- 1Department of Information Systems, University of Haifa, Haifa, Israel
- 2Department of Geography and Environmental Studies, Shamir Research Institute, University of Haifa, Haifa, Israel
Citizen science, whereby ordinary citizens participate in scientific endeavors, is widely used for biodiversity monitoring, most commonly by relying on unstructured monitoring approaches. Notwithstanding the potential of unstructured citizen science to engage the public and collect large amounts of biodiversity data, observers’ considerations regarding what, where and when to monitor result in biases in the aggregate database, thus impeding the ability to draw conclusions about trends in species’ spatio-temporal distribution. Hence, the goal of this study is to enhance our understanding of observer-based biases in citizen science for biodiversity monitoring. Toward this goals we: (a) develop a conceptual framework of observers’ decision-making process along the steps of monitor – > record and share, identifying the considerations that take place at each step, specifically highlighting the factors that influence the decisions of whether to record an observation (b) propose an approach for operationalizing the framework using a targeted and focused questionnaire, which gauges observers’ preferences and behavior throughout the decision-making steps, and (c) illustrate the questionnaire’s ability to capture the factors driving observer-based biases by employing data from a local project on the iNaturalist platform. Our discussion highlights the paper’s theoretical contributions and proposes ways in which our approach for semi-structuring unstructured citizen science data could be used to mitigate observer-based biases, potentially making the collected biodiversity data usable for scientific and regulatory purposes.
Introduction
The world’s ecosystems are undergoing rapid and significant changes, characterized by a continuous decline in the abundance of insects, birds and mammals. From a centennial perspective, these changes are clearly evident (Attenborough, 2020). To take action in time and help in species conservation, scientists must be able to detect changes and identify warning signs much quicker (Robinson et al., 2021). However, several factors limit the ability of traditional methods to detect these changes. Traditional scientific monitoring methods rely on systematic protocols and professionally trained observers, and are thus costly and difficult to scale (Robinson et al., 2021). As a consequence, long-term and wide-scale monitoring initiatives are often limited to very few sampling sites within limited regions and to particular times, deeming the attempt to generalize to different places and times problematic. Furthermore, given the budget constraints and scientists’ focus on particular species, most of a region’s species are not monitored systematically, limiting ecologists ability to consider inter-species interactions and thus making it difficult to assess long-term trends in the ecological system.
Citizen science (CS), based on public participation in scientific research (Vohland et al., 2021), presents an alternative to traditional systematic protocols in ecological monitoring (Conrad and Hilchey, 2011; Haklay, 2013; Bonney et al., 2014; Crain et al., 2014; Wiggins and Crowston, 2015). Citizen science has been applied to multiple conservation purposes, such as estimating species dynamics, mapping species distributions and studying climate change ecology (Dickinson et al., 2010; Powney and Isaac, 2015; Callaghan et al., 2020). More broadly, citizen science is becoming a powerful means for addressing complex scientific challenges (Cooper et al., 2014; Ries and Oberhauser, 2015). The scope of CS projects for ecological monitoring has increased immensely in recent years, providing an important means for data collection, and is playing a pivotal role in conservation, management and restoration of natural environments (Bonney et al., 2009; Dickinson et al., 2010; Skarlatidou and Haklay, 2021). It is estimated that the number of CS projects increases annually by 10% (Pocock et al., 2017).
Citizen science projects can be loosely categorized along a structured-unstructured continuum (Welvaert and Caley, 2016; Kelling et al., 2019). Participants of structured projects must adhere to a formal sampling protocol, which defines all the aspects of the sampling events, including location, duration, timing, target species, etc. In contrast, unstructured, non-systematic, CS projects facilitate reporting that do not impose any guidance, and participants are free to report any species they observe without any spatio-temporal restrictions (i.e., monitoring is opportunistic). As the degree of lack of structure of a project increases, the ability to deduce statistically sound inferences substantially decreases (Kelling et al., 2019). Structured CS projects provide more verifiable data, suitable for scientific analyses, but as a trade-off might suffer from a lack of participants or funding due to the complexity of policies. In contrast, unstructured monitoring, which characterize many of CS biodiversity monitoring projects (Pocock et al., 2017), is preferable for the wide audience due to data collection flexibility, but is more susceptible to observer-based biases (Tulloch and Szabo, 2012; Isaac and Pocock, 2015; Boakes et al., 2016; Callaghan et al., 2019; Kirchhoff et al., 2021). These biases may be broadly classified into three categories: temporal, spatial and species-related biases (Isaac and Pocock, 2015). For example, observers’ reports may be spatially clustered due to ease of access to some areas, such as those close to the observer’s residence or commute route (Leitão et al., 2011; Geldmann et al., 2016; Neyens et al., 2019), and the difficulty in accessing other areas (Lawler et al., 2003; Tulloch and Szabo, 2012). Such reporting patterns yield spatial redundancies or gaps in the collected data (Callaghan et al., 2019). Similarly, observers’ temporal activity patterns and their tendency to report some species more than others may introduce additional biases.
Semi-structured projects represent a middle point between structured and unstructured protocols, allowing participants much autonomy in selecting what, where and when to monitor, but require that details of the monitoring process be reported in order to account for variation and bias in the data-collection process (Kelling et al., 2019). Such semi-structured approaches have proved highly effective in some areas. Namely, building on the long history of citizen’s involvement in birdwatching, initiatives like eBird1 (Sullivan et al., 2009, 2014) are playing an important role in tracking trends in bird population, much owing to the valuable work by the Cornell Lab of Ornithology. Still, for species other than birds, semi-structured CS projects have had a limited scientific impact. Conversely, unstructured CS projects, such as iNaturalist2, with their wide coverage of taxa, present an alternative. However, due to their inherent biases, there are relatively few scientific reports of species abundance that are based on unstructured presence-only CS data.
The untapped potential of unstructured CS provides the impetus for our research, and the objective for our research program is to develop tools and methods for accounting for biases, so as to utilize unstructured CS data to meet scientific objectives. Toward this wide-ranging objective, the goal of this paper is to provide a framework for understanding biases associated with the reporting process of unstructured citizen projects, with the expectation that our approach would be utilized to quantify biases and account for them in statistical ecological models. We propose a method for semi-structuring unstructured citizen science data by collecting additional data from observers using a questionnaire.
Prior studies proposed conceptualizations of citizen science projects, by offering a variety of typologies, for example distinguishing between unstructured, semi-structured and structured monitoring protocols (Welvaert and Caley, 2016; Kelling et al., 2019), whether reporting is intentional or not (Welvaert and Caley, 2016), and classifying projects based on their organization and governance (e.g., the degree of citizen involvement is the scientific project) (Cooper et al., 2007; Wiggins and Crowston, 2011; Shirk et al., 2012; Haklay, 2013). Here we propose an alternative to the typological perspective, offering a process-based framework that considers an individual observer’s decision-making steps. We study individual observers’ considerations, which in aggregate yield biases in the communal database of observations. To the best of our knowledge, this is the first study to introduce such a process-oriented framework for studying observer-based biases.
We draw a distinction between biodiversity monitoring citizen science projects that base their quality assurance and provenance procedures on observers’ expertise and reputation (“expertise-based”) as opposed to projects that require evidence and are based on a communal deliberation process (“evidence-based”) (Guillera-Arroita et al., 2015). As we show later, this distinction has important implications for observers’ decision-making process, as well as to the biases that are introduced during this process.
The paper continues as follows: in the next section we introduce our decision-based conceptualizations of the observation process in unstructured citizen science; we then proceed to offer an approach for semi-structuring unstructured citizen science data; we follow with an empirical illustration of our approach using data from iNaturalist; finally, we conclude with a discussion of the study’s contributions, highlighting the paper’s practical implications and discussing ways in which the study could be extended in future research.
The Proposed Framework
Our proposed framework for understanding observer-based biases is grounded in the reporting process that an observer goes through (Kéry and Schmid, 2004; Kelling et al., 2015, 2019). The framework was developed through a synthesis of the literature on the existing practices in citizen science, and then validated and refined through feedback received from practitioners. Our framework takes a decision-making approach, treating an observer’s monitoring activity as a series of decisions. These decisions may be influenced by both species-related features (e.g., species abundance in the region, the features that determine how easy it is to detect a species) and observer-related factors, such as their expertise, preferences, and monitoring equipment. Our framework is focused on the latter—observer-related biases—accounting for observer’s considerations regarding: selecting the spatial, temporal and taxonomic target for monitoring, detecting and identifying the species, and recording and sharing the observation. Figure 1 below illustrates our proposed framework.
