- 1Marine Science Group, Department of Biological, Geological and Environmental Sciences, University of Bologna, Bologna, Italy
- 2Fano Marine Center, The Inter-Institute Center for Research on Marine Biodiversity, Resources and Biotechnologies, Fano, Italy
- 3Animal and Environmental Physiology Laboratory, Department of Biological, Geological and Environmental Sciences, University of Bologna, Ravenna, Italy
The quality of data collected by non-professional volunteers in citizen science programs is crucial to render them valid for implementing environmental resources management and protection plans. This study assessed the reliability of data collected by non-professional volunteers during the citizen science project Scuba Tourism for the Environment (STE), carried out in mass tourism facilities of the Red Sea between 2007 and 2015. STE involved 16,164 volunteer recreational divers in data collection on marine biodiversity using a recreational citizen science approach. Through a specifically designed questionnaire, volunteers indicated which of the seventy-two marine taxa surveyed were observed during their recreational dive, giving an estimate of their abundance. To evaluate the validity of the collected data, a reference researcher randomly dived with the volunteers and filled in the project questionnaire separately. Correlation analyses between the records collected by the reference researcher and those collected by volunteers were performed based on 513 validation trials, testing 3,138 volunteers. Data reliability was analyzed through 7 parameters. Consistency showed the lowest mean score (51.6%, 95% Confidence Interval CI 44.1–59.2%), indicating that volunteers could direct their attention to different taxa depending on personal interests; Percent Identified showed the highest mean score (66.7%, 95% CI 55.5–78.0), indicating that volunteers can correctly identify most surveyed taxa. Overall, results confirmed that the recreational citizen science approach can effectively support reliable data for biodiversity monitoring, when carefully tailored for the volunteer skills required by the specific project. The use of a recreational approach enhances massive volunteer participation in citizen science projects, thus increasing the amount of sufficiently reliable data collected in a reduced time.
Introduction
Institutions and natural resource managers are often under fund restrictions, which odds with the need to collect fundamental data to implement conservation strategies (Lewis, 1999; Foster-Smith and Evans, 2003; Jetz et al., 2012; Forrester et al., 2015; McKinley et al., 2017). Effective conservation strategies must also integrate public input and engagement in designing solutions (McKinley et al., 2017). Involving volunteers in data collection for monitoring activities can be a cost-effective strategy to complement or replace the information collected by professionals (Starr et al., 2014). Citizen science projects can improve environmental education of volunteers, increase scientific knowledge and allow the collection of large datasets (Foster-Smith and Evans, 2003; Bonney et al., 2009; Sullivan et al., 2009; Jordan et al., 2011; Branchini et al., 2015b; Callaghan et al., 2019). Participating in a citizen science project can have an educational role both in the short and long term, with the retention of acquired environmental awareness after years (Branchini et al., 2015a; Meschini et al., 2021).
Observations of the natural world, including weather information, plants and animals distribution, astronomical phenomena and many other data have been recorded for decades by citizens (Miller-Rushing et al., 2012; Bonney et al., 2014). One emblematic example come from ornithology, with the Audubon Society’s annual Christmas bird counts, started in 1900 and it still engaging 60–80,000 volunteers annually (Forrester et al., 2015). Nowadays millions of volunteers are participating in many scientific research projects by collecting, categorizing, transcribing and analyzing data (Dickinson et al., 2012; Callaghan et al., 2019). Ultimately, citizen science presents an enormous potential to influence policy and guide resource management by producing datasets that would be otherwise unobtainable (Kosmala et al., 2016).
Citizen science is blooming across a range of disciplines in natural and social sciences, as well as humanities (Lukyanenko et al., 2019). A large body of environmental research is based on citizen science (e.g., biology, conservation and ecology); anyway, the development of information and communication technologies (ICT) have expanded the scale and scope of data collection from geographic information research (e.g., projects for geographic data collection) to social sciences and epidemiology studies (e.g., projects that study the relationship between environmental issues and human health) (Kullenberg and Kasperowski, 2016; Hecker et al., 2018). Citizen science is becoming of central importance to reinforce literacy and societal trust in science and foster participatory and transparent decision-making1. It is also gaining an increasing interest for policy makers, government officials and non-governmental organizations (Turbé et al., 2019). Data collected through citizen science are a non-traditional data source that is giving a contribution to measure the United Nations (UN) Sustainable Development Goals (Fritz et al., 2019). The role of citizens is becoming central also in European Union (EU) policies, such as the Horizon 2020 funding program2. The next European Research and Innovation Program Horizon Europe includes a specific mission supporting this process by connecting citizens with science and public policy3. In the Mission Starfish 2030 program, citizens are protagonists of one of the five overarching objectives for 2030 and one goal of this program for the 2025 checkpoint, is that 20% of data collection comes from citizen science initiatives4. Those are some examples of the increasing importance that citizen science is gaining in European funding programs, where citizen science will be a transversal topic to all missions.
Citizen science projects vary extensively in subject matter, objectives, activities, and scale, but the common goal is collecting reliable data to be used for scientific and policy making purposes for implementing environmental management and protection plans (Forrester et al., 2015; Van der Velde et al., 2017). Volunteers involved in citizen science projects can produce data with sufficient to high accuracy (Foster-Smith and Evans, 2003; Goffredo et al., 2010; Kosmala et al., 2016), although some cases of insufficient volunteer data quality have been reported (Foster-Smith and Evans, 2003; Galloway et al., 2006; Delaney et al., 2008; Silvertown, 2009; Hunter et al., 2013).
Data collection in citizen science projects usually addresses easy-to-recognize organisms, with interest on qualitative and semi-quantitative data that can be useful for management plans (Bramanti et al., 2011). The marine environment data collection is particularly challenging because it requires swimming or scuba diving skills in addition to the usual sampling difficulties (Goffredo et al., 2004, 2010; Gillett et al., 2012; Forrester et al., 2015). Citizen science in the marine environment can be used to monitor shallow water organisms (up to 40 meters depth, the Professional Association of Diving Instructors (PADI) limit for recreational scuba skills) over a large geographical and temporal extension (Goffredo et al., 2010; Bramanti et al., 2011; Gommerman and Monroe, 2012). Several studies analyzed the correlation between data collected by professionals and volunteers on a single taxonomic group, such as fishes (Darwall and Dulvy, 1996; Holt et al., 2013), e.g., sharks (Ward-Paige and Lotze, 2011) or corals (Bramanti et al., 2011; Marshall et al., 2012; Forrester et al., 2015) showing that volunteers were able to collect good quality data that could be used to complement professional data and describe population trends in spatial and temporal scales.
The aim of this study was to replicate the standardized methodology used in Goffredo et al. (2010) and Branchini et al. (2015b) to assess the quality of data collected by non-specialist volunteers on seventy-two Red Sea taxa during the recreational citizen science project Scuba Tourism for the Environment (STE). Previous reported studies were, respectively, based on 38 and 61 validation trials, in this study we analyzed 513 validation trials mainly performed in Egypt between 2007 and 2015. Our study used a recreational survey protocol based on casual diver observations. This protocol allowed divers to carry out their normal recreational activities and ensured the reliability of collected data through standardized data collection (Branchini et al., 2015b). To evaluate the possible influence of independent variables (date, team size, diving certification level, depth and dive time on volunteers data quality, we used correlation analyses using Spearman rank correlation and distance-based redundancy linear modeling (DISTLM) to test the contributions of independent variables to data variability.
Materials and Methods
From 2007 to 2015 16,164 recreational scuba divers in mass tourism facilities and diving centers in the Red Sea were involved in the citizen science project Scuba Tourism for the Environment (STE). Project goal was to monitor coral reef biodiversity in the Red Sea, using specifically developed illustrated questionnaires. A first section of the questionnaire was dedicated to volunteer environmental education to limit human impact on the reef and increase volunteer awareness on the vulnerability of coral reefs (Supplementary Figure 1). The second section of the questionnaire consisted in seventy-two photographs of target taxa, chosen because they are: (i) representative of the main ecosystem trophic levels, (ii) expected to be common and abundant in the Red Sea, and (iii) easily recognizable by non-specialist volunteers (Supplementary Figure 2). These characteristics were selected to increase the accuracy of data collected by volunteers (Goffredo et al., 2004, 2010). The third section of the questionnaire was dedicated to the collection of personal information (i.e., name, address, email, level of diving certification and diving agency), technical information about the dive (i.e., place, date, depth, dive time, duration of the dive), type of habitat explored (i.e., rocky bottom, sandy bottom or other habitat) and the data collection table about sighted taxa with an estimation of their abundance (Supplementary Figure 3). The abundance estimation of each taxon was based on literature (Wielgus et al., 2004) and databases5, and expressed in the three categories “rare,” “frequent” or “abundant.” Completing questionnaires shortly after the dive facilitated the quality control of collected data. The STE project used a recreational citizen science approach (Goffredo et al., 2004, 2010; Branchini et al., 2015b) in which normal recreational diving features and volunteer behavior are not modified by project participation. Researchers of the STE project performed an annual training session for scuba instructors of the diving centers involved in the project, based on the methodology used for the study and obtained results. This allowed scuba instructors to directly involve their clients in data collection. The STE project received the approval of the Bioethics Committee of the University of Bologna (prot. 2.6). Data were treated confidentially, exclusively for institutional purposes (art. 4 of Italian legislation D.R. 271/2009 – single text on privacy and the use of IT systems). Data treatment and reporting took place in aggregate form.
