- 1Department of Integrated Mathematical Oncology, Moffitt Cancer Center and Research Institute, Tampa, FL, United States
- 2Department of Medical Engineering, College of Engineering, University of South Florida, Tampa, FL, United States
- 3Dynamic Game Theory Team, Department of Data Science and Knowledge Engineering, Maastricht University, Maastricht, Netherlands
- 4Department of Integrative Biology, University of South Florida, Tampa, FL, United States
- 5Plant Evolutionary Ecology Group, University of Tübingen, Tübingen, Germany
- 6Department of Biological Sciences, University of Illinois at Chicago, Chicago, IL, United States
- 7Delft Institute of Applied Mathematics, Delft University of Technology, Delft, Netherlands
Rapid evolution is ubiquitous in nature. We briefly review some of this quite broadly, particularly in the context of response to anthropogenic disturbances. Nowhere is this more evident, replicated and accessible to study than in cancer. Curiously cancer has been late - relative to fisheries, antibiotic resistance, pest management and evolution in human dominated landscapes - in recognizing the need for evolutionarily informed management strategies. The speed of evolution matters. Here, we employ game-theoretic modeling to compare time to progression with continuous maximum tolerable dose to that of adaptive therapy where treatment is discontinued when the population of cancer cells gets below half of its initial size and re-administered when the cancer cells recover, forming cycles with and without treatment. We show that the success of adaptive therapy relative to continuous maximum tolerable dose therapy is much higher if the population of cancer cells is defined by two cell types (sensitive vs. resistant in a polymorphic population). Additionally, the relative increase in time to progression increases with the speed of evolution. These results hold with and without a cost of resistance in cancer cells. On the other hand, treatment-induced resistance can be modeled as a quantitative trait in a monomorphic population of cancer cells. In that case, when evolution is rapid, there is no advantage to adaptive therapy. Initial responses to therapy are blunted by the cancer cells evolving too quickly. Our study emphasizes how cancer provides a unique system for studying rapid evolutionary changes within tumor ecosystems in response to human interventions; and allows us to contrast and compare this system to other human managed or dominated systems in nature.
1. Introduction
Organisms can respond rapidly to contingencies and changes in their environment. When they cannot extinction may follow. The Stephens Island wren was flightless and free of mammalian predators until the lighthouse keeper introduced Tibbles the house cat (Galbreath and Brown, 2004; Medway, 2004). Extinction of the wren followed shortly thereafter. The birds either did not or could not muster behavioral responses, and did not have the time needed to evolve appropriate responses. Similarly, the introduction of brown tree snakes (Boiga irregularis) has threatened a number of birds, bats and reptiles on islands such as Guam leading to dramatic losses of species diversity (Savidge, 1987; Fritts and Rodda, 1998; Wiles et al., 2003). On the other hand, many species respond quickly to dramatic changes in their environment or even colonize novel environments. Examples include responses to size selective harvesting of fish (Conover and Munch, 2002; Salvioli et al., 2021); re-emergence of anti-predator behaviors with the reintroduction of predators (Laundré et al., 2001); rapid evolution of body size, behavior and other traits in invasive organisms (Huey et al., 2000; Whitney and Gabler, 2008; Turner et al., 2014; Vandepitte et al., 2014; Rollins et al., 2015; Selechnik et al., 2019) or members of invaded communities (Chapuis et al., 2017); and shifts in reproduction and migration in animals and plants in response to climate (Parmesan et al., 1999; Franks et al., 2007; Geerts et al., 2015).
Rapid evolution may permit species to adjust to rapid changes in their environments, but rapid evolution can also be consequential to human welfare and health. For example, herbicide and pesticide resistance threatens the productivity of crops (Kuester et al., 2014; Baucom, 2019; Hawkins et al., 2019). The boll weevil (Anthonomus grandis Boheman) was reported as a serious pest in U.S. cotton production as far back as 1892 and developed resistance to insecticides within a few years in the 1950's (Perkins, 1980). Today, boll weevil control involves an integrated pest management (IPM) approach using pheromone traps and insecticides timed around the weevil's reproductive cycles (Shipman, 2017). For upwards of 50 years now, IPM strategies have employed resistance management plans which can include application of targeted pheromones and allelochemicals, leading to a reduction in the use of broad-spectrum insecticides and ensuring that non-target and beneficial insects are not adversely affected (Tewari et al., 2014; Brown and Staňková, 2017; Cunningham, 2019). Similarly, drug resistance poses direct threats to patient health. Chloroquine resistant strains of malaria have become particularly prevalent in West Africa and Papua New Guinea (Wellems and Plowe, 2001). Antibiotic resistance threatens the advances that have been made in controlling infectious diseases. The emergence of methicillin-resistant Staphylococcus aureus (MRSA) strains of pathogenic bacteria have been the subject of epidemiological, experimental and mathematical-modeling studies (Robinson and Enright, 2003).
While the idea that cancer progression is an evolutionary process has been discussed for several decades (Cairns, 1975; Nowell, 1976), the application of ecological and evolutionary principles to understanding rapid evolution in cancer has only recently become a major objective [e.g., the classic by Nowell (1976) has been cited over 7,000 times in Google Scholar; more than half of that in the last 10 years]. Cancers provide a unique study of rapid evolution because within a matter of months or years, cancer within its host will evolve adaptations for evading the immune system, increasing vasculature, co-opting the signaling pathways of normal cells, and gathering scarce nutrients more quickly and efficiently (Hanahan and Weinberg, 2000, 2011). This trajectory of extremely rapid evolution begins de novo in each patient. In addition to the rapid evolution of cancer in the host, resistance to therapy can also evolve quickly (Dujon et al., 2020; Gatenby and Brown, 2020a). When metastatic, like the cotton boll weevil, the cancer will evolve resistance to all available drugs. Cancer then represents a microcosm for studying and managing rapid evolution that is replicated across patients (Pienta et al., 2020).
There are some important differences between studying rapid evolution in ecosystems compared to in human disease, and cancer in particular. Evolutionary speed in wild populations, for instance, can be sensitive to sex ratios that determine effective population sizes (Allendorf et al., 2008), an issue that does not apply to asexual reproduction through mitotic cell division. For antibiotic resistance, the fear is not that the current patient will succumb because of rapid evolution, but that the application of antibiotics across millions of patients will result in a strain emerging from a subset of these patients that will go on to infect others (Ventola, 2015). In contrast, the concern in cancer is not that a resistant strain will jump from patient to patient. Instead, the problem lies entirely within the patient and the eco-evolutionary dynamics that lead to therapy failure.
Here, we are interested in addressing and modeling the consequences of two features of eco-evolutionary models of adaptive therapy (AT). These features are the speed of evolution, and whether the cancer cell population is monomorphic (where treatment-induced resistance evolves as a quantitative trait) vs. polymorphic (sensitive vs. resistant cell types where treatment-induced resistance evolves only in resistant cells, also as a quantitative trait). In what follows, we elaborate more fully on the determinants of evolutionary speed (section 2) and the broader contexts of rapid evolution (section 3). In section 4, we introduce therapeutic strategies in cancer as a special form of integrated pest management. We then develop a model of therapy that includes the ecological dynamics of tumor burden and the evolutionary dynamics of changes in the composition of cancer cell types within the patient. We analyze the consequences of evolutionary speed in determining the efficacy of a standard form of AT relative to continuous drug delivery at maximum tolerable dose (MTD) (section 5). We model this in the context of monomorphic and polymorphic cancer cell populations, and in the context of having no cost of resistance, a cost of resistance manifested in intrinsic growth rates, and a cost of resistance manifested in the carrying capacities. Section 6 concludes by summarizing the main outcomes of the cancer model and discussing how our results could be transferable to other fields. In addition to adding to the modeling results for AT in cancers, we hope to show evolutionary biologists and ecologists just how similar resistance management in cancer is to managing evolving species (that may be pests or resources), and to show cancer biologists how the challenge of therapy resistance is kindred to conservators and managers of biodiversity and pests in nature.
2. Determinants of Evolutionary Speed
A better understanding of the evolution of resistance to therapy in cancer can be informed by theory and examples of rapid evolution in several ecological contexts. Evolution occurs over many timescales. The domain of evolutionary science classically has involved taxa with vertical inheritance, so discussions of “rapid” evolution by many scholars emphasize the surprisingly small number of generations over which substantial changes in heritable phenotypes are observed. Hairston et al. (2005) defined rapid evolution as “genetic change occurring rapidly enough to have a measurable impact on simultaneous ecological change.” While the authors emphasize the change must be genetic, their analysis is actually based on heritable phenotypic change which could result from genetic, epigenetic or other forms of non-genetic inheritance (Bonduriansky and Day, 2009; Jablonka and Raz, 2009; Keller, 2014; Müller, 2017; Stoltzfus, 2017; Banta and Richards, 2018; Richards and Pigliucci, 2020; Mounger et al., 2021). Evolution is known to occur rapidly in wild populations abruptly subjected to novel selection pressures. Rapid evolution is well-documented in invasive populations (Bock et al., 2015; van Kleunen et al., 2018; Mounger et al., 2021), and wild populations experiencing intensive human intervention related to urbanization, agro-ecosystem management, wild species harvest, and pollution (Sullivan et al., 2017).
Researchers have long been interested in the mechanisms that allow for these rapid responses to environmental challenges. The frequencies of heritable phenotypes in wild populations may change within only a few generations when novel environmental conditions are highly lethal to some portion of existing trait variation. Sudden ecological and climatic changes are particularly effective at driving rapid phenotypic change and underlying change in genetic and non-genetic inheritance mechanisms. In response to climate change in the UK, for instance, some populations of the brown argus butterfly (Aricia agestis) have shifted female preference for host plant species, and exhibited reduced fitness in ancestral habitats within 10–15 years of the shift (Buckley and Bridle, 2014). Plenty of evidence suggests that these responses can be in part dictated by classic expectations of selection acting on genetic diversity (Hoffmann and Sgro, 2011). The type of intense selection that induces rapid evolutionary change, however, may be accompanied by a loss in genetic diversity and heritable variation.
On the other hand, many invasive species offer important counter evidence to the assumption that reduced genetic variance indicates reduced evolutionary potential (Colautti and Lau, 2015; Dlugosch et al., 2015; Stapley et al., 2015; Estoup et al., 2016; Selechnik et al., 2019). The population bottlenecks inherent to invasion have long been assumed to hinder evolutionary potential creating the “genetic paradox” of invasion (Estoup et al., 2016; Mounger et al., 2021), but recent studies have shown that in fact the genetic paradox may not be as severe as initially thought. This is due to a myriad of genomic possibilities. First, many invasive populations undergo only modest reductions in genetic variation due to multiple introductions, hybridization or de novo mutations (Estoup et al., 2016). But importantly, loss of genetic diversity measured by molecular markers does not reflect loss of quantitative trait variation or may reflect selection of fit genotypes or recombination among founding genotypes (e.g., Selechnik et al., 2019). Genetic bottlenecks can also contribute to performance by purging deleterious alleles, revealing beneficial cryptic variation or creating new beneficial interactions among genomic elements (Colautti and Lau, 2015; Dlugosch et al., 2015; Stapley et al., 2015; Estoup et al., 2016; van Kleunen et al., 2018).
In addition to genetic variants, the plasticity of morphological, physiological and behavioral traits are clearly important (West-Eberhard, 1989; Richards et al., 2006; Lankau, 2011; Ledón-Rettig et al., 2013; Rollins et al., 2015). Theoretical work suggests putative upper limits on rates of genetic evolution, and that rapid trait changes result in part from phenotypic plasticity (Kopp and Matuszewski, 2014). The distinction is complex, since phenotypic plasticity is genetically based but also underlain by epigenetic mechanisms that can be independent of genetic differences (Richards et al., 2006, 2010, 2017; Cortijo et al., 2014; Banta and Richards, 2018). Furthermore, the molecular-level mechanisms that contribute to such plastic responses can ultimately lead to genetic changes or non-genetic inheritance (West-Eberhard, 1989; Bonduriansky and Day, 2009, 2018; Klironomos et al., 2013; Kronholm and Collins, 2016; Kronholm et al., 2017; Wölfl et al., 2020). A particularly striking example of the disconnect between genetic variation and heritable phenotypic response is in the single octoploid clone of Japanese knotweed that has spread aggressively through a broad range of habitats in temperate Europe and North America (Beerling et al., 1994; Bailey and Conolly, 2000; Grimsby et al., 2007; Gerber et al., 2008; Bailey et al., 2009; Richards et al., 2012; Zhang et al., 2016). Several studies have linked the divergence in these populations to differences in DNA methylation (Richards et al., 2008, 2012; Zhang et al., 2016). Despite the potential importance of this type of clonal spread particularly in invasive plant species, our ability to understand the roles for existing mutations, de novo mutations, and epigenetics remains constrained by too few studies (Paun et al., 2019; Richards and Pigliucci, 2020; Mounger et al., 2021).
Extensive genomics studies in cancer have revealed that “genetic instability” is a hallmark of cancer (Coffey, 1998; Duesberg et al., 1998). While no universal driver mutations of metastases have been identified, Gerstung et al. (2020) demonstrated by analysis of 2,658 samples of 38 different cancers types in the Pan-Cancer Analysis of Whole Genomes (PCAWG) that very early events in cancer are limited to a common set of drivers. In fact, 50% of early mutations in cancers occur in just 9 genes. Mutations in epigenetic machinery can also be important in shaping genome dynamics in cancer (Feinberg et al., 2006; Timp and Feinberg, 2013). In particular, chromatin regulators are often mutated in cancer. Mutations in the SWI/SNF complex occur in over 20% of all cancers (Kadoch and Crabtree, 2015). Recent studies indicate that specific genetic mutations can instigate metastases but that completion of the process depends only on non-genetic changes, specifically epigenetic changes that complement the genetic mutations (Lambert et al., 2017).
The molecular basis of trait variation can have important impacts on the speed of evolution as evidenced by studies in herbicide and pesticide resistance. Hawkins et al. (2019) recently compared three major pesticide groups (insecticides, herbicides, and fungicides) to make this point. They argued that fungicide resistance evolves more often by de novo point mutations in functional genes, herbicide resistance evolves through selection on standing variation; and insecticide resistance evolves through a combination of standing variation and de novo mutations. The rate at which resistance evolves in these groups depends on the dynamics within the populations. They argue that de novo mutation must spread through the movement of insects, seeds, pollen, or spores, whereas a preexisting allele may already be present throughout the range. These arguments suggest that the pathway to resistance can determine the most effective containment strategy (Hawkins et al., 2019).
3. Contexts of Rapid Evolution
Humans are selective agents that influence the evolution of non-human wild populations by harvesting them, attempting to suppress or extirpate them, and by altering their biophysical environment (Hendry et al., 2017). These anthropogenic interventions often induce trait changes more rapidly and to a greater extent than observed in the evolution of populations inhabiting more natural contexts (Hendry et al., 2008), although even natural populations can undergo rapid evolution in real time (Weiner, 1995; Reznick et al., 2019). Human interventions drive evolution in traits such as body size in harvested fish (Olsen et al., 2004; Salvioli et al., 2021), and dispersal traits of urban plants (Cheptou et al., 2008). Accelerated rates of evolution may result from shifts in the adaptive landscapes resulting from anthropogenic changes or from increased variance in relative fitness among individuals (Fugère and Hendry, 2018). Human-induced evolutionary change may render populations resistant to future management and may endow populations with functional traits that feed back to affect the properties of ecosystems that benefit people (Rudman et al., 2017). This rapid evolution of management-resistant traits, and feedbacks on the health of ecosystems, are analogous to the management of therapy resistance, tumor burden, and patient well-being in oncological settings. Like the clinician and cancer, the practitioner/manager (e.g., in an agroecosystem or fishery) and non-human population (e.g., of weeds or fish) coevolve, one through rational decision making and one through selection (Staňková et al., 2019; Salvioli et al., 2021). Next, we briefly highlight some principles developed through the study of rapid evolution in anthropogenic contexts of urbanization, weed management in agrosystems, and wild animal harvest.
3.1. Urbanization
Urban evolutionary ecology and adaptive cancer therapies share a common interest in populations responding to large magnitude environmental changes. Urbanization is characterized by a host of changes in the biophysical environment, including accelerated cycling of nutrients and pollutants, altered energy budgets that induce warming via heat island effects, landscape fragmentation and the proliferation of impervious surface, modified soil structure and fertility, redistributions of water, changes to physical architecture, and homogenization of ecological communities and the attendant introduction of novel competitor, predator, and pathogen species (Grimm et al., 2008). Populations of plants, animals, and microorganisms experience this wide portfolio of changes under non-equilibrium conditions, as urbanization generates perpetual changes in environments rather than stable endpoints (Collins et al., 2000). Cancer cell populations, likewise, experience dramatic environmental change either when therapies are imposed on extant tumors, or during metastasis as cells migrate to distinct areas of the body. Like the environmental changes that constitute urbanization, adaptive therapies seek to impose non-equilibrium selection regimes on cancer populations to disrupt the emergence, or dominance of resistant cancer cells.
