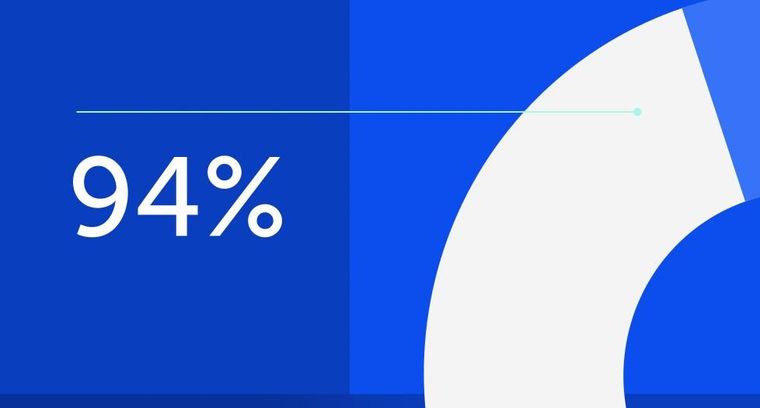
94% of researchers rate our articles as excellent or good
Learn more about the work of our research integrity team to safeguard the quality of each article we publish.
Find out more
REVIEW article
Front. Ecol. Evol., 08 July 2021
Sec. Behavioral and Evolutionary Ecology
Volume 9 - 2021 | https://doi.org/10.3389/fevo.2021.678533
This article is part of the Research TopicFrom Ecology to Cancer Biology and Back AgainView all 18 articles
All biological systems depend on signals for coordination: signals which pass information among agents that run the gamut from cells to organisms. However, their very importance makes signals vulnerable to subversion. How can a receiver know whether a signal is honest or deceptive? In other words, are signals necessarily a reliable indicator of agent quality or need? By drawing parallels to ecological phenomena ranging from begging by nestlings to social insects, we investigate the role of signal degradation in cancer. We thus think of cancer as a form of corruption, in which cells command huge resource investment through relatively cheap signals, just as relatively small bribes can leverage large profits. We discuss various mechanisms which prevent deceptive signaling in the natural world and within tissues. We show how cancers evolve ways to escape these controls and relate these back to evasion mechanisms in ecology. We next introduce two related concepts, co-option and collusion, and show how they play critical roles in ecology and cancer. Drawing on public policy, we propose new approaches to view treatment based on taxation, changing the incentive structure, and the recognition of corrupted signaling networks.
In an influential 1996 speech, the President of World Bank, James Wolfensohn, described corruption as a cancer standing in the way of equitable development (Wolfensohn, 2005). Without giving any biological details, he used the term “cancer” to stand in generically for a bad thing that will expand if uncontrolled and is difficult to root out. In response, he prescribed what can be seen as an international version of precision medicine: specific homegrown solutions for each country, complemented by support from the World Bank for anticorruption fighters and withdrawal of international support from corrupt governments.
In economic contexts, corruption is often defined as “the abuse of public office for private gain” (Wedel, 2012), with the focus on the use of government offices with control of a limited resource to demand bribes or tariffs from a second party (Levin and Tsirik, 1998) but can be extended to include corruption such as embezzlement involving only a single individual (Boisvert et al., 2014). Mathematical analysis began with Rose-Ackerman (Rose-Ackerman, 1975) who studied bribes using game theory and emphasized the role of information availability and reliability. Despite some special cases where corruption might “grease the wheels of commerce” by correcting distorted markets, the effects of corruption are widely agreed to be destructive (Wei, 1999), as argued by Wolfensohn. Corruption concentrates wealth and power in the hands of those with access, reducing resources for the environment and the poor (Joly, 2017).
The causes of corruption are perhaps as complex as those of cancer, involving internal forces that parallel growth and mutation, outside influences like colonialism that parallel carcinogens or oncogenic viruses, and a history that parallels how these forces develop in a tumor (Wedel, 2012). Incompetence can create supply gaps to be filled by corruption, excessive or ambiguous regulation might promote their evasion, recessions can increase need and increase incentives for corruption, and low pay or education of government officials might increase temptation (Levin and Tsirik, 1998). Corruption occurs within a complex social system, just as cancer occurs within the tightly knit environment of a tissue, and is promoted by a lack of transparency and accountability (Levin and Tsirik, 1998), but also by the very social pressures that make societies function (Kolokoltsov and Malafeyev, 2017). Once established, corruption can spread like a contagion (Nekovee and Pinto, 2019) or, of course, like a cancer.
Direct approaches to combating corruption address these causes by promoting greater transparency or increasing pay and training (Levin and Tsirik, 1998). More systemic approaches look to institutions. The benefit of modular network structures that can contain corruption in more isolated cells must be balanced against the risk that isolated units could receive less oversight (Luna-Pla and Nicolás-Carlock, 2020). In some cases, disrupting the structure of established corruption networks might even improve their functioning (Duijn et al., 2014). Fighting corruption, like fighting cancer, must thus deal with the Law of Unintended Consequences (Fisman and Golden, 2017). As anyone who watches crime movies knows, the most difficult corruption to detect and control goes all the way to the top, a modern variant of the ancient question “But who will guard the guardians?” (Hurwicz, 2007).
