- School of Environment, Beijing Normal University, Beijing, China
Grazing activities perhaps lead to habitat quality degradation and animal biodiversity loss while the effects on the Qinghai-Tibet Plateau (QTP) is still relatively poorly studied. Based on the Integrated Valuation of Ecosystem Services and Tradeoffs model, geographical detector model and generalized linear mixed model, the responses of habitat quality and animal biodiversity to grazing activities at 5 km grid scale were analyzed. Results showed that the overall habitat quality on the QTP was high with 76.43% of the total area, and poor level accounted for 19.56%. High level habitat was mainly distributed in the southern part, while the poor level in the northern part. The mean grazing activity explanatory ability to habitat quality, bird species richness and mammal richness were 0.346, 0.430, and 0.354. The interaction effects between slope and grazing activities on habitat quality, bird species richness and mammal richness were the most important interaction effects, and the area affected by the interaction was 73.82, 46.00, and 46.17% of habitat quality, bird species richness and mammal richness, respectively. The interaction effects on habitat quality, bird species richness and mammal richness all showed “low in the northwest and high in the southeast”. Grazing activities and habitat quality had a positive correlation while bird species richness, and mammal richness negative correlations. The spatial relationship of grazing activities of habitat quality was “higher in the middle and lower around the periphery”, while the spatial distribution of grazing activities of bird species richness and mammal richness was “higher in the east and lower in the west”. This study explicitly revealed the responses of habitat quality and animal biodiversity to grazing activities, thus providing references for biodiversity conservation on the QTP.
Introduction
Grassland is one of the most extensive ecosystems in the world (Li L. et al., 2019). As an important ecological land type in China, grassland not only serves a range of ecosystem services, but also provides livestock products, thus bringing economic income to residents (Wang Y. et al., 2020). In China, grazing activities are mainly distributed in the northern and western regions. With the development of economy, grazing activities are increasingly intensified, and overgrazing has occurred in many areas (Zhao et al., 2020a). However, intensive grazing activities can lead to grassland degradation, which in turn affects habitat quality and reduces biodiversity (Liu et al., 2020).
The Qinghai-Tibet Plateau (QTP), as the largest plateau in the world, has a grassland area of 1.59 × 106 km2, accounting for up to 60% of the total area (Liu et al., 2021b). It not only plays an important role in water retention, but also is an important ecological security barrier in China with great biodiversity protection value (Wang Y. et al., 2020). However, studies have shown that in recent years, the QTP is facing many threats with the intensification of human activities, such as excessive grazing, large-scale infrastructure construction, resulting in ecological degradation, wildlife habitat fragmentation, and biodiversity loss (Dong et al., 2020). Therefore, it is urgent to strengthen the protection of biodiversity on the QTP.
Habitat quality is an important indicator of regional ecological security, which can reflect the level of regional biodiversity and ecosystem services (Tang et al., 2020; Zhu C. et al., 2020). Therefore, habitat quality has become a hot issue in ecological security research. At present, habitat quality can be assessed based on the measured species diversity, or through the analysis of the evolution of the habitat by parameter substitution (Andrus et al., 2021). As the parameter substitution method has become the main method, the relationship between resources and habitat suitable land is mainly discussed from the perspective of landscape pattern (Zhu Z. et al., 2020). Generally, it is believed that construction land, cultivated land and roads are the main sources of threats to habitat quality, while natural ecosystems provide a relatively complete habitat for species, and their habitat quality is overall good (Zhang H. et al., 2020). Current studies on habitat quality are mainly based on the above threat factors, and the Integrated Valuation of Ecosystem Services and Tradeoffs (InVEST) model is used to evaluate the regional habitat quality (Moreira et al., 2018).
Current studies about the relationship between habitat quality and human disturbances mainly focus on the response of habitat quality to land use change (Roche et al., 2016; Yang H. et al., 2021). These studies are usually divided into two categories, one is about the impact of long-term land use change on habitat quality, and the second is mainly about simulating and predicting the impact of future land use change on habitat quality. Some of these studies revealed the impact of long-term land use change on habitat quality of coastal zones (Zhang X. et al., 2020), there were also studies on long-term land use change on habitat quality at county level (Tang et al., 2020). These studies all found that long-term increase of land use change would lead to continuous decline in habitat quality. The second category is mainly based on the current land use change to simulate and predict the impact of future land change on habitat quality. Previous studies have revealed the impact of future land use change on habitat quality across China, and found that the expansion of large cities had a greater impact on future habitat quality than that of small cities (Song et al., 2020). There were also studies on the direct and indirect impacts of urban expansion on habitat quality in the future, and it was found that the indirect impact of urban expansion on habitat quality was greater than the direct impact (Yang et al., 2020).
Grazing activities, as one of the most important human activities on the QTP, is the direct cause of grassland degradation (Zhang Y. et al., 2021). Grassland is the largest ecosystem on the QTP, and its degradation will have serious impacts on ecosystem services (Dong et al., 2020). However, there have been few studies on the ecological effects of grazing activities on the QTP at a large scale. Because of its special geographical environment, the QTP has a fragile ecological environment (Li L. et al., 2019). Overgrazing activities will affect the plant community and reduce the height and coverage of vegetation. Studies have shown that changes in vegetation structure characteristics have significant effects on the community structure, bird species richness and mammal richness (Leal et al., 2019).
In addition, the impact of grazing activities on habitat quality is also particularly obvious (Su et al., 2020). However, as far as the ecological effects of grazing activities are concerned, many studies have focused on the stress of grazing activities on plants or the impact on wild animal habitats (Zhang X. et al., 2021). Some studies have compared and analyzed the difference between long-term grazing exclusion and regular grazing activities on vegetation biodiversity, and found that long-term grazing exclusion would reduce vegetation biodiversity, while regular grazing activities was a better management strategy to restore and improve biodiversity (Yao et al., 2019). As for the impact of different grazing measures on habitat quality under the implementation of the policy, some studies have explored the impact of long-term grazing exclusion and sustainable grazing activities on habitat quality, and found that the longer the grazing exclusion lasted, the less the biodiversity of vegetation decreased, but moderate grazing activities would effectively restore vegetation biodiversity (Liu et al., 2020).
However, most studies focused on a single species or single habitat. Due to the particularity of the QTP and the availability of biodiversity data, the effect of grazing activities on habitat quality is still lacking. In addition, the QTP has a vast territory, so the effects of grazing activities on habitat quality and animal biodiversity are different (Dong et al., 2020; Liu et al., 2020). In addition, up to now, studies on mammal richness and bird species richness have mainly focused on forest ecosystems, and there are relatively few research topics about the responses of habitat quality and animal biodiversity to grazing activities on grassland ecosystems. Therefore, the study on the responses of habitat quality and animal biodiversity to grazing activities on the QTP will cover the gap of this field. Here, three issues should be addressed as follows: (1) how is the habitat quality distributed. (2) How are the independent and interaction effects of grazing activities on habitat quality and animal biodiversity distributed at the spatial scale? (3) What is the spatial relationship between grazing activities and habitat quality and animal biodiversity?
Most of the methods to analyze the impact of human activities on vegetation biodiversity and habitat quality were correlation analysis, linear regression (Gosselin and Callois, 2018; Yohannes et al., 2021). Some advanced methods were adopted to reveal more mechanism, such as generalized linear model (GLM) and censored linear regression to analyze the impact of grazing activities on habitat sustainability (Moore et al., 2018; Leal et al., 2019). However, there are generally two problems in the above methods, one is that the interaction between factors is not considered in the study of biodiversity conservation by grazing activities, the other is that the fixed and random effect of factors on biodiversity conservation and habitat quality is not taken into consideration. Based on these considerations, the geographical detector method could be a good solution to the first problem, as it is a spatial method for examining the interaction of two explanatory variables on dependent variables (Han et al., 2021). For the second problem, the generalized linear mixed model (GLMM) is very suitable because it takes the fixed and random effects of the factors into account. Studies have shown that the GLMM can improve the accuracy of the results more effectively than the traditional method (Evans and Holsinger, 2012; Hubin and Storvik, 2018).
Therefore, the InVEST model, geographical detector method and GLMM were applied to conduct research in this study to (1) explore the spatial distribution characteristics of habitat quality and animal biodiversity in grazing regions, (2) study the independent effects of grazing activities and interaction effects between grazing activities and various factors on habitat quality and animal biodiversity, and (3) analyze the relationship between grazing activities and habitat quality and animal biodiversity on the basis of considering fixed and random effects. Through the research on the responses of habitat quality and animal biodiversity to grazing activities on the QTP, we aim to provide effective references for the habitat quality improvement and biodiversity conservation.
Materials and Methods
Study Area
Located in southwest China (26°00′–39°47′N, 73°19′–104°47′E), the QTP is the largest plateau in the world, covering an area of about 2.57 × 106 km2 (Figure 1) (Cao et al., 2018). It is known as “the roof of the world” for its high altitude and “the third pole of the Earth” for its alpine environment (Chen et al., 2021). The climate of the QTP is characterized by intense radiation, much sunshine, low temperature, and small accumulated temperature (Jiang et al., 2020). The mean annual solar radiation varies from 5,000 to 8,500 MJ/m2, the average annual precipitation ranges from 415 to 515 mm, which mainly occurs from May to September, and the average temperature is between −2.2 and 0°C (Li et al., 2013). The vegetation types on the QTP are forest, meadow, grassland, desert, and alpine vegetation. Among them, the alpine grassland with the largest area is concentrated in the Northern Tibetan Plateau, accounting for 27.54% of the total plateau area (Zhan et al., 2021). The second is alpine meadow, mainly distributed in the headwaters of the Yarlung Zangbo River, Yellow River, Yangtze River, and Lancang River, accounting for more than 25% of the total area of the plateau. Alpine desert accounted for 8.96% of the total area, and the vegetation coverage decreases gradually from southeast to northwest (Wang D. et al., 2020). In general, grazing activities is one of the most important activities, although the extent of grazing activities shows large spatial differences on the QTP. The grazing activities is weak in the southern part of the QTP, and strong in the central and southern part of Qinghai province and the northeast part of the QTP (Li et al., 2019b). There are many precious animals on the QTP, such as the golden monkey, Tibetan antelope, wild yak, Tibetan wild donkey, argali sheep, snow leopard and so on (Huang et al., 2020). The main animal species are bird species and mammal richness, and the amphibians are very few (Yao et al., 2019).