Urban populations can exhibit sufficient trait variation for rapid evolution. For instance, variation in plant size and allocation traits among urban plant species often exceeds that of their non-urban conspecifics (Borowy and Swan, 2020). Urban environmental conditions alter phenotype frequencies in non-human wild populations by inducing plastic responses, as well as through both adaptive and non-adaptive evolutionary changes (Johnson and Munshi-South, 2017). For instance, acorn ants (Temnothorax curvispinosus) reared from urban populations inhabiting environments warmed 2°C by the heat island effect show higher heat tolerance and narrower thermal tolerance breadths than ants reared from rural populations. Yet, even rural ants can develop higher heat tolerance through acclimation, indicating both fixed and plastic phenotypic responses to the urban thermal environment (Diamond et al., 2017).
Landscape fragmentation in cities can isolate small populations, reducing gene flow and promoting genetic drift, resulting in potentially non-adaptive genetic differentiation among populations. Transcriptome differences among distinct urban populations of white-footed mice (Peromyscus leucopus) occupying isolated habitat patches in New York, for instance, suggest that both selection and genetic drift account for rapid evolutionary responses to urbanization (Harris et al., 2013). Populations inhabiting urban environments can exhibit adaptive changes in sexually selected traits when compared with their conspecific rural counterparts (Yeh, 2004). Important questions remain about the extent to which urban environmental properties induce mutation or affect genome-wide mutation rates, and whether adaptation to urban environments results more often from mutations that occur after populations are urbanized or from standing, pre-urban genetic variation (Barrett and Schluter, 2008). These pressing questions for non-human populations adapting to urbanization are similarly relevant for cancer cell populations evolving responses to diverse tumor microenvironments and therapy-induced selection.
Urban evolutionary ecology investigates not only phenotypic responses of populations to urban environmental conditions, but also examines how these altered populations and their traits affect urban ecosystem processes (Alberti, 2016). Ecosystems carry out processes such as primary production of biomass and organic energy, decomposition and nutrient recycling, hosting of biodiversity, and societally valued services like storm energy mitigation, food production, pollutant capture, and recreation. The degree and manner in which ecosystems carry out these processes depend strongly on the functional traits of an ecosystem's constituent species (Rudman et al., 2017). These traits are the products of evolution occurring under the selective regime imposed by ecosystem processes, setting up reciprocal eco-evolutionary feedbacks between population traits and the environment in which those traits emerged. Similarly, through mechanisms such as promoting vascularization, acidic pH, and cancer associated fibroblasts, cancer cells evolve traits that alter their environment creating eco-evolutionary feedbacks (De Groot et al., 2017).
3.2. Agroecosystem Weed Management
Tumor cells and agricultural pests both form undesirable populations that humans attempt to eradicate or manage through the application of biocides. Parallels to cancer therapies are arguably most evident in the battle against weed plants and other pests in agroecosystems. Pesticides and antibiotics, among other agricultural technologies, have afforded increases in food supply necessary for a growing and urbanizing human population. But, the intensive application of these chemicals select for resistance in weeds, insect pests, and crop and livestock pathogens (Pittendrigh et al., 2013; Kuester et al., 2014; Baucom, 2019). One strategy developed in cropping systems to inhibit rapid evolution of resistance in weeds is the application of herbicide mixtures (Wrubel and Gressel, 1994). This mixture approach is distinct from the sequential use of multiple herbicides, one at a time, until each has selected for resistance in the focal weed population. It is also distinct from the application of multiple herbicides that each target a separate weed species. Instead, this mixture approach consists of simultaneously applying multiple herbicides with different modes of action to control a single weed population. Similar approaches using multi-drug cocktails have become commonplace in cancer treatments. Toxicity to patients often dictates the doses and combinations of drugs that can be safely administered.
Theory and experience reveal several criteria for delaying the evolution of herbicide resistance in weeds (Wrubel and Gressel, 1994), with parallels comparable to mixed-therapy strategies in oncology that likewise seek to delay the rapid evolution of resistance in tumor cell populations. First, both (or more) herbicides in a mixture must control the same weed population, as an herbicide having no effect on a focal weed population will not influence the rate at which it evolves resistance to another herbicide. Second, both herbicides must be similarly effective in killing weeds (e.g., lethal to similar percentages of the focal weed population), and third, both must persist in the environment for similar durations; failure to meet these criteria leaves some portion of the focal weed population exposed to only one of the herbicides and thus prone to rapid evolution of resistance to it. Fourth, the two herbicides must have different biochemical targets within the focal weed population, such as inactivating different proteins or enzyme systems. Fifth, both must be degraded through different mechanisms; failure to meet these criteria may induce evolution of cross-resistance. Although the use of herbicide mixtures effectively inhibits the evolution of specialist resistance traits, one downside of this strategy appears to be selection for generalist resistance (Comont et al., 2020). Whether this outcome is unavoidable or results from failure to meet the above criteria is unclear, but it does raise a warning sign for extrapolating biocide mixture approaches to oncology. Lastly, a desirable property of mixtures is negative cross-resistance, in which one herbicide selects for alleles that confer hypersusceptibility to the other herbicide. In cancer, such negative cross-resistance are known as double-bind therapies in which drugs should be given sequentially rather than together (Gatenby et al., 2009a; Basanta et al., 2012; Gatenby and Brown, 2020b).
Pollutants, although not intentionally applied to wild populations, can mimic pesticides by acting as lethal poisons. As such, like pesticides, they have the capacity to impose intense selective pressure and drive rapid evolution. In temperate and boreal regions, for example, salts used to de-ice roads commonly run off into freshwater ecosystems. The resulting salinization of these water bodies raises the question of whether freshwater populations can adapt to the selective pressure imposed by this new water chemistry regime. Indeed, populations of the freshwater cladoceran zooplankton Daphnia, a critical link in most freshwater lake food webs, can adapt to higher (albeit not extreme) salinities in 5–10 generations (Coldsnow et al., 2017).
3.3. Harvested Animal Populations
Humans harvest wild animal populations to obtain food, furs and clothing materials, ornamental features such as horns and antlers, and collectable specimens (e.g., mollusc shells). Given these motivations, animals with particular traits or trait values (e.g., particular morph or size) are often targeted for harvesting, driving phenotypic change in harvested populations. Phenotypic responses to harvesting are well-documented in fishes, from freshwater recreational harvesting (Sutter et al., 2012) to marine commercial harvesting (Law, 2000), and in a wide variety of ungulates such as bighorn sheep (Pigeon et al., 2016) and elephants (Jachmann et al., 1995). More pervasively, selective harvest and associated phenotypic change is also documented in a variety of other mammalian and invertebrate taxa (Allendorf et al., 2008). Harvest reduces the frequency of desirable phenotypes in populations, quite opposite to the reinforcement of desired phenotypes under artificial selection in agriculture and aquaculture (Allendorf and Hard, 2009). While lack of additive genetic variance and plasticity in targeted traits may limit heritable responses to harvest, harvest is generally thought to drive evolution through three mechanisms: reduced local densities that open harvested subpopulations to immigration and concurrent genetic swamping and loss of local adaptation, selection on standing variants, and reduced genetic variation (Allendorf et al., 2008). Molecular genetic monitoring is recommended to detect harmful genetic change that results from selective mortality via harvest (Allendorf and Hard, 2009). Assessing and monitoring for specific mutations or overall genetic heterogeneity have become part of personalized medicine in cancer treatments. Genetic predispositions of the patient and the presence of specific driver mutations often permit early detection of cancer, indicate the presence of certain types of drug resistance, and dictate the course of therapy.
While there is consensus that harvesting changes phenotypic frequencies in harvested populations, the role of evolution in these changes remains uncertain, particularly in harvested fish populations. Harvest may indeed act on heritable variation and thereby drive evolution, but may also induce ecological changes (e.g., reduced population densities) that provoke plastic responses in harvested populations (Kuparinen and Festa-Bianchet, 2017). Some evidence suggests that adaptation is localized and occurs rapidly (within a few generations). Age-structured population models indicate that harvest strategies that ignore harvest-induced evolution can ultimately depress sustained yields because they irreversibly select for maturation at smaller sizes and younger ages (Heino, 1998). Probabilistic maturation reaction norms delineate the probability (usually 50%) of maturation for different combinations of age and size. Changes in the shape of reaction norms (as opposed to changes in location along a reaction norm) indicate evolutionary change, and, when such shifts coincide with harvest, provide evidence of harvest-induced evolution (Olsen et al., 2004). Converse arguments posit that relatively low heritability of relevant life history traits means that evolutionary responses to fish harvesting may require long time scales (Law, 2007). Evolutionary responses to harvesting may be difficult to detect because of counter-gradient variation, where, for example, alleles for fast growth are favored in cold environments, and vice versa, thereby reducing phenotypic variation among populations across environmental (e.g., climatic) gradients (Jorgensen et al., 2007).
A principle concern for wildlife managers is whether evolutionary responses to selective mortality via harvest undermines the ecological sustainability of the harvested population. Fishing, for example, may select for traits that are not adaptive with respect to natural and sexual selection regimes, leaving harvested populations without the phenotypic traits or variation needed to cope with their environment (Conover, 2000). Adaptive variation needed to recover during fishing moratoria may be limited in overharvested fish populations. For instance, overharvested populations of many species exhibit reduced allelic diversity and heterozygosity (Pinsky and Palumbi, 2014). Moreover, harvesting diminishes traits that correspond with fitness (weapon size in ungulates, size at maturity, and boldness in fish), yet when harvest is suspended to permit recovery, countervailing selection that favors the reemergence of these traits may be less intense, prolonging population recovery (Allendorf et al., 2008). This outcome was evident in northern populations of Atlantic cod (Gadus morhua), in which harvest selected for a younger age and smaller size at maturity. Population densities remained depressed even after a decade of fishing moratorium Olsen et al. (2004). While diminished sustainability of a wild population is an undesirable outcome of evolutionary responses to selective mortality, analogous outcomes in oncological settings would be favorable. Useful therapies may be those that impose evolutionary trade-offs on cancer cell populations by selecting for cancer cell phenotypes that are maladaptive to the natural selection regime imposed by the immune system, or that intensely select against antagonistic traits that are only modestly favored during AT holidays. These are key issues in designing, implementing and modeling AT.
4. Modeling Eco-evolutionary Dynamics of Cancer in Response to Treatment
Similar to human intervention in ecology, therapeutic intervention in cancer can favorably or unfavorably direct evolution. MTD is the standard of care in which therapy is given continuously for a predetermined amount of time. When MTD is unable to eliminate all cancerous cells it inevitably selects for the continued proliferation of treatment-resistant cells (Chabner and Roberts, 2005; Gatenby, 2009; Pepper et al., 2009; Aktipis et al., 2011; Greaves and Maley, 2012). In contrast, AT modulates therapy based on tumor dynamics in response to treatment. When there is a cost to resistance (a disadvantage to being resistant), therapy-sensitive cells outcompete their resistant counterparts in the absence of treatment. Therefore, drug withdrawal during AT suppresses the ability for resistant cells to dominate the tumor population (Gatenby et al., 2009b; Zhang et al., 2017; Staňková et al., 2019). The evolutionary capacity of cancer cell phenotypes to withstand therapy induced selection regulates the effectiveness of therapy. Specifically, how fast or slow evolution occurs may play a key role in therapeutic success. We develop mathematical models to analyze the impact of evolutionary speed on the success of AT when compared to MTD.
Combating resistance is prevalent in nature and medicine when dealing with an evolving population. Resistance mechanisms to biocides in pest species and therapies in cancer can be categorized as follows: (1) strictly qualitative (for instance, the presence of an upregulated or novel metabolic pathway in the resistant form), which can be modeled using a polymorphic population where one strain is sensitive while another strain possesses a resistance trait which is fixed and does not evolve; (2) strictly quantitative (for instance, the production of binding or detoxification enzymes, and for cancer and microorganisms an increase in the number of membrane pumps for eliminating the toxin), which can be modeled using a monomorphic population with an evolving resistance trait; or (3) a hybrid combination of contexts 1 and 2, which can be modeled using a polymorphic population where one strain remains sensitive while another strain possesses an evolving quantitative resistance trait. For example, in the case of abiraterone resistance in metastatic castrate-resistant prostate cancer there are three qualitative cell types: those requiring exogenous testosterone, those independent of testosterone, and those producing testosterone as a public good (You et al., 2017, 2019; Zhang et al., 2017). While the first two are strictly qualitative, the last type can also be quantitative in terms of the amount of testosterone produced. Strictly qualitative resistance traits have been explored in detail by modelers (You et al., 2017; Zhang et al., 2017; West et al., 2018; Cunningham et al., 2020; Bayer et al., 2021; Kim et al., 2021; Viossat and Noble, 2021), strictly quantitative less so (Staňková et al., 2019; Reed et al., 2020; Salvioli, 2020; Wölfl et al., 2020), and the combination, to our knowledge, has not been explored at all. Our models can be used to consider all three contexts (strictly qualitative, strictly quantitative or both). In what follows, we shall focus on comparing contexts 2 with 3. We do not model context 1 (polymorphic population, strictly qualitative resistance) as it is a special case of our context 3 (polymorphic population with resistance evolving as a quantitative trait of the resistant population). A final key element for all considerations of evolving pests and managed species concerns a cost of resistance. A cost of resistance will slow the evolution of resistance, and render more sensitive types more competitive than less sensitive types when the biocide is removed. In our models, we consider what happens when there is no cost of resistance, a cost of resistance in the intrinsic growth rate, or a cost of resistance in the carrying capacity.
Our models are generic in the sense that they can frame biological systems other than cancer and their eco-evolutionary responses to management by humans. However, we narrow our model analysis to cancer. We consider the superiority of AT relative to MTD as measured by time to progression (TTP). We consider jointly the effects of evolutionary speed, the context of resistance, and the nature of the cost of resistance. The model permits any level and spacing of dosing, but we focus on contrasting a continuous, fixed level of dosing with a form of AT where the dosing is either on or off depending on the patient's tumor burden.
4.1. Our Models
We model the evolution of resistance leading to treatment failure using ordinary differential equations (ODEs) for a monomorphic (context 2) and polymorphic (context 3) tumor cell population. In our monomorphic model, resistance is a quantitative trait. The resistance strategy exists on a continuum, and all cells can exhibit some magnitude of resistance u(t), which evolves in time. In our polymorphic model, we assume the entire tumor cell population is comprised of two distinct subpopulations, sensitive and resistant cells. In this model, only the resistant cell subpopulation has the capacity to evolve resistance as a quantitative trait uR(t). Table 1 displays all scenarios for each model and Table 2 indicates all parameters and their definitions. In the following, we assume that the tumor populations grow logistically and are suppressed by the presence of therapy and natural cell turnover.
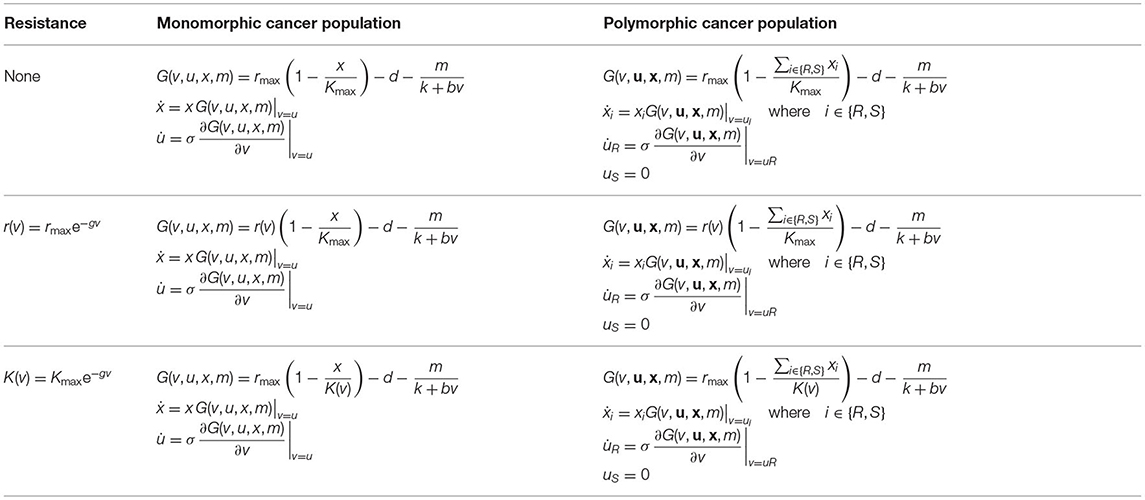
Table 1. Different models analyzed in this paper: The first, second, and third lines of this table describe eco-evolutionary cancer dynamics with no cost of resistance, cost of resistance in the growth rate, and cost of resistance in the carrying capacity of cancer cells, respectively.