Here, we ask whether the metaphor of corruption as a cancer can be inverted, and whether thinking of cancer as corruption might provide new approaches to treatment. This thinking seeks to extend the many ideas derived from cancer ecology (Pienta et al., 2008; Gatenby et al., 2009; Korolev et al., 2014; Kareva, 2015) to a behavioral ecology framework that sees cancer as a breakdown of the reliability of signaling and information transfer needed to coordinate complex biological systems.
Coordination of complex biological systems, from cells and tissues to societies, depends on reliable communication. This communication underlies behaviors ranging from the intricate intracellular signaling cascades that coordinate cell growth, movement, and division (Alberts et al., 2014) to the “ballerina dances” that male birds-of-paradise use to court females (Wilts et al., 2014). Communication requires signals, pieces of information transmitted from a signaler to a receiver (Table 1 provides precise definitions of key terms used throughout this paper). Signals can be chemical (cytokines in the immune system), aural (begging calls of nestlings), or visual (skin coloring of venomous snakes or body movements of courting birds). Following (Otte, 1974), we define a signal as a trait that plays an adaptive role through conveying information to other organisms. Traits like body size do convey information, but we treat as signals only when modified to alter perception through structures like frills (Shine, 1990). We discuss the blurry line between physical constraints and signals later.
Communication depends on the response of the signal receiver. When a signal is reliable, the receiver can accurately ascertain information about the state of the signaler. Drawing upon (Searcy and Nowicki, 2005), we classify a signal as reliable if: (1) a characteristic of the signal is consistently correlated with some attribute of the signaler or environment and (2) if receivers gain some benefit from having information about the attribute. For instance, if the call of a male frog is consistently correlated with its size and if knowing the size of male frogs allows females to choose appropriate mates, the male frog call is reliable. We define deception functionally, obviating the need to assume cognitive underpinnings for deceptive behavior (Hauser, 1996). Again, following (Searcy and Nowicki, 2005), we define deception as when (1) a receiver registers Y from a signaler, (2) the receiver responds in a manner that benefits the signaler and is appropriate if Y means X, but (3) it is not true that X is the case. For example, in great tits (Møller, 2010) and many other birds, alarm calls are sometimes given in the absence of predators to induce competitors to move away from a food source.
More broadly, deception can be categorized into three classes: exaggeration (use of a signal that differs from the corresponding condition), lies (use of the wrong signal), or withholding information (not signaling when appropriate) (Vehrencamp, 2009). Each of these is context-dependent. For example, if every frog were to sing as if it were larger than its true size, receivers could adjust their interpretation to accurately assess them, canceling the effects of exaggeration and making deception a function of the social environment. In this context, we think of corruption as being of the network of communication itself, such as when a government official conceals information to demand bribes for access. One can think of counterfeit money as a deceptive signal of value that corrupts the trust in the “legal tender” upon which the economy depends. Before taking on the broader concept of corruption, we focus on the mechanisms by which deception is deterred in ecology and in tissues. We then describe how cancers can evolve to evade these checks and relate these strategies to those used in ecology. Finally, we describe how viewing cancer through the lens of corruption, combined with appropriate mathematical models, can inspire new treatment strategies.
Deception degrades the reliability of the communication system, endangering the coordination on which complex systems depend. The fable of The Boy Who Cried Wolf provides a proverbial example. However, conflicts of interest between signallers and receivers can favor degrading reliability, as with alarm calls. How then can biological systems maintain the integrity of communication (Searcy and Nowicki, 2005; Vehrencamp, 2009)?
We review key mechanisms from behavioral ecology and discuss their application to tissues. First, and perhaps most obvious, biological systems can reduce or remove conflicts of interest (Krakauer and Pagel, 1996). The high relatedness of sterile worker ants within a colony and the segregation of germ and somatic tissue play similar roles: individual ants or cells gain no benefit from behaviors that fail to aid the centralized reproduction of the collective. However, the ability of many worker ants to lay unfertilized male eggs creates such a conflict, creating a challenge for colony functioning (Monnin and Ratnieks, 2001). Avoiding such genetic conflicts within the nest favors loss of kin recognition and the use of colony odor to distinguish nestmates from non-nestmates (Lenoir et al., 2001). To avoid attack, workers emerge with minimal odor, and are tolerated by older workers while they come to smell like their colony. Slave-making ants use this necessary acceptance of ambiguous workers to take over a colony. A queen from a slave-making species can infiltrate a host colony, kill the existing queen, and lay her own eggs to be taken care of by the accepting workers (Buschinger, 2009).