Data Validity Assessment
To assess the validity of data collected by volunteers, records of 3,138 volunteer were compared with those collected by a marine biologist of the Marine Science Group of the University of Bologna (“control diver”) during 513 validation trials mainly performed in Egypt (Figure 1). The characteristics of the validation trials were: (1) the control diver dived with at least three volunteers; (2) the validation trial did not affect the diving center normal choice of dive site; (3) the dive was conducted between 9.00 am and 4.00 pm; (4) after the dive, the control diver filled in the questionnaire apart from volunteers, as to avoid interference with volunteers data recording (Goffredo et al., 2010). For each trial, the inventory of each taxa (with abundance ratings) sighted by the control diver was correlated with that collected by each volunteer to verify their similarity (Darwall and Dulvy, 1996; Foster-Smith and Evans, 2003; Aceves-Bueno et al., 2017). To measure the quality of volunteer data, 7 reliability parameters were used: Accuracy, Consistency, Percent Identified, Correct Identification, Correctness of Abundance Ratings, Similarity, Reliability (Table 1). Non-parametric statistical tests were used for the analysis: (1) Spearman rank correlation coefficient, to evaluate the accuracy of data collected by volunteers in comparison to those obtained by the control diver; (2) Cronbach’s alpha (α) correlation, to evaluate the reliability of collected data between each volunteer and the control diver; and (3) Czekanowski proportional similarity index (SI) to obtain a measure of similarity between each volunteer and the control diver ratings (Goffredo et al., 2010). Tests results were reported as mean with 95% Confidence Interval (CI) (Sale and Douglas, 1981; Darwall and Dulvy, 1996). For the Similarity and Reliability parameters the lower bound (calculated from 95% Confidence Interval (CI) of the mean values) was used (Goffredo et al., 2010). We also examined the effect of date, team size (the number of participants present in each validation trial), diving certification level of each participant, depth and dive time on volunteer accuracy using the Spearman’s rank correlation coefficient. All these statistical analyses were computed using the SPSS 22.0 statistical software. Using PRIMER v6, distance-based redundancy linear modeling (DISTLM) with a test of marginality was also performed, based on Euclidean distance, to test the contributions of variables to data variability.
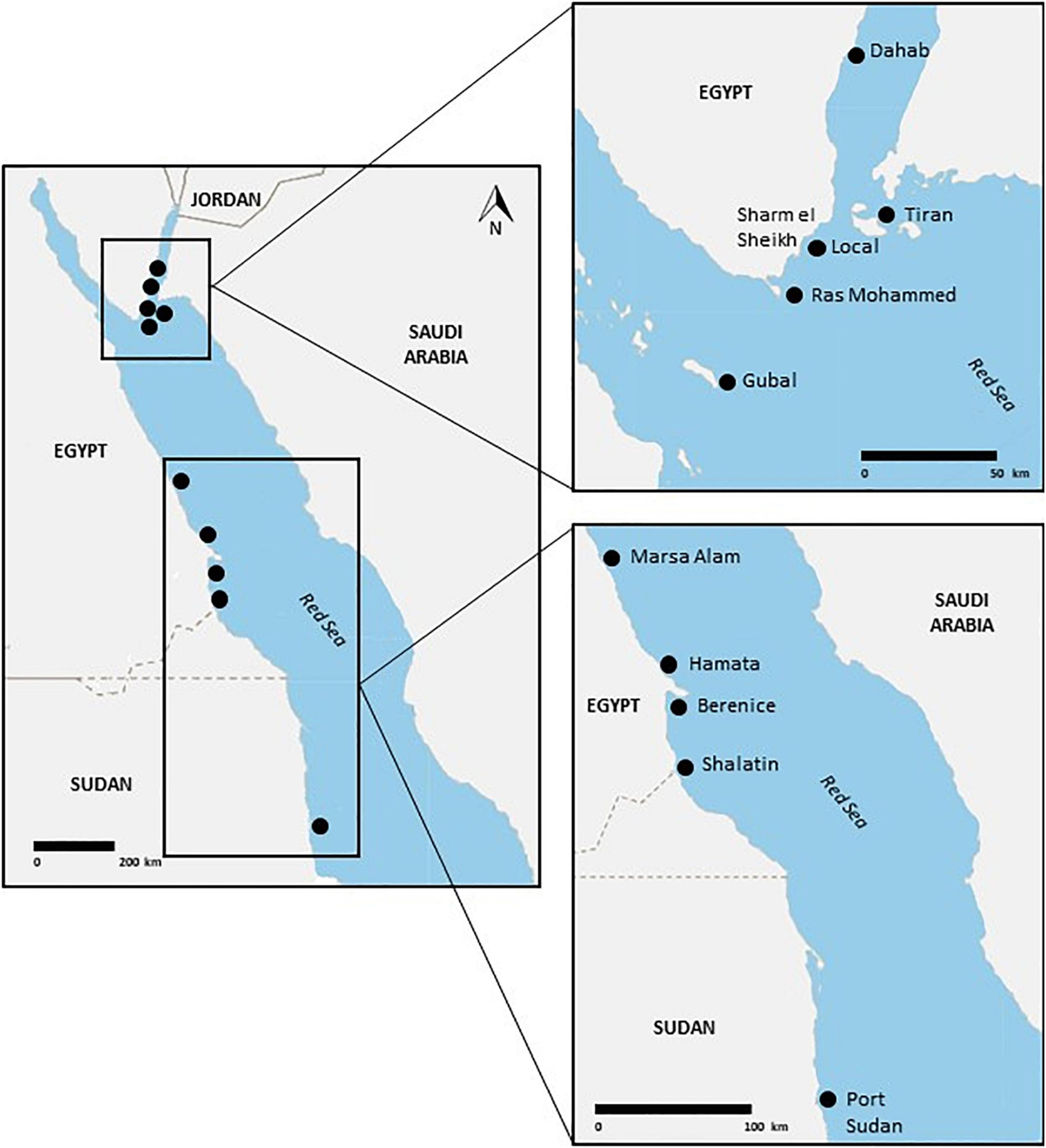
Figure 1. Red Sea map with black dots indicating sites in which data for the reliability analysis were collected.
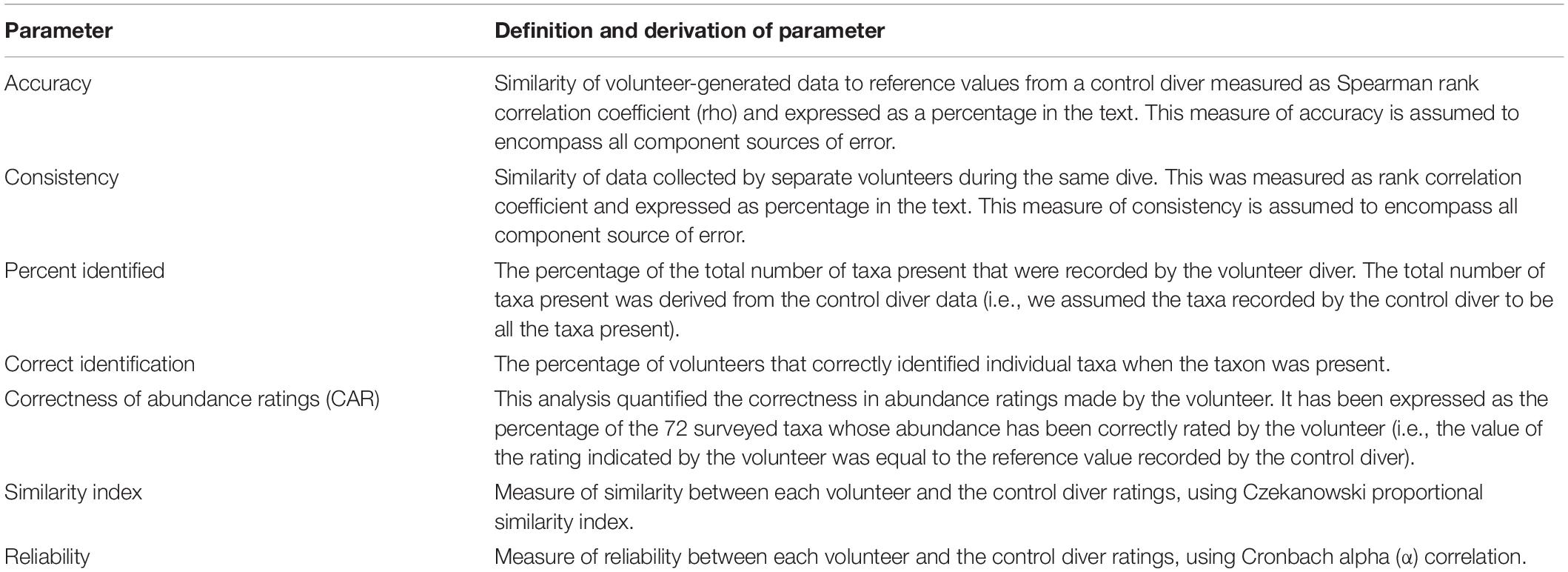
Table 1. Reliability parameters used to analyze data collected by volunteers (modified from Goffredo et al., 2010).