Data Sources
For the selection of animal biodiversity indicators, although there is amphibian richness on the QTP, but the numbers are very small, and there is no data on insects, so these two types of datasets were not taken into consideration. Therefore, we chose bird species richness with a spatial resolution of 10 km and mammal richness with a spatial resolution of 1 km as the representatives of animal on the QTP. Based on the differences in the spatial scale of animal biodiversity between bird species richness and mammal richness, the response of grazing activities to animal biodiversity is investigated at 5 km grid scale. The datasets of motor way, national way, provincial way and railway data are in the vector scale of 1:250,000. The remaining datasets are raster data with a spatial resolution of 1 km except for the dataset of bird species richness. In addition, the brief description, website, unit and time of data are listed in Table 1.
Methods
In this study, the InVEST model was applied with LULC dataset, threat factors, habitat sustainability of LULC types and their sensitivity to threat factors. Geographical detector model was used to analyze the independent effects of grazing activities and interaction effects between grazing activities and the factors on habitat quality and animal biodiversity. Finally, GLMM was used to analyze the relationship between grazing activities and habitat quality and animal biodiversity based on the consideration of random and fixed effects.
Habitat Quality Model
Habitat quality refers to the ability of an ecosystem to provide living conditions for individual organisms and populations, which is represented by the availability of biological resources, and the number of biological reproduction and existence (Caro et al., 2020). The habitat quality change is thought to be representative of changes in genes, species, or ecosystems (Tang et al., 2020). Habitat quality model was used to examine the spatial distribution of habitat quality. The total threat level of habitat is formulated by the Eq. 1 as follows (Song et al., 2020):
Where, Dxj represents for threat level of the xth grid in the jth LULC type; y represents for the total grids on threat factor r’s raster; wr stands for the weight of the threat factor r; ry is the threat intensity of the yth grid; irxy denotes the distance between the habitat and the threat source; βx is the accessibility level of the xth grid; Sjr represents for the jth land cover type’s sensitivity on the threat factor r. irxy has two decay functions, linear and exponential decay, which can be expressed as Eqs 2 and 3, shown as follows (Moreira et al., 2018):
Where, dxy is the straight-line distance between the xth grid and the yth grid; dr max is the maximum impact distance (Max_Dist) of threat factor r; The Max_Dist, distance attenuation function and weight of each stress factor are comprehensively referred to relevant literature, as shown in Table 2 (Tang et al., 2020; Li et al., 2021).
After the calculation of Dxj, the habitat quality of each grid can be calculated by the Eq 4 combining land cover and threat factors as follows (Ma et al., 2021):
Where Qxj stands for the habitat quality of x in the jth grid; Hj is the habitat sustainability of the jth grid; represents for threat level of the xth grid in the jth LULC type; z is a normalized constant, usually equal to 2.5 and k is half-saturation constant, with a default value of 0.5. The value of Qxj ranges from 0 to 1, and the higher the value, the better the habitat quality.
Based on the research of Tang et al. (2020) and Wei Y. et al. (2021), the habitat suitability of LULC types and their sensitivity to various threat factors on the QTP are obtained, shown in Table 3 (Tang et al., 2020; Wei Y. et al., 2021).
Geographical Detector Method
The geographical detector method, proposed by Wang et al. (2010), is usually applied to quantify the impact of influencing factors and determine whether the spatial distribution of the explanatory variable is the same as that of the dependent variable (Wang et al., 2010). The geographical detector method is not based on linear assumptions, but rather compares the spatial consistency of the distribution of independent variables with the geographic layer with the underlying factors (Liu et al., 2021a). The geographical detector consists of four kinds of detectors, which are factor detector, interaction detector, ecological detector, and risk detector (Fan et al., 2021). In this study, factor and interaction detector method are used to analyze the effects of grazing activities on habitat quality and animal biodiversity.
Factor Detector Method
The factor detector is applied to evaluate the independent effects of the explanatory variable on independent variable. The explanatory power of each factor is expressed by q value, and the formula is shown in the Eq. 5 as follows (Zhao et al., 2020b):
Where q is the explanatory ability of explanatory variable to independent variable; N is the total number of grids and h stands for the number of classifications of independent variable; Nh is the number of samples in h; σh2 and σ2 represent the variance of the independent variable for the units in class h and the entire region of different grids, respectively. The value of q is from 0 to 1. If h is produced by explanatory variable, then the q value means that the explanatory variable accounts for 100 × q% of independent variable. The greater the value of q, the more explanatory ability explanatory variable has on independent variable.
Interaction Detector Method
The interaction detector is used to investigate the interaction effects between two explanatory variables on independent variable, namely q(X1) and q(X2), in which the interaction effects (q (X1∩ X2)) can be roughly divided into three types: weakening, enhancement, and independence. The detailed description and interaction relationships are shown in Table 4 (Qiao et al., 2019).
Generalized Linear Mixed Model
Generalized linear mixed model is a method to explore the relationship between independent variables and dependent variables on the basis of considering the random and mixed effects of independent variables. As an extension of GLM, GLMM is mainly suitable for dealing with discrete random variables that do not obey the normal distribution, mainly including three parts: the distribution of the independent variable y, connection function and linear prediction of the system. One of the common connection function of exponential distribution family into five kinds, which are normal distribution, binomial distribution, Poisson distribution, exponential distribution, and gamma distribution, respectively (Li et al., 2012). The expression of GLMM is shown in the Eq 6 as follows (Venables and Dichmont, 2004):
Where y is an observation vector of n × 1, X is a known design matrix of n × p, z is a design matrix of n × q, β is an unknown parameter vector of p × 1, b is a random vector of q × 1, ε is a random error vector of n × 1, b, and ε are independent of each other.
Study Framework
The purpose of this study was to analyze the responses of habitat quality and animal biodiversity to grazing activities on the QTP. Firstly, the InVEST model was used to analyze the habitat quality. Secondly, the independent and interaction effects of grazing activities on habitat quality and animal biodiversity were explored by geographical detector method. Finally, based on the results of geographical detector method, the GLMM was applied to reveal the relationships between grazing activities and habitat quality and animal biodiversity in the case of random and fixed effects. The overall technical approach was illustrated in Figure 2.
Results
Spatial Distribution Characteristics of Habitat Quality and Animal Biodiversity
The spatial distribution characteristics of habitat quality, bird species richness and mammal richness in grazing regions on the QTP was shown in Figure 3. The proportion of poor, low, moderate, good, and high habitat quality of grazing regions on the QTP was 19.56, 0.55, 1.67, 1.79, and 76.43%, respectively, indicating that the habitat quality level of the QTP was mainly high, followed by poor habitat quality (Figure 3A). The areas with poor habitat quality were distributed throughout the whole area, but mainly in the central and northern regions, while the areas with low habitat quality were only distributed in part of the southern and eastern areas. The regions with good habitat quality were distributed in the southern part of the QTP, while the areas with high habitat quality were distributed in all regions, and mainly in the western, southern and southeastern regions.
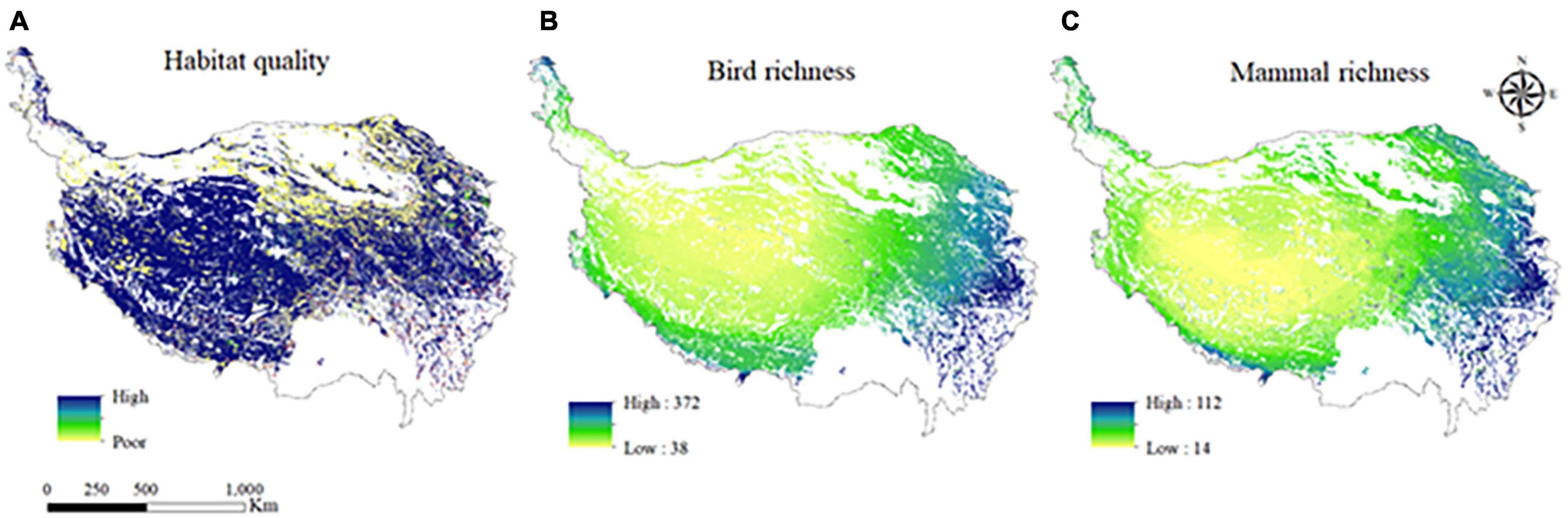
Figure 3. The spatial distribution of habitat quality (A); bird species richness (B); and mammal richness (C) in the grazing regions on the Qinghai-Tibet Plateau (QTP).
Although the number of mammal richness was smaller than that of bird species richness, the spatial distribution characteristics of bird species richness and mammal richness were generally similar, with the lowest in the central region and an upward trend from middle to all directions (Figures 3B,C). Both bird species richness and mammal richness reached their maximum in the southeastern region of the QTP.
Independent and Interaction Effects of Grazing Activities on Habitat Quality and Animal Biodiversity
The factor detector was applied to analyze the independent effects of grazing activities on habitat and animal biodiversity, and the spatial distribution of the influence was shown in Figure 4.
