- 1Chair of Silviculture, Institute of Forest Sciences, University of Freiburg, Freiburg, Germany
- 2Amt für Archäologie, Kanton Thurgau, Frauenfeld, Switzerland
- 3Chair of Forest History, Institute of Forest Sciences, University of Freiburg, Freiburg, Germany
- 4Chair of Forest Growth and Dendroecology, Institute of Forest Sciences, University of Freiburg, Freiburg, Germany
Tree-ring widths (TRW) of historical and archeological wood provide crucial proxies, frequently used for high-resolution multi-millennial paleoclimate reconstructions. Former growing conditions of the utilized trees, however, are largely unknown. Potential influences of historical forest management practices on climatic information, derived from TRW variability need to be considered but have not been assessed so far. Here, we examined the suitability of TRW series from traditionally managed oak forests (Quercus spp.) for climate reconstructions. We compared the climate signal in TRW chronologies of trees originating from high forests and coppice-with-standards (CWS) forests, a silvicultural management practice widely used in Europe for most of the common era. We expected a less distinct climate control in CWS due to management-induced growth patterns, yet an improved climate-growth relationship with TRW data from conventionally managed high forests. CWS tree rings showed considerably weaker correlations with hydroclimatic variables than non-CWS trees. The greatest potential for hydroclimate reconstructions was found for a large dataset containing both CWS and non-CWS trees, randomly collected from lumber yards, resembling the randomness in sources of historical material. Our results imply that growth patterns induced by management interventions can dampen climate signals in TRW chronologies. However, their impact can be minimized in well replicated, randomly sampled regional chronologies.
Introduction
Medieval societies in Europe developed the “Coppice-with-standards” (CWS) silvicultural system to ensure a sustainable supply with fuelwood and timber for the growing population. This two-story forest structure combines an understory of even-aged coppice harvested in short rotation for fuelwood and tanning with an uneven-aged partial upper story of standard trees growing at wide spacing for timber production (Mosandl et al., 2010). The earliest historical evidence for this practice dates back to the thirteenth century CE (Hausrath, 1982; Hasel and Schwartz, 2006). Dendroarcheological evidence for CWS management in central Europe during the first millennium CE suggests an even longer tradition, at least since the early medieval period (i.e., 500–1000 CE) (Muigg et al., 2020b). The CWS forest management system was used all over medieval and early modern Europe (Short and Hawe, 2012) until it disappeared in most regions by the mid-twentieth century, when fossil fuels had replaced wood as the primary energy source and modern forestry focused primarily on timber production (Groß and Konold, 2010). A small region in southern Germany (Franconia) (Figures 1A,B) provides one of the few places left in central Europe, where traditional CWS forest stands are still maintained (Albrecht and Abt, 2014).
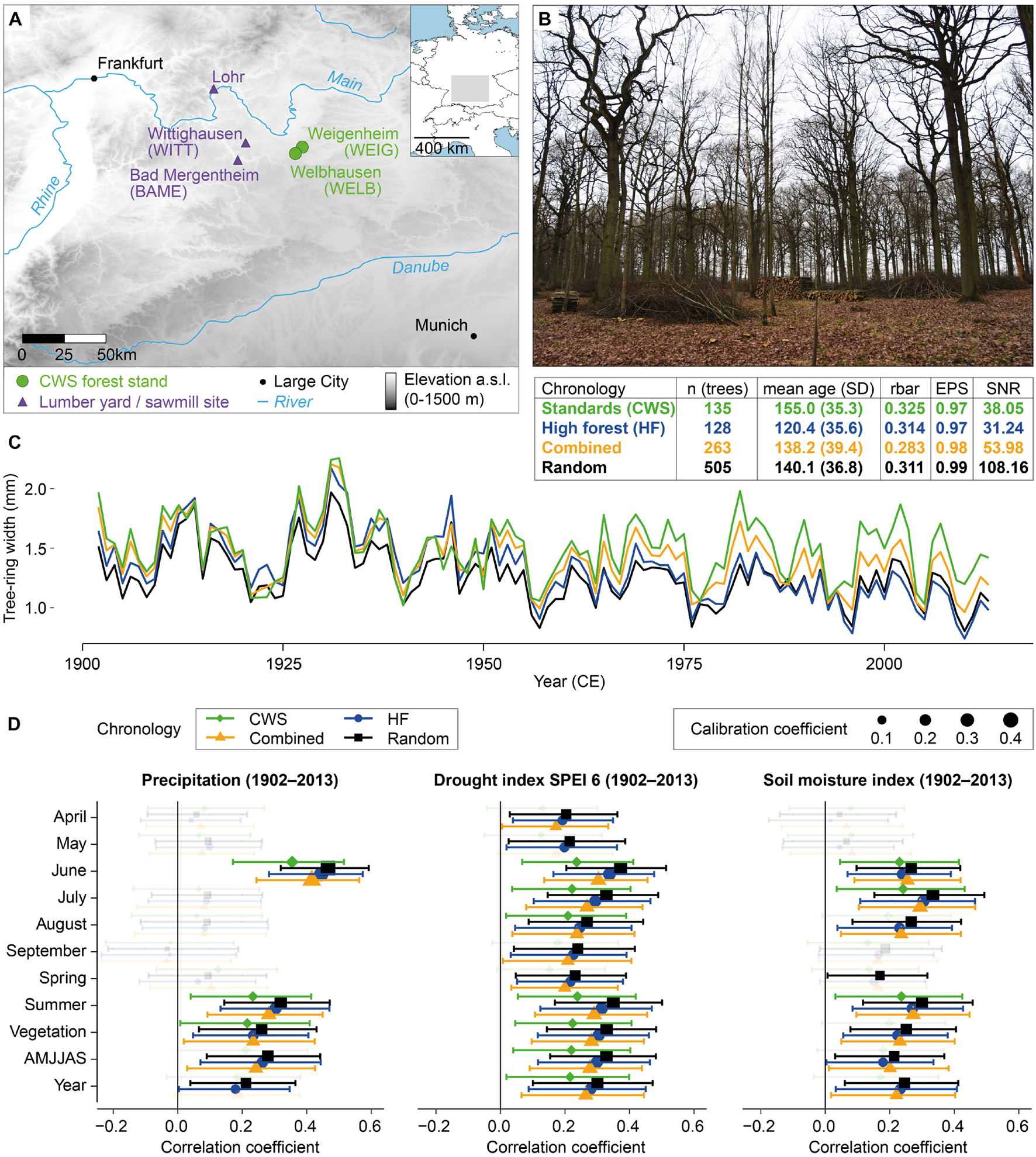
Figure 1. (A) Location of sampled forest stands for coppice-with-standards (CWS; green) and lumber yards/sawmills (purple). (B) Example of CWS forest structure in Welbhausen (during understory coppice in 2018). (C) Average tree-ring chronologies (raw TRW) and statistics (table) on the detrended tree-ring series. mean age in years; SD, standard deviation; rbar, mean correlation between individual tree series; EPS, expressed population signal and SNR, signal to noise ratio. (D) Climate-growth correlations for all detrended chronologies (using a 30-year spline with a 50% frequency cut off after removing autocorrelation) with hydroclimate parameters for the period 1902–2013. Bars denote upper and lower confidence intervals. Transparent points and bars indicate non-significant correlations. CWS: coppice with standards, HF: high forests, combined: dataset containing both the CWS and HF datasets, random: the random dataset of oak tree ring series collected from lumber yards and a veneer company in the region.
