- 1Département de Biologie, Centre d’Études Nordiques, Université du Québec à Rimouski, Rimouski, QC, Canada
- 2Polar Knowledge Canada, Canadian High Arctic Research Station, Cambridge Bay, NU, Canada
- 3Département de Biologie, Centre d’Études Nordiques, Université Laval, Québec City, QC, Canada
- 4Division of Migratory Bird Management, U.S. Fish and Wildlife Service, Anchorage, AK, United States
- 5Department of Integrative Biology, University of Windsor, Windsor, ON, Canada
- 6Canadian Wildlife Service, Environment and Climate Change Canada, Yellowknife, NT, Canada
- 7Department of Ecology, Montana State University, Bozeman, MT, United States
- 8Portland Audubon, Portland, OR, United States
- 9Wildlife Conservation Society, Arctic Beringia Program, Fairbanks, AK, United States
- 10Alberta Environment and Parks, Grande Prairie, AB, Canada
- 11Department of Biology, Trent University, Peterborough, ON, Canada
- 12South Carolina Department of Natural Resources, McClellanville, SC, United States
- 13Bilingual Biology Program, York University, Toronto, ON, Canada
- 14Wildlife Research Division, Environment and Climate Change Canada, Ottawa, ON, Canada
- 15Canadian Wildlife Service, Environment and Climate Change Canada, Delta, BC, Canada
- 16Canada Research Chair in Polar and Boreal Ecology, Centre d’Études Nordiques, Université de Moncton, Moncton, NB, Canada
- 17K.-C.-Irving Research Chair in Environmental Sciences and Sustainable Development, Université de Moncton, Moncton, NB, Canada
- 18Department of Bird Migration, Swiss Ornithological Institute, Sempach, Switzerland
- 19Molecular Ecology and Evolution Lab, Department of Biology, Lund University, Lund, Sweden
Long-distance migrants are under strong selection to arrive on their breeding grounds at a time that maximizes fitness. Many arctic birds start nesting shortly after snow recedes from their breeding sites and timing of snowmelt can vary substantially over the breeding range of widespread species. We tested the hypothesis that migration schedules of individuals co-occurring at the same non-breeding areas are adapted to average local environmental conditions encountered at their specific and distant Arctic breeding locations. We predicted that timing of breeding site availability (measured here as the average snow-free date) should explain individual variation in departure time from shared non-breeding areas. We tested our prediction by tracking American Golden-Plovers (Pluvialis dominica) nesting across the North-American Arctic. These plovers use a non-breeding (wintering) area in South America and share a spring stopover area in the nearctic temperate grasslands, located >1,800 km away from their nesting locations. As plovers co-occur at the same non-breeding areas but use breeding sites segregated by latitude and longitude, we could disentangle the potential confounding effects of migration distance and timing of breeding site availability on individual migration schedule. As predicted, departure date of individuals stopping-over in sympatry was positively related to the average snow-free date at their respective breeding location, which was also related to individual onset of incubation. Departure date from the shared stopover area was not explained by the distance between the stopover and the breeding location, nor by the stopover duration of individuals. This strongly suggests that plover migration schedule is adapted to and driven by the timing of breeding site availability per se. The proximate mechanism underlying the variable migration schedule of individuals is unknown and may result from genetic differences or individual learning. Temperatures are currently changing at different speeds across the Arctic and this likely generates substantial heterogeneity in the strength of selection pressure on migratory schedule of arctic birds migrating sympatrically.
Introduction
Long-distance migrants should adjust their spring migration schedule to arrive on their stopover areas and breeding grounds at the time that maximizes fitness (Lack, 1968; Marra, 1998). Yet, substantial variation exists in the migration timing of individuals, both within and among populations (Cristol et al., 1999; Briedis et al., 2016). For species with a broad geographical distribution, spatial variation in temporal availability of breeding habitat could partially explain variation in individual migration schedule, as individuals from different populations should have different optimal timing of migration (Alerstam and Lindström, 1990; Buehler and Piersma, 2008; Pedersen et al., 2018).
Studies performed on several temperate bird species provided evidence that latitudinally separated breeding populations vary in their migration schedules (Stanley et al., 2012; Briedis et al., 2016; Loon et al., 2017). Additionally, tracking data from Arctic-nesting shorebirds showed that breeding latitude is related to the timing of migration for individuals overwintering in sympatry (Conklin et al., 2010). Such relationships between breeding latitudes and migration schedules support the hypothesis that variation in the temporal availability of breeding habitat drives among and within population variation in timing of migration.
Although breeding latitude is considered a reliable indicator of the temporal availability of breeding habitat, differences in stopover behavior and migration schedule are typically correlated with migration distance (Fraser et al., 2013; Ketterson et al., 2015; Åkesson et al., 2017; Rakhimberdiev et al., 2018). For example, birds breeding at higher latitudes could potentially delay departure from non-breeding (wintering) or stopover areas to increase their body condition, allowing them to cover longer migration distances. Moreover, latitudinally separated breeding populations can experience variable environmental conditions during their northward migration when using different routes or stopover areas (e.g., Marra, 1998; Trierweiler et al., 2014), resulting in different migration schedules (Clausen et al., 2015; Ely et al., 2018). We propose that by comparing the migration schedule of sympatrically overwintering and migrating individuals that have breeding locations segregated by latitude and longitude, we may be able to disentangle potential drivers of migration schedule.
In northern ecosystems, most migratory birds start breeding shortly after snow recedes from their breeding sites (Grabowski et al., 2013; Liebezeit et al., 2014; van Gils et al., 2016; Saalfeld and Lanctot, 2017; Rakhimberdiev et al., 2018). Because the timing of snowmelt can vary substantially over the breeding range of widespread species (Niehaus and Ydenberg, 2006; Kwon et al., 2019), comparing migratory strategies used by a species across its breeding range can offer insight into drivers of migration timing.
Using the American Golden-Plover (Pluvialis dominica) as study model having large breeding range but common overwintering and stopover areas, we tested the hypothesis that migration schedule of individuals is adapted to average local environmental conditions encountered at their breeding locations. American Golden-Plovers are long-distance migrants faithful to their breeding sites located in the arctic and subarctic tundra and the breeding range of the species is spread from western Alaska (United States) to Eastern Nunavut (Canada) (Johnson et al., 2020a; Figure 1). All individuals share a common non-breeding (wintering) area in South America, and migrate north over the Amazon before all using a common stopover area in the nearctic temperate grasslands of the Midwestern United States (Johnson et al., 2020a). Across the breeding range, the timing of nest initiation varies substantially (from late-May to early July; Weiser et al., 2018) and coincides with the moment when breeding habitats become snow-free (Smith et al., 2010; Liebezeit et al., 2014; Saalfeld and Lanctot, 2017). When comparing plovers that co-occur at the same wintering and stopover sites, we predicted that individual migration schedule is mainly linked to variation in average snow-free date at the nesting location, and not to migration distance.