Results
The mean accuracy of each validation trial ranged from 38.2 to 81.5%, with 94.2% of trials with mean accuracy between 40 and 70% (Supplementary Table 1; Figure 2). Accuracy was positively correlated with: date (ρs = 0.120, N = 513, p < 0.01, Table 2; Figure 3), volunteers scores increased with years, with a score increase of 2.8% between the start and the end of the project (Table 3); volunteer diving certification level (ρs = 0.242, N = 513, p < 0.001, Table 2; Figure 4), volunteers scores increased with higher divers certification level, with an increase of 17.3% between beginners and professional divers (Table 3); dive time (ρs = 0.122, N = 513, p < 0.01, Table 2; Figure 4), volunteers scores increased with time spent underwater, with an increase of 11.6% between short and long dives (Table 3). Accuracy was not correlated with team size (ρs = 0.063, N = 513, p = 0.151, Table 2) and depth (ρs = −0.022, N = 513, p = 0.620, Table 2).
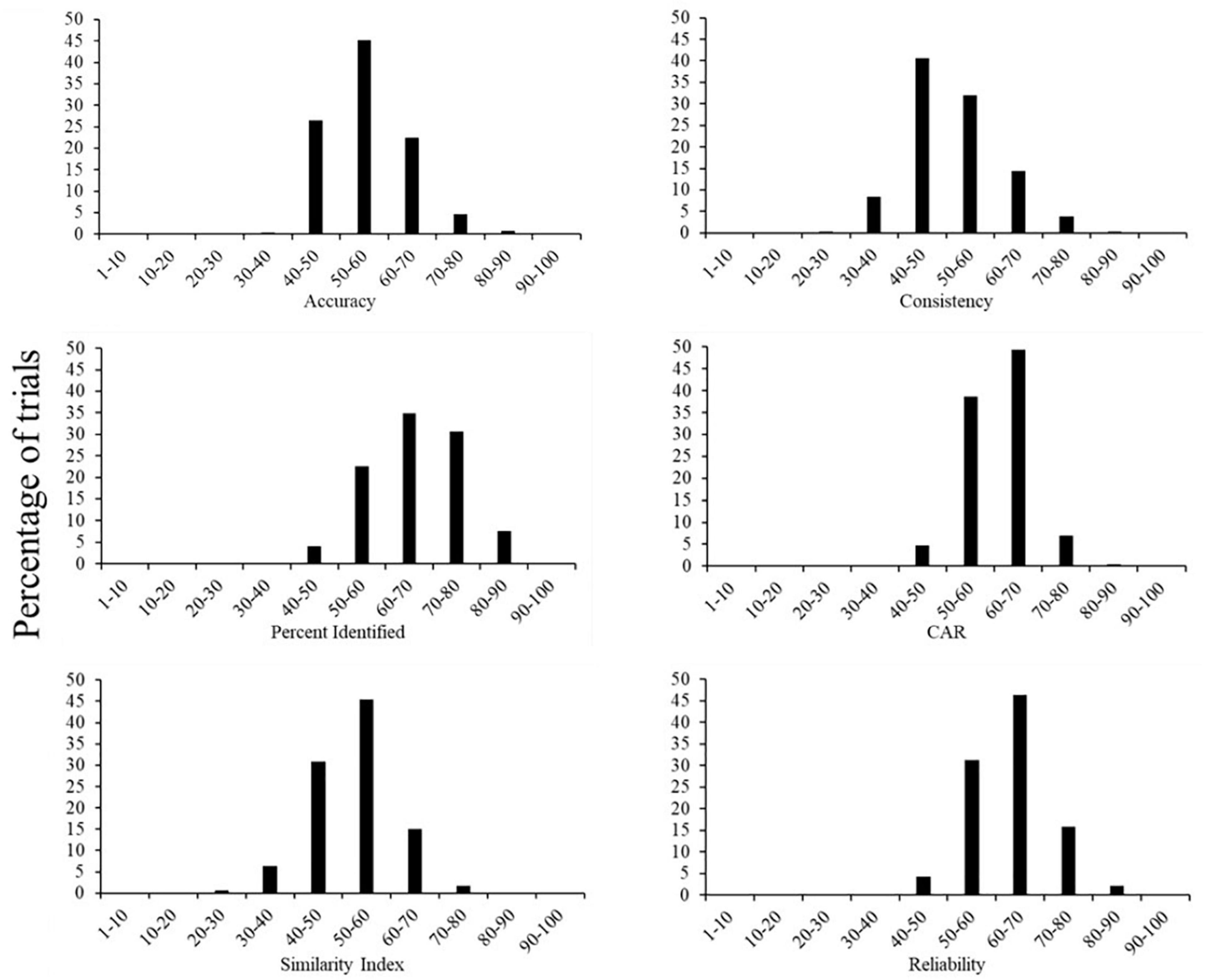
Figure 2. Quality of data collected by volunteers in the 513 validation trials performed during the 9-year research project STE (2007–2015). Distribution of data is divided in classes depending on the mean score percentage that each validation trial achieved for the studied parameters. For the parameters Similarity Index and Reliability the reference score is the lower bound calculated from 95% CI of the mean values.
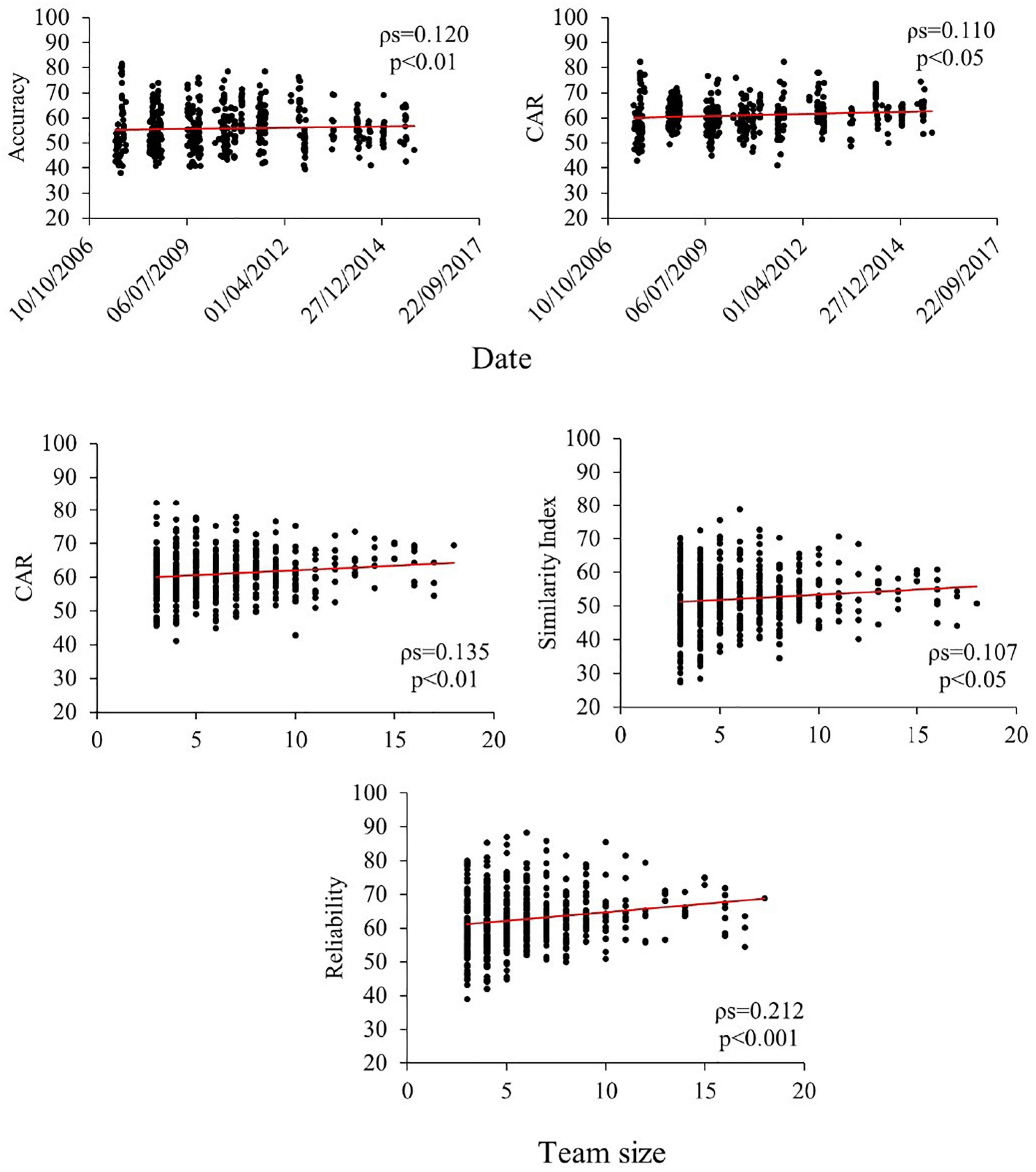
Figure 3. Significant correlations between reliability parameters (Accuracy, CAR, Reliability, and Similarity Index) and independent variables (Date and Team Size). Results based on the 513 validation trials. Indicated in red the trendline of the correlations. ρs = Spearman correlation coefficient.