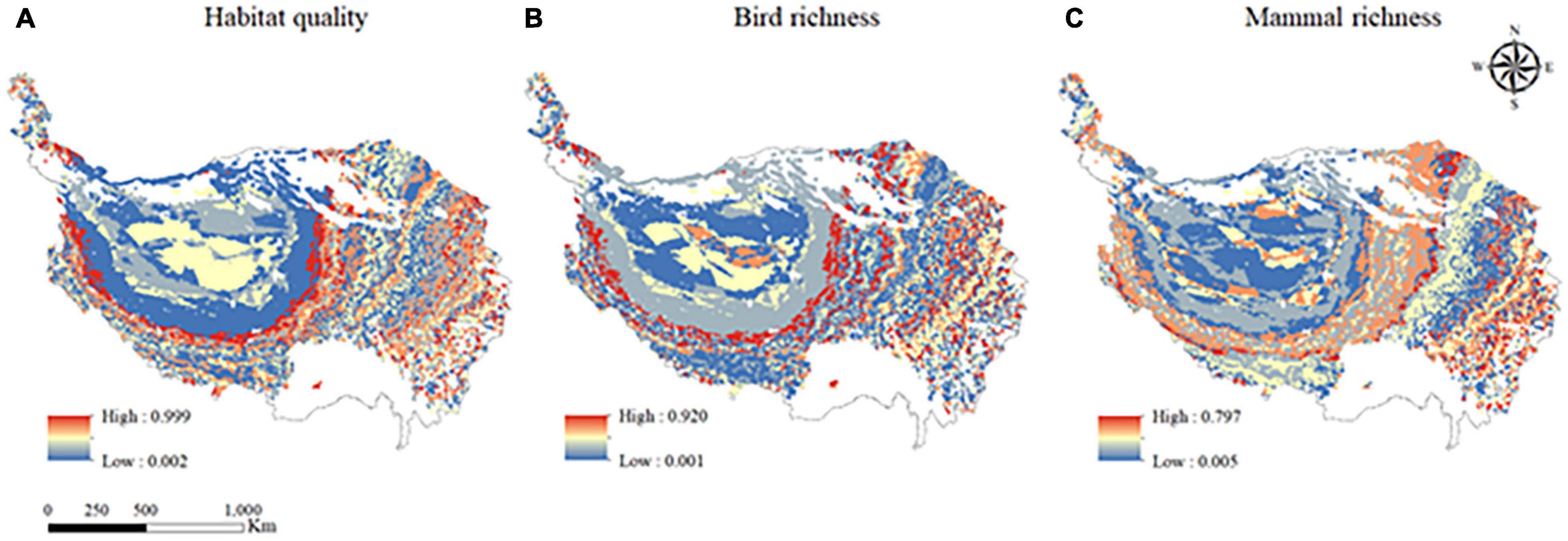
Figure 4. The spatial distribution of the independent effect of grazing activities on habitat quality (A); bird species richness (B); and mammal richness (C).
The influence of grazing activities on habitat quality ranged from 0.002 to 0.999, and the distribution characteristics showed a decreasing trend from east to west on the QTP (Figure 4A). The areas with the largest impact were mainly concentrated in the southeastern part of the QTP, while the areas with the least impact were mainly concentrated in some areas of Tibet province on the QTP.
The influence range of grazing activities on bird species richness was 0.001–0.920, and the distribution characteristics mainly showed a trend of decreasing first, then increasing, and finally decreasing from east to west on the QTP (Figure 4B). The areas with the largest impact were mainly concentrated in part of Qinghai province and the southwest region of Tibet province on the QTP, while the areas with small impact were mainly concentrated in the western region of the QTP.
The influence of grazing activities on mammal richness ranged from 0.005 to 0.797 (Figure 4C). The regions with the largest impact were mainly concentrated in the southeastern part of the QTP, while the regions with small impact were mainly concentrated in some areas of Qinghai and Tibet provinces.
The interaction effects between grazing activities and various influencing factors on habitat quality and animal biodiversity were analyzed, and it was found that the interaction between grazing activities and all factors all showed bivariate enhancements or nonlinear enhancements, indicating that the interaction effects between grazing activities between all factors were all greater than the single action of the grazing activities. The factors with the greatest interaction with grazing activities in different grids were screened out, and the result was shown in Figure 5.
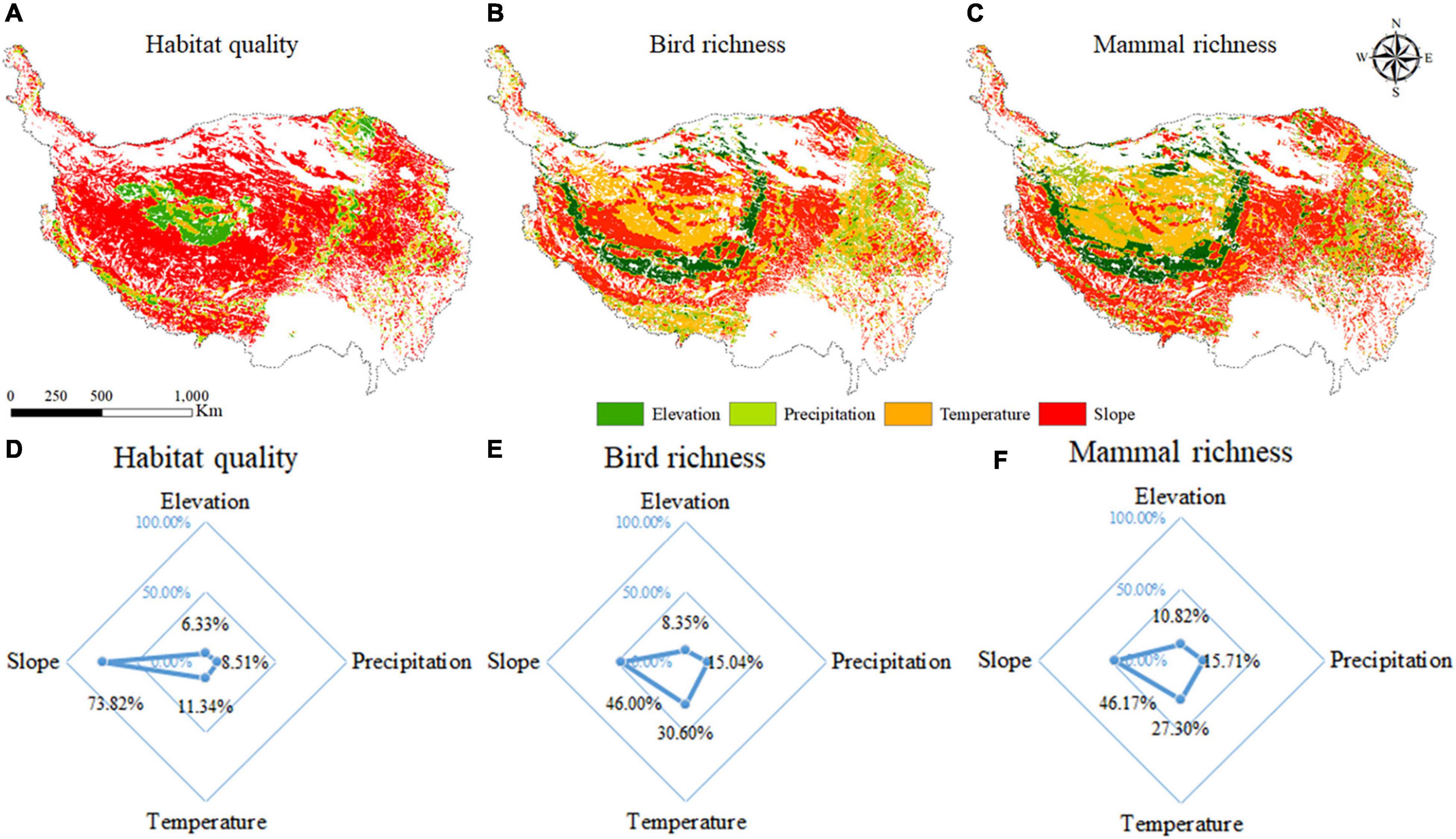
Figure 5. The spatial distribution of the maximum interaction between grazing activities and the factor on habitat quality (A); bird species richness (B); and mammal richness (C); and the area proportion with the largest interaction effects between grazing activities and the factor on habitat quality (D); bird species richness (E); and mammal richness (F).
The results showed that the interaction effects between grazing activities and slope were the main interaction on the QTP, and the areas affected by the interaction effects between grazing activities and slope were mainly distributed in most regions except the central region of the QTP (Figure 5A). The interaction effects between grazing activities and elevation, precipitation, temperature, and slope had the greatest impact on habitat quality, with the area proportions of 6.33, 8.51, 11.34, and 73.82%, respectively (Figure 5D).
The interaction effects between slope and grazing activities were the largest, which were mainly distributed in the central and northeastern regions, followed by the interaction effects between grazing activities and temperature, which was mainly distributed in the northwest region (Figure 5B). The interaction effects between grazing activities and elevation, precipitation, temperature, and slope had the greatest impact on bird species richness, with the area proportions being 8.35, 15.04, 30.60, and 46.00%, respectively (Figure 5E).
Among them, the largest interaction effect between precipitation and slope were mainly distributed in the central to eastern and central to southwestern regions (Figure 5C). The interaction effects between grazing activities and altitude, rainfall, temperature, and slope had the largest impact on mammal richness, with the area proportions being 10.82, 15.71, 27.30, and 46.17%, respectively (Figure 5F).
The influence of the maximum interaction effects between grazing activities and the factor on habitat quality, bird species richness, and mammal richness was shown in Figure 6. The interaction effects on habitat quality ranged from 0.068 to 1.000 (Figure 6A). The areas with the weakest interaction effects were mainly concentrated in the central part to the west on the QTP, while the regions with the strongest interaction effects were mainly distributed in the central, eastern, and southeastern parts of the QTP.