In evolutionary ecology, costly signaling is perhaps the best-known deterrence mechanism; a signal constrained in this way is called a handicap signal (Vehrencamp, 2000). With condition-dependent handicaps, low quality individuals pay a greater cost to signal than high quality individuals. If the benefit for a given signal intensity is identical for all signalers, the optimal signaling level that maximizes the difference between signal benefit and cost will be greater for high quality individuals (Figure 1) (Grafen, 1990).
Figure 1. Lower signaling costs support higher signal intensities for high quality signalers (inspired by Johnstone, 1977). The black curve represents the fitness benefit of signaling for a signaler. The blue curve represents the fitness cost of signaling for a low quality signaler: the cost of signaling increases rapidly with signal intensity. The red curve represents the fitness cost of signaling for a high quality signaler: the cost of signaling increases more gradually with signal intensity. Signalers will signal at an intensity that maximizes the difference between benefits and costs (indicated by blue and red stars). Because the cost curve is less steep for high quality signalers, the intersection point will occur at a higher signal intensity.
Ecologists have established many cases of this mechanism. Territorial song by male birds is an energetically expensive and time-consuming signal of physical state and territory quality. Males with higher quality territories have more access to food, and thus pay a lower cost to sing more frequently because they need to dedicate less time to foraging. Experiments across bird species have confirmed this prediction by modifying food availability in male territories and quantifying changes in song frequency (Hoi-Leitner et al., 1995; Manica et al., 2014). For females, this signal then conveys reliable information about which males can provide the best access to food and potentially greater investment in offspring care (Rytkonen et al., 1997). Signals play a key role in the other component of sexual selection, male-male competition. In red deer, energetically costly roaring contests use muscles and actions similar to those used in fighting and thus provide reliable information to assess male fighting condition (Clutton-Brock and Albon, 1979). In our own bodies, cells may also use costly signals to display their phenotypic state to other cells in competitive environments. During development of the neural system and of eggs, for example, costly signals identify the healthiest cells that should be maintained for the good of the body despite the lack of a conflict of interest (Krakauer and Pagel, 1996; Madan et al., 2018).
Costly signals of need follow a similar logic if the greatest benefits accrue to those with the greatest need. If all signalers pay the same cost to signal but differ in their benefit curves, we expect the signaler with the higher benefit curve to maximize fitness by signaling at a higher intensity (Figure 2). Birds provide the well-studied example of begging by nestlings. A starving nestling should receive a greater benefit from a morsel of food than a well-fed nestling and will thus beg more intensely from its parents who receive a reliable signal of need. Experimental manipulation of hunger by artificial feeding or short-term food deprivation in a variety of bird species has shown that begging reliably signals short-term need (Sacchi, 2002; Watson and Ritchison, 2018), with birds that were deprived of food begging at significantly higher rates than those that were not (Cotton et al., 1996; Kilner et al., 1999). In our cells, a similar low benefit mechanism may help suppress uncontrolled cell proliferation. Cells require growth factors to divide, and can signal for these dependent on need. The larger array of internal controls, including cell cycle checkpoints (Kastan and Bartek, 2004; Barnum and O'Connell, 2014), programmed cell death (Elmore, 2007; Fuchs and Steller, 2011), and oncogene-induced senescence (Gorgoulis and Halazonetis, 2010; Zhu et al., 2020) mean that the effects of growth factors saturate. Like a fully fed nestling, a cell that takes up massive amounts of growth factor would grow no faster, removing selection for deceptive signaling.
Figure 2. Higher signaling benefits support higher signal intensities for needy signalers (inspired by Johnstone, 1977). The black curve represents the fitness cost of signaling for signalers. The blue curve represents the fitness benefit of signaling for a low need signaler: the benefits of signaling quickly saturate with signal intensity. The red curve represents the fitness benefit of signaling for a high need signaler: the benefit of signaling saturates more gradually with signal intensity. Signalers will signal at an intensity that maximizes the difference between cost and benefits (indicated by blue and red stars). Because the benefit curve saturates less quickly for high need signalers, the intersection point will occur at a higher signal intensity.
These cost-benefit mechanisms are complemented by enforcement. We thus distinguish the energetic or production costs characteristic of condition-dependent handicaps from externally imposed costs through punishment or retaliation. With repeated interactions, if receivers can identify and remember the sources of deceptive signals, they can discriminate against them in the future and reduce or reverse the benefits of deception (Searcy and Nowicki, 2005). This individually directed skepticism can enforce signal reliability because the benefit a signaler receives from one deceptive interaction can be outweighed by the cost of lower receiver responses in the future (Maynard-Smith, 1991; Silk et al., 2000). As we have seen, some animals use false alarm calls to lure competitors away from food. In an experiment with Richardson's ground squirrels, when the alarm calls of one squirrel were consistently paired with the approach of a badger and the calls of another squirrel were not, receiver squirrels displayed much higher levels of vigilance to the alarms of the honest squirrel than to the dishonest one (Hare and Atkins, 2001). Similar results have been seen in vervet monkeys (Cheney and Seyfarth, 1988) and food calls in domestic chickens (Evans and Evans, 2002). One could speculate that the lack of alarm calls in the gregarious zebra finch could be the endpoint of this breakdown, where birds have abandoned such calls to be replaced by visual cues (Butler et al., 2017).