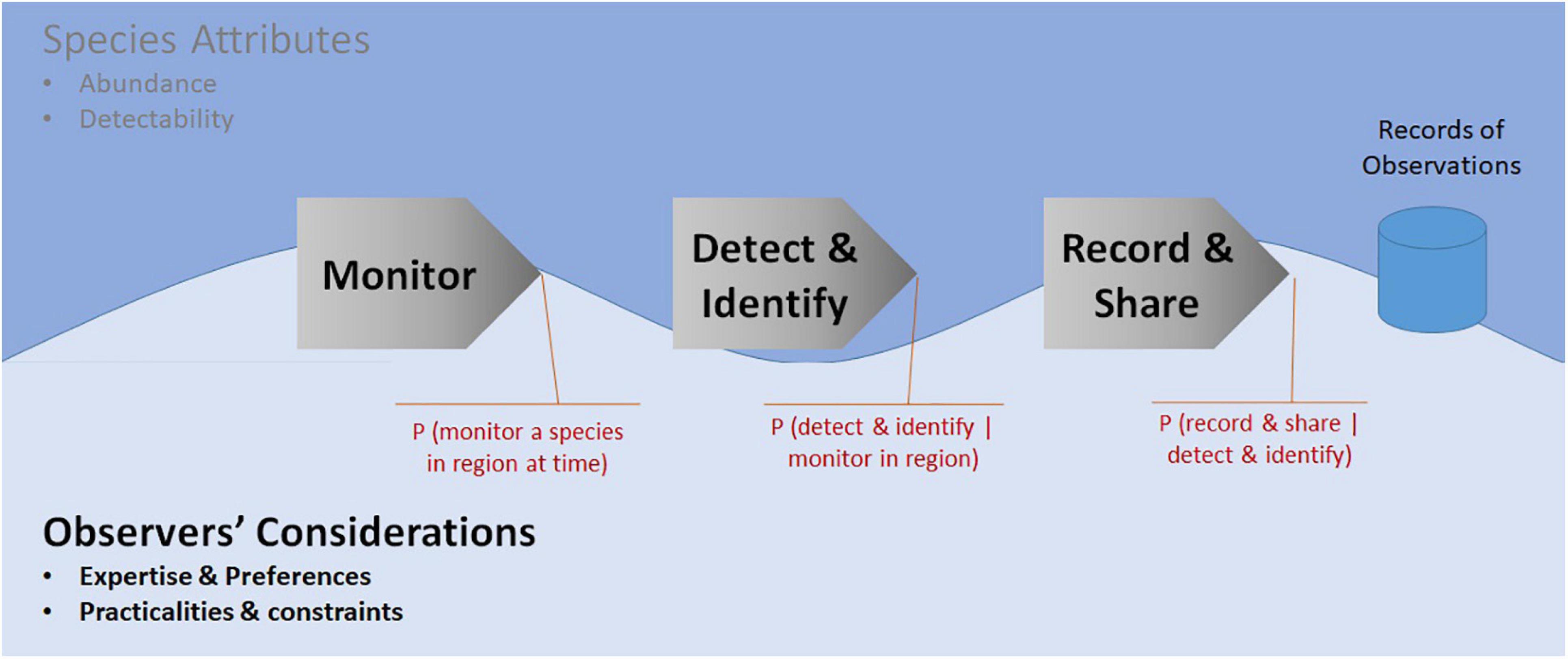
Figure 1. Our proposed framework: an observer’s participation process as a series of consideration regarding: monitoring, detecting and identifying, and recording and sharing. Assuming known species, spatial and temporal attributes, the results of each consideration is a relevant probability.
We note that this 3-phase decision-making process does not apply equally to all types of citizen science projects. For example, expertise-based projects, such as eBird, emphasize the ability to taxonomically identify the detected bird (Bonney et al., 2009; Sullivan et al., 2014). In contrast, evidence-based projects, such as iNaturalist, do not require that species are identified by the observer, as they could be identified later by other community members, based on the photo (Wiggins and He, 2016). Evidence-based projects highlight the considerations that determine the recording and sharing of species (e.g., personal preferences). Thus, the salience of each decision-making step in our proposed framework may differ between citizen science projects. During the reporting process, first an observer commonly makes a decision regarding the species or taxon one is interested in recording, the place and time where the observer would monitor (Welvaert and Caley, 2016). Once decisions regarding monitoring were made, observers’ ability to detect and identify species is influenced by the observer’s expertise and the technical equipment’s affordances, such as the camera’s zooming capabilities. While prior studies have treated detection and identification as distinct processes (Kelling et al., 2015), we opted to combine the two in a single step, because not all unstructured projects require that the species be identified prior to recording the observation. Moreover, similar factors affect observers’ considerations pertaining to detection and identification (more below). Finally, once detected and identified, observers’ inclinations (e.g., preference for a species) and practicalities will determine the decision of whether to record and share the observation. Most often, when using current reporting methods (i.e., smartphone app), the observation is automatically shared within a common database as it is recorded. However, in some cases, such as when recording the observation with a professional camera, the observation is recorded only at a later time. The equipment used and the method for sharing the observation have a significant impact on the observation’s reliability (Wiggins and He, 2016). We introduce the notion of “recordability” to refer to the likelihood of recording and sharing the observation, once the species has been detected and identified.
Putting aside species-related factors (e.g., species abundance that are outside the scope of the current analysis), observers’ considerations will influence: (a) the likelihood of monitoring a particular species in a certain place and time; (b) the likelihood of detecting and identifying the species, conditional on the probability of monitoring; and (c) the likelihood of recording and sharing the observation, conditional on the detected and identified species (i.e., recordability) (Figure 1).
Each of the three steps in our framework is influenced by considerations related to species, the geography of the region (e.g., vegetation, weather) and time (season, time of day) (August et al., 2020). The decision to monitor is shaped by the target species (or alternately, a non-targeted observation outing), the area to be monitored, and the time (season, day a week, and time of day) when the observer choses to go out for a monitoring excursion (Callaghan et al., 2019; Neyens et al., 2019). Detection and identification are influenced by the observers’ attention to a particular species, their expertise (Yu et al., 2010; Johnston et al., 2019) and the photography equipment used (e.g., the use of zoom-enabled cameras), as well as by the conditions at the place and time of observations and the factors determining visibility, such as weather conditions. For example, an expert observer is able to both detect species more easily (e.g., by relying on auditory cues) and more accurately identify them (August et al., 2020). Likewise, an equipment that enhances eyesight (e.g., zoom-enabled camera) may facilitate both easier detection and a more accurate identification (Wiggins and He, 2016). Finally, we suggest that the decision whether to record and share the observation and upload it to the online archive is determined by three factors: (a) the observer’s perceptions, for example believing it is more important to report certain species; (b) technical constraints, for example the difficulty of uploading photos that were taken using a professional camera; and (c) spatial and temporal factors, such as limited internet connection at certain areas and times. Table 1 below provides examples of how species-related, spatial and temporal factors affect observers’ considerations.
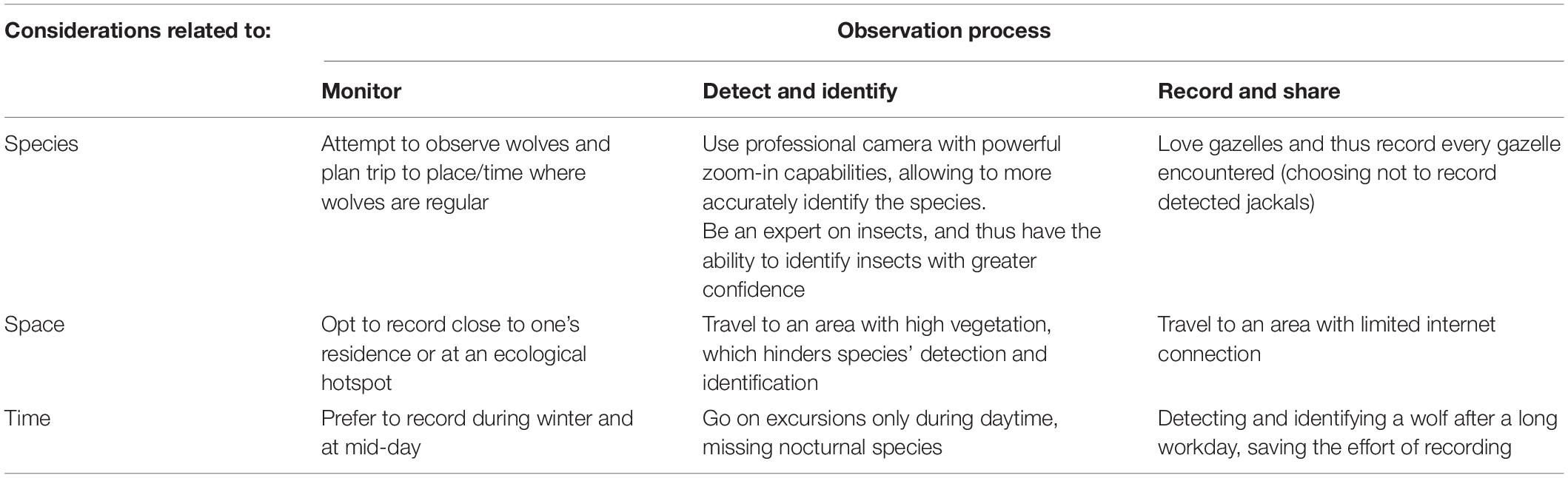
Table 1. Examples of how species-related, spatial and temporal considerations affect observers’ decisions in the three phases of the monitoring process.
Operationalizing Our Framework: Semi-Structuring Unstructured Monitoring Protocols
In this section we offer an approach for semi-structuring opportunistic citizen science protocols by collecting a limited set of basic information about how participants make their observations. Namely, we assume that the reporting protocol remains entirely unstructured, and propose to collect additional meta-data about the reporting process that would help in the interpretation of citizens’ reports. We focus our attention on data that directly corresponds to observers’ considerations related to decisions regarding: monitoring, detecting and identifying and recording and sharing species observations. Semi-structuring of opportunistic citizen science protocols is essential for mitigating various biases, such as the ones associated with estimating sampling effort, which is required for studying species richness (Walther and Martin, 2001) and abundance (Delabie et al., 2000; Aagaard et al., 2018). Doing so has the potential to dramatically improve the scientific value of citizens’ reports (Kelling et al., 2019).
Broadly speaking, data regarding observers’ decision-making process could be gathered either by directly asking observers (using a prompt in the reporting application, an interview or a questionnaire) or alternatively, by using a data-driven approach to approximate observers’ considerations through their reporting activity. Here we present an approach that is primarily based on a short questionnaire; later in this paper, where we discuss future research directions, we will present opportunities for replacing the questionnaire with a data-driven approach. In presenting the structure of the questionnaire, we will follow the three phases of our proposed framework (Figure 1), and pay particular attention to considerations that are pertinent to wide-scope and evidence-based projects.
Monitor
Observers’ choices regarding what, when, and where to go out for a monitoring trip are evident through their actual reporting activity (Boakes et al., 2016). We loosely use the term “monitoring trip” to include any trip that yields reports on observations, including observations that are taken when the initial purpose was not monitoring, such as encountering wildlife when commuting. The location of one’s observations is commonly recorded in most monitoring applications, particularly in evidence-based platforms, such as iNaturalist, that rely on photos’ automatic geo-tagging. Similarly, observers’ choices regarding when to monitor are reflected in the times when observations were taken (Callaghan et al., 2019). However, decisions regarding what species or taxon to record cannot be directly deduced from one’s reporting patterns, as species reports are also influenced by a variety of species- and observer-related considerations (see below). Thus, in order to better understand observers’ choices regarding monitoring decisions, the questionnaire includes the following question: “In your nature monitoring excursions, do you actively go out seeking a particular species? if yes, what are the species, or species categories, that you usually target?”.