The long tradition of CWS forest management in Europe led to various studies from the fields of forest history and dendroarchaeology (e.g., Bernard et al., 2014; Szabó et al., 2015; Vandekerkhove et al., 2016). As wood samples originating from archeological excavations or historical constructions lack information on site and stand conditions, it is likely that, across Europe, a substantial part of oaks originates from CWS managed forests.
Historical tree-ring chronologies are unique archives of past climate variability and as such are frequently used for multi-centennial to multi-millennial paleoclimate reconstructions with annual resolution (e.g., Büntgen et al., 2011; Tegel et al., 2020). However, forest management interventions that might influence tree growth and its sensitivity to climate variability are typically unknown for such samples. So far, possible influences of management-induced alterations in tree-growth patterns on the suitability of CWS standards for reconstructing climate have not been studied. The aim of this study was to compare climate-growth relationships in CWS standards and trees from nearby high forests (HF) (forests consisting mainly of large, tall mature trees with a closed canopy), to critically evaluate their potential for climate reconstructions. We expected a weaker climate signal in CWS standards compared to oak trees from HF. However, we hypothesized that the hydroclimatic signal of a well replicated regional chronology would not be severely impacted by management-induced growth patterns present in a low proportion of tree-ring series.
Methods
Study Design and Development of Chronologies
Four datasets of oak [Quercus robur L. and Quercus petraea (Matt.) Liebl.] TRW series were utilized for the purposes of this study (Figure 1A), comprising (a) oak standards from two actively managed CWS forests (CWS dataset), (b) TRW series of oaks, originating from high forests (HF dataset), (c) a dataset containing both previous (CWS and HF) datasets (combined dataset), and (d) a larger dataset of randomly sampled oak trees (random dataset) (see also Supplementary Figure S2).
The CWS dataset consisted of TRW series from northern Bavaria (Germany), sampled by Muigg et al. (2020b). Stem disks were collected from two actively managed CWS forest stands near Weigenheim (WEIG) and Welbhausen (WELB). To detect signals of CWS in the individual TRW series, we first detected growth releases by using the adapted growth averaging method as described by Muigg et al. (2020b). Following the methods proposed in the study by Muigg et al. (2020b), we applied a standardized scanning for growth releases at an average chronological interval of 26–36 years with less than 5 years standard deviation (SD) on the TRW data from WEIG and WELB to select 135 trees that showed the strongest CWS signal (hereinafter referred to as CWS dataset).
The rest of the datasets (HF, combined and random) that were used here for comparison consisted entirely (or partly in the case of the combined dataset) of oak trees (505 trees in total) from nearby lumber yards in Bad Mergentheim (BAME; 210 individuals) and Wittighausen (WITT; 233 individuals) and a veneer company in Lohr (62 samples). The stem disks (CWS, HF, combined and random) used in this study were collected close to the lower crown height following the random sampling approach (Tegel et al., 2010). The HF dataset consisted only of those trees (128 in total) that did not show cyclic and temporally aligned growth release events (cf. Muigg et al., 2020b) and therefore presumably originated from high forests. Additionally, we merged the CWS and HF datasets (combined dataset) to assess the hydroclimate signal of a hypothetical chronology containing a relatively large proportion of trees (ca. 50% in this case) with periodic management-induced growth patterns. Finally, we assembled a “random dataset” (see Tegel et al., 2010) by merging the full TRW datasets of WITT, BAME, and Lohr (in total 505 oaks of unknown management) to simulate the random composition of samples from archeological and historical material (Figure 1A).
