists the input, output, and carry-over variables.
The China government approved the Action Plan For the Prevention and Control of Air Pollution8 in 2013, which marked the establishment of a more comprehensive and perfect air quality indicator system in the country. Since then, PM2.5 has been gradually included for air quality measurement in Chinese cities. Before 2013, only large particles such as PM10, etc., in air were measured. Therefore, 2013 is a meaningful year, and we choose the data span from 2013 to 2017 in this study.
The extensive growth mode adopted by China in the past has resulted in high energy consumption and heavy pollution, and GDP growth has also brought about intensified air pollution. GDP can reflect the relationship between the economy and air pollution in China’s three major urban agglomerations. Therefore, we use GDP together with other air pollutants as output indicators to better assess the impact of economic development on air quality and the correlation between the two.
Definitions of input, output, and carry-over indicators in this study are shown in the Appendix. We use the indicators in the existing research literature for reference and analyze the significance of the results after employing these indicators. The analysis results show good significance. Among these indicators, we note that after the long-term efforts of the China government, SO2 has been effectively controlled and is no longer the main air pollution source domestically. However, SO2 emissions are still a problem worthy of attention in China’s industrial enterprises. Therefore, we still select SO2 as an indicator in our research to make our research more comprehensive.
The research samples are collected from 60 cities in China’s three major urban agglomerations. The relationships between urban agglomerations and cities appear in Table 2. We have made Figure 2 to more display the specific location of each city in the three urban agglomerations.
Statistical Analysis of Input, Output, and Carry-Over Indicators
Overall Analysis
Table 3 shows the maximum and minimum (including related cities) of each indicator in this study. From Table 3, we see that no positive correlation appears between the economic development of individual cities and the emissions of pollutants. For example, Shenzhen, whose economic aggregate ranks first among all cities in the three major urban agglomerations, has the minimum amount of Industrial SO2 Emissions and Industrial Smoke Dust Emissions among all cities (Industrial SO2 Emission, 2017; Industrial Smoke Dust Emission, 2013–2015). Another example is Shanghai, whose GDP ranked first among all cities in 2013–2017, but its pollutant emissions are only in the middle level, reflecting the importance of optimizing the industrial structure for energy conservation and emission reduction.
The data of some cities in BTH are worth paying attention to as well. Beijing’s Energy Consumption per Unit of GDP is the best, ranking first among all cities in 5 years, but Tangshan’s performances in Industrial SO2 Emissions and Industrial Smoke Dust Emissions are the worst among all cities. This may be related to the transfer of the industrial layout from Beijing to surrounding cities in this agglomeration.
Input Indicators’ Analysis
From 2013 to 2017, the average and minimum of Employment Population in each of the three major urban agglomerations in China show a slight upward trend, but the maximum drops sharply in 2016. This closely relates to the significant decrease in the Employed Population in Beijing, the capital city, in 2016 (range of score change: from 1729.076 to 1270.836; variance: 26.50%; period: 2015–2016). Beijing had the largest Employment Population in China during 2013–2015. The average number of Employed Population in China rose by about 20% in 2017 from 2013. Overall, energy consumption conservation and environmental protection face partial upward pressure.
The average, maximum, and minimum Energy Consumption per Unit of GDP of each city show a significant downward trend in 5 years. From the perspective of the maximum Energy Consumption per Unit of GDP, a significant decrease occurs in 2014 compared with the previous year (range of score change: from 1.700 to 1.432; variance: 15.76%; period: 2013–2014), which may relate to the significant increase in energy conservation and emission reduction in Tangshan, the city with the largest indicator in that year. The standard deviation (SD) shows a slight downward trend. In combination with the average value, it indicates that the overall trend of energy conservation and emission reduction in each city is positive.
Output Indicators’ Analysis
The average value and maximum value of GDP in each city in 2013–2017 have increased significantly, which closely relate to the rapid economic development of China in recent years. The rising SD indicates that the economic development of each city is unbalanced and the gap is expanding. This may relate to the suppression effect of economically developed cities upon backward cities by continuously attracting resources from them based on their existing advantages.
Over the 5-year period, the average value of Industrial SO2 Emissions in each city has decreased significantly, indicating that the Industrial SO2 Emission reduction measures in each city have achieved remarkable results. The maximum decreased significantly after 2013 (from 2828.06 to 1254.32, 55.65%, 2013–2016). In the next 2 years, it gradually leveled off, indicating that the governance of Tangshan, the city with the largest Industrial SO2 Emissions, has had a positive effect versus the previous 2 years, but at the same time, the problem of Industrial SO2 Emissions is still severe. The SD also shows a decreasing trend year by year, indicating that the gap of Industrial SO2 Emissions in each city has been narrowing year by year.
The PM2.5 mean value, maximum value, and SD of each city exhibit a similar trend to those of SO2. The maximum value slows down after a significant decrease in 2014. The governance effect of the city with the most serious PM2.5 pollution (Tangshan) for PM2.5 has a great breakthrough in 2013, but falls back in 2014, while the minimum value rebounds after 2015, due to the increase of PM2.5 in the city with the lowest emissions (Huaibei) in the previous period. It is noteworthy that PM2.5 has been gradually included among air quality indicators in cities of China since 2013,9 indicating that the country’s understanding of air quality has gradually improved, and that monitoring technology is also progressing year by year.
The statistical indicators of Industrial Smoke Dust Emissions all peaked in 2014 and gradually decreased in the following 3 years. We can see that the governance effect of Industrial Smoke Dust Emissions in the various cities has improved year by year since 2014, especially in 2017.
Carry-Over Indicator Analysis
Table 3 shows the capital stock of Fixed Asset investment (excluding farmers) in each city of the three major urban agglomerations from 2013 to 2017. As can be seen from the table, the average value increased slightly and steadily, the maximum value increased rapidly from 2013 to 2015 and then decreased, the minimum value increased slightly, and the SD fluctuated slightly. In general, the maximum and minimum of all input, output, and carry-over indicators have a large gap, indicating significant regional differences among the three major urban agglomerations.
Efficiency Scores and Ranking
Overall Efficiency Scores and Ranking
Table 4 shows the overall efficiency score and ranking of air quality in each of the three major urban agglomerations. Among the 57 prefecture-level cities and 3 municipalities directly under the central government in China’s three major urban agglomerations, 5 cities have an efficiency value of 1: Beijing, Tianjin, Shanghai, Guangzhou, and Shenzhen. The output efficiency of energy input in these cities is higher and the air pollution is lower. Beijing and Tianjin belong to BTH, accounting for 15.38% of the total number of BTH. Shanghai belongs to YRD, accounting for 3.13% of the total number of YRD. Guangzhou and Shenzhen belong to PRD, accounting for 13.3% of the total number of PRD. Huaibei, Lu’an, Xuancheng, Fuyang, Yunfu, and Chuzhou, and other cities have low comprehensive efficiency scores (score <0.2), ranking at the tail end and indicating that their energy utilization efficiency is low and their air quality is poor. Measures need to be taken to improve the unreasonable status of input and output and to improve the overall efficiency of energy utilization and air quality level. According to the proportion of the number of cities with a score of 1, the performances of BTH and PRD are better than that of YRD. From the perspective of the number and proportion of cities with a low score (score <0.2), the performance of YRD is worse than that of the other two urban agglomerations.
From the regional perspective as a whole (Figure 3), the change trend of the average efficiency score of the three major urban agglomerations is basically similar. It has risen slowly and fluctuated slightly since the lowest point in 2014. BTH has the best score performance, and its 5-year average efficiency fluctuates within the range of 0.46–0.61, with fluctuation rates of −23.42, 20.25, 1.67, and −2.38%, respectively. This relates to the fact that BTH, as the manufacturing base of China, has a strong industrial foundation. Moreover, BTH includes the locations of China’s political center, cultural center, and economic center, bringing with it more advantages. Although the total efficiency score of BTH is the highest, many heavy industrial cities in Hebei Province (Tangshan, Shijiazhuang, Xingtai, etc.) also rank in the forefront of pollutant emissions. In second place is PRD. Its average efficiency fluctuates within the range of 0.34–0.46, with fluctuation rates of −38.55, 20.33, −1.45, and 9.06%, respectively. The overall trend is the most stable among the three major urban agglomerations. The main reason may be that all cities in PRD are relatively developed coastal cities with similar levels of social and economic development. The performance of YRD is the worst, and its average efficiency fluctuates within the range of 0.26–0.44, with fluctuation rates of −24.56, 16.30, 2.79, and 6.18%, respectively. The main reason for the low average efficiency is that YRD, including less developed inland cities such as those in Anhui Province, is at a disadvantage in energy conservation, emission reduction, industrial development level, and other aspects, thus lowering its overall score.