Detect and Identify
The literature discusses the factors affecting species detectability, paying particular attention to species-related features (e.g., animal size, fur pattern) and behavior (e.g., diurnal or nocturnal) (Boakes et al., 2016; Robinson et al., 2021). Nonetheless, observer-related factors also influence the ability to detect a species, namely: the amount of attention devoted to monitoring, observers’ expertise and the equipment used. Wide-scope and opportunistic platforms such as iNaturalist, relay on reports taken by observers which are heterogeneous in terms of the attention they devote (some report when on leisurely nature strolls, others actively seeking wildlife) and their equipment (some use smartphones, others professional cameras with powerful zooming capabilities)3 (Kirchhoff et al., 2021). The ability to identify species is most critical in expertise-based platforms (e.g., eBird), in contrast to evidence-based platforms where the observer’s initial identification is less critical, as the community is involved in the identification process based on the photos taken. Hence, expertise-based platforms collect meta-data of observers’ expertise, or alternatively, use data-driven proxies to gauge expertise (Barata et al., 2017; Johnston et al., 2018; August et al., 2020). Thus, we suggest to include in the questionnaire questions pertaining to observers’ equipment (“What type of equipment do you use for detecting species?”) and their context when making observations (“What sort of activity are you regularly engaged in when making observations (e.g., work, leisure, actively seeking wildlife”). In addition, we suggest to include in the questionnaire the question: “What is your level of expertise in the various species categories that you report on (e.g., plants, insects, birds, mammals)? what is your source of expertise (e.g., formal education, practice, self-taught)?”.
Record and Share
The literature pays little attention to considerations pertaining to the decision whether to record and share an observation, perhaps because much of it has focused on birdwatching, where (a) the process of recording what has been identified is rather simple, and (b) observers go on excursions with the intent of reporting their observations. Wide-scope and evidence-based projects, on the other hand, differ in two fundamental ways. First, sharing of the observations once it has been identified and documented may not be simple, especially in platforms that require evidence (i.e., photos) and when one is using a professional camera (rather than the smartphone app), requiring the manual upload of the photos through a website. Second, observers make choices about what they perceive as important to record. For example, observers may have a stronger affinity to certain species and others may consider rare species as more important to document (Welvaert and Caley, 2016); in both cases, such considerations result in that many of the observations are neither recorded nor shared. To capture these preferences, we included in the questionnaire two types of questions. The first asks observers to specify their preference and affinity to a series of species (either using a Likert scale, or in ranking the species by the observer’s preference). The second asks observers “What is the likelihood that you will detect a _____ and opt not to record it [remote, low, about even, high, almost certain]?”. To limit the effort required for filling-in the questionnaire, we propose that both these questions be limited to a restricted set of species. Later, when discussing the practical implications of our study, we discuss the ability to extrapolate this information to other species.
In addition to observers’ considerations regarding what, when and where to observe species, data regarding the effort or time invested in each observation excursion is essential for utilizing citizens reports for scientific purposes (Delabie et al., 2000; Walther and Martin, 2001; Geldmann et al., 2016; Aagaard et al., 2018; Boersch-Supan et al., 2019). Quantifying effort is crucial information required for ultimately assessing species richness or abundance. Effort could be estimated automatically from reporting logs (e.g., the time from first to last observation on a particular day) or obtained directly from observers (e.g., indicating in the monitoring app when the excursion begins and ends) (Kelling et al., 2019). Alternatively, we propose to include in the questionnaire a question about the time typically spent in observation excursions, for example by indicating the percentage of excursions that are: less than an hour long, 1–2 h, 2–4 h, and more than 4 h.
Demonstrating Our Proposed Framework Using Data From an Inaturalist Project
In this section we report on a small-scale empirical study that is intended to illustrate the questionnaire’s ability to capture the factors driving observer-based biases by employing data from a local project on the iNaturalist platform.
Research Setting
The setting for this study is “Tatzpiteva” (in Hebrew, a portmanteau of “nature” and “observation”), a citizen science project that allows observers complete reporting autonomy, namely allowing them to report on any species they choose, at any place or time, while providing limited guidance and direction (i.e., the need to accurately represent species spatio-temporal distribution). Hence, such a setting is likely to reveal a broad range of observer-based considerations and biases. Namely, the observation protocols are opportunistic–as opposed to systematic monitoring that is commonly used in scientific research. Tatzpiteva, launched in January 2016, is a local citizen science initiative focused on the Golan region in northern Israel, a rural area the size of 1,200 square km, where the dominant land use are open rangelands and residents live in small towns and communities. The project is operated by the Golan Regional Council together with the University of Haifa. Observations are reported by a local community of volunteers. Tatzpiteva employs the iNaturalist4 online citizen science platform (Wiggins and He, 2016; Kirchhoff et al., 2021), whereby observers use a mobile phone (both Android and iPhone applications) and a web site. Observations are recorded using a camera and then recorded (or uploaded) to the online database; when using a smartphone app recording and sharing are performed simultaneously, unless limited internet connection delays upload; and when using a standalone camera to record observations, reporting to the website is performed at a later stage. The observer may choose to identify the species in an observation; in any case, the observation is later subject to a community-based validation process, intended to accurately identify the species. As of February 2020, approximately 33,000 observations have been reported on Tatzpiteva by 400 residents of the Golan, making up roughly half of all iNaturalist observations in Israel.
Data for this study was collected through a questionnaire that was administered by the research team, and data of observers’ activity was gathered through iNaturalist’s data export utility5. The questionnaires were sent to the 38 members comprising the local community’s core: all participants contributed a minimum of 25 observations and 8 were formally assigned “curator” privileges to the Tatzpiteva project. Twenty-seven responses were returned, where survey participants accounted for 82% of the recorded observations in this project. Insights were gained by linking observers’ activity patterns to their responses in questionnaire and interviews, where participants were given the option to provide their iNaturalist user name, assuring them anonymity (all participants have consented).
Illustrating Observers’ Considerations and the Resulting Biases
In this section we seek to demonstrate observers’ considerations by showing patterns that link their responses to a questionnaire and the observations they reported to the online system. The section is organized according to the proposed three steps in observers’ decision-making process: monitor, detect and identify and record and share. Our aim is to illustrate the concepts from our framework and make them concrete, rather than to provide strong statistical evidence for trends in species behavior or to draw conclusions regarding causality. Our analyses combine data from observers’ questionnaire and from iNaturalist logs of reported observations. In highlighting patterns that reflect observers’ considerations, we attempt to informally control for other potentially confounding factors, namely species’ characteristics and behavior. For example, when illustrating observers’ preference for species, we compare the records of two observers who live in the same village, and mostly report from the immediate vicinity of their residence at similar times (and thus are likely to encounter the same species), use similar equipment (controlling for differences in detectability) and have similar level of expertise (controlling for the ability to identify species).
Choosing Where and When to Monitor
When questioned about monitoring decisions, observers’ answers exhibit considerable variability, whereas some are going on monitoring excursions seeking to record specific species, others simply go out to nature with no particular target in mind. The differences in observations’ location, as illustrated in Figure 2, hint at observers’ spatial preferences.
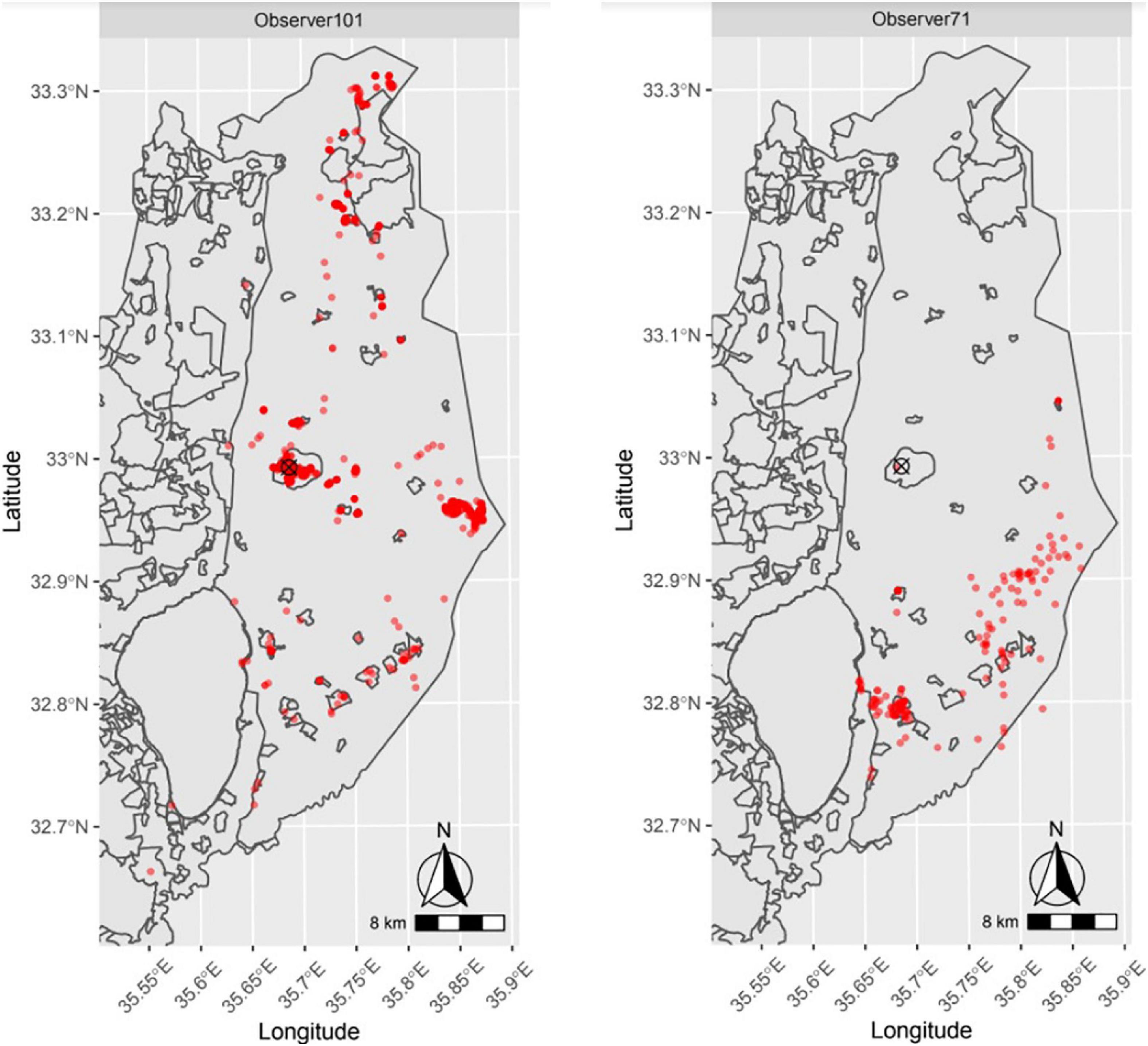
Figure 2. The geographical spread of observations for two observers who use similar photography equipment and live in the 314 same town (marked by a).
