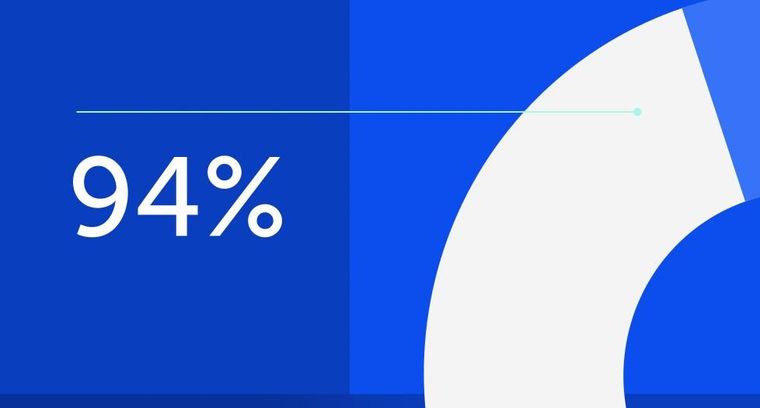
94% of researchers rate our articles as excellent or good
Learn more about the work of our research integrity team to safeguard the quality of each article we publish.
Find out more
REVIEW article
Front. Ecol. Evol., 23 June 2021
Sec. Population, Community, and Ecosystem Dynamics
Volume 9 - 2021 | https://doi.org/10.3389/fevo.2021.693781
This article is part of the Research TopicFrom Ecology to Cancer Biology and Back AgainView all 18 articles
Advanced metastatic cancer is currently not curable and the major barrier to eliminating the disease in patients is the resistance of subpopulations of tumor cells to drug treatments. These resistant subpopulations can arise stochastically among the billions of tumor cells in a patient or emerge over time during therapy due to adaptive mechanisms and the selective pressures of drug therapies. Epigenetic mechanisms play important roles in tumor cell diversity and adaptability, and are regulated by metabolic pathways. Here, I discuss knowledge from ecology, evolution, infectious disease, species extinction, metabolism and epigenetics to synthesize a roadmap to a clinically feasible approach to help homogenize tumor cells and, in combination with drug treatments, drive their extinction. Specifically, cycles of starvation and hyperthermia could help synchronize tumor cells and constrain epigenetic diversity and adaptability by limiting substrates and impairing the activity of chromatin modifying enzymes. Hyperthermia could also help prevent cancer cells from entering dangerous hibernation-like states. I propose steps to a treatment paradigm to help drive cancer extinction that builds on the successes of fasting, hyperthermia and immunotherapy and is achievable in patients. Finally, I highlight the many unknowns, opportunities for discovery and that stochastic gene and allele level epigenetic mechanisms pose a major barrier to cancer extinction that warrants deeper investigation.
Cancer is a disease that results from fundamental biological processes and mechanisms that enable diversity, adaptation and evolution (Merlo et al., 2006; Maley et al., 2017; McGranahan and Swanton, 2017). Stage IV metastatic cancer is currently not curable, and clinical treatment regimens are typically palliative, aiming to maximize patient quality and duration of life. Malignant cells arise most frequently in tissues with high cell division rates and from cell populations that have the capacity for cell division (i.e., reproduction) (Tomasetti et al., 2017). Tumor cell populations in a single 1 cm3 tumor can reach 107–109 cells, with a doubling rate of every ∼15 days for a rapidly growing tumor and every 100 or more days for a slow growing tumor (Tubiana, 1989; Del Monte, 2009). Thus, a metastatic patient with multiple lesions and circulating tumor cells can have tens of billions of actively dividing malignant cells. With an average cell cycle time of ∼48 h and mutation rate of 1.14 mutations per genome per cell division (Werner et al., 2019), every gene in the cancer cell genome is affected by coding and non-coding genetic mutations multiple independent times in a patient with years of metastatic disease. All enemies are at the gate. Nonetheless, cancer cell evolution converges on a handful of specific driver mutations that are the major genetic drivers of malignancy (McGranahan et al., 2015; Tomasetti et al., 2015; Tokheim et al., 2016; Reiter et al., 2018). This observation led to the proposal that a curative combinatorial treatment strategy attacking these driver mutations could be developed (Reiter et al., 2019). However, while the identification of core genetic driver mutations in cancer is foundational and exciting, understanding epigenetic mechanisms driving cancer cell diversity and adaptability is another major barrier. Epigenetic mechanisms contribute to the evolution of multi-drug resistance (MDR), preventing us from curing metastatic cancer (Easwaran et al., 2014; Baylin and Jones, 2016; McGranahan and Swanton, 2017; Guo et al., 2019). The development of cancer cell subpopulations with MDR, or the ability to enter dormant, persistent states that evade treatment and immune predation, ultimately lead to disease progression and patient death (Recasens and Munoz, 2019; Shen et al., 2020). No single drug, new or old, will ever overcome these evolutionary forces and cure the disease. Currently, many chemotherapies and endocrine therapies target the reproductive capabilities of cancer cells by affecting cell division processes or signaling pathways that control cell division and tumor growth. Immunotherapies are distinct in that they enhance immune cell predation on cancer cells. However, the field lacks interventions aimed at solving the fundamental problem of how to constrain cancer cell diversity, adaptability and evolvability. Interventions that could homogenize cancer cell populations and constrain adaptability would help enable a chance at a cure using existing drugs.
Recent articles propose innovative treatment regimens for curing metastatic cancer that are inspired by the factors that drive the extinction of species in nature (Gatenby and Brown, 2018; Gatenby et al., 2019, 2020; Reed et al., 2020). Species extinctions often involve such complex interactions between unrelated stressors, rather than single catastrophic events. Central to these ideas are the application of aggressive, unpredictable, successive and combinatorial chemo, endocrine and immunotherapy treatment strikes that fragment cell populations and continue even after the cancer becomes clinically undetectable. Combinatorial approaches that are designed to be curative would benefit from interventions that help constrain cancer cell diversity and adaptability during drug treatment strikes. Here, I discuss how epigenetic gene regulatory mechanisms and allele-specific expression effects create cellular diversity and enable adaptability in cancer, creating barriers to its extinction. I highlight opportunities to learn from ecology, evolution, biochemistry, metabolism, genomics and conserved responses to bacterial infection to develop clinically relevant strategies to constrain cancer cell diversity and adaptability. My goal is to lay the conceptual groundwork and areas for further study to devise feasible treatment programs to make cancer cell populations more vulnerable to combinatorial chemotherapy strikes designed to cure metastatic disease.
Previous decades have largely focused on cancer genetics and the identification of important genetic mutations. Less is known about gene regulatory and epigenetic mechanisms in cancer, though it is emerging as a major field of study and a new area for therapy development. From ecology and species evolution, we know that protein-coding genes are relatively well conserved across species, while gene regulatory mechanisms and cis-regulatory elements (CREs) are rapidly evolving. Changes to gene regulatory network (GRNs) play the major roles in the development of new phenotypes in different lineages (Davidson and Erwin, 2006; Wray, 2007; Carroll, 2008; Davidson, 2010). Recent reviews have covered the emerging and likely important roles that epigenetic mechanisms also play in cancer initiation and progression, metastasis, and drug resistance (Guo et al., 2019), as well as opportunities for targeting these mechanisms to treat the disease (Bennett and Licht, 2018; Cheng et al., 2019; Hogg et al., 2020). Epigenetic mechanisms and stochastic changes to GRNs and gene expression enable a dynamic range of phenotypic possibilities for a population of cells or organisms.
Pioneering studies in bacteria first showed that isogenic cells held in constant conditions occupy a wide-range of different states due to stochastic gene expression and transcriptional bursts (Li, 2002; Ozbudak et al., 2002). Subsequent studies of eukaryotic cells reached similar conclusions (Blake et al., 2003). It is now well established that, at baseline, cells exist in flux, creating molecularly diverse populations through stochastic gene expression (Kærn et al., 2005; Levine et al., 2013), transcriptional bursting and transitions between active, reversibly silent and irreversibly silent chromatin states (Singer et al., 2014; Bintu et al., 2016; Figure 1). This has the important effect of creating diversity for adaptability and “bet hedging” so that at least some cells are in the right molecular state to receive and correctly respond to unpredictable signals from the environment (Raj and van Oudenaarden, 2008; Feinberg and Irizarry, 2010; Raj et al., 2010; Balázsi et al., 2011). In the context of stressors, this diversity may help prokaryotic and eukaryotic cells survive acute insults by helping to ensure that at least some cells exist in a state that is resilient to the stressor.
Figure 1. Schematic depiction of how stochastic gene expression and epigenetic regulation creates mosaics of cells in different molecular states. Studies by Bintu et al. (2016) show that histone acetylation is dynamic and mediates short-term epigenetic memory, while histone and DNA methylation is more stable, mediating long-term epigenetic memory. These stochastic gene expression and epigenetic effects contribute to cellular diversity and may enable enhanced tumor cell diversity and adaptability.
This type of stochastic epigenetic cellular diversity has been proposed to have important roles in cancer evolution and drug resistance (Pujadas and Feinberg, 2012). However, we currently have little understanding of the mechanisms involved or how to modulate or constrain the effect. Few studies have directly examined chromatin dynamics at the cellular level over time. Chromatin biochemical modifications are diverse and new forms are constantly being discovered. Major epigenetic mechanisms involved in stochastic epigenetic diversity likely include histone acetylation, DNA methylation and histone methylation. A brief overview of these mechanisms is described below, but I refer the reader to excellent recent reviews for further detail (Campbell and Wellen, 2018; Dai et al., 2020; Trefely et al., 2020).
In brief, histone tails are biochemically modified post-translationally to regulate gene expression. The chromatin modifiers (writers) that establish these marks use metabolic intermediate molecules, including acetyl-CoA and S-adenosylmethionine (SAM). Acetyl-CoA is the metabolite used by histone acetyltransferases (HATs) to place acetyl groups on lysine residues of the N-terminal tails of H3 and H4 canonical histones. Acetyl groups are a subtype of acyl organic molecules distinguished by the inclusion of a –CH3 group. In the absence of acetylation, the positive charges on H3 and H4 histones combine with the negative charge on the surface of H2A histone fold domains to enable the formation of nucleosomes and compact chromatin. One effect of H3 and H4 lysine acetylation is to change the overall histone charge to neutral, which reduces histone affinity, creating open chromatin sites in which transcriptional regulatory proteins can bind. The creation of these open chromatin sites through lysine acetylation is important for the activation of non-coding enhancers, gene promoters, gene bodies and alternative exon usage through splicing variation (Rajagopal et al., 2014). In addition to promoting open chromatin states, bromo-domain containing chromatin “reader” proteins recognize lysine acetylation and bind to promote gene expression. Recently, other histone acylations have been uncovered, which also have activating effects on gene expression (Dai et al., 2020). On the other hand, histone deacetylation by histone deacetylases (HDACs) promotes gene silencing and the formation of heterochromatin. Acetyl groups are added to histone tails by HATs, which are divided into three groups, including GNAT, MYST, and p300/CBP. The HDACs that remove acetyl groups in mammals are divided into 4 groups, including the zinc-dependent class I, II, and IV HDACs, and the NAD-dependent class III HDACs, which are also known as sirtuins.
In addition to Acetyl-CoA dependent chromatin acetylation dynamics, SAM dependent chromatin methylation dynamics are primary players in orchestrating cellular epigenetic states and GRNs. De novo DNA methylation is performed by DNMT (DNA methyltransferase) 3a and DNMT3b, and maintenance of DNA methylation is performed by DNMT1, which recognizes hemi-methylated DNA. DNA methylation frequently occurs on cytosines located in sets of CpG dinucleotide repeats called CpG islands, which are located near transcription start sites. Methylation of these regions contributes to gene silencing. Removal of the silencing can be achieved by active DNA demethylation, which is primarily regulated by the TET family of DNA demethylase enzymes, as well as by passive DNA demethylation due do inhibition of DNMT1 in dividing cells, which causes the methylation mark to be lost over successive cell divisions.