All samples were prepared with standard dendrochronological methods (Speer, 2010). TRW was measured with a precision of 0.01 mm using a movable object table (Megatron) and recorded using the program Berlin Muehle 4 1.0 (developed by Tobias Heussner). The TRW series were crossdated with PAST software (Knibbe, 2008). Three different detrending methods were tested on the combined dataset after removing auto-correlation (AR), including a 30-year and a 100-year spline (Cook and Peters, 1981; Büntgen et al., 2012) and a modified negative exponential model. Finally, four average TRW chronologies were developed by using the bi-weight robust mean (Supplementary Figure S1). The datasets used in this study include oaks with individual tree ages over 200 years. However, we limited our analyses to the period 1902–2013 due to the availability of instrumental climate data for the study region (starting in 1902) and the availability of tree ring records (ending in 2013 for the WITT, BAME, and Lohr datasets).
The quality of the developed chronologies (Figure 1C for the 30-year spline) as well as the relationships between hydroclimate variables and growth (Supplementary Figure S1) were tested on the raw TRW data and on different detrending methods after removing auto-correlation. The strongest correlations with hydroclimate variables were obtained when applying a 30-year cubic spline detrending. Hence, this detrending method was chosen for further analyses. The quality of the detrended chronologies was assessed by calculating the descriptive statistics EPS (expressed population signal), rbar (mean interseries correlation) and SNR (signal to noise ratio). EPS is an indicator of how well a chronology represents a theoretical infinite population (Wigley et al., 1984) and is used as an indicator of common variability in detrended tree-ring chronologies. Rbar is the mean correlation between series within a chronology and indicates the common signal strength in chronologies (Speer, 2010). Finally, SNR is a measure of the desired signal in each chronology versus the amount of unwanted information and random variation (Speer, 2010). All four chronologies displayed high EPS, ranging from 0.97 for the CWS and HF chronologies, 0.98 for the combined to 0.99 for the random chronology. Rbar was found to be lowest for the combined (0.283) and highest for the CWS (0.325) chronology.
Climate, Soil Moisture Content, and Drought Indices
We obtained gridded monthly temperature and precipitation data for the study region from the Royal Netherlands Meteorological Institute (KNMI) climate explorer,1 which were available at a 0.5 × 0.5o resolution (CRU TS.03). Based on these data, we calculated the Standardized Precipitation Evapotranspiration Index (SPEI) using the SPEI package in R (Vicente-Serrano et al., 2010). Although we calculated different accumulation periods of the SPEI index (1, 3, 6, and 12 months) we selected the SPEI index calculated over an accumulation period of 6 months since it was found to correlate best with growth of oaks in the region. In addition, we acquired monthly data of modeled soil moisture content in the upper 100 cm of the soil from the NOAA-CIRES-DOE Twentieth Century Reanalysis V3 datasets (Slivinski et al., 2019). Twentieth Century Reanalysis data accessed by the NOAA/OAR/ESRL PSL, Boulder, Colorado, United States, from their Website on 14/3/2021. All the hydroclimatic variables used in the study were available for the period 1902–2013.
Climate-Growth Relationships and Evaluation of Reconstruction Skills
Hydro-climate sensitivity of the four developed chronologies was assessed by computing bootstrapped Pearson correlation functions between the detrended chronologies and monthly as well as seasonal means for all hydroclimatic variables (average monthly temperature, monthly precipitation sums, SPEI-6 and monthly soil moisture index). Correlation functions were computed for the individual months from April to September and seasonal means for spring (March–May), summer (June–August), as well as for the vegetation period (March–September), the period between April and September (AMJJAS) and the whole year. This analysis was performed for the period between the years 1902 and 2013 for which all the hydroclimatic variables were available. Once the strongest relationship between hydro-climatic variables and oak growth in the region was identified, we applied the commonly used split period (early and late, 1902–1957 and 1958–2013, respectively) ordinary least squares (OLS) regression approach to calibrate the reconstructions. The strength of the relationship between reconstructed and observed values was evaluated by the reduction of error (RE) and coefficient of efficiency (CE), with positive values for these statistics indicating that the reconstruction contains useful information (Fritts, 1976; Cook et al., 1994). The uncertainty in the reconstructions was assessed by calculating the root mean square error (RMSE). We used the functions implemented in the treeclim package in R (Zang and Biondi, 2015) to assess the relationships between annual growth and hydroclimate variability as well as to evaluate the reconstruction skills of each developed TRW chronology. All the analyses were performed using R software (R Core Team, 2013).
Results
The average tree age in the four TRW chronologies ranged from 120.4 (SD = 35.6) years for the HF dataset and 155.0 years (SD = 35.3 years) for the CWS dataset. Average tree age of the combined and random chronologies was 138.2 (SD = 39.4) and 140.1 (SD = 36.8) years, respectively.
Climate-growth correlations for the period 1902–2013 revealed not significant correlations with temperature (Supplementary Figure S1B) but significant positive correlations with all hydroclimate variables that were considered in this study (precipitation, SPEI-6 and soil moisture content) in annual and different monthly and seasonal time windows (Figure 1D). Climate growth correlations were overall strongest for the random chronology, followed by HF and combined chronologies and weakest for CWS. Note that correlations with annual precipitation were only significant for the HF and random chronologies, while mean precipitation in the months from April to September showed significant positive correlations with all chronologies except for the CWS. SPEI-6 showed insignificant correlations with growth of CWS for the early months of the vegetation period (April, May) and soil moisture content only showed significant correlations with CWS for June, July and the summer season. A significant positive correlation was observed between average soil moisture content in the spring months and the random chronology. September soil moisture showed insignificant correlations with all four chronologies. The strongest correlations for all four chronologies were found with June precipitation. Hence, this parameter was used to test the reconstruction skills of the developed chronologies for the period 1902–2013 (Figure 2).