A key factor determining where observers monitor is the proximity to their residence. Observers’ residence data was obtained from the questionnaire, given that this data is not recorded on iNaturalist. As illustrated in Figure 3 below, the majority of observations are in locations close to one’s residence, with 32.5% of observations are within 5 km from residence and 18% within 1 km from residence.
The hour in the day when observations are recorded exhibit a bell-shaped distribution, with the mean at around noon time. Observers also differ in terms of the time they choose to monitor, as illustrated in Figure 4 below. When considering species daily activity patterns, it is clear that observers’ choice when to record influence the species they encounter.
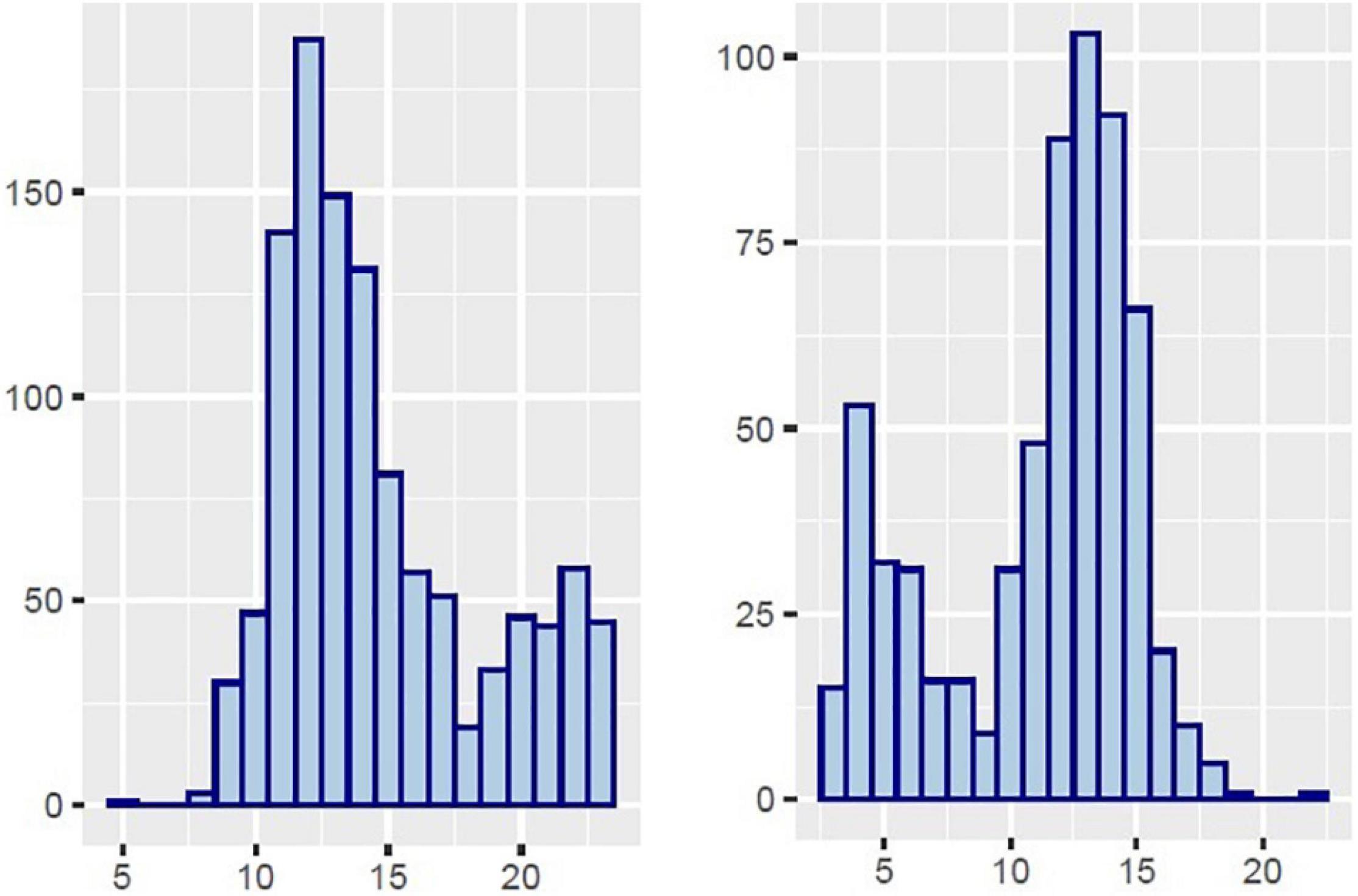
Figure 4. The percentage of observations per the hour of the day for two different observers: Observer 101 (left; active middays and nights) and Observer15 (right; active early morning and middays).
Detecting and Identifying a Species: Observers’ Expertise and Photography Equipment
iNaturalist employs a communal identification process, whereby observations move up a quality scale as more community members confirm the identification (independent of members’ expertise or tenure in the community), where the highest quality grade is “Research Grade.” Hence, if a research or a government agency were to employ an analysis only observations that have reached Research Grade status, the expertise of the person that made the observation are less relevant. Nonetheless, in the cases when all observations are used in an analysis independent of their research grade, the observer’s level of expertise may become more important. Our analysis sought to identify whether experts’ observations are of a higher quality. We compared the percent of observations to reach a Research Grade between experts and non-experts. Within the Tatzpiteva project, “curator” privileges are given to some of the experts6, with the main responsibility of helping correct the identification of others’ observations. We found that whereas 69.5% of the observations by non-experts’ (i.e., regular community members) reached Research Grade status, the percentage of curators’ observations to reach this quality grade was substantially higher: 87.9%. Furthermore, experts also contributed significantly to the quality assurance process of others’ observations: 81.1% of the observations that received a feedback on species identification by an expert reached Research Grade, compared to 53.0% that reached this quality grade after receiving feedback from non-experts.
The questionnaire responses revealed that a key factor affecting observers’ actions is the equipment they use, where the primary distinction is between those using smartphone camera (observation instantly uploaded to iNaturalist) and others who use a professional camera with powerful zoom capabilities (observations uploaded later to the web site). Those using professional cameras more often report on birds’ observations, whereas those using smartphone cameras are more likely to report on reptiles, arachnids and insects (Figure 5). Interestingly, no differences are seen in the likelihood of reporting mammals.
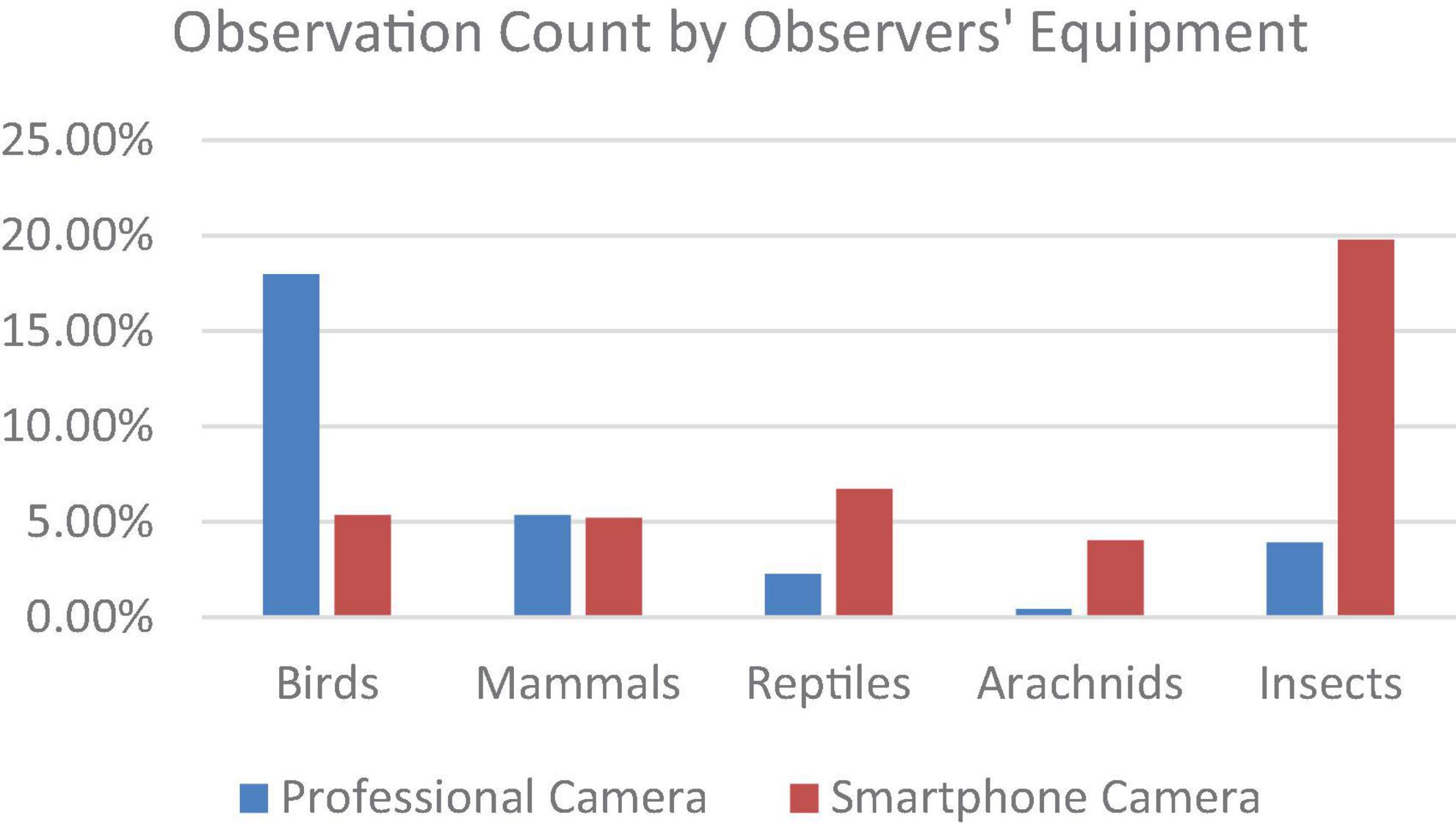
Figure 5. Differences in species distribution between observers using smartphone camera ad those using a professional camera.