All models describe Darwinian dynamics of cancer in response to treatment, with a fitness-generating function, “G-function” (Vincent and Brown, 2005). A G-function considers how the fitness of a focal cancer cell using a strategy v in the population is influenced by the environment and by the strategies and population sizes of the resident phenotypes. The set of phenotypic strategies present in the tumor are represented by u. The population size of cells with a particular strategy is indicated by x. In the polymorphic context, the vector encompasses the strategy for resistant and sensitive cells and their population sizes. In the monomorphic context u and x are reduced to scalars as only a single evolutionary strategy defines the entire tumor population. We assume that the physician applies a treatment dose m(t)∈[0, 1] at time t≥0, where m(t) = 0 and m(t) = 1 correspond to no dose and MTD at time t, respectively. For simplicity, the drug is assumed to be maximally effective at MTD. The efficacy of the drug is reduced by a focal cell's resistance strategy v, innate drug immunity k, and the benefit b of the resistance trait in reducing therapy efficacy. The G-function is used to derive the evolutionary dynamics that describe how the resident strategies (i.e., phenotypes) of the tumor change with time. Note that in this case the fitness function for a rare mutant does not directly depend on the current resident strategies. Following Fisher's fundamental theorem of natural selection, the resistance strategies change in the direction of the fitness gradient with respect to the fitness of a rare mutant v (Fisher, 1930). This derivative is then evaluated at the current resident strategies u, giving an equation defining the evolutionary dynamics for each resident strategy (Table 1) (Vincent and Brown, 2005). The rate at which the strategies change is scaled by an evolutionary speed term σ. In our model, large values of evolutionary speed σ correspond to enhanced phenotypic variance which could result from increased genetic variance or phenotypic plasticity. Innate immunity k suggests that prior to drug exposure cells possess a mechanism that inhibits the potency of treatment. This parameter is the only value that reduces drug efficacy for the sensitive population in our polymorphic model as the sensitive cells cannot evolve resistance. Treatment efficacy is further diminished by the magnitude of the benefit b of the resistance strategy for the monomorphic population and the resistant population in the polymorphic model. For a general introduction to our modeling framework, see Appendix B.
Although resistance decreases treatment efficacy, it may be that a resistance strategy comes at a cost (Staňková, 2019). When a cost to resistance is present, resistance confers a selective advantage during treatment. In the absence of therapy, a cost of resistance confers a fitness disadvantage. In our model, we consider that resistance either carries no cost (K(v) = Kmax, r(v) = rmax), carries a cost in the intrinsic growth rate ( K(v) = Kmax), or carries a cost in the carrying capacity (r(v) = rmax, ). These costs are relevant when modeling the monomorphic population, and for the resistant population when modeling the polymorphic context.
4.2. Case Studies
We analyze the impact of two treatment strategies (MTD and AT) on TTP. We define TTP as the first time at which the tumor burden reaches δ = 70% of the maximum carrying capacity Kmax, during treatment.
The treatment schedule for each strategy is as follows:
• Maximum tolerable dose (MTD): m(t) = 1 for all t;
• Adaptive therapy (AT): Initially, MTD is administered (m(0) = 1) until the tumor cell population size x reaches half of its initial density. Treatment is then discontinued until the tumor recovers to its initial size where treatment is re-administered beginning a new treatment cycle (Zhang et al., 2017). We will also, at times, consider a 20% reduction in tumor volume as the switch threshold. While this has not been tried in any clinical trial, we include it as several authors have shown that it gives superior results as compared to a 50% reduction (Kim et al., 2021; Strobl et al., 2021; Viossat and Noble, 2021).
We first consider the case of a monomorphic tumor cell population, which has evolved resistance u(t) at time t≥0 in response to treatment m(t). Here, u(t) = 0 corresponds to no resistance. We do not impose an upper bound on the resistance trait, thus u(t) is a quantitative trait achieving non-negative values. For the sake of simplicity of expressions, we will drop the time variable t whenever this does not compromise the clarity. For this case, we always consider initial conditions x(0)= 6,000 and u(0) = 0.
Next, we consider the case of a polymorphic tumor cell population. We assume that the tumor is composed of distinct sensitive xS and resistant xR subpopulations of cells. The sensitive subpopulation utilizes a fixed resistance strategy uS = 0, and this value does not evolve with time. The resistant subpopulation, initially expresses almost no resistance uR(0) = 0.01 and evolves treatment-induced resistance (uR>0) over time. The initial conditions for each subpopulation are xS(0) = 5, 990, and xR(0) = 10.
We also analyze how different assumptions regarding the cost of resistance in cancer cells (no cost of resistance, cost of resistance in cancer cells' growth rate (), and cost of resistance in cancer cells' carrying capacity ()) impact the success of MTD and AT in terms of TTP. Altogether, our modeling efforts investigate how the TTP varies between AT and MTD therapy, dependent on the following:
• The type of cancer population (monomorphic vs. polymorphic),
• The cost of resistance (none, on r, on K), and
• Evolutionary speed σ.
The models were solved numerically through the odeint function of the Python 3.6 Scipy package, using three-stage Adams-Bashforth method with adaptive stepsize and backward differentiation formula for stiff and non-stiff problems, respectively. All results were also duplicated in Mathematica, to validate their correctness.
5. Results
In this section, we begin by investigating how the cost of resistance impacts tumor burden and TTP during continuous therapy at MTD. Subsequently, we compare TTP under MTD vs. AT for each of our eco-evolutionary models introduced in the previous section. The ability to extend TTP signifies a greater treatment efficacy and the treatment strategy exhibiting a longer TTP is deemed superior. We show that in all of our model scenarios, AT is superior to MTD. The magnitude of this superiority (increase in TTP compared to MTD) is influenced by the context (monomorphic vs. polymorphic tumor composition), evolutionary speed, and to a lesser extent by the cost of resistance.
5.1. A Cost of Resistance Manifested in the Carrying Capacity Comprises Tumor Growth the Most
Typically, MTD leads to an initial decrease in the tumor burden, followed by the evolution of resistance and ultimately treatment failure. Figure 1 depicts these dynamics for both our polymorphic (top row) and monomorphic (bottom row) tumor cell populations exhibiting different resistance costs. For all cases, MTD results in evolutionary dynamics where there is a monotonic increase in the level of resistance of the evolving cancer cell populations. When there is a cost of resistance (influenced by parameter g), TTP increases, resistance evolves more slowly, and maximum tumor burden declines. In both population contexts, when there is no cost to resistance the tumor burden stabilizes at a population density 4% greater than when the cost of resistance is expressed in the intrinsic growth rate r and almost 20% greater than when the cost of resistance is expressed in the carrying capacity K. Thus, a cost applied to the carrying capacity compromises tumor regrowth the most, leading to an extended TTP. As our intuition would suggest, when the cost of resistance is very high, the population dynamics stabilize at much lower tumor burdens (Figure A1). Based on model formulation and parameterization, there are instances when tumor regrowth stabilizes at a survivable tumor burden that does not result in progression. In Figure 1, the tumor burdens of both population contexts recover to reach what we consider disease progression, however in the monomorphic context, the initial decrease in the population density is much less than in the polymorphic context. In some cases, continuous therapy will not reduce the tumor burden sufficiently to allow for AT. For the purposes of our investigation, we focus on scenarios where continuous treatment results in at least a 50% decline in the initial tumor burden, and where resistance evolution under continuous therapy will result in a tumor burden that exceeds δ=70% of the maximum carrying capacity (which we consider to represent disease progression). With these two stipulations, we restrict ourselves to conditions where AT can be applied, and where continuous MTD will eventually result in disease progression.
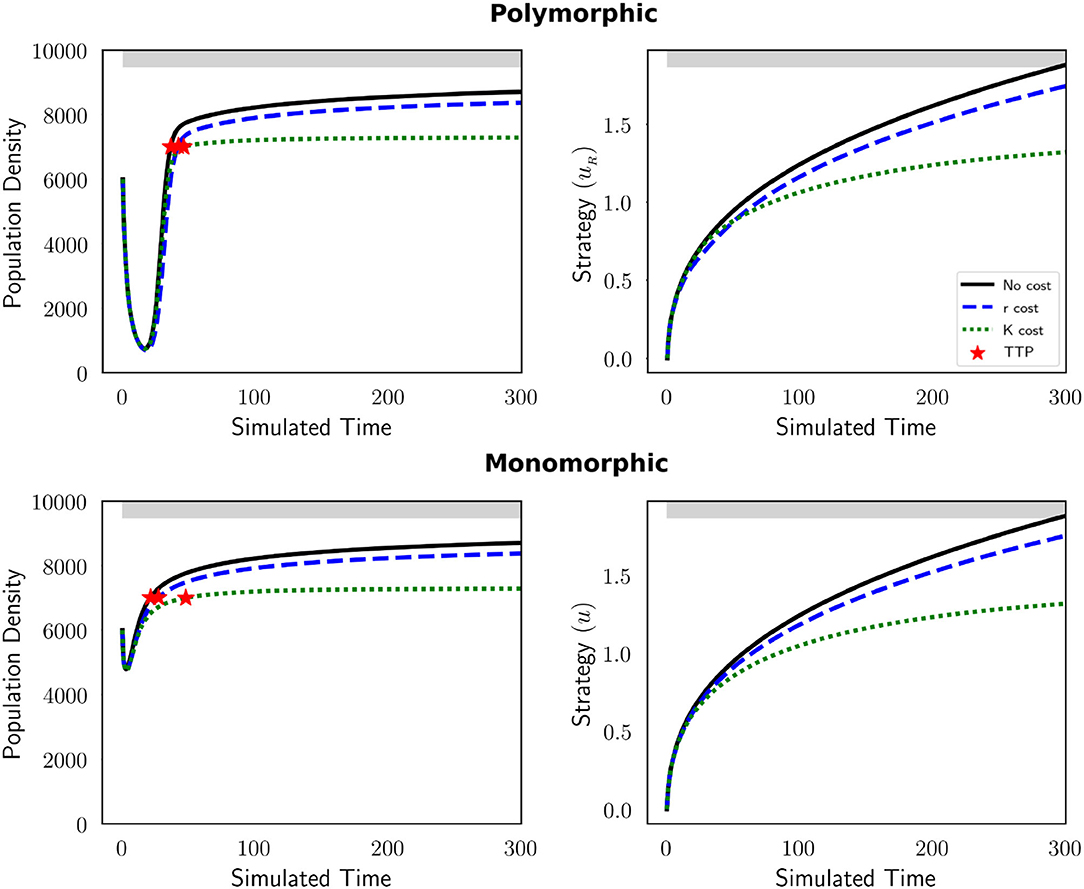
Figure 1. The presence of a resistance cost can improve the short-lived therapeutic success of MTD treatment. Drug administration is shown at the top of each plot in gray. Population dynamics (left) and the evolutionary dynamics (right) are shown in the absence of a resistance cost (black solid line), and when the cost is manifested in the intrinsic growth rate (blue dashed) and carrying capacity (green dot dashed line) for a polymorphic (top) and monomorphic (bottom) tumor cell population. The strategy in the polymorphic context is that of the resistant population of cells. Red stars indicate progression defined as x≥7,000. When a cost of resistance is imposed on carrying capacity there is a greater extension in TTP. The parameters used in these figures are: m = 1, Kmax = 10, 000, rmax = 0.45, k = 2, b = 10, d = 0.01, g = 0.1, σ = 0.1.
5.2. AT Improves TTP With or Without a Cost of Resistance, Where the Improvement Is Greatest When the Cost Is Applied to the Carrying Capacity
Independent of the presence of a cost of resistance, AT is superior to MTD when we do not consider evolutionary speeds. In Figure 2, we see that in the polymorphic context AT leads to a clear improvement in treatment efficacy in terms of TTP with respect to MTD. The left panel exhibits the population dynamics for this polymorphic population during each treatment schedule and the right panel displays the strategy dynamics of the resistant cell type. TTP is represented by red stars which identify when the tumor burden reaches 7,000. Regardless of the resistance cost, AT extends TTP compared to MTD. In the absence of a resistance cost, AT increases TTP by 33%. With a cost of resistance, AT increases TTP by 34 and 45% when manifested in the growth rate and carrying capacity, respectively. Drug holidays during AT lead to disruptions in the strategy dynamics of the resistant cells, increasing the time it takes for them to reach levels of resistance that result in disease progression. We further analyze how therapy influences the frequency of sensitive and resistant cells within the tumor in Figure 3. Here we observe the dynamics of each subpopulation and the total population of cancer cells with MTD (top row) and AT (bottom row). There is no decrease in the resistant subpopulation when therapy is not applied, but a drug holiday through adaptive scheduling decreases the speed of its growth. When therapy is applied continuously there is no opportunity for the sensitive subpopulation to regrow and maintain a tumor composition that is majority drug sensitive. We note that eventually, also with AT, the resistant subpopulation outcompetes the sensitive one and the disease progresses. Furthermore, we explore a parameterization that allows us to compare AT to MTD for a monomorphic and polymorphic population (Figure A2). The dynamics are similar to Figure 2, AT remains advantageous for both contexts. The TTP for all cases is increased significantly due to a reduction in the cost of resistance (g = 0.01) and benefit of resistance (b = 1).
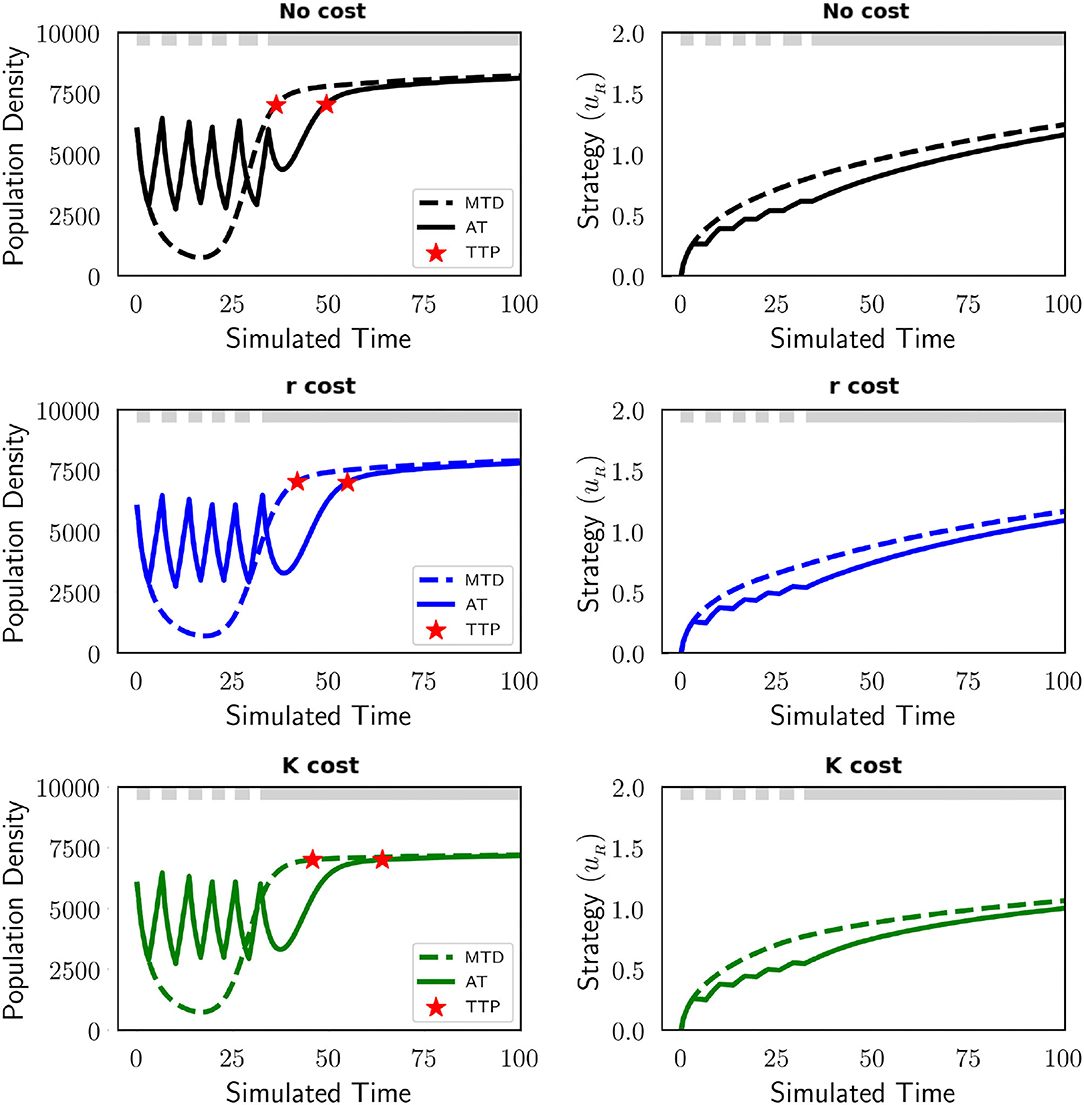
Figure 2. AT is superior to MTD independent of the cost of resistance. Simulations of AT (solid line) and MTD (dashed line) for a polymorphic population are shown in the absence of a resistance cost (black), and when the cost impacts the intrinsic growth rate (blue) and carrying capacity (green). Population dynamics (left) and evolutionary dynamics (right) illustrate the advantage of AT compared to MTD. The protocol for AT is to withdraw the drug once the population density ≤ 3, 000 (50% of the initial population size). Drug administration for AT is shown at the top of each plot in gray. TTP for each is illustrated by red stars. Only the strategy for the resistant population is shown as the sensitive population has a fixed strategy (us = 0). Regardless of the cost of resistance, AT always corresponds to a longer TTP. The parameters used in this figure are: m = 1, Kmax = 10, 000, rmax = 0.45, k = 2, b = 10, d = 0.01, g = 0.1, σ = 0.1.
