- 1School of Food and Agriculture, University of Maine, Orono, ME, United States
- 2Museum of Vertebrate Zoology, University of California, Berkeley, Berkeley, CA, United States
- 3Movement Ecology Laboratory, Department of Ecology, Evolution, and Behavior, Alexander Silberman Institute of Life Sciences, The Hebrew University of Jerusalem, Jerusalem, Israel
- 4Division of Avian Diseases, Kimron Veterinary Institute, Bet Dagan, Israel
- 5Azrieli Faculty of Medicine, Bar Ilan University, Safed, Israel
- 6Department of Environmental Science Policy and Management, University of California, Berkeley, Berkeley, CA, United States
- 7School of Mathematical Sciences University of KwaZulu-Natal, Durban, South Africa
- 8Department of Integrative Biology, University of California, Berkeley, Berkeley, CA, United States
Studies in both humans and model organisms suggest that the microbiome may play a significant role in host health, including digestion and immune function. Microbiota can offer protection from exogenous pathogens through colonization resistance, but microbial dysbiosis in the gastrointestinal tract can decrease resistance and is associated with pathogenesis. Little is known about the effects of potential pathogens, such as Salmonella, on the microbiome in wildlife, which are known to play an important role in disease transmission to humans. Culturing techniques have traditionally been used to detect pathogens, but recent studies have utilized high throughput sequencing of the 16S rRNA gene to characterize host-associated microbial communities (i.e., the microbiome) and to detect specific bacteria. Building upon this work, we evaluated the utility of high throughput 16S rRNA gene sequencing for potential bacterial pathogen detection in barn swallows (Hirundo rustica) and used these data to explore relationships between potential pathogens and microbiota. To accomplish this, we first compared the detection of Salmonella spp. in swallows using 16S rRNA data with standard culture techniques. Second, we examined the prevalence of Salmonella using 16S rRNA data and examined the relationship between Salmonella-presence or -absence and individual host factors. Lastly, we evaluated host-associated bacterial diversity and community composition in Salmonella-present vs. -absent birds. Out of 108 samples, we detected Salmonella in six (5.6%) samples based on culture, 25 (23.1%) samples with unrarefied 16S rRNA gene sequencing data, and three (2.8%) samples with both techniques. We found that sex, migratory status, and weight were correlated with Salmonella presence in swallows. In addition, bacterial community composition and diversity differed between birds based on Salmonella status. This study highlights the value of 16S rRNA gene sequencing data for monitoring pathogens in wild birds and investigating the ecology of host microbe-pathogen relationships, data which are important for prediction and mitigation of disease spillover into domestic animals and humans.
Introduction
The gut microbiome, defined as the community of living microorganisms (e.g., bacteria) and non-living genetic elements (e.g., relic DNA) inhabiting the gastrointestinal tract (Berg et al., 2020), plays an important role in an individual’s development, digestion, and immune function (van der Waaij, 1989; Kohl, 2012). Intestinal microbial communities provide different functions for the host and are influenced by host diet, physiology, environment, and taxonomy (Kohl, 2012; Hird et al., 2015). Further, the microbiome is a key within-host trait that is associated with host-pathogen interactions. Infection with pathogens is linked to changes in the microbiome, as seen in mallards infected with low-pathogenic avian influenza virus (Ganz et al., 2017), as well as Marek’s disease virus (Perumbakkam et al., 2014) and Salmonella infection in chickens (Videnska et al., 2013). It is unclear whether microbial dysbiosis is a result of or a precursor to pathogen infection: commensal microbiota can promote colonization resistance, but changes in the normal microbial community can decrease colonization resistance allowing for pathogen infection (Sorbara and Pamer, 2019). Alternatively, pathogen infection can disrupt the microbial balance in the gut, or a state of dysbiosis (Lupp et al., 2007). In either case, the relationship between pathogen infection and microbiome composition highlights the need to improve our understanding of the how and when the microbiome is influenced by pathogen infections.
The introduction of massive-parallel genetic sequencing methods has dramatically advanced the field of microbial ecology, allowing for a deeper examination of the microbial communities in both humans and other animals. Traditional culture techniques are time-intensive and tend to favor microbial species that thrive in laboratory settings (Davies et al., 2000), thereby excluding the vast majority of microbial diversity present within a community (Rhoads et al., 2012). Molecular techniques, such as low- and high-throughput 16S rRNA gene metabarcoding, have helped address the underestimation of community microbial diversity using culture methods, because they increase sensitivity through sequencing a small DNA region from all bacteria, and are more time efficient (Felske et al., 1998; Schwieger and Tebbe, 1998). The advent of next-generation sequencing has greatly accelerated the number of studies characterizing the microbiome of specific organisms and environments, with some projects achieving this at a global scale (e.g., The Earth Microbiome Project, Thompson et al., 2017). This has led to many new insights into host-microbe interactions at the molecular, individual, and community levels (Rosario and Breitbart, 2011), as well as the role of the microbiome in fighting disease and stimulating the host immune response (Kohl, 2012).
Recent studies have expanded the use of 16S rRNA gene amplicon data beyond the characterization of host-associated microbiomes to the detection of bacterial pathogens with greater taxonomic specificity (Srinivasan et al., 2014; Banskar et al., 2016). Many studies comparing traditional culture techniques to sequencing methods have found that 16S rRNA gene sequencing is more sensitive and can capture a greater proportion of the microbial diversity than culture techniques (Westergren et al., 2009; Rhoads et al., 2012; Park et al., 2014; Gupta et al., 2019), although comparative results were more equivocal (Wilson et al., 2018) with differing results based on the study. In part, 16S rRNA gene amplicon sequencing might increase sensitivity because DNA can be detected from living and dead cells, as well as from residual DNA present in the environment, whereas culturing is restricted to living cells. Most studies comparing culture to 16S sequencing have been limited to humans and have focused on commensal bacteria (Rhoads et al., 2012; Gupta et al., 2019), rather than bacterial pathogens (Westergren et al., 2009). Those studies outside of humans have focused on livestock (Park et al., 2014; Wilson et al., 2018) and not wildlife, despite wild populations being important reservoirs of zoonotic disease that can spill over into humans or livestock (e.g., COVID-19 likely originated from a bat host; Lu et al., 2020; Zhou et al., 2020).
Salmonella is a genus of bacteria that contains several pathogenic strains capable of being transmitted among wildlife, domestic animals, and humans as well as through the environment where it naturally resides in the soil (Wiedemann et al., 2014; Aung et al., 2020). Salmonella may be naturally occurring in the gastrointestinal tract and harmless in small quantities, but can become pathogenic when significantly increased in abundance, as is the case with some strains of Escherichia coli (Tizard, 2004). When Salmonella becomes pathogenic, it causes the disease known as salmonellosis and can result in symptoms such as diarrhea, fever, and lethargy, although some infected individuals may be asymptomatic. Infections can occur through fecal-oral contact via contaminated food or water, direct animal contact, and occasionally from person to person (Tizard, 2004). Wild avian hosts often serve as reservoirs for Salmonella (Gargiulo et al., 2018), and sometimes are the source of outbreaks in human and livestock populations (e.g., Foti et al., 2009). Furthermore, Salmonella outbreaks have been known to rapidly emerge in songbird (Passeriformes) populations largely due to the use of bird feeders, where high-density aggregations of birds increase the likelihood of transmission to other wild and domestic species (Tizard, 2004; Giovannini et al., 2012). A recent Salmonella outbreak resulted in die-offs of songbirds across multiple states within the United States (Machemer, 2021; Mansfield and Lehman, 2021). Therefore, pathogen monitoring in birds is critical for reducing the likelihood of spillover events to susceptible wildlife, domestic animals, and humans. Monitoring can entail not just estimating the prevalence of a bacterial pathogen, but also examining its relationship with the host’s microbial community.
A healthy microbiome may affect host health and immune response by preventing successful colonization of invading bacteria (colonization resistance), acting as a first line of defense against pathogens, and by modulating immune signaling (Sorbara and Pamer, 2019; Kogut et al., 2020; Rogers et al., 2020). Infection by pathogens, such as Salmonella, triggers an immune response leading to inflammation within the gut that alters the microbiome composition to favorable conditions (e.g., changes in pH) for pathogen colonization and reproduction, reducing competitors and decreasing the overall community diversity (i.e., α-diversity; Lupp et al., 2007). This dysbiosis allows invading pathogens to persist and may lead to differences in the microbiome between infected and uninfected individuals, as seen in waterfowl infected with avian influenza viruses (Ganz et al., 2017; Hird et al., 2018). While studies on the relationship between Salmonella infections and the microbiome are extensive in vertebrates (Bratburd et al., 2018, reviewed by Rogers et al., 2020), little is known on the effects of the presence of Salmonella itself on the microbiome of wild species regardless of pathogen status (i.e., carriers of Salmonella that may or may not be diseased). With this in mind, we sought to explore the microbiome of barn swallows (Hirundo rustica) with or without the presence of Salmonella. We hypothesized that the microbiome of Salmonella-present (pathogenic or non-pathogenic) barn swallows will have decreased alpha diversity compared to the microbiome of Salmonella-absent birds.
The barn swallow is a widespread ubiquitous passerine species well studied in terms of its life history (Balbontin et al., 2012; Møller, 2014), behavior (Saino et al., 2002; Lifjeld et al., 2011), physiology (de Ayala et al., 2006; Schmidt-Wellenburg et al., 2007; Safran et al., 2008), migration ecology (Altwegg et al., 2011; Liechti et al., 2014; Pancerasa et al., 2018), and host-associated microbiomes (Kreisinger et al., 2015, 2017; Ambrosini et al., 2019; Turjeman et al., 2020), which make the species an ideal exemplar for this study. The breadth of knowledge available on barn swallows allows for the integration of information across fields and more in-depth conclusions from our findings. In particular, the migratory distance of barn swallows could serve as an indicator of the potential for transmission of Salmonella spp. across broad geographic scales, as seen in bar-headed geese (Anser indicus) infected with highly pathogenic avian influenza virus H5N1 (Prosser et al., 2011) and passerine birds with the parasite Babesia venatorum (Hasle et al., 2011). Although Salmonella spillover into humans and domestic animals has not been linked with barn swallows, one study found barn swallows carried strains of the bacterial pathogen, Clostridium difficile, that were also found in humans and farm animals, implicating swallows as a potential source of spillover (Bandelj et al., 2014). Further, barn swallows migrate along the Palearctic-African flyway, the world’s largest bird migration network. Israel, our sampling area, serves as a migratory bottleneck along the flyway linking Eurasia and Africa, where birds are able to avoid crossing large ecological barriers along their migration route (Collins-Kreiner et al., 2013). More specifically, an estimated 500 million birds travel through the Hula Valley region of Israel each season (Gophen, 2015) where dense congregations of birds create opportunities for pathogen transmission and spillover to agricultural operations in the area and beyond.