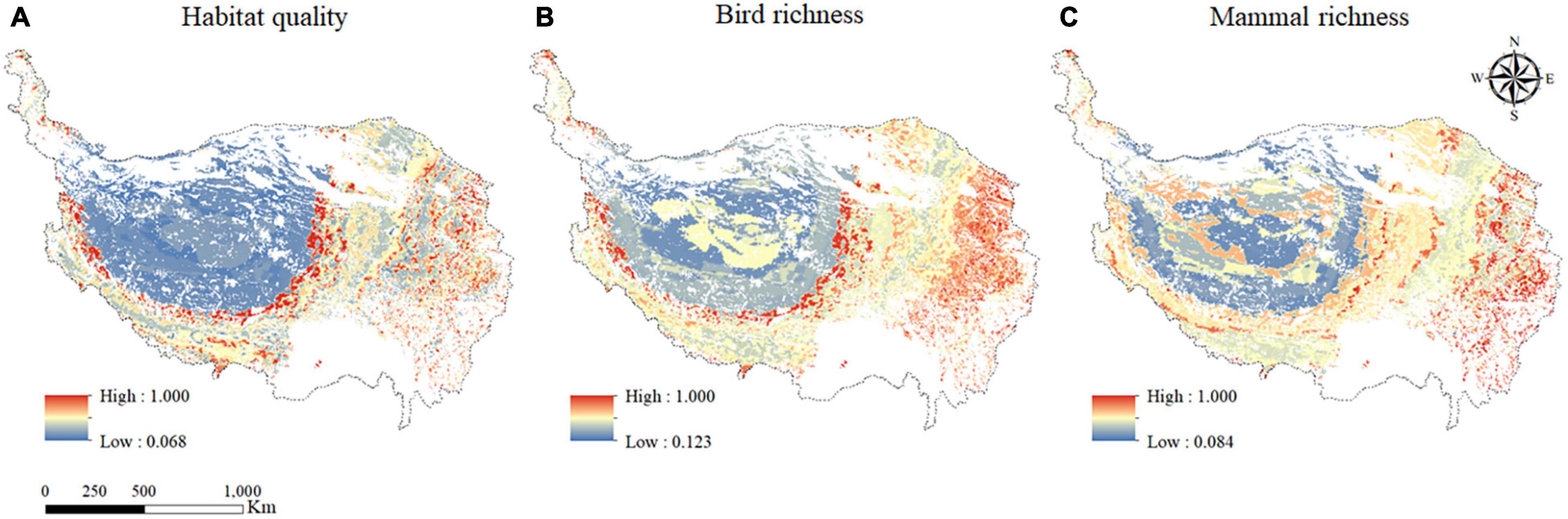
Figure 6. The spatial distribution of maximum interaction effects between grazing activities and the factor on habitat quality (A); bird species richness (B); and mammal richness (C) in the grazing regions on the QTP.
The interaction effects on bird species richness ranged from 0.123 to 1.000 (Figure 6B). The areas with the weakest interaction effects were mainly concentrated in the north and part of the west, while the regions with the strongest interaction effects were mainly concentrated in the east and southeast.
The interaction effects on mammal richness ranged from 0.084 to 1.000 (Figure 6C). The areas with the weakest interaction effects were concentrated in the northwest part of the QTP, while the regions with the strongest effect were mainly concentrated in the southeast of the QTP.
In general, although the factors of maximum interaction effects affected by habitat quality, bird species richness, and mammal richness in each region were different, the distribution of maximum interaction degree on habitat quality, bird species richness, and mammal richness were roughly the same, with the characteristics of “lower in the northwest and higher in the southeast”.
Relationships Between Grazing Activities and Habitat Quality and Animal Biodiversity
Based on the results of the geographical detector, the factor with the least interaction of grazing activities in each grid was selected, and the factor and grid number were assumed as the random effect of the GLMM of different grid. The other four variables were set as the fixed effect of different grids, and then the GLMM of different grids was established. After the significance analysis of the parameter estimation of the fixed effect items, the fixed variables were gradually removed, the modeling was carried out. The obtained model was analyzed by variance to determine the final random effect factors, and the optimal GLMM of each grid was screened out. Since grazing activities was the main influencing factor of habitat quality and animal biodiversity and was fixed effect in each grid, only the regression coefficient distribution of grazing activities was displayed in Figure 7.
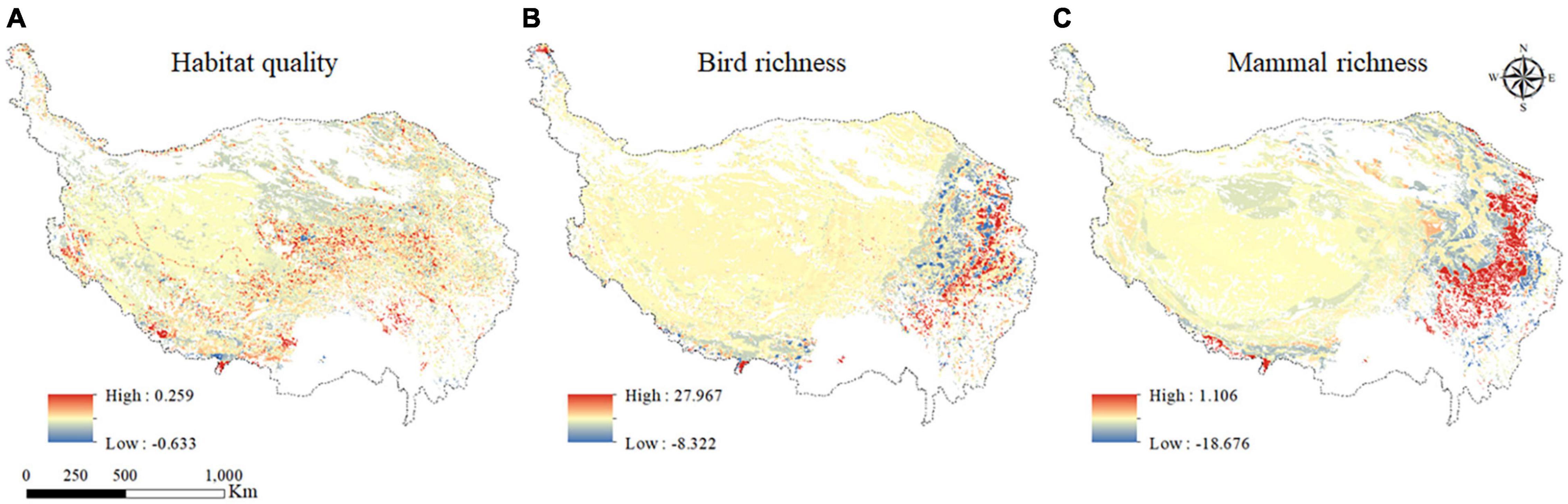
Figure 7. Regression coefficient distribution of grazing activities of habitat quality (A); bird species richness (B); and mammal richness (C).
Grazing activities was the main influencing factor of habitat quality, the regression coefficients of grazing activities ranged from −0.633 to 0.259, and there were both positive and negative distribution in the study area, indicating that the impact of grazing activities on habitat quality was not stable (Figure 7A). According to the absolute value of the regression coefficient of grazing activities, the spatial distribution of grazing activities was generally “high in the middle and low around the periphery”. There was a positive correlation between grazing activities and habitat quality, and the positive correlation regions were mainly distributed in the south, northwest, and southeast of the QTP.
The regression coefficient of grazing activities of bird species richness ranged from −8.322 to 27.967 (Figure 7B), with both positive and negative distribution, indicating that the influence of grazing activities on bird species richness was not stable. According to the absolute value of the regression coefficient of grazing activities, the spatial distribution of grazing activities showed a downward trend from east to west, with the highest value in the east and the lowest value in the west. In general, there was a negative correlation between grazing activities and bird species richness, and the positive correlation regions were distributed in all regions, but mainly in the eastern and central parts of the QTP.
The regression coefficients of grazing activities of mammal richness were between −18.676 and 1.106, with both positive and negative distribution, indicating that grazing activities had an unstable influence on mammal richness (Figure 7C). According to the absolute value of the regression coefficient of grazing activities, the spatial distribution characteristics of grazing activities mainly showed a trend of low in the west and high in the east, with the lowest value in the west and the highest value in the east. In general, grazing activities and mammal richness were mainly negatively correlated, and the areas with positive correlation were mainly distributed in the eastern and central regions of the QTP.
For bird species richness and mammal richness, the spatial distribution characteristics of regression coefficients of grazing activities on the QTP were generally similar, and both were mainly negatively correlated, and the areas with positive correlation were mainly distributed in the eastern region.
Discussion
Rationality of Indicators and Model Selection
In this study, we applied InVEST model to estimate the habitat quality on the QTP. Although the parameter setting of InVEST model was subjective to a certain extent, it was still a good method to explore the habitat quality of the QTP for the following two reasons. First, it can replace the detailed method to quickly examine the habitat quality changes (Zhu C. et al., 2020). Second, due to the large area of the QTP, there was a lack of species distribution data in many areas, and this method could well solve the problem of missing data which cannot be estimated (Zhang X. et al., 2020). Third, the variability of different habitats to the same environment under threat was considered (Tang et al., 2020).
Generally, cultivated land and construction land were selected as indicators in the habitat quality module of InVEST model (Shaffer et al., 2019). However, with the deepening of the study on habitat quality, road factors were added to the selection of threat factors from the initial cultivated land and construction land (Zhu C. et al., 2020). In recent years, GDP and population factors have been gradually added into the research, making the indicators selection more comprehensive. Therefore, for a comprehensive consideration, this study selected cultivated land, construction land, road factors, GDP and population as threat sources (Zhao and Li, 2020).
In traditional studies, only single factor is considered to affect the biodiversity conservation. However, in addition to the individual effect of the factor, biodiversity conservation is also affected by interaction effect between the factors, which is often ignored (Fan et al., 2021). In addition, the influence of factor on biodiversity conservation at grid scale is not necessarily a completely fixed part. Some factors may have individual differences and thus become random parts, and their influence on habitat quality may be random effects (Venables and Dichmont, 2004). However, in this kind of research, the influence of factor is usually unified as fixed effect, and the possible random effect of factors is often ignored. Therefore, geographical detector and GLMM were applied in this study.