Instead of removing the benefit of deception, receivers could directly retaliate against deceptive signalers. For example, the highly variable black clypeus patterns on the female paper wasp Polistes dominulus strongly predict body size and social dominance (Tibbetts and Dale, 2004). Specifically, wasps with no marks or single marks are less aggressive and defer to the more aggressive wasps that have “broken” facial patterns (Clark and Kimbrough, 2017). However, the reliability of this signal is constantly tested in contests between wasps. Experimental results show that wasps with experimentally altered clypeus patterns (deceptive signalers) received considerbly more aggression from rivals and were less able to establish dominance relationships, suggesting that a mismatch between signal and state causes social punishment (Tibbetts and Dale, 2004; Tibbetts and Izzo, 2010). The constant testing of host tissues for self-antigens such as MHC and proteins like decay-accelerating factor that regulates the complement system quickly identifies any cells that fail to provide appropriate signals. Immunological memory serves as a form of repeated interaction. When a tissue is exposed to an antigen on several occasions, the host produces memory B and T cells that enable a more rapid and effective adaptive immune response to subsequent antigen insults (Janeway et al., 2001).
Most simply, physical constraints can prevent deceptive signaling (Vehrencamp, 2000) if signal intensity is tightly correlated with the quality being signaled, and cannot be faked (Maynard-Smith and Harper, 2003). For example, the frequency of vocalization depends on the size of vocal folds in vertebrates, which in turn depends on body size. This size-frequency allometry has been experimentally confirmed across many mammalian species, from primates to carnivores (Morton, 1977; Schmidt-Nielsen, 1984; Bowling et al., 2017). If body size is a marker for male quality, females can use vocalization frequency signals to choose the best mate (Glaudas et al., 2020). Similarly, song repertoire size in great reed warblers is an index of age, with older males having a greater repertoire size (Hasselquist, 1994). However, no correlation is perfect, and any trait that allows a signaler to sound larger without being larger would be favored, leading to a shift in the whole signal. In the context of tissue homeostasis, pathogens may carry signals (pathogen-associated molecular patterns or PAMPs) as seemingly essential parts of their physical structure, such as LPS in the cell wall of gram negative bacteria or a production of double-stranded RNA during replication of most RNA viruses (Maverakis et al., 2015). These PAMPS are reliably recognized by innate mechanisms of the immune system, such as toll-like receptors (TLRs), triggering an immune response. (Kumar et al., 2011). Of course, pathogens have no interest in signaling their presence, and have evolved many ways to modify or conceal these signals. Similarly, as we will see, cancer cells may modify their cell surface markers to hide from immune cells. More simply, pathogens, by definition, damage their hosts and this physical damage creates an unambiguous signal. A summary of these prevention mechanisms and examples in ecology and tissue homeostasis is captured in Table 2.
When signaling systems include conflicts of interest between signalers and receivers, the reliability of communication is in constant flux. Cancers emerge by corrupting these control measures perhaps first and foremost by creating a conflict of interest within a tissue. Even when control begins to unravel, as seems inevitable with aging (Martincorena et al., 2015), mechanisms based on costs, benefits, repeated interactions, enforcement, and constraints can maintain effective communication. But breakdown of the systems through systematic deception or evasion is always possible. In this section, we outline the ways cancers have evolved to partially or completely circumvent each of these deterrence mechanisms, and relate these evasion strategies back to ecology.
We begin by proposing that evasion of high cost and low benefit mechanisms is central to the rise of corruption in cancer. For costs, we propose that there are three main types: internal regulation, external enforcement, and energetic costs. Internal regulation refers to internal controls that cells have to govern proliferation such as cell-cycle checkpoints and oncogene-induced senescence. External enforcement describes interactions of cancer cells with other agents in their microenvironment, most notably immune cells. Energetic costs refer to the actual costs cells incur to grow and divide, including ATP, pathway intermediates, and synthesis of critical molecules. We here focus on the energetic costs of cells and consider internal regulation and external enforcement later. Assume there are two agents identical in quality, but differing in the cost of signaling (Figure 1). The individual with a lower signaling cost would be expected to signal at higher intensities and benefit from this cost differential in a deceptive fashion. Similarly, if one individual receives a higher benefit from signaling, we expect this individual to signal at a higher intensity (Johnstone, 1977; Grafen, 1990). In cancer, we hypothesize that both of these cases occur, resulting in an “exaggeration” evasion mechanism.