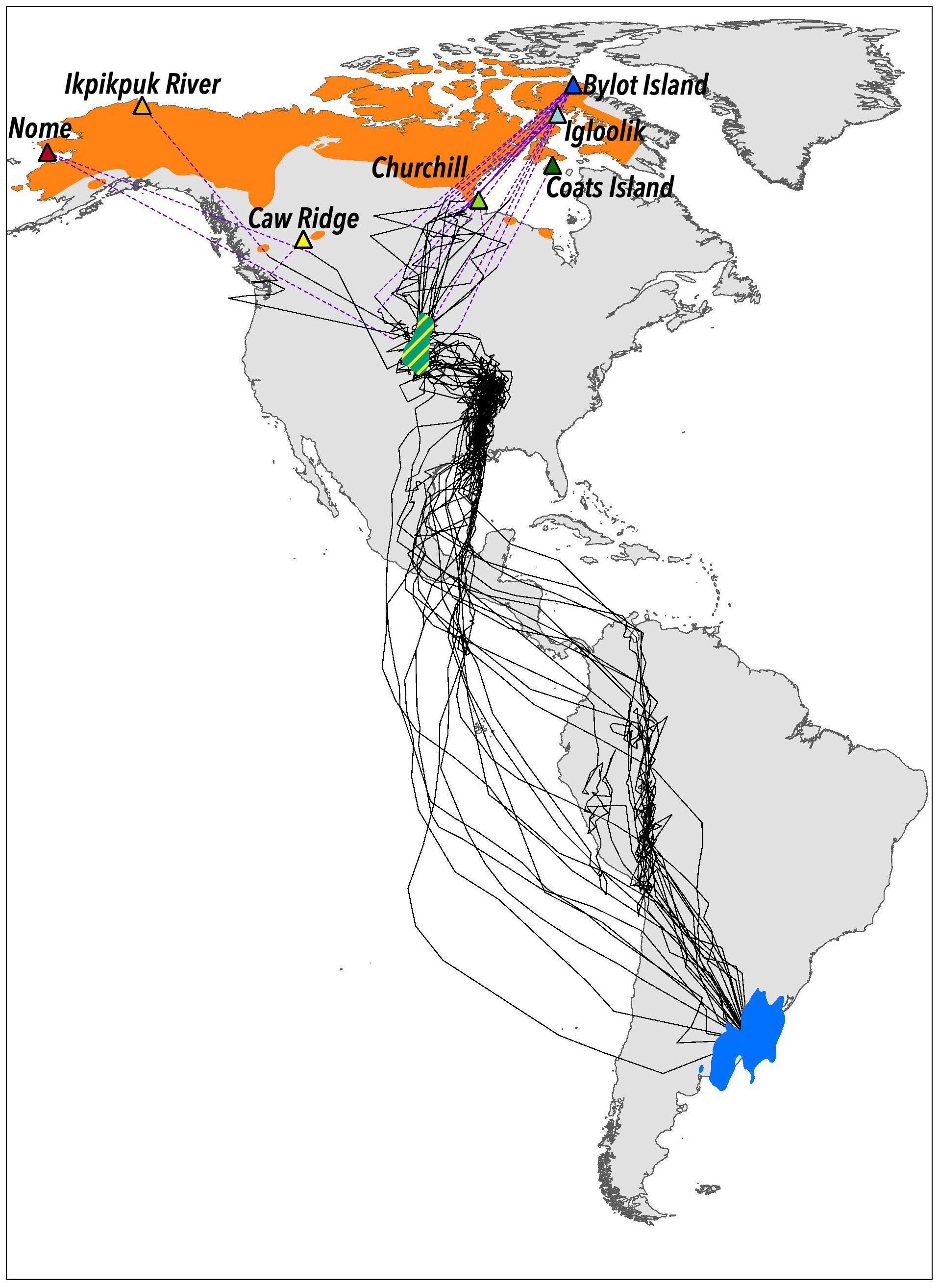
Figure 1. American Golden-Plover (Pluvialis dominica) breeding range (orange; based on Johnson et al., 2020a), non-breeding (wintering) area (blue polygon) and last shared spring stopover area (green polygon with yellow stripes) located in Nearctic temperate grasslands of the Midwestern United States (mostly South Dakota, Nebraska, and Kansas). Wintering and shared stopover areas were defined with Kernel density estimation (75%) based on locations of individuals marked with light-level geolocators (n = 23; black lines illustrate individual tracks). Purple dashed lines represent straight lines between last location obtained for an individual and its breeding location. Birds were captured and marked at study areas (triangles) distributed across the breeding range.
Materials and Methods
Capturing and Marking Plovers
Incubating American Golden-Plovers (hereafter referred to as plovers) were trapped at seven study areas distributed across the entire breeding range of the species (Figure 1). Nests were located by searching appropriate habitats and birds were trapped with a bownet (60 cm in diameter) placed over their nest. Each bird was equipped with metal and plastic color bands for individual identification and with an archival light-level geolocator (British Antarctic Survey MK10b, weight ∼1.1 g or Migrate Technology Ltd., Intigeo geolocators, W65A9RK, weight ∼ 0.87 g; Figure 2). The sex of the plovers was identified using breeding plumage characteristics (Johnson et al., 2020a). A total of 262 geolocators were deployed from 2009 to 2015, and 45 of them (∼17%) were retrieved by recapturing individuals 1–4 years after their deployment. Plovers are faithful to their breeding sites (Johnson et al., 2020a). We detected marked individuals during subsequent nesting seasons by conducting visual surveys in each study area. Distance between nest locations of marked individuals monitored more than 1 year was 319 m on average (range 54–1,119 m; n = 22). Spring migration paths (from “wintering” to breeding sites) were obtained for 23 plovers. A total of 1–11 geolocators were recovered per study area: Alaska: Nome, n = 2; Ikpikpuk River, n = 1; Alberta: Caw Ridge, n = 1; Manitoba, Churchill, n = 3; Nunavut: Coats Island, n = 1; Igloolik, n = 4; Bylot Island, n = 11 (Figure 1). Sampled individuals were from different breeding pairs. Birds were also equipped with tags at Utqiagvik (Alaska, 71.287 −156.744, ∼110 km North-West of Ikpikpuk river – Figure 1), but geolocation information for the northbound trip was insufficient to be included in this study.
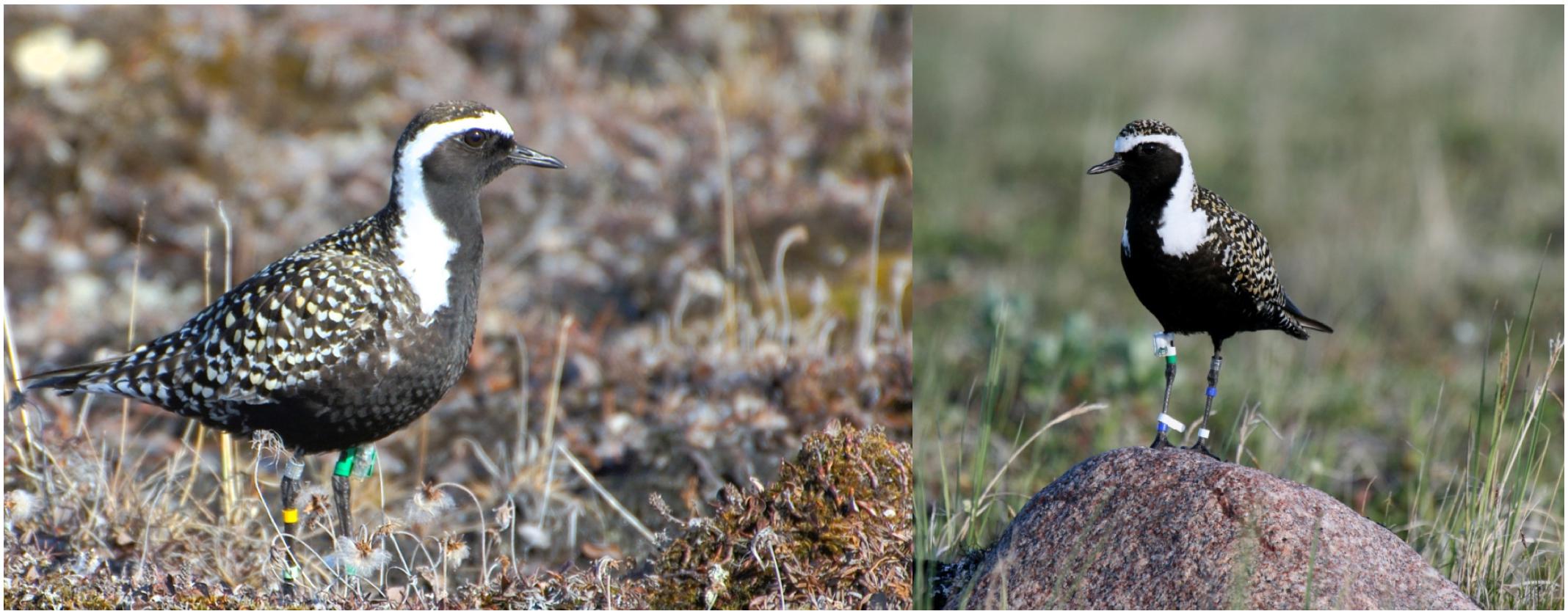
Figure 2. Breeding American Golden-Plover (Pluvialis dominica) equipped with leg bands and a geolocator (left tibia). Photo by Andréanne Beardsell (left) and FS (right).