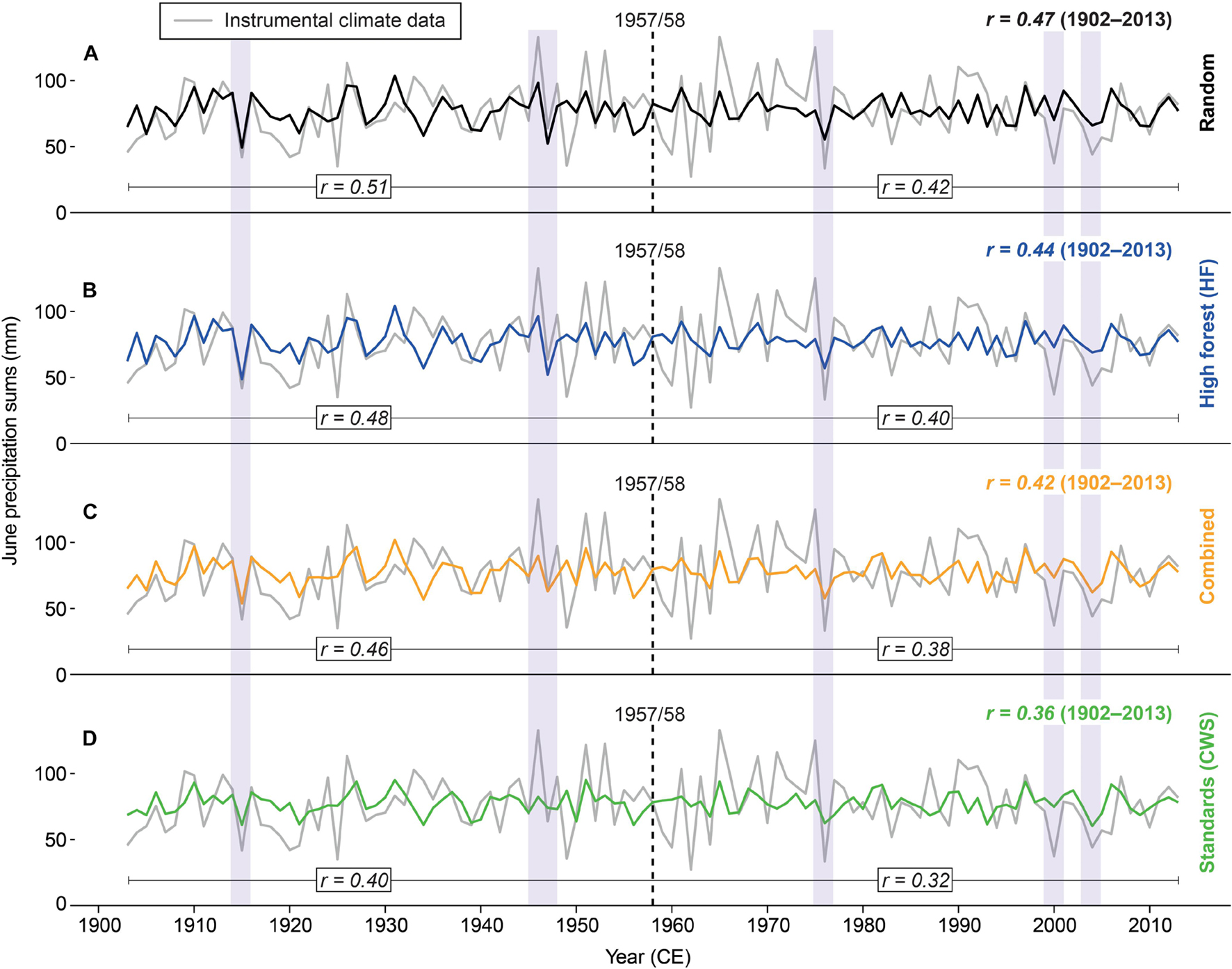
Figure 2. Different regional June precipitation reconstructions for the period 1902–2013 (split calibration/verification periods 1902–1957 and 1958–2013, respectively) and comparison with instrumental climate data (gray). (A) Random chronology (black), (B) high forest (HF) chronology (blue), (C) combined chronology (orange), and (D) standards (CWS) chronology (green). Highlighted gray areas denote positive and negative hydroclimatic extremes that were used for visually assessing the accuracy of the reconstructions in years with extremely low and high June precipitation.
To compare the skills of the four chronologies in reconstructing past climate variability, we performed reconstructions of June precipitation over the period 1902–2013 and compared the reconstructed values against instrumental records from the region (Figure 2). All four models (OLS regressions) used for reconstructions displayed a significance level of 99.9% (p < 0.001). Robustness over time was tested for all reconstructions by splitting the period 1902–2013 into two equal 56-year sub-periods for calibration (1958–2013) and verification (1902–1957). The strongest correlation for the full period (1902–2013) was obtained for the random chronology (Figure 2A; r = 0.47). The correlation coefficient was slightly lower for the calibration period (1958–2013; r = 0.42) than for the verification period (1902–1957; r = 0.51) but still statistically significant. The skill of the reconstruction in capturing precipitation variability is demonstrated with positive RE and CE of 0.26 and 0.25, respectively. Similar results were obtained for HF and combined chronologies (Figures 2B,C), with full-period correlation coefficients 0.44 and 0.42, respectively. In both cases, the correlation coefficients were lower for the calibration period (r = 0.40 and 0.38, respectively) than for the verification period (r = 0.48 and 0.46, respectively). RE and CE were slightly higher for the combined (0.22 and 0.21) than for the HF chronology (0.21 and 0.20). The lowest correlation coefficient for the full period was observed for the CWS chronology (Figure 2D; r = 0.36). Again, correlations remained significant over time, showing lower values for the calibration period (r = 0.32) than the validation period (r = 0.40). RE (0.16) and CE (0.15) were considerably lower compared to the other reconstructions but still larger than zero and therefore confirming the skills of the reconstruction.