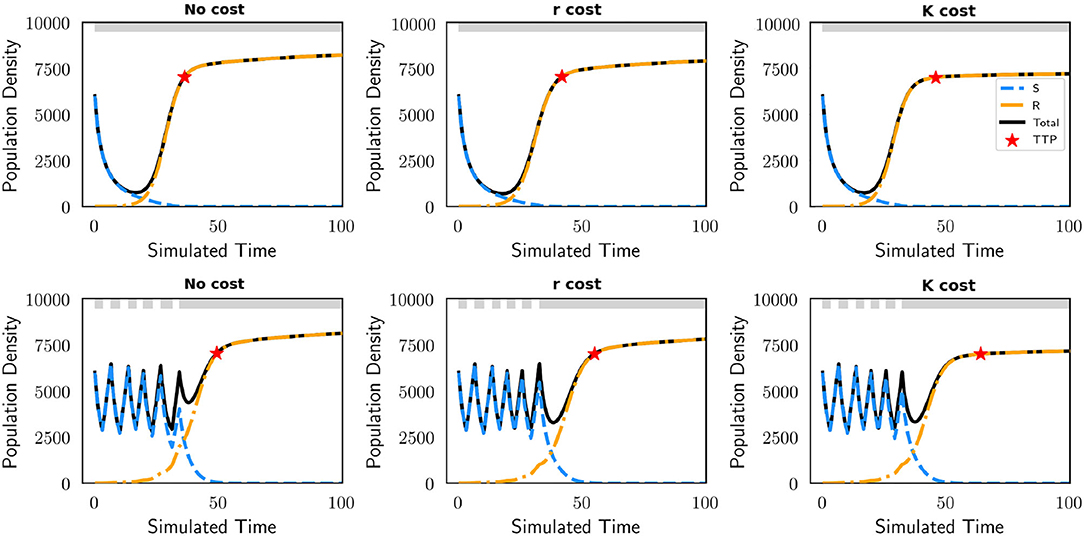
Figure 3. Changes in the frequency of sensitive (blue) and resistant (yellow) subpopulations in the polymorphic context during MTD (top) and AT (bottom). At the top of each plot, drug administration is shown in gray. These dynamics are shown for three different models of costs of resistance: no cost, cost applied to the growth rate (r cost), and cost applied to the carrying capacity (K cost). AT delays TTP (red star) by maintaining a tumor composition of mostly sensitive cells for a longer time than MTD. The parameters used in this figure are: m = 1, Kmax = 10, 000, rmax = 0.45, k = 2, b = 10, d = 0.01, g = 0.1, σ = 0.1.
5.3. Faster Speeds of Evolution Reduce the Improvement in TTP Provided by AT
Evolutionary speed σ contributes to the effectiveness of AT. At faster speeds of evolution, tumor regrowth occurs quicker, decreasing TTP (Figure A3). The impact of increasing evolutionary speed on TTP for MTD and AT in a polymorphic population is shown in Figure 4. At very slow speeds of evolution, we see significantly longer TTPs for all contexts of resistance, both for MTD and AT. We observe again that when the resistance cost is manifested in carrying capacity of resistant cells, TTP is longer, for both MTD and AT, than when resistance cost is manifested in intrinsic population growth rate or when there is no cost. When σ>0.01 the relative TTP for each treatment strategy decreases dramatically. Nonetheless, under all evolutionary speeds and resistance cost scenarios, AT remains superior to MTD via lengthening TTP (Figure 4).
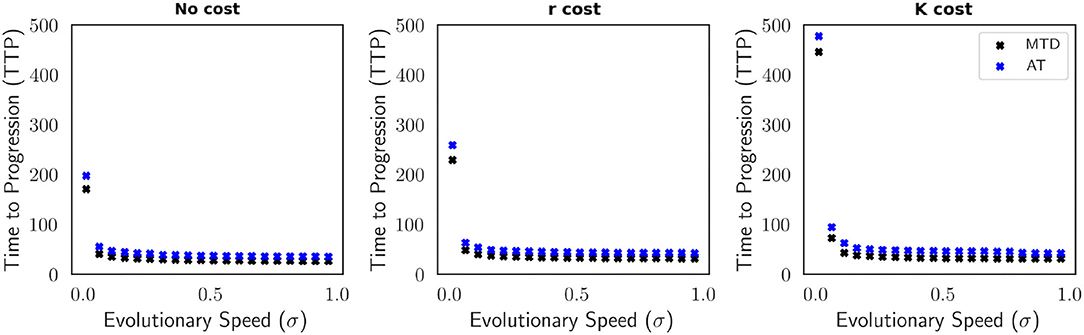
Figure 4. TTP for MTD (black) and AT (blue) as a function of the evolutionary speed for a polymorphic tumor cell population. This is illustrated for each model of cost of resistance (no cost, r cost, and K cost). At faster speeds of evolution, AT remains favorable but TTP decreases for both AT and MTD. Of the three models of cost of resistance, the TTP for MTD and AT is shortest in the absence of a cost and greatest when the cost of resistance impacts the carrying capacity K. The parameters used in this figure are: m = 1, Kmax = 10, 000, rmax = 0.45, k = 2, b = 10, d = 0.01, g = 0.1.
In absolute terms (TTPAT−TTPMTD) this benefit declines as evolutionary speed increases, while proportionally ((TTPAT−TTPMTD)/TTPMTD), the benefit of AT increases with evolutionary speed (Figure 5).
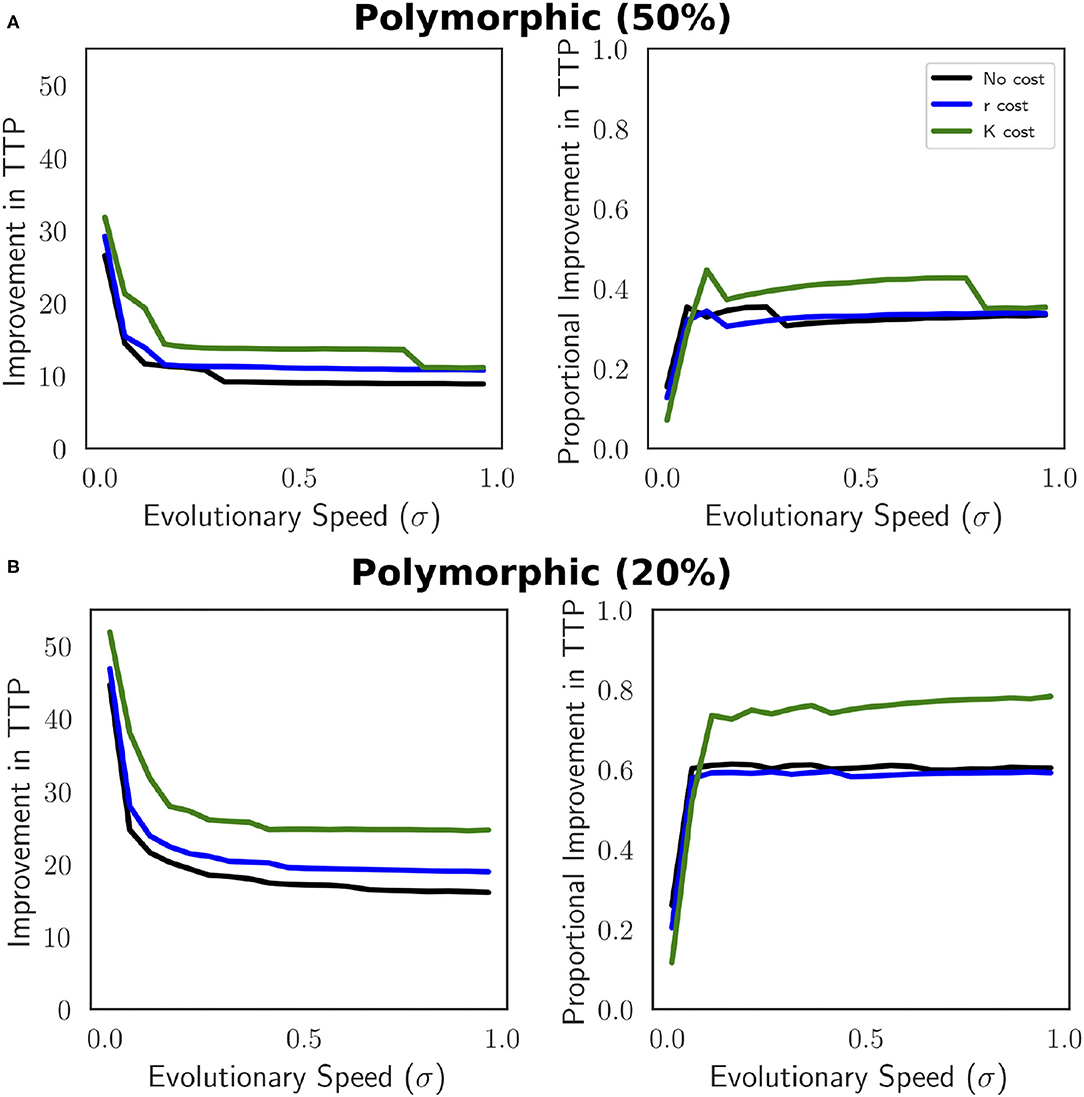
Figure 5. Improvement in TTP (TTPAT−TTPMTD) and proportional improvement in TTP ((TTPAT−TTPMTD)/TTPMTD) as a function of evolutionary speed for a polymorphic tumor cell population. (A) Therapy is withdrawn once the population density ≤ 3, 000 (50% of the initial population size) and re-administered once the population ≥6, 000 (initial population size). (B) The threshold to remove therapy is reduced from 50% to 20%. Therapy is withdrawn once the population density ≤ 4, 800 (20% decrease from initial population size) and re-administered once the population ≥6, 000 (initial population size). The benefit of AT increases at faster speeds of evolution. A reduction in the magnitude the tumor population decreases before therapy can be withdrawn makes AT more effective. The parameters used in this figure are: m = 1, Kmax = 10, 000, rmax = 0.45, k = 2, b = 10, d = 0.01, g = 0.1.
Relative to MTD, AT will extend TTP by a greater proportion at faster evolutionary speeds due to the already short-lived therapeutic success of MTD at those greater speeds. AT does not confer a proportionally large increase in TTP when TTP under MTD is relatively long. Under this parameterization, AT relative to MTD provides a greater proportional improvement in TTP when a resistance cost is expressed in carrying capacity than when it is expressed in population growth rate or not at all. However, when evolutionary speed is fast, this advantage is lost. Since the original AT trial protocol was introduced, subsequent studies suggest that withholding therapy sooner provides a greater benefit. We explore this by removing treatment once the tumor burden drops 20% of initial density (Figure 5B). This change in protocol amplifies the results shown when using a 50% threshold. The overall trends remain the same, the relative superiority of AT is greater at faster speeds of evolution.
5.4. Improvement in TTP Provided by AT Is Greater for a Polymorphic Population
As previously stated, the AT regimen cannot be applied to a monomorphic population using the same parameterization. This is due to an insufficient decrease in tumor burden during continuous treatment (Figure 1). By reducing the benefit (b is decreased from 10 to 1) and cost of resistance (g is decreased from 0.1 to 0.01) we are able to achieve population dynamics during MTD that satisfy our requirements for AT. In Figure 6, we compare improvement in TTP when AT is applied to the polymorphic and monomorphic contexts, and to different forms of the cost of resistance. As before, having a cost of resistance in carrying capacity produces a longer TTP than having no cost or a cost in intrinsic population growth rate (Figure A4). Here we observe a trend similar to Figure 5 in terms of improvement in TTP for both population contexts, independent of the cost of resistance. As evolutionary speed increases the improvement AT provides decreases.
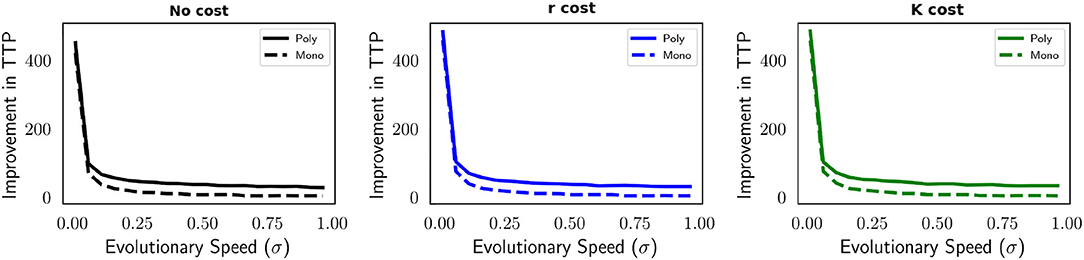
Figure 6. The impact of evolutionary speed on the improvement in TTP for a monomorphic and polymorphic tumor cell population compared between different models of cost of resistance. Compared to Figures 1–5, b is reduced from 10 to 1 and g is reduced from 0.1 to 0.01. Rapid evolution decreases the improvement in TTP provided by AT independent of a resistance cost. Despite the decline, there remains a greater effectiveness of AT when the population is polymorphic. All parameter values used in this figure are: m = 1, Kmax = 10, 000, rmax = 0.45, k = 2, b = 1, d = 0.01, g = 0.01.
Our results show that there is a significant difference in the improvement AT bestows based on tumor composition. Improvement in TTP with AT is not as advantageous for a monomorphic population as it is for a polymorphic population. The proportional improvement of AT is minimal when the population is monomorphic (Figure 7). At faster speeds of evolution, the relative superiority of AT decreases in this population. In contrast, there is a greater proportional improvement in TTP under AT than under MTD when the population is polymorphic than when it is monomorphic. The proportional AT advantage is shown to correlate positively with evolutionary speed. In the polymorphic case, the proportional improvement in TTP under AT vs. under MTD is greatest where TTP under MTD is short (Figure 5). The altered therapy protocol (Figure 7B), again, shows that the overall results remain the same. Notably, maintaining the tumor burden at a therapy switching threshold of 20% its original size allows AT to be more effective. Although rapid evolution is unfavorable in terms of delaying TTP, AT is proportionally more successful when the evolutionary speed is faster.