Processing Geolocator Data
Light data were converted into locations (latitude and longitude) using a multi-step approach. As light data output is different between models of geolocators, we used device-specific light thresholds (three for British Antarctic Survey geolocators and two for Intigeo geolocators) to identify sunrise and sunset (after Finch et al., 2015). Light data were analyzed using the R package GeoLight 2.0 (Lisovski et al., 2015). Night-time light noise was reduced using the lightFilter function of GeoLight 2.0. We identified residency and movement periods with the ChangeLight function of GeoLight 2.0 (quantile = 0.9, minimum residency length = 2 days). We performed a rooftop calibration and we used the derived sun elevation angle [mean = −6.76° (range: −7.33, −4.17), n = 16] to initiate the Hill-Eckstrom method and obtain residency site-specific sun elevation angles in order to increase location estimates accuracy. When no sun elevation angle could be obtained by either rooftop or Hill-Eckstrom calibration, civil twilight was used (i.e.,−6°, n = 7). We applied a loess filter (k = 2) using the loessFilter function of GeoLight 2.0 to remove outliers.
Following Hobson and Kardynal (2015), each migratory path was smoothed with a state-space Kalman filter and the most probable path was obtained with kftrack (Sibert and Nielsen, 2002) in R. Kalman filtering reduces observer bias when dealing with raw location estimates obtained with geolocators and provides the most probable track from location data (Hobson and Kardynal, 2015; Gow, 2016). Estimated flight speeds of American Golden-Plovers vary widely (see Johnson et al., 2020a). To set the diffusion component of the model, we used a relatively high flight speed estimate (104.6 km/h, Johnson and Morton, 1976), which corresponds to a maximum of 2,510 km per day. Kftrack uses an asymmetric error structure peaking on the winter side of the equinoxes, which is typical for geolocator data.
Migration Parameters
We performed a visual inspection of each animal track to assign a geographic designation to each cluster of points when movements were reduced, became erratic and lost directionality (e.g., staging or stopover site). The wintering area corresponded to the southernmost site used by individuals, while the last shared stopover corresponded to an area in the Midwestern United States in which all individuals showed limited movements before resuming their northward movement. Departure dates was the date of the first location out of an area for birds initiating unidirectional northward movement, and arrival date at the last stopover was the date of the first location within the stopover area. Stopover duration was the difference between departure and arrival date at the last shared stopover area. The size of wintering and shared stopover areas were summarized with Kernel Density Estimation (75%) with the kde function in the R package ks (Duong et al., 2021) by pooling locations obtained from all individuals. Arrival date at the nesting location and migration routes used during the final leg of migration could not be determined for most birds with geolocators because areas above the Arctic Circle receive 24 h of sunlight in summer. The distance from the last shared stopover area and the nesting location of each individual was calculated using the geodesic distance (Hijmans et al., 2015) between the last location of a given individual within the stopover area prior to the northward departure and the nest location. Total distance of spring migration was estimated by measuring the sum of geodesic distances from the wintering area to the breeding site via the centroids of all stopovers areas.
Breeding Parameters
Nest searching was conducted during egg laying and early incubation. The onset of incubation was estimated using the observed laying or hatching date (Brown et al., 2014), or using egg floatation if necessary (accurate to within ±4 days; Liebezeit et al., 2007). We also used light data patterns provided by geolocators to confirm the first nesting attempt and to identify onset of incubation for some individuals lacking nest monitoring data (n = 9). As an incubating bird shades the leg-mounted geolocator, a dramatic shift toward more dark periods (i.e., geolocators recording shorter day lengths) after a gradual increase in day length (associated with birds’ northward migration toward areas of longer day length) indicated the start of incubation (see Bulla et al., 2016). The onset of incubation estimated using light data was highly correlated with estimates based on nest monitoring data (r = 0.83, p < 0.001, n = 13 birds for which estimates were obtained using both methods).
Breeding Site Availability
Plovers are ground nesters and thus require snow-free patches to build their nests and to access their main food source (surface active arthropods), allowing them to produce and lay eggs (Byrkjedal and Thompson, 1998; Hobson and Jehl, 2010). As a proxy of breeding site availability, we used the average snow-free date (from 2001 to 2015) from a 500 m resolution map produced with MODIS for North America (data and methodological details provided in O’Leary et al., 2017). Snow-free date can vary spatially, even within a given breeding area, and hence we used data obtained near each plover’s nesting site. Although snowmelt patterns were recorded in the field at some study area, they were not available for specific nesting locations or were obtained using different protocols. Hence, we used standardized remote sensing data for consistency.
Analysis
We built linear mixed models using the R package lme4 (Bates et al., 2015), in association with the R package blme (Chung et al., 2013) when singularity was detected, to investigate the relationships between timing of spring migration and breeding site availability (explanatory variable: average snow-free date from 2001 to 2015 at nesting location). Our response variables representing timing of spring migration included: (a) departure date from the wintering ground, (b) arrival date at the last shared stopover area, and (c) departure date from the last shared stopover area. Year was included as a random factor. Although migration parameters can differ between sexes in some plover species (Byrkjedal and Thompson, 1998), our limited sample size (12 males, three females, eight individuals with unknown sex) prevented us from adding sex as a covariate in the model. We report on models with all individuals because the model results for the male-only sample did not differ. Outliers were identified with Grubbs’ test (p < 0.05, R library outliers; Komsta, 2011). When relevant, we used the methods described by Nakagawa and Schielzeth (2013) to calculate the amount of variance explained by the fixed effects only (Marginal R2: R2m) and the entire model (Conditional R2: R2c). All correlations presented are Pearson correlation coefficients (r). All values are presented with 95% confidence intervals (CI) unless specified otherwise and we used the day of year (day 1 = January 1st) to report migration schedules. All analyses respected the assumptions of normality and homoscedasticity of residuals (Zuur, 2009) and were performed using R version 3.5.3 (R Core Team, 2019).