In this study, we aimed to: (1) explore the prevalence of Salmonella from fecal microbiome data within barn swallows, (2) assess the utility of microbiome data for accurately detecting potentially pathogenic bacteria (Salmonella spp.), by comparing results from traditional culture techniques to those from 16S rRNA gene sequencing, (3) evaluate different host ecological factors predicting Salmonella presence or absence, and (4) analyze the diversity of total (living and relic) microbial communities with and without the presence of Salmonella in barn swallows. Our results highlight the value of 16S rRNA gene sequencing not just for monitoring potential bacterial pathogens, but also for better understanding the ecology and role of microbial communities in pathogen infections.
Materials and Methods
Study Area and Sample Collection
We captured 159 adult birds with mist-nets from four sites (Beit She’an, Haifa, Hula, Shefayim, Figure 1) in the northern half of Israel from November of 2016 to the end of November 2017. All fieldwork was conducted using permit number 2017/41764 issued by the Israel National Protection Authority and approved by Hebrew University ethics committee according to institutional and national guidelines. We placed captured birds in clean plastic cups inside individual bags to collect fecal samples for microbiome characterization. All fecal samples were collected using a sterile swab and stored in 95% EtOH and immediately frozen in a −20°C portable freezer for up to 7 days in the field before transfer to a −80°C freezer for long-term storage until extraction. For all individuals, an additional swab (fecal) was taken and stored in a glycerol-LB mixture for culture detection of Salmonella spp. Where possible, we collected associated data on age, sex, season of capture, and site for all the birds. Sexing was completed according to standard protocols described in Turjeman et al. (2020). We estimated, as described below, Salmonella prevalence for each site using a filtered dataset (n = 108) and visualized the prevalence distribution over our study area by generating a map using QGIS version 2.16.3 with the Apple iPhoto basemap (QGIS Development Team, 2009). Sample sizes, summary statistics on the number of Salmonella reads, and GPS location details for each site are available in Supplementary Table 1.
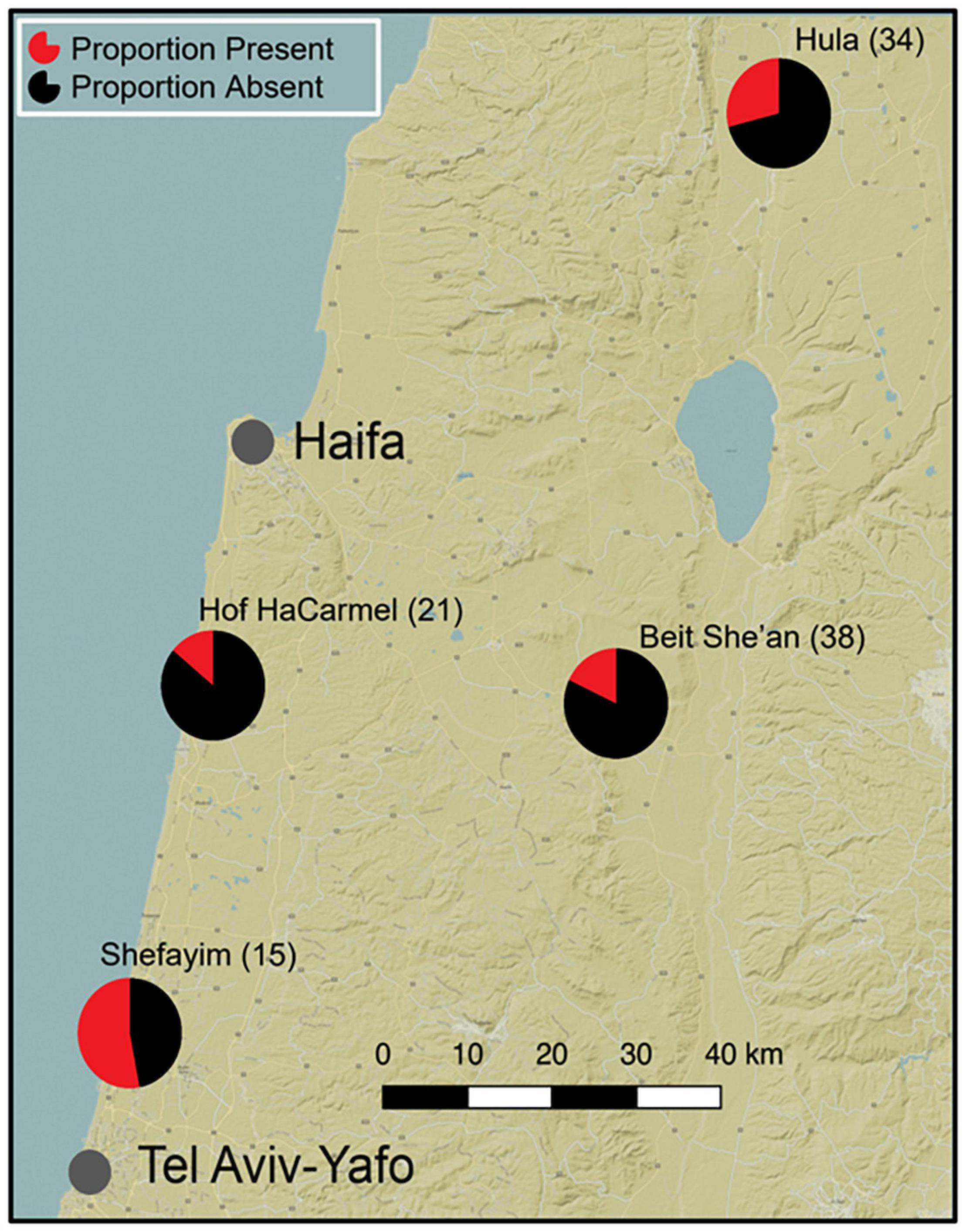
Figure 1. The distribution of Salmonella in barn swallows (Hirundo rustica) across four sampling locations in Israel. The proportion of birds with Salmonella (red) and without Salmonella (black), as determined by 16S rRNA gene sequencing (pre-rarefying), is shown for each site, with samples sizes in parentheses.
Culture Techniques for Salmonella Detection
Fecal swabs were stored in glycerol-LB and cultured to test for Salmonella spp. through one of two culturing workflows. The first workflow (workflow 1), done at the Kimron Veterinary Institute, Israel, used a culture-based approach and was performed on a subset of samples (n = 74) prior to 16S rRNA gene sequencing and did not detect Salmonella in any of the samples. However, sequencing revealed the presence of Salmonella in some samples and therefore we utilized a second culturing workflow (workflow 2) on the remaining samples (n = 34) to determine if Salmonella could be detected using a different culturing protocol. For the workflow 1, swabs were incubated in 10 mL buffered peptone water, followed by 1 mL tetrathionate-brilliant green broth, then XLT4 agar and Enteroplus slant agar. Each step included a 24 h incubation at 37°C. Cultures were considered Salmonella-present if they exhibited a characteristic tri-color appearance of Salmonella (red, black, yellow; ISO 6579-1 2017). Workflow 2 was completed at the Hebrew University of Jerusalem, Israel. Swabs were incubated in 10 mL buffered peptone water, followed by 1 mL Rappaport-Vassiliadis broth, then brilliant green agar, and triple sugar iron agar slants. Each step included an 18–24 h incubation step at 37°C. Colonies suspected of being Salmonella were verified through PCR. DNA was extracted using DNeasy Blood and Tissue kits (Qiagen Inc., Germantown, MD) following the gram-negative bacteria protocol. PCR was conducted following Halatsi et al. (2006) using primer pair SdiA1 (AATATCGCTTCGTACCAC) and SdiA2 (GTAGGTAAACGAGGAGCAG). Reaction volume was 20 μL: 3 μL DNA template, 1 μL of each primer, 10 μL of OneTaq Master Mix, and 5 μL of molecular grade water. Cycling conditions were as follows: 5 min of denaturation at 94°C, then 30 cycles of 94°C for 30 s, 52°C for 40 s, and 72°C for 30 s ending with a final extension at 72°C for 7 min. PCR products were visualized by gel electrophoresis for the determination of the presence/absence of Salmonella.
Microbiome Extraction and Sequencing
A detailed protocol for 16S rRNA gene sequencing and processing of resulting reads is available in Corl et al. (2020) and Turjeman et al. (2020). Briefly, frozen swabs stored in 95% EtOH were extracted using DNeasy PowerLyzer PowerSoil kits (Qiagen Inc., Germantown, MD). Each DNA extraction contained a set of individuals randomized across sites and times of collection to control for any batch effects during extraction. Along with the barn swallow samples, three negative control samples were processed through the DNA extraction workflow in the same manner as for the fecal samples. These negative controls were included to offset the ubiquitous nature of bacteria and to account for possible contamination from laboratory plastics and consumables used throughout the collection and extraction processes. DNA extractions were shipped to Argonne National Laboratory (Lemont, IL) for PCR-amplification and DNA sequencing. Primers 515F and 806R (Caporaso et al., 2012), targeting the variable V4 region of the bacterial 16S rRNA gene were used for Illumina sequencing. Paired-end reads of 151 base pairs (bp) sequences were generated on two runs of an Illumina MiSeq.