Relationship Between Habitat Quality and Animal Biodiversity
Traditionally, there has been a high correlation between habitat quality and animal biodiversity (Edmonds et al., 2021). However, the responses of habitat quality and animal biodiversity to grazing activities on the QTP has not been studied. As the largest plateau in the world, the QTP had a large area and a relatively complex ecological environment (de Lima Filho et al., 2021). Due to the great differences in natural environment, the distribution of grazing activities, animal biodiversity, and habitat quality in different regions on the QTP were all spatially heterogenous (Su et al., 2020), therefore, some studies have inferred that the responses of habitat quality and animal biodiversity to grazing activities varies with different regional locations. In addition, the spatial resolution of raster data used in this study was 1 km except for the spatial resolution of bird species richness, which was 10 km. Studies have shown that grazing activities would directly affect the plant community on grassland and reduce the height and coverage of vegetation, leading to the reduction of vegetation types, and indirectly interfering with the activities of birds on grassland (Li L. et al., 2018). The ecological environment of the QTP was complex, the bird species richness varied in different regions because of the different tolerance of different birds to grazing activities (Wang Y. et al., 2020). Based on comprehensive consideration of the above research conclusions, the responses of habitat quality and animal biodiversity to grazing activities were selected at 5 km grid scale.
The spatial relationship between habitat quality and animal biodiversity was analyzed, and the result was shown in Figure 8. The correlation coefficients between habitat quality and bird species richness ranged from −0.354 to 0.666 (Figure 8A), and that between habitat quality and mammal richness ranged from −0.256 to 0.708 (Figure 8B). The regions with higher positive correlation between habitat quality and bird species richness and mammal richness were mainly concentrated in some regions in the eastern and southwest of the QTP. The regions with low correlation mainly concentrated in some areas in central and southern Tibet province and the northern and western part of Qinghai province. The area with negative correlation between habitat quality and bird species richness accounted for 30.38%, which was mainly distributed in the northern region of Tibet, while the areas with negative correlation between habitat quality and mammal richness accounted for 53.54%, which were mainly distributed in the western region of QTP. Above results were consistent with the results of existing studies. The studies have shown that the vegetation cover of the QTP showed a decreasing trend from southeast to northwest, and the higher the vegetation cover, the better the habitat quality (Wei H. et al., 2021). As a place for animals to survive, the quality of habitat would affect animal biodiversity (Chabuz et al., 2019). However, because of the special characteristics of the QTP, the eastern region, although it would take longer to recover after being damaged by human activities, also became a more suitable habitat for animals on the QTP due to its relatively suitable habitat conditions (Shi et al., 2018).
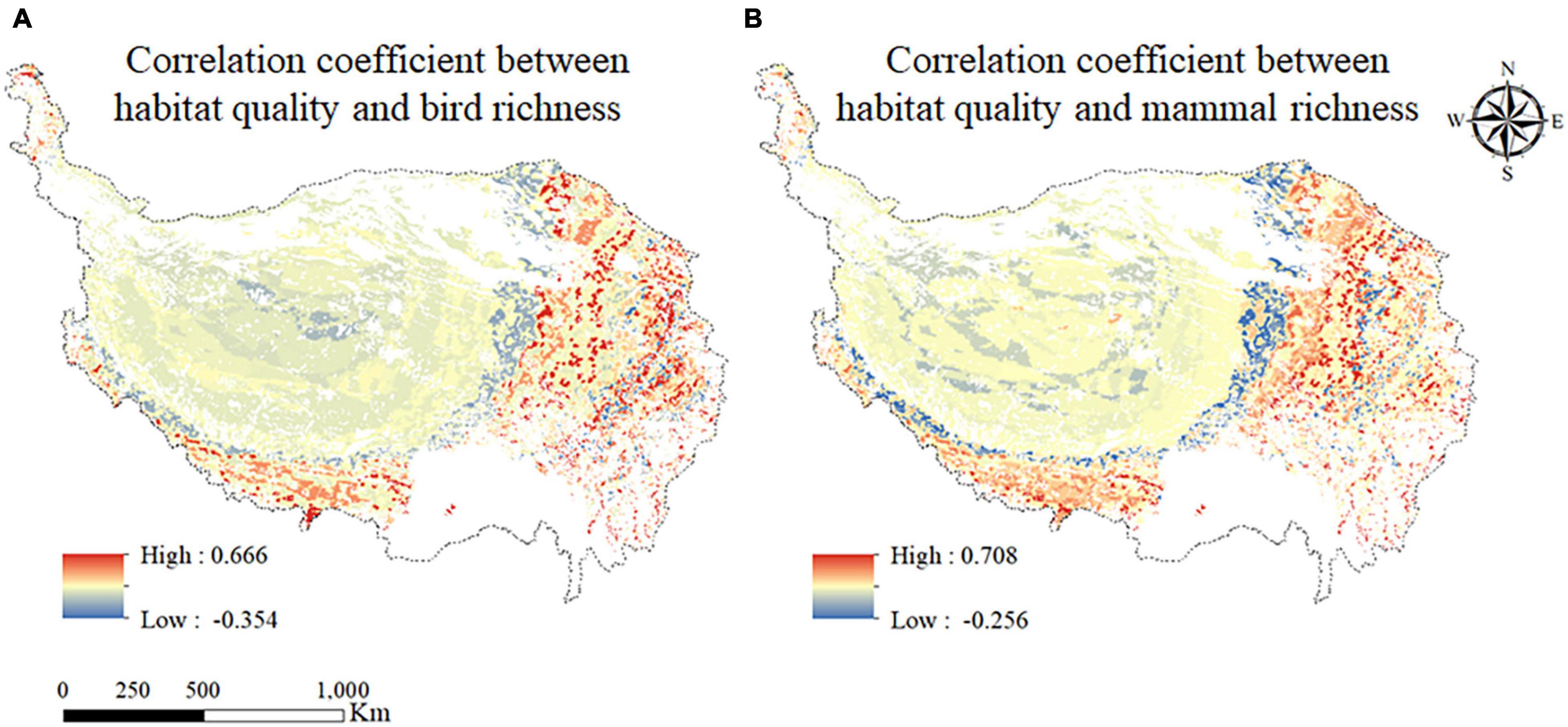
Figure 8. Correlation coefficient between habitat quality and bird species richness (A); and between habitat quality and mammal richness (B).
Therefore, it can be concluded that the relationship between habitat quality and animal biodiversity on the QTP was indeed complex. In general, the correlation relationships between habitat quality and bird species richness and between habitat quality and mammal richness were all higher in the eastern part of the region, but weaker in the central and western regions. The correlations were different among different grids. This result also proved that the selection of the index of animal biodiversity should be considered comprehensively in the study of animal biodiversity conservation on the QTP.
Response of Habitat Quality to Grazing Activities
In this study, we used the InVEST model to estimate the habitat quality on the QTP, and found that the habitat quality was generally good, and the areas with poor habitat quality were mainly distributed in the central part of Tibet, the northern part, and the northwestern part of the QTP. These conclusions were similar to the research of Sun et al. (2020), in 2015, the habitat quality in the northern and central parts of the QTP was poor (Sun et al., 2020b). The research of Li et al. (2018a, b) found that the eastern basin of the QTP and the central region of Tibet were more vulnerable to human disturbances (Li et al., 2018a,b). By overlay analysis of grassland types and habitat quality on the QTP, it was found that the areas with poor habitat quality in this study were consistent with areas with alpine desert on the QTP. According to the study of Li H. et al. (2019), it was found that the lower the vegetation was, the lower the habitat quality was (Li H. et al., 2019).
In this study, the geographical detector and GLMM were used to analyze the impact of grazing activities on habitat quality, the results showed that although the habitat quality in the southeast on the QTP was high, it was more sensitive to grazing activities. When grazing activities intensified, the habitat quality in this region would decline. In addition, the northern part of the QTP showed the same response to grazing activities. The independent effect of grazing activities on habitat quality was the largest in the eastern region. The interaction effects between grazing activities and slope were the main interaction. This conclusion was related to the study of Li L. et al. (2019) and Yang C. et al. (2021), in the southeastern part of the QTP, the vegetation was relatively lush and mostly forest (Li et al., 2019b; Yang C. et al., 2021). The forest ecosystem was the dominant ecosystem in the above regions. Since the restoration ability of the forest ecosystem was worse than that of the grassland and rational grazing capacity was relatively low (Jian et al., 2021). Therefore, the intensification of grazing activities would lead to the habitat quality degradation. The results of Dong et al. (2020) showed that grazing activities was the main external disturbance factors that led to habitat quality degradation in the central and northern parts of the QTP. However, moderate grazing activities in these areas did not have negative effect on habitat quality, but can moderately improve habitat quality (Dong et al., 2020). In recent years, as the state attached great importance to the ecological environment of the QTP, many measures have been carried out to benefit the ecological environment, such as pastureland rehabilitation, grazing exclusion and so on (Wu et al., 2017; Sun et al., 2019). According to the research of Su et al. (2020), because the grazing exclusion and rotation grazing policies have been implemented in most of the central and western regions of the QTP, the grazing intensity have decreased year by year, and the situation of overgrazing in most areas was gradually improving (Sun et al., 2020). Therefore, grazing activities had a positive impact on habitat quality in the above areas. However, the pressure of overgrazing still remained in the central and northern parts of the QTP for a long time (Liu et al., 2021). Hence, grazing activities had a negative impact on habitat quality in these regions.
Response of Animal Biodiversity to Grazing Activities
In this study, two indicators, bird species richness and mammal richness, were selected to represent animal biodiversity. The distribution characteristics of the two types of animals were consistent. The areas where grazing activities had the greatest impact on bird species richness were mainly in the alpine desert region and the southwestern part of the QTP, while the impact on mammal richness was mainly distributed in the southeastern part of the QTP. Both bird species richness and mammal richness were mainly affected by the interaction between grazing activities and slope. Different was that the area proportion of bird species richness affected by the interaction between grazing activities and temperature was much higher than that of mammal richness. Moreover, the areas where bird species richness were mainly affected by the interaction between temperature and grazing activities were mainly in the southwest, central, and northeast of the QTP, while the areas where mammal richness were mainly affected by the interaction between temperature and grazing activities were mainly in the northern part of Tibet. Based on GLMM, it was found that the grazing activities mainly had a negative impact on bird species richness, and the areas affected by the positive impact were mainly concentrated in the southeastern area of the QTP, while the grazing activities mainly had a negative impact on mammal richness, and the areas affected by the positive impact were mainly distributed in the middle of Tibet province and part of Qinghai province. There was a certain degree of uncertainty in bird species richness, so only there may be potential regularities on large scales.