Results
Plovers departed from their wintering area between late January and mid-March [median day of year – hereafter DOY: 54, range: (28, 74), n = 23]. All American golden plovers in this study used a last shared stopover area in the nearctic temperate grasslands of the Midwestern United States (Figure 1). This last shared stopover area was mostly located in South Dakota, Nebraska, and Kansas. Arrival dates to the last shared stopover area were between early April and mid-May [median DOY: 133, range: (106, 142), n = 23, Figure 3]. Individuals had stopover lengths ranging from 2 to 22 days (median: 8 days, n = 23) at the shared stopover area. Departure dates from the last shared stopover area were between early-May and late-May [median DOY: 142, range: (123, 148), n = 23]. The average snow-free date at individual nesting locations ranged from late-May to late-June [median DOY: 168, range: (142, 179), n = 23]. The onset of egg incubation ranged between late-May and early-July [median DOY: 169, range (148, 183) n = 23]. The distance between the last shared stopover area and the nesting location varied from 1,836 km to 4,788 km (mean = 3,263 km, n = 23) and was correlated with the latitude of the nesting location (r = 0.59, p < 0.01, n = 23). This distance was not correlated with the average snow-free date at the nesting location (r = 0.19, p = 0.4, n = 23). This remaining distance to reach the breeding ground represented between 14 and 35% (mean: 25%) of the total estimated spring migration, which varied from 11,094 km to 13,956 km (mean: 12,695 km; individual tracks are illustrated in Figure 1). The individual’s onset of incubation was correlated with the nesting location latitude (r = 0.77, p < 0.001, n = 23) and positively related to the average snow-free date at the nesting location [B: 0.56, CI: (0.45, 0.69), n = 23, R2m = 0.52, R2c = 0.92]. The average number of days between nest initiation date and mean snow-free date at the nesting locations was 5.2 [CI: (1.9, 8.4), n = 23].
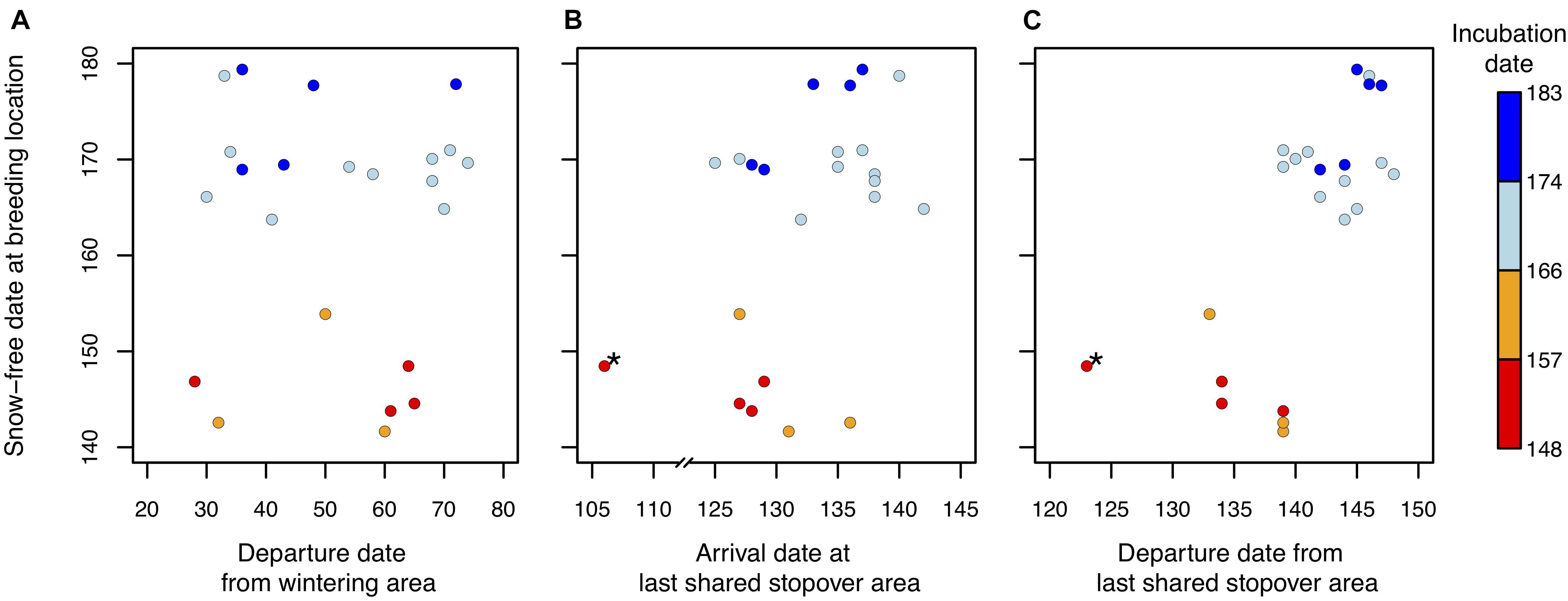
Figure 3. Relationships between timing of breeding site availability (mean snow-free date) and (A) departure date from the non-breeding (wintering) area, (B) arrival or (C) departure date from the last shared stopover site in the nearctic temperate grasslands of the Midwestern United States in tundra nesting American Golden-plover (Pluvialis dominica). To help visualize geographical relationships, axis were transposed. Colors indicate the onset of incubation for each individual (n = 23). A slight neutral offset was added to overlapping points to facilitate interpretation. Day 1 = January 1st. “*” signifies an outlier (Grubb’s test, p < 0.05, see section “Materials and Methods”).
The departure date from the wintering grounds was not related to the average snow-free date at the nesting location of an individual [B: 0.01, CI: (−0.53, 0.55), n = 23, Figure 3]. The arrival date at the last shared stopover area was positively related to the average snow-free date at the nesting location [B: 0.25, CI: (0.03, 0.50), n = 23, R2m = 0.16, R2c = 0.35, Figure 3]. However, an outlier was identified using Grubb’s test (Figure 3). After removing the outlier, we only detected a marginal positive relationship as the confidence interval on the slope overlapped zero [B: 0.18, CI: (−0.001, 0.32), n = 22, R2m = 0.15, R2c = 0.35, Figure 3]. The departure date from the last shared stopover area was positively related to the average snow-free date at the nesting location [B: 0.25, CI: (0.11, 0.40), n = 23; R2m = 0.31, R2c = 0.57, Figure 3]. The relationship remained strong after removing the same individual again identified as an outlier [B: 0.22, CI: (0.11, 0.34), n = 22, R2m = 0.37, R2c = 0.59]. Sample size was too low to rigorously test for annual variation in the observed relationship. However, analyses performed separately for a few years with more data points yielded to very similar results (see Supplementary Material).
The stopover duration was longer for individuals arriving earlier at the shared stopover [B: −0.51, CI: (−0.73, −0.29), n = 23]. However, it was not related to the distance between the last shared stopover and the nesting location [B: 0.001, CI: (−0.002, 0.003), n = 23], nor to snow-free date at the nesting location [B: 0.04, CI: (−0.14, 0.22), n = 23]. The departure date from the last shared stopover area was also independent of the remaining distance to reach the nesting location [B: −0.001, CI: (−0.004, 0.002), n = 23], even when removing the outlier [B = 0.000, CI: (−0.002, 0.002)]. Finally, the distance between the stopover and nest location was unrelated to the onset of incubation of individuals [B: 50.35, CI: (−26.11, 91.34), n = 23].