Microbiome Quality Control, and Data Filtering
Sequences were demultiplexed using QIIME2 (Bolyen et al., 2019). Analyses of the data were conducted in R 3.6.3 (R Core Team, 2020) following the R workflow for processing 16S data published by Callahan et al. (2016b). The first 10 bases were removed from all reads and DADA2 (Callahan et al., 2016a) was used to identify amplicon sequence variants (ASVs), which were unique sequences that were statistically unlikely to be due to sequencing error. The SILVA 132 taxonomy database was used to assign taxonomy, DECIPHER (Wright, 2015) was used to align sequences and the package phangorn (Schliep, 2010) was used to build a maximum likelihood phylogenetic tree. All parts of the data (sequence variant table, taxonomy table, phylogentic tree) were combined along with the metadata using the phyloseq package (McMurdie and Holmes, 2013) for statistical analyses. Contaminants were removed from the data set using the decontam package (Davis et al., 2018). The prevalence filter was used with a threshold set at 0.5 to remove all sequences that were more prevalent in the three negative controls than in samples from birds. The negative control samples did not have any Salmonella ASVs within them. In total, 518 ASVs were removed as contaminants. In addition, any ASVs not of the kingdom Bacteria were also removed, as well as any sequences matching mitochondria or chloroplasts. Lastly, we removed samples with poor quality PCR (n = 45) and samples without culture results (n = 2). The remaining 108 samples were analyzed to compare the sensitivity of culturing to 16S rRNA gene sequencing in detecting Salmonella. Throughout this manuscript, we use Salmonella presence/absence to indicate Salmonella spp. ASVs present/absent in the microbiome and note that it is unknown whether the Salmonella strains detected are pathogenic.
We examined the bacterial community diversity within and across samples after rarefying the data to a sampling depth of 12,000 reads. A threshold of 12,000 reads was chosen after examining rarefaction curves (Supplementary Figure 1, rngseed = 711) that showed even the most diverse samples leveled off at this threshold and we would only lose seven samples, five of which had fewer than 5,000 reads. Therefore, our analyses of microbial diversity were conducted on 101 samples, after removing these seven samples from the dataset. To test for sampling effects during rarefaction, we ran the rarefaction analyses 100 times using different random seeds (1:100) and then determined the consistency across runs in detecting Salmonella.
Salmonella Prevalence and Comparison of Detection Methods
We estimated the prevalence of Salmonella spp. by detection method for all swallows with paired culture and 16S rRNA gene data (n = 108). Given that we used two different culture workflows, we ran separate sets of analyses for each workflow (nworkflow 1 = 74, nworkflow 2 = 34). A McNemar’s exact test, assuming non-independence of samples that is appropriate for smaller sample sizes (Fagerland et al., 2013), was used to compare detection probabilities of culture and 16S rRNA gene sequencing for both workflow one and two. We used a generalized linear model (GLM) with a quasipoisson distribution from the car package (Fox and Weisberg, 2011) followed by a Type II ANOVA with the smaller dataset of workflow 2 and the combined dataset to test two hypotheses. First, we tested whether culture success or failure could predict the absolute abundance of Salmonella as measured by the number of 16S rRNA sequencing reads, including the total number of reads per sample as a covariate to account for sequencing depth. We used absolute abundance for this model to maximize detection power while acknowledging that samples did not have equal sequencing effort (unrarefied). Second, to address how sequencing depth affects our detection probability, we tested whether Salmonella-present vs. -absent status in the host, as measured by the 16S data, was correlated with the total number of reads sequenced for the sample. We do not report the results for rarefied data as the results did not differ for either the culture success and absolute abundance or Salmonella presence and total reads tests. To determine the sensitivity of Salmonella detection to 16S sequencing depth, we used all samples with at least a single read for Salmonella and greater than 45,000 reads (n = 18). We chose 45,000 as a cutoff because at higher depths many samples were lost during rarefaction as they did not meet the required number of reads. We rarefied the data for these 18 Salmonella-positive samples between depths of 5,000–45,000, in increments of 5,000 reads, using the default seed (711). For each depth, we calculated the proportion of samples with at least a single read of Salmonella. Salmonella detection, prevalence estimation, and statistical analyses were conducted in R 3.6.3 (R Core Team, 2020). Figures displaying prevalence between detection methods were generated using the ggplot2 (Wickham, 2016) and phyloseq (McMurdie and Holmes, 2013) packages in R.
Relationships Between Host Ecology and Salmonella Status
We explored whether Salmonella presence in the gut microbiome was correlated with host characteristics to highlight how 16S data can enhance our understanding of host ecology in relation to the presence of potential pathogens. We tested whether the presence or absence of Salmonella was dependent on three host factors: migratory status, weight, and sex. A generalized linear model with a binomial distribution was run for each factor, with the total number of reads for every sample included as a covariate to control for sequencing depth, which likely influences the detection of Salmonella in the hosts. For this analysis and all subsequent analyses, we initially assigned Salmonella-present samples as samples that had one or more Salmonella reads. We also repeated each analysis with a higher threshold of two or more Salmonella reads to assign a Salmonella-present sample. We did this to account for possible false positives due to PCR or sequencing error. When using a two read threshold, we removed seven samples that had only a single Salmonella read from the analyses. We were not able to explore thresholds greater than two reads due to the need to maintain a sufficient sample size of Salmonella-present samples. Data was available for 92 samples to test for the effects of weight and sex at read threshold one, while 88 samples were used for read threshold two. Data for migratory status was available for 37 (one read) and 33 (two reads) samples that were classified as migrant or resident using both feather molt pattern evaluated during field capture and stable isotope analyses (see Turjeman et al., 2020). For each model, we used an ANOVA to identify significant relationships.
Relationships Between the Microbiome and Salmonella Status
We estimated the diversity and bacterial species composition in all samples post rarefaction (rngseed = default of 711, n = 101) to examine host microbiome relationships with the presence of Salmonella. We used the Chao1 estimator of the number of species (Chao, 1984) to measure alpha diversity and used a Mann-Whitney-Wilcoxon test (MWW) to test for differences in alpha diversity of the microbiota between birds with and without Salmonella sequence reads. We chose to use Chao1 as an estimator for alpha diversity, because it accounts for rare and missing species (Chao and Shen, 2003). To test for confounding factors that may also influence alpha diversity, we used a linear model and ANOVA, including sex, site, and season as additional factors. Migration status was not included because our previous work showed no differences in alpha diversity between migrants and residents in our study population (Turjeman et al., 2020).
Differences in bacterial communities between Salmonella-present and -absent birds were visualized using bar plots and principal coordinates analysis (PCoA) with the phyloseq package. PCoA chooses axes that explain most of the variation in the entire dataset without reference to the particular factors that may distinguish two groups of samples. Permutational multivariate analysis of variance (PERMANOVA) tests were calculated with adonis from the vegan package (Oksanen et al., 2019) with 9,999 permutations. This was done on both weighted and unweighted UniFrac metrics. UniFrac is a distance metric comparing bacterial communities that uses phylogenetic information to measure distance between samples (Lozupone and Knight, 2005). The unweighted UniFrac uses only presence/absence data, whereas weighted UniFrac also incorporates relative abundance of ASVs to measure distance between samples (Lozupone et al., 2007). To account for potentially significant differences based on location or dispersion, we tested for homogeneity of group dispersions using the betadisper function in the vegan package. When dispersions are significantly different, then significant differences in communities as found by PERMANOVA can be the result of differences in either dispersion or both dispersion and location.
Results
16S rRNA Gene Sequencing Detected Higher Salmonella Prevalence Than Culture-Based Methods
Culture results revealed a relatively low prevalence of Salmonella, with zero out of 74 birds positive for Salmonella presence in workflow 1 (0%, Figure 2A) and six out of 34 birds positive in workflow 2 (17.6%; Figure 2B). In comparison, results based on unrarefied 16S rRNA gene data identified Salmonella in ten swallows (13.5%, Figure 2A) for workflow 1 and 15 swallows (44.1%, Figure 2B) in workflow 2. The McNemar’s exact test revealed a significant difference in Salmonella detection probability between culturing vs. 16S rRNA gene sequencing for both culture workflow 1 (Figure 2A, p = 0.002) and workflow 2 (Figure 2B, p = 0.035).
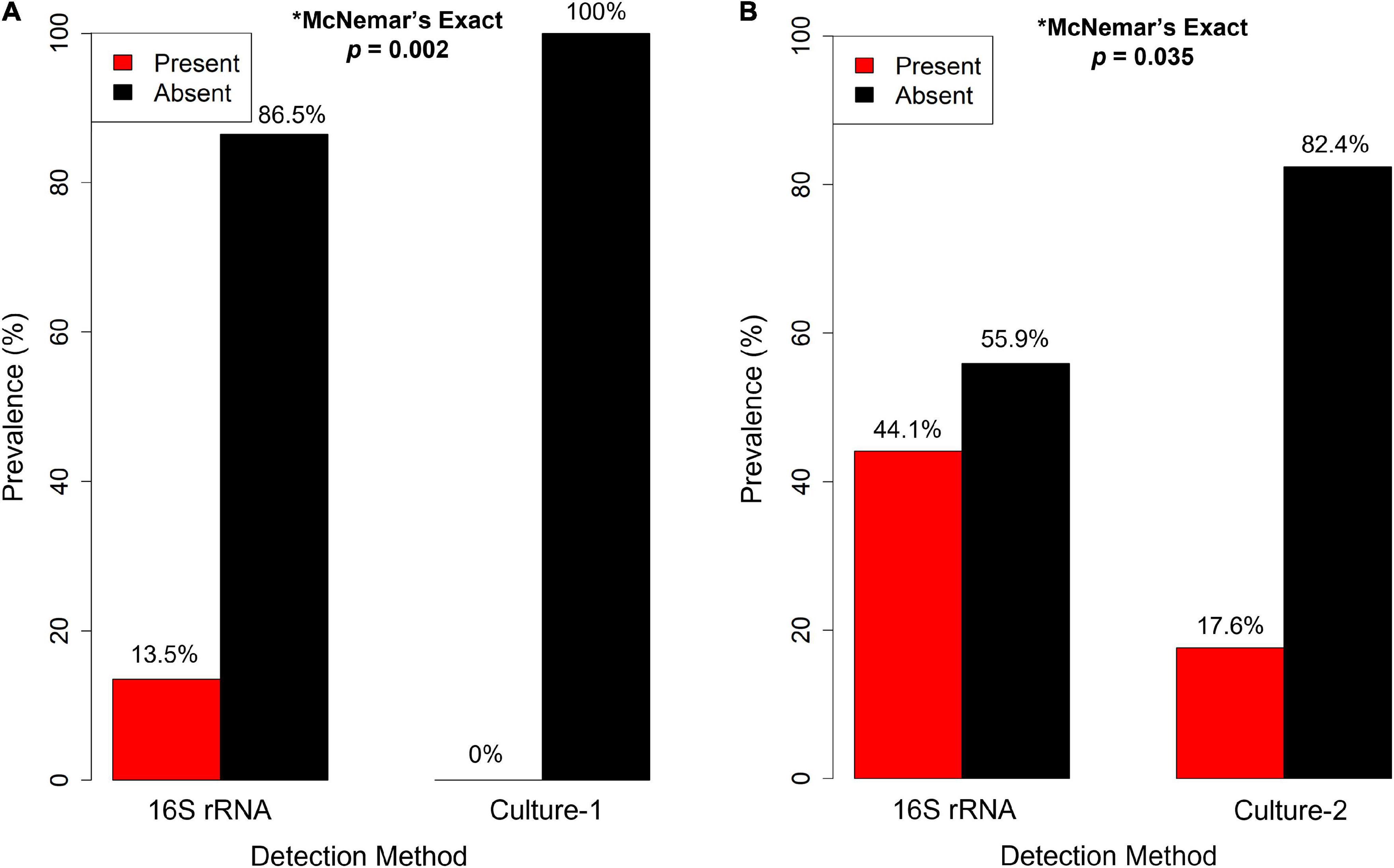
Figure 2. Salmonella spp. prevalence in barn swallows (Hirundo rustica) from Israel, estimated using unrarefied 16S rRNA gene sequencing (16S rRNA) and two culture methods: (A) culture workflow 1 (Culture-1; n = 74) and (B) culture workflow 2 (Culture-2; n = 34). The proportion of samples positive for the presence of Salmonella (red bars) detected by 16S rRNA gene sequencing was significantly higher than those obtained by culturing. This analysis was conducted on paired samples from the same individuals that had both culture and 16S rRNA gene data collected.