Observers’ Decision What Observations to Record and Share
We studied observers’ questionnaire responses regarding recordability: the likelihood of detecting and identifying a particular species, and their decision not to record the observations. We compared observers’ responses regarding recordability for four species—gazelle, wild boar, jackal and tortoise—to their iNaturalist reporting patterns. We found that recordability is strongly correlated with observers’ count of reports, as illustrated in Figure 6 below. When comparing recordability to iNaturalist observation logs for each species distinctively, we found that the correlation persisted for each of the four species.
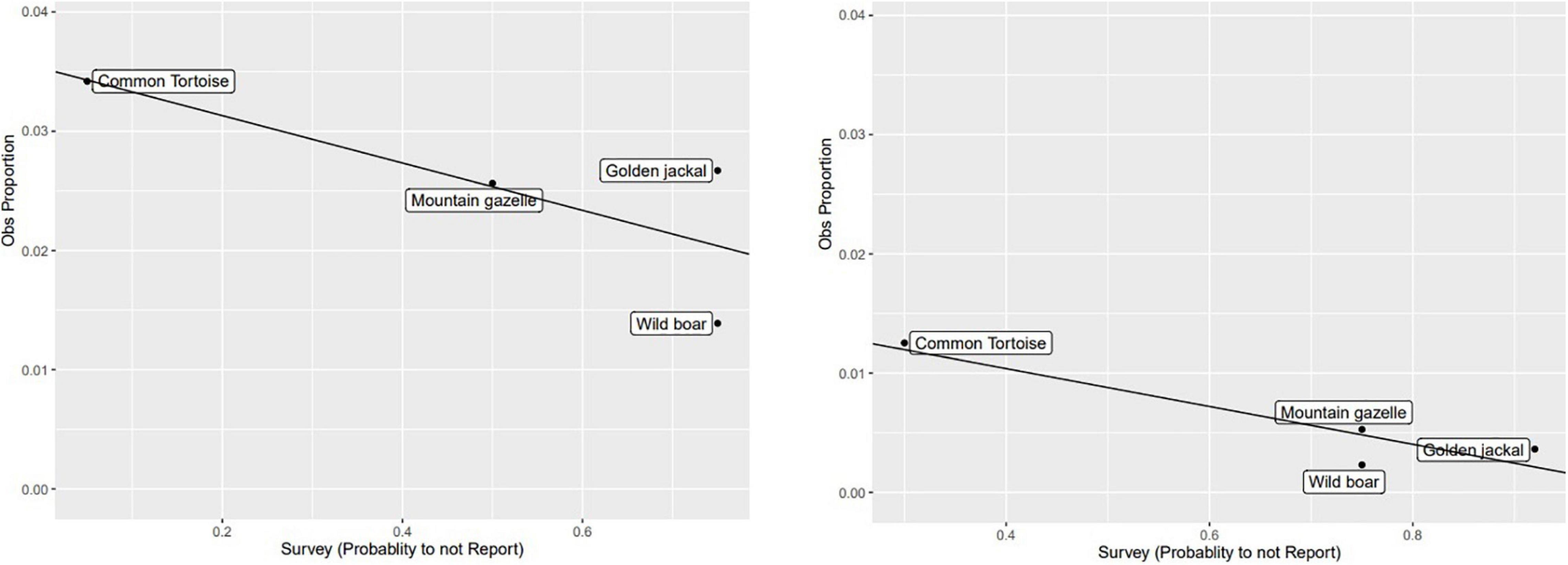
Figure 6. Compared observers’ questionnaire responses regarding recordability (X-axis) to their observation count (in percentages; Y-axis).
Observers’ Preference for Species
A key insight from the questionnaire is that observers’ preference for species or a taxon is a factor that influences all three steps of the reporting process. These preferences may reflect an emphasis on communal goals (e.g., preferring flagship species, such as gazelles in Israel), one’s hobby, an inclination to favor rare species, or the observers’ expertise. Regardless of the source of these preferences, they affect decisions regarding monitoring, detection and identification, and the recording and sharing of the observation. When deciding to monitor, the observer may choose a place and time where the species of preference is most likely to appear. Similarly, the preference to a particular species may influence observers’ attention (Dukas, 2002) and thus their ability to detect and identify the species. Lastly, observers may choose to record and share their species of preference more often than recording other species they encounter.
The questionnaires revealed that most often peoples’ preferences are articulated at the taxon level and less commonly they have a special affinity to a particular species. The differences in observers’ reporting patterns, as illustrated in Figure 7, hint at preferences for species categories.
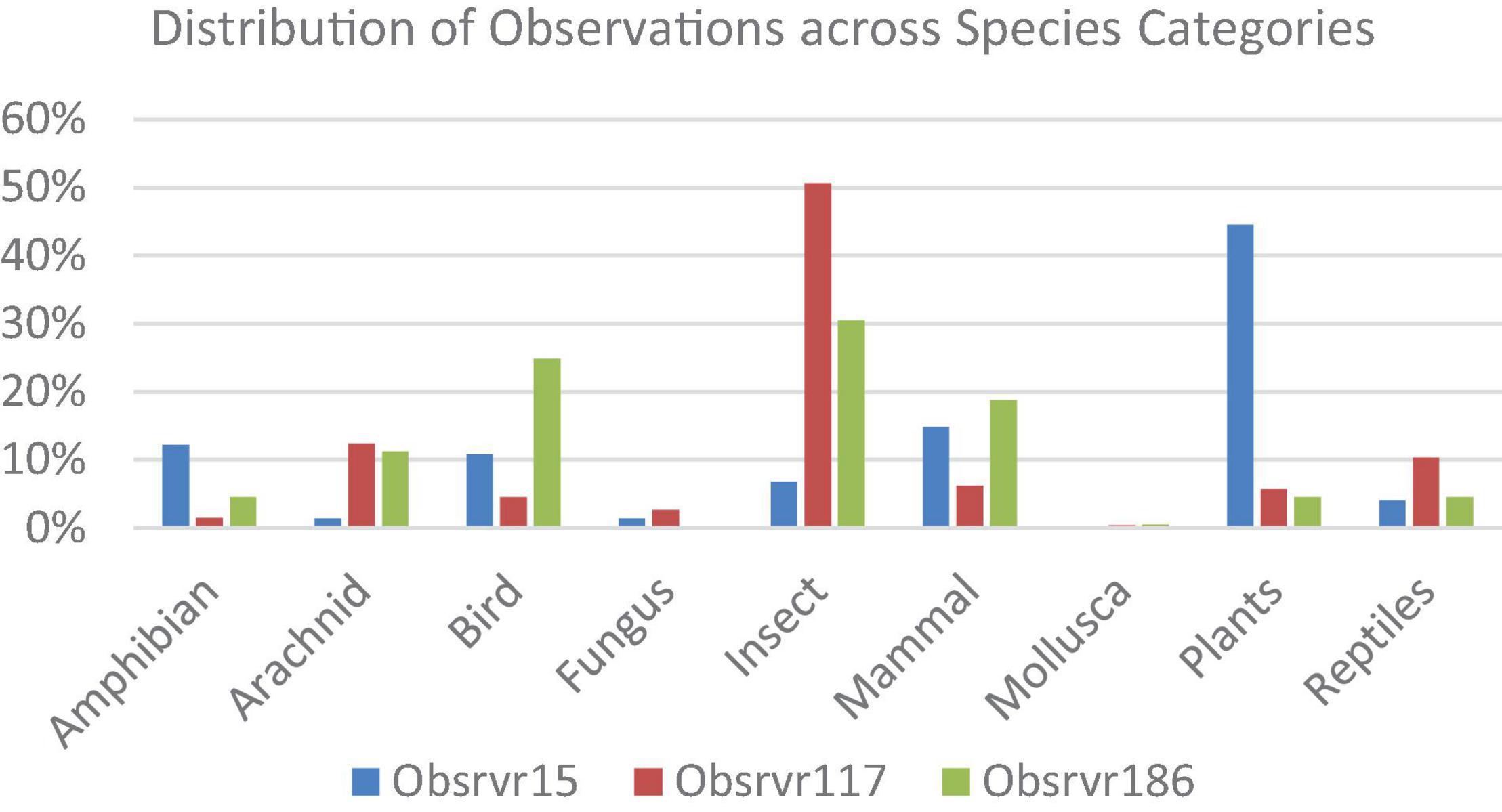
Figure 7. Distribution of observations across species categories for three observers who reside in the same settlement and use similar photography equipment.
Delving deeper, we sought evidence in the data for observers’ preference for particular species, focusing on 9 quadruped species that are common in the region: Jackal, Wild Boar, Tortoise, Porcupine, Mole Rat, Hedgehog, Fox, Gazelle, and Mongoose. We compared the observes’ reports against the questionnaire data regarding their affinity to the 9 species. Looking at the patterns for individual observers, we note substantial differences: for some the affinity to species is correlated with the observation count (Figure 8, left side), whereas for others there is no evidence for such correlation (Figure 8, right side). Interestingly, differences were also observed between species. For some species (e.g., wild boar) there is an evident relation between observers’ affinity and their reporting patters, whereas for others no such relation is observed (mole rat).