Methylation also occurs on histone tails, including lysine and arginine residues, which alters the affinity of histone-methylation reader proteins to bind and affect gene expression. Some forms of histone methylation are associated with gene activation, such as H3K4, H3K79, or H3K36 methylation. On the other hand, H3K9, H3K27, and H4K20 methylation are associated with gene silencing and different methylation states, including mono-, di- or tri- methylation of the same amino acid residue can have different effects on gene expression. Histone methylation is mediated by histone methyltransferases. For example, the EZH2 (enhancer of zeste 2) enzymatic subunit of the polycomb repressor complex 2 (PRC2) catalyzes the formation of H3K27me3, while KRAB (Kruppel associated box) domain containing zinc finger protein transcription factors can catalyze the formation of H3K9me3. Both of these chromatin modifications have potent silencing effects and important roles in the formation of stable heterochromatin.
Building on this understanding of epigenetic gene regulation, a pioneering study by Bintu et al. created an elegant reporter assay to study the temporal dynamics of histone acetylation, DNA methylation and histone methylation at the cellular level (Bintu et al., 2016). They compared the cellular repression induction and reactivation kinetics for DNA methylation (DNMT3B), H3K9me3 (KRAB), H3K27me3 (EED-EZH2 component of PRC2) and H3/H4 histone deacetylation (HDAC4). Their results show that DNMT3B, KRAB, and EED-EZH2 induce stable chromatin changes that cause permanent epigenetic memory. DNMT3B in particular showed slow induction kinetics, but caused stable and permanent epigenetic memory in affected cells. These results are consistent with previous work showing that DNA methylation is a relatively stable biochemical mark. In contrast, HDAC4 effects are highly dynamic, such that activation of HDAC4 caused the induction of faster chromatin changes than the other enzymes tested and the effects are transient and rapidly reversible. The rapid and dynamic roles of histone acetylation in these hamster ovary cell lines are consistent with previous work in yeast, which showed that histone acetylation and deacetylation states can switch within minutes (Katan-Khaykovich and Struhl, 2002). Overall, these results suggest that reducing the enzymatic activity of HATs and HDACs could constrain short-term, dynamic stochastic cellular diversity and adaptability. Further, reducing the activity of enzymes controlling DNA and histone methylation could help constrain long-term, stable stochastic cellular diversity and adaptability (Figure 1). Below, I next discuss how stochastic epigenetic effects not only occur at the gene level, but also at the allele level, which potentially further create primary epigenetic and gene expression barriers to cancer “extinction.”
Stochastic gene regulatory effects also occur at the allele level, which could further contribute to cancer cell diversity and evolvability. Indeed, the diploid and, in some cases, polyploid nature of eukaryotic cells creates added cellular epigenetic, gene expression and genetic diversity. The advantage of diploidy over haploidy has typically been proposed to be to mask the effects of partially recessive mutations (Orr, 1995). Interestingly, however, periods associated with catastrophic extinction events, such as the comet or asteroid strike and volcanic eruptions at the Cretaceous–Paleogene boundary, were previously shown to be associated with evolutionary bursts of new species with whole genome duplication events (Madlung, 2012; Van de Peer et al., 2017). In other words, increased ploidy was associated with improved survival during a mass extinction event. Polyploidy is proposed to have been beneficial because it increases allelic diversity and species adaptability (Fox et al., 2020). In mammals, most cells are diploid, though specialized cell-types, such as liver hepatocytes, are polyploid. It has been observed that normal diploid cells in the body can increase their ploidy in response to stress (Fox et al., 2020). For cancer, tumor cell acquisition of polyploidy or aneuploidy are major features of the disease that are thought to contribute to rapid tumor evolution (Krajcovic and Overholtzer, 2012; Coward and Harding, 2014). Typically, increases in ploidy are considered to drive increased genetic variation, however, through allele-specific epigenetic regulatory effects, increased ploidy could also promote increased epigenetic variation (Figure 2).
Figure 2. Allele-specific epigenetic and expression create complex and diverse cellular states. (A) Classic biallelic (allele co-expression) and no expression states typically associated with autosomal genes, and clonal random monoallelic states (RME) typically associated with random X-inactivation in females. (B) Newly described stochastic differential allele expression (DAE), which causes biallelic and stochastic allelic expression states at the cellular level for thousands of autosomal genes in mice. (C) DAE interacts with heterozygous mutations in diploid and polyploidy cells to create widespread cellular diversity and potential for adaptability to different conditions. This phenomenon could be a major blocker to cancer cell extinction.
Previous in vitro studies of cell lines have suggested the existence of random monoallelic expression (RME) for thousands of autosomal genes in mice and humans due to non-genetic mechanisms (Gimelbrant et al., 2007; Eckersley-Maslin et al., 2014; Gendrel et al., 2014). RME in cell lines is mitotically heritable and has been shown to be regulated by levels of the insulator protein, CTCF, in some cases (Chandradoss et al., 2020). However, the prevalence of widespread autosomal RME that is clonal (mitotically heritable) is currently debated and little is known about the existence of such effects in vivo (Reinius and Sandberg, 2015; Rv et al., 2021; Vigneau et al., 2018). Some have suggested that bona fide clonal RME is rare on the autosomes and that most observed cases are linked to transcriptional bursting and low expression (Deng et al., 2014; Reinius et al., 2016; Larsson et al., 2018; Symmons et al., 2019). Nonetheless, stochastic allele-specific expression for some autosomal genes appears stable at the cellular level. For example, one study directly imaged allelic expression at the cellular level over time for Bcl11b in T cell lineages (Ng et al., 2018). The authors found that monoallelic (or biallelic) expression states can be stable at the protein level for at least one hundred hours in single cells, amounting to several days (Ng et al., 2018). This type of allele-specific regulatory effect might be sufficient to have biological consequences, affecting gene dosage and/or the cellular effects of a single mutated allele.
Focusing on the in vivo context, we previously uncovered hundreds of genes in mouse tissues that display stochastic differential allelic expression (DAE) at the cellular level (Huang et al., 2017). We found that DAE affects the cellular expression of heterozygous mutations such that mosaics of mixed cells arise in tissues in which some cells monoallelically express the mutated allele, some express the wild-type allele and others are biallelic and express both parental alleles (Huang et al., 2017). Stochastic DAE may be an important feature of diploid (or polyploidy) genomes that increases cellular diversity and adaptability to stress (Huang et al., 2018; Kravitz and Gregg, 2019).
With regard to nomenclature, we differentiate stochastic DAE from bona fide RME, because RME is typically used to describe clonal, mitotically-heritable and stable monoallelic states, such as random X-inactivation, olfactory receptor or protocadherin monoallelic expression states (Lomvardas and Maniatis, 2016; Monahan et al., 2019; Figures 2A, 3). In the case of stochastic DAE, we found that some cells are monoallelic and others are biallelic, and the temporal stability of each allelic state and the clonal relationships between cells are not yet known (Figure 2B). Mechanistically, stochastic DAE and clonal RME likely involve different epigenetic mechanisms, including different roles for short-term (e.g., histone acetylation) versus long-term (e.g., methylation or CTCF) epigenetic memory (Figure 3). This new area is expected to reveal important allele-specific gene regulatory mechanisms that enable increased phenotypic variability and adaptable metabolic phenotypes (Huang et al., 2018; Kravitz and Gregg, 2019). Currently, we do not understand the nature of these different allelic effects in tumor cells or how they may change and contribute to tumor initiation, metastasis, evolution and drug resistance, and affect patient survival.
Figure 3. A decision classification tree for different subtypes of allele-specific epigenetic and expression effects according to heritability, clonality and stability. The decision tree shows that different allele-specific expression effects arise according to genetic, genomic imprinting, clonal RME and stochastic DAE. Each of these increases cellular diversity and adaptability and are potential barriers to eliminating metastatic cancer in patients.
Given that polyploidy and aneuploidy are linked to cancer progression and evolution, and appear to predict worse outcomes (Coward and Harding, 2014; Krajcovic and Overholtzer, 2012), it is possible that cancer cells benefit from increases in ploidy by increasing cellular diversity through stochastic allele-specific epigenetic and gene expression effects. Previous studies found that progenitor versus differentiated cellular states are associated with dramatic differences in RME, suggesting these mechanisms have roles in defining different cellular proliferative versus differentiated states (Miyanari and Torres-Padilla, 2013; Eckersley-Maslin et al., 2014; Gendrel et al., 2014; Jeffries et al., 2016; Branciamore et al., 2018; Ng et al., 2018). Recent studies have also begun to show how stochastic allele-specific epigenetic effects can interact with allele-specific genetic variants to create allelic diversity (Onuchic et al., 2018; Zhang S. et al., 2020). In summary, the studies above show the enormous potential for stochastic epigenetic and gene expression effects to drive cellular diversity in cancer and the different potential forms of these effects at the gene and allele levels. Next, I discuss findings that show that stochastic epigenetic effects are, in fact, the primary drivers of tumor cell evolution and drug resistance, which motivates discussions for new studies and clinical solutions.
Intra-tumor cellular epigenetic and gene expression heterogeneity is proposed play the primary roles in the acquisition of drug resistance in cancer compared genetic mutations (Flavahan et al., 2017; Marusyk et al., 2020). A leading model in the field is that stochastic and semi-stable changes to gene expression and chromatin states cause drug-resistant phenotypes to arise dynamically within tumor cell populations. In this model, stochastic epigenetic and phenotypic cellular diversity creates an ecosystem in which a drug treatment can “discover” a pre-existing tumor cell in a state that enables it to tolerate the drug and persist. This epigenetic state is therefore advantageous for survival and proliferation compared to other tumor cell states in the microenvironment. Not only will this cell survive, persist and continue to reproduce, but its advantageous chromatin state can become enhanced, stabilized and mitotically heritable through further induced chromatin and gene expression changes. This evolutionary process drives the formation of dangerous new lineages of drug resistant tumor cells.
Support for this model of tumor evolution and drug resistance is strong. Indeed, epigenetic stochasticity has been shown to be a central driver of cellular phenotypic variability and mechanism of plasticity (Jenkinson et al., 2017). A seminal paper by Sharma et al. showed that individual tumor cells stochastically and transiently acquire and then relinquish chromatin-mediated drug resistant states (Sharma et al., 2010). Moreover, they showed that chromatin-modifying agents selectively ablated the resistant cell population. Subsequently, others found that the histone demethylases, KDM5A and KDM6B, regulate phenotypic heterogeneity in estrogen receptor positive breast cancer (Hinohara et al., 2018). High KDM5B activity promotes increased intra-tumor gene expression heterogeneity, creating pre-existing cell populations that increase chances for drug resistance (Hinohara et al., 2018). Further, studies of DNA methylation dynamics in chronic lymphocytic leukemia (CLL) found that stochastic epimutations form the basis of intratumor cellular methylome variability (Landau et al., 2014). More recently, single-cell DNA methylome analyses in CLL revealed that the cellular inheritance of stochastic DNA methylation epimutations reveal a lineage tree for the cellular evolution of the disease and that epigenetic drift occurs rapidly following B cell transformation and greater proliferation rates (Gaiti et al., 2019). Moreover, increases in stochastic epigenetic diversification in CLL appear to contribute to a larger diversification of gene expression and cellular molecular identities in the disease (Gaiti et al., 2019; Pastore et al., 2019). Thus, primary roles for stochastic and dynamic cellular epigenetic and gene expression variability in the selection, formation and evolution of drug resistant tumor cells are now well supported (Huang et al., 2009; Huang, 2013; Pisco et al., 2013; Knoechel et al., 2014; Flavahan et al., 2017; Liau et al., 2017; Shaffer et al., 2017; Risom et al., 2018; Marusyk et al., 2020). We now have a maturing conceptual framework for understanding “mutation-independent” evolution and the primary roles that epigenetic and gene expression diversity play in the development of new phenotypes (Huang et al., 2009; Huang, 2011, 2012, 2021). One of the most glaring facts supporting this model is that genetic mutations are not required for cells to “evolve” into different cell lineages during organismal development—this diversity is entirely grounded in epigenetic and gene expression changes (Huang, 2012).