A GLM showed no significant relationship between Salmonella culture results and the absolute abundance of Salmonella as measured by unrarefied reads of 16S rRNA data for workflow 2 (p = 0.58) and both workflows combined (p = 0.96). We did not use workflow 1 for this analysis as there were no culture positive samples for comparison. Samples that were identified as having at least one Salmonella sequence read in the 16S rRNA data had significantly higher sequencing depths than samples where no Salmonella sequences were detected (Supplementary Figure 2, p = 0.002). The mean absolute abundance of Salmonella, as measured by unrarefied 16S rRNA in culture-positive samples for workflow 2 (mean = 3.67, std dev = 7.12) and both workflows combined (same as workflow 2 as culture-positive samples did not change), did not significantly differ from mean abundance in culture-negative samples for workflow 2 (mean = 10.71, std dev = 38.87) (ANOVA, F = 0.19, p = 0.66) and the combined workflows (mean = 3.44, std dev = 20.83) (ANOVA, F = 0.00069, p = 0.98), respectively. Salmonella abundance was low, ranging from 1–199 reads (<0.007%, mean = 14.92) for samples positive for its presence based on 16S rRNA sequencing (Supplementary Figure 3A). Seven out of the 25 samples with Salmonella present in the microbiome had only a single Salmonella read.
We explored how Salmonella was affected by rarefaction, given that Salmonella presence status was related to sequencing depth. After rarefying to 12,000 reads, we detected Salmonella in an average of 12.95 samples (down from 25 samples pre-rarefying), with variability across runs (9–17 samples positive per run) due to random sampling (Supplementary Figure 3). The median value was 13 positive samples with a 95% confidence interval range of 12.6–13.3 samples after 100 iterations, so rarefaction reduced the number of Salmonella-present samples by about half. Samples with higher numbers of Salmonella reads before rarefying consistently had higher numbers of reads after rarefying. Post-rarefaction, one to three cultured samples were confirmed for Salmonella presence with 16S rRNA data, whereas three of the six culture positive samples were confirmed prior to rarefaction. In terms of sequencing depth and Salmonella detection, a high depth (>32,000) was required to obtain 80% detection within this system (Supplementary Figure 4) suggesting that a very high sequencing depth is required to consistently detect Salmonella in our data.
Salmonella Presence Differed Between Birds by Migratory Status and Weight
Using a Salmonella detection threshold of one read, we found a marginally significant difference in Salmonella presence (p = 0.056) between male and female birds. For host weight, Salmonella-present birds were slightly heavier (mean = 19.1 grams) in weight than those without Salmonella (mean = 18.1 grams) (p = 0.012). For migratory status, migrant swallows were significantly more likely to have Salmonella in their microbiome than resident swallows (p = 0.041), with a Salmonella prevalence of 52% in migrants and 16.7% in resident swallows.
When applying a Salmonella detection threshold of two reads, there was similarly a significant difference in Salmonella presence (p = 0.015) between male and female birds. Salmonella was present in seventeen of 37 (31.5%) male birds, in comparison to four of 39 (9.3%) females. For both weight and migratory status, there was no significant difference between Salmonella-present and -absent birds (p = 0.081 and 0.099, respectively).
Alpha Diversity Differed Among Birds Based on Salmonella Presence and Absence
When examining relationships between Salmonella and microbial communities, our set of positive samples were all samples with Salmonella sequence reads identified by 16S rRNA sequencing (pre-rarefaction; n = 25), plus the samples that were only determined to be positive by culture (n = 3). One sample with Salmonella identified pre-rarefaction did not meet the required threshold inclusion and was removed during rarefaction; this left 27 Salmonella-present samples for the remainder of the analyses, when applying a detection threshold of one read. For a detection threshold of two reads, six additional samples were removed, leaving 21 Salmonella-present samples in the remaining analyses. At Salmonella read threshold one, individuals with Salmonella (n = 27) had higher levels of alpha diversity than individuals without Salmonella (Figure 3, MWW, p = 0.001). Both Salmonella presence/absence (ANOVA, p = 0.047, F = 4.044, df = 1) and site (ANOVA, p = 0.048, F = 2.739, df = 3) were significant predictors of microbial community alpha diversity (Model R2 = 0.18). Sex (ANOVA, p = 0.509, F = 0.44, df = 1), age (ANOVA, p = 0.55, F = 0.553, df = 1) and season (ANOVA, p = 0.92, F = 0.084, df = 2) were not significantly correlated with alpha diversity. Salmonella read threshold two yielded similar results: individuals with Salmonella had higher levels of alpha diversity than individuals without Salmonella (Supplementary Figure 5, MWW, p = 0.003), and both Salmonella presence/absence (ANOVA, p = 0.028, F = 4.970, df = 1) and site (ANOVA, p = 0.042, F = 2.852, df = 3) were significant predictors of microbial community alpha diversity (Model R2 = 0.112). In addition, sex (ANOVA, p = 0.225, F = 1.49, df = 1), age (ANOVA, p = 0.60, F = 0.280, df = 1), and season (ANOVA, p = 0.93, F = 0.074, df = 2) were not significantly correlated with alpha diversity.
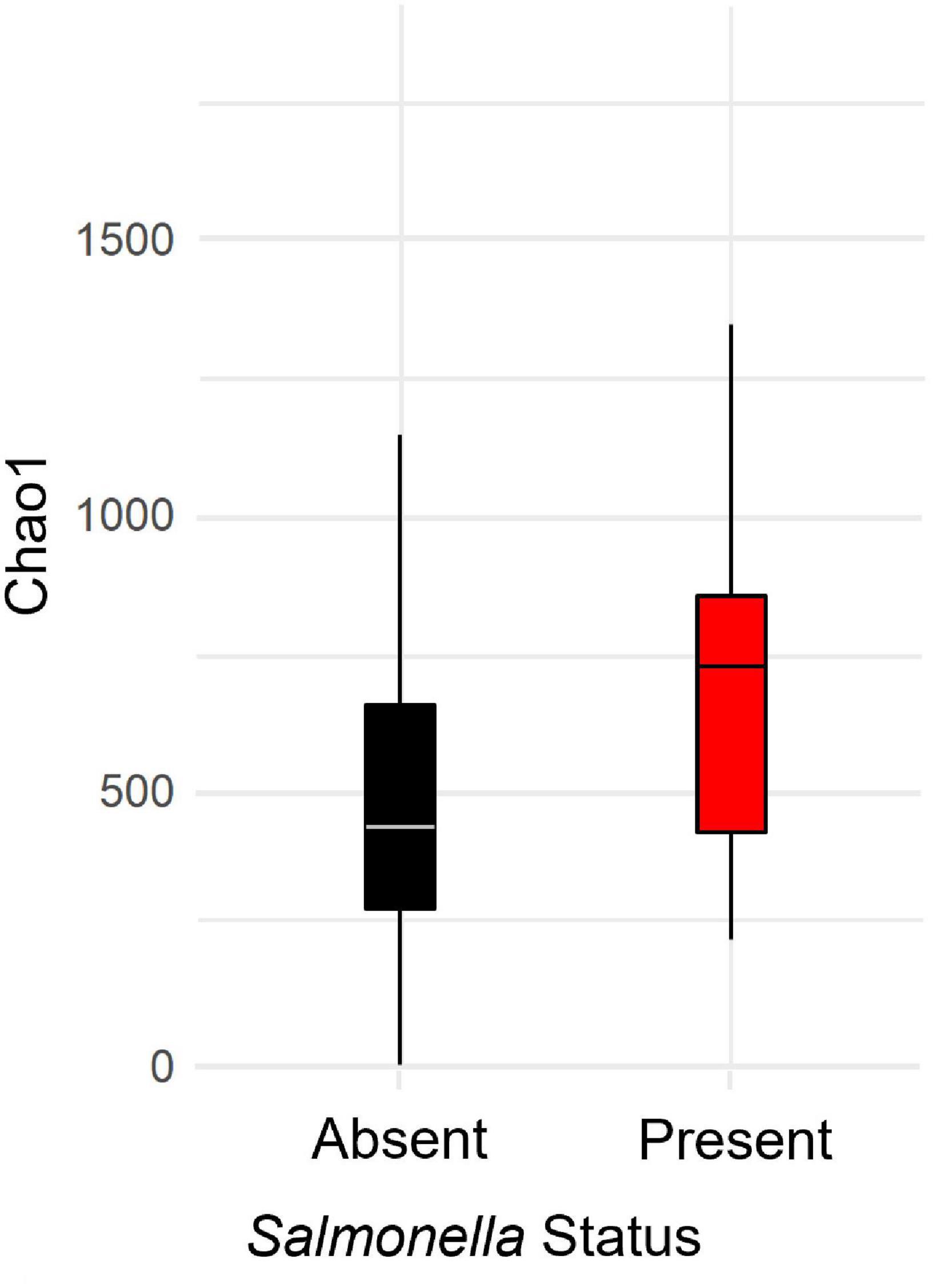
Figure 3. Bacterial alpha diversity, as estimated by the Chao1 statistic, by Salmonella spp. status (absent, present) in barn swallow (Hirundo rustica) fecal samples collected in Israel. Salmonella-absent samples had significantly lower diversity than -present samples (Mann-Whitney-Wilcoxon test, p = 0.008).