Discussion
Dataset Composition and Sample Size
Four TRW chronologies were created based on datasets, which contained differing proportions of trees with periodic management-induced growth releases (sustained growth increases). The CWS dataset consisted entirely of trees (135 in total) from actively managed CWS stands (see also Muigg et al., 2020b for further information). In a CWS forest, the reduced competition for light, nutrients and water after periodic understory coppice causes an increased growth in the remaining standards, commonly referred to as release (Müllerová et al., 2016). The absence of such periodic and temporally aligned release events in the HF dataset suggests the absence of substantial alterations in the canopies or understories of target trees, indicative of growing conditions in a high forest (Nowacki and Abrams, 1997; Bergès et al., 2000). To obtain such release-free TRW series a large dataset of 505 oak trees sampled in lumber yards and a veneer company in the same region was scanned for the absence of characteristic growth releases, providing a high forest (HF) dataset with a sample size of 128 trees and therefore equally well-replicated as the CWS dataset. The combined dataset, including all CWS and HF trees, consisted of 263 trees. With half of the trees originating from CWS forests we expected a strong influence of management interventions also in this dataset. For the random dataset, the highest replication of 505 samples was achieved by including all available oak trees, randomly sampled from regional lumber yards and sawmills to simulate the sampling conditions characteristic in historical and archeological samples, i.e., lack of stand information. Note that the lack of information on historical management practices also applies for trees from old-grown stands, where different and changing silvicultural measures in the past cannot be ruled out, especially before the onset of modern forestry, i.e., before the second half of the nineteenth century (Hausrath, 1982; Hasel and Schwartz, 2006).
Climate Reconstruction
Not significant climate-growth correlations found with temperature for all four chronologies are explicable with the prevalence of hydroclimate, primarily controlling oak growth in low elevation sites (Büntgen et al., 2010; Tegel et al., 2020). The strong correlations found for June precipitation for all four chronologies, are in accordance with previous studies on the climatic sensitivity of oak growth (e.g., Büntgen et al., 2010). It is, however, striking that the random chronology showed the best correlation results for all climate parameters and seasonal windows, consistently followed by the HF and combined chronologies, while the CWS chronology showed the weakest and, in most cases, not significant correlations (Figure 1D). This provides strong evidence for the prevalence of CWS management-induced signals in the TRW chronology over the climate signal and therefore, raises objections to the suitability of CWS standards for climate reconstructions.
The pronounced differences in the correlation strength with hydro-climate variables as well as in the reconstruction skill among the four chronologies revealed the influence of past forest management on the climate signals present in TRW chronologies. The random chronology showed the strongest correlations with all hydroclimate variables and provided the best reconstruction skills for June precipitation, followed by HF and the combined chronologies, while the CWS chronology showed the weakest correlations with hydroclimate variables and reconstruction skill. This implies that trees from CWS managed forests are less suitable for TRW based climate reconstructions than trees from high forests. However, combining TRW series which are strongly influenced by management interventions with TRW series from high forests improves the strength of the climate signals present in an average TRW chronology, as was observed here for the combined chronology.
It is worth noting here that the chronology with the highest replication (random; 505 samples) provided the strongest associations with instrumental climate data. The importance of sample replication for tree-ring based reconstructions has already been highlighted by earlier studies and for other tree species (Büntgen et al., 2012). However, in our study replication alone cannot explain the observed differences in the correlation strength and reconstruction skills among the developed chronologies. The combined chronology (263 samples) showed weaker correlations with hydroclimate variables and demonstrated a lower performance in reconstructing June precipitation than the HF chronology (128 samples). These results further demonstrate the importance of testing individual TRW series for the presence of strong management signals in TRW series (for instance, see Muigg et al., 2020b).
The reconstruction based on the random chronology was also more accurate in years with extremely low and high precipitation sums than the rest of the chronologies. Several years illustrate that the reconstruction reflected the actual precipitation sums best based on the random chronology, successively less in the HF and combined chronologies, while the least accurate was the CWS chronology. Exemplary years, which are also confirmed by other studies (highlighted in Figure 2) include 1915, 1947, 1976, and 2000 (Brázdil et al., 2016; Hänsel et al., 2019). It is, however, striking that June precipitation in 2003 (highlighted in Figure 2) is most clearly reflected in the reconstruction based on the CWS chronology (Figure 2D) and successively less pronounced in the reconstructions based on the combined, HF and random chronologies (Figures 2A–C).