Discussion
By tracking individual plovers breeding across a broad geographic range, our study provides strong evidence that migration schedule of long-distance migrants stopping-over in sympatry is adapted to and driven by the timing of breeding site availability per se. Indeed, we found that departure time from the last shared stopover area in the Midwestern United States was positively associated with our index of the temporal availability of breeding sites (average snow-free date at the individual nesting location), but that departure from the non-breeding (wintering) area was not. Thus, individuals nesting in areas available earlier within the species’ breeding range departed earliest from the final shared stopover area, which is located 1,836–4,788 km away from their nesting locations. The temporal availability of breeding resources is expected to affect the optimal timing of migration in long-distance migrants (Alerstam and Lindström, 1990; Pedersen et al., 2018). Neither stopover duration nor distance from stopover to breeding locations explained individual variation in departure time. Hence, we can exclude the potential confounding effects of migration distance, which is typically correlated with breeding latitude (Stanley et al., 2012; Briedis et al., 2016; Loon et al., 2017). The proximate mechanism underlying the shifted migration schedule of individuals breeding at nesting locations available earlier is unknown and may result from genetic differences or individual learning (see below; Åkesson and Helm, 2020).
Our study indicates that only the last leg of the spring migration is shifted temporally for individuals using breeding sites available at different times. This result differs from previous studies that documented a shift in the departure date from non-breeding (wintering) areas or differences in stopover duration (Godwit: Conklin et al., 2010; Flycatcher: Briedis et al., 2016). Such differences among species could be explained by constraints faced during spring migration. Birds crossing major obstacles with limited possibilities to refuel en route may be more constrained during migration and may have to time their entire spring migration schedule to coincide with breeding site conditions (Conklin et al., 2010). Species facing fewer resource-constrained environments during migration, like American Golden-Plovers migrating mostly overland, may have the ability to exhibit more flexibility in migration schedules (Cornelius et al., 2013). Indeed, American Golden-Plovers can likely refuel regularly and adjust spring migration speed from the non-breeding areas to their last major stopover area in the nearctic temperate grasslands. Interestingly, the departure time from the non-breeding area seems negatively related to the breeding site latitude in the Pacific Golden-Plover (Pluvialis fulva), with birds wintering farther south and nesting later at higher latitudes, leaving their wintering areas earlier (Johnson et al., 2020b). Although this species is similar to the American Golden-Plover, it has a different migratory context and constraints, including a section of the migration over the Pacific Ocean, as well as population specific migratory routes and wintering locations (Johnson et al., 2020b).
A key step to unravel processes driving migratory movements involves determining the influence of endogenous control mechanisms that allows for optimal scheduling through the annual cycle (Robinson et al., 2010). Little is known about how migration schedules develop within individuals, and we still need to disentangle the effect of the environmental component from the genetic components (van Noordwijk et al., 2006). Although departure time from the last shared stopover areas could be partly inherited in American Golden-Plovers stopping-over in sympatry, we cannot exclude the possibility that individuals adjust their migration schedule based on their early life experience and past years breeding attempts (Mueller et al., 2013; Åkesson and Helm, 2020). Our study does not allow us to disentangle the potential role of such mechanisms. However, there is little support for the idea that individuals can learn from previous experience to better time nest initiation with snowmelt on the breeding grounds in shorebirds (Saalfeld and Lanctot, 2017).
If the timing of migration is inherited and adapted to the average timing of snowmelt at individual nesting locations, we could expect that a relatively high level of philopatry is required for such local adaptation to evolve as high gene flow could prevent local adaptation (Postma and van Noordwijk, 2005). Adults American Golden-Plovers, especially males, are faithful to their breeding area and usually return to the same breeding territory every year (Johnson et al., 2020a). High natal philopatry has been observed in some bird species (Wheelwright and Mauck, 1998) but the information available to date on American Golden-Plovers indicates low rates of natal philopatry (Saalfeld and Lanctot, 2015) and juvenile dispersal remains poorly documented (Johnson et al., 2020a). Under rapidly warming climatic conditions, if the rate of adjustment of migration schedule is not fast enough to follow environmental changes on the breeding grounds (Berteaux, 2004), dispersing individuals could have higher individual fitness if they could track suitable climatic breeding conditions (Visser, 2008).
Temperatures are rising faster in polar regions than anywhere else on earth (Hodgkins, 2014; Intergovernmental Panel on Climate Change, 2018), leading to earlier, warmer, and longer summers (Richter-Menge et al., 2019). Under a warmer climate, snowmelt advances (Eythorsson et al., 2019) with potential cascading effects on the timing of resource availability and reproductive events for arctic-nesting birds (Saalfeld and Lanctot, 2017; McGuire et al., 2020). However, the timing of snowmelt is advancing at different rates across the Arctic and a range-wide cline in the level of advancement in breeding resource availability is expected (Kwon et al., 2019). If the variation in the migration schedule of individuals co-occurring at the same non-breeding stopover areas is partly caused by genetic differences, we should expect substantial intraspecific heterogeneity in the strength of selection pressure on timing of migration. Our study helps to identify one critical phenological event that should be under strong selection (i.e., the schedule of the last northward migration segment) for the American Golden-Plover. We expect a stronger selection pressure for an earlier departure in breeding populations located in Alaska and western Canadian Arctic, where rapid increase in temperature should speed up snowmelt compared to populations breeding in northeastern Canada (Bush and Lemmen, 2019; Lenssen et al., 2019; GISTEMP Team, 2021). The ability for shorebirds to adapt and cope with rapid environmental changes currently occurring in the Arctic is poorly known. A better knowledge of their ability to learn from previous experiences and of the level of heritability of critical phenological events is needed.
Data Availability Statement
The datasets (animal tracks and metadata) for this study can be found in the Movebank repository [Movebank ID: 1454359936, Study Name: American Golden-Plover (Pluvialis dominica) migration from breeding range wide individuals].
Ethics Statement
The animal study was reviewed and approved by the Comité de Protection des Animaux de l’Université du Québec à Rimouski. Field methods, including handling and marking were approved by the relevant committees for animal care and all required permits for wildlife research were obtained (Parks Canada, Environment and Climate Change Canada, U.S. Fish and Wildlife Service, Government of Nunavut, Government of Alberta, United States and Canada Bird Banding Office).
Author Contributions
J-FL and JB conceptualized the study and analyzed the data. J-FL wrote the manuscript. J-FL, GG, JB, and RL contributed significantly to data interpretation and writing with contributions from all authors. All authors approved the final manuscript.