Across all samples, 16S rRNA data revealed that bacterial communities were comprised mainly of the phyla Proteobacteria, Tenericutes, Firmicutes, and Actinobacteria (Supplementary Figure 6). The microbial communities of Salmonella-present and -absent birds were significantly different in PERMANOVA tests using both unweighted (Figure 4A, p = 0.001, F = 2.637, df = 1, R2 = 0.260) and weighted (Figure 4B, p = 0.013, F = 2.821, df = 1, R2 = 0.028) UniFrac distances at read threshold one. Homogeneity of dispersion between the two groups was rejected for both unweighted and weighted UniFrac distances (unweighted: F = 24.786, df = 1, p = 0.001; weighted: F = 12.506, df = 1, p = 0.001). Thus, significant differences in the bacterial communities of Salmonella-present and -absent birds are driven at least in part by different dispersion of the two groups. However, the principal coordinates analysis plot for the unweighted UniFrac (Figure 4A) suggests that there may also be differences in the location of points within the plot for the two groups, because many birds with Salmonella reads have bacterial communities that occupy a space that is distinct from birds without Salmonella reads. Read threshold two yielded similar results and thus are not reported here (Supplementary Figure 7).
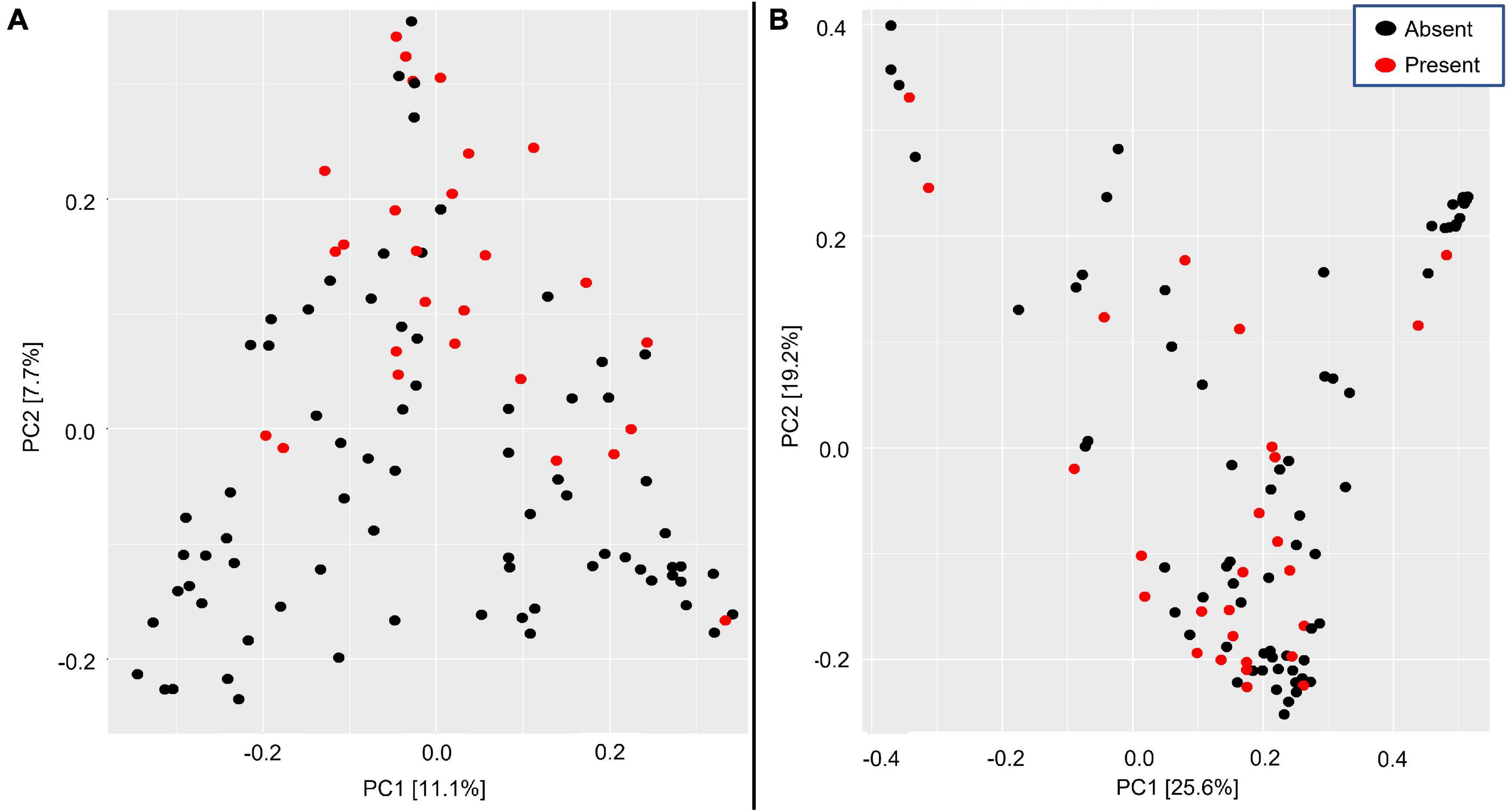
Figure 4. Principal coordinate analysis plots showing the bacterial communities in barn swallow (Hirundo rustica) fecal samples with Salmonella (present: red dots) and without (absent: black dots) by (A) unweighted UniFrac and (B) weighted UniFrac metrics. The amount of the variation explained by each axis is in brackets. Bacterial beta diversity significantly differed for both metrics (p = 0.001 for both) between Salmonella-present and -absent birds.
Discussion
Salmonella is a bacterial genus that includes several pathogenic serotypes of concern to both public health and agriculture. As wild bird species can act as reservoirs or carriers for these pathogenic bacteria, continued Salmonella surveillance is critical for preventing spillovers into humans and domestic animals (Giovannini et al., 2012). In barn swallows, Salmonella was not previously detected when using a culture diagnostic approach for screening (Haemig et al., 2008). However, our data suggest that Salmonella can be missed by culturing (e.g., culture workflow 1), and therefore microbiome data could enhance efforts to monitor Salmonella and other bacterial pathogens in wild birds for the purposes of both human and avian health. Further, our results demonstrate an approach that can be used not only for monitoring potential pathogens, but also for addressing key questions in disease ecology, such as the relationship between individual host factors (e.g., age, sex, and microbial community diversity) and pathogen infection.
We found that detection of Salmonella was significantly better with 16S rRNA gene sequencing data than using culture techniques (Figure 2). Our finding that 16S rRNA sequence data detected Salmonella in more birds than either respective culture workflow suggests that sequencing approaches could be an important tool for the detection and surveillance of bacterial pathogens like Salmonella in wildlife populations. Higher sensitivity of microbe detection using 16S rRNA gene sequencing data could be due to the detection of both residual or dead cells. For the purposes of this study, dead cell DNA is still of interest in terms of the potential for carrier status, because these cells could represent bacteria that are alive in the gastrointestinal tract, but unable to survive the semi-aerobic conditions of the cloaca (Grond et al., 2018), where fecal material passes prior to sample collection. However, if detection of only live potential pathogens is desired, a pre-enrichment step using ethidium monoazide (Nogva et al., 2003) or propidium monoazide (Nocker et al., 2006) can be included to remove non-viable DNA.
The detection of Salmonella or other bacteria in 16S rRNA sequencing data will depend on the sequencing depth and the abundance of the bacteria in the sample. We found that Salmonella presence in barn swallows was related to sequencing depth (Supplementary Figure 2), that rarefaction to 12,000 reads resulted in Salmonella detection in only half the total samples observed when using the full data set, and that a high sequencing depth was required for reliable detection of Salmonella spp. (Supplementary Figures 4, 5). This high sequencing depth requirement may explain the discrepancy in Salmonella detection for the three false negative samples that were culture positive, but 16S negative. Further, these data highlight two vital factors to keep in mind when using 16S rRNA gene data for disease detection. First, sequencing depth will determine the power to detect taxa, especially those in low abundance, as we observed here when increasing the Salmonella detection threshold. The minimum sequencing depth would change for other host species, which may not have a similarly low level of Salmonella abundance, especially if they are in a diseased state. Our results are consistent with these swallows potentially being infected or carriers of Salmonella, rather than experiencing disease, due to extremely low levels of abundance. Thus, we recommend high sequencing depths unless it is known a priori that the pathogen is abundant. A cost-effective approach would be to first sequence a subset of samples at high sequencing depth to determine the optimal level of sequencing for the remaining samples. Second, we recommend that all reads be used for detection of rare pathogens, because this approach maximizes the power for detection within a dataset. If this approach is taken, it could be useful to withhold some DNA before 16S library preparation that could be used for further PCR or sequencing of the target pathogen to confirm positive samples that have very few reads from the bacteria of interest. A previous study showed that with a proper enrichment step, PCR detected Salmonella from as little as one colony forming unit (CFU) in food (Ferretti et al., 2001), suggesting that even in low abundance, Salmonella can be detected. PCR and Sanger sequencing of 16S positive samples could also be useful for determining whether the bacterial strain is pathogenic, which often requires longer reads than are typical with 16S rRNA amplicon sequencing.