The major implication of all of the findings in the above sections is that uncovering ways to constrain tumor cell epigenetic regulatory activity and dynamics will help constrain cancer cell diversity, adaptability and evolution during treatment. Placing constraints on cellular acetylation and methylation dynamics could be especially effective, but no single molecular target will solve this problem because of redundancy and adaptability. Next, I discuss clues for how to effectively constrain cellular epigenetic diversity and dynamics in a clinically relevant manner that could be feasibly integrated into a chemotherapy treatment regimen.
Since both prokaryotic and eukaryotic cells use stochastic gene regulatory mechanisms and transcriptional noise for promoting cellular diversity and adaptability, we can potentially learn solutions to constrain these effects by analyzing how vertebrates evolved solutions to prokaryotic infections. The numbers of bacteria involved in an infection can exceed the population sizes that cancer cells reach in a body, yet the body can drive them to extinction. To achieve this, vertebrates evolved a highly conserved set of sickness responses that help to effectively eliminate infections in combination with activation of the immune system (Hart, 1988; Aubert, 1999; Dantzer and Kelley, 2007). This combinatorial response involves: (1) fasting (loss of appetite), (2) fever, (3) sleepiness and fatigue, (4) social withdrawal and irritability, and (5) altered motivations (inhibited foraging and exploration) (Hart, 1988; Aubert, 1999; Adelman and Martin, 2009). This adaptation for infection turns out to be highly relevant to cancer elimination. A history of the immunotherapy field reveals that interest in immune system predation on tumor cells began with early observations of tumors disappearing in patients following a bacterial infection with a high fever (Dobosz and Dzieciątkowski, 2019). Subsequently, William Coley showed that cancer patients enter spontaneous remission after a streptococcal skin infection (i.e., erysipelas). Moreover, bacterial infections can induce complete remission in several cancer types (Dobosz and Dzieciątkowski, 2019). So far, this work has largely inspired the development of targeted immunotherapies that aim to improve immune cell detection and killing of cancer cells, but there may be more to learn.
Vertebrate sickness responses are typically proposed to function for diverting energy from activities peripheral to surviving infection to immune responses that combat the infection (Hart, 1988; Aubert, 1999; Dantzer and Kelley, 2007). However, if the goal was only to increase resources for immune defense, namely boost the concentrations of the substrates and cofactors needed to support biochemical reactions for effective immunity, one might instead expect the animal to display increased appetite and caloric intake, rather than fasting. Recently, fasting, and the associated shift to ketone metabolism, was shown to reduce the damaging effects of reactive oxidative species (ROS) generated by bacterial inflammation, indicating an important function for this component of the sickness response that is different from the energy conservation model (Wang et al., 2016). However, as I discuss below, fasting may have additional benefits that involve constraining capabilities for stochastic cellular diversity and adaptability by limiting the availability of key substrates and cofactors.
From the perspective of preserving energy for the immune attack, coupling fasting with fever during sickness responses might seem to be counterproductive. How fever offers a protective mechanism against pathogenic microbes is a long-standing mystery (Evans et al., 2015). Fever is currently thought to create conditions that are inhospitable for microbe proliferation by raising the body’s temperature above optimal growth conditions, while potentiating the immune response by increasing neutrophil activity and lymphocyte proliferation and activation (Hart, 1988; Evans et al., 2015). However, with the possible exception of unique immune cells, fever could constrain biochemical reaction kinetics in cells and thereby further constrain capabilities for creating a range of different stochastic cellular states. By constraining the diversity and adaptability of microbes, immune cell predation would be more effective. As I discuss below, fever could especially help block dangerous hypometabolic hibernation-like states that enable disease persistence.
Thus, by combining fasting and fever with increased immune predation, the body has not only evolved an effective combinatorial strategy for driving the extinction of invading microbes, but apparently also cancer. We now know that nutrients and metabolic processes affect epigenetic gene regulatory mechanisms, suggesting an important link exists between fasting, fever and the epigenetic mechanisms that enable cellular diversity and adaptability. Noticeably, the vertebrate sickness response combines the starvation, climate change and predation conditions that frequently cause species extinctions in the wild. It is a highly effective recipe for this outcome.
The benefits of fasting for cancer and other diseases have been carefully reviewed elsewhere (Nencioni et al., 2018; de Cabo and Mattson, 2019; Caffa et al., 2020; Tajan and Vousden, 2020; Zhang J. et al., 2020). Pre-clinical (Lee et al., 2012; Brandhorst et al., 2015; Wei et al., 2017; de Cabo and Mattson, 2019; Caffa et al., 2020), and early clinical studies (Caffa et al., 2020; de Groot et al., 2020), show benefits for coupling fasting or fasting-mimicking diets (FMDs) with chemotherapy and/or endocrine therapy, including enhanced treatment efficacy, reduce side effects and the prevention of drug resistance. The beneficial effects of fasting in cancer have so far been largely attributed to reductions in circulating glucose, insulin, IGF-1, PI3 kinase, mTOR and leptin signaling, which reduces growth signals that can drive cancer cell proliferation and survival. Beneficial effects of increased autophagy and stem cell activation are also apparent. Different durations and patterns of fasting induce different biological effects in a cell-type dependent manner. It has been suggested that the induction of a starvation response, in which cellular autophagy is strongly activated, is important for deriving anti-cancer benefits (Brandhorst et al., 2015; Nencioni et al., 2018). Important for this article, is that starvation and nutrient deprivation affect epigenetic mechanisms. Such effects could help constrain tumor cell diversity and adaptability. Starvation states can affect the availability of essential substrates and cofactors necessary for enzymatic modifications to chromatin and chromatin binding transcriptional regulatory proteins. Starvation states also block global protein translation by inhibiting mTOR complex 1 (mTORC1), which in turn limits cellular capabilities for intra and intercellular signaling and gene expression dynamics (Holcik and Sonenberg, 2005; Wullschleger et al., 2006). Indeed, starvation state translational and transcriptional programs involve a shift to specific stress response mechanisms that are essential for cell survival.
To my knowledge, no study has yet determined whether starvation reduces stochastic epigenetic dynamics within a cell over time, epigenetic diversity across populations of cells, or capabilities for epigenetic and gene expression adaptability in response to new and additional stressors (e.g., chemotherapy strikes). However, there are reasons to expect such effects. An enzyme’s Km is the concentration of a substrate needed for the rate of its catalytic reaction to be half of the maximum rate (Vmax) and can further depend on the concentration of necessary cofactors. Relative to their Km, the physiological concentrations of the substrates and cofactors needed for HAT, HMT, and DNMT mediated chromatin-modifying reactions are low (Reid et al., 2017). As a result, the kinetics of histone acetylation and methylation biochemical reactions are sensitive to changes in these substrate concentrations and inhibited by reduced cellular concentrations of nutrient-derived cofactors and substrates (Su et al., 2016; Reid et al., 2017; Figure 4A). This differs from phosphorylation and ubiquitination reactions that are not as responsive to metabolic changes because their substrate, ATP, does not reach cellular levels low enough to limit enzymatic activity (Locasale and Cantley, 2011). Consequently, starvation limits the availability of essential SAM and Acetyl-CoA substrates needed for methylation and acetylation dynamics, respectively, while simultaneously activating nutrient stress response epigenetic and gene expression programs for survival. This may constrain the range of different epigenetic states cells can occupy, in addition to the other anti-cancer benefits of fasting/FMD/starvation (Figure 4B).
Figure 4. Physiological concentrations of Acetyl-CoA and SAM are rate limiting for HATs, DNMTs, and HMTs and affect chromatin modifications. (A) Dietary changes that limit cellular SAM and Acetyl-CoA substrate concentrations are expected to reduce biochemical reactions mediating chromatin acetylation and methylation dynamics because these substrates lie within concentrations that are enzymatically rate limiting. (B) The hypothesized benefits of starvation periods or fasting-mimicking diets (FMD) are summarized and could include decreased acetylation and methylation reaction kinetics that help impair tumor cell diversity and adaptability.
SAM is an essential cofactor for histone and DNA methyltransferases and is an intermediate of one-carbon metabolism derived from dietary methionine and synthesized through the methionine and folate metabolic cycles (Figure 5). Dietary methionine restriction causes significant alterations to cellular DNA methylation, histone methylation and gene expression (Mentch et al., 2015; Su et al., 2016), though we know less about effects on cellular epigenetic diversity or temporal dynamics. Recent work in mice found that a methionine-restricted diet rapidly altered methionine and sulfur metabolism, inhibiting tumor growth and increasing tumor susceptibility to chemotherapy and radiation (Gao et al., 2019). These results suggest decreased capabilities for tumor cells to adapt to the treatment strikes. However, further studies are needed to determine whether a methionine-restricted diet can help prevent the development of drug resistance either by reducing cellular epigenetic diversity and/or adaptability over time. Starvation or FMD cycles in mice have been shown to significantly improve survival, increase time to progression and even restore drug responsiveness to previously resistant tumors (Lee et al., 2012; Brandhorst et al., 2015; Caffa et al., 2020). Currently, we do not fully understand the mechanisms involved or to what degree methionine-restriction alone can achieve the benefits of starvation or FMD treatments.
Figure 5. A summary of how starvation-triggered nutrient deprivations converge on epigenetic pathways for short-term and long-term epigenetic memory. Nutrient deprivations that affect glucose, acetate, serine and methionine levels are predicted to impact the efficiency of cellular chromatin modifying reactions. Acteyl-CoA reductions are expected to most strongly affect epigenetic dynamics for short-term memory, while changes to methionine and SAM will affect epigenetic mechanisms mediating longer-term epigenetic effects. Adapted from Reid et al. (2017).
Methionine-restriction is not expected to directly alter histone acetylation dynamics. However, nutrient deprivation or inhibition of glycolysis causes significantly decreased acetyl-CoA levels, which in turn reduces histone acetylation (Wang et al., 2009; Lee et al., 2014; Mariño et al., 2014; Cluntun et al., 2015). Acetyl-CoA abundance is a key factor controlling gene expression by affecting chromatin structure to create open chromatin sites for gene activation. In addition, Acetyl-CoA abundance affects the acetylation of transcription factors by altering their stability, subcellular localization, or abilities to bind to DNA (Choudhary et al., 2014). Acetyl-CoA is produced from pyruvate through the tricarboxylic acid (TCA) cycle or by beta-oxidation of fatty acids (Campbell and Wellen, 2018). Little is known about the effects of starvation, fasting or FMD on histone acetylation dynamics at the cellular level or over time within a cell. One study tested whether a high fat diet (HFD) affects acetyl-CoA levels and global histone acetylation in mice, uncovering tissue specific effects after a 4 week HFD (Carrer et al., 2017). They found that white adipose tissue showed significantly decreased Acetyl-CoA and histone acetylation for specific lysine residues. Thus, diet can affect global histone acetylation in a tissue dependent manner (Figure 5).
AMP-activated protein kinase (AMPK) is phosphorylated in response to starvation and inhibits anabolic glucose, lipid and protein synthesis pathways, and activates autophagy and mitophagy for the breakdown of cellular macromolecules (Herzig and Shaw, 2017). Cellular lipid stores are consumed through lipid metabolic pathways and, in an attempt to increase glucose uptake, AMPK promotes increased glucose transporter functions. Along with this energy stress response, AMPK inhibits the HAT, p300, and glucagon release during fasting causing the activation of class II HDACs and localization to the nucleus to activate transcriptional stress responses. Finally, sirtuins are activated in response to nutrient stress and use NAD+ as a substrate for deacetylation. I refer readers to a previous review detailing how Acetyl-CoA levels are dynamically responsive to nutrient availability, affecting histone acetylation and gene expression (Sivanand et al., 2018). Additionally, starvation blocks global protein translation by inhibiting mTOR complex 1 (mTORC1), which in turn limits cellular capabilities for intra and intercellular signaling and gene expression dynamics (Holcik and Sonenberg, 2005; Wullschleger et al., 2006). Recently, it has been further shown that fasting effects on gene transcription and translation depend upon the nature of the specific nutrients that are deprived (Gameiro and Struhl, 2018). Currently, we know almost nothing about how global or specific nutrient deprivations affect stochastic cellular epigenetic diversity and adaptability, indicating an important area for study.