Above conclusions were similar to the research of Li L. et al. (2018), it was found that areas with strong grazing activities exerted greater pressure on bird species richness, and the distribution of bird species richness on the QTP was related to the complexity of vegetation zones and the height of sea level (Li L. et al., 2018). However, according to the research results of Päckert et al. (2015) and Jian et al. (2021), slope had a more significant impact on bird species richness than other environmental factors (Päckert et al., 2015; Jian et al., 2021). According to the study of Zou et al. (2020), it was found that vegetation flourished in the southeastern part of the QTP, and the reasonable storage capacity was low (Zou et al., 2020). The density of mammal richness in this region was high, and once grazing was overdone, the grassland recovery time in this region would be longer than that in other areas, and the habitat of mammal richness would be destroyed, which would lead to the decrease of mammal richness (Wilson and Smith, 2015; Huang et al., 2020). Due to the particularity of the distribution of mammal richness, with the increase of the elevation on the QTP, temperature gradually decreased, and mammal richness were gradually affected by the interaction of elevation, temperature, and grazing activities (Zhang et al., 2016; Li X. et al., 2018). Studies have shown that the habitat selection of birds tends to focus on vegetation structure, vegetation coverage and other living conditions, and the higher the altitude is, the more suitable survival of birds (Jian et al., 2021). However, when it reaches a certain height, the sudden drop in temperature is not conducive to the habitat of birds, and the bird species richness will decrease. Compared with slope and altitude, the effects of temperature and precipitation are relatively small (Päckert et al., 2015). The results were relatively consistent with the study of Jian et al. (2021) and Päckert et al. (2015). According to the study of Liu (2004), the distribution of mammal richness was closely related to the quality of their habitats. The abundance of mammals decreased with the increase of the elevation gradient of 1,000 m and the decrease of air temperature by 0.6° (Liu, 2004). Moreover, with the increase of the average temperature, it showed significant fluctuations and tended to decrease. However, the response of mammal richness to precipitation increased first and then decreased with the increase of precipitation (Zhang et al., 2016).
The environment of the QTP is complex, and the effects of grazing activities on different bird species richness and mammal richness are different. However, the species and number of birds and mammal richness in some areas are not completely clear, so it is necessary to conduct research in depth in the future.
Limitations and Future Works
In this study, based on consideration of interaction effects between the factors and consideration of fixed or random effects of factors, we explored the responses of habitat quality and animal biodiversity to grazing activities. Thus, the results of the study can provide references for biodiversity conservation on the QTP. However, there were still some limitations in this study. First, in this study, we investigated the responses of habitat quality and animal biodiversity to grazing activities at 5 km grid scale. Studies have shown that there was a scale effect of human activities on habitat quality and animal biodiversity (Li et al., 2019a; Su et al., 2019). For the QTP, which was a large area with a more complex environment, the effects of grazing activities on habitat quality and animal biodiversity may vary greatly at different raster scales (Li et al., 2020). However, the responses of habitat quality and animal biodiversity to grazing activities at other scales was not considered in this study.
Second, although the main human activity on the QTP was grazing activities, only grazing activities was selected as the index of human activity in this study. However, previous studies showed that land use change (Wei H. et al., 2021), such as infrastructure construction and tourism (Ni et al., 2021), was also a part of human activities, which was not considered. In addition, in recent years, the government has implemented a series of ecological engineering measures to effectively restore the environment on the QTP, such as grazing exclusion (Sun et al., 2020). Some studies have shown that some counties on the northern Tibet were implementing a 10-years grazing exclusion in 2015 (Wu et al., 2017). However, some of the human activities described above were also major human activities other than grazing activities, but were not taken into account. Therefore, the consideration and selection of human factors were not sufficient and complete in this study.
Third, the two methods had their own advantages. Geographical detector could well consider the interaction effects between two factors, but the obtained results cannot quantitatively determine the impact of the interaction (Liu et al., 2021a). Moreover, there may be some interaction effects between various factors, which may also have impacts on biodiversity conservation. However, there may still be some problems in this study. Fewer factors affecting biodiversity conservation were selected, which may lead to certain errors in the random factors and fixed factors screened out by the model, thus leading to inaccurate results (Hubin and Storvik, 2018).
Finally, the habitat quality in this study was obtained by using the InVEST model based on a combination of the effects of various threat factors on different LULC types (Song et al., 2020). Although the Invest model was the main method in studies of habitat quality assessment, the parameters such as the weight of threat factors and habitat suitability were mainly obtained based on the existing literature or expert scoring method, which may be subjective (Andrus et al., 2021), and the ecological environment of the QTP was complex, and the parameters may vary from region to region. Not only that, since a large part of the QTP is a nature reserve or uninhabited area, it has certain particularity, and the distribution characteristics of various threat factors may be similar, so there may be some errors in the obtained results. In addition, this habitat quality did not refer to the habitat quality of specific organisms (Zhang H. et al., 2020), so the relationship between this habitat quality and animal biodiversity may not be significant.
Therefore, considering the above deficiencies, we will explore the impact of grazing activities on habitat quality and animal biodiversity at different scales, and combine with field research to make the results more accurate and reliable.
Conclusion
The responses of habitat quality and animal biodiversity and their interaction mechanisms were revealed spatially on the QTP in this study. Grazing activities lead to degradation of habitat quality and a sharp decline in biodiversity. In this study, based on the InVEST model, the habitat quality in grazing areas on the QTP was evaluated, and it was found that the overall habitat quality was good. The habitat quality level was mainly high, accounting for 76.43% of the area, and mainly distributed in the southern part. Then geographical detector method elucidated the independent effects of grazing activities and interaction effects between grazing activities and the factors on habitat quality and animal biodiversity. Finally, based on GLMM, considering the random effects and fixed effects of different grids, we found the relationship between grazing activities and habitat quality and animal biodiversity was mainly positive and negative, respectively. The regression coefficients distribution of grazing activities of habitat quality and animal biodiversity showed great spatial difference. The results showed that the areas where grazing activities had the greatest impact on habitat quality, bird species richness and mammal richness were mainly concentrated in the southeast, northeast, and southeast of the QTP, respectively.
The results of our study are of great significance for the study of responses of habitat quality and animal biodiversity to grazing activities and can provide effective guidance for biodiversity conservation on the QTP.
Data Availability Statement
The original contributions presented in the study are included in the article/supplementary material, further inquiries can be directed to the corresponding author.
Ethics Statement
Ethical review and approval was not required for the study on human participants in accordance with the local legislation and institutional requirements. The patients/participants provided their written informed consent to participate in this study. Ethical review and approval was not required for the animal study because the animal studies are based on animal biodiversity data, which is freely available on the website.
Author Contributions
SL and YL designed the research. FW, YS, ML, QW, and LY analyzed the data. All authors interpreted results and wrote the manuscript.
Funding
The work was funded by the second scientific expedition to the Qinghai-Tibet Plateau (No. 2019QZKK0405-05), National Natural Sciences Foundation of China (No. 41571173), and National Key Research and Development Project (No. 2016YFC0502103).
Conflict of Interest
The authors declare that the research was conducted in the absence of any commercial or financial relationships that could be construed as a potential conflict of interest.
References
Andrus, R. A., Martinez, A. J., Jones, G. M., and Meddens, A. J. H. (2021). Assessing the quality of fire refugia for wildlife habitat. For. Ecol. Manage. 482:118868. doi: 10.1016/j.foreco.2020.118868