Large amounts of archeological findings of oak wood demonstrate the preference of the species for various constructions throughout past epochs and enabled the development of long and well replicated oak tree-ring chronologies in Europe, providing one of the most important proxy records for high-precision multi-millennial climate reconstructions. During the recent decade, several oak tree-ring based paleoclimate reconstructions have been published by various authors (Büntgen et al., 2010, 2011; Cooper et al., 2013; Wilson et al., 2013; Dobrovolný et al., 2014; Cook et al., 2015; Prokop et al., 2016; Muigg et al., 2020a; Tegel et al., 2020). Several authors have stressed the importance of enhanced understanding of past climate to predict future climate variability (Hegerl and Russon, 2011; Woodhouse et al., 2016; Tierney et al., 2020). As oak wood has been the preferred raw material for various purposes in historical times, particularly for construction timber, it was regarded as a valuable forest resource and hence, oak forests have been extensively managed (Haneca, 2011). The CWS management system has been applied on oak forests for at least the past 1,500 years (Muigg et al., 2020b), most likely with varying intensities in different regions and periods (Short and Hawe, 2012; Short and Campion, 2014). Other forms of historical forest management have been practiced all over Europe, e.g., simple coppice management (Stajic et al., 2009; Unrau et al., 2018). Samples from archeological excavations and historical buildings lack information on the individual tree stands (Tegel et al., 2010). As the growing conditions of such samples remain unknown, potential effects of historical forest management cannot be ruled out. However, they can be compensated for by massive sample replication (cf. Büntgen et al., 2010). Having said this, uncertain silvicultural treatment in the early phases of modern oak trees, which are commonly used to calibrate climate-proxy relationships, could easily lead to under- or overestimation of past climate variability and therefore need to be considered as a potential bias in future studies. Similar to the CWS system, other management interventions, such as thinning can influence climate-growth relationships (see for example Cescatti and Piutti, 1998; Pérez-de-Lis et al., 2011; D’Amato et al., 2013) and should be also considered in tree-ring based reconstructions. Based on the results of this study, scanning of modern reference datasets for management-induced growth patterns provides an important tool to improve future tree-ring based climate reconstructions.
Conclusion
Our results imply that the influence of historical forest management on TRW does affect their suitability for climate reconstructions and should be considered in future paleoclimate reconstructions. It also shows, however, that the negative effects of historical forest management can be mitigated with sufficient sample replication, compensating for the management-induced effects of CWS management and presumably other forms of historical forest management practices. This information is all the more important for future paleoclimate reconstructions in regions with long historical significance and constant human impact on the cultural landscape. Further consideration regarding previous forest management should be given when selecting modern reference material for climate-growth calibration. Historical forest management practices and their influence on tree-ring based reconstructions should be considered in future studies. The field of forest history might provide substantial information for combined studies (Brázdil et al., 2016).
Data Availability Statement
The datasets generated for this study are available on request to the corresponding author. Requests to access these datasets should be directed to GS, Z2Vvcmdpb3Muc2tpYWRhcmVzaXNAd2FsZGJhdS51bmktZnJlaWJ1cmcuZGU=.
Author Contributions
GS and BM designed the study, performed the analyses, and developed the datasets. BM, GS, and WT sampled the modern CWS stands. BM and GS wrote the manuscript with input from WT. All authors provided critical discussion, helped writing the manuscript, and approved its submission.
Funding
The article processing charge was funded by the Baden-Wuerttemberg Ministry of Science, Research and Art and the University of Freiburg in the funding program Open Access Publishing.
Conflict of Interest
The authors declare that the research was conducted in the absence of any commercial or financial relationships that could be construed as a potential conflict of interest.
Publisher’s Note
All claims expressed in this article are solely those of the authors and do not necessarily represent those of their affiliated organizations, or those of the publisher, the editors and the reviewers. Any product that may be evaluated in this article, or claim that may be made by its manufacturer, is not guaranteed or endorsed by the publisher.
Supplementary Material
The Supplementary Material for this article can be found online at: https://www.frontiersin.org/articles/10.3389/fevo.2021.727651/full#supplementary-material
Footnotes
References
Albrecht, L., and Abt, A. (2014). Die Bedeutung der Eiche im bäuerlichen Mittelwald. LWF Wissen 75, 41–47.
Bergès, L., Dupouey, J.-L., and Franc, A. (2000). Long-term changes in wood density and radial growth of Quercus petraea Liebl. in northern France since the middle of the nineteenth century. Trees 14, 398–408. doi: 10.1007/s004680000055
Bernard, V., Le Digol, Y., and Couturier, Y. (2014). “Production de bois d’oeuvre et pratiques sylvicoles entre forêt et bocage: Dendroarchéologie des charpentes du territoire de Sainte-Suzanne (XIIe–XVIIIe siècles),” in Sainte-Suzanne -un Territoire remarquable en Mayenne, eds C. Davy and N. Foisneau (Nantes: Revue 303), 243–257.
Brázdil, R., Raška, P., Trnka, M., Zahradníček, P., Valášek, H., Dobrovolný, P., et al. (2016). The Central European drought of 1947: causes and consequences, with particular reference to the Czech Lands. Clim. Res. 70, 161–178. doi: 10.3354/cr01387
Büntgen, U., Tegel, W., Heussner, K.-U., Hofmann, J., Kontic, R., Kyncl, T., et al. (2012). Effects of sample size in dendroclimatology. Clim. Res. 53, 263–269. doi: 10.3354/cr01107
Büntgen, U., Tegel, W., Nicolussi, K., McCormick, M., Frank, D., Trouet, V., et al. (2011). 2500 years of European climate variability and human susceptibility. Science 331, 578–582. doi: 10.1126/science.1197175
Büntgen, U., Trouet, V., Frank, D., Leuschner, H. H., Friedrichs, D., Luterbacher, J., et al. (2010). Tree-ring indicators of German summer drought over the last millennium. Quat. Sci. Rev. 29, 1005–1016. doi: 10.1016/j.quascirev.2010.01.003
Cescatti, A., and Piutti, E. (1998). Silvicultural alternatives, competition regime and sensitivity to climate in a European beech forest. For. Ecol. Manag. 102, 213–223.