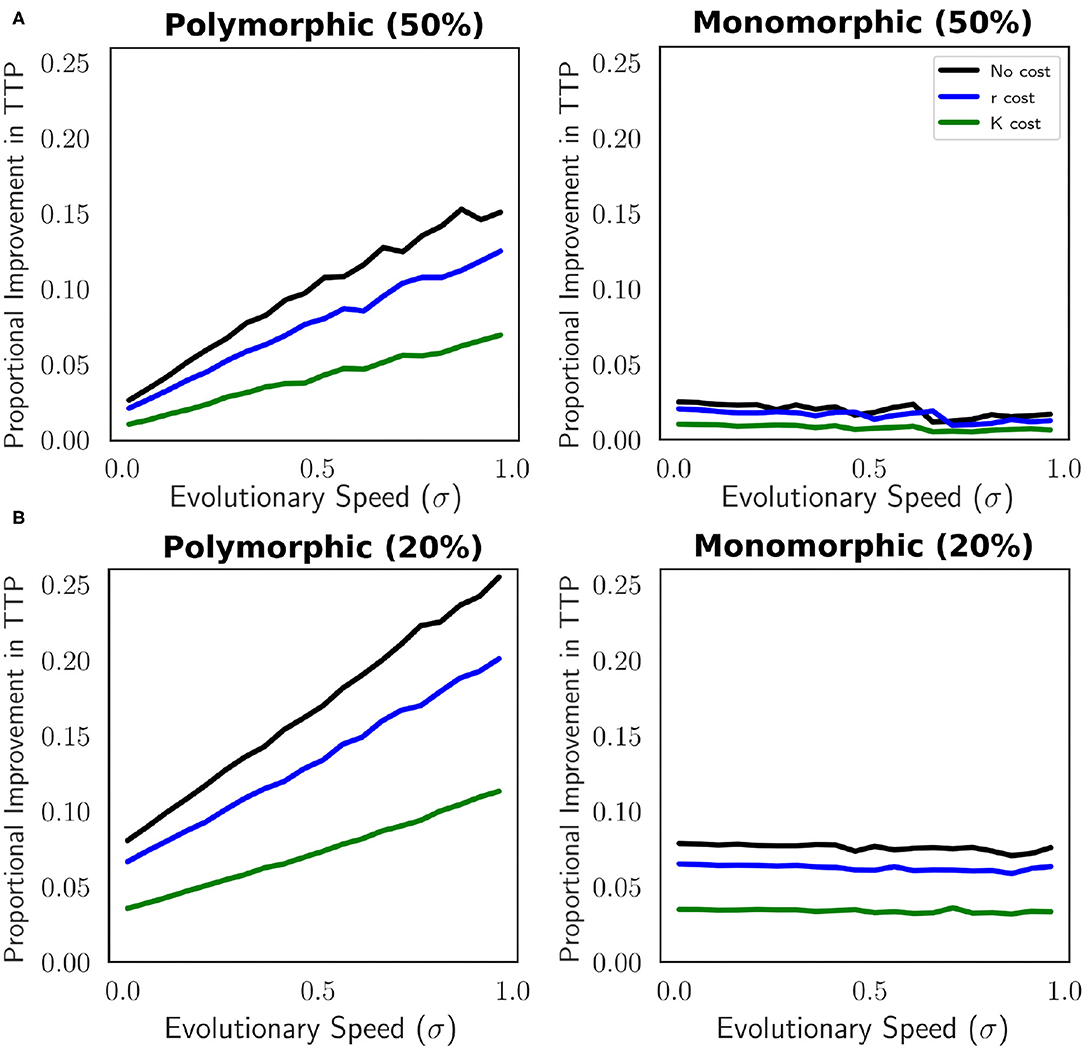
Figure 7. Comparison of proportional improvement in TTP as a function of evolutionary speed for a monomorphic and polymorphic tumor cell population. Three models of resistance are compared: no cost of resistance, cost of resistance expressed in r, and cost of resistance expressed in K. (A) Therapy is withdrawn once the population density ≤ 3, 000 (50% of the initial population size) and re-administered once the population ≥6, 000 (initial population size). (B) The threshold to remove therapy is reduced thus from 50% to 20%. Therapy is withdrawn once the population density ≤ 4, 800 (20% decrease from initial population size) and re-administered once the population ≥6, 000 (initial population size). The superiority of AT when the population is monomorphic is minimal and as evolutionary speed increases the relative benefit of AT declines. A polymorphic population of cells allows for a greater effectiveness of AT. In this case, when the speed of evolution is quick AT provides a greater proportional benefit. Withdrawing therapy at 20% opposed to 50% improves the proportional increase in the effectiveness of AT. The parameters used in this figure are: m = 1, Kmax = 10, 000, rmax = 0.45, k = 2, b = 10, d = 0.01, g = 0.1.
6. Discussion
6.1. Main Outcomes
In this paper, we model how the speed of evolution of treatment-induced resistance in cancer cells impacts the patient's TTP under two treatment regimens: (i) maximum tolerable dose (MTD) and (ii) adaptive therapy (AT) following the Zhang protocol, where MTD is discontinued when the tumor reaches half of its initial volume and is re-administered only once the tumor recovers (Zhang et al., 2017). We considered two eco-evolutionary contexts. In the first, there is a monomorphic population of cancer cells, with treatment-induced resistance being a quantitative trait that evolves in accord with standard models of quantitative genetics and adaptive dynamics. In the second, the population of cancer cells is assumed to be polymorphic with a strictly sensitive subpopulation that does not evolve, and a resistant subpopulation of cancer cells that can evolve increasing resistance as a quantitative trait.
There is an existing tradition of cancer models that treat the evolving trait of the cancer cells as quantitative in ordinary differential equation models (Orlando et al., 2012; Staňková et al., 2019; Reed et al., 2020; Salvioli, 2020), partial differential equation models (Lorenzi et al., 2016; Almeida et al., 2019) and in agent-based models (Gallaher et al., 2018). There is also a tradition of modeling resistance evolution by presupposing pre-existing populations of therapy resistant and sensitive populations (Sun et al., 2016; You et al., 2017; Zhang et al., 2017; Cunningham et al., 2020; Strobl et al., 2020; Kim et al., 2021; Viossat and Noble, 2021). In these models, resistance is a qualitative trait and it does not exist on a continuum. Our second model and eco-evolutionary context represents a new approach to the resistance strategy. Like models with a quantitative trait, we let the resistant population start with a positive but low level of the resistance trait. This subpopulation evolved its quantitative trait according to Darwinian dynamics. Like models with qualitative traits we assumed a sensitive, non-evolving subpopulation that had only some level of innate resistance to the therapy. It remains an open empirical question in all fields that involve managing evolving pests and resources whether the population under management is (1) polymorphic with sensitive and resistant subpopulations with fixed values for their resistance traits, (2) monomorphic with a quantitative trait, or (3) polymorphic with an evolving resistant population and a fixed sensitive population. We suspect examples of all three exist in nature, in disease management, and in pest management. For the second and third eco-evolutionary contexts (where in fact the first context mentioned is a special case of the third one), we considered three different modeling forms for the cost of resistance: (i) no cost of resistance, (ii) the cost of resistance manifests as a decrease in the growth rate of cancer cells r, and (iii) the cost of resistance manifests as a decline in the cancer cells' carrying capacity K.
Our first main observation concerns the eco-evolutionary context with a monomorphic cancer population with a continuous resistance trait. While AT outperforms MTD, it does so only very slightly and only at slow evolutionary speeds. Above a certain rate of evolution, the tumor burden never decreases to half of its initial volume and as such, AT TTP coincides with that of standard of care. This outcome is independent of whether or not the cost of resistance is considered. TTP is slower with a cost of resistance than without. In terms of the proportional increase of the TTP when compared to the MTD regimen, AT with no cost of resistance outperforms AT when the cost of resistance acts on r (intrinsic growth rate) which outperforms AT when the cost of resistance impacts K (carrying capacity). The relatively lackluster benefits of AT under a quantitative resistance strategy has to do with the fitness gradients of the adaptive landscape. The slope of the logistic term of the fitness function is either a drag on evolving resistance when therapy is on or the force that evolves greater sensitivity when therapy is off. This aspect of the fitness gradient is always active. Not so for the effect of therapy. The mortality term from therapy is active and decisive in evolving resistance when therapy is on, but it becomes neutral, not negative, when therapy is off. Thus, in general, therapy being on propels the evolution of resistance more strongly than the evolution of increased sensitivity when therapy is off. This finding parallels a potential frustration in the management of evolving fisheries stocks, where selection for younger age and smaller size at maturity (which ultimately reduce yield) imposed by harvest is much faster and more intense than the reverse process where natural selection for older age and larger size at maturity during harvest moratoriums may restore a stock (Allendorf et al., 2008).
The second main observation concerns context with a sensitive and a resistant subpopulation of cancer cells, where resistance in the resistant subpopulation evolves. Here, AT always outperforms the MTD protocol by large margins. Regardless of AT or MTD therapies, time to treatment failure (progression) declines as evolutionary speed increases. However the proportional benefit of AT over MTD increases with high evolutionary speeds. The model predicted a 5% improvement with AT at a very low evolutionary speed and about a 55% improvement with a very high evolutionary speed. This is again independent of whether or not there is a cost of resistance. The absolute TTP is for most model parameterization shortest when there is no cost of resistance, followed by a cost of resistance in r, and longest when the cost of resistance effects K. Whether AT or MTD, TTP is fastest when there is no cost of resistance and slowest when the cost of resistance effects K. The greater effectiveness of AT over MTD with a polymorphic population derives from two sources of evolution. First, the resistant subpopulation of cancer cells evolves similarly to that of a monomorphic population. Second, there is a change in the frequency of the sensitive (non-evolving) and resistant subpopulations with each cycle of switching therapy on and off. This reservoir of sensitive cells already has the optimal resistance strategy of uS = 0 when therapy stops, and therefore their population rebounds faster than if one is waiting for the resistant cells to evolve greater sensitivity. And when therapy resumes they are least able to survive.
To summarize, in determining the superiority of AT over MTD it matters most whether the cancer population is monomorphic or polymorphic. While a slower evolutionary speed renders both AT and MTD more effective, the relative superiority of AT decreases with evolutionary speed in the monomorphic population, and increases with evolutionary speed in the polymorphic population. Overall, evolutionary speed matters more in the context of the polymorphic cancer population than the monomorphic one. Interestingly the impact of the cost of resistance on the superiority of AT is not that large. While having a cost of resistance increases TTP regardless of AT and MTD, the superiority of AT over MTD is generally greatest when there is no cost of resistance. This result was anticipated by others who noted that AT can be effective relative to MTD even when there is no cost of resistance (Strobl et al., 2021; Viossat and Noble, 2021).
6.2. Relation of Our Models and Results to Those Related to Managing/Controlling Other Evolving Systems
Models analyzed within this paper are generic in the sense that they can frame any situation where actions of a human are aimed at controlling, containing, or preserving biological systems evolving according to natural selection, such as fisheries (Salvioli et al., 2021), pest systems (Brown and Staňková, 2017; Cunningham, 2019), or parasites systems (Hastings and d'Alessandro, 2000; Bushman et al., 2018; Scott et al., 2018). In such systems, the human player impacts the eco-evolutionary response in the biological system under management, while the biological system adapts to the human's actions. The control corresponding to the AT in cancer treatment can be considered as the simplest form of evolutionary control of evolving biological systems, where maximum exploitation/control is paused when the population of the biological systems shrinks to half of its initial size. Similarly as we discussed for our model, the impact of evolution of resistance in other biological systems, such as in bacteria and virus strains, will depend on the speed of this evolution (Sandoval-Motta and Aldana, 2016). Our results imply that when the biological system is monomorphic and the evolutionary trait is quantitative, there is little difference between an adaptive and continuous-mortality approach. However, when the biological system is polymorphic, adaptive approaches may be more effective at prolonging the evolution of resistance to the source of mortality than is maintaining a constant preservation/exploitation strategy all the time.
The applicability and desirability of this outcome for wild populations depend on the objectives of management. In the case of harvested (fished, hunted) populations, it seems unlikely that continuous harvest (the MTD approach) could select for a resistant population that “progresses” to immunity from the mortality source and thus to high population density (like a cancer cell population escaping therapy) before harvest simply extirpates the population. In this case, an “AT” approach with harvest moratoria simply allows ecological population recovery with potentially minimal impact on the selective landscape and population genetic variation. In the case of selection inadvertently imposed by human activities (e.g., by urbanization), rapid evolution and short “TTP” of imperiled native plant and animal populations is desirable from a conservation standpoint, and a continuous “MTD” context is unavoidable given the persistent nature of the selective agent (e.g., unrelenting land development, pollution in the Anthropocene). The wild population context most informed by our contrast of mono- and polymorphic cancer populations is arguably that of pests and pathogens where, as with adaptive cancer therapy, human managers directly regulate the application of the mortality source (biocidal agent) and a management goal is learning to live with chronic, small, treatment-sensitive pest populations (Wrubel and Gressel, 1994). While not feasible for cancer as yet, the benefits of AT can be achieved in space as is done in some agricultural applications, where the pesticide is applied to some fraction of the field (e.g., 3/4) while a bit of field is left untreated so as to maintain a sensitive population (Denholm and Rowland, 1992; Weisz et al., 1996).
6.3. Other Evolutionary Approaches for Managing Evolving Resources
In this article we considered a classical protocol for AT introduced by Zhang et al. (2017). Other types of evolutionary therapy, i.e., therapy anticipating and steering evolution of treatment-induced resistance, exist, many of which are inspired by ecological literature. Such include double-bind, evolutionary gambit, extinction therapies, therapies targeting aggregation effects, or those aiming at tumor stabilization (Gatenby et al., 2009a, 2019; Brown and Staňková, 2017; Cunningham et al., 2020). A double-bind therapy works if in evolving resistance to drug A the cancer cells become more susceptible to drug B and vice-versa (Gatenby et al., 2009a; Basanta et al., 2012). An evolutionary gambit, or Sucker's gambit, involves applying a treatment that, while promoting or not inhibiting tumor growth, sets up an evolutionary trap by selecting for cancer cells more susceptible to the planned therapy (Merlo et al., 2006; Basanta and Anderson, 2013). While not yet tested, therapies may be able to exploit aggregation effects. Much of the time, cancer cells, by detoxifying a therapy or by providing protective groups, provide a public good to each other. But, there is a flip side. If the resistance strategy involves pumping the still toxic agent into the interstitial fluid, then the cancer cells may engage in a game of “hot potato”; each is under selection to escalate the number of pumps or other membrane mechanisms for removing the toxin. It becomes an evolutionary race that is driven by natural selection but harmful to the entire population of cancer cells (Brown and Staňková, 2016).
In extinction therapy, the idea is to use a first strike set of drugs, radiation and/or surgery to render the remaining cancer cell population fragmented and dispersed in what may be undetectably small remnant populations. Rather than continuing these therapies, one immediately switches to second strike therapies aimed at “kicking the cancer while it's down.” The goal is to apply and then swiftly change up therapies before the cancer cells can evolve resistance. The second strike therapies may be older, out-of-favor drugs (Dinić et al., 2020) or part of the broader pool of current therapy options. They just need to be effective at continuing to drive the cancer cell populations to smaller and smaller sizes, and ideally offer diverse modes of action. The triple-drug overkill strategy used effectively for HIV treatment anticipates this sort of first-strike—second-strike therapies. Termed “pyramiding” (Palumbi, 2001), similar overkill strategies are utilized in pesticide management programs. These treatment strategies apply the maximum application possible, as the intent is curative or the elimination of the pest. If the curative intent is unrealistic, focusing on maintaining the disease/pest burden at acceptable levels becomes a better strategy and should involve less treatment (“minimum effective dose,” Cunningham, 2019).
Some of these evolutionary strategies have been successful at managing other evolving biological systems other than cancer. For example, Guiver et al. (2016) controlled pests by cycling between different pest management strategies. Acheson and Gardner (2014) successfully adopted evolutionary harvesting of lobsters, by releasing the smallest and the largest individuals. When targeting antibiotic resistance, Kim et al. (2014), Yoshida et al. (2017), and Imamovic and Sommer (2013) successfully slowed the evolution of a resistant strain by cycling between certain combinations of antibiotics.
It is important to note that an evolutionarily informed management strategy must consider and constantly update the eco-evolutionary state of the system under management. Hence, management differs from metronomic cancer treatments where predefined periods of treatment are punctuated by predefined drug holidays (Cunningham et al., 2018). The failure of metronomic regimens can be seen outside of the cancer domain. For example, when targeting viruses, applying more frequent but shorter predefined antibiotic courses favors the resistant strains (Blanquart et al., 2018). The most advanced evolutionary therapy optimizes treatment objectives with respect to the predefined treatment goals, adopting the Stackelberg evolutionary game theory approach (Staňková et al., 2019; Salvioli, 2020; Wölfl et al., 2020; Salvioli et al., 2021). If successful, this approach leads to the best possible evolutionary control.