In a baseline fed state, diverse epigenetic writers, readers and erasers are available with readily available substrates and co-factors. However, after ∼3 days of fasting, the human body transitions into an early starvation state designed to preserve cellular proteins and this involves a major switch to ketone metabolism, which shifts epigenetic regulatory processes and triggers protective stress responses (Dai et al., 2020). It is reasonable to expect that this can help to constrain and inhibit biochemical reactions that promote cellular epigenetic diversity and adaptability, but remains to be tested. New single cell omics technologies, including single cell RNASeq and ATAC-Seq can help test these predictions directly. The implication of identifying interventions that can constrain cellular diversity and adaptability is that we can couple these interventions with chemotherapy strikes and improved drugs to increase chances for a cure and complete disease elimination. If such constraints can be induced, we should expect that the type and duration of nutrient deprivations can be tailored to different cancer types, stages and lesion locations.
As described above, chromatin methylation and acetylation biochemical reaction kinetics can be controlled by limiting their substrates and cofactors through nutrient deprivation. However, another important factor constraining these biochemical reactions is body temperature, which controls reaction kinetics (e.g., Kd, dissociation constant). Whole organism metabolic rate scales with the 3/4-power of body mass and increases exponentially with temperature, up to ∼40°C when catabolic processes increase (Gillooly et al., 2001). Body temperature profoundly affects metabolic rates and vice versa. A 1% increase in body temperature has been linked to a 10–15% increase in metabolic rate in endotherms (Evans et al., 2015). On the other hand, decreasing body temperature slows biochemical reaction kinetics. Most physiological processes and biochemical reactions function optimally at ∼37°C (98°F). What temperature state might best help to drive cancer cell extinction?
As noted above, the body responds to microbial infections through a combination of fasting and fever, and the overall sickness response can eliminate cancer in patients. A high-grade fever in an adult is an oral temperature of > 39.4°C (103°F), which can begin to promote catabolic processes and the denaturation of enzymes. In contrast, reducing body temperature slows cellular reaction kinetics and decreases the metabolic rate (Evans et al., 2015). For example, hibernation (torpor) involves a profoundly decreased metabolic rate in order to survive harsh environmental conditions with low nutrient availability (Carey et al., 2003). For torpor to occur, a first step is to trigger a decrease the hypothalamic set point for body temperature, and then in turn drop the body temperature for the induction of torpor and decreased metabolic rate (Gillooly et al., 2001; Geiser, 2004). A tumor in a hibernating animal stops growing during hibernation, but then resumes growth after torpor (Lyman and Fawcett, 1954). Dangerous, transient hypometabolic hibernation-like states can also occur in human cancers and these subpopulations of persistent, dormant cancer cells are major causes of mortality, disease recurrence and treatment failure (Recasens and Munoz, 2019; Shen et al., 2020). Recently, it was shown that most cancer cells have the capability to enter a dormant (or diapause) state (Recasens and Munoz, 2019). Out of the many adaptive phenotypes cancer cell subpopulations might occupy, this hypometabolic state is one of the most dangerous. Previous studies have shown that chronic cold stress, which depresses metabolic rate and immunity, is associated with elevated risks for cancer (Bandyopadhayaya et al., 2020). Cold stress is associated with accelerated tumor growth and treatment resistance in mice, which appears to involve enhanced tumor cell survival pathways as well as suppressed anti-tumor immunity (Kokolus et al., 2013; Messmer et al., 2014). This suggests that increased temperature could help prevent cancer cell dormancy and hibernation-like states in the body (Figure 6A).
Figure 6. Fever/hyperthermia is predicted to block cellular hypometabolic states and constrain epigenetic diversity and adaptability by impairing enzyme activity. (A) Cold stress depresses metabolic rate and promotes hypometabolic states, while warming increases metabolic rates. Warming could help block hibernation-like states in cancer cell subpopulations. (B) Fever/hyperthermia triggers rapid decreases in biochemical reaction rates due to denaturation of enzyme active sites. Thus, fever-level body temperatures may help inhibit the activity of chromatin-modifying enzymes and block epigenetic diversity and adaptability. (C,D) Compare the effects of hyperthermia versus hypothermia and suggest that hyperthermic treatments could have multiple beneficial effects for cancer elimination. (E) Plot shows that combining starvation/FMD with fever/hyperthermia could be a potent intervention constraining tumor cell epigenetic diversity and adaptability. (F) A hypothesis for the benefits of combining starvation/FMD with fever/hyperthermia.
So far, thinking in the cancer field has primarily focused on uncovering molecular targets that might help block cancer cell dormancy using targeted drugs (Recasens and Munoz, 2019). However, transiently elevating body temperature and metabolic rates might be effective, particularly when coupled with fasting and chemotherapy. Moreover, the activation of the immune system, which occurs in response to elevated body temperature, might beneficially boost anti-tumor immunity (Evans et al., 2015). There is clinical evidence and multiple independent studies showing that hyperthermia has therapeutically beneficial effects in cancer patients (Hildebrandt et al., 2002; van der Zee, 2002; Wust et al., 2002; Jha et al., 2016). Efforts to use hyperthermia clinically have included local delivery of microwaves or radiowaves, as well as approaches to use arrays of antennas to heat entire body parts. For metastatic patients, whole body heating approaches using radiant thermal isolation systems have been developed that can achieve systemic temperatures of 41.8–42.0°C. Perfect thermal isolation is sufficient in-and-of itself to raise the body temperature from 37.5 to 42 in a 70 kg patient in 180 min (Wust et al., 2002). In mice, simply raising the ambient temperature from 22°C to 30°C causes measurable improvements in tumor sensitivity to chemotherapy treatment (Eng et al., 2015). The benefits of hyperthermia are thought to include immune activation and the enhancement of anti-tumor immunity, increased blood perfusion and drug delivery into tumor sites, and cytotoxic effects on tumor cells growing in low pO2 and low pH conditions. Effectiveness for hyperthermia for different cancers has been reported in some randomized clinical trials (Jha et al., 2016).
In addition to beneficial immune activation, perfusion and drug uptake effects, fever/hyperthermia promotes increased cellular metabolic rates and biochemical reaction kinetics, and then at ∼40°C, causes enzyme denaturation and a rapid decline in reaction kinetics. Such effects could be applied precisely to help prevent cancer cells from occupying dangerous hypometabolic states, and at > 40°C, to help narrow the dynamic range of biochemical reaction kinetics in cells and thereby the diversity of epigenetic states cancer cells can occupy (Figures 6B–D). Overall, high-grade fever/hyperthermia may reduce cancer cell diversity and adaptability. When applied in combination with starvation/FMD, cells are struck with the combination of limited substrates and cofactors plus a forced increase in metabolic rates and enzymatic denaturation (Figures 6E,F, 7). In patients, simple approaches to transiently increase body temperature and metabolic rate could be vigorous exercise, which is difficult for patients, or dry sauna treatments (Hussain and Cohen, 2018). Dry saunas begin to raise core body temperature within 15 min (Zalewski et al., 2014). More aggressive approaches could involve pyrogen treatments, like LPS (lipopolysaccharide). Further studies are needed to test whether mimicking the vertebrate sickness response with a fasting + fever combinatorial therapy has the effect of helping to constrain tumor cell epigenetic diversity and adaptability and making cancer cells more vulnerable to chemotherapy treatment strikes.
Figure 7. Schematic depiction of the hypothesized effects of combining starvation/FMD and fever/hyperthermia on tumor cell epigenetic diversity. (A) At baseline, enzyme kinetics are optimal and all possible stochastic epigenetic cellular states can be occupied in a population of cells. (B) Starvation limits essential substrates and cofactors for chromatin modifying enzymes, constraining epigenetic dynamics the number of states that a cell can occupy. (C) Fever/hyperthermia denatures chromatin modifying enzymes, inhibiting their activity and constraining the number of different epigenetic states that a cell can potentially occupy. (D) The combination of starvation and fever/hyperthermia creates a state that severely constrains cancer cell potential for epigenetic diversity.
For decades, scientists have used nutrient withdrawal or temperature changes to synchronize the proliferation of dividing bacterial, yeast or mammalian cells in culture for experiments. The removal of serum from culture media causes cells to withdraw from the cell cycle (Pardee, 1974; Zetterberg and Larsson, 1985; Balsalobre et al., 1998). Then, the subsequent re-introduction of serum triggers the cell population to synchronously re-enter the cell cycle, homogenizing the cell population. Repeated serum removal cycles can synchronize the division of 80% of cells in culture (Bánfalvi, 2011; Tian et al., 2012). Similarly, reducing and then increasing the ambient temperature can synchronize dividing cells in culture (Rieder and Cole, 2002; Bánfalvi, 2017). This suggests that cycles of starvation, re-feeding and increased temperature could be combined in patients and timed with chemotherapy treatments to help reduce cellular diversity by synchronizing tumor cell populations for maximum killing during a chemotherapy treatment strike (Figure 8). Different schedules might be developed for different phases of treatment for complete elimination of metastatic disease.
Figure 8. Cycles of transient starvation/FMD are predicted to help synchronize tumor cell division and further reduce heterogeneity. At baseline tumor cells are dividing at different rates and are in different stages of the cell cycle. Following starvation, the division of tumor cells that are sensitive to environmental nutrient concentrations is stalled. Many tumor cells are not sensitive to nutrients and continue to divide and are sensitive to chemotherapy. Subpopulations of tumor cells that are sensitive to nutrients, may stop division and enter persistent, hypometabolic states during starvation. However, upon re-feeding, nutrient sensitive tumor cells are expected to synchronously enter the cell cycle, as is observed for some cancer cell lines in vitro upon serum-induced cell cycle activation. This activation is expected to make the cell population more homogeneous and vulnerable to chemotherapy.
For a treatment regimen to have the potential to be curative, it is predicted that the treatment needs to achieve NED (no evidence of disease), thereby eliminating the majority of the disease, and then continue with diverse strikes to ultimately eliminate the remaining, yet undetectable disease (Gatenby et al., 2020). If patients are to be cured, maintaining health for a rich, long life must be integral to the approach and the functionality of the immune and digestive systems should be preserved. Thus, the major initial objectives are to (1) maximize tumor cell killing to reach NED as quickly as possible and with as few drugs as possible, and (2) to reach NED with paradigms that protect the long-term health and quality of life of the patient as much as possible (Figure 9A). By reaching NED quickly, safely and efficiently, the tumor cell population is fragmented and vulnerable to extinction with continued treatments (see below). Ideally, the approach involves delivering successive combinations of treatment strikes using different drugs that attack different mechanisms and switching to each new treatment prior to progression. Switching treatments at the disease nadir, but prior to progression potentially helps to preserve drug efficacy for future use if needed.