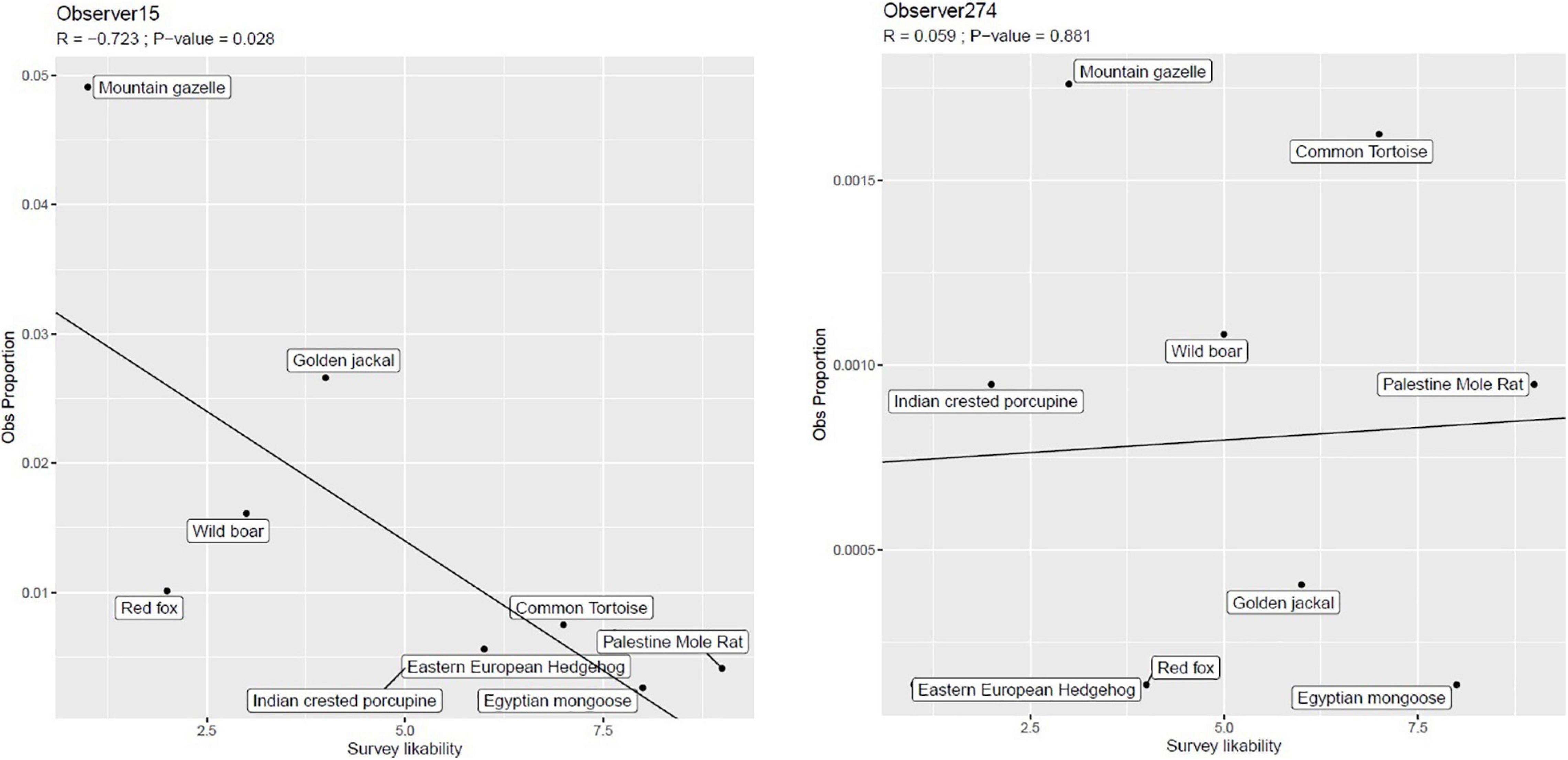
Figure 8. Observation count by the observers’ preference ranking of species (low is most preferred) for two observers. On the left, Observer15 with a clear association between preference and observation counts; on the right, Observer274 with no relation between preferences and observation count.
Discussion and Conclusion
Citizen science is widely used for biodiversity monitoring, commonly relying on unstructured monitoring protocols (Pocock et al., 2017). Notwithstanding the potential of unstructured citizen science to engage the public and to collect large amounts of biodiversity data, observers’ make various considerations regarding what, where and when to monitor, and these considerations aggregate into biases, whereby the archive of citizens’ reports does not reflect the actual species’ spatio-temporal distribution in the environment (Leitão et al., 2011; Tulloch and Szabo, 2012; Isaac and Pocock, 2015; Boakes et al., 2016; Neyens et al., 2019; August et al., 2020; Robinson et al., 2021). Our focus in this article has been to provide a framework for collecting meta-data which will facilitate more sound statistical analyses of the data. Specifically, our focus was on the biases in the data caused by variation in the observation process. We maintain that by semi-structuring unstructured citizen science data it may be possible to engage volunteers in citizen science monitoring through broad participation, while gathering sufficiently robust data which will enable rigorous analyses and allow meeting scientific objectives.
This study adds to the literature in the area by enhancing our understanding of observer-based biases in citizen science for biodiversity monitoring. Specifically, this study makes three contributions: (I) conceptual, by developing a framework of observers’ decision-making process along the steps of monitor–> detect and identify–> identify–> record and share, pointing to the considerations that take place at each step; (II) methodological, by offering an approach for semi-structuring unstructured monitoring approaches, using a targeted and focused questionnaire, and (III) empirical, by illustrating the questionnaire’s ability to capture the factors driving observer-based biases.
An important contribution of this study is in conceptualizing observers’ participation in citizen science as a sequential decision-making process. Prior studies have offered of conceptualizations of citizen science projects, by offering a variety of typologies, for example distinguishing between unstructured, semi-structured and structured monitoring protocols (Welvaert and Caley, 2016; Kelling et al., 2019), whether reporting is intentional or not (Welvaert and Caley, 2016), and classifying projects based on their organization and governance (e.g., the degree of citizen involvement in the scientific project) (Cooper et al., 2007; Wiggins and Crowston, 2011; Shirk et al., 2012; Haklay, 2013). Other conceptualizations categorize observers based on their reporting activity signatures, or profiles (Boakes et al., 2016; August et al., 2020). Here we take a somewhat different approach by offering a process-based framework that considers an individual observer’s decision-making steps. Namely, we propose a formal structure to the reporting process, which follows several cognitive stages, beginning with the decision to leave one’s home to monitor and ending in the decision to press the “report” button. From a statistical perspective, our framework suggests that observers’ decision-making process could be represented trough a sequence of conditional probabilities for observes’ actions: (1) the likelihood of monitoring a particular species at a given place and time; (2) the likelihood of detecting and identifying a species, conditional on monitoring that species at a place and time; and (3) the likelihood of recording and sharing a species’ observation, conditional on detecting and identifying the species. A statistical approach for mitigating observer-based biases, hence, should account for this sequence of probabilities.
The proposed framework is based on a synthesis of the literature, and many of the concepts we examine have been discussed in prior works (Kéry and Schmid, 2004; Kelling et al., 2015, 2019; Welvaert and Caley, 2016; Wiggins and He, 2016). Thus, the value of this framework is in offering a novel perspective for organizing these concepts in a manner that highlights the factors underlying observer-based biases. Whereas our framework is applicable to most, if not all, biodiversity monitoring projects, some important differences are worth noting. For example, the identify step in our framework precedes an observer’s decision of whether to record and share an observation, but observers in iNaturalist often report an observation absent of an identification (e.g., with the goal of learning).
While much of the research to date on biases in citizen science has focused on expert-based semi-structured projects (Sullivan et al., 2009; Fink et al., 2010; Johnston et al., 2018; Kelling et al., 2019), we focus here on wide-scope evidence-based opportunistic citizen science projects. This shift in scope brought into light less explored biases. Namely, prior research has focused primarily on biases related to detection (i.e., detectability; e.g., vegetation and species traits) and identification of observations (e.g., observers’ expertise), whereas our study emphasizes other factors. Specifically, given the great variability in the observer population and the tools they use to collect evidence, has called into attention observers’ practicalities related to detection and identification, namely observers’ photography equipment: those with professional cameras are able to better identify species. More importantly, treating the step of recording the observation as a distinct phase has underscored the importance of recordability: observers’ perceptions regarding what is important to record and the effort involved in uploading and sharing observations (more onerous for photos that are uploaded to the website after-the-fact) influence what observations end up in the database. To the best of our knowledge, this is the first study that identifies recordability as a distinct construct. The ability to capture observations’ recordability may prove essential in developing methods for mitigating observer-based biases (see below). It is important to note that an observer’s recordability for a species may change over time; for example, the observer may always report the first gazelle observations of the season, but after encountering many gazelles, may opt not to record their observations. Another important insight that emerged from our study is that the preference to a particular species or taxon is an overriding factor that drives an observer’s decisions throughout the three reporting stages we defined.
A second contribution of this study is in the methodological approach for semi-structuring unstructured citizen science data. We propose questionnaires that are highly focused and targeted at revealing the factors that underlie observers’ decisions regarding what, where and when to report. In contrast to traditional questionnaires that are designed to capture well-established psychological constructs, our questionnaire is shaped by practical considerations, especially designed to unravel the factors influencing observers’ decisions-making process. We expect that the questionnaire information could later be used for mitigating observer-based biases, thus making the citizen science data usable for scientific purposes. The proposed questionnaire somewhat resembles the metadata that is collected in semi-structured projects such as eBird (Sullivan et al., 2009). For example, Kelling et al. (2019) has recently proposed a set of metadata that should be collected in semi-structured projects, including data that is often recorded automatically (time, location, observer’s identity) and data that requires additional data entry (duration or effort, method of surveying). However, we attempt to capture, beyond this metadata, observers’ preference for species/taxon, their particular domain of expertise and considerations related to the decision of whether to record the observation (i.e., recordability). To the best of our knowledge, this is the first study to propose methods for estimating the factors underlying the decisions of what observations are reported.
We also contribute to the literature by empirically illustrating observer-based biases and by linking observers’ considerations to their actual reporting patterns. Prior studies have analyzed the spatial distribution of observers and their observations (Isaac and Pocock, 2015; Boakes et al., 2016). Here, the questionnaire offered unique data about observers, which we utilized in comparing iNaturalist reporting logs to observers’ preference for species and to their likelihood of reporting certain species once detected, allowing us to expose observers’ considerations and biases in novel ways. For example, we show that the observers’ reporting patterns are often correlated with observers’ preference for species and demonstrate that observers’ self-reported recordability data (i.e., the likelihood of recording or not, when detecting a particular species) are indicative of their actual reporting patterns.