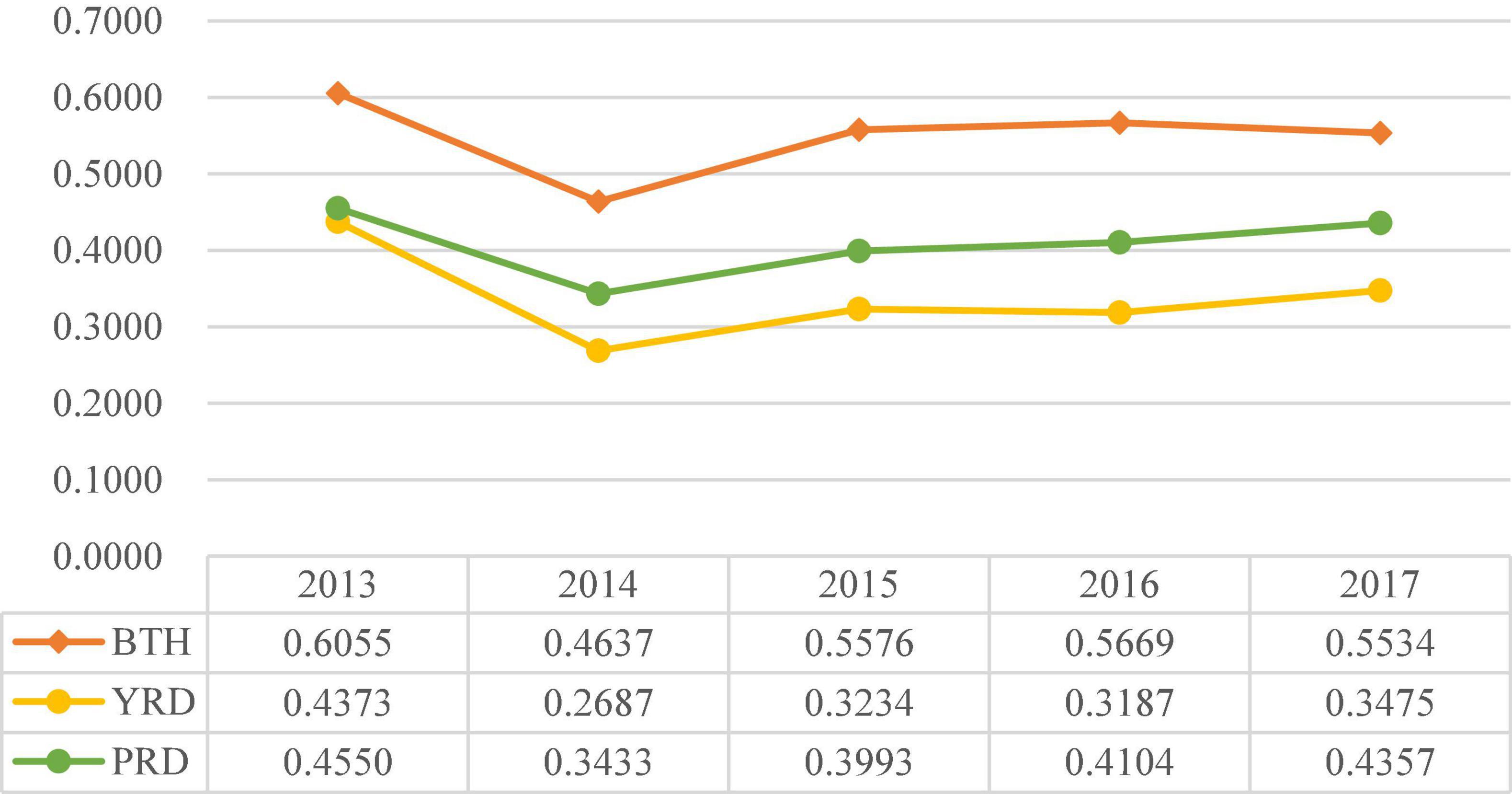
Figure 3. Change of annual average efficiency of China’s three major urban agglomerations, 2013–2017.
From the perspective of the three major urban agglomerations, a large difference appears in the efficiency score of air quality among the cities, but the change trend is generally consistent. Within BTH, only Beijing and Tianjin score a 1 for the five consecutive years; the efficiency scores of Cangzhou and Tangshan decrease from 1 first and then increase back to 1; only Chengde (increases by 0.0225) and Qinhuangdao (increases by 0.0022) show a weak upward trend in efficiency score, while the other cities show a downward trend, with the score of Shijiazhuang dropping the most seriously by −0.3463. Within YRD, except for Shanghai’s efficiency score of 1 for five consecutive years, Bengbu’s performance is more prominent. Its efficiency score increases from 0.6117 at the beginning to 1, increasing by 0.3883 points. Considering that Bengbu’s economic size is small, the improvement of energy conservation and the emission reduction governance effect can be reflected more clearly. Within PRD, Guangzhou and Shenzhen achieve an efficiency score of 1 for 5 consecutive years, while Foshan has the largest growth in the overall efficiency of all cities, with the fastest growth rate of 55.84%. In terms of overall efficiency score, except for Beijing, Tianjin, Shanghai, Guangzhou, and Shenzhen, whose efficiency scores are always 1, other cities experience a sharp drop in efficiency score during 2014.
In terms of city score ranking, no city shows a full upward trend over the 5 years, but Fuyang and Taizhou (in Zhejiang) show a full downward trend in the 5 years. For the overall ranking in 5 years (2017 compared with 2013), those that increase by more than 10 rankings are Qingyuan (23), Zhongshan (22), Qinhuangdao (17), Zhuhai (15), Hengshui (14), Shaoguan (13), Chengde (12), Xingtai (12), and Foshan (11). For the overall ranking in 5 years (2017 compared with 2013), those that decrease by more than 10 rankings are Tongling (21), Anqing (17), Hefei (16), Langfang (15), Huangshan (15), Heyuan (13), and Shijiazhuang (10). The other 44 cities’ rankings fluctuate within 10 positions.
The efficiency scores of each of the three major urban agglomerations in China overall show a trend of fluctuation and decline, with the declining cities accounting for 68.33% of the total number of all cities in the three urban agglomerations. This indicates that the current situation of air quality in China is not optimistic. Further measures are needed to improve governance efficiency and strengthen energy conservation and emission reduction efforts.
Figure 4 shows the cluster analysis spectrum of the average annual efficiency score of the three major urban agglomerations. In the first cluster analysis, the score is used as the variable, the cluster method of inter-group connection is adopted, and the square Euclidean distance is employed as the measurement interval. Cluster analysis automatically divides the efficiency score of each city into four clusters. The first cluster is Beijing, Shanghai, Guangzhou, Shenzhen, Tianjin, Cangzhou, Tangshan, and Shijiazhuang, for a total of eight cities with an average efficiency in the optimal range. Although the total efficiency of Tangshan is in the best range, the sub-efficiency of Industrial SO2 Emissions and Industrial Smoke Dust Emissions is lower, which reflects that Tangshan is at a relative disadvantage in these two indicators. It may be caused by Tangshan being close to Beijing in geographical location and related to Beijing’s industrial transfer to its surrounding cities. The second cluster is Zhoushan, Foshan, and Bengbu or three cities. The third cluster is Wuxi, Suzhou, Hangzhou, Ningbo, and Dongguan, while the remaining 44 cities are in the fourth cluster.