Our data showed that the presence of Salmonella was correlated with multiple differences in host ecology and microbiota. When using a detection threshold of one read, birds with Salmonella weighed more than birds without Salmonella, and Salmonella was present in more migrants than resident barn swallows. These two factors were related to one another, because migrant birds were heavier than resident barn swallows (mean 20.2 vs. 17.5 grams). Barn swallows have been known to accumulate fat in preparation for migration (Pilastro and Spina, 1999), which suggests that migrants might have differing foraging behaviors that could lead to a higher prevalence of Salmonella. Another potential reason why migrants have a higher prevalence of Salmonella is that they travel through multiple environments, which could increase their encounters with Salmonella species. This sort of pattern has been observed in barn owls (Tyto alba), where owls that traveled greater distances from their nests had more diverse microbiota (Corl et al., 2020). However, migration does not necessarily lead to changes in the microbiota as microbial diversity did not vary between fecal samples collected from common cranes (Grus grus) before and after migration (Pekarsky et al., 2021). Dietary, physiological, or subspecies differences might also explain differences in Salmonella prevalence between migrants and resident barn swallows. A caveat to the models correlating Salmonella presence with host ecological variables is they were sensitive to the read threshold and/or sample size. With read threshold two, the relationships with both migratory status and weight were not significant (migratory status, p = 0.099, weight p = 0.081), whereas a read threshold of one revealed a significant relationship (p < 0.05) between these factors and Salmonella status. In contrast, the relationship with sex changed from marginally significant (p = 0.056) to significant (p = 0.012) when increasing the Salmonella detection threshold to two reads. Thus, similar trends were found for all three traits, but the statistical significance of the correlations is tentative. However, these results do highlight a set of traits to target for future study to elucidate the relationship between host ecology, microbiota, and Salmonella status. In addition, these results underscore the need for high sequencing depths and sufficient numbers of positive samples when investigating relationships between Salmonella status and host ecology.
We found that birds with Salmonella had more diverse bacterial communities, suggesting that Salmonella may alter community level interactions among bacterial taxa in the gut microbiome or that Salmonella-presence is correlated with other factors that alter bacterial communities. Increased diversity in Salmonella-present birds may be related to the vital role the gut microbiome plays in immune response and health. Hosts and their microbiota can work together to promote colonization resistance (Sorbara and Pamer, 2019; Rogers et al., 2020), while infection by Salmonella spp. can cause dysbiosis in favor of Salmonella growth (Lupp et al., 2007) and a decrease in diversity that is not seen here. For example, microbial diversity was reduced in American white ibis (Eudocimus albus) shedding Salmonella enterica, relative to the diversity observed in healthy ibis (Murray et al., 2020). Similarly, microbial diversity was reduced in mallard ducks infected with avian influenza viruses (Ganz et al., 2017). However, pathogen relationships with microbial diversity are species-specific, as seen in waterfowl where influenza A virus infection was negatively correlated with alpha diversity in only two of five species (Hird et al., 2018). However, our results contrast with these previous studies and the apparent increased microbial diversity in individuals with Salmonella may be due to avirulent or low abundance strains failing to trigger colitis, and thus being outcompeted by other bacterial species (Stecher et al., 2007). Another explanation is that hosts with Salmonella could have more varied diets, resulting in an increased chance of being colonized by diverse bacteria, including Salmonella. In addition, hosts may differ in their movement patterns (e.g., migrant vs. resident), which could affect their exposure and colonization by a particular bacterium: the use of more diverse habitats (long-distance migrants) may lead to more exposures and colonization by diverse bacteria as supported by our finding that migrants were more likely to have Salmonella.
We found that bacterial communities differed in individuals with and without Salmonella, but we were unable to disentangle whether the significance of these tests is driven in part by community-level differences or by significant differences in dispersion between Salmonella-present and -absent groups. With that caveat in mind, the PcoA of unweighted UniFrac suggests that there are both differences in location (i.e., position of the points) and dispersion (i.e., the spread of the points) of the two bacterial communities. Therefore, dispersion might not be the only factor separating bacterial communities with and without Salmonella, and Salmonella-present hosts may vary in their bacterial communities.
Our work demonstrates the potential for 16S rRNA gene sequencing data collected for microbiome studies to also be used for monitoring Salmonella and other pathogens in wild bird populations. The observed variation in microbial communities and host traits by Salmonella status also suggests that studies on pathogen transmission and host microbial ecology can mutually inform one another. Pathogens should be considered as one of the many plausible causal explanations for the differences in the host microbiota. The few studies focused on pathogen-microbiome interactions in wildlife highlight a need for further research to elucidate the relationships between microbiota, pathogen infections, and disease. We propose that future microbiome studies of wild animals have great potential to be used to better understand disease epidemiology and ecology in wild populations, as well as aid in the identification of potential reservoir species for pathogenic bacteria. Microbiome studies could thus be a rich, but untapped source of data for better understanding the distribution and ecological dynamics of pathogens in the environment.
Data Availability Statement
Part of the sequence data generated for this study were previously deposited in the Sequence Read Archive (BioProject ID: PRJNA578383, accession nos. SRX9094853 and SRX9094908) along with associated metadata (Turjeman et al., 2020) and the remainder of the sequence in the same Sequence Read Archive BioProject (PRJNA578383) along with associated metadata (BioSamples SAMN21551599 and SAMN21551658, accession numbers SRX12293439 and SRX12293498). Both datasets can be found at https://www.ncbi.nlm.nih.gov/sra/PRJNA578383.
Ethics Statement
The animal study was reviewed and approved by the Israel National Protection Authority (#2017/41764) and approved by an ethics committee according to institutional and national guidelines.
Author Contributions
PK, RB, RN, and WG obtained funding and provided resources and facilities that were used in this study. AC, OC, RB, and PK designed the study. AW collected samples. ST developed sampling protocols, helped coordinate field and laboratory work, and prepared sampling material and media. AC, AL, OC, and ST completed laboratory work (AC: microbiome extractions, AL and OC: culture workflows, ST: determining the sex of the samples). AC completed bioinformatics processing. AC and SI contributed code for analyses. OC completed statistical analyses and wrote the first draft of the manuscript with help from AC, SI, and PK. All authors read and revised the manuscript.
Funding
This research was funded by National Science Foundation grant #1617982 to WG, RB, and PK, United States-Israel Binational Science Foundation grant: 2015904 to RN and WG, and USDA-National Institute of Food and Agriculture Hatch Project: ME021908 through the Maine Agricultural and Forest Experimentation Station to PK.
Conflict of Interest
The authors declare that the research was conducted in the absence of any commercial or financial relationships that could be construed as a potential conflict of interest.
Publisher’s Note
All claims expressed in this article are solely those of the authors and do not necessarily represent those of their affiliated organizations, or those of the publisher, the editors and the reviewers. Any product that may be evaluated in this article, or claim that may be made by its manufacturer, is not guaranteed or endorsed by the publisher.
Acknowledgments
We would like to thank members of the Movement Ecology Lab at the Hebrew University of Jerusalem for assistance with sample collection, especially Yoav Bartan for his help in the field as well as the lab. All fieldwork was conducted using permit number 2017/41764 by the Israel National Protection Authority and approved by an ethics committee according to institutional and national guidelines. We thank Candace Wang, Hannah Newcombe, and Lydia Smith for assistance with laboratory work, and the Wildlife Disease Genetics Laboratory at the University of Maine for feedback on the manuscript. This is Maine Agricultural and Forest Experiment Station Publication no. 3853.
Supplementary Material
The Supplementary Material for this article can be found online at: https://www.frontiersin.org/articles/10.3389/fevo.2021.683183/full#supplementary-material
References
Altwegg, R., Broms, K., Erni, B., Barnard, P., Midgley, G. F., and Underhill, L. G. (2011). Novel methods reveal shifts in migration phenology of barn swallows in South Africa. Proc. R. Soc. B 279, 1485–1490. doi: 10.1098/rspb.2011.1897
Ambrosini, R., Corti, M., Franzetti, A., Caprioli, M., Rubolini, D., Motta, V. M., et al. (2019). Cloacal microbiomes and ecology of individual barn swallows. FEMS Microbiol. Ecol. 95:fiz061.
Aung, K. T., Khor, W. C., Octavia, S., Ye, A., Leo, J., Chan, P. P., et al. (2020). Distribution of Salmonella serovars in humans, food, farm animals and environment, companion and wildlife animals in Singapore. Int. J. Res. Public Health 17:5774. doi: 10.3390/ijerph17165774
Balbontin, J., Møller, A. P., Hermosell, I. G., Marzal, A., Reviriego, M., and de Lope, F. (2012). Geographical variation in reproductive ageing patterns and life-history strategy of a short-lived passerine bird. J. Evolution. Biol. 25, 2298–2309. doi: 10.1111/j.1420-9101.2012.02606.x
Bandelj, P., Trilar, T., Blagus, R., Ocepek, M., Rousseau, J., Weese, J. S., et al. (2014). Prevalence and molecular characterization of Clostridium difficile isolated from European barn swallows (Hirundo rustica) during migration. BMC Vet. Res. 14:40. doi: 10.1186/1746-6148-10-40
Banskar, S., Bhute, S. S., Suryavanshi, M. V., Punekar, S., and Shouche, Y. S. (2016). Microbiome analysis reveals the abundance of bacterial pathogens in Rousettus leschenaultii guano. Sci. Rep. 6:36948. doi: 10.1038/srep36948
Berg, G., Rybakova, D., Rischer, D., Cernava, T., Vergés, M. C. C., Charles, T., et al. (2020). Microbiome definition re-visited: old concepts and new challenges. Microbiome 8:103. doi: 10.1186/s40168-020-00875-0
Bolyen, E., Rideout, J. R., Dillon, M. R., Bokulich, N. A., Abnet, C. C., Al-Ghalith, G. A., et al. (2019). Reproducible, interactive, scalable and extensible microbiome data science using QIIME 2. Nat. Biotech. 37, 852–857. doi: 10.1038/s41587-019-0209-9
Bratburd, J. R., Keller, C., Vivas, E., Gemperline, E., Li, L., Rey, F. E., et al. (2018). Gut microbial and metabolic response to Salmonella enterica serovar Typhimurium and Candida albicans. mBio 9, e2032–e2118. doi: 10.1128/mBio.02032-18
Callahan, B. J., Sankaran, K., Fukuyama, J. A., McMurdie, P. J., and Holmes, S. P. (2016b). Bioconductor workflow for microbiome data analysis: from raw reads to community analyses. F1000Res.5:1492. doi: 10.12688/f1000research.8986.2
Callahan, B. J., McMurdie, P. J., Rosen, M. J., Han, A. W., Johnson, A. J., and Holmes, S. P. (2016a). DADA2: high-resolution sample inference from Illumina amplicon data. Nat. Methods 13, 581–583. doi: 10.1038/nmeth.3869
Caporaso, J. G., Lauber, C. L., Walters, W. A., Berg-Lyons, D., Huntley, J., Fierer, N., et al. (2012). Ultra-high-throughput microbial community analysis on the Illumina HiSeq and MiSeq platforms. ISME J. 6, 1621–1624. doi: 10.1038/ismej.2012.8
Chao, A. (1984). Nonparametric estimation of the number of classes in a population. Scand. J. Stat. 11, 265–270.