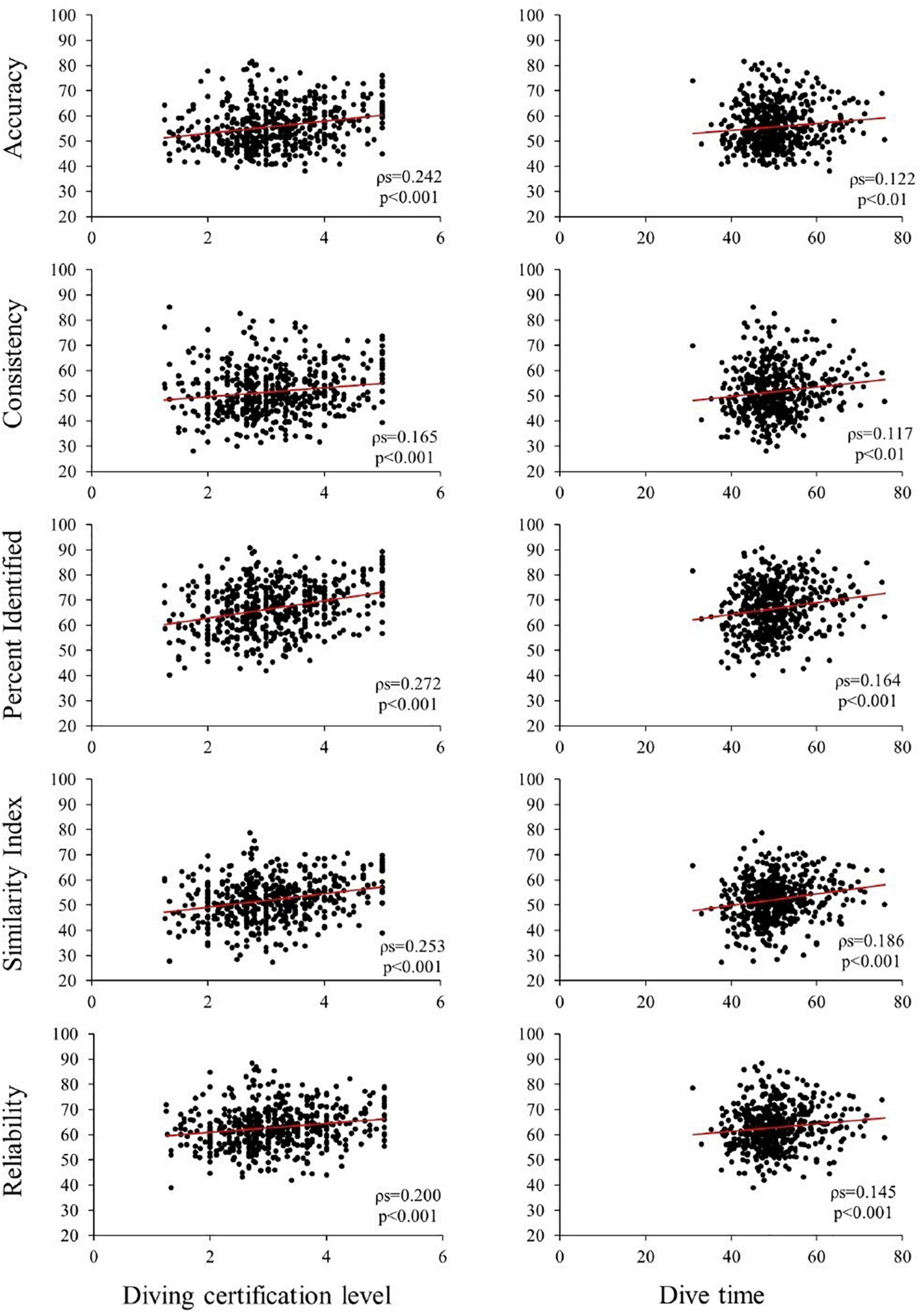
Figure 4. Significant correlations between the studied reliability parameters (Accuracy, Consistency, Percent Identified, Similarity Index, and Reliability) and the independent variables Diving certification level and Dive time. Results based on the 513 validation trials. Indicated in red the trendline of the correlations. ρs = Spearman coefficient value.
The mean consistency of each validation trial ranged from 28.0 to 85.3%, with 86.9% of trials with mean consistency between 40 and 70% (Supplementary Table 1; Figure 2). Consistency was positively correlated with: volunteer diving certification level (ρs = 0.165, N = 513, p < 0.001, Table 2; Figure 4), volunteers scores increased with higher divers certification level, with a score increase of 13.6% between beginners and professional divers (Table 3); dive time (ρs = 0.117, N = 513, p < 0.01, Table 2; Figure 4), volunteers scores increased with time spent underwater, with an increase of 17.7% between short and long dives (Table 3). Consistency was not correlated with date (ρs = −0.022, N = 513, p = 0.615, Table 2), team size (ρs = −0.077, N = 513, p = 0.81, Table 2) and depth (ρs = −0.049, N = 513, p = 0.271, Table 2).
The mean percent identified of each validation trial ranged from 40.2 to 90.9%, with 88.1% of trials with mean percentage of identified between 50 and 80% (Supplementary Table 1; Figure 2). Percent identified was positively correlated with: volunteer diving certification level (ρs = 0.272, N = 513, p < 0.001, Table 2; Figure 4), volunteers scores increased with higher divers certification level, with a score increase of 21.4% between beginners and professional divers (Table 3); dive time (ρs = 0.164, N = 513, p < 0.001, Table 2; Figure 4), volunteers scores increased with time spent underwater, with an increase of 17.1% between short and long dives (Table 3). Percent identified was not correlated with date (ρs = −0.005, N = 513, p = 0.904, Table 2), team size (ρs = −0.020, N = 513, p = 0.656, Table 2) and depth (ρs = 0.009, N = 513, p = 0.831, Table 2).
The mean correct identification of each taxon varied from 3.8 to 94.7%, with a positive correlation between the number of validation trials in which the taxon was present and the level of correct identification performed by volunteers (ρs = 0.610, N = 77, p < 0.001), with a score increase of 21.5% between less present and most present taxa (Table 4; Figure 5).
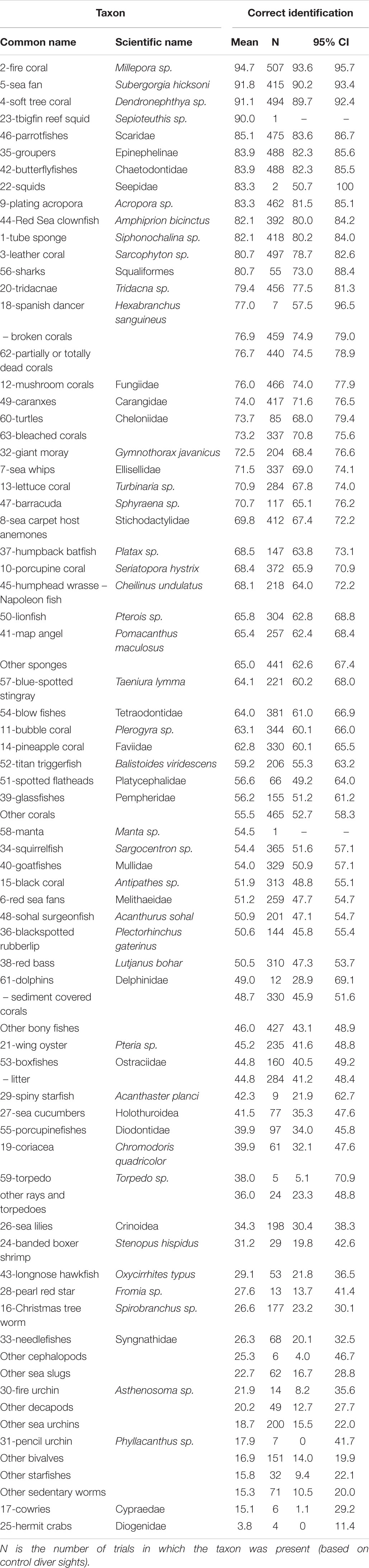
Table 4. Results of the correct identification analysis with mean score of correct identification performed by volunteers for each taxon.