The proposed approach for semi-structuring unstructured monitoring protocols offers important implications for researchers and government agencies that are interested in utilizing citizen science data for analyzing trends in species spatio-temporal distribution. As has been suggested in prior studies, an understanding of observer-based biases may be valuable in directing the observers in a way that mitigates theses biases. For example, citizen observers may be guided to report on all species, independent of their rarity, or even be directed to monitor under-monitored areas (Callaghan et al., 2019). Alternatively, our proposed approaches for estimating biases could be leveraged by statistical methods that attempt to correct biases in citizen science data (Wikle, 2003; Royle et al., 2012; Dorazio, 2014; Koshkina et al., 2017; Aagaard et al., 2018; Horns et al., 2018; Boersch-Supan et al., 2019; Neyens et al., 2019). In particular, we foresee that data regarding observers’ preference for species or recordability will be incorporated into statistical models that utilize citizen science data. To infer the ecological process from citizen science data it is essential to account for species’ characteristics, in particular detectability (beyond the observer-based biases that were discussed above). We note that detectability is a complex construct, as it depends on the landscape characteristics, such as vegetation cover, weather conditions and species’ morphology. Our findings point to much variability in peoples’ responses: for some, the preference for species is reflected at the taxon category, whereas others preferences are also manifested within a taxon category (e.g., strongly prefer a gazelle over a jackal). Moreover, the extent to which a preference for species predicts observers’ reporting pattern differ between species (the relation is evident for wild boar much more than it is for mole rat). These variations suggest that any bias-correction statistical model should include observer-specific and species-specific parameters.
Our proposed approach comes with its limitations, opening the door for future research in the area. Most importantly, our approach for semi-structuring unstructured citizen science data requires that a questionnaire be administered to collect data regarding observers’ considerations. Whereas the questionnaire was designed to be concise, many participants may choose not to complete it. This limitation may be addressed by future research in different ways. For example, the questionnaire may target the most active observers, thus cover a large portion of the observations in the area. In addition, we believe that statistical models may be able to extrapolate from the information about few observers, and their reporting patterns, to other observers for which this information is not available. Alternatively, it may be possible to develop methods for automatically inferring observers’ considerations. For example, prior studies have suggested that observers’ reporting patterns may be indicative of their preferences or expertise (August et al., 2020). However, given that citizens’ reports are used as evidence for species distribution, we caution against using this same data for proxying observers’ considerations (and accounting for biases), as such an approach may result in circular logic. Perhaps other types of data—e.g., from community discussion forums and the communal quality assurance process—are better suited for estimating observers’ characteristics such as their preference for species. Future research could also investigate ways for improving the questionnaire, for example by utilizing methods from behavioral psychology to more accurately represent observers’ preferences. Another limitation of our study is that it was restricted to a particular region (northern Israel) and a single citizen science platform (iNaturalist). Although the empirical data was merely employed to illustrate the biases, it is possible that an analysis of other regions and projects would reveal a different array of observer considerations and biases. Future research could also conduct large scale empirical research so as to statistically analyze the extent to which various observer considerations predict their reporting patterns, attempting to assign weights to these various biasing factors (Robinson et al., 2021). Such future research would need to operationalize some of the constructs that were loosely defined in this paper, for instance what constitutes “a region.”
An additional interesting avenue for future research is to investigate the motivational processes underlying observers’ considerations. Prior research on the motivation for participation in citizen science projects (Nov et al., 2014; Tiago et al., 2017) has employed generic frameworks such as Self Determination Theory (Ryan and Deci, 2000) or the model for collective action (Klandermans, 1997). We suggest that future research move beyond these generic conceptualizations to studying the specific motivational factors that are directly linked to observer-based biases. For example, the affinity to a particular species may be linked to a fond childhood memory, or alternatively, to an identification with national symbols. Similarly, recordability may be linked to observers’ preference for species, or alternatively, to animal-related features such as shyness and the speed at which it flees when encountering humans. We believe that a deeper understanding of the motivational dynamics underlying observers’ behavior could yield insights that may be relevant for mitigating the biases.
In conclusion, we believe that citizen science has the potential to become an important approach for biodiversity monitoring, which will overcome the limitations of traditional monitoring methods. Unstructured CS data reflects both the ecological process that determines species presence in a given location and observers’ decision-making process. Hence, for citizen science’s potential to materialize, it is essential that we deepen our understanding of the various biases that are associated with observers’ considerations, and that we identify ways for accounting for these biases in statistical models of species distribution. We hope that this study will encourage future research on the development of tools that assist scientists’ efforts for tracking trends in the worlds’ biodiversity. By enhancing our ability to detect such trends in species population, we may be able to intervene promptly, to protect the environment.
Data Availability Statement
The raw data supporting the conclusions of this article will be made available by the authors, without undue reservation.
Author Contributions
OA led the conceptual development. DM led the empirical analysis. Both authors contributed to the article and approved the submitted version.
Funding
This research was funded in part by the University of Haifa’s Data Science Research Center (DSRC).
Conflict of Interest
The authors declare that the research was conducted in the absence of any commercial or financial relationships that could be construed as a potential conflict of interest.
Acknowledgments
We thank Yuval Nov, Ariel Shamir, Keren Kaplan Mintz and Carrie Seltzer for their insightful comments on drafts of this manuscript. We also thank students at the University of Haifa - Ofri Sheelo and Maria Nitsberg - for help in data analyses.
Footnotes
- ^ https://ebird.org/, with over 700 million observations as of March 2021.
- ^ https://www.inaturalist.org/, with close to 60 million observations as of March 2021.
- ^ In contrast, the observers contributing to species-focused applications such as eBird are more homogeneous: they commonly share the goal of seeking observations, are watchful when observing nature, and often use professional photography gear.
- ^ https://www.inaturalist.org/
- ^ https://www.inaturalist.org/observations/export
- ^ It is important to draw the distinction between project-level curators (as in Tatzpiteva) and platform-level curatorship on iNaturalist. The latter entails administrative responsibilities, rather than domain-specific expertise. Namely, curators on iNaturalist employ iNaturalist’s tools to manage taxonomy and assist with various flags.
References
Aagaard, K., Lyons, J. E., and Thogmartin, W. E. (2018). Accounting for surveyor effort in large-scale monitoring programs. J. Fish Wildl. Manag. 9, 459–466. doi: 10.3996/022018-jfwm-012
Attenborough, D. A. (2020). Life on Our Planet: My Witness Statement and a Vision for the Future New York: Penguin Random House
August, T., Fox, R., Roy, D. B., and Pocock, M. J. (2020). Data-derived metrics describing the behaviour of field-based citizen scientists provide insights for project design and modelling bias. Sci. Rep. 10:11009.
Barata, I. M., Griffiths, R. A., and Ridout, M. S. (2017). The power of monitoring: optimizing survey designs to detect occupancy changes in a rare amphibian population. Sci. Rep. 7:16491.
Boakes, E. H., Gliozzo, G., Seymour, V., Harvey, M., Smith, C., Roy, D. B., et al. (2016). Patterns of contribution to citizen science biodiversity projects increase understanding of volunteers’ recording behaviour. Sci. Rep. 6:33051.
Boersch-Supan, P. H., Trask, A. E., and Baillie, S. R. (2019). Robustness of simple avian population trend models for semi-structured citizen science data is species-dependent. Biol. Conserv. 240:108286. doi: 10.1016/j.biocon.2019.108286
Bonney, R., Cooper, C. B., Dickinson, J., Kelling, S., Phillips, T., Rosenberg, K. V., et al. (2009). Citizen science: a developing tool for expanding science knowledge and scientific literacy. BioScience 59, 977–984. doi: 10.1525/bio.2009.59.11.9
Bonney, R., Shirk, J. L., Phillips, T. B., Wiggins, A., Ballard, H. L., Miller-Rushing, A. J., et al. (2014). Next steps for citizen science. Science 343, 1436–1437. doi: 10.1126/science.1251554
Callaghan, C. T., Ozeroff, I., Hitchcock, C., and Chandler, M. (2020). Capitalizing on opportunistic citizen science data to monitor urban biodiversity: a multi-taxa framework. Biol. Conserv. 251:108753. doi: 10.1016/j.biocon.2020.108753
Callaghan, C. T., Rowley, J. J., Cornwell, W. K., Poore, A. G., and Major, R. E. (2019). Improving big citizen science data: moving beyond haphazard sampling. PLoS Biol. 17:e3000357. doi: 10.1371/journal.pbio.3000357
Conrad, C. C., and Hilchey, K. G. (2011). A review of citizen science and community-based environmental monitoring: issues and opportunities. Environ. Monit. Assess. 176, 273–291. doi: 10.1007/s10661-010-1582-5
Cooper, C. B., Dickinson, J., Phillips, T., and Bonney, R. (2007). Citizen science as a tool for conservation in residential ecosystems. Ecol. Soc. 12:11.
Cooper, C. B., Shirk, J., and Zuckerberg, B. (2014). The invisible prevalence of citizen science in global research: migratory birds and climate change. PLoS One 9:e106508. doi: 10.1371/journal.pone.0106508.
Crain, R., Cooper, C., and Dickinson, J. L. (2014). Citizen science: a tool for integrating studies of human and natural systems. Ann. Rev. Environ. Resour. 39, 641–665. doi: 10.1146/annurev-environ-030713-154609
Delabie, J. H., Fisher, B. L., Majer, J. D., and Wright, I. W. (2000). “Sampling effort and choice of methods,” in Ants: Standard Methods for Measuring and Monitoring Biodiversity. Biological Diversity Handbook Series, eds D. Agosti, J. Majer, E. Alonso, and T. Schultz (Washington D.C: Smithsonian Institution Press), 145–154.