In order for a cell to divide or secrete growth factors for example, the coordination and contribution of a multitude of signaling components are needed. These components are tightly regulated through both environmental and intracellular factors to ensure that the cell does not divide at an inappropriate time. However, in cancer, due to the presence of oncogenes, these signaling components can be overexpressed or constitutively active, removing the need to meet certain intracellular or extracellular conditions and leading to uncontrolled levels of proliferation. For example, MYC codes for c-Myc, which induces cellular proliferation (Dang, 2012; Tansey, 2014). Normally, it becomes activated upon receiving mitogenic signals like serum stimulation, Wnt, Shh, or EGF via the MAPK/ERK pathway (Campisi et al., 1984). However, in many cancers, most notably in Burkitt's lymphoma, Myc is constitutively expressed. This removes the need for the cells to receive external serum stimulation or expend energy to produce these signals, leading to increased expression of many downstream genes that govern cell proliferation (Finver et al., 1988). Similar situations occur with NFκB and STAT3, key players in cell proliferation, apoptosis, migration, and angiogenesis. Normally, these are activated by a plethora of cytokines and growth factors; however, due to constitutive activation in cancer, the cells do not need to invest in the production of these cytokines and growth factors to activate them, reducing the cost of proliferation, movement, and blood vessel recruitment (Garcia et al., 2001; Nagel et al., 2014). More generally, cancer cells seem to operate with a greater degree of modularity; rather than having tightly integrated gene signaling networks, pathways may act more independently of each other, and thus avoiding some of the energetic costs involved in growth and division.
Quite generally, cancers escape by removing the controls that create saturating benefits. When cells lose tumor suppressors such as Rb, they can grow more quickly for a given amount of nutrient or growth factor, while a normal cell would be prevented from dividing too quickly. Cancer cells that acquire mutations to signal at higher intensities would then grow more quickly, creating selection for deception (Vehrencamp, 2009). One can see these cancer cells as exaggerating their phenotypic state and need for growth and division factors. Cancer cells can also evade saturating benefits by modifying their metabolic pathways. Most cancers exhibit the Warburg effect, the use of glycolysis rather than oxidative phosphorylation to generate ATP (Liberti and Locasale, 2016). Although glycolysis is less efficient at generating ATP, it creates more intermediates for biosynthetic and anabolic purposes (e.g., through the pentose phosphate pathway) and greater metabolic flexibility (e.g., catabolism of macromolecules) when nutrients are limited (Vander Heiden et al., 2009). This production of proteins, amino acids, and lipids is crucial for proliferation. Unlike normal cells whose proliferation is partially constrained, intrinsically and extrinsically, by the rate at which they can produce molecules needed for growth and division, cancer cells can rapidly produce those needed molecules. One example of subversion of enforcement of honesty through the saturating benefit mechanism in ecology, cowbirds lay their eggs in the nests of other often smaller birds, and continue to beg and grow far beyond their hapless nestmates (Dearborn, 1998).
Even the most tightly knit societies, such as social insects, have interaction intensity vastly exceeded by that of cells in a body. Due to the huge number of cells in the body or in a tumor, individual cells are not encountered multiple times and remembered. However, the whole tumor does present novel antigens in the context of damage, triggering an immune response. Cancers capitalize on one essential feature of this response, T cell exhaustion. If the immune system indefinitely attacked every repeated challenge, autoimmunity would be almost unavoidable in the face of low level inflammation. When faced with slow-growing tumors, immune cells interact repeatedly with antigens on the surface of cancer cells, promoting the progressive loss of function of effector T cells (Wherry, 2011; Schietinger and Greenberg, 2014). In addition, when chronically exposed to antigen, tumor-specific T cells develop an increased expression of many inhibitory receptors such as PD-1 and CTLA-4 and an altered cellular metabolic and transcriptomic profile. As a result, these T cells have lowered proliferation, effector cytokine secretion, and cytolytic activity, aiding tumor-immune escape (Jiang et al., 2015; Catakovic et al., 2017; Zhang et al., 2020). Tumor cells amplify these natural controls in at least two ways, first by reducing expression of class I MHC (Vinay et al., 2015) (a form of deception through withholding information) and through production or induction of production of immune suppressing signals like PDL1 (Cha et al., 2019). As described earlier, slave-making ants and certain beetles capitalize on the tolerance workers show to bland-smelling intruders (Geiselhardt et al., 2007).
Finally, cancers find ways to evade even the physical constraints that prevent deception. Normally, immune cells circulate through the body, detect, and destroy malignant or premalignant cells in a process called immune surveillance (Swann and Smyth, 2007). They do so by identifying tumor-specific antigens that are present on a cancer cell's surface, which triggers killing of the cancer cell through such mechanisms as release of cytotoxic molecules like granzymes and perforin (Tsukumo and Yasutomo, 2018). Tumors use many signal corrupting mechanisms to avoid the immune system, including the release of immunosuppressive cytokines (Seliger, 2005) and recruiting regulatory T cell function (Zindl and Chaplin, 2010). Cancer cells can also mask their identity through the modification of antigen presentation (Vinay et al., 2015). In particular, cancer cells can downregulate MHCI expression (the molecule used to present antigens to the immune system) or lack the requisite costimulatory molecules for antigen presentation. In this way, the cancer cell becomes invisible to the T cells, which cannot recognize it as “non-self.”