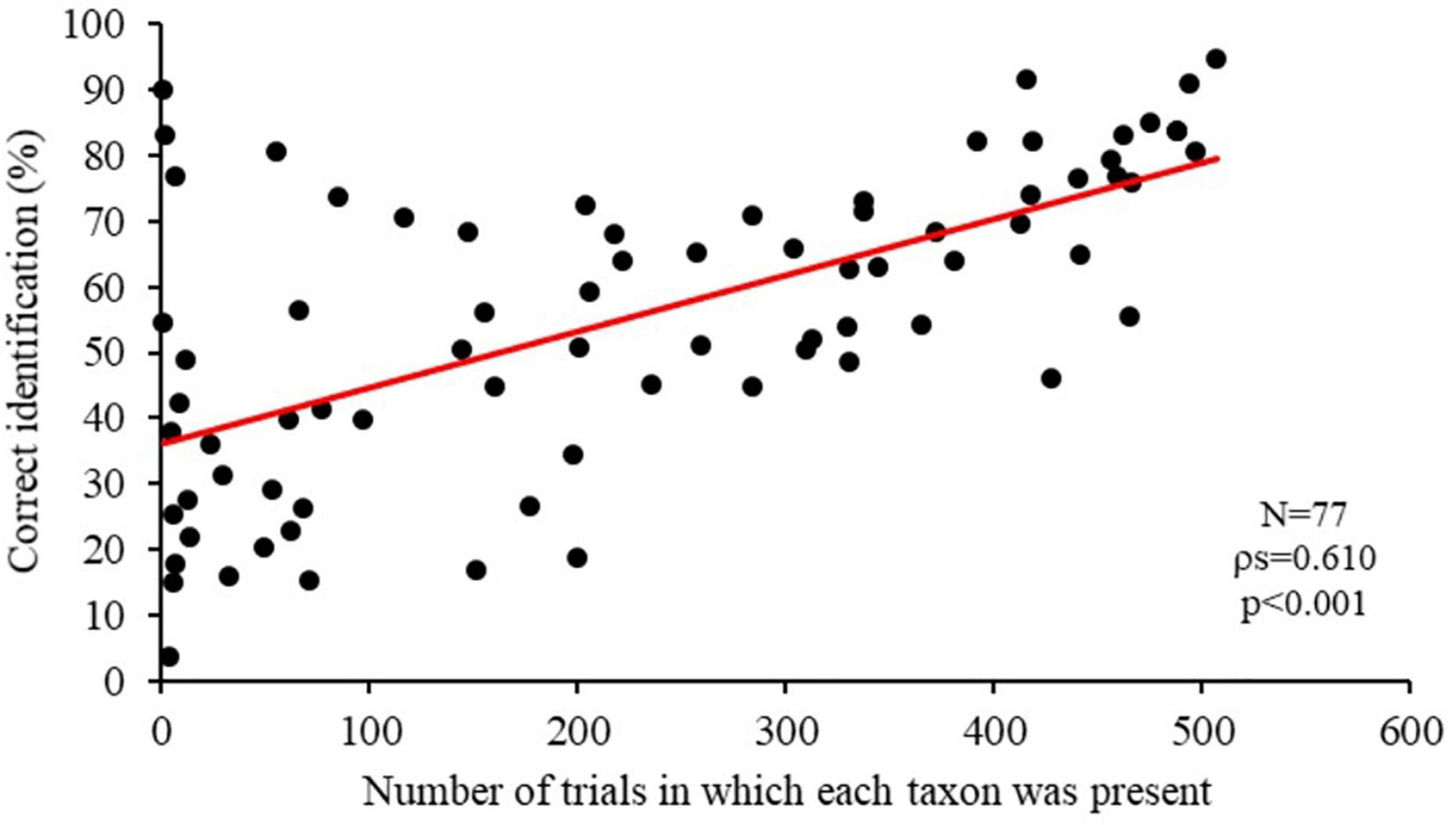
Figure 5. Significant correlation between the percentage of correct identification performed by volunteers (expressed as mean percentage for each taxon) and number of trials in which each taxon was present (based on the control diver sighted). Based on 72 studied taxa, litter presence and sight of damaged corals (see Table 3). Indicated in red the trendline of the correlations. N = number analyzed organisms; ρs = Spearman coefficient value.
The mean correctness of abundance ratings (CAR) of each validation trial ranged from 41.1 to 82.3%, with 94.9% of trials with mean CAR between 50 and 80% (Supplementary Table 1; Figure 2). CAR was positively correlated with: date (ρs = 0.110, N = 513, p < 0.05, Table 2; Figure 3), volunteers scores increased with years, with a score increase of 7.8% between the start and the end of the project (Table 3) and team size (ρs = 0.135, N = 513, p < 0.01, Table 2; Figure 3), volunteers scores increased with number of present divers, with a score increase of 6.9% between small and big groups (Table 3). CAR was not correlated with volunteer diving certification level (ρs = −0.020, N = 513, p = 0.657, Table 2), depth (ρs = −0.084, N = 513, p = 0.057, Table 2) and dive time (ρs = 0.016, N = 513, p = 0.721, Table 2).
The mean lower bound of the Czekanowski proportional similarity index (SI) of each validation trial ranged from 27.3 to 78.8%, with 91.2% of trials with mean SI between 40 and 70% (Supplementary Table 1; Figure 2). A 194 trials (37.8%) performed with levels of precision below the sufficiency threshold (SI, 95% CI lower bound ≤ 50%); 317 trials (61.8%) scored a sufficient level of precision (SI, 95% CI lower bound > 50% ≤ 75%), and 2 trials (0.4%) scored high levels of precision (SI, 95% CI lower bound > 75% ≤ 100%). SI was positively correlated with: team size (ρs = 0.107, N = 513, p < 0.05, Table 2; Figure 3), volunteers scores increased with number of present divers, with a score increase of 8.7% between small and big groups (Table 3); volunteer diving certification level (ρs = 0.253, N = 513, p < 0.001, Table 2; Figure 4), volunteers scores increased with higher divers certification level, with a score increase of 21.2% between beginners and professional divers (Table 3); dive time (ρs = 0.186, N = 513, p < 0.001, Table 2; Figure 4), volunteers scores increased with time spent underwater, with an increase of 21.4% between short and long dives (Table 3). SI was not correlated with date (ρs = 0.032, N = 513, p = 0.465, Table 2) and depth (ρs = −0.004, N = 513, p = 0.924, Table 2).
The mean lower bound reliability (α) of each validation trial ranged from 38.9 to 88.4%, with 93.4% of trials with mean reliability between 50 and 80% (Supplementary Table 1; Figure 2). Only 23 trials (4.5%) performed with an insufficient level of reliability (α, 95% CI lower bound ≤ 50%); 160 trials (31.2%) scored acceptable relationship with the control diver census (α, 95% CI lower bound > 50% ≤ 60%); 238 trials (46.4%) scored an effective reliability level census (α, 95% CI lower bound > 60% ≤ 70%); 92 trials (17.9%) performed from definitive to very high levels of reliability census (α, 95% CI lower bound > 70% ≤ 100%). Reliability was positively correlated with: team size (ρs = 0.212, N = 513, p < 0.001, Table 2; Figure 3), volunteers scores increased with number of present divers, with a score increase of 12.4% between small and big groups (Table 3); volunteer diving certification level (ρs = 0.200, N = 513, p < 0.001, Table 2; Figure 4), volunteers scores increased with higher divers certification level, with an increase of 11.1% between beginners and professional divers (Table 3); dive time (ρs = 0.145, N = 513, p < 0.001, Table 2; Figure 4), volunteers scores increased with time spent underwater, with an increase of 11.0% between short and long dives (Table 3). Reliability was not correlated with date (ρs = 0.029, N = 513, p = 0.515) and depth (ρs = −0.024, N = 513, p = 0.591) (Table 2).
Distance-based redundancy linear modeling analysis showed that the two variables “diving certification level” and “dive time” comprehensively explained about 82.7% of data variability, while the variable “team size” explained 13% of variability (Table 5; Figure 6).