Cao, J. J., Li, M. T., Deo, R. C., Adamowski, J. F., Cerda, A., Feng, Q., et al. (2018). Comparison of social-ecological resilience between two grassland management patterns driven by grassland land contract policy in the Maqu, Qinghai-Tibetan Plateau. Land Use Policy 74, 88–96. doi: 10.1016/j.landusepol.2017.07.027
Caro, C., Marques, J. C., Cunha, P. P., and Teixeira, Z. (2020). Ecosystem services as a resilience descriptor in habitat risk assessment using the InVEST model. Ecol. Indic. 115:106426. doi: 10.1016/j.ecolind.2020.106426
Chabuz, W., Kulik, M., Sawicka-Zugaj, W., Żółkiewski, P., Warda, M., Pluta, M., et al. (2019). Impact of the type of use of permanent grasslands areas in mountainous regions on the floristic diversity of habitats and animal welfare. Glob. Ecol. Conserv. 19:e00629. doi: 10.1016/j.gecco.2019.e00629
Chen, X., Zhang, T., Guo, R., Li, H., Zhang, R., Degen, A. A., et al. (2021). Fencing enclosure alters nitrogen distribution patterns and tradeoff strategies in an alpine meadow on the Qinghai-Tibetan Plateau. Catena 197:104948. doi: 10.1016/j.catena.2020.104948
de Lima Filho, J. A., Vieira, R. J. A. G., de Souza, C. A. M., Ferreira, F. F., and de Oliveira, V. M. (2021). Effects of habitat fragmentation on biodiversity patterns of ecosystems with resource competition. Phys. A Stat. Mech. Appl. 564:125497. doi: 10.1016/j.physa.2020.125497
Dong, S., Shang, Z., Gao, J., and Boone, R. B. (2020). Enhancing sustainability of grassland ecosystems through ecological restoration and grazing management in an era of climate change on Qinghai-Tibetan Plateau. Agric. Ecosyst. Environ. 287:106684. doi: 10.1016/j.agee.2019.106684
Edmonds, N. J., Al-Zaidan, A. S., Al-Sabah, A. A., Le Quesne, W. J. F., Devlin, M. J., Davison, P. I., et al. (2021). Kuwait’s marine biodiversity: qualitative assessment of indicator habitats and species. Mar. Pollut. Bull. 163:111915. doi: 10.1016/j.marpolbul.2020.111915
Evans, M. E. K., and Holsinger, K. E. (2012). Estimating covariation between vital rates: a simulation study of connected vs. separate generalized linear mixed models (GLMMs). Theor. Popul. Biol. 82, 299–306. doi: 10.1016/j.tpb.2012.02.003
Fan, Z., Duan, J., Lu, Y., Zou, W., and Lan, W. (2021). A geographical detector study on factors influencing urban park use in Nanjing, China. Urban For. Urban Green. 59:126996. doi: 10.1016/j.ufug.2021.126996
Gosselin, F., and Callois, J.-M. (2018). Relationships between human activity and biodiversity in Europe at the national scale: spatial density of human activity as a core driver of biodiversity erosion. Ecol. Indic. 90, 356–365. doi: 10.1016/j.ecolind.2018.03.010
Han, J., Wang, J., Chen, L., Xiang, J., Ling, Z., Li, Q., et al. (2021). Driving factors of desertification in Qaidam Basin, China: an 18-year analysis using the geographic detector model. Ecol. Indic. 124:107404. doi: 10.1016/j.ecolind.2021.107404
Huang, X., Zhang, J., Storozum, M., Liu, S., Gill, J. L., Xiang, L., et al. (2020). Long-term herbivore population dynamics in the northeastern Qinghai-Tibetan Plateau and its implications for early human impacts. Rev. Palaeobot. Palynol. 275:104171. doi: 10.1016/j.revpalbo.2020.104171
Hubin, A., and Storvik, G. (2018). Mode jumping MCMC for Bayesian variable selection in GLMM. Comput. Stat. Data Anal. 127, 281–297. doi: 10.1016/j.csda.2018.05.020
Jian, Y., Zhang, X., Li, X., Schou, C., Charalambidou, I., Ma, L., et al. (2021). Occurrence of Cryptosporidium and Giardia in wild birds from Qinghai Lake on the Qinghai-Tibetan Plateau, China. Parasitol. Res. 120, 615–628. doi: 10.1007/s00436-020-06993-w
Jiang, W., Lü, Y., Liu, Y., and Gao, W. (2020). Ecosystem service value of the Qinghai-Tibet Plateau significantly increased during 25 years. Ecosyst. Serv. 44:101146. doi: 10.1016/j.ecoser.2020.101146
Leal, A. I., Acácio, M., Meyer, C. F. J., Rainho, A., and Palmeirim, J. M. (2019). Grazing improves habitat suitability for many ground foraging birds in Mediterranean wooded grasslands. Agric. Ecosyst. Environ. 270−271, 1–8. doi: 10.1016/j.agee.2018.10.012
Li, H., Gao, J., Hu, Q., Li, Y., Tian, J., Liao, C., et al. (2019). Assessing revegetation effectiveness on an extremely degraded grassland, southern Qinghai-Tibetan Plateau, using terrestrial LiDAR and field data. Agric. Ecosyst. Environ. 282, 13–22. doi: 10.1016/j.agee.2019.05.013
Li, J. X., Jeske, D. R., and Klein, J. A. (2012). Sequential analysis methodology for a Poisson GLMM with applications to multicenter randomized clinical trials. J. Stat. Plan. Inference 142, 3225–3234. doi: 10.1016/j.jspi.2012.04.014
Li, L., Tietze, D. T., Fritz, A., Lü, Z., Bürgi, M., and Storch, I. (2018). Rewilding cultural landscape potentially puts both avian diversity and endemism at risk: a Tibetan Plateau case study. Biol. Conserv. 224, 75–86. doi: 10.1016/j.biocon.2018.05.008
Li, L., Zhang, Y., Wu, J., Li, S., Zhang, B., Zu, J., et al. (2019). Increasing sensitivity of alpine grasslands to climate variability along an elevational gradient on the Qinghai-Tibet Plateau. Sci. Total Environ. 678, 21–29. doi: 10.1016/j.scitotenv.2019.04.399
Li, M., Liang, D., Xia, J., Song, J., Cheng, D., Wu, J., et al. (2021). Evaluation of water conservation function of Danjiang River Basin in Qinling Mountains, China based on InVEST model. J. Environ. Manage. 286:112212. doi: 10.1016/j.jenvman.2021.112212
Li, S., Wu, J., Gong, J., and Li, S. (2018a). Human footprint in Tibet: assessing the spatial layout and effectiveness of nature reserves. Sci. Total Environ. 621, 18–29. doi: 10.1016/j.scitotenv.2017.11.216
Li, S., Zhang, H., Zhou, X., Yu, H., and Li, W. (2020). Enhancing protected areas for biodiversity and ecosystem services in the Qinghai-Tibet Plateau. Ecosyst. Serv. 43:101090. doi: 10.1016/j.ecoser.2020.101090
Li, S., Zhang, Y., Wang, Z., and Li, L. (2018b). Mapping human influence intensity in the Tibetan Plateau for conservation of ecological service functions. Ecosyst. Serv. 30, 276–286. doi: 10.1016/j.ecoser.2017.10.003
Li, X., Perry, G. L. W., and Brierley, G. J. (2018). A spatial simulation model to assess controls upon grassland degradation on the Qinghai-Tibet Plateau, China. Appl. Geogr. 98, 166–176. doi: 10.1016/j.apgeog.2018.07.003
Li, Y., Dong, S., Liu, S., Su, X., Wang, X., Zhang, Y., et al. (2019a). Relationships between plant diversity and biomass production of alpine grasslands are dependent on the spatial scale and the dimension of biodiversity. Ecol. Eng. 127, 375–382. doi: 10.1016/j.ecoleng.2018.12.015
Li, Y., Dong, S., Wen, L., Wang, X., and Wu, Y. (2013). The effects of fencing on carbon stocks in the degraded alpine grasslands of the Qinghai-Tibetan Plateau. J. Environ. Manage. 128, 393–399. doi: 10.1016/j.jenvman.2013.05.058
Li, Y., Li, J., Are, K. S., Huang, Z., Yu, H., and Zhang, Q. (2019b). Livestock grazing significantly accelerates soil erosion more than climate change in Qinghai-Tibet Plateau: evidenced from 137Cs and 210Pbex measurements. Agric. Ecosyst. Environ. 285:106643. doi: 10.1016/j.agee.2019.106643
Liu, C., Li, W., Xu, J., Wei, W., Xue, P., and Yan, H. (2021). Response of soil nutrients and stoichiometry to grazing management in alpine grassland on the Qinghai-Tibet Plateau. Soil Tillage Res. 206:104822. doi: 10.1016/j.still.2020.104822
Liu, S. H. (2004). Studies on the Species Diveristy of Mammals in Southern Hengduanshan: Spatial Patters of Species Richness of Mammals in Nature Reserves in Southern Yunnan, Ph.D.thesis. Zhejian: Zhejiang University.
Liu, X., Ma, Z., Huang, X., and Li, L. (2020). How does grazing exclusion influence plant productivity and community structure in alpine grasslands of the Qinghai-Tibetan Plateau? Glob. Ecol. Conserv. 23:e01066. doi: 10.1016/j.gecco.2020.e01066
Liu, Y., Chen, Y., Wu, Z., Wang, B., and Wang, S. (2021a). Geographical detector-based stratified regression kriging strategy for mapping soil organic carbon with high spatial heterogeneity. Catena 196:104953. doi: 10.1016/j.catena.2020.104953
Liu, Y., Liu, S., Sun, Y., Li, M., An, Y., and Shi, F. (2021b). Spatial differentiation of the NPP and NDVI and its influencing factors vary with grassland type on the Qinghai-Tibet Plateau. Environ. Monit. Assess. 193:48. doi: 10.1007/s10661-020-08824-y
Ma, S., Wang, L., Zhu, D., and Zhang, J. (2021). Spatiotemporal changes in ecosystem services in the conservation priorities of the southern hill and mountain belt, China. Ecol. Indic. 122:107225. doi: 10.1016/j.ecolind.2020.107225
Moore, E. K., Iason, G. R., Pemberton, J. M., Bryce, J., Dayton, N., Britton, A. J., et al. (2018). Habitat impact assessment detects spatially driven patterns of grazing impacts in habitat mosaics but overestimates damage. J. Nat. Conserv. 45, 20–29. doi: 10.1016/j.jnc.2018.07.005
Moreira, M., Fonseca, C., Vergílio, M., Calado, H., and Gil, A. (2018). Spatial assessment of habitat conservation status in a Macaronesian island based on the InVEST model: a case study of Pico Island (Azores, Portugal). Land Use Policy 78, 637–649. doi: 10.1016/j.landusepol.2018.07.015
Ni, J., Wu, T., Zhu, X., Wu, X., Pang, Q., Zou, D., et al. (2021). Risk assessment of potential thaw settlement hazard in the permafrost regions of Qinghai-Tibet Plateau. Sci. Total Environ. 776:145855. doi: 10.1016/j.scitotenv.2021.145855
Päckert, M., Martens, J., Sun, Y., and Tietze, D. T. (2015). Evolutionary history of passerine birds (Aves: Passeriformes) from the Qinghai-Tibetan plateau: from a pre-Quarternary perspective to an integrative biodiversity assessment. J. Ornithol. 156, 355–365. doi: 10.1007/s10336-015-1185-6
Qiao, P., Yang, S., Lei, M., Chen, T., and Dong, N. (2019). Quantitative analysis of the factors influencing spatial distribution of soil heavy metals based on geographical detector. Sci. Total Environ. 664, 392–413. doi: 10.1016/j.scitotenv.2019.01.310