Funding
Funding was provided by: Fonds de Recherche du Québec – Nature et Technologies (FRQNT), Indigenous and Northern Affairs Canada, Natural Resources Canada (Polar Continental Shelf Program), Université du Québec à Rimouski, Natural Sciences and Engineering Research Council of Canada (NSERC), W. Garfield Weston Award for Northern Research and EnviroNord (NSERC CREATE Training Program in Northern Environmental Sciences), U.S. Fish and Wildlife Service, and Arctic Landscape Conservation Cooperative. Funding for the geolocators came notably from an NSERC – Research Tools and Instruments (RTI) award. Funding was also provided by the Liz Claiborne and Art Ortenberg Foundation, Bureau of Land Management, NMBCA U.S. Fish and Wildlife Service (Ikpikpuk River), Manomet Center for Conservation Sciences, NSERC grants to EN, Northern Research Fund, CNSC (Churchill), Environment and Climate Change Canada, NSERC, the W. Garfield Weston Foundation, and the Nunavut Wildlife Management Board National Fish and Wildlife Foundation, US Fish and Wildlife Service, Neotropical Migratory Bird Conservation Act, Manomet Inc. (Coats Island), Canada Research Program, NSERC, EnviroNord (NSERC CREATE Training Program in Northern Environmental Sciences), Canadian Foundation for Innovation, New Brunswick Innovation Foundation, Indigenous and Northern Affairs Canada, and Université de Moncton (Igloolik). This research was supported logistically by: Sirmilik National Park (Parks Canada), and by the Bylot Island Field Station of the Centre d’Études Nordiques (CEN) (Bylot Island), Barrow Arctic Science Commission and the UMIAQ, LLC (Utqiaġvik), Bureau of Land Management (Ikpikpuk River), Government of Alberta, Department of Environment and Parks, Fish and Wildlife Division (Caw Ridge), Churchill Northern Studies Centre (Churchill), Aiviit Hunters and Trappers Organization (Coats Island), Government of Nunavut, the Hunters and Trappers Organization of Igloolik (Igloolik).
Conflict of Interest
The authors declare that the research was conducted in the absence of any commercial or financial relationships that could be construed as a potential conflict of interest.
Publisher’s Note
All claims expressed in this article are solely those of the authors and do not necessarily represent those of their affiliated organizations, or those of the publisher, the editors and the reviewers. Any product that may be evaluated in this article, or claim that may be made by its manufacturer, is not guaranteed or endorsed by the publisher.
Acknowledgments
The Ukpeaġvik Iñupiat Corporation and the North Slope Borough kindly permitted us to conduct this research on their lands (Utqiaġvik). Most Canadian sites benefited from logistical support by the Polar Continental Shelf Program. For their help in the field we are extremely grateful particularly to: A. Beardsell, E. Chalifour, J. Otis, L. Paquette, M. Trudel, P. Royer-Boutin, C. Doucet, D.-J. Léandri-Breton and all that contributed to the Ecological Studies and Environmental Monitoring at Bylot Island, J. Cunningham, A. Doll, R. Dugan, P. Herzog, B. Hill, Y.-H. Hsu, and M. McConnell at Utqiaġvik, K. Overdujin, P. Brusseau, N. Brusseau, S. Gold, M. Weber, and R. Goodwill at Nome, K. Pietrzak, M. Mudge, R. Hammond, P. Chilton, and M. Dodds at Ikpikpuk River, D. Stepnisky, S. Robertson, D. Hobson, M. Bacon, D. Albright, R. Arbuckle, S. Stamler, M. Hervieux, and H. McKenzie at Caw Ridge, G. Holmes, A. Veldhoen, N. MacDonald, J. Robinson, J. Perz, C. Anderson, A. Johnson, R. Addley, and additional contributions from researchers based at the Churchill Northern Studies Centre at Churchill, S. Brown, S. Schulte, B. Winn, S. Neima, K. Reishke, M. Boothroyd, and S-L. Masson at Coats Island, J. Otis, M. C. Frenette, K. Peck, L. Gauvin, E. Brisson-Curadeau, M. Trottier-Paquet, M. Favreau, N. Uttak, M. Qrunuk, the whole community of Igloolik and all team members contributing to the ecosystem study at Igloolik Island, R. Porter, M.-C. Cadieux, E. Bolduc, and N. Casajus provided support with geolocator preparation, field logistics, data handling, and/or analysis. The Centre Intégré de Santé et de Services Sociaux du Bas-Saint-Laurent assisted with the ground truthing of geolocators. We also thank the external reviewers for their constructive comments on the manuscript.
Supplementary Material
The Supplementary Material for this article can be found online at: https://www.frontiersin.org/articles/10.3389/fevo.2021.710007/full#supplementary-material
References
Åkesson, S., and Helm, B. (2020). Endogenous programs and flexibility in bird migration. Front. Ecol. Evol. 8:78. doi: 10.3389/fevo.2020.00078
Åkesson, S., Ilieva, M., Karagicheva, J., Rakhimberdiev, E., Tomotani, B., and Helm, B. (2017). Timing avian long-distance migration: from internal clock mechanisms to global flights. Philos. Trans. R. Soc. B Biol. Sci. 372:20160252. doi: 10.1098/rstb.2016.0252
Alerstam, T., and Lindström, Å (1990). “Optimal bird migration: the relative importance of time, energy, and safety,” in Bird Migration, ed. P. D. E. Gwinner (Heidelberg: Springer Berlin Heidelberg), 331–351. doi: 10.1007/978-3-642-74542-3_22
Bates, D., Mächler, M., Bolker, B., and Walker, S. (2015). Fitting linear mixed effects models using lme4. J. Stat. Softw. 67, 1–48. doi: 10.18637/jss.v067.i01
Berteaux, D. (2004). Keeping pace with fast climate change: can arctic life count on volution? Integr. Comp. Biol. 44, 140–151. doi: 10.1093/icb/44.2.140
Briedis, M., Hahn, S., Gustafsson, L., Henshaw, I., Träff, J., Král, M., et al. (2016). Breeding latitude leads to different temporal but not spatial organization of the annual cycle in a long-distance migrant. J. Avian Biol. 47, 743–748. doi: 10.1111/jav.01002
Brown, S. C., Gates, H. R., Liebezeit, J. R., Smith, P. A., Hill, B. L., and Lanctot, R. B. (2014). Arctic Shorebird Demographics Network Breeding Camp Protocol, Version 5, April 2014. U.S. Fish and Wildlife Service and Manomet Center for Conservation Sciences (Unpublished Paper), 118. Available online at: https://www.manomet.org/project/arctic-shorebird-demographics-network-asdn/
Buehler, D. M., and Piersma, T. (2008). Travelling on a budget: predictions and ecological evidence for bottlenecks in the annual cycle of long-distance migrants. Philos. Trans. R. Soc. B 363, 247–266. doi: 10.1098/rstb.2007.2138
Bulla, M., Valcu, M., Dokter, A. M., Dondua, A. G., Kosztolányi, A., Rutten, A. L., et al. (2016). Unexpected diversity in socially synchronized rhythms of shorebirds. Nature 540, 109–113. doi: 10.1038/nature20563
Bush, E., and Lemmen, D. S. (2019). Canada’s Changing Climate Report. Ottawa, Ont: Government of Canada.
Byrkjedal, I., and Thompson, D. B. A. (1998). Tundra Plovers: The Eurasian, Pacific and American Golden Plovers and Grey Plover. London: T & AD Poyser Ltd.