Chao, A., and Shen, T.-J. (2003). Nonparametric estimation of Shannon’s index of diversity when there are unseen species in sample. Environ. Ecol. Stat. 10, 429–443.
Collins-Kreiner, N., Malkinson, D., Labinger, Z., and Shtainvarz, R. (2013). Are birders good forbirds? Bird conservation through tourism management in the Hula Valley, Israel. Tourism Manage. 38, 31–42. doi: 10.1016/j.tourman.2013.01.009
Corl, A., Charter, M., Rozman, G., Toledo, S., Turjeman, S., Kamath, P. L., et al. (2020). Movement ecology and sex are linked to barn owl microbial community composition. Mol. Ecol. 29, 1358–1371. doi: 10.1111/mec.15398
Davies, S., Zadik, P. M., Mason, C. M., and Whittaker, S. J. (2000). Methicillin-resistant Staphyloccocus aureus: evaluation of five selective media. Br. J. Biomed. Sci. 57, 269–272.
Davis, N. M., Proctor, D. M., Holmes, S. P., Relman, D. A., and Callahan, B. J. (2018). Simple statistical identification and removal of contaminant sequences in marker-gene and metagenomics data. Microbiome 6:226. doi: 10.1186/s40168-018-0605-2
de Ayala, R. M., Martinelli, R., and Saino, N. (2006). Vitamin E supplementation enhances growth and condition of nestling barn swallows (Hirundo rustica). Behav. Ecol. Sociobiol. 60, 619–630. doi: 10.1007/s00265-006-0206-0
Fagerland, M. W., Lydersen, S., and Laake, P. (2013). The McNemar test for binary matched-pairs data: mid-p and asymptotic are better than exact conditional. BMC Med. Res. Methodol. 13:91. doi: 10.1186/1471-2288-13-91
Felske, A., Akkermans, A. D., and De Vos, W. M. (1998). Quantification of 16S rRNAs in complex bacterial communities by multiple competitive reverse transcription-PCR in temperature gradient gel electrophoresis fingerprints. Appl. Environ. Microbiol. 64, 4581–4587. doi: 10.1128/AEM.64.11.4581-4587.1998
Ferretti, R., Mannazzu, I., Cocolin, L., Comi, G., and Clementi, F. (2001). Twelve-hour PCR-based method for detection of Salmonella spp. in food. Appl. Environ. Microbiol. 67, 977–978. doi: 10.1128/AEM.67.2.977-978.2001
Foti, M., Daidone, A., Aleo, A., Pizzimenti, A., Giacopello, C., and Mammina, C. (2009). Salmonella bongori 48:z35:- in migratory birds, Italy. Emerg. Infect. Dis. 15, 502–503. doi: 10.3201/eid1503.080039
Ganz, H. H., Doroud, L., Firl, A. J., Hird, S. M., Eisen, J. A., and Boyce, W. M. (2017). Community-level differences in the microbiome of healthy wild mallards and those infected by influenza A viruses. mSystems 2:188. doi: 10.1128/mSystems.00188-16
Gargiulo, A., Fioretti, A., Russo, T. P., Varriale, L., Rampa, L., Paone, S., et al. (2018). Occurrence of enteropathogenic bacteria in birds of prey in Italy. Lett. Appl. Microbiol. 66, 202–206. doi: 10.1111/lam.12836
Giovannini, S., Pewsner, M., Hüssy, D., Hächler, H., Ryser Degiorgis, M.-P., von Hirschheydt, J., et al. (2012). Epidemic of Salmonellosis in passerine birds in Switzerland with spillover to domestic cats. Vet. Path. 50, 597–606. doi: 10.1177/0300985812465328
Gophen, M. (2015). Management improvement of the Agmon wetlands system (Hula Valley. Israel) aimed at the enhancement of bird populations and Kinneret protection. Open J. Modern Hydrol. 5, 1–9. doi: 10.4236/ojmh.2015.51001
Grond, K., Sandercock, B. K., Jumpponen, A., and Zeglin, L. H. (2018). The avian gut microbiota: community physiology and function in wild birds. J. Avian Biol. 49:e01788. doi: 10.1111/jav.01788
Gupta, S., Mortensen, M. S., Schjorring, S., Trivedi, U., Vestergaard, G., Stokholm, J., et al. (2019). Amplicon sequencing provides more accurate microbiome information in healthy children compared to culturing. Commun. Biol. 2:291. doi: 10.1038/s42003-019-0540-1
Haemig, P. D., Hernandez, K., Waldenström, J., Bonnedahl, J., and Olsen, B. (2008). Barn swallows (Hirundo rustica) test negative for Salmonella. Vector Borne Zoonotic Dis. 8, 451–453. doi: 10.1089/vbz.2007.0233
Halatsi, K., Oikonomou, I., Lambiri, M., Mandilara, G., Vatopoulos, A., and Kyriacou, A. (2006). PCR detection of Salmonella spp. using primers targeting the quorum sensing gene sdiA. FEMS Microbiol. Lett. 259, 201–207. doi: 10.1111/j.1574-6968.2006.00266.x
Hasle, G., Leinaas, H. P., Røed, K. H., and Øines, Ø (2011). Transport of Babesia venatorum-infected Ixodes ricinus to Norway by northward migrating passerine birds. Acta Vet. Scand. 53:41.
Hird, S. M., Ganz, H., Eisen, J. A., and Boyce, W. M. (2018). The cloacal microbiome of five wildduck species varies by species and influenza A virus infection status. mSphere 3, e382–e418. doi: 10.1128/mSphere.00382-18
Hird, S. M., Sánchez, C., Carstens, B. C., and Brumfield, R. T. (2015). Comparative gut microbiota of 59 neotropical bird species. Front. Microbiol. 6:1403. doi: 10.3389/fmicb.2015.01403
Kogut, M. H., Lee, A., and Santin, E. (2020). Microbiome and pathogen interaction with the immune system. Poultry Sci. 99: 1906–1913. doi: 10.1016/j.psj.2019.12.011
Kohl, K. (2012). Diversity and function of the avian gut microbiota. J. Comp. Phys. B 182, 591–602. doi: 10.1007/s00360-012-0645-z
Kreisinger, J., Čížková, D., Kropáčková, L., and Albrecht, T. (2015). Cloacal microbiome structure in a long-distance migratory bird assessed using deep 16sRNA pyrosequencing. PLoS One 10:e0137401. doi: 10.1371/journal.pone.0137401
Kreisinger, J., Kropáčková, L., Petrželková, A., Adámková, M., Tomášek, O., Martin, J.-F., et al. (2017). Temporal stability and the effect of transgenerational transfer on fecal microbiota structure in a long-distance migratory bird. Front. Microbiol. 8:50. doi: 10.3389/fmicb.2017.00050
Liechti, F., Scandolara, C., Rubolini, D., Ambrosini, R., Korner-Nievergelt, F., Hahn, S., et al. (2014). Timing of migration and residence areas during the non-breeding period of barn swallows Hirundo rustica in relation to sex and population. J. Avian Biol. 46, 254–265. doi: 10.1111/jav.00485
Lifjeld, J. T., Kleven, O., Jacobsen, F., McGraw, K. J., Safran, R. J., and Robertson, R. J. (2011). Age before beauty? Relationships between fertilization success and age-dependent ornaments in barn swallows. Behav. Ecol. Sociobiol. 65, 1687–1697. doi: 10.1007/s00265-011-1176-4
Lozupone, C. A., Hamady, M., Kelley, S. T., and Knight, R. (2007). Quantitative and qualitative diversity measures lead to different insights into factors that structure microbial communities. Appl. Environ. Microb. 71, 8228–8235. doi: 10.1128/AEM.01996-06
Lozupone, C., and Knight, R. (2005). UniFrac: a new phylogenetic method for comparing microbial communities. Appl. Environ. Microb. 73, 1576–1585. doi: 10.1128/AEM.71.12.8228-8235.2005
Lu, R., Zhao, X., Li, J., Niu, P., Yang, B., Wu, H., et al. (2020). Genomic characterization and epidemiology of 2019 novel coronavirus: implications for virus origins and receptor binding. Lancet 395, 565–574. doi: 10.1016/S0140-6736(20)30251-8
Lupp, C., Robertson, M. L., Wickham, M. E., Sekirov, I., Champion, O. L., Gaynor, E. C., et al. (2007). Host-mediated inflammation disrupts the intestinal microbiota and promotes the overgrowth of Enterobacteriaceae. Cell Host Microbe 2, 119–129. doi: 10.1016/j.chom.2007.06.010
Machemer, T. (2021). Songbirds Are Spreading A Deadly Disease At Birdfeeders. Smithsonian Magazine Available online at: https://www.smithsonianmag.com/smart-news/songbirds-are-spreading-deadly-disease-birdfeeders-180977222/ (accessed March 12, 2021).
Mansfield, K., and Lehman, S. (2021). Help Protect Wild Birds From Deadly Salmonellosis. Availale online at: https://wdfw.wa.gov/news/help-protect-wild-birds-deadly-salmonellosis (accessed Feb 15, 2021).