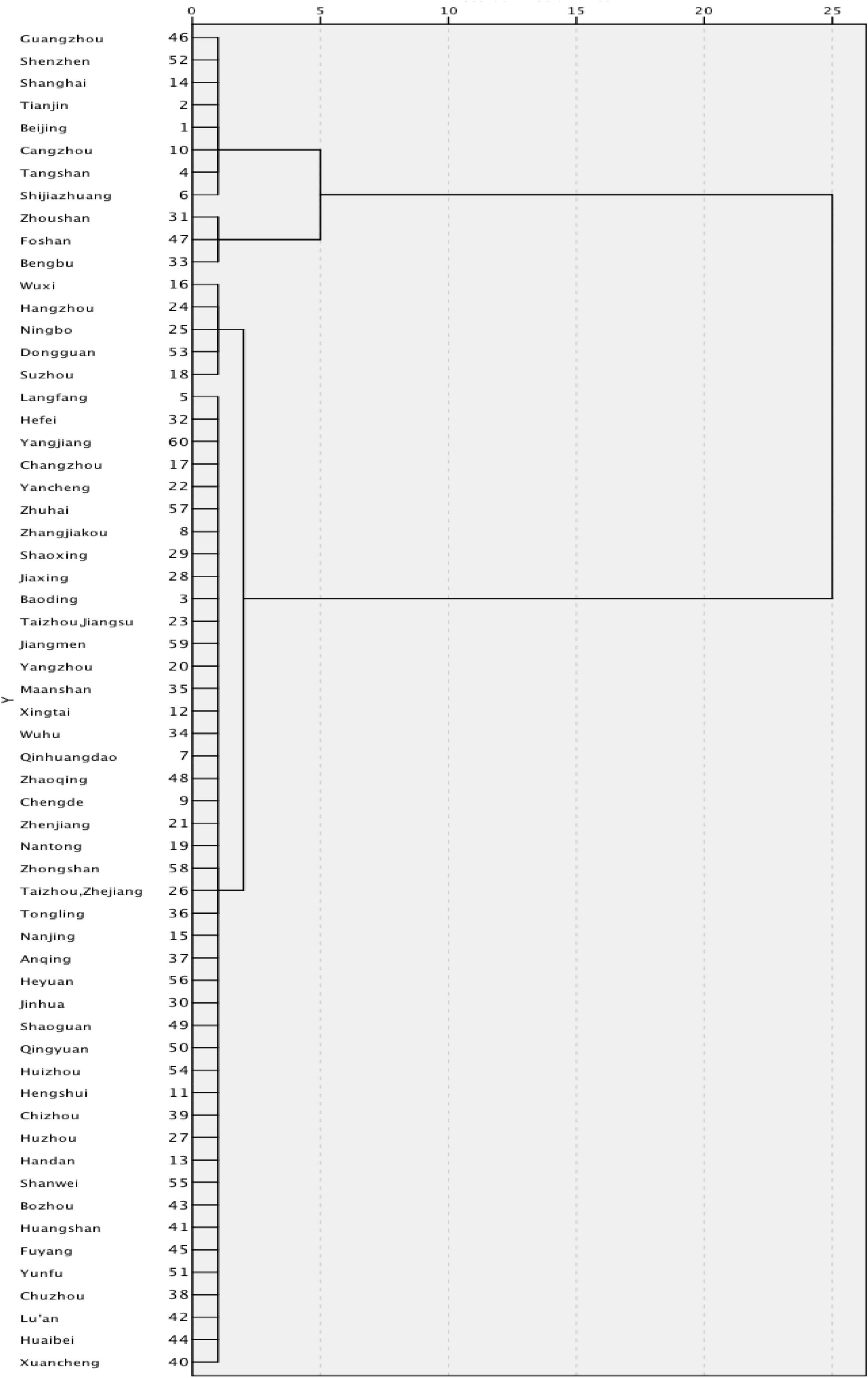
Figure 4. Cluster analysis using average links (group spacing), re-scaled distance clustering combination.
In order to show the specific differentiation of the cluster analysis more clearly, the second cluster analysis is carried out by using K-means. Taking the score as the variable, the iterative classification method is adopted, with the maximum number of iterations of 10 times and manually divided into 2 clusters. The results are as follows. The first cluster has a cluster center of 0.9054 and has 11 cities. The second cluster has a cluster center of 0.2891 and has 49 clusters. The two clusters show a clear, obvious differentiation.
From the results of the two cluster analysis, we see that the polarization of the total efficiency value of each city in the three major urban agglomerations is very significant. The main reason lies in the uneven comprehensive capacity of these agglomerations. Among the three, the mega-cities include Beijing, Shanghai, Shenzhen, and Guangzhou as well as 3- or 4-tier cities such as Lu’an, Xuancheng, and Bengbu. The differences among the three major urban agglomerations are not only reflected in the uneven level of industrial development, but also via the “Matthew effect” brought by different policies and national strategic positioning enjoyed by mega-cities and first-tier cities. The effect of internal polarization is the most obvious, which closely relates to YRD, including the largest number of cities and the largest development level gap between cities.
Analysis of MetaFrontier and Group Frontier Efficiency Scores
Table 5 shows the results of the meta-frontier method. MFE reflects the distance between the actual output of the DMU and the common frontier boundary. GFE reflects the distance between the actual output of the DMU and the frontier boundary. TGR reflects the gap between the group technology level and the potential common frontier technology level of DMUs.
Under the metafrontier, the overall air quality efficiency of China’s three major urban agglomerations is at a low level, and there is a significant gap between YRD, PRD, and BTH. BTH shows the best performance, with an average MTE of 0.5091; YRD and PRD show the worst performance, with an average MTE of 0.3002 and 0.3461, respectively. The efficiency gaps in the three clusters are distinct. By comparing GFE with TGR, we can see cities in YRD have great gaps. Many cities have lower MFE scores and higher GFE scores. It is the same as the above finding, confirming that there is a huge polarization in YRD. Most cities in BTH and PRD have generally higher TGR scores (score >0.8), while more than two-thirds of the cities in YRD have TGR scores that hover between 0.3 and 0.7. Compared to the frontier cities, the cities with lower scores have great room to improve their air quality efficiency.
Analysis of Sub-Index Efficiency
Energy consumption per GDP, Industrial SO2 Emissions (undesirable), Absorptive Fine Particulates – PM2.5 (undesirable), and Industrial Smoke Dust Emissions (undesirable) reflect the actual economic development and air pollution of urban agglomeration, which can be used as the basis for sub-item efficiency evaluation. Table 6 reveals the efficiency scores for each indicator of the three major urban agglomerations from 2013 to 2017. Each urban agglomeration has several core cities, such as Beijing and Tianjin of BTH, Shanghai of YRD, and Guangzhou and Shenzhen of PRD, whose efficiency scores are 1. In addition, there are some sub-level core cities. The efficiency scores of Changzhou, Tangshan, and Shijiazhuang of BTH are almost 1 except for some years. Bengbu in YRD scores 1 except for some indictors in some years. The efficiency score of Foshan in PRD gradually increases from 2013 to 2015 and reaches 1 in 2016–2017. The remaining cities have low efficiency scores and present huge room for improvement.
The polarizations of efficiency scores of energy consumption per GDP and PM2.5 are the most conspicuous. The energy consumption per GDP efficiency scores of cities in BTH are lower than 0.2, except for Beijing, Tianjin, Changzhou, Tangshan, and Shijiazhuang. The PM2.5 efficiency scores of almost 60% of the cities of the three urban agglomerations are below 0.2.
Analysis of Average Scores of Each Index
It can be seen from Figures 5A–D that the efficiency scores of each sub-index of BTH are the best, followed by PRD and YRD. It reveals that, along with economic development, air pollution control of BTH has also strengthened in recent years, but air pollution of YRD is the most serious.