Cancer cells can also gain a competitive advantage over neighboring cells by modifying their expression of certain membrane proteins. Cell selection based on “fitness fingerprints” is used in development and maintenance to identify and eliminate cells with low fitness relative to their neighboring cells (Madan et al., 2018). The membrane bound protein Flower has different isoforms termed “Win” and “Lose” to signal cell quality. Cells that express high levels of Lose isoforms are marked as low fitness and, if surrounded by cells expressing high levels of Win isoforms, are eliminated (Madan et al., 2019). This process allows the body to delay aging (Merino et al., 2015), prevent developmental malformation (Merino et al., 2013, 2015), and replace old tissues during regeneration (Moreno et al., 2015). However, this cell selection mechanism can be hijacked by premalignant cancer cells, which upregulate Win isoform expression regardless of cell quality, to gain a competitive advantage at the expense of neighboring stromal cells that express the Lose isoform, increasing the cancer cell's proliferative and metastatic potential (Madan et al., 2019).
Cancer cells avoid a different physical constraint through the loss of contact inhibition. Non-cancerous cells initiate cell cycle arrest and reduce proliferation and mitogen signaling pathways when cellular density is too high and cells are in contact with each other, regardless of their cellular metabolism or extracellular factors (Levine et al., 1965). However, this response is weakened in cancer cells, allowing them to proliferate uncontrollably and grow on top of each other, leading to the high density characteristic of solid tumors (Pavel et al., 2018). We summarize of the evasion strategies associated with each of these prevention mechanisms along with examples in ecology and cancer in Table 3.
Two related concepts enhance the danger of cancer corruption: co-option and collusion. co-option means diverting resources or assistance in roles different from usual, and instead adopting them for one's own sake. Cancer cells can co-opt normal cells in the tumor microenvironment to work for them. Viewing cancer as “the wound that never heals” (Hua and Bergers, 2019) reveals this process: the chronic inflammation that often accompanies cancer progression brings with it a variety of inflammatory agents which can lead to the infiltration and activation of different myeloid cells such as macrophages that contribute to growth (Schmid and Varner, 2012; Stegelmeier et al., 2019). Thus, the physical damage that should be an unambiguous signal of danger is not concealed but is instead turned to the tumor's own advantage. As part of this process, some cancer cells release cytokines that promote polarization of nearby immune cells to a pro-tumor role, dampening the anti-tumor immune response and stimulating cancer cell survival and proliferation (Cheng et al., 2019; Strauss et al., 2020). Immune cells can promote cancer progression through an “angiogenic switch” by producing proteases, proteins that break down the extracellular matrix, that in turn can activate latent molecules to drive angiogenesis (Ribatti et al., 2007; Baeriswyl and Christofori, 2009). Furthermore, recent evidence in the context of breast cancer suggests that metastatic cancer cells can induce regression of normal non-cancerous cells in their local environment into a stem-like state, further promoting tumorigenesis (Ombrato et al., 2019). In ecology, some forms of sexual selection co-opt prior preferences, such as those of female birds for brightly-colored fruit, to create attractive males (Ryan et al., 1990). As we have seen, brood parasitism provides an example of how one species co-opts another. Brood parasites like cuckoos and cowbirds manipulate a host to care for their offspring, leaving them with time and energy to spend on feeding and producing more offspring (Dearborn, 1998). Egg-dumping is common within species, where individuals again co-opt the parental care instincts of others (Yom-Tov, 1980).
When corruption becomes systemic, multiple individuals can work together in complementary roles and collude to garner resources and subvert the signaling environment. Cancer cells, both within and among tumors, can “collude” by exchanging information, such as RNA, DNA, and proteins, through exosomes and other mechanisms (Li et al., 2006; Hough et al., 2017; Maziveyi et al., 2019). Although far from fully understood, the proteins, metabolites, and nucleic acids delivered in this way are thought to facilitate survival, differentiation, and proliferation, promote angiogenesis and wound healing, contribute to metastasis and migration, and reprogram metabolic profiles of receiving cells (Kalluri and LeBleu, 2020). For example, cancer cells in hypoxic conditions secrete exosomes with increased angiogenic and metastatic potential to engineer a more favorable environment or move to a new one (Park et al., 2010). Exosomes from tumor cells mediate the metastasis of cancer to distant organs through uptake by resident cells that prepare the pre-metastatic niche (Hoshino et al., 2015). Poly-aneuploid cancer cells (PACCs) are a recently discovered form of collusion in cancer. During times of microenvironmental stress, aneuploid cancer cells can fuse together to form PACCs, entering a state of quiescence or reversible therapy-induced senescence to protect their genome and avoid apoptosis (Pienta et al., 2021). Due to their high levels of genomic content, PACCs that enter the cell cycle and divide into non-polyploid cells can produce new phenotypic variants of cancer cells that contribute to cancer heterogeneity and lethality (Bukkuri et al., under review; Bukkuri et al., under preparation).