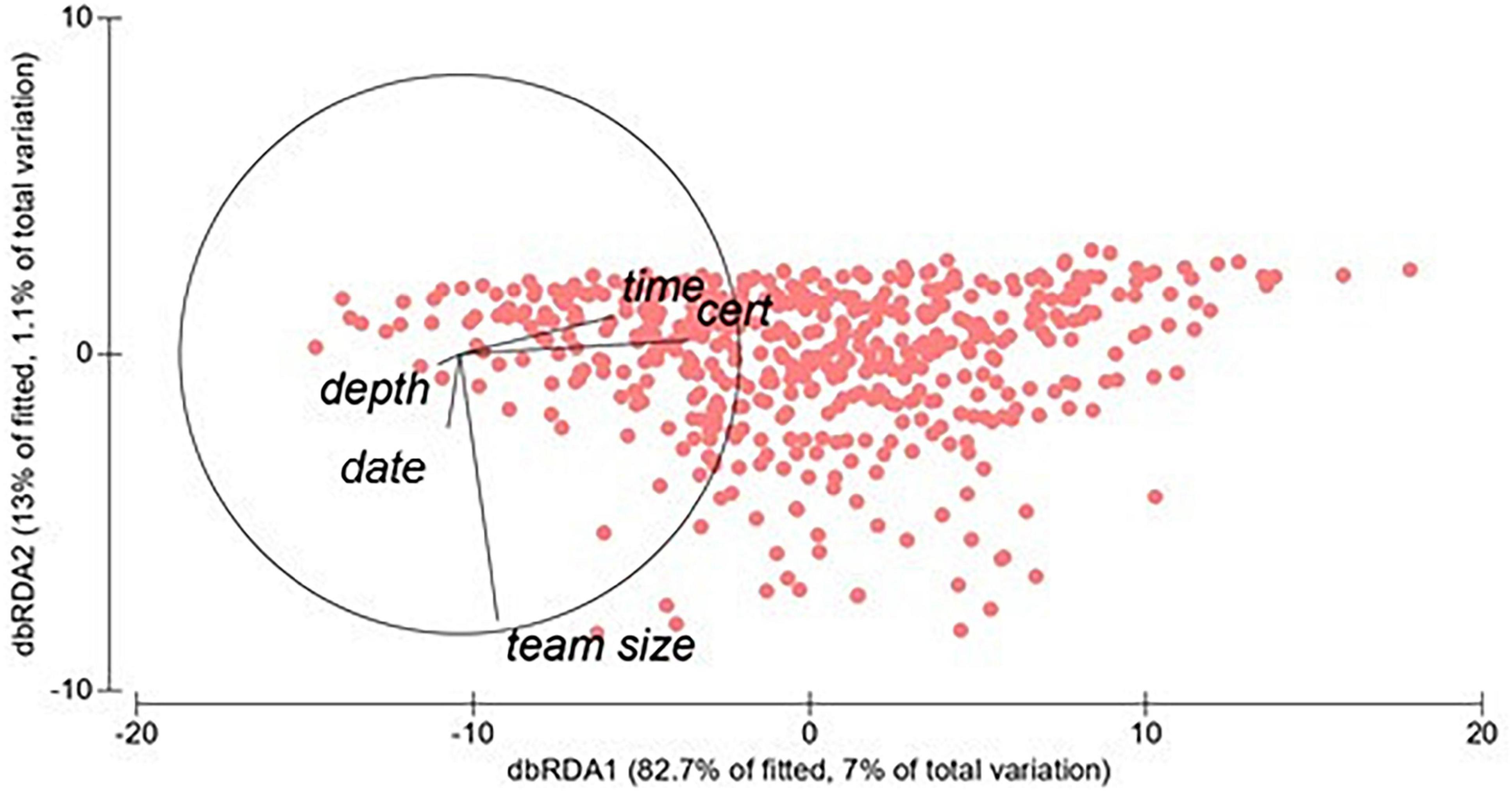
Figure 6. Results of distance-based linear modeling analysis. Variables in the graphs: depth of the scuba diving activity), date of the validation trial, time is the amount of minutes spent underwater, cert is the diving certification level of volunteers and team size is the number of divers present in each validation trial.
Discussion
Notwithstanding the large number of studied species, the accuracy of validation trials was promising, with most trials achieving a mean score between 50 and 70%. As pointed out by correlation and DISTLM analyses, most reliability parameters were positively correlated with the diving certification level, indicating that more experienced divers collected more accurate data. A possible explanation could be that expert divers have major confidence with the diving equipment and their underwater skills in comparison to beginner divers, allowing them focus more on the surrounding environment (Goffredo et al., 2010; Branchini et al., 2015b). Also, the dive time was positively correlated with most reliability parameters, suggesting that longer dives lead to higher data accuracy possibly because divers have more time to look around them and identify organisms.
Two reliability parameters (Accuracy and CAR) showed a positive correlation with the date. Although they are only two of seven parameters, this could suggest that citizen science projects should aim at a long-term duration due to the possibility to improve its implementation through feedbacks from volunteers, thus improving data quality.
Three reliability parameters (CAR, Similarity Index and Reliability) were positively correlated with team size, differently from previous studies where these relationships were not significant (Goffredo et al., 2010; Branchini et al., 2015b). This result could likely be related to presence of big groups belonging to the same diving school, that may be more guided by the instructor while filling in the questionnaire after the dive respect to single independent divers. Moreover, big groups of divers that stay close to each other to prevent the group from dispersing, could survey the marine environment in a more similar way to the control diver compared to small groups in which divers are free to dive. The anonymous data analysis did not allow us to test this aspect.
The lowest score within the analyzed reliability parameters was obtained by the Consistency parameter, with 86.9% of trials with mean consistency between 40 and 70%. This result is in line with previous studies that used the recreational approach and is likely related to the different personal interests of volunteers which made them focus on different species (Branchini et al., 2015b). For example, divers interested in macro photography may have focused their attention on small benthic organisms, while others interested in large pelagic fish (e.g., sharks) may have focused their attention away from the reef. Higher consistency results have been found using intensive training program in marine life identification and survey techniques (Mumby et al., 1995; Forrester et al., 2015). While an intense training could increase the consistency of data collected, it will drastically reduce the number of volunteers involved. This could limit the educational role of citizen science projects on volunteers for the lower number of involved volunteers.
The Czekanowski proportional similarity index (SI) showed that volunteers abundance ratings were below the sufficiency threshold in 37.8% validation trials, indicating that volunteers could encounter difficulties in abundance estimation as already found in other studies (Gillett et al., 2012; Done et al., 2017).
The wide variability of mean scores of the Correct Identification parameter could be due to the difficulty for volunteers to see and report the presence of less common or evident taxa (e.g., hermit crab that is frequently found between the rocks and blends in very well), while they performed better in recording the most common, well-known and straightforward species, as previously observed (Goffredo et al., 2010; Cox et al., 2012; Bernard et al., 2013; Branchini et al., 2015b; Forrester et al., 2015; Kosmala et al., 2016).
Previous studies that used the same methodology were performed, respectively, on 38 (Goffredo et al., 2010) and 61 validation trials (Branchini et al., 2015b). This study analyzed 513 validation trials that confirms previous trends permitting to generalize our results. A new result of this study is the team size variable as possible predictor for volunteers data quality, indicating that future data reliability studies should also consider this parameter.
As highlighted by different authors (Lewandowski and Specht, 2015; Kosmala et al., 2016; Specht and Lewandowski, 2018), a limitation of the approach used in this and other studies (Bell, 2007; Oscarson and Calhoun, 2007; Delaney et al., 2008; Aceves-Bueno et al., 2017) is that using professional or expert data, in the case of our study the “control diver,” as reference for evaluating volunteer data would also need an evaluation of correctness of the data collected by professionals or experts (Specht and Lewandowski, 2018). In this study control divers were marine biologist of the Marine Science Group trained in the project specifics that spent some weeks monitoring the biodiversity of the surveyed sites, which should assure a good quality of collected data.
In citizen science projects it is fundamental to develop suitable tasks for volunteers to assure good data quality collection (Schmeller et al., 2009; Magurran et al., 2010; Tulloch et al., 2013; Kosmala et al., 2016; Brown and Williams, 2019). In the present study data quality was assured: (1) by asking volunteers to fill the questionnaire soon after the dive, to avoid possible species oversight; (2) by training scuba instructors on the methodology of STE data collection on an annual basis (during public events) or on site when the control diver was present in the diving centers.
Moreover the overall data accuracy of this study was comparable to that performed in other projects by volunteer divers on precise transects (Mumby et al., 1995; Darwall and Dulvy, 1996; Goffredo et al., 2010; Done et al., 2017). This suggest that data from citizen science programs can complement professional datasets with sufficiently accurate data, increasing the possibility of researchers to estimate species richness and providing valuable information on species distributions that are relevant for the detection of the biological consequences of global change (Soroye et al., 2018).
Volunteers quality of data varies with tasks, they perform better at identifying iconic or well-known species while they can be confused by cryptic, rare or unknown specie (Kosmala et al., 2016; Swanson et al., 2016). Some of the methods used to improve the quality of data collected by volunteers are training programs or the request of prequalification via a skill test and the use of ongoing feedback on the volunteers identification for long-term engaged volunteers (Danielsen et al., 2014; Kosmala et al., 2016; van der Wal et al., 2016). Volunteers improve their data accuracy by gaining experience with a project, so a long-term engagement could bring to higher quality of data collected (Weir et al., 2005; Crall et al., 2010; Kelling et al., 2015).
Scuba Tourism for the Environment project was developed in collaboration with several mass tourism facilities and diving centers. During the project, annual meetings with Ministry of Tourism of the Arab Republic of Egypt were carried out to give management and conservation suggestions based on project results.
Conclusion
This project provided additional evidence that “recreational” (Goffredo et al., 2004, 2010) and “easy and fun” (Dickinson et al., 2012) citizen science is an efficient and effective method to recruit many volunteers and provide reliable data if well designed (Branchini et al., 2015b). The recreational citizen science approach used in the present study can be exported to different countries and used as a valuable tool by local governments and marine managers to achieve large-scale and long-term data collection, required in a fast-changing world where climate change and anthropogenic pressure on natural resources are leading to fast environmental changes worldwide.
Data Availability Statement
The raw data supporting the conclusions of this article will be made available by the authors, without undue reservation.
Ethics Statement
The studies involving human participants were reviewed and approved by the Bioethics Committee of the University of Bologna. The patients/participants provided their written informed consent to participate in this study.
Author Contributions
SG, SB, MMe, CM, AM, and EC collected data during the STE project. MMe, MMa, LL, MD, MTr, EN, MTi, RB, SB, PN, and SG analyzed the data. MMe, MMa, CM, EC, FP, AM, SF, and SG wrote the manuscript. SG supervised the research. All authors discussed the results and participated to the scientific discussion.
Conflict of Interest
The authors declare that the research was conducted in the absence of any commercial or financial relationships that could be construed as a potential conflict of interest.