Chung, Y., Rabe-Hesketh, S., Dorie, V., Gelman, A., and Liu, J. (2013). A non-degenerate penalized likelihood estimator for variance parameters in multilevel models. Psychometrika 78, 685–709. doi: 10.1007/s11336-013-9328-2
Clausen, K. K., Madsen, J., and Tombre, I. M. (2015). Carry-over or compensation? The impact of winter harshness and post-winter body condition on spring-fattening in a migratory goose species. Plos One 10:e0132312. doi: 10.1371/journal.pone.0132312
Conklin, J. R., Battley, P. F., Potter, M. A., and Fox, J. W. (2010). Breeding latitude drives individual schedules in a trans-hemispheric migrant bird. Nat. Commun. 1:67. doi: 10.1038/ncomms1072
Cornelius, J. M., Boswell, T., Jenni-Eiermann, S., Breuner, C. W., and Ramenofsky, M. (2013). Contributions of endocrinology to the migration life history of birds. Gen. Comp. Endocrinol. 190, 47–60. doi: 10.1016/j.ygcen.2013.03.027
Cristol, D. A., Baker, M. B., and Carbone, C. (1999). “Differential migration revisited,” in Current Ornithology, eds V. Nolan, E. D. Ketterson, and C. F. Thompson (Boston, MA: Springer US), 33–88. doi: 10.1007/978-1-4757-4901-4_2
Duong, T., Wand, M., Chacon, J., and Gramacki, A. (2021). ks: Kernel Smoothing. Available online at: https://CRAN.R-project.org/package = ks (accessed February 9, 2021).
Ely, C. R., McCaffery, B. J., and Gill, R. E. (2018). “Shorebirds adjust spring arrival schedules with variable environmental conditions: four decades of assessment on the Yukon–Kuskokwim Delta, Alaska,” in Trends and Traditions: Avifaunal Change in Western North America, eds W. D. Shuford, R. E. Gill, and C. M. Handel (Long Beach, CA: Western Field Ornithologists), 296–311. doi: 10.21199/SWB3.16
Eythorsson, D., Gardarsson, S. M., Ahmad, S. K., Hossain, F., and Nijssen, B. (2019). Arctic climate and snow cover trends comparing global circulation models with remote sensing observations. Int. J. Appl. Earth Obs. Geoinf. 80, 71–81. doi: 10.1016/j.jag.2019.04.003
Finch, T., Saunders, P., Avilés, J. M., Bermejo, A., Catry, I., de la Puente, J., et al. (2015). A pan-european, multipopulation assessment of migratory connectivity in a near-threatened migrant bird. Divers. Distrib. 21, 1051–1062. doi: 10.1111/ddi.12345
Fraser, K. C., Silverio, C., Kramer, P., Mickle, N., Aeppli, R., and Stutchbury, B. J. M. (2013). A trans-hemispheric migratory songbird does not advance spring schedules or increase migration rate in response to record-setting temperatures at breeding sites. PLoS One 8:e64587. doi: 10.1371/journal.pone.0064587
GISTEMP Team. (2021). Data.GISS: GISS Surface Temperature Analysis (v4): Global Maps. Available online at: https://data.giss.nasa.gov/gistemp/maps/index_v4.html (accessed April 14, 2021).
Gow, E. A. (2016). Analyzing geolocator data for birds that roost in cavities year-round. J. Field Ornithol. 87, 74–83. doi: 10.1111/jofo.12130
Grabowski, M. M., Doyle, F. I., Reid, D. G., Mossop, D., and Talarico, D. (2013). Do arctic-nesting birds respond to earlier snowmelt? A multi-species study in north Yukon, Canada. Polar Biol. 36, 1097–1105. doi: 10.1007/s00300-013-1332-6
Hijmans, R. J., Williams, E., Vennes, C., and Hijmans, M. R. J. (2015). Package ‘Geosphere. Wien: R Foundation.(R Foundation Rapport) Tillgänglig.
Hobson, K. A., and Jehl, J. R. Jr. (2010). Arctic waders and the capital-income continuum: further tests using isotopic contrasts of egg components. J. Avian Biol. 41, 565–572. doi: 10.1111/j.1600-048x.2010.04980.x
Hobson, K. A., and Kardynal, K. J. (2015). Western Veeries use an eastern shortest-distance pathway: new insights to migration routes and phenology using light-level geolocators. The Auk 132, 540–550. doi: 10.1642/AUK-14-260.1
Hodgkins, R. (2014). The twenty-first-century arctic environment: accelerating change in the atmospheric, oceanic and terrestrial spheres: commentary. Geogr. J. 180, 429–436. doi: 10.1111/geoj.12112
Intergovernmental Panel on Climate Change (2018). Global Warming of 1.5°C. Available online at: http://www.ipcc.ch/report/sr15/ (accessed April 1, 2019).
Johnson, O. W., and Morton, M. L. (1976). Fat content and flight range in shorebirds summering on Enewetak Atoll. Condor 78, 144–145. doi: 10.2307/1366949
Johnson, O. W., Connors, P. G., and Pyle, P. (2020a). “American golden-plover (Pluvialis dominica),” in Birds of the World, ed. P. G. Rodewald (Ithaca, NY: Cornell Lab of Ornithology), doi: 10.2173/bow.amgplo.01
Johnson, O. W., Tibbitts, T. L., Weber, M. F., Bybee, D. R., Goodwill, R. H., Bruner, A. E., et al. (2020b). Tracking the migration of Pacific Golden-Plovers from non-breeding grounds at Moorea, French Polynesia, using pinpoint GPS-Argos tags. Wader Study 127, 53–59. doi: 10.18194/ws.00172
Ketterson, E. D., Fudickar, A. M., Atwell, J. W., and Greives, T. J. (2015). Seasonal timing and population divergence: when to breed, when to migrate. Curr. Opin. Behav. Sci. 6, 50–58. doi: 10.1016/j.cobeha.2015.09.001
Komsta, L. (2011). Outliers: Tests for Outliers. Available online at: https://CRAN.R-project.org/package = outliers (accessed August 8, 2018).
Kwon, E., Weiser, E. L., Lanctot, R. B., Brown, S. C., Gates, H. R., Gilchrist, G., et al. (2019). Geographic variation in the intensity of warming and phenological mismatch between Arctic shorebirds and invertebrates. Ecol. Monogr. 89, 1–20. doi: 10.1002/ecm.1383
Lenssen, N. J., Schmidt, G. A., Hansen, J. E., Menne, M. J., Persin, A., Ruedy, R., et al. (2019). Improvements in the GISTEMP uncertainty model. J. Geophys. Res. Atmospheres 124, 6307–6326. doi: 10.1029/2018jd029522
Liebezeit, J. R., Gurney, K. E. B., Budde, M., Zack, S., and Ward, D. (2014). Phenological advancement in arctic bird species: relative importance of snow melt and ecological factors. Polar Biol. 37, 1309–1320. doi: 10.1007/s00300-014-1522-x
Liebezeit, J. R., Smith, P. A., Lanctot, R. B., Schekkerman, H., Tulp, I., Kendall, S. J., et al. (2007). Assessing the development of shorebird eggs using the flotation method: species-specific and generalized regression models. Condor 109, 32–47. doi: 10.1650/0010-5422(2007)109[32:atdose]2.0.co;2
Lisovski, S., Wotherspoon, S., Sumner, M., Bauer, S., and Emmenegger, T. (2015). GeoLight: Analysis of Light Based Geolocator Data. Available online at: https://cran.r-project.org/web/packages/GeoLight/index.html (accessed February 3, 2017).