Figure 9. A three-phase paradigm for combining starvation/FMD/diet with hyperthermia and drug treatments to promote metastatic disease extinction. (A) The schematic depicts two phases of combinatorial treatment to eliminate the disease. In Phase I, the goal is to rapidly drive the disease to NED using combinations of drug treatments, starvation and hyperthermia. In Phase II, the goal is to activate, sensitive and kill persistent and dormant tumor cells in the body that will lead to the recurrence of the disease in the future. In Phase III, the goal is to drive the disease to extinction by combining a continuous drug treatment that enables a high quality of life with randomized starvation, intermittent fasting, dietary and hyperthermic interventions that cause unpredictable and different metabolic states to eliminate remaining disease and prevent recurrence. (B) In Phase I, by delivering drug treatment strikes during starvation periods (day 4 of starvation or FMD), tumor cells are sensitized to treatment and healthy cells are protected, improving drug efficacy and protecting patient health and quality of life. By including hyperthermia (HT) during drug treatment strikes, drug penetration into tumor sites may increase, hypometabolic states may be blocked and tumor cell diversity and adaptability are expected to be impaired. (C) In Phase II, the drug strikes and HT are applied during the re-feeding period after a starvation/FMD cycle, where the goal is to flush out persistent hypometabolic cancer cells and drive them into cell division, homogenize them and kill them with treatment. (D) In Phase III, continuous oral chemo or targeted therapies are used in combination with randomized and unpredictable metabolic switches to disrupt the epigenetic and gene expression cancer cell landscapes to break emerging drug resistant phenotypes and stochastically induce drug sensitive states over time, ultimately leading to complete disease extinction.
Toward achieving these goals in actual patients, coupling starvation and hyperthermia cycles with strikes of cytotoxic chemotherapy infusions is expected to enhance tumor cell killing by (1) sensitizing the tumor to treatment, (2) reducing growth signals driving tumor proliferation (e.g., glucose, insulin, IGF1, leptin), (3) impairing capabilities to sustain metabolically demanding drug resistance mechanisms, (4) disrupting the Warburg effect, (5) boosting anti-tumor immunity through hyperthermia, (6) improving vasodilation and drug delivery to tumor cells, and finally, (7) constraining capabilities for cellular epigenetic diversity, adaptability and dormancy during treatment (Figure 9B). Moreover, starvation appears to protect normal cells from the cytotoxic effects of chemotherapies by reducing division and metabolic activity, while a majority of tumor cells continue to divide and be affected, resulting in differential sensitivity to chemotherapy treatment (Lee et al., 2012). Thus, integrating starvation and hyperthermia cycles into the first phase of attack and applying the chemotherapy strikes during the starvation response could help the patient reach NED quickly and with fewer drugs and treatment cycles. Moreover, it may help protect the health of the patient during the aggressive treatment or even enable a more aggressive regimen to achieve NED.
Once NED is achieved, the next major challenge is to eliminate any persistent, hibernating tumor cells that entered dormancy and have evaded treatment, but will cause disease recurrence in the future (Figures 9A,C). During this stage, when the tumor cell population is fragmented and vulnerable, chemotherapy and hyperthermia strikes might be best timed with the re-feeding phase following a starvation cycle to help activate dormant cancer cells and make them sensitive to treatment. Re-feeding after starvation appears to drive progenitor cells, stem cells and tumor cells into a rebound of increased, synchronous cell division (Stragand et al., 1979; Brandhorst et al., 2015), though more studies are needed. As noted above, cells in culture synchronously re-enter the cell cycle upon the return of serum or shift from cold to warm temperature. However, while this approach is predicted to help activate and sensitize persistent, hibernating tumor cells to chemotherapy treatment, it is also expected to increase unwanted damage to healthy cell populations that also enter the cell cycle upon re-feeding. Therefore, this hypothetical approach for eliminating hibernating tumor cells might be best applied in a limited and strategic manner when NED has been reached and there is a chance to now cure the disease with continued aggressive therapy.
Finally, I propose a third and final phase of adjuvant attack during NED that uses remaining drugs in the clinical arsenal that are designed for continuous chronic delivery and have low side effects, such as oral chemotherapies (e.g., Capecitabine) or targeted therapies (e.g., aromatase inhibitors and/or Palbociclib, etc.). Frequently, these would be the first line therapies, but would be delivered chronically until progression. Instead, here they are delivered in this final adjuvant phase to drive disease extinction. The continuous chronic delivery is potentially advantageous for treating slow dividing and dormant tumor cells. Importantly however, this chronic drug treatment is now coupled with randomized and unpredictable starvation, fasting, dietary and hyperthermic metabolic switches
(Figure 9D). The idea is that by inducing randomized and different metabolic states during treatment, the metabolic, epigenetic and gene expression states of the tumor cells are forced into flux and occupy many different molecular states during the chronic drug treatment. Selection for drug resistance is strongly driven by the application of chronic, unchanging treatment conditions and unpredictable environmental changes are primary factor in driving extinction (Gatenby et al., 2019, 2020). Therefore, by enforcing randomized metabolic switching, one aims to (i) force any drug resistant cellular states to be transient and disrupted before a dangerous new resistant cell lineage becomes stable, (ii) drive dormant tumor cells into states of vulnerability to the treatment, and (iii) stochastically “screen” different molecular states in the remaining tumor cell population to uncover those that sensitize different cells to the drug. Randomly perturbing metabolic pathways during chronic treatment over time likely offers one of the best chances of driving tumor cells throughout the body into different molecular states that break evolutionary trajectories and expose drug-sensitive vulnerabilities that ultimately lead to extinction. Devising optimal approaches to do this is an important area for study and we already have an understanding of different dietary and fasting strategies for metabolic switching that the field can start with (Nencioni et al., 2018; Tajan and Vousden, 2020).
Most importantly for clinical applications, there are reasons to believe that this type of program will be tolerable and safe and easily to integrate into the care setting. Pre-clinical studies of FMD in mice involved treatments that are typically chronic in patients, including fulvestrant and palbociclib, and are safe and effective (Caffa et al., 2020). Further, in FMD clinical studies of patients taking drugs during the fasting and re-feeding periods, the approach appears safe (de Groot et al., 2020). Nonetheless, the starvation-to-re-feeding pulse with drug strikes warrants further investigation. The addition of hyperthermia during re-feeding, which will increase perfusion and drug delivery, might be best reserved for drugs with low probabilities of causing neuropathy. The timing of starvation + hyperthermic interventions might also be most effective when timed with the known pharmacokinetics of the drugs to maximize the tumor cell-killing window. For example, palbociclib reaches peak plasma concentrations 6–12 h after oral administration and a steady state in the body after 8 days of treatment, suggesting that starvation, re-feeding and/or hyperthermic interventions are best done 8 days into the typical 21 day treatment cycle and 6–12 h after taking a dose. One question is whether the re-feeding period can be optimized with specific nutrients intended to activate cancer cell populations that especially depend on glucose, amino acid or fatty acid metabolism, or other nutrients. Additionally, supplementing fasting, hyperthermia and chemotherapy strikes with small molecule drugs that impair major metabolic pathways, like inhibitors of heat shock proteins, ubiquitin mediated protein degradation, mTOR signaling or others, may increase the epigenetic homogenization of tumor cells and/or help disrupt epigenetic and gene expression states of drug resistance. These general concepts need to be tested for safety and efficacy in mouse models and tested for associations with effects on tumor cell synchronization and constrained epigenetic diversity using recent paired single cell RNASeq and ATAC-Seq technologies. Finally, treating patients that have reached NED by scan and tumor markers leaves the clinician in the dark regarding how to gage the efficacy of such treatments. Circulating tumor DNA might be a more sensitive and effective marker during this phase to help define the bona fide extinction of the disease.
Stochastic epigenetic and gene expression states are primary drivers of the evolution of drug resistance in patients. Moreover, the diploid/polyploid genome of tumor cells likely enables profound epigenetic, allelic and gene expression diversity at the cellular level through stochastic DAE and clonal RME. These gene and allele level epigenetic and gene expression effects are barriers to the elimination of metastatic disease. To create clinical solutions, we need an understanding of the short-term and long-term epigenetic mechanisms involved, their regulation by different metabolic mechanisms and their roles in the initiation and persistence of cancer and the evolution of drug resistance. No single drug will solve the cancer evolution problem. Here, I have attempted to draw on the fields of evolution, ecology, infectious disease, epigenetics, metabolism, biochemistry, genomics and oncology to synthesize a clinically feasible path forward to help homogenize tumor cell populations and constrain cellular epigenetic diversity and adaptability in patients. The rationale for pursuing this objective is to improve the efficacy of combinatorial chemotherapy strikes to cure metastatic disease. I propose an approach that involves combining cycles of starvation and hyperthermia to synchronize tumor cell division and constrain tumor cell epigenetic diversity and adaptability by transiently limiting essential nutrients, substrates and cofactors and impairing the optimal enzymatic activity of chromatin modifying enzymes when drug treatment strikes are delivered. I then propose inducing unpredictable different metabolic states during continuous oral drug treatments to drive the disease to extinction. In addition, I speculate that strategic increases in body temperature may help block cancer cells from entering dangerous hypometabolic, hibernation-like states during treatment, which are major barriers to disease elimination. Whether these manipulations can actually reduce tumor cell epigenetic diversity, stochastic variability and adaptability and improve outcomes is not known and remains to be tested using state-of-the-art single cell genomics methods and pre-clinical studies. Nonetheless, based on simple biochemical principles, it is reasonable to expect that carefully timed, transient starvation and hyperthermic cycles will limit the range of molecular states tumor cells can occupy during chemotherapy infusion treatments. Further, given the evidence that drug resistance is a stochastic and dynamic molecular state that cells are able to move in and out of, rather than a genetically hardwired state, it is also reasonable to expect that unpredictable switches of metabolic states over time during continuous treatment can help to disrupt drug resistant epigenetic and gene expression states and induce drug sensitive states in tumor cells. This especially important when the available drugs in the arsenal are limited.
In summary, to achieve cancer cell extinction, I propose a three-phase paradigm. In Phase I, starvation and hyperthermia cycles are timed with chemotherapy strikes in a manner that is anticipated to protect the patient and enable aggressive treatments that help to rapidly drive metastatic disease to NED. In Phase II, chemotherapy treatment strikes and hyperthermia are timed with the re-feeding phase after a starvation cycle, where the goal is to help activate, sensitize and eliminate persistent, hibernating cancer cells and help completely wipe out the disease. In Phase III, continuous chemo or targeted therapies that enable improved quality of life are combined with randomized and diverse metabolic state switches using different starvation, fasting, dietary and hyperthermia treatments to drive extinction. This paradigm integrates knowledge from different fields, including the ability of the vertebrate infection response to eliminate cancer. There are many unknowns and opportunities for study in this area to help patients.
CG conceived and wrote the article.
This work was supported by grants to CG from the National Institutes of Health, including R01MH109577, R01AG064013, R21AG067350, R21MH118570, and R21MH120468.
CG was a co-founder and has financial interests in Storyline Health Inc. www.storylinehealth.com, which is creating artificial intelligence technologies to improve behavioral health research and patient care, including for cancer patient care.
The author declares that the research was conducted in the absence of any commercial or financial relationships that could be construed as a potential conflict of interest.
I thank Elliott Ferris and Stephanie Kravitz in the Gregg Lab, and Bob Gatenby, Alana Welm, and Saundra Buys for reading and commenting on this article. I thank Joel Brown, Dawn Lemanne, and Valter Longo for helpful discussions.