In ecology, “collusion” is usually seen as cooperation that does not subvert the existing order, such as food sharing among vampire bats (Carter and Wilkinson, 2013). Coalitions of males working together to oust an existing leader is perhaps closer to the human sense of the term, but hardly subverts an already violent social order (De Waal and Waal, 2007). The “dear enemy” effect, where neighboring territory owners cooperate by reducing aggression (Temeles, 1994) is not a breakdown of the territorial system itself, but a modification through cooperation that can even enhance defense against intruders (Detto et al., 2010). One could view sexual reproduction as a form of genetic collusion. Similar to the mixing of genetic material in the PACC state through cell fusion that produces increased heritable variation, many asexually reproducing species engage in sexual recombination when under stress, ranging from the crustacean Daphnia magna that produces males and sexual eggs when facing high population density, starvation, or bacterial infection (Kleiven et al., 1992; Mitchell et al., 2004) to the perennial herb Trifolium repens that increases investment in sexual reproduction when subject to herbivory. As an interesting parallel with cancer, this response was observed solely in sensitive plants and not resistant ones (Griffiths and Bonser, 2013). This view of cancer as a corruption of the signaling system aligns remarkably well, although not perfectly, with the established hallmarks of cancer (Hanahan and Weinberg, 2011) (Table 4).
Does viewing cancer through the lens of signaling and corruption help us design treatments? Our goal is to re-establish the broken control system, either through some form of punishment (the stick) in parallel with strengthening enforcement mechanisms, or restoring the incentive structure created by costs and benefits of signaling so that the corrupt behavior is no longer beneficial (the carrot).
One way to abolish corruption is by punishing corrupt behavior directly, including regulation and taxation. Regulation takes many forms in the body, both within and across cells, such as the immune system detecting cancer cells by their novel antigens and destroying them. Immunotherapies, drugs that boost the immune system, can restore regulation often weakened through deceptive signals by the cancer. In this section, we focus instead on taxation. In society, taxes are a tool of the public sector to guide behavior in socially preferred ways, which has no direct parallel in the self-organized and decentralized body. We here think of taxation as having been guided by natural selection that has evolved policies to control corrupt actors much as laws and societies constantly develop and learn to achieve the same ends. In both cases, corrupt actors almost by definition are not playing by the rules, and can find ways to change their strategy to evade or subvert the strategies, as the constant effort to suppress new forms of tax fraud illustrates. However, such fraud requires altered behaviors, such as hiring clever lawyers and fixers, that create inefficiencies that parallel the costs of developing drug resistance.
We begin with two brief examples of the unintended consequences of anti-corruption efforts in public policy (Fisman and Golden, 2017). Due to rampant cheating on high school exit exams in Romania, security cameras were introduced to monitor students and teachers. Although cheating overall was reduced, the policy disproportionately impacted the poorer students because more affluent students were able to bribe the enforcers individually without having to engage in more detectable collusion like poorer students (Borcan et al., 2017). In Ghana, the placement of observers at select polling locations did succeed in reducing fraud at these locations, fraudulent activity increased in neighboring, unobserved polling locations. These examples illustrate two key points: (1) anti-corruption measures can disproportionately impact certain groups and (2) anti-corruption efforts are always under threat of subversion by corrupt actors who find a way to modify their behavior to evade them.
A related challenge arises with the use of targeted therapies to treat cancer. Targeted therapies, from monoclonal antibodies to small molecule inhibitors, have been at the forefront of the precision medicine revolution, promising effective treatments tailored to each patient's unique genetic profile. In contrast to chemotherapy that affects all rapidly dividing normal and cancerous cells, targeted therapies attack pathways specifically associated with the patient's cancer. This reduces side effects and should be more potent than standard chemotherapy. Like specific anti-corruption policies however, targeted therapies only attack a specific form of corruption, and cancer cells can modify their corruption strategy through mutation or plasticity to avoid effects of the drug, creating an opportunity to evade the treatment. In a simple graphical model, an untreated cancer might grow most quickly with a particular pathway tuned to an intermediate value (Figure 3). Chemotherapy (blue line) reduces growth of all cells below that needed for replacement (dashed gray line). Targeted therapy (red line) lowers growth of the most rapidly growing phenotype even further, but leaves a window of escape for corrupt cells.
Figure 3. Hypothetical fitness landscape in response to therapy. The black curve represents the fitness of the cancer cells in the absence of therapy, which is maximized for an intermediate value of some pathway trait. Targeted therapy, represented by the red curve, greatly diminishes the fitness of cancer cells with intermediate trait values. However, in so doing, it leaves room for cancer cells with more extreme trait values to survive. The blue curve depicts the effects of chemotherapy, which broadly reduces the fitness of all cells below a critical threshold, although not as dramatically as the targeted therapy on cells with intermediate trait values.