6.4. Resistance as a Qualitative Trait
In our polymorphic model, the resistant subpopulation carries a “hurdle” of evolvability, as it starts at initially low resistance levels and may evolve to a high resistance rate. In that sense, a limiting case of our polymorphic model is the situation where the resistance trait evolves to the fitness maximum and resistance becomes a qualitative trait. There are multiple cancer models considering such a situation. For example, models of metastatic castrate-resistant prostate cancer consider three different types of cancer cells differing in their sensitivity to androgen deprivation and abiraterone acetate (Cunningham et al., 2011, 2018, 2020; You et al., 2017; Zhang et al., 2017). For these models, AT extends patient TTP. Similarly, Strobl et al. (2021) and Viossat and Noble (2021) demonstrated that AT extends TTP even without a resistance cost.
6.5. Different Forms of Cost of Resistance and Its Management
Typically, when a cost of resistance in cancer cells, pests, viruses or bacteria is considered/studied, it is assumed that resistance comes at a cost such as a decreased maximum growth rate. Xu et al. (2014), Kam et al. (2015) and Gallaher et al. (2018) showed that doxorubicin-resistant MCF-7/Dox breast cancer cells replicate slower compared to their sensitive counterparts. Almost a decade earlier, Andersson and Hughes (2010) demonstrated cost of resistance in laboratory experiments with different bacterial strains.
Salvioli (2020) considered how the cost of resistance decreases carrying capacity of cancer cells, focusing on equilibrium behavior instead of on the transient dynamics. Independently whether the cost of resistance impacts growth rate or carrying capacity of the evolving population, it becomes essential to introduce a resistance management plan that defines how resistance can be targeted (Staňková et al., 2019). Levy and Marshall (2004) focused on managing microbial resistance. They proposed tracking the frequency of resistant bacterial strains among patients, isolating hospitalized individuals with potentially dangerous resistant bacteria, and providing such patients with new therapeutic approaches to specifically target resistance. While the second suggestion may be difficult to follow when targeting treatment-induced resistance in cancer, the first and third suggestions cannot be followed as usefully in the treatment of cancer.
On the other hand, while in some cancers, cells may mutate from being resistant to being sensitive, reversibility of evolutionary traits is expected to be slow or non-existent elsewhere, as already shown for antibiotics (Andersson and Hughes, 2010), fish (Enberg et al., 2009), and pests (Mallet, 1989).
6.6. Future Research
In this research, we established a modeling protocol and partially answered the question “What is the best treatment choice based on the speed of evolution of resistance in cancer cells?”, listed as one the key questions of ecology and evolution of cancer (Dujon et al., 2020). We also identified the importance of knowing whether the resistance mechanism manifests as a quantitative trait in a monomorphic population or as a resistant vs. sensitive polymorphic population.
Here we focused on the impact of different modeling assumptions on the time to progression of MTD when compared to that of adaptive therapy with a pre-specified schedule. However, future work can include optimizing the treatment schedule for the models introduced here with respect to prespecified criteria, as has been done in other works (Martin et al., 1992; Carrére, 2017; Muros et al., 2017; Cunningham et al., 2018, 2020; Almeida et al., 2019; Gluzman et al., 2020; Wölfl et al., 2020).
Analysis of our modeling results are based on tumor burden. In many clinical instances neoadjuvant therapy such as surgery is applied to reduce tumor burden before or after drug exposure. Changing the population density could ultimately impact the better choice in therapeutic strategy. In an ideal situation, neoadjuvant therapy would reduce the tumor burden to a size such that the subsequent treatment is sufficient in removing all cancer cells. Our model can be used to explore different initial population sizes to analyze the consequences of neoadjuvant therapy. Also, we can use our model to explore timing between therapeutic strategies.
Our model can further be expanded to consider other tumor constituents related to tumor growth and progression. For example, the tumor microenvironment consists of fibroblasts, endothelial cells, immune cells, and non-cellular components that are not explicitly included in our model. Parameters in the model encompass what these cells and factors contribute to overall tumor dynamics. For example, cancer associated fibroblasts (CAFs) and tumor associated macrophages (TAMs) secrete proteins that promote angiogenesis and therefore could be included in the carrying capacity as the abundance of resources (blood vessels) place limits on tumor size. The parameters used in this work were chosen for illustrative purposes. Future research will focus on validating the model using patient data and on tailoring the model to describe specific types of cancer. Stochastic elements, such as mutation of cancer cells into cells of different type, may be included in our model as well. Moreover, future research on collectively evaluating evolutionary speed, the contexts of resistance evolution, and the role of costs of resistance, might profitably investigate how alternative evolutionary therapies, such as those stabilizing the tumor burden (Cunningham et al., 2020), double-bind therapies (Gatenby et al., 2009a), or those motivated by theories of extinction (Gatenby et al., 2019) compare to standard of care and the AT analyzed in this paper.
Data Availability Statement
The original contributions presented in the study are included in the article/Supplementary Material, further inquiries can be directed to the corresponding author/s.
Author Contributions
MP and MS carried out modeling. DL and CR put the results into the context of the large body of the literature. JB and KS managed the project and organized its different sub-tasks. All authors contributed to writing the manuscript and its final editing.
Funding
This research was supported by the European Union's Horizon 2020 research and innovation program under the Marie Sklodowska- Curie grant agreement No's 690817 and 955708, the Dutch National Foundation (NWO) Grant number OCENW.KLEIN.277, the James S. McDonnell Foundation grant, Cancer therapy: Perturbing a complex adaptive system, a V Foundation grant, NIH/National Cancer Institute (NCI) R01CA170595, Application of Evolutionary Principles to Maintain Cancer Control (PQ21), NIH/NCI U54CA143970-05 Physical Science Oncology Network (PSON) Cancer as a complex adaptive system, Alfred P. Sloan Foundation University Center of Exemplary Mentoring (UCEM) #G-2017-9717, and NSF Florida-Georgia Louis Alliance for Minority Participation (FGLSAMP) Bridge to the Doctorate Activity HRD #1906518. The funders had no role in study design, data collection and analysis, decision to publish, or preparation of the manuscript.
Conflict of Interest
The authors declare that the research was conducted in the absence of any commercial or financial relationships that could be construed as a potential conflict of interest.
Publisher's Note
All claims expressed in this article are solely those of the authors and do not necessarily represent those of their affiliated organizations, or those of the publisher, the editors and the reviewers. Any product that may be evaluated in this article, or claim that may be made by its manufacturer, is not guaranteed or endorsed by the publisher.
Supplementary Material
The Supplementary Material for this article can be found online at: https://www.frontiersin.org/articles/10.3389/fevo.2021.681121/full#supplementary-material
References
Acheson, J., and Gardner, R. (2014). Fishing failure and success in the gulf of maine: lobster and groundfish management. Marit. Stud. 13:8. doi: 10.1186/2212-9790-13-8
Aktipis, C. A., Kwan, V. S., Johnson, K. A., Neuberg, S. L., and Maley, C. C. (2011). Overlooking evolution: a systematic analysis of cancer relapse and therapeutic resistance research. PLoS ONE 6(11):e26100. doi: 10.1371/journal.pone.0026100
Alberti, M. (2016). Cities that Think Like Planets: Complexity, Resilience, and Innovation in Hybrid Ecosystems. Seattle, WA: University of Washington Press.
Allendorf, F. W., England, P. R., Luikart, G., Ritchie, P. A., and Ryman, N. (2008). Genetic effects of harvest on wild animal populations. Trends Ecol. Evol. 23, 327–337. doi: 10.1016/j.tree.2008.02.008
Allendorf, F. W., and Hard, J. J. (2009). Human-induced evolution caused by unnatural selection through harvest of wild animals. Proc. Natl. Acad. Sci. U.S.A. 106(Suppl 1), 9987–9994. doi: 10.1073/pnas.0901069106
Almeida, L., Bagnerini, P., Fabrini, G., Hughes, B. D., and Lorenzi, T. (2019). Evolution of cancer cell populations under cytotoxic therapy and treatment optimisation: insight from a phenotype-structured model. ESAIM Mathemat. Modell. Numer. Anal. 53, 1157–1190. doi: 10.1051/m2an/2019010
Andersson, D. I., and Hughes, D. (2010). Antibiotic resistance and its cost: is it possible to reverse resistance? Nat. Rev. Microbiol. 8, 260–271. doi: 10.1038/nrmicro2319
Bailey, J., and Conolly, A. (2000). Prize-winners to pariahs-a history of Japanese knotweed sl (polygonaceae) in the British Isles. Watsonia 23, 93–110. Available online at: http://archive.bsbi.org.uk/Wats23p93.pdf
Bailey, J. P., Bimová, K., and Mandák, B. (2009). Asexual spread versus sexual reproduction and evolution in Japanese knotweed sl sets the stage for the “battle of the clones”. Biol. Invas. 11, 1189–1203. doi: 10.1007/s10530-008-9381-4
Banta, J. A., and Richards, C. L. (2018). Quantitative epigenetics and evolution. Heredity 121, 210–224. doi: 10.1038/s41437-018-0114-x
Barrett, R. D., and Schluter, D. (2008). Adaptation from standing genetic variation. Trends Ecol. Evol. 23, 38–44. doi: 10.1016/j.tree.2007.09.008
Basanta, D., and Anderson, A. R. (2013). Exploiting ecological principles to better understand cancer progression and treatment. Interface Focus 3:20130020. doi: 10.1098/rsfs.2013.0020
Basanta, D., Gatenby, R. A., and Anderson, A. R. (2012). Exploiting evolution to treat drug resistance: combination therapy and the double bind. Mol. Pharmaceut. 9, 914–921. doi: 10.1021/mp200458e
Baucom, R. S. (2019). Evolutionary and ecological insights from herbicide-resistant weeds: what have we learned about plant adaptation, and what is left to uncover? N. Phytol. 223, 68–82. doi: 10.1111/nph.15723
Bayer, P., Gatenby, R. A., McDonald, P. H., Derek, K., Duckett, R., Staňková, K., et al. (2021). Coordination games in cancer. submitted. bioRxiv. doi: 10.1101/2021.06.22.449436
Beerling, D. J., Bailey, J. P., and Conolly, A. P. (1994). Fallopia japonica (houtt.) ronse decraene. J. Ecol. 82, 959–979. doi: 10.2307/2261459
Blanquart, F., Lehtinen, S., Lipsitch, M., and Fraser, C. (2018). The evolution of antibiotic resistance in a structured host population. J. R. Soc. Interface 15:20180040. doi: 10.1098/rsif.2018.0040
Bock, D. G., Caseys, C., Cousens, R. D., Hahn, M. A., Heredia, S. M., Hübner, S., et al. (2015). What we still don't know about invasion genetics. Mol. Ecol. 24, 2277–2297. doi: 10.1111/mec.13032
Bonduriansky, R., and Day, T. (2009). Nongenetic inheritance and its evolutionary implications. Annu. Rev. Ecol. Evol. Systemat. 40, 103–125. doi: 10.1146/annurev.ecolsys.39.110707.173441
Bonduriansky, R., and Day, T. (2018). Extended Heredity: A New Understanding of Inheritance and Evolution. Princeton, NJ: Princeton University Press.
Borowy, D., and Swan, C. M. (2020). A multi-trait comparison of an urban plant species pool reveals the importance of intraspecific trait variation and its influence on distinct functional responses to soil quality. Front. Ecol. Evol. 8:68. doi: 10.3389/fevo.2020.00068
Brown, J., Cunningham, J., and Gatenby, R. (2016). Aggregation effects and population-based dynamics as a source of therapy resistance in cancer. IEEE Trans. Biomed. Eng. 64, 512–518. doi: 10.1109/TBME.2016.2623564
Brown, J., and Staňková, K. (2017). Game theory as a conceptual framework for managing insect pests. Curr. Opin. Insect Sci. 21, 26–32. doi: 10.1016/j.cois.2017.05.007
Buckley, J., and Bridle, J. R. (2014). Loss of adaptive variation during evolutionary responses to climate change. Ecol. Lett. 17, 1316–1325. doi: 10.1111/ele.12340
Bushman, M., Antia, R., Udhayakumar, V., and de Roode, J. C. (2018). Within-host competition can delay evolution of drug resistance in malaria. PLoS Biol. 16:e2005712. doi: 10.1371/journal.pbio.2005712
Cairns, J. (1975). Mutation selection and the natural history of cancer. Nature 255, 197–200. doi: 10.1038/255197a0
Carrére, C. (2017). Optimization of an in vitro chemotherapy to avoid resistant tumours. J. Theoret. Biol. 413, 24–33. doi: 10.1016/j.jtbi.2016.11.009
Chabner, B. A., and Roberts, T. G. (2005). Chemotherapy and the war on cancer. Nat. Rev. Cancer 5, 65–72. doi: 10.1038/nrc1529
Chapuis, E., Lamy, T., Pointier, J.-P., Juillet, N., Ségard, A., Jarne, P., et al. (2017). Bioinvasion triggers rapid evolution of life histories in freshwater snails. Am. Nat. 190, 694–706. doi: 10.1086/693854
Cheptou, P.-O., Carrue, O., Rouifed, S., and Cantarel, A. (2008). Rapid evolution of seed dispersal in an urban environment in the weed crepis sancta. Proc. Natl. Acad. Sci. U.S.A. 105, 3796–3799. doi: 10.1073/pnas.0708446105
Coffey, D. S. (1998). Self-organization, complexity and chaos: the new biology for medicine. Nat. Med. 4, 882–885. doi: 10.1038/nm0898-882
Colautti, R. I., and Lau, J. A. (2015). Contemporary evolution during invasion: evidence for differentiation, natural selection, and local adaptation. Mol. Ecol. 24, 1999–2017. doi: 10.1111/mec.13162
Coldsnow, K. D., Mattes, B. M., Hintz, W. D., and Relyea, R. A. (2017). Rapid evolution of tolerance to road salt in zooplankton. Environ. Pollut. 222, 367–373. doi: 10.1016/j.envpol.2016.12.024
Collins, J. P., Kinzig, A., Grimm, N. B., Fagan, W. F., Hope, D., Wu, J., et al. (2000). A new urban ecology: modeling human communities as integral parts of ecosystems poses special problems for the development and testing of ecological theory. Am. Sci. 88, 416–425. doi: 10.1511/2000.5.416
Comont, D., Lowe, C., Hull, R., Crook, L., Hicks, H. L., Onkokesung, N., et al. (2020). Evolution of generalist resistance to herbicide mixtures reveals a trade-off in resistance management. Nat. Commun. 11, 1–9. doi: 10.1038/s41467-020-18079-3
Conover, D. O. (2000). Darwinian fishery science. Mar. Ecol. Prog. Ser. 208, 303–307. Available online at: https://www.jstor.org/stable/24863828
Conover, D. O., and Munch, S. B. (2002). Sustaining fisheries yields over evolutionary time scales. Science 297, 94–96. doi: 10.1126/science.1074085