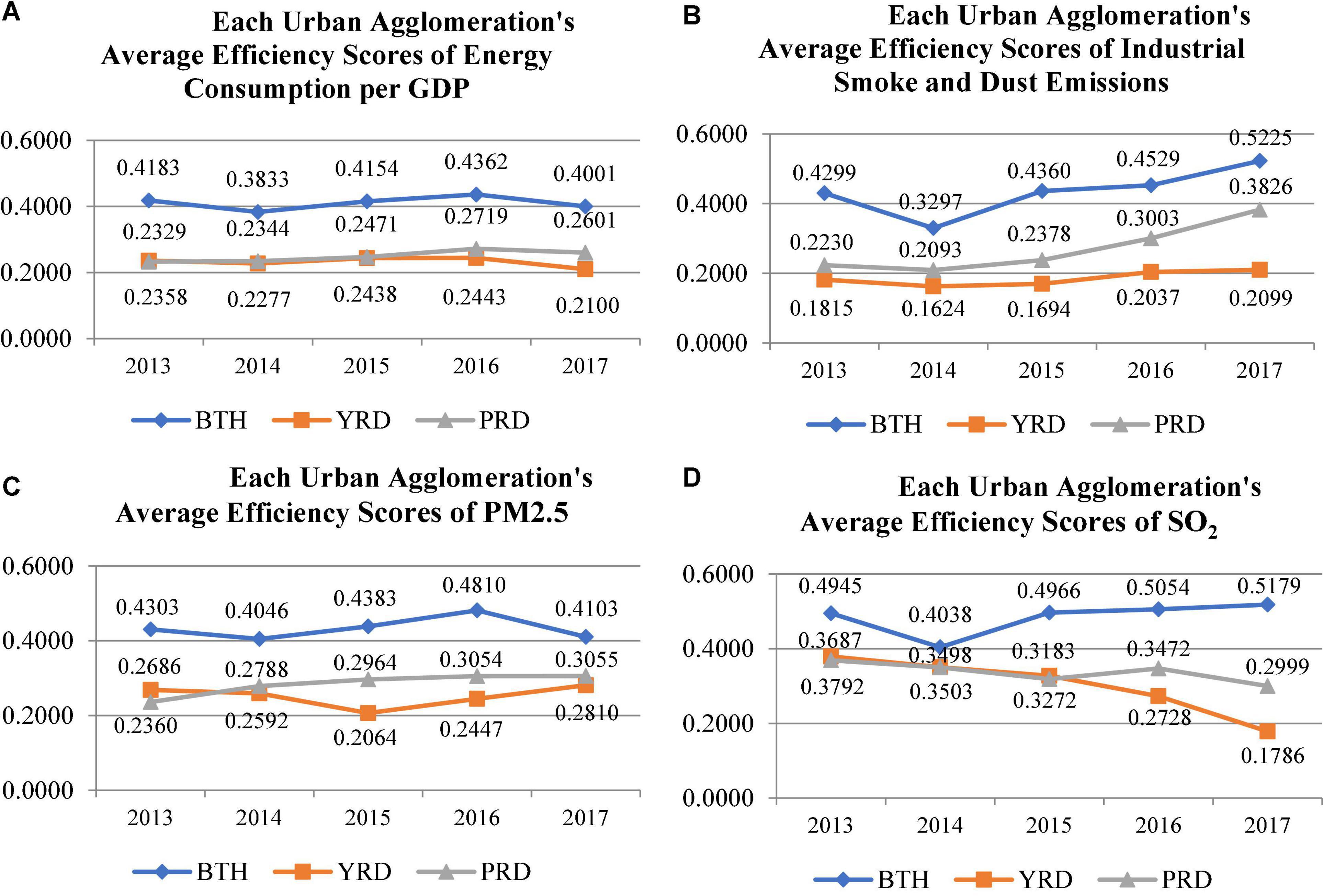
Figure 5. (A–D) Average scores of each index of the three major urban agglomerations. (A) Each urban agglomeration’s average efficiency scores of energy consumption per GDP. (B) Each urban agglomeration’s average efficiency scores of industrial smoke and dust emissions. (C) Each urban agglomeration’s average efficiency scores of PM2.5. (D) Each urban agglomeration’s average efficiency scores of SO2.
In terms of Energy Consumption per Unit GDP, the efficiency scores of the three major urban agglomerations tend to fluctuate around 0.41, 0.23, and 0.25 in BTH, YRD, and PRD, respectively. In terms of SO2, the score of BTH drops to 0.40 in 2014, but increases steadily from 2015 to 2017, with an average annual growth rate of 8.6%, while YRD and PRD show an obvious downward trend, with declines of 34.5 and 13.6% in 2017. In terms of PM2.5, the efficiency scores of BTH and YRD fluctuate around 0.43 and 0.24, respectively. The efficiency score of PRD shows an upward trend, but the growth decreases year by year and is approximately zero by 2017. In terms of industrial smoke and dust emissions, the three major urban agglomerations decrease their amounts in 2014, with a significant drop of 23.3% in BTH. In 2015–2017 they all show an upward trend, with BTH and PRD increasing by 16.6 and 22.3%, respectively, while YRD only rises 8.9%. In combination with Figure 4, the reason why overall efficiency scores decline in 2014 are the reduction of average efficiency scores of SO2 and a drop in industrial smoke and dust emissions.
Analysis of Sub-Index Efficiency
Figures 6A,B show the scores of SO2 and industrial smoke and dust emissions in each city of the three major urban agglomerations. Generally speaking, the score of SO2 is higher than that of PM2.5, and the score of industrial smoke and dust emissions is the lowest. It shows that the three urban agglomerations have some experience at SO2 control, but there is still a lot of room for improvement in the efficiencies of PM2.5 and industrial smoke and dust control.
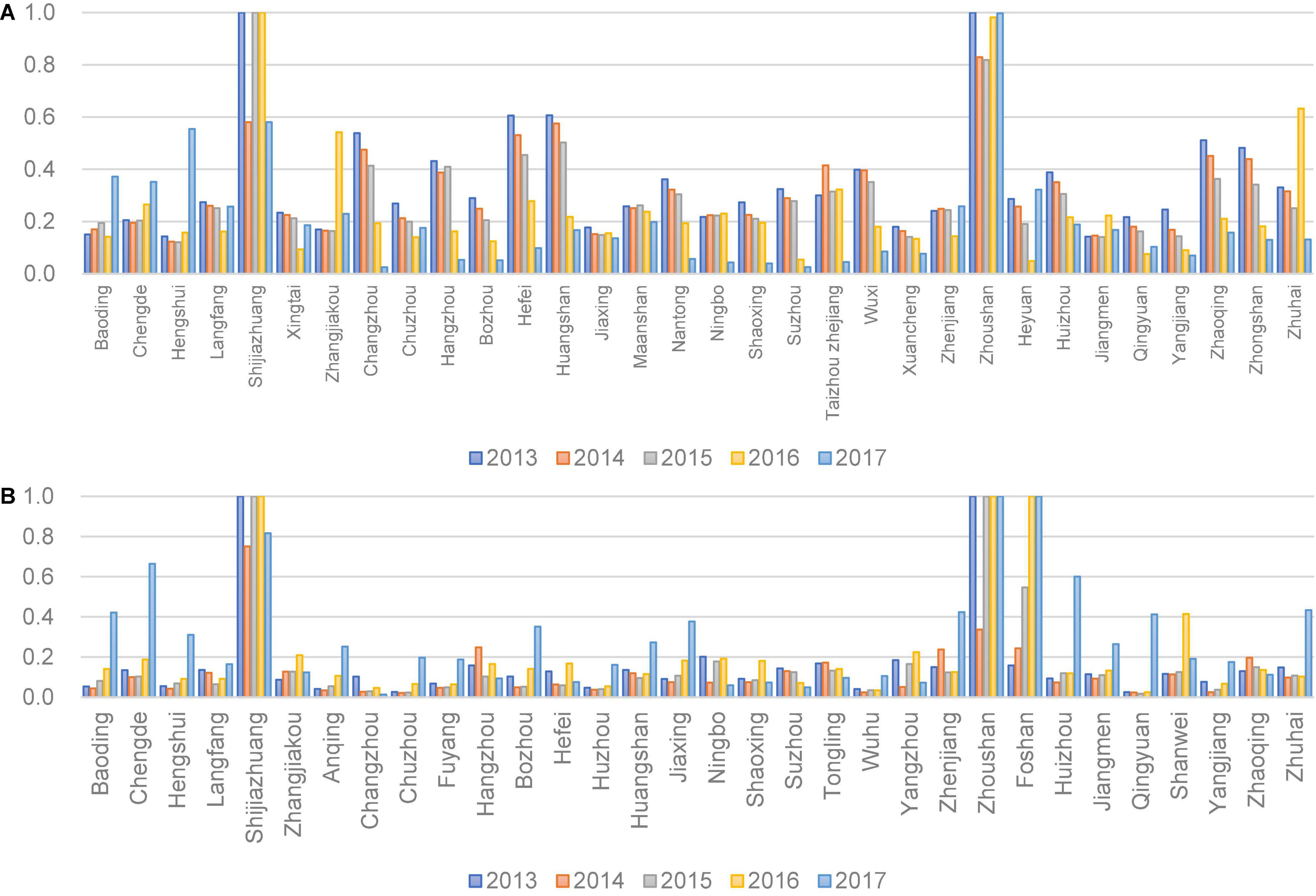
Figure 6. (A) SO2 efficiency scores of typical cities. (B) Industrial smoke and dust emission efficiency scores of typical cities.