Funding
Sources of funding have been the Italian Government (Ministry of Education, University and Research; www.istruzione.it), the Egyptian Government (Ministry of Tourism of the Arab Republic of Egypt and the Egyptian Tourist Authority; www.egypt.travel), ASTOI (Association of Italian Tour Operators; www.astoi.com), the tour operator Settemari (www.settemari.it), the diving agencies SNSI, Scuba Nitrox Safety International (www.Scubasnsi.com) and SSI, Scuba School International (www.divessi.com), the traveling magazine TuttoTurismo, the airline Neos (www.neosair.it), the association Underwater Life Project (www.underwaterlifeproject.it), the Project Aware Foundation (www.projectaware.org) and the diving centers Viaggio nel Blu (www.viaggionelblu.org), and Holiday Service (www.holidaydiving.org). The project has had the patronage of Ministry of the Environment and Land and Sea Protection (www.minambiente.it).
Acknowledgments
The research leading to these results has been conceived under the International Ph.D. Program “Innovative Technologies and Sustainable Use of Mediterranean Sea Fishery and Biological Resources (www.FishMed-PhD.org). This study represents partial fulfilment of the requirements for the Ph.D. thesis of MMe at the FishMed Ph.D. Program (University of Bologna, Italy). Special thanks go to all the divers who have made this study possible, all the marine biologists and to G. Neto (www.giannineto.it) who shot the pictures for the survey questionnaire.
Supplementary Material
The Supplementary Material for this article can be found online at: https://www.frontiersin.org/articles/10.3389/fevo.2021.694258/full#supplementary-material
Footnotes
- ^ https://cordis.europa.eu/programme/id/H2020_IBA-SWAFS-Citizen-2019
- ^ https://ec.europa.eu/programmes/horizon2020/en/h2020-section/science-and-society
- ^ https://ec.europa.eu/info/horizon-europe_en
- ^ https://ec.europa.eu/info/publications/mission-starfish-2030-restore-our-ocean-and-waters_en
- ^ http://www.gbif.org; http://www.marinespecies.org
References
Aceves-Bueno, E., Adeleye, A. S., Feraud, M., Huang, Y., Tao, M., Yang, Y., et al. (2017). The accuracy of citizen science data: a quantitative review. Bull. Ecol. Soc. Am. 98, 278–290. doi: 10.1002/bes2.1336
Bell, J. J. (2007). The use of volunteers for conducting sponge biodiversity assessments and monitoring using a morphological approach on Indo-Pacific coral reefs. Aquat. Conserv. Mar. Freshw. Ecosyst. 17, 133–145. doi: 10.1002/aqc.789
Bernard, A. T. F., Götz, A., Kerwath, S. E., and Wilke, C. G. (2013). Observer bias and detection probability in underwater visual census of fish assemblages measured with independent double-observers. J. Exp. Mar. Bio. Ecol. 443, 75–84. doi: 10.1016/j.jembe.2013.02.039
Bonney, R., Cooper, C. B., Dickinson, J., Kelling, S., Phillips, T., Rosenberg, K. V., et al. (2009). Citizen science: a developing tool for expanding science knowledge and scientific literacy. Bioscience 59, 977–984. doi: 10.1525/bio.2009.59.11.9
Bonney, R., Shirk, J. L., Phillips, T. B., Wiggins, A., Ballard, H. L., Miller-Rushing, A. J., et al. (2014). Next steps for citizen science. Science. 343, 1436–1437. doi: 10.1126/science.1251554
Bramanti, L., Vielmini, I., Rossi, S., Stolfa, S., and Santangelo, G. (2011). Involvement of recreational scuba divers in emblematic species monitoring: the case of Mediterranean red coral (Corallium rubrum). J. Nat. Conserv. 19, 312–318. doi: 10.1016/j.jnc.2011.05.004
Branchini, S., Meschini, M., Covi, C., Piccinetti, C., Zaccanti, F., and Goffredo, S. (2015a). Participating in a citizen science monitoring program: implications for environmental education. PLoS One 10:e0131812. doi: 10.1371/journal.pone.0131812
Branchini, S., Pensa, F., Neri, P., Tonucci, B. M., Mattielli, L., and Collavo, A. (2015b). Using a citizen science program to monitor coral reef biodiversity through space and time. Biodivers. Conserv. 24, 319–336. doi: 10.1007/s10531-014-0810-7
Brown, E. D., and Williams, B. K. (2019). The potential for citizen science to produce reliable and useful information in ecology. Conserv. Biol. 33, 561–569. doi: 10.1111/cobi.13223
Callaghan, C. T., Rowley, J. J. L., Cornwell, W. K., Poore, A. G. B., and Major, R. E. (2019). Improving big citizen science data: moving beyond haphazard sampling. PLoS Biol. 17:e3000357. doi: 10.1371/journal.pbio.3000357
Cox, T. E., Philippoff, J., Baumgartner, E., and Smith, C. M. (2012). Expert variability provides perspective on the strengths and weaknesses of citizen-driven intertidal monitoring program. Ecol. Appl. 22, 1201–1212. doi: 10.1890/11-1614.1
Crall, A. W., Newman, G. J., Jarnevich, C. S., Stohlgren, T. J., Waller, D. M., and Graham, J. (2010). Improving and integrating data on invasive species collected by citizen scientists. Biol. Invasions. 12, 3419–3428. doi: 10.1007/s10530-010-9740-9
Danielsen, F., Jensen, P. M., Burgess, N. D., Altamirano, R., Alviola, P. A., Andrianandrasana, H., et al. (2014). A multicountry assessment of tropical resource monitoring by local communities. Bioscience 64, 236–251. doi: 10.1093/biosci/biu001
Darwall, W. R. T., and Dulvy, N. K. (1996). An evaluation of the suitability of non-specialist volunteer researchers for coral reef fish surveys. mafia island, tanzania — a case study. Biol. Conserv. 78, 223–231. doi: 10.1016/0006-3207(95)00147-6
Delaney, D. G., Sperling, C. D., Adams, C. S., and Leung, B. (2008). Marine invasive species: validation of citizen science and implications for national monitoring networks. Biol. Invasions 10, 117–128. doi: 10.1007/s10530-007-9114-0
Dickinson, J. L., Shirk, J., Bonter, D., Bonney, R., Crain, R. L., Martin, J., et al. (2012). The current state of citizen science as a tool for ecological research and public engagement. Front. Ecol. Environ. 10:291–297. doi: 10.1890/110236
Done, T., Roelfsema, C., Harvey, A., Schuller, L., Hill, J., Schläppy, M. L., et al. (2017). Reliability and utility of citizen science reef monitoring data collected by Reef Check Australia, 2002–2015. Mar. Pollut. Bull. 117, 148–155. doi: 10.1016/j.marpolbul.2017.01.054
Forrester, G., Baily, P., Conetta, D., Forrester, L., Kintzing, E., and Jarecki, L. (2015). Comparing monitoring data collected by volunteers and professionals shows that citizen scientists can detect long-term change on coral reefs. J. Nat. Conserv. 24, 1–9. doi: 10.1016/j.jnc.2015.01.002
Foster-Smith, J., and Evans, S. M. (2003). The value of marine ecological data collected by volunteers. Biol. Conserv. 113, 199–213. doi: 10.1016/S0006-3207(02)00373-7
Fritz, S., See, L., Carlson, T., Haklay, M., Oliver, J. L., Fraisl, D., et al. (2019). Citizen science and the United Nations Sustainable Development Goals. Nat. Sustain. 2, 922–930. doi: 10.1038/s41893-019-0390-3
Galloway, A. W. E., Tudor, M. T., and Vander Haegen, W. M. (2006). The reliability of citizen science: a case study of oregon white oak stand surveys. Wildl. Soc. Bull. 34, 1425–1429.
Gillett, D. J., Pondella, D. J., Freiwald, J., Schiff, K. C., Caselle, J. E., Shuman, C., et al. (2012). Comparing volunteer and professionally collected monitoring data from the rocky subtidal reefs of southern California USA. Environ. Monit. Assess. 184, 3239–3257. doi: 10.1007/s10661-011-2185-5
Goffredo, S., Pensa, F., Neri, P., Orlandi, A., Gagliardi, M. S., Velardi, A., et al. (2010). Unite research with what citizens do for fun: “recreational monitoring” of marine biodiversity. Ecol. Appl. 20, 2170–2187. doi: 10.1890/09-1546.1
Goffredo, S., Piccinetti, C., and Zaccanti, F. (2004). Volunteers in marine conservation monitoring: a study of the distribution of seahorses carried out in collaboration with recreational scuba divers. Conserv. Biol. 18, 1492–1503. doi: 10.1111/j.1523-1739.2004.00015.x
Gommerman, L., and Monroe, M. C. (2012). Lessons Learned From Evaluations of Citizen Science Programs. Gainesville, FL: IFAS Extension University of Florida, 1–5.