Roche, D. V., Cardilini, A. P. A., Lees, D., Maguire, G. S., Dann, P., Sherman, C. D. H., et al. (2016). Human residential status and habitat quality affect the likelihood but not the success of lapwing breeding in an urban matrix. Sci. Total Environ. 556, 189–195. doi: 10.1016/j.scitotenv.2016.03.008
Shaffer, J. A., Roth, C. L., and Mushet, D. M. (2019). Modeling effects of crop production, energy development and conservation-grassland loss on avian habitat. PloS One 14:e0198382. doi: 10.1371/journal.pone.0198382
Shi, J., Li, X., Dong, S., Zhuge, H., and Mu, Y. (2018). Trans-boundary conservation of Chiru by identifying its potential movement corridors in the alpine desert of Qinghai-Tibetan Plateau. Glob. Ecol. Conserv. 16:e00491. doi: 10.1016/j.gecco.2018.e00491
Song, S., Liu, Z., He, C., and Lu, W. (2020). Evaluating the effects of urban expansion on natural habitat quality by coupling localized shared socioeconomic pathways and the land use scenario dynamics-urban model. Ecol. Indic. 112:106071. doi: 10.1016/j.ecolind.2020.106071
Su, X., Han, W., Liu, G., Zhang, Y., and Lu, H. (2019). Substantial gaps between the protection of biodiversity hotspots in alpine grasslands and the effectiveness of protected areas on the Qinghai-Tibetan Plateau, China. Agric. Ecosyst. Environ. 278, 15–23. doi: 10.1016/j.agee.2019.03.013
Su, X., Liu, Y., Zhou, W., and Liu, G. (2020). “An overview on status and importance of Tibetan Plateau steppe, China,” in Reference Module in Earth Systems and Environmental Sciences, (Amsterdam: Elsevier). doi: 10.1016/B978-0-12-821139-7.00006-4
Sun, J., Hou, G., Liu, M., Fu, G., Zhan, T., Zhou, H., et al. (2019). Effects of climatic and grazing changes on desertification of alpine grasslands, Northern Tibet. Ecol. Indic. 107:105647. doi: 10.1016/j.ecolind.2019.105647
Sun, J., Liu, M., Fu, B., Kemp, D., Zhao, W., Liu, G., et al. (2020). Reconsidering the efficiency of grazing exclusion using fences on the Tibetan Plateau. Sci. Bull. 65, 1405–1414. doi: 10.1016/j.scib.2020.04.035
Sun, Y., Liu, S., Liu, Y., Dong, Y., Li, M., Sun, Y. X., et al. (2020a). Grazing intensity and human activity intensity datasets on the Qinghai-Tibet Plateau during 1990-2015. Sci. Data Bank doi: 10.11922/sciencedb.00171
Sun, Y., Liu, S., Shi, F., An, Y., Li, M., and Liu, Y. (2020b). Spatio-temporal variations and coupling of human activity intensity and ecosystem services based on the four-quadrant model on the Qinghai-Tibet Plateau. Sci. Total Environ. 743:140721. doi: 10.1016/j.scitotenv.2020.140721
Tang, F., Fu, M., Wang, L., and Zhang, P. (2020). Land-use change in Changli County, China: predicting its spatio-temporal evolution in habitat quality. Ecol. Indic. 117:106719. doi: 10.1016/j.ecolind.2020.106719
Venables, W. N., and Dichmont, C. M. (2004). GLMs, GAMs and GLMMs: an overview of theory for applications in fisheries research. Fish. Res. 70, 319–337. doi: 10.1016/j.fishres.2004.08.011
Wang, D., Li, X., Zou, D., Wu, T., Xu, H., Hu, G., et al. (2020). Modeling soil organic carbon spatial distribution for a complex terrain based on geographically weighted regression in the eastern Qinghai-Tibetan Plateau. Catena 187:104399. doi: 10.1016/j.catena.2019.104399
Wang, J., Li, X., Christakos, G., Liao, Y., Zhang, T., Gu, X., et al. (2010). Geographical detectors-based health risk assessment and its application in the neural tube defects study of the Heshun Region, China. Int. J. Geogr. Inf. Sci. 24, 107–127. doi: 10.1080/13658810802443457
Wang, Y., Ren, Z., Ma, P., Wang, Z., Niu, D., Fu, H., et al. (2020). Effects of grassland degradation on ecological stoichiometry of soil ecosystems on the Qinghai-Tibet Plateau. Sci. Total Environ. 722:137910. doi: 10.1016/j.scitotenv.2020.137910
Wei, H., Duan, R., Xu, Q., Yang, S., Fan, Q., Hou, G., et al. (2021). Fungal spore indicators of vegetation and highland pastoralism in modern topsoil and dung, eastern Tibetan Plateau. Catena 202:105231. doi: 10.1016/j.catena.2021.105231
Wei, Y., Zhang, L., Wang, J., Wang, W., Niyati, N., Guo, Y., et al. (2021). Chinese caterpillar fungus (Ophiocordyceps sinensis) in China: current distribution, trading, and futures under climate change and overexploitation. Sci. Total Environ. 755:142548. doi: 10.1016/j.scitotenv.2020.142548
Wilson, M. C., and Smith, A. T. (2015). The pika and the watershed: the impact of small mammal poisoning on the ecohydrology of the Qinghai-Tibetan Plateau. Ambio 44, 16–22. doi: 10.1007/s13280-014-0568-x
Wu, J., Feng, Y., Zhang, X., Wurst, S., Tietjen, B., Tarolli, P., et al. (2017). Grazing exclusion by fencing non-linearly restored the degraded alpine grasslands on the Tibetan Plateau. Sci. Rep. 7:15202. doi: 10.1038/s41598-017-15530-2
Yang, C., Tsedan, G., Fan, Q., Wang, S., Wang, Z., Chang, S., et al. (2021). Behavioral patterns of yaks (Bos grunniens) grazing on alpine shrub meadows of the Qinghai-Tibetan Plateau. Appl. Anim. Behav. Sci. 234:105182. doi: 10.1016/j.applanim.2020.105182
Yang, H., Huang, Q., Zhang, J., Songer, M., and Liu, J. (2021). Range-wide assessment of the impact of China’s nature reserves on giant panda habitat quality. Sci. Total Environ. 769:145081. doi: 10.1016/j.scitotenv.2021.145081
Yang, Y., Nan, Y., Liu, Z., Zhang, D., and Sun, Y. (2020). Direct and indirect losses of natural habitat caused by future urban expansion in the transnational area of Changbai Mountain. Sustain. Cities Soc. 63:102487. doi: 10.1016/j.scs.2020.102487
Yao, X., Wu, J., Gong, X., Lang, X., Wang, C., Song, S., et al. (2019). Effects of long term fencing on biomass, coverage, density, biodiversity and nutritional values of vegetation community in an alpine meadow of the Qinghai-Tibet Plateau. Ecol. Eng. 130, 80–93. doi: 10.1016/j.ecoleng.2019.01.016
Yohannes, H., Soromessa, T., Argaw, M., and Dewan, A. (2021). Spatio-temporal changes in habitat quality and linkage with landscape characteristics in the Beressa watershed, Blue Nile basin of Ethiopian highlands. J. Environ. Manage. 281:111885. doi: 10.1016/j.jenvman.2020.111885
Zhan, Q., Zhao, W., Yang, M., and Xiong, D. (2021). A long-term record (1995–2019) of the dynamics of land desertification in the middle reaches of Yarlung Zangbo River basin derived from Landsat data. Geogr. Sustain. 2, 12–21. doi: 10.1016/j.geosus.2021.01.002
Zhang, H., Zhang, C., Hu, T., Zhang, M., Ren, X., and Hou, L. (2020). Exploration of roadway factors and habitat quality using InVEST. Trans. Res. D Trans. Environ. 87:102551. doi: 10.1016/j.trd.2020.102551
Zhang, X., Song, W., Lang, Y., Feng, X., Yuan, Q., and Wang, J. (2020). Land use changes in the coastal zone of China’s Hebei Province and the corresponding impacts on habitat quality. Land Use Policy 99:104957. doi: 10.1016/j.landusepol.2020.104957
Zhang, X., Wang, J., Gao, Y., and Wang, L. (2021). Variations and controlling factors of vegetation dynamics on the Qingzang Plateau of China over the recent 20 years. Geogr. Sustain. 2, 74–85. doi: 10.1016/j.geosus.2021.02.001
Zhang, Y., Zhao, R., Liu, Y., Huang, K., and Zhu, J. (2021). Sustainable wildlife protection on the Qingzang Plateau. Geogr. Sustain. 2, 40–47. doi: 10.1016/j.geosus.2021.02.005
Zhang, Z., Xu, D., Wang, L., Hao, J., Wang, J., Zhou, X., et al. (2016). Convergent evolution of rumen microbiomes in high-altitude mammals. Curr. Biol. 26, 1873–1879. doi: 10.1016/j.cub.2016.05.012
Zhao, J., and Li, C. (2020). Investigating spatiotemporal dynamics and trade-off/synergy of multiple ecosystem services in response to land cover change: a case study of Nanjing city, China. Environ. Monit. Assess. 192:701. doi: 10.1007/s10661-020-08663-x
Zhao, Y., Chen, D., and Fan, J. (2020a). Sustainable development problems and countermeasures: a case study of the Qinghai-Tibet Plateau. Geogr. Sustain. 1, 275–283. doi: 10.1016/j.geosus.2020.11.002
Zhao, Y., Deng, Q., Lin, Q., Zeng, C., and Zhong, C. (2020b). Cadmium source identification in soils and high-risk regions predicted by geographical detector method. Environ. Pollut. 263:114338. doi: 10.1016/j.envpol.2020.114338
Zhu, C., Zhang, X., Zhou, M., He, S., Gan, M., Yang, L., et al. (2020). Impacts of urbanization and landscape pattern on habitat quality using OLS and GWR models in Hangzhou, China. Ecol. Indic. 117:106654. doi: 10.1016/j.ecolind.2020.106654
Zhu, Z., Li, Y., Li, K., Cheng, B., Yang, S., Liu, Q., et al. (2020). Study of quality maintenance of fish habitats in small- and medium-sized mountain rivers with low flow rate. Ecol. Eng. 147:105780. doi: 10.1016/j.ecoleng.2020.105780
Keywords: grazing intensity, biodiversity conservation, interaction relationship, random effect, fixed effect
Citation: Liu Y, Liu S, Wang F, Sun Y, Li M, Wang Q and Yu L (2021) Responses of Habitat Quality and Animal Biodiversity to Grazing Activities on the Qinghai-Tibet Plateau. Front. Ecol. Evol. 9:681775. doi: 10.3389/fevo.2021.681775
Received: 17 March 2021; Accepted: 10 May 2021;
Published: 02 June 2021.
Edited by:
Jian Sun, Institute of Tibetan Plateau Research (CAS), ChinaReviewed by:
Dongliang Luo, Northwest Institute of Eco-Environment and Resources, Chinese Academy of Sciences (CAS), ChinaJunbang Wang, Institute of Geographic Sciences and Natural Resources Research, Chinese Academy of Sciences, China
Minghao Zhuang, China Agricultural University, China
Copyright © 2021 Liu, Liu, Wang, Sun, Li, Wang and Yu. This is an open-access article distributed under the terms of the Creative Commons Attribution License (CC BY). The use, distribution or reproduction in other forums is permitted, provided the original author(s) and the copyright owner(s) are credited and that the original publication in this journal is cited, in accordance with accepted academic practice. No use, distribution or reproduction is permitted which does not comply with these terms.
*Correspondence: Shiliang Liu, c2hpbGlhbmdsaXVAYm51LmVkdS5jbg==