Adelman, J. S., and Martin, L. B. (2009). Vertebrate sickness behaviors: Adaptive and integrated neuroendocrine immune responses. Integr. Comp. Biol. 49, 202–214. doi: 10.1093/icb/icp028
Aubert, A. (1999). Sickness and behaviour in animals: a motivational perspective. Neurosci. Biobehav. Rev. 23, 1029–1036. doi: 10.1016/s0149-7634(99)00034-2
Balázsi, G., van Oudenaarden, A., and Collins, J. J. (2011). Cellular decision making and biological noise: from microbes to mammals. Cell 144, 910–925. doi: 10.1016/j.cell.2011.01.030
Balsalobre, A., Damiola, F., and Schibler, U. (1998). A serum shock induces circadian gene expression in mammalian tissue culture cells. Cell 93, 929–937. doi: 10.1016/s0092-8674(00)81199-x
Bandyopadhayaya, S., Ford, B., and Mandal, C. C. (2020). Cold-hearted: A case for cold stress in cancer risk. J. Therm. Biol. 91:102608. doi: 10.1016/j.jtherbio.2020.102608
Bánfalvi, G. (2017). Overview of cell synchronization. Methods Mol. Biol. 1524, 3–27. doi: 10.1007/978-1-4939-6603-5_1
Baylin, S. B., and Jones, P. A. (2016). Epigenetic determinants of cancer. Cold Spring Harb. Perspect. Biol. 8:a019505. doi: 10.1101/cshperspect.a019505
Bennett, R. L., and Licht, J. D. (2018). Targeting epigenetics in cancer. Annu. Rev. Pharmacol. Toxicol. 58, 187–207. doi: 10.1146/annurev-pharmtox-010716-105106
Bintu, L., Yong, J., Antebi, Y. E., McCue, K., Kazuki, Y., Uno, N., et al. (2016). Dynamics of epigenetic regulation at the single-cell level. Science 351, 720–724. doi: 10.1126/science.aab2956
Blake, W. J., Kærn, M., Cantor, C. R., and Collins, J. J. (2003). Noise in eukaryotic gene expression. Nature 422, 633–637. doi: 10.1038/nature01546
Branciamore, S., Valo, Z., Li, M., Wang, J., Riggs, A. D., and Singer-Sam, J. (2018). Frequent monoallelic or skewed expression for developmental genes in CNS-derived cells and evidence for balancing selection. Proc. Natl. Acad. Sci. U.S.A. 115, E10379–E10386. doi: 10.1073/pnas.1808652115
Brandhorst, S., Choi, I. Y., Wei, M., Cheng, C.-W., Sedrakyan, S., Navarrete, G., et al. (2015). A Periodic diet that mimics fasting promotes multi-system regeneration, enhanced cognitive performance, and healthspan. Cell Metab. 22, 86–99. doi: 10.1016/j.cmet.2015.05.012
Caffa, I., Spagnolo, V., Vernieri, C., Valdemarin, F., Becherini, P., Wei, M., et al. (2020). Fasting-mimicking diet and hormone therapy induce breast cancer regression. Nature 583, 620–624. doi: 10.1038/s41586-020-2502-7
Campbell, S. L., and Wellen, K. E. (2018). Metabolic signaling to the nucleus in cancer. Mol. Cell 71, 398–408. doi: 10.1016/j.molcel.2018.07.015
Carey, H. V., Andrews, M. T., and Martin, S. L. (2003). Mammalian hibernation: cellular and molecular responses to depressed metabolism and low temperature. Physiol. Rev. 83, 1153–1181. doi: 10.1152/physrev.00008.2003
Carrer, A., Parris, J. L. D., Trefely, S., Henry, R. A., Montgomery, D. C., Torres, A., et al. (2017). Impact of a high-fat diet on tissue acyl-coa and histone acetylation levels. J. Biol. Chem. 292, 3312–3322. doi: 10.1074/jbc.M116.750620
Carroll, S. B. (2008). Evo-devo and an expanding evolutionary synthesis: a genetic theory of morphological evolution. Cell 134, 25–36. doi: 10.1016/j.cell.2008.06.030
Chandradoss, K. R., Chawla, B., Dhuppar, S., Nayak, R., Ramachandran, R., Kurukuti, S., et al. (2020). CTCF-mediated genome architecture regulates the dosage of mitotically stable mono-allelic expression of autosomal genes. Cell Rep. 33:108302. doi: 10.1016/j.celrep.2020.108302
Cheng, Y., He, C., Wang, M., Ma, X., Mo, F., Yang, S., et al. (2019). Targeting epigenetic regulators for cancer therapy: mechanisms and advances in clinical trials. Signal Trans. Target. Ther. 4:62. doi: 10.1038/s41392-019-0095-0
Choudhary, C., Weinert, B. T., Nishida, Y., Verdin, E., and Mann, M. (2014). The growing landscape of lysine acetylation links metabolism and cell signalling. Nat. Rev. Mol. Cell Biol. 15, 536–550. doi: 10.1038/nrm3841
Cluntun, A. A., Huang, H., Dai, L., Liu, X., Zhao, Y., and Locasale, J. W. (2015). The rate of glycolysis quantitatively mediates specific histone acetylation sites. Cancer Metab. 3:10. doi: 10.1186/s40170-015-0135-3
Coward, J., and Harding, A. (2014). Size does matter: why polyploid tumor cells are critical drug targets in the war on cancer. Front. Oncol. 4:123. doi: 10.3389/fonc.2014.00123
Dai, Z., Ramesh, V., and Locasale, J. W. (2020). The evolving metabolic landscape of chromatin biology and epigenetics. Nat. Rev. Genet. 21, 737–753. doi: 10.1038/s41576-020-0270-8
Dantzer, R., and Kelley, K. W. (2007). Twenty years of research on cytokine-induced sickness behavior. Brain Behav. Immun. 21, 153–160. doi: 10.1016/j.bbi.2006.09.006
Davidson, E. H. (2010). Emerging properties of animal gene regulatory networks. Nature 468, 911–920. doi: 10.1038/nature09645
Davidson, E. H., and Erwin, D. H. (2006). Gene regulatory networks and the evolution of animal body plans. Science 311, 796–800. doi: 10.1126/science.1113832
de Cabo, R., and Mattson, M. P. (2019). Effects of intermittent fasting on health, aging, and disease. N. Engl. J. Med. 381, 2541–2551. doi: 10.1056/NEJMra1905136
de Groot, S., Lugtenberg, R. T., Cohen, D., Welters, M. J. P., Ehsan, I., Vreeswijk, M. P. G., et al. (2020). Fasting mimicking diet as an adjunct to neoadjuvant chemotherapy for breast cancer in the multicentre randomized phase 2 DIRECT trial. Nat. Commun. 11:3083. doi: 10.1038/s41467-020-16138-3
Del Monte, U. (2009). Does the cell number 10(9) still really fit one gram of tumor tissue? Cell Cycle 8, 505–506. doi: 10.4161/cc.8.3.7608
Deng, Q., Ramskold, D., Reinius, B., and Sandberg, R. (2014). Single-cell RNA-seq reveals dynamic, random monoallelic gene expression in mammalian cells. Science 343, 193–196. doi: 10.1126/science.1245316
Dobosz, P., and Dzieciątkowski, T. (2019). The Intriguing History of Cancer Immunotherapy. Front. Immunol. 10:2965. doi: 10.3389/fimmu.2019.02965
Easwaran, H., Tsai, H.-C., and Baylin, S. B. (2014). Cancer epigenetics: tumor heterogeneity, plasticity of stem-like states, and drug resistance. Mol. Cell 54, 716–727. doi: 10.1016/j.molcel.2014.05.015
Eckersley-Maslin, M. A., Thybert, D., Bergmann, J. H., Marioni, J. C., Flicek, P., and Spector, D. L. (2014). Random monoallelic gene expression increases upon embryonic stem cell differentiation. Dev. Cell 28, 351–365. doi: 10.1016/j.devcel.2014.01.017
Eng, J. W. L., Reed, C. B., Kokolus, K. M., Pitoniak, R., Utley, A., Bucsek, M. J., et al. (2015). Housing temperature-induced stress drives therapeutic resistance in murine tumour models through β2-adrenergic receptor activation. Nat. Commun. 6:6426. doi: 10.1038/ncomms7426
Evans, S. S., Repasky, E. A., and Fisher, D. T. (2015). Fever and the thermal regulation of immunity: the immune system feels the heat. Nat. Rev. Immunol. 15, 335–349. doi: 10.1038/nri3843
Feinberg, A. P., and Irizarry, R. A. (2010). Stochastic epigenetic variation as a driving force of development, evolutionary adaptation, and disease. Proc. Natl. Acad. Sci. U.S.A. 107, 1757–1764. doi: 10.1073/pnas.0906183107
Flavahan, W. A., Gaskell, E., and Bernstein, B. E. (2017). Epigenetic plasticity and the hallmarks of cancer. Science 357:eaal2380. doi: 10.1126/science.aal2380
Fox, D. T., Soltis, D. E., Soltis, P. S., Ashman, T.-L., and Van de Peer, Y. (2020). Polyploidy: a biological force from cells to ecosystems. Trends Cell Biol. 30, 688–694. doi: 10.1016/j.tcb.2020.06.006
Gaiti, F., Chaligne, R., Gu, H., Brand, R. M., Kothen-Hill, S., Schulman, R. C., et al. (2019). Epigenetic evolution and lineage histories of chronic lymphocytic leukaemia. Nature 569, 576–580. doi: 10.1038/s41586-019-1198-z
Gameiro, P. A., and Struhl, K. (2018). Nutrient deprivation elicits a transcriptional and translational inflammatory response coupled to decreased protein synthesis. Cell Rep. 24, 1415–1424. doi: 10.1016/j.celrep.2018.07.021
Gao, X., Sanderson, S. M., Dai, Z., Reid, M. A., Cooper, D. E., Lu, M., et al. (2019). Dietary methionine influences therapy in mouse cancer models and alters human metabolism. Nature 572, 397–401. doi: 10.1038/s41586-019-1437-3
Gatenby, R., and Brown, J. (2018). The evolution and ecology of resistance in cancer therapy. Cold Spring Harb. Perspect. Med. 8:a033415. doi: 10.1101/cshperspect.a033415
Gatenby, R. A., Artzy-Randrup, Y., Epstein, T., Reed, D. R., and Brown, J. S. (2020). Eradicating metastatic cancer and the eco-evolutionary dynamics of anthropocene extinctions. Cancer Res. 80, 613–623. doi: 10.1158/0008-5472.CAN-19-1941
Gatenby, R. A., Zhang, J., and Brown, J. S. (2019). First strike-second strike strategies in metastatic cancer: lessons from the evolutionary dynamics of extinction. Cancer Res. 79, 3174–3177. doi: 10.1158/0008-5472.CAN-19-0807
Geiser, F. (2004). Metabolic rate and body temperature reduction during hibernation and daily torpor. Annu. Rev. Physiol. 66, 239–274. doi: 10.1146/annurev.physiol.66.032102.115105
Gendrel, A.-V., Attia, M., Chen, C.-J., Diabangouaya, P., Servant, N., Barillot, E., et al. (2014). Developmental dynamics and disease potential of random monoallelic gene expression. Dev. Cell 28, 366–380. doi: 10.1016/j.devcel.2014.01.016
Gillooly, J. F., Brown, J. H., West, G. B., Savage, V. M., and Charnov, E. L. (2001). Effects of size and temperature on metabolic rate. Science 293, 2248–2251. doi: 10.1126/science.1061967
Gimelbrant, A., Hutchinson, J. N., Thompson, B. R., and Chess, A. (2007). Widespread monoallelic expression on human autosomes. Science 318, 1136–1140. doi: 10.1126/science.1148910
Guo, M., Peng, Y., Gao, A., Du, C., and Herman, J. G. (2019). Epigenetic heterogeneity in cancer. Biomark Res 7:23. doi: 10.1186/s40364-019-0174-y
Hart, B. L. (1988). Biological basis of the behavior of sick animals. Neurosci. Biobehav. Rev. 12, 123–137. doi: 10.1016/s0149-7634(88)80004-6
Herzig, S., and Shaw, R. J. (2017). AMPK: guardian of metabolism and mitochondrial homeostasis. Cell Res. 19, 121–135. doi: 10.1038/nrm.2017.95