Cortijo, S., Wardenaar, R., Colomé-Tatché, M., Gilly, A., Etcheverry, M., Labadie, K., et al. (2014). Mapping the epigenetic basis of complex traits. Science 343, 1145–1148. doi: 10.1126/science.1248127
Cunningham, J. (2019). A call for integrated metastatic management. Nat. Ecol. Evol. 3, 996–998. doi: 10.1038/s41559-019-0927-x
Cunningham, J. J., Brown, J. S., Gatenby, R. A., and Staňková, K. (2018). Optimal control to develop therapeutic strategies for metastatic castrate resistant prostate cancer. J. Theoret. Biol. 459, 67–78. doi: 10.1016/j.jtbi.2018.09.022
Cunningham, J. J., Gatenby, R. A., and Brown, J. S. (2011). Evolutionary dynamics in cancer therapy. Mol. Pharmaceut. 8, 2094–2100. doi: 10.1021/mp2002279
Cunningham, J. J., Thuijsman, F., Peeters, R., Viossat, Y., Brown, J. S., Gatenby, R. A., et al. (2020). Optimal control to reach eco-evolutionary stability in metastatic castrate resistant prostate cancer. PLoS ONE 15:e0243386. doi: 10.1371/journal.pone.0243386
De Groot, A. E., Roy, S., Brown, J. S., Pienta, K. J., and Amend, S. R. (2017). Revisiting seed and soil: examining the primary tumor and cancer cell foraging in metastasis. Mol. Cancer Res. 15, 361–370. doi: 10.1158/1541-7786.MCR-16-0436
Denholm, I., and Rowland, M. (1992). Tactics for managing pesticide resistance in arthropods: theory and practice. Annu. Rev. Entomol. 37, 91–112. doi: 10.1146/annurev.en.37.010192.000515
Diamond, S. E., Chick, L., Perez, A., Strickler, S. A., and Martin, R. A. (2017). Rapid evolution of ant thermal tolerance across an urban-rural temperature cline. Biol. J. Linnean Soc. 121, 248–257. doi: 10.1093/biolinnean/blw047
Dieckmann, U., and Law, R. (1996). The dynamical theory of coevolution: a derivation from stochastic ecological processes. J. Math. Biol. 34, 579–612. doi: 10.1007/s002850050022
Dinić, J., Efferth, T., Garcia-Sosa, A. T., Grahovac, J., Padrón, J. M., Pajeva, I., et al. (2020). Repurposing old drugs to fight multidrug resistant cancers. Drug Resist. 52:100713. doi: 10.1016/j.drup.2020.100713
Dlugosch, K. M., Anderson, S. R., Braasch, J., Cang, F. A., and Gillette, H. D. (2015). The devil is in the details: genetic variation in introduced populations and its contributions to invasion. Mol. Ecol. 24, 2095–2111. doi: 10.1111/mec.13183
Duesberg, P., Rausch, C., Rasnick, D., and Hehlmann, R. (1998). Genetic instability of cancer cells is proportional to their degree of aneuploidy. Proc. Natl. Acad. Sci. U.S.A. 95, 13692–13697. doi: 10.1073/pnas.95.23.13692
Dujon, A. M., Aktipis, A., Alix-Panabiéres, C., Amend, S. R., Boddy, A. M., Brown, J. S., et al. (2020). Identifying key questions in the ecology and evolution of cancer. Evol. Appl. 14, 877–892. doi: 10.1111/eva.13190
Enberg, K., Jørgensen, C., Dunlop, E. S., Heino, M., and Dieckmann, U. (2009). Implications of fisheries-induced evolution for stock rebuilding and recovery. Evol. Appl. 2, 394–414. doi: 10.1111/j.1752-4571.2009.00077.x
Estoup, A., Ravigné, V., Hufbauer, R., Vitalis, R., Gautier, M., and Facon, B. (2016). Is there a genetic paradox of biological invasion? Annu. Rev. Ecol. Evol. Syst. 47, 51–72. doi: 10.1146/annurev-ecolsys-121415-032116
Feinberg, A. P., Ohlsson, R., and Henikoff, S. (2006). The epigenetic progenitor origin of human cancer. Nat. Rev. Genet. 7, 21–33. doi: 10.1038/nrg1748
Fisher, R. (1930). The Genetical Theory of Natural Selection. Oxford, UK: Clarendon Press. doi: 10.5962/bhl.title.27468
Franks, S. J., Sim, S., and Weis, A. E. (2007). Rapid evolution of flowering time by an annual plant in response to a climate fluctuation. Proc. Natl. Acad. Sci. U.S.A. 104, 1278–1282. doi: 10.1073/pnas.0608379104
Fritts, T. H., and Rodda, G. H. (1998). The role of introduced species in the degradation of island ecosystems: a case history of guam. Annu. Rev. Ecol. Syst. 29, 113–140. doi: 10.1146/annurev.ecolsys.29.1.113
Fugére, V., and Hendry, A. P. (2018). Human influences on the strength of phenotypic selection. Proc. Natl. Acad. Sci. U.S.A. 115, 10070–10075. doi: 10.1073/pnas.1806013115
Galbreath, R., and Brown, D. (2004). The tale of the lighthouse-keeper's cat: discovery and extinction of the Stephens Island wren (Traversia lyalli). Notornis 51, 193–200. Available online at: http://notornis.osnz.org.nz/system/files/Notornis_51_4_193.pdf
Gallaher, J., Enriquez-Navas, P., Luddy, K., Gatenby, R., and Anderson, A. (2018). Spatial heterogeneity and evolutionary dynamics modulate time to recurrence in continuous and adaptive cancer therapies. Cancer Res. 78, 2127–2139. doi: 10.1158/0008-5472.CAN-17-2649
Gatenby, R. A. (2009). A change of strategy in the war on cancer. Nature 459:508. doi: 10.1038/459508a
Gatenby, R. A., Brown, J., and Vincent, T. (2009a). Lessons from applied ecology: cancer control using an evolutionary double bind. Cancer Res. 69, 7499–7502. doi: 10.1158/0008-5472.CAN-09-1354
Gatenby, R. A., and Brown, J. S. (2020a). The evolution and ecology of resistance in cancer therapy. Cold Spring Harbor Perspect. Med. 10:a040972. doi: 10.1101/cshperspect.a040972
Gatenby, R. A., and Brown, J. S. (2020b). Integrating evolutionary dynamics into cancer therapy. Nat. Rev. Clin. Oncol. 17, 675–686. doi: 10.1038/s41571-020-0411-1
Gatenby, R. A., Silva, A. S., Gillies, R. J., and Frieden, B. R. (2009b). Adaptive therapy. Cancer Res. 69, 4894–4903. doi: 10.1158/0008-5472.CAN-08-3658
Gatenby, R. A., Zhang, J., and Brown, J. S. (2019). First strike-second strike strategies in metastatic cancer: lessons from the evolutionary dynamics of extinction. Cancer Res. 79, 3174–3177. doi: 10.1158/0008-5472.CAN-19-0807
Geerts, A., Vanoverbeke, J., Vanschoenwinkel, B., Van Doorslaer, W., Feuchtmayr, H., Atkinson, D., et al. (2015). Rapid evolution of thermal tolerance in the water flea daphnia. Nat. Clim. Change 5, 665–668. doi: 10.1038/nclimate2628
Gerber, E., Krebs, C., Murrell, C., Moretti, M., Rocklin, R., and Schaffner, U. (2008). Exotic invasive knotweeds (Fallopia spp.) negatively affect native plant and invertebrate assemblages in european riparian habitats. Biol. Cons. 141, 646–654. doi: 10.1016/j.biocon.2007.12.009
Geritz, S. A., Mesze, G., Metz, J. A., et al. (1998). Evolutionarily singular strategies and the adaptive growth and branching of the evolutionary tree. Evol. Ecol. 12, 35–57. doi: 10.1023/A:1006554906681
Gerstung, M., Jolly, C., Leshchiner, I., Dentro, S. C., Gonzalez, S., Rosebrock, D., et al. (2020). The evolutionary history of 2,658 cancers. Nature 578, 122–128. doi: 10.1038/s41586-019-1907-7
Gluzman, M., Scott, J. G., and Vladimirsky, A. (2020). Optimizing adaptive cancer therapy: dynamic programming and evolutionary game theory. Proc. R. Soc. B. 287:20192454. doi: 10.1098/rspb.2019.2454
Greaves, M., and Maley, C. C. (2012). Clonal evolution in cancer. Nature 481, 306–313. doi: 10.1038/nature10762
Grimm, N. B., Faeth, S. H., Golubiewski, N. E., Redman, C. L., Wu, J., Bai, X., et al. (2008). Global change and the ecology of cities. Science 319, 756–760. doi: 10.1126/science.1150195
Grimsby, J. L., Tsirelson, D., Gammon, M. A., and Kesseli, R. (2007). Genetic diversity and clonal vs. sexual reproduction in Fallopia spp.(polygonaceae). Am. J. Bot. 94, 957–964. doi: 10.3732/ajb.94.6.957
Guiver, C., Edholm, C., Jin, Y., Mueller, M., Powell, J., Rebarber, R., et al. (2016). Simple adaptive control for positive linear systems with applications to pest management. SIAM J. Appl. Math. 76, 238–275. doi: 10.1137/140996926
Hairston, N. G. Jr., Ellner, S. P., Geber, M. A., Yoshida, T., and Fox, J. A. (2005). Rapid evolution and the convergence of ecological and evolutionary time. Ecol. Lett. 8, 1114–1127. doi: 10.1111/j.1461-0248.2005.00812.x
Hanahan, D., and Weinberg, R. (2011). Hallmarks of cancer: the next generation. Cell 144, 646–674. doi: 10.1016/j.cell.2011.02.013
Hanahan, D., and Weinberg, R. A. (2000). The hallmarks of cancer. Cell 100, 57–70. doi: 10.1016/S0092-8674(00)81683-9
Harris, S. E., Munshi-South, J., Obergfell, C., and O'Neill, R. (2013). Signatures of rapid evolution in urban and rural transcriptomes of white-footed mice (Peromyscus leucopus) in the New York metropolitan area. PLoS ONE 8:e74938. doi: 10.1371/journal.pone.0074938
Hastings, A., and Gross, L. (2012). Encyclopedia of Theoretical Ecology. Berkeley: University of California Press. doi: 10.1525/9780520951785
Hastings, I., and d'Alessandro, U. (2000). Modelling a predictable disaster:: the rise and spread of drug-resistant malaria. Parasitol. Tdy 16, 340–347. doi: 10.1016/S0169-4758(00)01707-5
Hawkins, N. J., Bass, C., Dixon, A., and Neve, P. (2019). The evolutionary origins of pesticide resistance. Biol. Rev. 94, 135–155. doi: 10.1111/brv.12440
Heino, M. (1998). Management of evolving fish stocks. Can. J. Fish. Aquat. Sci. 55, 1971–1982. doi: 10.1139/f98-089
Hendry, A., Gotanda, K., and Svensson, E. (2017). Human influences on evolution, and the ecological and societal consequences. Philos. Trans. R. Soc. Lond. Ser. B Bio. Sci. 372:1712. doi: 10.1098/rstb.2016.0028
Hendry, A. P., Farrugia, T. J., and Kinnison, M. T. (2008). Human influences on rates of phenotypic change in wild animal populations. Mol. Ecol. 17, 20–29. doi: 10.1111/j.1365-294X.2007.03428.x
Hoffmann, A. A., and Sgro, C. M. (2011). Climate change and evolutionary adaptation. Nature 470, 479–485. doi: 10.1038/nature09670
Huey, R. B., Gilchrist, G. W., Carlson, M. L., Berrigan, D., and Serra, L. (2000). Rapid evolution of a geographic cline in size in an introduced fly. Science 287, 308–309. doi: 10.1126/science.287.5451.308
Imamovic, L., and Sommer, M. O. (2013). Use of collateral sensitivity networks to design drug cycling protocols that avoid resistance development. Sci. Transl. Med. 5:204ra132. doi: 10.1126/scitranslmed.3006609
Jablonka, E., and Raz, G. (2009). Transgenerational epigenetic inheritance: prevalence, mechanisms, and implications for the study of heredity and evolution. Q. Rev. Biol. 84, 131–176. doi: 10.1086/598822
Jachmann, H., Berry, P., and Imae, H. (1995). Tusklessness in african elephants: a future trend. Afr. J. Ecol. 33, 230–235. doi: 10.1111/j.1365-2028.1995.tb00800.x
Johnson, M. T., and Munshi-South, J. (2017). Evolution of life in urban environments. Science 358:6363. doi: 10.1126/science.aam8327
Jorgensen, C., Enberg, K., Dunlop, E. S., Arlinghaus, R., Boukal, D. S., Brander, K., et al. (2007). Ecology-managing evolving fish stocks. Science 318, 1247–1248. doi: 10.1126/science.1148089
Kadoch, C., and Crabtree, G. R. (2015). Mammalian SWI/SNF chromatin remodeling complexes and cancer: Mechanistic insights gained from human genomics. Sci. Adv. 1:e1500447. doi: 10.1126/sciadv.1500447
Kam, Y., Das, T., Tian, H., Foroutan, P., Ruiz, E., Martinez, G., et al. (2015). Sweat but no gain: inhibiting proliferation of multidrug resistant cancer cells with “ersatzdroges”. Int. J. Cancer 136, E188–E196. doi: 10.1002/ijc.29158
Keller, E. F. (2014). From gene action to reactive genomes. J. Physiol. 592, 2423–2429. doi: 10.1113/jphysiol.2014.270991
Kim, E., Brown, J. S., Eroglu, Z., and Anderson, A. R. A. (2021). Adaptive therapy for metastatic melanoma: predictions from patient calibrated mathematical models. Cancers 13:823. doi: 10.3390/cancers13040823
Kim, S., Lieberman, T. D., and Kishony, R. (2014). Alternating antibiotic treatments constrain evolutionary paths to multidrug resistance. Proc. Natl. Acad. Sci. U.S.A. 111, 14494–14499. doi: 10.1073/pnas.1409800111
Klironomos, F. D., Berg, J., and Collins, S. (2013). How epigenetic mutations can affect genetic evolution: model and mechanism. Bioessays 35, 571–578. doi: 10.1002/bies.201200169
Kopp, M., and Matuszewski, S. (2014). Rapid evolution of quantitative traits: theoretical perspectives. Evol. Appl. 7, 169–191. doi: 10.1111/eva.12127
Kronholm, I., Bassett, A., Baulcombe, D., and Collins, S. (2017). Epigenetic and genetic contributions to adaptation in chlamydomonas. Mol. Biol. Evol. 34, 2285–2306. doi: 10.1093/molbev/msx166
Kronholm, I., and Collins, S. (2016). Epigenetic mutations can both help and hinder adaptive evolution. Mol. Ecol. 25, 1856–1868. doi: 10.1111/mec.13296
Kuester, A., Conner, J. K., Culley, T., and Baucom, R. S. (2014). How weeds emerge: a taxonomic and trait-based examination using united states data. N. Phytol. 202, 1055–1068. doi: 10.1111/nph.12698
Kuparinen, A., and Festa-Bianchet, M. (2017). Harvest-induced evolution: insights from aquatic and terrestrial systems. Philos. Trans. R. Soc. B Biol. Sci. 372:20160036. doi: 10.1098/rstb.2016.0036
Lambert, A. W., Pattabiraman, D. R., and Weinberg, R. A. (2017). Emerging biological principles of metastasis. Cell 168, 670–691. doi: 10.1016/j.cell.2016.11.037
Lankau, R. A. (2011). Rapid evolutionary change and the coexistence of species. Annu. Rev. Ecol. Evol. Systemat. 42, 335–354. doi: 10.1146/annurev-ecolsys-102710-145100
Laundré, J. W., Hernández, L., and Altendorf, K. B. (2001). Wolves, elk, and bison: reestablishing the “landscape of fear” in Yellowstone National Park, USA. Can. J. Zool/ 79, 1401–1409. doi: 10.1139/z01-094
Law, R. (2000). Fishing, selection, and phenotypic evolution. ICES J. Mar. Sci. 57, 659–668. doi: 10.1006/jmsc.2000.0731
Law, R. (2007). Fisheries-induced evolution: present status and future directions. Mar. Ecol. Prog. Ser. 335, 271–277. doi: 10.3354/meps335271
Ledón-Rettig, C. C., Richards, C. L., and Martin, L. B. (2013). Epigenetics for behavioral ecologists. Behav. Ecol. 24, 311–324. doi: 10.1093/beheco/ars145
Levy, S. B., and Marshall, B. (2004). Antibacterial resistance worldwide: causes, challenges and responses. Nat. Med. 10, S122–S129. doi: 10.1038/nm1145
Lorenzi, T., Chisholm, R. H., and Clairambault, J. (2016). Tracking the evolution of cancer cell populations through the mathematical lens of phenotype-structured equations. Biol. Direct 11, 1–17. doi: 10.1186/s13062-016-0143-4
Mallet, J. (1989). The evolution of insecticide resistance: have the insects won? Trends Ecol. Evol. 4, 336–340. doi: 10.1016/0169-5347(89)90088-8
Martin, R. B., Fisher, M. E., Minchin, R. F., and Teo, K. L. (1992). Optimal control of tumor size used to maximize survival time when cells are resistant to chemotherapy. Math. Biosci. 110, 201–219. doi: 10.1016/0025-5564(92)90038-X
Medway, D. G. (2004). The land bird fauna of Stephens Island, New Zealand in the early 1890s, and the cause of its demise. Notornis 51, 201–211. Available online at: http://notornis.osnz.org.nz/system/files/Notornis_51_4_201.pdf
Merlo, L. M., Pepper, J. W., Reid, B. J., and Maley, C. C. (2006). Cancer as an evolutionary and ecological process. Nat. Rev. Cancer 6, 924–935. doi: 10.1038/nrc2013
Metz, J. A., Geritz, S. A., Meszéna, G., Jacobs, F. J., and Van Heerwaarden, J. S. (1995). “Adaptive dynamics: a geometrical study of the consequences of nearly faithful reproduction,” in Stochastic and Spatial Structures of Dynamical Systems, Proceedings of the Royal Dutch Academy of Science (KNAW Verhandelingen) (Amsterdam), 183–231.