From Figure 6A, we note that the polarization of efficiency scores of SO2 is quite conspicuous. The scores of YRD and PRD have an obvious downward trend. Values less than 0.2 cover nearly half of the cities. The distribution of efficiency scores in PRD and YRD is relatively uniform, but the downward trend is obvious, and YRD declines further. In the aspect of SO2 treatment, YRD and PRD have low efficiencies.
From Figure 6B, we can see the industrial smoke and dust emission efficiency scores are low from 2013 to 2015, and cities with low scores account for more than half. The polarization of efficiency scores of industrial smoke and dust emissions is very serious. However, the data from 2016 to 2017 show that the three major urban agglomerations have greatly improved in industrial smoke and dust control. Cities with scores between 0.2 and 0.7 began to appear, thus alleviating the polarization to a certain extent.
As a traditional air pollution index, SO2 has a relatively mature governance system, and the efficiency score is in the middle. However, following economic development, the efficiency score has tended to decrease, which requires more attention and improvement. PM2.5, as a new index proposed in recent years, is not yet mature in terms of prevention and control, but the scores of PM2.5 efficiency tend to be stable, indicating that a series of prevention and control measures taken by the China government have achieved remarkable results.
Table 7 shows the Friedman test score for the average technology gap. In 2013–2017 the average total efficiencies of the three major urban agglomerations of China all pass the significance test.
Discussion and Limitations
Discussion
Comparing our results with previous studies, we find some similarities and differences.
1. From 2006 to 2015, the average static efficiency of total factor air pollution emissions in China’s provinces is relatively low, and the gap between its provinces may continue to widen in the future (Wang X. et al., 2019). This is similar to our conclusion. However, their research focus is on 30 provincial administrative regions in China, which is different from the analysis of 60 specific cities contained in China’s three major urban agglomeration. The low total efficiency of air quality reflects that air pollution improvement by the China government should be further carried down to the city level, and the system mechanism of refinement and grid should be adopted.
2. The atmospheric environmental efficiency (AEE) of BTH, YRD, and PRD is low (Zhang et al., 2020), which is similar to our research conclusion. However, their study shows that the AEE of the three urban agglomerations has been increasing annually. The lowest AEE and pollutant shadow prices are for BTH. In our article, the efficiency scores of the three major urban agglomerations in China overall show a trend of fluctuation and decline, and BTH has the highest efficiency. This may be due to the adoption of different methods. In addition, the feasibility of using a shadow price to regulate efficiency and to promote policies needs to be further verified.
3. No matter for short-term or long-term energy efficiency and CO2 emissions efficiency, China within BRICS has the worst efficiency (Chang and Hu, 2018), which is similar to our research conclusion. However, their research analyzes long-term potential energy savings, potential emission reduction, and long-term technology gaps on energy utilization and CO2 emissions in G7 and BRICS, conducts an overall analysis of carbon emission efficiency from the perspective of energy utilization, and puts forward suggestions. This article focuses on the evaluation and improvement of the air quality of the three major urban agglomerations in China and puts forward suggestions for the specific situation of different urban agglomerations from the perspective of a number of air quality indicators.
4. China has not achieved coordinated development between the environment and economic growth in the past decade (Cheng et al., 2019). This is similar to our conclusion. We find that some cities of the three urban agglomerations, such as Langfang, Ningbo, Wuhu, Tongling, Chizhou, Lu’an, Zhaoqing, Heyuan, and Jiangmen, tend to focus on developing their economy rather than controlling air pollution. With the annual increase of GDP, although the emissions of pollutants have been decreasing year by year, the efficiency of pollution control remains at a low level, even showing a downward trend. This fully means that the economic development and pollution control of these cities cannot be effectively coordinated together.
Limitations
We only research the air quality problems of the three major urban agglomerations in China from their existing air index data and do not consider the specific reasons for the differences in air control efficiency of these agglomerations. In a follow-up study, we shall carry out a specific analysis of the influencing factors of air quality, so as to further explore the reasons for the differences as well as the advantages and disadvantages of air quality between urban agglomerations and within cities.
Conclusion and Recommendation
Conclusion
This study uses the Modified MetaFrontier Dynamic SBM model to evaluate and measure the air quality of 57 prefecture-level cities and 3 municipalities directly under the central government included in the three major urban agglomerations. The empirical results are as follows.
1. The level of air pollution prevention and control in China’s three major urban agglomerations is relatively low, and YRD, where most economic development is taking place, has the worst effect of air pollution prevention and control. Under the metafrontier, BTH shows the best performance, with an average MFE of 0.5091, while YRD and PRD show the worst performance, with an average MFE of 0.3002 and 0.3461, respectively. The average development of YRD’s 5-year total efficiency score illustrates a “W” shape, with an average annual decline of 5.58%. There is still a lot of room to improve the development level of air pollution prevention and control, which still needs policy guidance and technical support.
2. The cities show obvious polarization in air pollution prevention and control. We note that 68.33% of the urban air pollution control level is low and shows a downward trend. In the cluster analysis, all cities are divided into two clusters. The first cluster has a cluster center of 0.9054 and has 11 cities. The second cluster has a cluster center of 0.2891 and has 49 cities. The two clusters show a clear, obvious differentiation. With the implementation of pollution prevention and control policies by the China government, polarization has improved, but remains stagnant.
1. No positive correlation appears between the economic development of individual cities and the emissions of pollutants. The core cities’ GDP come out in front in 2013–2017, but their pollutant emissions are only in the middle level. The overall efficiencies and sub-index efficiencies of the core cities are 1, representing the frontier of the three urban agglomerations.
2. Due to the reduction of SO2 and industrial smoke and dust emission efficiencies, the air quality efficiency of the three urban agglomerations decreased in 2014. After that, with the improvement of industrial smoke and dust efficiency, air quality efficiency gradually improved. The air quality efficiency of the three urban agglomerations shows a V-shape, which is consistent with the sub efficiency-trend of industrial smoke and dust emissions. However, we also note that SO2 efficiency shows a downward trend. The central government should strengthen the control of industrial smoke, dust, and SO2 to reduce emissions and improve the efficiency of air quality control.
Recommendations
Based on the above conclusions, we believe that the central government should formulate different policies according to the different characteristics of the three major urban agglomerations, so as to improve the efficiency of air pollution control. We present some suggestions as follows.
The three major urban agglomerations should promote economic development in order to improve the positive effect of economic growth on reducing emissions. At the same time, the industrial smoke and dust emissions of the three urban agglomerations are very serious and need to be strictly controlled.
Air pollution in BTH is relatively serious. The regional governments of BTH should accelerate the adjustment of industrial structure, vigorously develop a low-carbon economy and a green economy, and reduce air pollution while at the same time developing the overall economy. For prevention and control of air pollution, the governments should improve existing dust removal technology and equipment in order to strictly manage the PM2.5 generated by industrial production and reduce vehicle exhaust emissions to reduce this pollution further.