Hildebrandt, B., Wust, P., Ahlers, O., Dieing, A., Sreenivasa, G., Kerner, T., et al. (2002). The cellular and molecular basis of hyperthermia. Critic. Rev. Oncol. Hematol. 43, 33–56. doi: 10.1016/s1040-8428(01)00179-2
Hinohara, K., Wu, H.-J., Vigneau, S., McDonald, T. O., Igarashi, K. J., Yamamoto, K. N., et al. (2018). KDM5 histone demethylase activity links cellular transcriptomic heterogeneity to therapeutic resistance. Cancer Cell 34, 939–953.e9. doi: 10.1016/j.ccell.2018.10.014
Hogg, S. J., Beavis, P. A., Dawson, M. A., and Johnstone, R. W. (2020). Targeting the epigenetic regulation of antitumour immunity. Nat. Rev. Drug Discov. 19, 776–800. doi: 10.1038/s41573-020-0077-5
Holcik, M., and Sonenberg, N. (2005). Translational control in stress and apoptosis. Nat. Rev. Mol. Cell Biol. 6, 318–327. doi: 10.1038/nrm1618
Huang, S. (2011). On the intrinsic inevitability of cancer: from foetal to fatal attraction. Semin. Cancer Biol. 21, 183–199. doi: 10.1016/j.semcancer.2011.05.003
Huang, S. (2012). Tumor progression: chance and necessity in darwinian and lamarckian somatic (mutationless) evolution. Prog. Biophys. Mol. Biol. 110, 69–86. doi: 10.1016/j.pbiomolbio.2012.05.001
Huang, S. (2013). Genetic and non-genetic instability in tumor progression: link between the fitness landscape and the epigenetic landscape of cancer cells. Cancer Metastasis Rev. 32, 423–448. doi: 10.1007/s10555-013-9435-7
Huang, S. (2021). Reconciling non-genetic plasticity with somatic evolution in cancer. Trends Cancer 7, 309–322. doi: 10.1016/j.trecan.2020.12.007
Huang, S., Ernberg, I., and Kauffman, S. (2009). Cancer attractors: a systems view of tumors from a gene network dynamics and developmental perspective. Semin. Cell Dev. Biol. 20, 869–876. doi: 10.1016/j.semcdb.2009.07.003
Huang, W.-C., Bennett, K., and Gregg, C. (2018). Epigenetic and cellular diversity in the brain through allele-specific effects. Trends Neurosci. 41, 925–937. doi: 10.1016/j.tins.2018.07.005
Huang, W.-C., Ferris, E., Cheng, T., Hörndli, C. S., Gleason, K., Tamminga, C., et al. (2017). Diverse non-genetic, allele-specific expression effects shape genetic architecture at the cellular level in the mammalian brain. Neuron 93, 1094–1109.e7. doi: 10.1016/j.neuron.2017.01.033
Hussain, J., and Cohen, M. (2018). Clinical effects of regular dry sauna bathing: a systematic review. Evid. Based Complement. Alternat. Med. 2018:1857413. doi: 10.1155/2018/1857413
Jeffries, A. R., Uwanogho, D. A., Cocks, G., Perfect, L. W., Dempster, E., Mill, J., et al. (2016). Erasure and reestablishment of random allelic expression imbalance after epigenetic reprogramming. RNA 22, 1620–1630. doi: 10.1261/rna.058347.116
Jenkinson, G., Pujadas, E., Goutsias, J., and Feinberg, A. P. (2017). Potential energy landscapes identify the information- theoretic nature of the epigenome. Nat. Genet. 49, 719–729. doi: 10.1038/ng.3811
Jha, S., Sharma, P. K., and Malviya, R. (2016). Hyperthermia: role and risk factor for cancer treatment. ALS 10, 161–167. doi: 10.1016/j.als.2016.11.004
Kærn, M., Elston, T. C., Blake, W. J., and Collins, J. J. (2005). Stochasticity in gene expression: from theories to phenotypes. Nat. Rev. Genet. 6, 451–464. doi: 10.1038/nrg1615
Katan-Khaykovich, Y., and Struhl, K. (2002). Dynamics of global histone acetylation and deacetylation in vivo: rapid restoration of normal histone acetylation status upon removal of activators and repressors. Genes. Dev. 16, 743–752. doi: 10.1101/gad.967302
Knoechel, B., Roderick, J. E., Williamson, K. E., Zhu, J., Lohr, J. G., Cotton, M. J., et al. (2014). An epigenetic mechanism of resistance to targeted therapy in T cell acute lymphoblastic leukemia. Nat. Genet. 46, 364–370. doi: 10.1038/ng.2913
Kokolus, K. M., Capitano, M. L., Lee, C.-T., Eng, J. W. L., Waight, J. D., Hylander, B. L., et al. (2013). Baseline tumor growth and immune control in laboratory mice are significantly influenced by subthermoneutral housing temperature. Proc. Natl. Acad. Sci. U.S.A. 110, 20176–20181. doi: 10.1073/pnas.1304291110
Krajcovic, M., and Overholtzer, M. (2012). Mechanisms of ploidy increase in human cancers: a new role for cell cannibalism. Cancer Res. 72, 1596–1601. doi: 10.1158/0008-5472.CAN-11-3127
Kravitz, S. N., and Gregg, C. (2019). New subtypes of allele-specific epigenetic effects: implications for brain development, function and disease. Curr. Opin. Neurobiol. 59, 69–78. doi: 10.1016/j.conb.2019.04.012
Landau, D. A., Clement, K., Ziller, M. J., Boyle, P., Fan, J., Gu, H., et al. (2014). Locally disordered methylation forms the basis of intratumor methylome variation in chronic lymphocytic leukemia. Cancer Cell 26, 813–825. doi: 10.1016/j.ccell.2014.10.012
Larsson, A. J. M., Johnsson, P., Hagemann-Jensen, M., Hartmanis, L., Faridani, O. R., Reinius, B., et al. (2018). Genomic encoding of transcriptional burst kinetics. Nature 565, 251–254. doi: 10.1038/s41586-018-0836-1
Lee, C., Raffaghello, L., Brandhorst, S., Safdie, F. M., Bianchi, G., Martin-Montalvo, A., et al. (2012). Fasting cycles retard growth of tumors and sensitize a range of cancer cell types to chemotherapy. Sci. Transl. Med. 4:124ra27. doi: 10.1126/scitranslmed.3003293
Lee, J. V., Carrer, A., Shah, S., Snyder, N. W., Wei, S., Venneti, S., et al. (2014). Akt-dependent metabolic reprogramming regulates tumor cell histone acetylation. Cell Metab. 20, 306–319. doi: 10.1016/j.cmet.2014.06.004
Levine, J. H., Lin, Y., and Elowitz, M. B. (2013). Functional roles of pulsing in genetic circuits. Science 342, 1193–1200. doi: 10.1126/science.1239999
Li, X. (2002). Tissue-specific regulation of retinal and pituitary precursor cell proliferation. Science 297, 1180–1183. doi: 10.1126/science.1073263
Liau, B. B., Sievers, C., Donohue, L. K., Gillespie, S. M., Flavahan, W. A., Miller, T. E., et al. (2017). Adaptive chromatin remodeling drives glioblastoma stem cell plasticity and drug tolerance. Stem Cell 20, 233–246.e7. doi: 10.1016/j.stem.2016.11.003
Locasale, J. W., and Cantley, L. C. (2011). Metabolic flux and the regulation of mammalian cell growth. Cell Metab. 14, 443–451. doi: 10.1016/j.cmet.2011.07.014
Lomvardas, S., and Maniatis, T. (2016). Histone and DNA modifications as regulators of neuronal development and function. Cold Spring Harb. Perspect. Biol. 8:a024208. doi: 10.1101/cshperspect.a024208
Lyman, C. P., and Fawcett, D. W. (1954). The effect of hibernation on the growth of sarcoma in the hamster. Cancer Res. 14, 25–28.
Madlung, A. (2012). Polyploidy and its effect on evolutionary success: old questions revisited with new tools. Heredity 110, 99–104. doi: 10.1038/hdy.2012.79
Maley, C. C., Aktipis, A., Graham, T. A., Sottoriva, A., Boddy, A. M., Janiszewska, M., et al. (2017). Classifying the evolutionary and ecological features of neoplasms. Nat. Rev. Cancer 17, 605–619. doi: 10.1038/nrc.2017.69
Mariño, G., Pietrocola, F., Eisenberg, T., Kong, Y., Malik, S. A., Andryushkova, A., et al. (2014). Regulation of autophagy by cytosolic acetyl-coenzyme A. Mol. Cell 53, 710–725. doi: 10.1016/j.molcel.2014.01.016
Marusyk, A., Janiszewska, M., and Polyak, K. (2020). Intratumor heterogeneity: the rosetta stone of therapy resistance. Cancer Cell 37, 471–484. doi: 10.1016/j.ccell.2020.03.007
McGranahan, N., Favero, F., de Bruin, E. C., Birkbak, N. J., Szallasi, Z., and Swanton, C. (2015). Clonal status of actionable driver events and the timing of mutational processes in cancer evolution. Sci. Transl. Med. 7:283ra54. doi: 10.1126/scitranslmed.aaa1408
McGranahan, N., and Swanton, C. (2017). Clonal heterogeneity and tumor evolution: past. present, and the future. Cell 168, 613–628. doi: 10.1016/j.cell.2017.01.018
Mentch, S. J., Mehrmohamadi, M., Huang, L., Liu, X., Gupta, D., Mattocks, D., et al. (2015). Histone methylation dynamics and gene regulation occur through the sensing of one-carbon metabolism. Cell Metab. 22, 861–873. doi: 10.1016/j.cmet.2015.08.024
Merlo, L. M. F., Pepper, J. W., Reid, B. J., and Maley, C. C. (2006). Cancer as an evolutionary and ecological process. Nat. Rev. Cancer 6, 924–935. doi: 10.1038/nrc2013
Messmer, M. N., Kokolus, K. M., Eng, J. W. L., Abrams, S. I., and Repasky, E. A. (2014). Mild cold-stress depresses immune responses: implications for cancer models involving laboratory mice. Bioessays 36, 884–891. doi: 10.1002/bies.201400066
Miyanari, Y., and Torres-Padilla, M.-E. (2013). Control of ground-state pluripotency by allelic regulation of Nanog. Nature 483, 470–473. doi: 10.1038/nature10807
Monahan, K., Horta, A., and Lomvardas, S. (2019). LHX2- and LDB1-mediated trans interactions regulate olfactory receptor choice. Nature 565, 448–453. doi: 10.1038/s41586-018-0845-0
Nencioni, A., Caffa, I., Cortellino, S., and Longo, V. D. (2018). Fasting and cancer: molecular mechanisms and clinical application. Nat. Rev. Cancer 18, 707–719. doi: 10.1038/s41568-018-0061-0
Ng, K. K., Yui, M. A., Mehta, A., Siu, S., Irwin, B., Pease, S., et al. (2018). A stochastic epigenetic switch controls the dynamics of T-cell lineage commitment. Elife 7:e37851. doi: 10.7554/eLife.37851
Onuchic, V., Lurie, E., Carrero, I., Pawliczek, P., Patel, R. Y., Rozowsky, J., et al. (2018). Allele-specific epigenome maps reveal sequence-dependent stochastic switching at regulatory loci. Science 361:eaar3146. doi: 10.1126/science.aar3146
Ozbudak, E. M., Thattai, M., Kurtser, I., Grossman, A. D., and van Oudenaarden, A. (2002). Regulation of noise in the expression of a single gene. Nat. Genet. 31, 69–73. doi: 10.1038/ng869
Pardee, A. B. (1974). A restriction point for control of normal animal cell proliferation. Proc. Natl. Acad. Sci. U.S.A. 71, 1286–1290. doi: 10.1073/pnas.71.4.1286
Pastore, A., Gaiti, F., Lu, S. X., Brand, R. M., Kulm, S., Chaligne, R., et al. (2019). Corrupted coordination of epigenetic modifications leads to diverging chromatin states and transcriptional heterogeneity in CLL. Nat. Commun. 10:1874. doi: 10.1038/s41467-019-09645-5