Mounger, J., Ainouche, M., Bossdorf, O., Cave-Radet, A., Li, B., Parepa, M., et al. (2021). Epigenetics and the success of invasive plants. Philos. Trans. R. Soc. B. 376:20200117. doi: 10.1098/rstb.2020.0117
Müller, G. B. (2017). Why an extended evolutionary synthesis is necessary. Interface Focus 7:20170015. doi: 10.1098/rsfs.2017.0015
Muros, F., Maestre, J., You, L., and Stankova, K. (2017). “Model predictive control for optimal treatment in a spatial cancer game,” in Proceedings of the 56th IEEE Conference on Decision and Control (Melbourne, VIC). doi: 10.1109/CDC.2017.8264481
Nowell, P. (1976). The clonal evolution of tumor cell populations. Science 194, 23–28. doi: 10.1126/science.959840
Olsen, E. M., Heino, M., Lilly, G. R., Morgan, M. J., Brattey, J., Ernande, B., et al. (2004). Maturation trends indicative of rapid evolution preceded the collapse of northern cod. Nature 428:932. doi: 10.1038/nature02430
Orlando, P., Gatenby, R., and Brown, J. (2012). Cancer treatment as a game: integrating evolutionary game theory into the optimal control of chemotherapy. Phys. Biol. 9:065007. doi: 10.1088/1478-3975/9/6/065007
Palumbi, S. R. (2001). Humans as the world's greatest evolutionary force. Science 293, 1786–1790. doi: 10.1126/science.293.5536.1786
Parmesan, C., Ryrholm, N., Stefanescu, C., Hill, J. K., Thomas, C. D., Descimon, H., et al. (1999). Poleward shifts in geographical ranges of butterfly species associated with regional warming. Nature 399, 579–583. doi: 10.1038/21181
Paun, O., Verhoeven, K. J., and Richards, C. L. (2019). Opportunities and limitations of reduced representation bisulfite sequencing in plant ecological epigenomics. N. Phytol. 221, 738–742. doi: 10.1111/nph.15388
Pepper, J. W., Scott Findlay, C., Kassen, R., Spencer, S. L., and Maley, C. C. (2009). Cancer research meets evolutionary biology. Evol. Appl. 2, 62–70. doi: 10.1111/j.1752-4571.2008.00063.x
Perkins, J. H. (1980). Boll weevil eradication. Science 207, 1044–1050. doi: 10.1126/science.207.4435.1044
Pienta, K. J., Hammarlund, E. U., Axelrod, R., Amend, S. R., and Brown, J. S. (2020). Convergent evolution, evolving evolvability, and the origins of lethal cancer. Mol. Cancer Res. 18, 801–810. doi: 10.1158/1541-7786.MCR-19-1158
Pigeon, G., Festa-Bianchet, M., Coltman, D. W., and Pelletier, F. (2016). Intense selective hunting leads to artificial evolution in horn size. Evol. Appl. 9, 521–530. doi: 10.1111/eva.12358
Pinsky, M. L., and Palumbi, S. R. (2014). Meta-analysis reveals lower genetic diversity in overfished populations. Mol. Ecol. 23, 29–39. doi: 10.1111/mec.12509
Pittendrigh, B., Olds, B., Yoon, K., Lee, S., Sun, W., and Clark, J. (2013). The genomics of human lice: from the genome to the potential for future control strategies. Pesticide Biochem. Physiol. 106, 172–176. doi: 10.1016/j.pestbp.2013.03.010
Reed, D. R., Metts, J., Pressley, M., Fridley, B. L., Hayashi, M., Isakoff, M. S., et al. (2020). An evolutionary framework for treating pediatric sarcomas. Cancer 126, 2577–2587. doi: 10.1002/cncr.32777
Reznick, D. N., Losos, J., and Travis, J. (2019). From low to high gear: there has been a paradigm shift in our understanding of evolution. Ecol. Lett. 22, 233–244. doi: 10.1111/ele.13189
Richards, C. L., Alonso, C., Becker, C., Bossdorf, O., Bucher, E., Colomé-Tatché, M., et al. (2017). Ecological plant epigenetics: evidence from model and non-model species, and the way forward. Ecol. Lett. 20, 1576–1590. doi: 10.1101/130708
Richards, C. L., Bossdorf, O., Muth, N. Z., Gurevitch, J., and Pigliucci, M. (2006). Jack of all trades, master of some? On the role of phenotypic plasticity in plant invasions. Ecol. Lett. 9, 981–993. doi: 10.1111/j.1461-0248.2006.00950.x
Richards, C. L., Bossdorf, O., and Pigliucci, M. (2010). What role does heritable epigenetic variation play in phenotypic evolution? Bioscience 60, 232–237. doi: 10.1525/bio.2010.60.3.9
Richards, C. L., and Pigliucci, M. (2020). Epigenetic inheritance. A decade into the extended evolutionary synthesis. Paradigmi 38, 463–494. doi: 10.30460/99624
Richards, C. L., Schrey, A. W., and Pigliucci, M. (2012). Invasion of diverse habitats by few japanese knotweed genotypes is correlated with epigenetic differentiation. Ecol. Lett. 15, 1016–1025. doi: 10.1111/j.1461-0248.2012.01824.x
Richards, C. L., Walls, R. L., Bailey, J. P., Parameswaran, R., George, T., and Pigliucci, M. (2008). Plasticity in salt tolerance traits allows for invasion of novel habitat by Japanese knotweed sl (Fallopia japonica and f. × bohemica, polygonaceae). Am. J. Bot. 95, 931–942. doi: 10.3732/ajb.2007364
Robinson, D. A., and Enright, M. C. (2003). Evolutionary models of the emergence of methicillin-resistant staphylococcus aureus. Antimicrob. Agents Chemother. 47, 3926–3934. doi: 10.1128/AAC.47.12.3926-3934.2003
Rollins, L. A., Richardson, M. F., and Shine, R. (2015). A genetic perspective on rapid evolution in cane toads (Rhinella marina). Mol. Ecol. 24, 2264–2276. doi: 10.1111/mec.13184
Rudman, S. M., Kreitzman, M., Chan, K. M., and Schluter, D. (2017). Evosystem services: rapid evolution and the provision of ecosystem services. Trends Ecol. Evol. 32, 403–415. doi: 10.1016/j.tree.2017.02.019
Salvioli, M. (2020). Game theory for improving medical decisions and managing biological systems (Ph.D. thesis). Politecnico di Milano, Milano, Italy.
Salvioli, M., Dubbeldam, J., Staňková, K., and Brown, J. S. (2021). Fisheries management as a Stackelberg evolutionary game: finding an evolutionarily enlightened strategy. PLoS ONE 16:e0245255. doi: 10.1371/journal.pone.0245255
Sandoval-Motta, S., and Aldana, M. (2016). Adaptive resistance to antibiotics in bacteria: a systems biology perspective. Wiley Interdiscipl. Rev. Syst. Biol. Med. 8, 253–267. doi: 10.1002/wsbm.1335
Savidge, J. A. (1987). Extinction of an island forest avifauna by an introduced snake. Ecology 68, 660–668. doi: 10.2307/1938471
Scott, N., Ataide, R., Wilson, D. P., Hellard, M., Price, R. N., Simpson, J. A., et al. (2018). Implications of population-level immunity for the emergence of artemisinin-resistant malaria: a mathematical model. Malaria J. 17, 1–11. doi: 10.1186/s12936-018-2418-y
Selechnik, D., Richardson, M. F., Shine, R., DeVore, J. L., Ducatez, S., and Rollins, L. A. (2019). Increased adaptive variation despite reduced overall genetic diversity in a rapidly adapting invader. Front. Genet. 10:1221. doi: 10.3389/fgene.2019.01221
Shipman, M. (2017). The Boll Weevil War, or How Farmers and Scientists Saved Cotton in the South. Available online at: https://news.ncsu.edu/2017/05/boll-weevil-war-2017/ (accessed March 12, 2021).
Staňková, K., Brown, J. S., Dalton, W. S., and Gatenby, R. A. (2019). Optimizing cancer treatment using game theory: a review. JAMA Oncol. 5, 96–103. doi: 10.1001/jamaoncol.2018.3395
Stapley, J., Santure, A. W., and Dennis, S. R. (2015). Transposable elements as agents of rapid adaptation may explain the genetic paradox of invasive species. Mol. Ecol. 24, 2241–2252. doi: 10.1111/mec.13089
Stoltzfus, A. (2017). Why we don't want another “synthesis”. Biol. Direct 12, 1–12. doi: 10.1186/s13062-017-0194-1
Strobl, M. A., Gallaher, J., West, J., Robertson-Tessi, M., Maini, P. K., and Anderson, A. (2020). Spatial structure impacts adaptive therapy by shaping intra-tumoral competition. bioRxiv. doi: 10.1101/2020.11.03.365163
Strobl, M. A., West, J., Viossat, Y., Damaghi, M., Robertson-Tessi, M., Brown, J. S., et al. (2021). Turnover modulates the need for a cost of resistance in adaptive therapy. Cancer Res. 81, 1135–1147. doi: 10.1158/0008-5472.CAN-20-0806
Sullivan, A. P., Bird, D. W., and Perry, G. H. (2017). Human behaviour as a long-term ecological driver of non-human evolution. Nat. Ecol. Evol. 1, 1–11. doi: 10.1038/s41559-016-0065
Sun, X., Bao, J., and Shao, Y. (2016). Mathematical modeling of therapy-induced cancer drug resistance: connecting cancer mechanisms to population survival rates. Sci. Rep. 6, 1–12. doi: 10.1038/srep22498
Sutter, D. A., Suski, C. D., Philipp, D. P., Klefoth, T., Wahl, D. H., Kersten, P., et al. (2012). Recreational fishing selectively captures individuals with the highest fitness potential. Proc. Natl. Acad. Sci. U.S.A. 109, 20960–20965. doi: 10.1073/pnas.1212536109
Tewari, S., Leskey, T. C., Nielsen, A. L., Piñero, J. C., and Rodriguez-Saona, C. R. (2014). “Use of pheromones in insect pest management, with special attention to weevil pheromones,” in Integrated Pest Management ed D. P. Abrol (New York, NY: Elsevier), 141–168. doi: 10.1016/B978-0-12-398529-3.00010-5
Timp, W., and Feinberg, A. P. (2013). Cancer as a dysregulated epigenome allowing cellular growth advantage at the expense of the host. Nat. Rev. Cancer 13, 497–510. doi: 10.1038/nrc3486
Turner, K. G., Hufbauer, R. A., and Rieseberg, L. H. (2014). Rapid evolution of an invasive weed. N. Phytol. 202, 309–321. doi: 10.1111/nph.12634
van Kleunen, M., Bossdorf, O., and Dawson, W. (2018). The ecology and evolution of alien plants. Annu. Rev. Ecol. Evol. Systemat. 49, 25–47. doi: 10.1146/annurev-ecolsys-110617-062654
Vandepitte, K., De Meyer, T., Helsen, K., Van Acker, K., Roldán-Ruiz, I., Mergeay, J., et al. (2014). Rapid genetic adaptation precedes the spread of an exotic plant species. Mol. Ecol. 23, 2157–2164. doi: 10.1111/mec.12683
Ventola, C. L. (2015). The antibiotic resistance crisis: part 1: causes and threats. P T Peer Review. J. Formul. Manage. 40, 277–283.
Vincent, T. L., and Brown, J. S. (2005). Evolutionary Game Theory, Natural Selection, and Darwinian Dynamics. New York, NY: Cambridge University Press. doi: 10.1017/CBO9780511542633
Viossat, Y., and Noble, R. (2021). A theoretical analysis of tumour containment. Nat. Ecol. Evol. 5, 826–835. doi: 10.1038/s41559-021-01428-w
Weiner, J. (1995). The Beak of the Finch: A Story of Evolution in Our Time. New York, NY: Vintage Books.
Weisz, R., Fleischer, S., and Smilowitz, Z. (1996). Site-specific integrated pest management for high-value crops: impact on potato pest management. J. Econ. Entomol. 89, 501–509. doi: 10.1093/jee/89.2.501
Wellems, T. E., and Plowe, C. V. (2001). Chloroquine-resistant malaria. J. Infect. Dis. 184, 770–776. doi: 10.1086/322858
West, J., Ma, Y., and Newton, P. K. (2018). Capitalizing on competition: an evolutionary model of competitive release in metastatic castration resistant prostate cancer treatment. J. Theoret. Biol. 455, 249–260. doi: 10.1016/j.jtbi.2018.07.028
West-Eberhard, M. J. (1989). Phenotypic plasticity and the origins of diversity. Annu. Rev. Ecol. Systemat. 20, 249–278. doi: 10.1146/annurev.es.20.110189.001341
Whitney, K. D., and Gabler, C. A. (2008). Rapid evolution in introduced species, “invasive traits” and recipient communities: challenges for predicting invasive potential. Divers. Distribut. 14, 569–580. doi: 10.1111/j.1472-4642.2008.00473.x
Wiles, G. J., Bart, J., Beck, R. E. Jr., and Aguon, C. F. (2003). Impacts of the brown tree snake: patterns of decline and species persistence in Guam's Avifauna. Conserv. Biol. 17, 1350–1360. doi: 10.1046/j.1523-1739.2003.01526.x
Wölfl, B., te Rietmole, H., Salvioli, M., Kaznatchev, A., Thuijsman, F., Brown, J. S., et al. (2020). The contribution of evolutionary game theory to understanding and treating cancer. medRxiv. doi: 10.1101/2020.12.02.20241703
Wrubel, R. P., and Gressel, J. (1994). Are herbicide mixtures useful for delaying the rapid evolution of resistance? A case study. Weed Technol. 8, 635–648. doi: 10.1017/S0890037X00039828
Xu, H. B., Shen, Z. L., Fu, J., and Xu, L. Z. (2014). Reversal of doxorubicin resistance by guggulsterone of commiphora mukul in vivo. Phytomedicine 21, 1221–1229. doi: 10.1016/j.phymed.2014.06.003
Yeh, P. J. (2004). Rapid evolution of a sexually selected trait following population establishment in a novel habitat. Evolution 58, 166–174. doi: 10.1111/j.0014-3820.2004.tb01583.x
Yoshida, M., Reyes, S. G., Tsuda, S., Horinouchi, T., Furusawa, C., and Cronin, L. (2017). Time-programmable drug dosing allows the manipulation, suppression and reversal of antibiotic drug resistance in vitro. Nat. Commun. 8, 1–11. doi: 10.1038/ncomms15589
You, L., Brown, J. S., Thuijsman, F., Cunningham, J. J., Gatenby, R. A., Zhang, J., et al. (2017). Spatial vs. non-spatial eco-evolutionary dynamics in a tumor growth model. J. Theoret. Biol. 435, 78–97. doi: 10.1016/j.jtbi.2017.08.022
You, L., von Knobloch, M., Lopez, T., Peschen, V., Radcliffe, S., Koshy Sam, P., et al. (2019). Including blood vasculature into a game-theoretic model of cancer dynamics. Games 10:13. doi: 10.3390/g10010013
Zhang, J., Cunningham, J. J., Brown, J. S., and Gatenby, R. A. (2017). Integrating evolutionary dynamics into treatment of metastatic castrate-resistant prostate cancer. Nat. Commun. 8:1816. doi: 10.1038/s41467-017-01968-5
Keywords: metastatic cancer, adaptive therapy, evolutionary speed, resistance, game theory, Stackelberg evolutionary game
Citation: Pressley M, Salvioli M, Lewis DB, Richards CL, Brown JS and Staňková K (2021) Evolutionary Dynamics of Treatment-Induced Resistance in Cancer Informs Understanding of Rapid Evolution in Natural Systems. Front. Ecol. Evol. 9:681121. doi: 10.3389/fevo.2021.681121
Received: 15 March 2021; Accepted: 23 June 2021;
Published: 06 August 2021.
Edited by:
Frederick R. Adler, The University of Utah, United StatesReviewed by:
Mark Chaplain, University of St Andrews, United KingdomChiara Villa, University of St Andrews, United Kingdom, in collaboration with reviewer MC
Christopher Carroll, Lund University, Sweden
Copyright © 2021 Pressley, Salvioli, Lewis, Richards, Brown and Staňková. This is an open-access article distributed under the terms of the Creative Commons Attribution License (CC BY). The use, distribution or reproduction in other forums is permitted, provided the original author(s) and the copyright owner(s) are credited and that the original publication in this journal is cited, in accordance with accepted academic practice. No use, distribution or reproduction is permitted which does not comply with these terms.
*Correspondence: Kateřina Staňková, k.stankova@tudelft.nl
†These authors share first authorship