The polarization of the efficiency scores in YRD is extraordinarily serious. In order to improve the overall economic strength of this region, it is necessary to enhance the infrastructure construction, improve the relationship between cities within the urban agglomeration, and give play to the radiative driving role of core cities and sub-core cities. In terms of air pollution prevention and control, attention should be paid to the treatment of SO2. New and highly efficient desulfurization technology can be developed and vigorously promoted to reduce SO2 emissions. In accordance with the requirements of new technology and environmental quality, emission fees should be raised. At the same time, the use of traditional fossil energy should be cut, and the use of clean energy should be promoted. Moreover, relevant laws and standards can be formulated to regulate the treatment of industrial smoke and dust emissions.
The air quality of PRD is generally good, and so attention should be paid to economic development, but air pollution should still not be ignored. In terms of the economy, the governments there should make full use of scientific and technological innovation and strategic advantages to develop high-tech industries and improve the overall economic development efficiency with a better industrial structure. In terms of air pollution prevention and control, due to the better overall air quality, smaller emission reduction targets can be set up to ensure rapid economic development.
Data Availability Statement
The original contributions presented in the study are included in the article/Supplementary Material, further inquiries can be directed to the corresponding author.
Author Contributions
ZC: conception, visualization, and supervision. F-RR: methodology, software, and funding acquisition. FY: validation, resources, and project administration. ZC and F-RR: formal analysis and writing – review and editing. QW: investigation. ZX: data curation. QW and ZX: writing – original draft preparation. All authors read and contributed to the manuscript.
Funding
This study was supported by the Jiangsu Education Department University Philosophy and Social Science Research Project (2021SJA1589).
Conflict of Interest
The authors declare that the research was conducted in the absence of any commercial or financial relationships that could be construed as a potential conflict of interest.
Publisher’s Note
All claims expressed in this article are solely those of the authors and do not necessarily represent those of their affiliated organizations, or those of the publisher, the editors and the reviewers. Any product that may be evaluated in this article, or claim that may be made by its manufacturer, is not guaranteed or endorsed by the publisher.
Supplementary Material
The Supplementary Material for this article can be found online at: https://www.frontiersin.org/articles/10.3389/fevo.2021.729012/full#supplementary-material
Abbreviations
BTH, Beijing-Tianjin-Hebei; YRD, Yangtze River Delta; PRD, Pearl River Delta; DEA, data envelopment analysis; SBM, slack-based measures; AQI, Air Quality Index; DMU, decision making unit; GDP, gross domestic product; MFE, MetaFrontier Efficiency; GFE, Group Frontier Efficiency; TGR, technology gap ratio.
Footnotes
- ^ New York is the center.
- ^ Chicago is the center.
- ^ Tokyo, Nagoya, and Osaka are the centers.
- ^ London is the center.
- ^ Source: China National Bureau of Statistics, 2017.
- ^ Source: 2010 China Urban Agglomeration Development Report, Science Press (2011), Chuanglin Fang.
- ^ Source: Bulletin on the State of China’s Ecological Environment in 2019, Ministry of Ecological Environment of China.
- ^ Action Plan For the Prevention and Control of Air Pollution, the State Council of the People’s Republic of China, 2013.
- ^ On February 29, 2012, the standing meeting of the State Council of China approved the newly revised ambient air quality standard, officially including PM2.5 into the air quality standard. The meeting required the monitoring of fine particles and ozone in key regions such as Beijing-Tianjin-Hebei, Yangtze River Delta, and Pearl River Delta, as well as municipalities directly under the central government and provincial capital cities in 2012, in 113 key cities for environmental protection and national model cities for environmental protection in 2013, and in all cities above the prefecture level in 2015.
References
Aleksandropoulou, V., Eleftheriadis, K., Diapouli, E., Torseth, K., and Lazaridis, M. (2012). Assessing PM10 source reduction in urban agglomerations for air quality compliance. J. Environ. 14, 266–278. doi: 10.1039/c1em10673b
Alemdar, K. D., Kaya, Ö, Canale, A., Çodur, M. Y., and Campisi, T. (2021). Evaluation of air quality index by spatial analysis depending on vehicle traffic during the COVID-19 outbreak in Turkey. Energies 14:5729.
Bogetoft, P., Christensen, D. L., Damgard, I., Geisler, M., Jakobsen, T. P., Krøigaard, M., et al. (2008). “Multiparty computation goes live,” in Financial Cryptography and Data Security, eds R. Dingledine and P. Golle (Berlin: Springer), 325–343.
Chang, M.-C., and Hu, J.-L. (2018). A long-term meta-frontier analysis of energy and emission efficiencies between G7 and BRICS. Energy Effic. 12, 879–893.
Chang, M.-C., Hu, J.-L., and Chen, C.-H. (2019). A metafrontier pollution efficiency analysis of Taiwan’s administrative regions. J. Clean. Prod. 222, 393–406. doi: 10.1016/j.jclepro.2019.03.046
Chen, C. M. (2009). Network-DEA, a model with new efficiency measures to incorporate the dynamic effect in production networks. Eur. J. Oper. Res. 194, 687–699. doi: 10.1016/j.ejor.2007.12.025
Chen, N., Xu, L., and Chen, Z. (2017). Environmental efficiency analysis of the Yangtze river economic zone using super efficiency data envelopment analysis (SEDEA) and tobit models. Energy 134, 659–671.
Cheng, S., Xie, J., Xiao, D., and Zhang, Y. (2019). Measuring the environmental efficiency and technology gap of PM2.5 in China’s ten city groups: an empirical analysis using the EBM meta-frontier model. Int. J. Environ. Res. Public Health 16:675. doi: 10.3390/ijerph16040675
Czechowski, P. O., Da̧browiecki, P., Oniszczuk-Jastrza̧bek, A., Bielawska, M., Czermański, E., Owczarek, T., et al. (2019). A preliminary attempt at the identification and financial estimation of the negative health effects of urban and industrial air pollution based on the agglomeration of Gdańsk. Sustainability 12:42. doi: 10.3390/su12010042
Dang, Y., Zhang, X., Rao, X., Kang, P., He, J., Lu, N., et al. (2021). Evaluation of air pollution characteristics and air quality improvement effect in Beijing and Chengdu. Environ. Sci. 42, 3622–3632. doi: 10.13227/j.hjkx.202012104
Ding, T., Wu, H. Q., Jia, J. J., Wei, Y. Q., and Liang, L. (2020). Regional assessment of water-energy nexus in China’s industrial sector: an interactive meta-frontier DEA approach. J. Clean. Prod. 244:118797. doi: 10.1016/j.jclepro.2019.118797
Färe, R., and Grosskopf, S. (1996). Productivity and intermediate products: a frontier approach. Econ. Lett. 50, 65–70. doi: 10.1016/0165-1765(95)00729-6
Färe, R., Grosskopf, S., Norris, M., and Zhang, Z. (1994). Productivity growth, technical progress and efficiency change in industrialized countries. Am. Econ. Rev. 84, 66–83.
Feng, D., Li, J., Li, X., and Zhang, Z. (2019). The Effects of Urban sprawl and industrial agglomeration on environmental efficiency: evidence from the Beijing–Tianjin–Hebei Urban agglomeration. Sustainability 11:3042. doi: 10.3390/su11113042
Figueiredo, M. L., Monteiro, A., Lopes, M., Ferreira, J., and Borrego, C. (2013). Air quality assessment of Estarreja, an urban industrialized area, in a coastal region of Portugal. Environ. Monit. Assess. 185, 5847–5860. doi: 10.1007/s10661-012-2989-y
Gottmann, J. (1957). Megalopolis or the urbanization of the Northeastern Seaboard. Urban Plan. Int. 33:189. doi: 10.2307/142307
Halkos, G. E., and Polemis, M. L. (2018). The impact of economic growth on environmental efficiency of the electricity sector: a hybrid window DEA methodology for the USA. J. Environ. Manag. 211, 334–346. doi: 10.1016/j.jenvman.2018.01.067
Hu, J. L., Chang, M. C., and Tsay, H. W. (2018). Disaggregate energy efficiency of regions in Taiwan. Manag. Environ. Qual. 29, 34–48. doi: 10.1108/meq-07-2016-0053
Hu, J. L., and Wang, S. C. (2006). Total-factor energy efficiency of regions in China. Energy Policy 34, 3206–3217. doi: 10.1016/j.enpol.2005.06.015
Jacobs, E. T., Burgess, J. L., and Abbott, M. B. (2018). The donora smog revisited: 70 Years after the event that inspired the clean air act. Am. J. Public Health 108, S85–S88. doi: 10.2105/AJPH.2017.304219
Kassomenos, P. A., Kelessis, A., Petrakakis, M., Zoumakis, N., and Christidis, Th, and Paschalidou, A. K. (2012). Air quality assessment in a heavily polluted urban Mediterranean environment through air quality indices. Ecol. Indic. 18, 259–268. doi: 10.1016/j.ecolind.2011.11.021
Klopp, G. (1985). The Analysis of the Efficiency of Production System with MultiPle InPuts and outPuts. Chicago, IL: University of llinois at Chieago Industrial and Systems Engineering College.