Hecker, S., Bonney, R., Haklay, M., Hölker, F., Hofer, H., Goebel, C., et al. (2018). Innovation in citizen science – perspectives on science-policy advances. Citiz. Sci. Theory Pract. 3:4. doi: 10.5334/cstp.114
Holt, B. G., Rioja-Nieto, R., Aaron Macneil, M., Lupton, J., and Rahbek, C. (2013). Comparing diversity data collected using a protocol designed for volunteers with results from a professional alternative. Methods Ecol. Evol. 4, 383–392. doi: 10.1111/2041-210X.12031
Hunter, J., Alabri, A., and Ingen, C. (2013). Assessing the quality and trustworthiness of citizen science data. Concurr. Comput. Pract. Exp. 25, 454–466. doi: 10.1002/cpe.2923
Jetz, W., McPherson, J. M., and Guralnick, R. P. (2012). Integrating biodiversity distribution knowledge: toward a global map of life. Trends Ecol. Evol. 27, 151–159. doi: 10.1016/j.tree.2011.09.007
Jordan, R. C., Gray, S. A., Howe, D. V., Brooks, W. R., and Ehrenfeld, J. G. (2011). Knowledge gain and behavioral change in citizen-science programs. Conserv. Biol. 25, 1148–1154. doi: 10.1111/j.1523-1739.2011.01745.x
Kelling, S., Johnston, A., Hochachka, W. M., Iliff, M., Fink, D., Gerbracht, J., et al. (2015). Can observation skills of citizen scientists be estimated using species accumulation curves? PLoS One. 10:e0139600. doi: 10.1371/journal.pone.0139600
Kosmala, M., Wiggins, A., Swanson, A., and Simmons, B. (2016). Assessing data quality in citizen science. Front. Ecol. Environ. 14:551–560. doi: 10.1002/fee.1436
Kullenberg, C., and Kasperowski, D. (2016). What is citizen science? – A scientometric meta-analysis. PLoS One 11:e0147152. doi: 10.1371/journal.pone.0147152
Lewandowski, E., and Specht, H. (2015). Influence of volunteer and project characteristics on data quality of biological surveys. Conserv. Biol. 29, 713–723. doi: 10.1111/cobi.12481
Lewis, J. R. (1999). Coastal zone consersation and management?: a biological indicator of climatic influences. Aqua. Conserv. Mar. Freshw. Ecosyst. 405, 401–405.
Lukyanenko, R., Wiggins, A., and Rosser, H. K. (2019). Citizen science: an information quality research frontier. Inf. Syst. Front. 22, 961–983. doi: 10.1007/s10796-019-09915-z
Magurran, A. E., Baillie, S. R., Buckland, S. T., Dick, J. M. P., Elston, D. A., Scott, E. M., et al. (2010). Long-term datasets in biodiversity research and monitoring: assessing change in ecological communities through time. Trends Ecol. Evol. 25, 574–582. doi: 10.1016/j.tree.2010.06.016
Marshall, N. J., Kleine, D. A., and Dean, A. J. (2012). CoralWatch: education, monitoring, and sustainability through citizen science. Front. Ecol. Environ. 10:332–334. doi: 10.1890/110266
McKinley, D. C., Miller-Rushing, A. J., Ballard, H. L., Bonney, R., Brown, H., Cook-Patton, S. C., et al. (2017). Citizen science can improve conservation science, natural resource management, and environmental protection. Biol. Conserv. 208, 15–28. doi: 10.1016/j.biocon.2016.05.015
Meschini, M., Prati, F., Simoncini, G. A., Airi, V., Caroselli, E., Prada, F., et al. (2021). Environmental awareness gained during a citizen science project in touristic resorts is maintained after 3 years since participation. Front. Mar. Sci. 8:92.
Miller-Rushing, A., Primack, R., and Bonney, R. (2012). The history of public participation in ecological research. Front. Ecol. Environ. 10:285–290. doi: 10.1890/110278
Mumby, P. J., Harborne, A. R., Raines, P. S., and Ridley, J. M. (1995). A critical assessment of data derived from coral cay conservation volunteers. Bull. Mar. Sci. 56, 737–751.
Oscarson, D. B., and Calhoun, A. J. K. (2007). Developing vernal pool conservation plans at the locallevel using citizen-scientists. Wetlands 27, 80–95. doi: 10.1672/0277-5212
Sale, P. F., and Douglas, W. A. (1981). Precision and accuracy of visual census technique for fish assemblages on coral patch reefs. Environ. Biol. Fishes 6, 333–339. doi: 10.1007/BF00005761
Schmeller, D. S., Henry, P. Y., Julliard, R., Gruber, B., Clobert, J., Dziock, F., et al. (2009). Ventajas del monitoreo de biodiversidad basado en voluntarios en Europa. Conserv. Biol. 23, 307–316. doi: 10.1111/j.1523-1739.2008.01125.x
Silvertown, J. (2009). A new dawn for citizen science. Trends Ecol. Evol. 24, 467–471. doi: 10.1016/j.tree.2009.03.017
Soroye, P., Ahmed, N., and Kerr, J. T. (2018). Opportunistic citizen science data transform understanding of species distributions, phenology, and diversity gradients for global change research. Glob. Chang. Biol. 24, 5281–5291. doi: 10.1111/gcb.14358
Specht, H., and Lewandowski, E. (2018). Biased assumptions and oversimplifications in evaluations of citizen science data quality. Bull. Ecol. Soc. Am. 99, 251–256. doi: 10.1002/bes2.1388
Starr, J., Schweik, C. M., Bush, N., Fletcher, L., Finn, J., Fish, J., et al. (2014). Lights, camer citizen science: assessing the effectiveness of smartphone-based video training in invasive plant identification. PLoS One 9:e111433. doi: 10.1371/journal.pone.0111433
Sullivan, B. L., Wood, C. L., Iliff, M. J., Bonney, R. E., Fink, D., and Kelling, S. (2009). eBird: a citizen-based bird observation network in the biological sciences. Biol. Conserv. 142, 2282–2292. doi: 10.1016/j.biocon.2009.05.006
Swanson, A., Kosmala, M., Lintott, C., and Packer, C. (2016). A generalized approach for producing, quantifying, and validating citizen science data from wildlife images. Conserv. Biol. 30, 520–531. doi: 10.1111/cobi.12695
Tulloch, A. I. T., Possingham, H. P., Joseph, L. N., Szabo, J., and Martin, T. G. (2013). Realising the full potential of citizen science monitoring programs. Biol. Conserv. 165, 128–138. doi: 10.1016/j.biocon.2013.05.025
Turbé, A., Barba, J., Pelacho, M., Mugdal, S., Robinson, L. D., Serrano-Sanz, F., et al. (2019). Understanding the citizen science landscape for european environmental policy: an assessment and recommendations. Citiz. Sci. Theory Pract. 4, 34. doi: 10.5334/cstp.239
Van der Velde, T., Milton, D. A., Lawson, T. J., Wilcox, C., Lansdell, M., Davis, G., et al. (2017). Comparison of marine debris data collected by researchers and citizen scientists: is citizen science data worth the effort? Biol. Conserv. 208, 127–138. doi: 10.1016/j.biocon.2016.05.025
van der Wal, R., Sharma, N., Mellish, C., Robinson, A., and Siddharthan, A. (2016). The role of automated feedback in training and retaining biological recorders for citizen science. Conserv. Biol. 30, 550–561. doi: 10.1111/cobi.12705
Ward-Paige, C. A., and Lotze, H. K. (2011). Assessing the value of recreational divers for censusing elasmobranchs. PLoS One 6:e25609. doi: 10.1371/journal.pone.0025609
Weir, L. A., Royle, J. A., Nanjappa, P., and Jung, R. E. (2005). Modeling anuran detection and site occupancy on North American Amphibian Monitoring Program (NAAMP) routes in Maryland. J. Herpetol. 39, 627–639.
Keywords: citizen science, reliability, data quality, volunteers, biodiversity monitoring
Citation: Meschini M, Machado Toffolo M, Marchini C, Caroselli E, Prada F, Mancuso A, Franzellitti S, Locci L, Davoli M, Trittoni M, Nanetti E, Tittarelli M, Bentivogli R, Branchini S, Neri P and Goffredo S (2021) Reliability of Data Collected by Volunteers: A Nine-Year Citizen Science Study in the Red Sea. Front. Ecol. Evol. 9:694258. doi: 10.3389/fevo.2021.694258
Received: 12 April 2021; Accepted: 28 May 2021;
Published: 24 June 2021.
Edited by:
Reuven Yosef, Ben-Gurion University of the Negev, IsraelReviewed by:
Danilo Cialoni, Dan Europe Foundation, ItalyJakub Kosicki, Adam Mickiewicz University, Poland
Nadav Shashar, Ben-Gurion University of the Negev, Israel
Copyright © 2021 Meschini, Machado Toffolo, Marchini, Caroselli, Prada, Mancuso, Franzellitti, Locci, Davoli, Trittoni, Nanetti, Tittarelli, Bentivogli, Branchini, Neri and Goffredo. This is an open-access article distributed under the terms of the Creative Commons Attribution License (CC BY). The use, distribution or reproduction in other forums is permitted, provided the original author(s) and the copyright owner(s) are credited and that the original publication in this journal is cited, in accordance with accepted academic practice. No use, distribution or reproduction is permitted which does not comply with these terms.
*Correspondence: Stefano Goffredo, cy5nb2ZmcmVkb0B1bmliby5pdA==
†These authors have contributed equally to this work