Cook, E. R., and Peters, K. (1981). The smoothing spline: a new approach to standardizing forest interior tree-ring width series for dendroclimatic studies. Tree-Ring Bulletin 41, 45–53.
Cook, E. R., Briffa, K. R., and Jones, P. D. (1994). Spatial regression methods in dendroclimatology: a review and comparison of two techniques. Int. J. Climatol. 14, 379–402. doi: 10.1002/joc.3370140404
Cook, E. R., Seager, R., Kushnir, Y., Briffa, K. R., Büntgen, U., Frank, D., et al. (2015). Old World megadroughts and pluvials during the Common Era. Sci. Adv. 1:e1500561. doi: 10.1126/sciadv.1500561
Cooper, R. J., Melvin, T. M., Tyers, I., Wilson, R. J. S., and Briffa, K. R. (2013). A tree-ring reconstruction of East Anglian (UK) hydroclimate variability over the last millennium. Clim. Dyn. 40, 1019–1039. doi: 10.1007/s00382-012-1328-x
D’Amato, A. W., Bradford, J. B., Fraver, S., and Palik, B. J. (2013). Effects of thinning on drought vulnerability and climate response in north temperate forest ecosystems. Ecol. Appl. 23, 1735–1742. doi: 10.1890/13-0677.1
Dobrovolný, P., Brázdil, R., Rezníčková, L., Rybníček, M., Kolář, T., and Büntgen, U. (2014). New millennium long oak tree-ring width chronology and its potential for climate reconstruction for the Czech Lands (EGU General Assembly 2014). Geophys. Res. Abstr. 16:EGU2014-5838-2.
Groß, P., and Konold, W. (2010). The “Mittelwald” - an agroforestry system between rigid sustainability and creative options. A historical study/Mittelwald als Agroforstsystem zwischen geordneter Nachhaltigkeit und Gestaltungsvielfalt – Eine historische Studie. Allg. Forst u. J. Ztg. 181, 64–71.
Haneca, K. (2011). “The influence of historic forest management practices on oak chronology building in Flanders,” in Tree Rings, Art, Archaeology. Proceedings of an international Conference, Scientia Artis 7, Brussels, 10-12 February 2010, ed. P. Fraiture (Brussels: Royal Institute for Cultural Heritage), 67–75.
Hänsel, S., Ustrnul, Z., Łupikasza, E., and Skalak, P. (2019). Assessing seasonal drought variations and trends over Central Europe. Adv. Water Resour. 127, 53–75. doi: 10.1016/j.advwatres.2019.03.005
Hasel, K., and Schwartz, E. (2006). Forstgeschichte. Ein Grundriß für Studium und Praxis, 3rd Edn. Remagen: Kessel.
Hausrath, H. (1982). Geschichte des deutschen Waldbaus. Von seinen Anfängen bis 1850. Freiburg im Breisgau: Hochschulverlag.
Hegerl, G. C., and Russon, T. (2011). Using the Past to Predict the Future? Science 334, 1360–1361. doi: 10.1126/science.1214828
Knibbe, B. (2008). PAST4 – Personal Analysis System for Treering Research version 4.5. Instruction Manual. Vienna: SCIEM.
Mosandl, R., Summa, J., and Stimm, B. (2010). Coppice-with-standards: management options for an ancient forest system. For. Ideas 16, 65–74.
Muigg, B., Seim, A., Tegel, W., Werther, L., Herzig, F., Schmidt, J., et al. (2020a). Tree rings reveal dry conditions during Charlemagne’s Fossa Carolina construction in 793 CE. Quat. Sci. Rev. 227:106040. doi: 10.1016/j.quascirev.2019.106040
Muigg, B., Skiadaresis, G., Tegel, W., Herzig, F., Krusic, P. J., Schmidt, U. E., et al. (2020b). Tree rings reveal signs of Europe’s sustainable forest management long before the first historical evidence. Sci. Rep. 10:21832. doi: 10.1038/s41598-020-78933-8
Müllerová, J., Pejcha, V., Altman, J., Plener, T., Dörner, P., and Doležal, J. (2016). Detecting Coppice Legacies from Tree Growth. PLoS One 11:e0147205. doi: 10.1371/journal.pone.0147205
Nowacki, G. J., and Abrams, M. D. (1997). Radial-growth averaging criteria for reconstructing disturbance histories from presettlement-origin oaks. Ecol. Monogr. 67, 225–249. doi: 10.1890/0012-9615(1997)067[0225:rgacfr]2.0.co;2
Pérez-de-Lis, G., García-González, I., Rozas, V., and Arévalo, J. R. (2011). Effects of thinning intensity on radial growth patterns and temperature sensitivity in Pinus canariensis afforestations on Tenerife Island, Spain. Ann. For. Sci. 68, 1093–1104. doi: 10.1007/s13595-011-0125-8
Prokop, O., Kolář, T., Büntgen, U., Kyncl, J., Kyncl, T., Bošel’a, M., et al. (2016). On the palaeoclimatic potential of a millennium-long oak ring width chronology from Slovakia. Dendrochronologia 40, 93–101. doi: 10.1016/j.dendro.2016.08.001
R Core Team. (2013). R: A Language and Environment for Statistical Computing. Vienna, Austria: R Foundation for Statistical Computing.