Li, Y., Chiu, Y. H., and Lu, L. C. (2019). Urban energy environment efficiency in China: based on dynamic meta-frontier slack-based measures. J. Air Waste Manag. Assoc. 69, 320–332. doi: 10.1080/10962247.2018.1537942
Li, Y., Lin, T. Y., and Chiu, Y. H. (2020). Dynamic linkages among economic development, environmental pollution and human health in Chinese. Cost Eff. Resour. Alloc. 18:32. doi: 10.1186/s12962-020-00228-6
Lu, Y., Chiu, Y., Chiu, C., and Wang, Y. (2018). Metafrontier analysis of the high-tech industry’s environmental effciency in Japan And Taiwan. Hitotsubashi J. Econ. 59, 9–24.
Mishra, R. K., Shukla, A., and Parida, M. (2016). Air quality assessment along urban transport corridor in megacity. Int. J. Environ. Technol. Manag. 19, 257–267. doi: 10.1504/ijetm.2016.082244
Mohammed, Z., Ziboon, A., Kamal, A., and Alfaraj, M. (2018). Urban air quality evaluation over Kut city using field survey and Geomatic techniques. MATEC Web Conf. 162:05023. doi: 10.1051/matecconf/201816205023
Park, K. S., and Park, K. (2009). Measurement of multiperiod aggregative efficiency. Eur. J. Oper. Res. 193, 567–580. doi: 10.1016/j.ejor.2020.09.044
Polivka, B. J. (2018). The great London Smog of 1952. Am. J. Nurs. 118:57. doi: 10.1097/01.naj.0000532078.72372.c3
Poupkou, A., Nastos, P., Melas, D., and Zerefos, C. (2011). Climatology of discomfort index and air quality index in a large urban mediterranean agglomeration. Water Air Soil Pollut. 222, 163–183. doi: 10.1007/s11270-011-0814-9
Qian, H., Xu, S., Cao, J., Ren, F., Wei, W., Meng, J., et al. (2021). Air pollution reduction and climate co-benefits in China’s industries. Nat. Sustain. 4, 417–425. doi: 10.1038/s41893-020-00669-0
Shen, J., Wang, S., Liu, W., and Chu, J. (2019). Does migration of pollution-intensive industries impact environmental efficiency? Evidence supporting “Pollution haven hypothesis”. J. Environ. Manag. 242, 142–152. doi: 10.1016/j.jenvman.2019.04.072
Shen, N., Deng, R., and Wang, Q. (2019). Influence of agglomeration of manufacturing and the producer service sector on energy efficiency. Polish J. Environ. Stud. 28, 3401–3417. doi: 10.15244/pjoes/94389
Song, M., and Wang, J. (2018). Environmental efficiency evaluation of thermal power generation in China based on a slack-based endogenous directional distance function model. Energy 161, 325–336. doi: 10.1016/j.energy.2018.07.158
Sueyoshi, T., and Yuan, Y. (2015). China’s regional sustainability and diversified resource allocation: DEA environmental assessment on economic development and air pollution. Energy Econ. 49, 239–256. doi: 10.1016/j.eneco.2015.01.024
Sueyoshi, T., Yuan, Y., and Goto, M. (2017). A literature study for DEA applied to energy and environment. Energy Econ. 62, 104–124. doi: 10.1016/j.eneco.2016.11.006
Sun, X.-W., Guo, X.-R., and Cheng, S.-Y. (2018). Impact of the electric power industry on air quality in winter of urban agglomerations along the middle reaches of the Yangtze River. Environ. Sci. 39, 3476–3484. doi: 10.13227/j.hjkx.201711186
Sun, Y., Zhang, J., Pan, Y., Wang, Y., Liao, T., and Song, T. (2014). Observations of air quality on the outskirts of an urban agglomeration during the implementation of pollution reduction measures. Atmos. Pollut. Res. 5, 789–795. doi: 10.5094/apr.2014.088
Tone, K. (2003). Dealing with Undesirable Outputs in DEA: A Slacks-Based Measure (SBM) Approach. GRIPS Research Report Series. Toronto, ON: North American Productivity Workshop.
Tone, K., and Tsutsui, M. (2010). Dynamic DEA: a Slacks-based measure approach. Omega 38, 145–156. doi: 10.1016/j.omega.2009.07.003
Wang, K., Yu, S., and Zhang, W. (2013). China’s regional energy and environmental efficiency: a DEA window analysis based dynamic evaluation. Math. Comput. Model. 58, 1117–1127. doi: 10.1016/j.mcm.2011.11.067
Wang, K. L., Ding, L. L., Wang, J. M., and Yang, M. (2020). Analysis of provincial total-factor air pollution efficiency in China by using context-dependent slacks-based measure considering undesirable outputs. Nat. Hazards 104, 1899–1921. doi: 10.1007/s11069-020-04253-9
Wang, K.-L., Miao, Z., Zhao, M.-S., Miao, C.-L., and Wang, Q.-W. (2019). China’s provincial total-factor air pollution emission efficiency evaluation, dynamic evolution and influencing factors. Ecol. Indic. 107:105578. doi: 10.1016/j.ecolind.2019.105578
Wang, X., Shao, Q., Nathwani, J., and Zhou, Q. (2019). Measuring wellbeing performance of carbon emissions using hybrid measure and meta-frontier techniques: empirical tests for G20 countries and implications for China. J. Clean. Prod. 237:117758. doi: 10.1016/j.jclepro.2019.117758
Wang, Y., Liu, H., Mao, G., Zuo, J., and Ma, J. (2017). Inter-regional and sectoral linkage analysis of air pollution in Beijing–Tianjin–Hebei (Jing-Jin-Ji) urban agglomeration of China. J. Clean. Prod. 165:1436–1444. doi: 10.1016/j.jclepro.2017.07.210
Wang, Y., and Wang, J. (2019). Does industrial agglomeration facilitate environmental performance: new evidence from urban China? J. Environ. Manag. 248:109244. doi: 10.1016/j.jenvman.2019.07.015
Wang, Z.-B., Liang, L.-W., Lin, X.-B., and Liu, H.-M. (2017). Control Models and effect evaluation of air pollution in Jing-Jin-Ji Urban Agglomeration. Environ. Sci. 38, 4005–4014. doi: 10.13227/j.hjkx.201701007
Xu, L., Chen, G., Wiedmann, T., Wang, Y., Geschke, A., and Shi, L. (2019). Supply-side carbon accounting and mitigation analysis for Beijing-Tianjin-Hebei urban agglomeration in China. J. Environ. Manag. 248:109243. doi: 10.1016/j.jenvman.2019.07.014
Xu, W. B., Zhang, X. H., Wang, X. S., and Zhang, B. A. (2019). Assessing the efficiency of JING-JIN-JI collaborative development in different low carbon economy strategies around Xiongan with SD and fractal model. Ecol. Environ. Res. 17, 11835–11849.