Pisco, A. O., Brock, A., Zhou, J., Moor, A., Mojtahedi, M., Jackson, D., et al. (2013). Non-darwinian dynamics in therapy-induced cancer drug resistance. Nat. Commun. 4:2467. doi: 10.1038/ncomms3467
Pujadas, E., and Feinberg, A. P. (2012). Regulated noise in the epigenetic landscape of development and disease. Cell 148, 1123–1131. doi: 10.1016/j.cell.2012.02.045
Raj, A., Rifkin, S. A., Andersen, E., and van Oudenaarden, A. (2010). Variability in gene expression underlies incomplete penetrance. Nature 463, 913–918. doi: 10.1038/nature08781
Raj, A., and van Oudenaarden, A. (2008). Nature, nurture, or chance: stochastic gene expression and its consequences. Cell 135, 216–226. doi: 10.1016/j.cell.2008.09.050
Rajagopal, N., Ernst, J., Ray, P., Wu, J., Zhang, M., Kellis, M., et al. (2014). Distinct and predictive histone lysine acetylation patterns at promoters, enhancers, and gene bodies. G3 (Bethesda) 4, 2051–2063. doi: 10.1534/g3.114.013565
Recasens, A., and Munoz, L. (2019). Targeting cancer cell dormancy. Trends Pharmacol. Sci. 40, 128–141. doi: 10.1016/j.tips.2018.12.004
Reed, D. R., Metts, J., Pressley, M., Fridley, B. L., Hayashi, M., Isakoff, M. S., et al. (2020). An evolutionary framework for treating pediatric sarcomas. Cancer 126, 2577–2587. doi: 10.1002/cncr.32777
Reid, M. A., Dai, Z., and Locasale, J. W. (2017). The impact of cellular metabolism on chromatin dynamics and epigenetics. Cell Res. 19, 1298–1306. doi: 10.1038/ncb3629
Reinius, B., Mold, J. E., Ramsköld, D., Deng, Q., Johnsson, P., Michaëlsson, J., et al. (2016). Analysis of allelic expression patterns in clonal somatic cells by single-cell RNA-seq. Nat. Genet. 48, 1430–1435. doi: 10.1038/ng.3678
Reinius, B., and Sandberg, R. (2015). Random monoallelic expression of autosomal genes: stochastic transcription and allele-level regulation. Nat. Rev. Genet. 16, 653–664. doi: 10.1038/nrg3888
Reiter, J. G., Baretti, M., Gerold, J. M., Makohon-Moore, A. P., Daud, A., Iacobuzio-Donahue, C. A., et al. (2019). An analysis of genetic heterogeneity in untreated cancers. Nat. Rev. Cancer 19, 639–650. doi: 10.1038/s41568-019-0185-x
Reiter, J. G., Makohon-Moore, A. P., Gerold, J. M., Heyde, A., Attiyeh, M. A., Kohutek, Z. A., et al. (2018). Minimal functional driver gene heterogeneity among untreated metastases. Science 361, 1033–1037. doi: 10.1126/science.aat7171
Rieder, C. L., and Cole, R. (2002). Cold-shock and the mammalian cell cycle. Cell Cycle 1, 168–174. doi: 10.4161/cc.1.3.119
Risom, T., Langer, E. M., Chapman, M. P., Rantala, J., Fields, A. J., Boniface, C., et al. (2018). Differentiation-state plasticity is a targetable resistance mechanism in basal-like breast cancer. Nat. Commun. 9:3815. doi: 10.1038/s41467-018-05729-w
Rv, P., Sundaresh, A., Karunyaa, M., Arun, A., and Gayen, S. (2021). Autosomal Clonal Monoallelic Expression: natural or Artifactual? Trends Genet 37, 206–211. doi: 10.1016/j.tig.2020.10.011
Shaffer, S. M., Dunagin, M. C., Torborg, S. R., Torre, E. A., Emert, B., Krepler, C., et al. (2017). Rare cell variability and drug-induced reprogramming as a mode of cancer drug resistance. Nature 546, 431–435. doi: 10.1038/nature22794
Sharma, S. V., Lee, D. Y., Li, B., Quinlan, M. P., Takahashi, F., Maheswaran, S., et al. (2010). A chromatin-mediated reversible drug-tolerant state in cancer cell subpopulations. Cell 141, 69–80. doi: 10.1016/j.cell.2010.02.027
Shen, S., Vagner, S., and Robert, C. (2020). Persistent cancer cells: the deadly survivors. Cell 183, 860–874. doi: 10.1016/j.cell.2020.10.027
Singer, Z. S., Yong, J., Tischler, J., Hackett, J. A., Altinok, A., Surani, M. A., et al. (2014). Dynamic heterogeneity and dna methylation in embryonic stem cells. Mol. Cell 55, 319–331. doi: 10.1016/j.molcel.2014.06.029
Sivanand, S., Viney, I., and Wellen, K. E. (2018). Spatiotemporal control of acetyl-coa metabolism in chromatin regulation. Trends Biochem. Sci. 43, 61–74. doi: 10.1016/j.tibs.2017.11.004
Stragand, J. J., Braunschweiger, P. G., Pollice, A. A., and Schiffer, L. M. (1979). Cell kinetic alterations in murine mammary tumors following fasting and refeeding. Eur. J. Cancer 15, 281–286. doi: 10.1016/0014-2964(79)90038-0
Su, X., Wellen, K. E., and Rabinowitz, J. D. (2016). Metabolic control of methylation and acetylation. Curr. Opin. Chem. Biol. 30, 52–60. doi: 10.1016/j.cbpa.2015.10.030
Symmons, O., Chang, M., Mellis, I. A., Kalish, J. M., Park, J., Suszták, K., et al. (2019). Allele-specific RNA imaging shows that allelic imbalances can arise in tissues through transcriptional bursting. PLoS Genet. 15:e1007874. doi: 10.1371/journal.pgen.1007874
Tajan, M., and Vousden, K. H. (2020). Dietary approaches to cancer therapy. Cancer Cell 37, 767–785. doi: 10.1016/j.ccell.2020.04.005
Tian, Y., Luo, C., Lu, Y., Tang, C., and Ouyang, Q. (2012). Cell cycle synchronization by nutrient modulation. Integr. Biol. (Camb) 4, 328–334. doi: 10.1039/c2ib00083k
Tokheim, C. J., Papadopoulos, N., Kinzler, K. W., Vogelstein, B., and Karchin, R. (2016). Evaluating the evaluation of cancer driver genes. Proc. Natl. Acad. Sci. U.S.A. 113, 14330–14335. doi: 10.1073/pnas.1616440113
Tomasetti, C., Li, L., and Vogelstein, B. (2017). Stem cell divisions, somatic mutations, cancer etiology, and cancer prevention. Science 355, 1330–1334. doi: 10.1126/science.aaf9011
Tomasetti, C., Marchionni, L., Nowak, M. A., Parmigiani, G., and Vogelstein, B. (2015). Only three driver gene mutations are required for the development of lung and colorectal cancers. Proc. Natl. Acad. Sci. U.S.A. 112, 118–123. doi: 10.1073/pnas.1421839112
Trefely, S., Lovell, C. D., Snyder, N. W., and Wellen, K. E. (2020). Compartmentalised acyl-CoA metabolism and roles in chromatin regulation. Mol. Metab. 38:100941. doi: 10.1016/j.molmet.2020.01.005
Tubiana, M. (1989). Tumor cell proliferation kinetics and tumor growth rate. Acta Oncol. 28, 113–121. doi: 10.3109/02841868909111193
Van de Peer, Y., Mizrachi, E., and Marchal, K. (2017). The evolutionary significance of polyploidy. Nat. Rev. Genet. 18, 411–424. doi: 10.1038/nrg.2017.26
van der Zee, J. (2002). Heating the patient: a promising approach? Ann. Oncol. 13, 1173–1184. doi: 10.1093/annonc/mdf280
Vigneau, S., Vinogradova, S., Savova, V., and Gimelbrant, A. (2018). High prevalence of clonal monoallelic expression. Nat. Genet. 50, 1198–1199. doi: 10.1038/s41588-018-0188-7
Wang, A., Huen, S. C., Luan, H. H., Yu, S., Zhang, C., Gallezot, J.-D., et al. (2016). Opposing effects of fasting metabolism on tissue tolerance in bacterial and viral inflammation. Cell 166, 1512–1525.e12. doi: 10.1016/j.cell.2016.07.026
Wang, P., González, M. C., Hidalgo, C. A., and Barabási, A.-L. (2009). Understanding the spreading patterns of mobile phone viruses. Science 324, 1071–1076. doi: 10.1126/science.1167053
Wei, M., Brandhorst, S., Shelehchi, M., Mirzaei, H., Cheng, C.-W., Budniak, J., et al. (2017). Fasting-mimicking diet and markers/risk factors for aging, diabetes, cancer, and cardiovascular disease. Sci. Transl. Med. 9:eaai8700. doi: 10.1126/scitranslmed.aai8700
Werner, B., Case, J., Williams, M. J., Chkhaidze, K., and Temko, D. (2019). Measuring single cell divisions in human cancers from multi-region sequencing data. biorxiv.[Preprint] doi: 10.1101/560243
Wray, G. A. (2007). The evolutionary significance of cis-regulatory mutations. Nat. Rev. Genet. 8, 206–216. doi: 10.1038/nrg2063
Wullschleger, S., Loewith, R., and Hall, M. N. (2006). TOR signaling in growth and metabolism. Cell 124, 471–484. doi: 10.1016/j.cell.2006.01.016
Wust, P., Hildebrandt, B., Sreenivasa, G., Rau, B., Gellermann, J., Riess, H., et al. (2002). Hyperthermia in combined treatment of cancer. Lancet Oncol. 3, 487–497. doi: 10.1016/s1470-2045(02)00818-5
Zalewski, P., Zawadka-Kunikowska, M., Słomko, J., Szrajda, J., Klawe, J. J., Tafil-Klawe, M., et al. (2014). Cardiovascular and thermal response to dry-sauna exposure in healthy subjects. Physiol. J. 2014:106049. doi: 10.1155/2014/106049
Zetterberg, A., and Larsson, O. (1985). Kinetic analysis of regulatory events in G1 leading to proliferation or quiescence of Swiss 3T3 cells. Proc. Natl. Acad. Sci. U.S.A. 82, 5365–5369. doi: 10.1073/pnas.82.16.5365
Zhang, J., Deng, Y., and Khoo, B. L. (2020). Fasting to enhance Cancer treatment in models: the next steps. J. Biomed. Sci. 27:58. doi: 10.1186/s12929-020-00651-0
Keywords: epigenetics, evolution, cancer, fasting, fever, stochastic gene expression, metabolism, adaptive therapy
Citation: Gregg C (2021) Starvation and Climate Change—How to Constrain Cancer Cell Epigenetic Diversity and Adaptability to Enhance Treatment Efficacy. Front. Ecol. Evol. 9:693781. doi: 10.3389/fevo.2021.693781
Received: 12 April 2021; Accepted: 02 June 2021;
Published: 23 June 2021.
Edited by:
Christopher J. Whelan, Moffitt Cancer Center, United StatesReviewed by:
Andriy Marusyk, Moffitt Cancer Center, United StatesCopyright © 2021 Gregg. This is an open-access article distributed under the terms of the Creative Commons Attribution License (CC BY). The use, distribution or reproduction in other forums is permitted, provided the original author(s) and the copyright owner(s) are credited and that the original publication in this journal is cited, in accordance with accepted academic practice. No use, distribution or reproduction is permitted which does not comply with these terms.
*Correspondence: Christopher Gregg, Y2hyaXMuZ3JlZ2dAbmV1cm8udXRhaC5lZHU=
Disclaimer: All claims expressed in this article are solely those of the authors and do not necessarily represent those of their affiliated organizations, or those of the publisher, the editors and the reviewers. Any product that may be evaluated in this article or claim that may be made by its manufacturer is not guaranteed or endorsed by the publisher.
Research integrity at Frontiers
Learn more about the work of our research integrity team to safeguard the quality of each article we publish.