Loon, A. V., Ray, J. D., Savage, A., Mejeur, J., Moscar, L., Pearson, M., et al. (2017). Migratory stopover timing is predicted by breeding latitude, not habitat quality, in a long-distance migratory songbird. J. Ornithol. 158, 745–752. doi: 10.1007/s10336-017-1435-x
Marra, P. P. (1998). Linking winter and summer events in a migratory bird by using stable-carbon isotopes. Science 282, 1884–1886. doi: 10.1126/science.282.5395.1884
McGuire, R. L., Lanctot, R. B., Saalfeld, S. T., Ruthrauff, D. R., and Liebezeit, J. R. (2020). Shorebird reproductive response to exceptionally early and late springs varies across sites in Arctic Alaska. Front. Ecol. Evol 8:577652. doi: 10.3389/fevo.2020.577652
Mueller, T., O’Hara, R. B., Converse, S. J., Urbanek, R. P., and Fagan, W. F. (2013). Social learning of migratory performance. Science 341, 999–1002. doi: 10.1126/science.1237139
Nakagawa, S., and Schielzeth, H. (2013). A general and simple method for obtaining R2 from generalized linear mixed-effects models. Methods Ecol. Evol. 4, 133–142. doi: 10.1111/j.2041-210x.2012.00261.x
Niehaus, A. C., and Ydenberg, R. C. (2006). Ecological factors associated with the breeding and migratory phenology of high-latitude breeding western sandpipers. Polar Biol. 30, 11–17. doi: 10.1007/s00300-006-0154-1
O’Leary, D. III, Hall, D. K., Medler, M., Matthews, R., and Flower, A. (2017). Snowmelt Timing Maps Derived from MODIS for North America, 2001-2015. Oak Ridge, TEN: ORNL DAAC. doi: 10.3334/ORNLDAAC/1504
Pedersen, L., Jackson, K., Thorup, K., and Tøttrup, A. P. (2018). Full-year tracking suggests endogenous control of migration timing in a long-distance migratory songbird. Behav. Ecol. Sociobiol 72:139. doi: 10.1007/s00265-018-2553-z
Postma, E., and van Noordwijk, A. J. (2005). Gene flow maintains a large genetic difference in clutch size at a small spatial scale. Nature 433, 65–68. doi: 10.1038/nature03083
R Core Team (2019). R: A Language and Environment for Statistical Computing. Vienna, WIEN: R Foundation for Statistical Computing.
Rakhimberdiev, E., Duijns, S., Karagicheva, J., Camphuysen, C. J., Castricum, V. R. S., Dekinga, A., et al. (2018). Fuelling conditions at staging sites can mitigate Arctic warming effects in a migratory bird. Nat. Commun. 9:4263. doi: 10.1038/s41467-018-06673-5
Richter-Menge, J., Druckenmiller, M. L., and Jeffries, M. (eds) (2019). Arctic Report Card 2019. Available online at: www.arctic.noaa.gov/Report-Card (accessed July 15, 2020).
Robinson, W. D., Bowlin, M. S., Bisson, I., Shamoun-Baranes, J., Thorup, K., Diehl, R. H., et al. (2010). Integrating concepts and technologies to advance the study of bird migration. Front. Ecol. Environ. 8, 354–361. doi: 10.1890/080179
Saalfeld, S. T., and Lanctot, R. B. (2015). Conservative and opportunistic settlement strategies in arctic-breeding shorebirds. The Auk 132, 212–234. doi: 10.1642/AUK-13-193.1
Saalfeld, S. T., and Lanctot, R. B. (2017). Multispecies comparisons of adaptability to climate change: a role for life-history characteristics? Ecol. Evol. 7, 10492–10502. doi: 10.1002/ece3.3517
Sibert, J., and Nielsen, A. (2002). kftrack: an add-on package for the statistical environment R to estimate most probable track from archival tagged individuals. Honolulu, HI: Pelagic Fisheries Research Program University of Hawaii at Manoa Honol.
Smith, P. A., Gilchrist, H. G., Forbes, M. R., Martin, J.-L., and Allard, K. (2010). Inter-annual variation in the breeding chronology of arctic shorebirds: effects of weather, snow melt and predators. J. Avian Biol. 41, 292–304. doi: 10.1111/j.1600-048X.2009.04815.x
Stanley, C. Q., MacPherson, M., Fraser, K. C., McKinnon, E. A., and Stutchbury, B. J. M. (2012). Repeat tracking of individual songbirds reveals consistent migration timing but flexibility in route. PLoS One 7:e40688. doi: 10.1371/journal.pone.0040688
Trierweiler, C., Klaassen, R. H., Drent, R. H., Exo, K.-M., Komdeur, J., Bairlein, F., et al. (2014). Migratory Connectivity and Population Specific Migration Routes in a Long-Distance Migratory Bird. Available online at: http://cees.eldoc.ub.rug.nl/FILES/root/2014/ProcRSocBTrierweiler/2014ProcRSocBTrierweiler.pdf (accessed May 21, 2015).
van Gils, J. A., Lisovski, S., Lok, T., Meissner, W., Ozarowska, A., de Fouw, J., et al. (2016). Body shrinkage due to arctic warming reduces red knot fitness in tropical wintering range. Science 352, 819–821. doi: 10.1126/science.aad6351
van Noordwijk, A. J., Pulido, F., Helm, B., Coppack, T., Delingat, J., Dingle, H., et al. (2006). A framework for the study of genetic variation in migratory behaviour. J. Ornithol. 147, 221–233. doi: 10.1007/s10336-005-0047-z
Visser, M. E. (2008). Keeping up with a warming world; assessing the rate of adaptation to climate change. Proc. R. Soc. B Biol. Sci. 275, 649–659. doi: 10.1098/rspb.2007.0997
Weiser, E. L., Lanctot, R. B., Brown, S. C., Gates, H. R., Bentzen, R. L., Bêty, J., et al. (2018). Environmental and ecological conditions at arctic breeding sites have limited effects on true survival rates of adult shorebirds. The Auk 135, 29–43. doi: 10.1642/AUK-17-107.1
Wheelwright, N. T., and Mauck, R. A. (1998). Philopatry, natal dispersal, and inbreeding avoidance in an island population of savannah sparrows. Ecology 79, 755–767. doi: 10.2307/176576
Keywords: phenology, snowmelt, trans-hemispheric migrant, arctic birds, timing of breeding, American Golden-Plover
Citation: Lamarre J-F, Gauthier G, Lanctot RB, Saalfeld ST, Love OP, Reed E, Johnson OW, Liebezeit J, McGuire R, Russell M, Nol E, Koloski L, Sanders F, McKinnon L, Smith PA, Flemming SA, Lecomte N, Giroux M-A, Bauer S, Emmenegger T and Bêty J (2021) Timing of Breeding Site Availability Across the North-American Arctic Partly Determines Spring Migration Schedule in a Long-Distance Neotropical Migrant. Front. Ecol. Evol. 9:710007. doi: 10.3389/fevo.2021.710007
Received: 14 May 2021; Accepted: 22 September 2021;
Published: 21 October 2021.
Edited by:
Cecilia Nilsson, University of Copenhagen, DenmarkReviewed by:
Theunis Piersma, University of Groningen, NetherlandsMartha Maria Sander, University of Turin, Italy
Copyright © 2021 Lamarre, Gauthier, Lanctot, Saalfeld, Love, Reed, Johnson, Liebezeit, McGuire, Russell, Nol, Koloski, Sanders, McKinnon, Smith, Flemming, Lecomte, Giroux, Bauer, Emmenegger and Bêty. This is an open-access article distributed under the terms of the Creative Commons Attribution License (CC BY). The use, distribution or reproduction in other forums is permitted, provided the original author(s) and the copyright owner(s) are credited and that the original publication in this journal is cited, in accordance with accepted academic practice. No use, distribution or reproduction is permitted which does not comply with these terms.
*Correspondence: Jean-François Lamarre, Jean-Francois.Lamarre@polar-polaire.gc.ca