McMurdie, P. J., and Holmes, S. (2013). Phyloseq: an R package for reproducible interactive analysis and graphics of microbiome census data. PLoS One 8:e61217. doi: 10.1371/journal.pone.0061217
Møller, A. P. (2014). Life history, predation and flight initiation distance in a migratory bird. J. Evolution. Biol. 27, 1105–1113. doi: 10.1111/jeb.12399
Murray, M. H., Lankau, E. W., Kidd, A. D., Welch, C. N., Ellison, T., Adams, H. C., et al. (2020). Gut microbiome shifts with urbanization and potentially facilitates a zoonotic pathogen in a wading bird. PLoS One 15:e0220926. doi: 10.1371/journal.pone.0220926
Nocker, A., Cheung, C. Y., and Camper, A. K. (2006). Comparison of propidium monoazide and ethidium monoazide for differentiation of live vs. dead bacteria by selective removal of DNA from dead cells. J. Microbiol. Meth. 67, 310–320. doi: 10.1016/j.mimet.2006.04.015
Nogva, H. K., Dromtorp, S. M., Nissen, H., and Rudi, K. (2003). Ethidium monoazide for DNA-based differentiation of viable and dead bacteria by 5’ nuclease, PCR. Biotechniques 810, 804–813. doi: 10.2144/03344rr02
Oksanen, J., Blanchet, F. G., Friendly, M., Kindt, R., Legendre, P., McGlinn, D., et al. (2019). Vegan: Community Ecology Package. R package version 2.5-6.
Pancerasa, M., Ambrosini, R., Saino, N., and Casagrandi, R. (2018). Barn swallows long-distance migration occurs between significantly temperature-correlated areas. Sci. Rep. 8:12359. doi: 10.1038/s41598-018-30849-0
Park, S. H., Aydin, M., Khatiwara, A., Dolan, M. C., Gilmore, D. F., Bouldin, J. L., et al. (2014). Current and emerging technologies for rapid detection and characterization of Salmonella in poultry and poultry products. Food Microbiol. 38, 250–252. doi: 10.1016/j.fm.2013.10.002
Pekarsky, S., Corl, A., Turjeman, S., Kamath, P. L., Getz, W. M., Bowie, R. C. K., et al. (2021). Drivers of change and stability in the gut microbiota of an omnivorous avian migrant exposed to artificial food supplementation. Mol. Ecol. doi: 10.1111/mec.16079 [Epub ahead of print].
Perumbakkam, S., Hunt, H. D., and Cheng, H. H. (2014). Marek’s disease virus influences the core gut microbiome of the chicken during the early and late phases of viral replication. FEMS Microbiol. Ecol. 90, 300–312. doi: 10.1111/1574-6941.12392
Pilastro, A., and Spina, F. (1999). “Fat accumulation in pre-migratory roosting barn swallows in Europe,” in Proceedings of the 22nd International Ornithological Congress, Durban, South Africa, eds. N. J. Adams, and R. H. Slotow (Johannesburg: BirdLife South Africa), 219–228.
Prosser, D. J., Cui, P., Takekawa, J. Y., Tang, M., Hou, Y., Collins, B. M., et al. (2011). Wild bird migration across the Qinghai-Tibetan Plateau: a transmission route for highly pathogenic H5N1. PLoS One 6:e117622. doi: 10.1371/journal.pone.0017622
QGIS Development Team (2009). QGIS Geographic Information System. Open Source Geospatial Foundation. Available online at: http://qgis.org
R Core Team (2020). R: A language and environment for statistical computing. R Foundation for Statistical Computing. Vienna, Austria.
Rhoads, D. D., Cox, S. B., Rees, E. J., Sun, Y., and Wolcott, R. D. (2012). Clinical identification of bacteria in human chronic wound infections: culturing vs. 16S ribosomal DNA sequencing. BMC Infect. Dis. 12:321. doi: 10.1186/1471-2334-12-321
Rogers, A. W. L., Tsolis, R. M., and Bäumier, A. J. (2020). Salmonella versus the microbiome. Microbiol. Mol. Biol. R 85, e27–e19. doi: 10.1128/MMBR.00027-19
Rosario, K., and Breitbart, M. (2011). Exploring the viral world through metagenomics. Curr. Opin. Virol. 1, 289–297. doi: 10.1016/j.coviro.2011.06.004
Safran, R. J., Adelman, J. S., McGraw, K. J., and Hau, M. (2008). Sexual signal exaggeration affects physiological state in male barn swallows. Curr. Biol. 18, R461–R462. doi: 10.1016/j.cub.2008.03.031
Saino, N., Incagli, M., Martinelli, R., and Møller, A. P. (2002). Immune response of male barn swallows in relation to parental effort, corticosterone plasma levels, and sexual ornamentation. Behav. Ecol. 13, 169–174. doi: 10.1093/beheco/13.2.169
Schliep, K. P. (2010). phangorn: phylogenetic analysis in R. Bioinformatics 27, 592–593. doi: 10.1093/bioinformatics/btq706
Schmidt-Wellenburg, C. A., Biebach, H., Daan, S., and Visser, G. H. (2007). Energy expenditure and wing beat frequency in relation to body mass in free flying Barn Swallows (Hirundo rustica). J. Comp. Physiol. B 177, 327–337. doi: 10.1007/s00360-006-0132-5
Schwieger, F., and Tebbe, C. C. (1998). A new approach to utilize PCR-single-strand-conformation polymorphism for 16S rRNA gene-based microbial community analysis. Appl. Environ. Microbiol. 64, 4870–4876. doi: 10.1128/AEM.64.12.4870-4876.1998
Sorbara, M. T., and Pamer, E. G. (2019). Interbacterial mechanisms of colonization resistance and the strategies pathogens use to overcome them. Mucosal Immunol. 12, 1–9. doi: 10.1038/s41385-018-0053-0
Srinivasan, R., Karaoz, U., Volegova, M., MacKichan, J., Kato-Maeda, M., Miller, S., et al. (2014). Use of 16SS rRNA gene for identification of a broad range of clinically relevant bacterial pathogens. PLoS One 10:e0117617. doi: 10.1371/journal.pone.0117617
Stecher, B., Robbiani, R., Walker, A. W., Westendorf, A. M., Barthel, M., Kremer, M., et al. (2007). Salmonella enterica serovar typhimurium exploits inflammation to compete with the intestinal microbiota. PLoS Biol. 5:e244. doi: 10.1371/journal.pbio.0050244
Thompson, L. R., Sanders, J. G., McDonald, D., Amir, A., Ladau, J., Locey, K. J., et al. (2017). A communal catalogue reveals Earth’s multiscale microbial diversity. Nature 551, 457–463. doi: 10.1038/nature24621
Tizard, I. (2004). Salmonellosis in wild birds. Semin. Avian Exot. Pet Med. 13, 50–66. doi: 10.1053/j.saep.2004.01.008
Turjeman, S., Corl, A., Wolfenden, A., Tsalyuk, M., Lublin, A., Choi, O., et al. (2020). Migration, pathogens, and the avian microbiome: a comparative study in sympatric migrants and residents. Mol. Ecol. 29, 4706–4720. doi: 10.1111/mec.15660
van der Waaij, D. (1989). The ecology of the human intestine and its consequences for overgrowth by pathogens such as Clostridium difficile. Annu. Rev. Microbiol. 43, 69–87. doi: 10.1146/annurev.mi.43.100189.000441
Videnska, P., Sisak, F., Havlickova, H., Faldynova, M., and Rychlik, I. (2013). Influence of Salmonella enterica serovar enteritidis infection on the composition of chicken cecal microbiota. BMC Vet. Res. 9:140. doi: 10.1186/1746-6148-9-140
Westergren, V., Bassiri, M., and Engstrand, L. (2009). Bacteria detected by culture and 16S rRNA sequencing in maxillary sinus samples from intensive care unit patients. Laryngoscope 113, 270–275. doi: 10.1097/00005537-200302000-00014
Wickham, H. (2016). ggplot2: Elegant Graphics for Data Analysis. New York, NY: Springer-Verlag. doi: 10.1007/978-0-387-98141-3
Wiedemann, A., Virlogeux-Payant, I., Chaussé, A.-M., Schikora, A., and Velge, P. (2014). Interactions of Salmonella with animals and plants. Front. Microbiol. 5:791. doi: 10.3389/fmicb.2014.00791
Wilson, K. M., Briggs, W. N., Duff, A. F., Chasser, K. M., Sun, X., and Bielke, L. R. (2018). Comparison of microbiome and culture techniques for determination of gastrointestinal microbial communities in ceca of chickens. bioRxiv [Preprint]. Available online at: https://doi:10.1101/494781 (accessed September 15, 2020).
Wright, E. S. (2015). DECIPHER: harnessing local sequence context to improve protein multiple sequence alignment. BMC Bioinformatics 16:2–14. doi: 10.1186/s12859-015-0749-z
Keywords: barn swallows, microbiome, Salmonella, culture, 16S rRNA sequencing, disease surveillance
Citation: Choi ON, Corl A, Wolfenden A, Lublin A, Ishaq SL, Turjeman S, Getz WM, Nathan R, Bowie RCK and Kamath PL (2021) High-Throughput Sequencing for Examining Salmonella Prevalence and Pathogen—Microbiota Relationships in Barn Swallows. Front. Ecol. Evol. 9:683183. doi: 10.3389/fevo.2021.683183
Received: 20 March 2021; Accepted: 14 September 2021;
Published: 05 October 2021.
Edited by:
Kimberly VanderWaal, University of Minnesota Twin Cities, United StatesReviewed by:
Alexandra DeCandia, Smithsonian Conservation Biology Institute (SI), United StatesSimone Sommer, University of Ulm, Germany
Copyright © 2021 Choi, Corl, Wolfenden, Lublin, Ishaq, Turjeman, Getz, Nathan, Bowie and Kamath. This is an open-access article distributed under the terms of the Creative Commons Attribution License (CC BY). The use, distribution or reproduction in other forums is permitted, provided the original author(s) and the copyright owner(s) are credited and that the original publication in this journal is cited, in accordance with accepted academic practice. No use, distribution or reproduction is permitted which does not comply with these terms.
*Correspondence: Olivia N. Choi, olivia.choi@maine.edu; Ammon Corl, ammoncorl@berkeley.edu; Rauri C. K. Bowie, bowie@berkeley.edu; Pauline L. Kamath, pauline.kamath@maine.edu
†These authors share first authorship