Ye, F. F., Yang, L. H., and Wang, Y. M. (2020). An interval efficiency evaluation model for air pollution management based on indicators integration and different perspectives. J. Clean. Prod. 245:118945. doi: 10.1016/j.jclepro.2019.118945
Yotova, G. I., Tsitouridou, R., Tsakovski, S. L., and Simeonov, V. D. (2016). Urban air quality assessment using monitoring data of fractionized aerosol samples, chemometrics and meteorological conditions. J. Environ. Sci. Health A Tox. Hazard Subst. Environ. Eng. 51, 544–552. doi: 10.1080/10934529.2016.1141620
Yu, X., Wu, Z., Zheng, H., Li, M., and Tan, T. (2020). How urban agglomeration improve the emission efficiency ? A spatial econometric analysis of the Yangtze River Delta urban agglomeration in China. J. Environ. Manag. 260:110061. doi: 10.1016/j.jenvman.2019.110061
Yu, Y., Hu, H., Zhang, Y., and Yin, Z. (2019). Metafrontier eco-efficiency and its convergence analysis for China: a multidimensional heterogeneity perspective. Emerg. Mark. Financ. Trade 55, 1531–1549. doi: 10.1080/1540496x.2018.1559142
Zaim, O. (2004). Measuring environmental performance of state manufacturing through changes in pollution intensities: a DEA framework. Ecol. Econ. 48, 37–47. doi: 10.1016/j.ecolecon.2003.08.003
Zhang, N., and Wu, Y. and Choi, Y. (2020). Is it feasible for China to enhance its air quality in terms of the efficiency and the regulatory cost of air pollution? Sci. Total Environ. 709:136149. doi: 10.1016/j.scitotenv.2019.136149
Zhao, P., Tuygun, G. T., Li, B., Liu, J., Yuan, L., Luo, Y., et al. (2019). The effect of environmental regulations on air quality: a long-term trend analysis of INDUSTRIAL SO2 EMISSION and NO2 in the largest urban agglomeration in southwest China. Atmos. Pollut. Res. 10, 2030–2039. doi: 10.1016/j.apr.2019.09.011
Zhou, Z., Chen, Y., Song, P., and Ding, T. (2020). China’s urban air quality evaluation with streaming data: a DEA window analysis. Sci. Total Environ. 727:138213. doi: 10.1016/j.scitotenv.2020.138213
Zhou, Z., Guo, X., Wu, H., and Yu, J. (2018). Evaluating air quality in China based on daily data: application of integer data envelopment analysis. J. Clean. Prod. 198, 304–311. doi: 10.1016/j.jclepro.2018.06.180
Appendix
Input variables:
Employed Population: Employed Population refers to the population within a certain age range, with labor capacity, engaged in certain social labor, and obtaining labor remuneration or operating income. In China, it specifically refers to the population aged 16 and above. This study uses the number of employees in each city each year as one of the input variables. Unit: persons.
Energy Consumption per Unit of GDP: According to the interpretation of energy consumption indicators by the National Bureau of Statistics of China, total energy consumption refers to the synthesis of various energy consumed in a certain period of time by each city’s national economy, which includes various industries and resident families. It is mainly calculated by compiling the energy balance table, which is divided according to the regional energy consumption. China’s energy includes primary energy, secondary energy, other fossil, renewable energy, and new energy. Primary energy mainly includes raw coal, crude oil, natural gas, hydro energy, nuclear energy, wind energy, solar energy, geothermal energy, biomass, etc., including petroleum energy consumed in the transportation process, but excluding energy fuels in the power industry. Energy Consumption per Unit of GDP is the main indicator reflecting the level of energy consumption, energy conservation, and consumption reduction. The ratio of total primary energy consumption to GDP is an indicator of energy utilization efficiency. This indicator shows the utilization degree of energy in a country’s economic activities, reflecting the changes of economic structure and energy utilization efficiency. Energy Consumption per Unit of GDP in this study is calculated from the ratio of total primary energy consumption to GDP in each city each year. Unit: 1 tce per 10,000 RMB, where tce is tons of standard coal equivalent.
Output variables:
GDP (Desirable): GDP refers to the final result of production activities of all resident units in a country (or region) in a certain period of time calculated according to the national market price, which is generally recognized as the best indicator to measure the national economic situation. GDP is an important comprehensive statistical index in an accounting system and also the core index in the new national economic accounting system. GDP in this study is the annual gross domestic product of each city. Unit: 10 billion RMB.
Industrial SO2 Emissions (Undesirable): Sulfur dioxide pollution sources include the combustion of sulfur-containing fuel (such as coal and oil), the combustion and emission of hydrogen sulfide in oil and gas well operations, smelting of sulfur-containing ore (especially non-ferrous metal ore with more sulfur), and production processes of the chemical industry, oil refining, sulfuric acid plant, etc. According to the Ecological Environment Statistical Investigation System and National Economic Industry Classification issued by the National Bureau of Statistics of China, the industries involved in sulfur dioxide emissions from industrial sources mainly include all industrial enterprises in three categories: mining, manufacturing, and production and supply of electricity, heat, gas, and power, excluding various transportation industries. In the statistical process, the statistical departments at all levels shall publish, fill in and summarize the key investigation units one by one, and make an overall estimation of the pollution discharge of non-key investigation units. Different industries have different calculation formulae for sulfur dioxide emissions. The total industrial sulfur dioxide emissions of a city are obtained by integrating the sulfur dioxide emissions of different industries. The SO2 indicator is each city’s annual Industrial SO2 Emission data. Unit: 100 tons.
Absorptive Fine Particulates – PM2.5 (Undesirable): The data of PM2.5 come from multiple environmental detection stations in each city – that is, the monitoring values of multiple sampling points. The environmental monitoring station mainly uses conical element oscillating microbalance, β X-ray, and other methods to measure PM2.5 (μg/m3). The calculation of PM2.5 mean value is from the mean value of the data of all monitoring points in a certain period of time in each city, then summing the mean value of each period, then calculating the mean value, and finally taking the average value of multiple sampling points of PM2.5. PM2.5 refers to particles with an aerodynamic equivalent diameter of 2.5 microns or less in ambient air. They have a small particle size, large surface area, strong activity, can easily be attached to toxic and harmful substances (such as heavy metals, microorganisms, etc.), spend a long time in the atmosphere, and can cover a long transportation distance. Thus, they have a greater impact on human health and atmospheric environment quality. The PM2.5 indicator in this study is the annual average concentration of PM2.5 in air in each city. Unit: μg/m3.
Industrial Smoke Dust Emissions (Undesirable): The variable is the annual industrial smoke dust emissions of each city. Industrial smoke dust may contain toxic metal dust and non-metal dust (chromium, manganese, cadmium, lead, mercury, arsenic, etc.). According to the interpretation of main statistical indicators in China’s National Statistical Yearbook, industrial smoke emissions are the sum of the total mass of particles entrained in the flue gas discharged into the atmosphere by industrial source industries in each city during fuel combustion and the production process and solid particles that can be suspended in the air for a certain time. The industries included are consistent with those in the industrial sulfur dioxide emission index. Unit: 100 tons.
Carry-over variable:
Fixed Assets (Capital Stock): Investment in fixed assets is the workload of the activities of building and purchasing fixed assets in the form of currency. This study calculates the Fixed Asset investment of each city each year (excluding peasants households’ investment). Unit: 10 billion yuan RMB.
Keywords: urban agglomeration, air quality, efficiency evaluation, Modified MetaFrontier Dynamic SBM model, PM2.5
Citation: Cui Z, Yang F, Ren F-r, Wei Q and Xi Z (2021) Air Quality Evaluation and Improvement of China’s Three Major Urban Agglomerations Based on the Modified MetaFrontier Dynamic Slack-Based Measures Model. Front. Ecol. Evol. 9:729012. doi: 10.3389/fevo.2021.729012
Received: 22 June 2021; Accepted: 12 November 2021;
Published: 09 December 2021.
Edited by:
Xunpeng (Roc) Shi, University of Technology Sydney, AustraliaReviewed by:
Richard W. Baldauf, United States Environmental Protection Agency (EPA), United StatesChangbo Wang, Nanjing University of Aeronautics and Astronautics, China
Copyright © 2021 Cui, Yang, Ren, Wei and Xi. This is an open-access article distributed under the terms of the Creative Commons Attribution License (CC BY). The use, distribution or reproduction in other forums is permitted, provided the original author(s) and the copyright owner(s) are credited and that the original publication in this journal is cited, in accordance with accepted academic practice. No use, distribution or reproduction is permitted which does not comply with these terms.
*Correspondence: Fang-rong Ren, MTgwMjEzMTIwMDA4QGhodS5lZHUuY24=