Short, I., and Campion, J. (2014). Coppice-with-standards: an old silvicultural system with new potential? For. Energy Rev. 4, 42–44.
Short, I., and Hawe, J. (2012). Possible silvicultural systems for use in the rehabilitation of poorly performing pole-stage broadleaf stands – Coppice-with-standards. Irish Forestry 69, 148–166.
Slivinski, L. C., Compo, G. P., Whitaker, J. S., Sardeshmukh, P. D., Giese, B. S., McColl, C., et al. (2019). Towards a more reliable historical reanalysis: improvements for version 3 of the Twentieth Century Reanalysis system. Q. J. R. Meteorol. Soc. 145, 2876–2908. doi: 10.1002/qj.3598
Stajic, B., Zlatanov, T., Velichkov, I., Dubravac, T., and Trajkov, P. (2009). Past and recent coppice forest management in some regions of South eastern Europe. Silva Balcanica 10, 9–19.
Szabó, P., Müllerová, J., Suchánková, S., and Kotačka, M. (2015). Intensive woodland management in the Middle Ages: spatial modelling based on archival data. J. Historical Geogr. 48, 1–10. doi: 10.1016/j.jhg.2015.01.005
Tegel, W., Seim, A., Skiadaresis, G., Ljungqvist, F. L., Kahle, H.-P., Land, A., et al. (2020). Higher groundwater levels in western Europe characterize warm periods in the Common Era. Sci. Rep. 10:16284. doi: 10.1038/s41598-020-73383-8
Tegel, W., Vanmoerkerke, J., and Büntgen, U. (2010). Updating historical tree-ring records for climate reconstruction. Quat. Sci. Rev. 29, 1957–1959. doi: 10.1016/j.quascirev.2010.05.018
Tierney, J. E., Poulsen, C. J., Montañez, I. P., Bhattacharya, T., Feng, R., Ford, H. L., et al. (2020). Past climates inform our future. Science 370:eaay3701. doi: 10.1126/science.aay3701
Unrau, A., Becker, G., Spinelli, R., Lazdina, D., Magagnotti, N., Nicolescu, V. N., et al. (eds) (2018). Coppice Forests in Europe. Freiburg: Albert-Ludwigs-Universität.
Vandekerkhove, K., Baeté, H., Van Der Aa, B., De Keersmaeker, L., Thomaes, A., Leyman, A., et al. (2016). 500 years of coppice-with-standards management in Meerdaal Forest (Central Belgium). iForest Biogeosci. Forestry 9, 509–517. doi: 10.3832/ifor1782-008
Vicente-Serrano, S. M., Beguería, S., and López-Moreno, J. I. (2010). A multiscalar drought index sensitive to global warming: the standardized precipitation evapotranspiration index. J. Clim. 23, 1696–1718. doi: 10.1175/2009jcli2909.1
Wigley, T. M. L., Briffa, K. R., and Jones, P. D. (1984). On the average value of correlated time series, with applications in dendroclimatology and Hydrometeorology. J. Clim. Appl. Meteorol. 23, 201–213. doi: 10.1175/1520-0450(1984)023<0201:otavoc>2.0.co;2
Wilson, R., Miles, D., Loader, N. J., Cunningham, L., Cooper, R., and Briffa, K. (2013). A millennial long March–July precipitation reconstruction for southern-central England. Clim. Dyn. 40, 997–1017. doi: 10.1007/s00382-012-1318-z
Woodhouse, C. A., Lukas, J. J., Morino, K., Meko, D. M., and Hirschboeck, K. K. (2016). “Using the past to plan for the future-the value of paleoclimate reconstructions for water resource planning,” in Water Policy and Planning in a Variable and Changing Climate, eds K. A. Miller, A. F. Hamlet, D. S. Kenney, and K. T. Redmond (Boca Raton: CRC Press), 162–182.
Keywords: Quercus spp., hydroclimate sensitivity, forest management, tree-rings, coppice-with-standards, climate reconstruction
Citation: Skiadaresis G, Muigg B and Tegel W (2021) Historical Forest Management Practices Influence Tree-Ring Based Climate Reconstructions. Front. Ecol. Evol. 9:727651. doi: 10.3389/fevo.2021.727651
Received: 19 June 2021; Accepted: 20 August 2021;
Published: 16 September 2021.
Edited by:
Olga Solomina, Institute of Geography (RAS), RussiaReviewed by:
Ionel Popa, National Institute for Research and Development in Forestry Marin Dracea (INCDS), RomaniaJesús Julio Camarero, Consejo Superior de Investigaciones Científicas (CSIC), Spain
Ignacio García-González, University of Santiago de Compostela, Spain
Bao Yang, Cold and Arid Regions Environmental and Engineering Research Institute, Chinese Academy of Sciences, China
Copyright © 2021 Skiadaresis, Muigg and Tegel. This is an open-access article distributed under the terms of the Creative Commons Attribution License (CC BY). The use, distribution or reproduction in other forums is permitted, provided the original author(s) and the copyright owner(s) are credited and that the original publication in this journal is cited, in accordance with accepted academic practice. No use, distribution or reproduction is permitted which does not comply with these terms.
*Correspondence: Georgios Skiadaresis, Z2Vvcmdpb3Muc2tpYWRhcmVzaXNAd2FsZGJhdS51bmktZnJlaWJ1cmcuZGU=