Dickinson, J. L., Zuckerberg, B., and Bonter, D. N. (2010). Citizen science as an ecological research tool: challenges and benefits, Annual review of ecology. Evol. Syst. 41, 149–172. doi: 10.1146/annurev-ecolsys-102209-144636
Dorazio, R. M. (2014). Accounting for imperfect detection and survey bias in statistical analysis of presence-only data. Glob. Ecol. Biogeogr. 23, 1472–1484. doi: 10.1111/geb.12216
Dukas, R. (2002). Behavioural and ecological consequences of limited attention. Philos. Trans. R. Soc. Lond. B Biol. Sci. 357, 1539–1547. doi: 10.1098/rstb.2002.1063
Fink, D., Hochachka, W. M., Zuckerberg, B., Winkler, D. W., Shaby, B., Munson, M. A., et al. (2010). Spatiotemporal exploratory models for broad-scale survey data. Ecol. Appl. 20, 2131–2147. doi: 10.1890/09-1340.1
Geldmann, J., Heilmann-Clausen, J., Holm, T. E., Levinsky, I., Markussen, B., Olsen, K., et al. (2016). What determines spatial bias in citizen science? Exploring four recording schemes with different proficiency requirements. Divers. Distrib. 22, 1139–1149. doi: 10.1111/ddi.12477
Guillera-Arroita, G., Lahoz-Monfort, J. J., Elith, J., Gordon, A., Kujala, H., Lentini, P. E., et al. (2015). Is my species distribution model fit for purpose? Matching data and models to applications. Glob. Ecol. Biogeogr. 24, 276–292. doi: 10.1111/geb.12268
Haklay, M. (2013). “Citizen science and volunteered geographic information: Overview and typology of participation,” in Crowdsourcing Geographic Knowledge, eds D. Sui, S. Elwood, and M. Goodchild. eds (Dordrecht: Springer), 105–122. doi: 10.1007/978-94-007-4587-2_7
Horns, J. J., Adler, F. R., and Şekercioğlu, ÇH. (2018). Using opportunistic citizen science data to estimate avian population trends. Biol. Conserv. 221, 151–159. doi: 10.1016/j.biocon.2018.02.027
Isaac, N. J., and Pocock, M. J. (2015). Bias and information in biological records. Biol. J. Linn. Soc. 115, 522–531. doi: 10.1111/bij.12532
Johnston, A., Fink, D., Hochachka, W. M., and Kelling, S. (2018). Estimates of observer expertise improve species distributions from citizen science data. Methods Ecol. Evol. 9, 88–97. doi: 10.1111/2041-210x.12838
Johnston, A., Hochachka, W., Strimas-Mackey, M., Gutierrez, V. R., Robinson, O., Miller, E., et al. (2019). Best practices for making reliable inferences from citizen science data: case study using eBird to estimate species distributions. BioRxiv 574392.
Kelling, S., Johnston, A., Bonn, A., Fink, D., Ruiz-Gutierrez, V., Bonney, R., et al. (2019). Using semistructured surveys to improve citizen science data for monitoring biodiversity. BioScience 69, 170–179. doi: 10.1093/biosci/biz010
Kelling, S., Johnston, A., Hochachka, W. M., Iliff, M., Fink, D., Gerbracht, J., et al. (2015). Can observation skills of citizen scientists be estimated using species accumulation curves? PLoS One 10:e0139600. doi: 10.1371/journal.pone.0139600
Kéry, M., and Schmid, H. (2004). Monitoring programs need to take into account imperfect species detectability. Basic Appl. Ecol. 5, 65–73. doi: 10.1078/1439-1791-00194
Kirchhoff, C., Callaghan, C. T., Keith, D. A., Indiarto, D., Taseski, G., Ooi, M. K., et al. (2021). Rapidly mapping fire effects on biodiversity at a large-scale using citizen science. Sci. Total Environ. 755:142348. doi: 10.1016/j.scitotenv.2020.142348
Koshkina, V., Wang, Y., Gordon, A., Dorazio, R. M., White, M., and Stone, L. (2017). Integrated species distribution models: combining presence-background data and site-occupany data with imperfect detection. Methods Ecol. Evol. 8, 420–430. doi: 10.1111/2041-210x.12738
Lawler, J. J., White, D., Sifneos, J. C., and Master, L. L. (2003). Rare species and the use of indicator groups for conservation planning. Conserv. Biol. 17, 875–882. doi: 10.1046/j.1523-1739.2003.01638.x
Leitão, P. J., Moreira, F., and Osborne, P. E. (2011). Effects of geographical data sampling bias on habitat models of species distributions: a case study with steppe birds in southern Portugal. Int. J. Geogr. Inform. Sci. 25, 439–454. doi: 10.1080/13658816.2010.531020
Neyens, T., Diggle, P. J., Faes, C., Beenaerts, N., Artois, T., and Giorgi, E. (2019). Mapping species richness using opportunistic samples: a case study on ground-floor bryophyte species richness in the Belgian province of Limburg. Sci. Rep. 9:19122.
Nov, O., Arazy, O., and Anderson, D. (2014). Scientists@ home: what drives the quantity and quality of online citizen science participation? PLoS One 9:e90375. doi: 10.1371/journal.pone.0090375
Pocock, M. J., Tweddle, J. C., Savage, J., Robinson, L. D., and Roy, H. E. (2017). The diversity and evolution of ecological and environmental citizen science. PLoS One 12:e0172579. doi: 10.1371/journal.pone.0172579
Powney, G. D., and Isaac, N. J. (2015). Beyond maps: a review of the applications of biological records. Biol. J. Linn. Soc. 115, 532–542. doi: 10.1111/bij.12517
Ries, L., and Oberhauser, K. (2015). A citizen army for science: quantifying the contributions of citizen scientists to our understanding of monarch butterfly biology. BioScience 65, 419–430. doi: 10.1093/biosci/biv011
Robinson, W. D., Hallman, T. A., and Hutchinson, R. A. (2021). Benchmark bird surveys help quantify counting errors and bias in a citizen-science database. Front. Ecol. Evol. 9:568278. doi: 10.3389/fevo.2021.568278
Royle, J. A., Chandler, R. B., Yackulic, C., and Nichols, J. D. (2012). Likelihood analysis of species occurrence probability from presence-only data for modelling species distributions. Methods Ecol. Evol. 3, 545–554. doi: 10.1111/j.2041-210x.2011.00182.x
Ryan, R., and Deci, E. (2000). Self-determination theory and the facilitation of intrinsic motivation, social development, and well-being. Am. Psychol. 55, 68–78. doi: 10.1037/0003-066x.55.1.68
Shirk, J. L., Ballard, H. L., Wilderman, C. C., Phillips, T., Wiggins, A., Jordan, R., et al. (2012). Public participation in scientific research: a framework for deliberate design. Ecol. Soci. 17:29.
Skarlatidou, A., and Haklay, M. (2021). Geographic Citizen Science Design: No One Left Behind. London: UCL Press.
Sullivan, B. L., Aycrigg, J. L., Barry, J. H., Bonney, R. E., Bruns, N., Cooper, C. B., et al. (2014). The eBird enterprise: an integrated approach to development and application of citizen science. Biol. Conserv. 169, 31–40. doi: 10.1016/j.biocon.2013.11.003
Sullivan, B. L., Wood, C. L., Iliff, M. J., Bonney, R. E., Fink, D., and Kelling, S. (2009). eBird: a citizen-based bird observation network in the biological sciences. Biol. Conserv. 142, 2282–2292. doi: 10.1016/j.biocon.2009.05.006
Tiago, P., Gouveia, M. J., Capinha, C., Santos-Reis, M., and Pereira, H. M. (2017). The influence of motivational factors on the frequency of participation in citizen science activities. Nat. Conserv. 18, 61–78. doi: 10.3897/natureconservation.18.13429
Tulloch, A. I., and Szabo, J. K. (2012). A behavioural ecology approach to understand volunteer surveying for citizen science datasets. Emu 112, 313–325. doi: 10.1071/mu12009
Vohland, K., Land-Zandstra, A., Ceccaroni, L., Lemmens, R., Perelló, J., Ponti, M., et al. (2021). The Science of Citizen Science. Basingstoke: Springer Nature.
Walther, B. A., and Martin, J. L. (2001). Species richness estimation of bird communities: how to control for sampling effort? Ibis 143, 413–419. doi: 10.1111/j.1474-919x.2001.tb04942.x
Welvaert, M., and Caley, P. (2016). Citizen surveillance for environmental monitoring: combining the efforts of citizen science and crowdsourcing in a quantitative data framework. SpringerPlus 5:1890.
Wiggins, A., and Crowston, K. (2011). “From Conservation to Crowdsourcing: A Typology of Citizen Science,” in Proceedings of the Hawaii International Conference on System Sciences. Piscataway: IEEE, 1–10.
Wiggins, A., and Crowston, K. (2015). Surveying the citizen science landscape. First Monday 20, 1–5. doi: 10.4324/9780203417515_chapter_1
Wiggins, A., and He, Y. (2016). “Community-based Data Validation Practices in Citizen Science,” in Proceedings Of The 19th Acm Conference On Computer-Supported Cooperative Work & Social Computing. New York: ACM. 1548–1559.
Wikle, C. K. (2003). Hierarchical Bayesian models for predicting the spread of ecological processes. Ecology 84, 1382–1394. doi: 10.1890/0012-9658(2003)084[1382:hbmfpt]2.0.co;2
Keywords: citizen science, biodiversity, monitoring, biases, framework
Citation: Arazy O and Malkinson D (2021) A Framework of Observer-Based Biases in Citizen Science Biodiversity Monitoring: Semi-Structuring Unstructured Biodiversity Monitoring Protocols. Front. Ecol. Evol. 9:693602. doi: 10.3389/fevo.2021.693602
Received: 11 April 2021; Accepted: 10 June 2021;
Published: 19 July 2021.
Edited by:
Reuven Yosef, Ben-Gurion University of the Negev, IsraelReviewed by:
Nuria Pistón, Federal University of Rio de Janeiro, BrazilMalamati Papakosta, Democritus University of Thrace, Greece
Copyright © 2021 Arazy and Malkinson. This is an open-access article distributed under the terms of the Creative Commons Attribution License (CC BY). The use, distribution or reproduction in other forums is permitted, provided the original author(s) and the copyright owner(s) are credited and that the original publication in this journal is cited, in accordance with accepted academic practice. No use, distribution or reproduction is permitted which does not comply with these terms.
*Correspondence: Ofer Arazy, oarazy@is.haifa.ac.il