As an example, trastuzumab is a targeted therapy for HER2+ breast cancer (Slamon et al., 2001). Despite being initially highly effective, the majority of patients still experience disease progression within 1 year (Ellis and Hicklin, 2009). This resistance emerges through a disturbingly wide array of mechanisms: (1) mutation of the HER2 target to prevent binding of the drug, (2) upregulation of downstream signaling pathways, (3) upregulation of alternate growth factor signaling pathways, and (4) inhibition of immune-mediated mechanisms (Pohlmann et al., 2009). Imatinib was one of the first targeted therapies, proving highly effective against BCR-ABL, a gene highly overexpressed in almost every case of chronic myeloid leukemia (Ellis and Hicklin, 2009). In this case, resistance could be caused by (1) amplification of the BCR-ABL target, (2) mutations in the BCR-ABL domain to prevent binding of the drug, and (3) the emergence of BCR-ABL independent pathways for signal transduction (Milojkovic and Apperley, 2009). Although targeted therapies can more efficiently kill cancer cells while sparing healthy cells, they disproportionately affect sensitive cells and provide opportunities for evolution of new strategies that bypass the drug's target molecule. Traditional chemotherapy which simply targets rapidly dividing cells may thus be more effective at keeping up with the cancer cell's evolution, recalling Haldane's wonderful remark “It is much easier for a mouse to get a set of genes which enable it to resist Bacillus typhimurium than a set which enable it to resist cats” (Lederberg, 1999).
In this context, we propose viewing cancer treatment as a form of taxation. In order for cells to continue to survive and proliferate under treatment, they must pay some cost, or tax, by developing a mechanism of drug resistance. This can take forms that include spending energy to upregulate production of the target molecule or utilizing a less energetically efficient signaling pathway to grow and divide. In economic terms, targeted therapies act on elastic goods, goods that can be easily replaced by alternatives if prices rise. Because targeted therapies focus on one small aspect of complex, multi-agent signaling pathways, cancer cells can evade the tax by shifting to an alternative. In contrast, standard chemotherapies affect all rapidly dividing cells, agnostic of the specific form of corruption of cancer cells. Thus, cancer cells are left with the option of dividing less, exactly as we hope, or mutating to defend against the drug's effects, such as through drug anti-porters (Lage et al., 1999; Tawbi and Buch, 2010; Jiang et al., 2011), defective apoptotic pathways (Bedikian et al., 2006), or the upregulation of survival signals (Lev et al., 2003). To continue our economic analogy, chemotherapy acts on inelastic goods, such as gasoline, whose consumption does not change much as a function of price. Rather than switching to an alternate pathway, standard chemotherapy forces cells to pay a price to continue using the same pathways for growth and division. A narrowly targeted tax is more effective when it works, but is easier to evade, while a broad tax affects the whole economy but is more difficult to avoid. With cancer, these arguments for and against traditional chemotherapy or targeted therapies depend on how quickly the cancer can evolve or alter behavior to escape treatment (Bukkuri et al., under review).
An alternative approach to prevent corruption changes the incentive structure to remove the benefits of corrupt behavior. In cancer, there are many ways to change the incentive structure to reduce the benefit of rapid proliferation. We discuss three approaches: oncogene-induced senescence (OIS), traditional chemotherapy, and the sucker's gambit. Although OIS can play both pro- and anti-tumor roles (Gorgoulis and Halazonetis, 2010; Liu et al., 2018), we focus on its role in suppressing excessive cell proliferation by arresting the cell cycle upon recognition of aberrant oncogenic signaling (Zhu et al., 2020). This effectively removes, or even reverses, any incentive to divide faster. This layer of control must be weakened by mutation or aging before oncogenes are selectively favored, and therapies that could restore or replace these controls could thus obviate the growth advantage of cancer cells. Chemotherapy that targets rapidly dividing cells provides a crude way to replace these controls, but at the cost of significant off-target side effects and evolution of resistance. The most explicit therapeutic use of this approach is the sucker's gambit (Merlo et al., 2006), which changes the selection pressures and incentive structures to select for phenotypes which are easier to treat. For example, increasing the concentration of glucose in a microenvironment changes the underlying incentive structure to favor cancer cells with high levels of GLUT1 receptors. Following this, administering glucose starvation or GLUT1 inhibitor treatment can force these cells to pay high and sometimes lethal costs for the production and maintenance of these receptors (Bukkuri and Brown, under review). Because cancer cells are short-sighted, successive administration of therapies that impose opposite evolutionary selection pressures on cancer cells can be effective. In ecology, conservation biologists seek to avoid “ecological traps,” where species choose poor habitats when faced by novel species or habitat modifications (Schlaepfer et al., 2002), but such traps could tempt unwanted species and help with their control.
Treatment necessarily alters the benefit structure, and ideally can be used to sucker cancers into traps. One goal of modern therapies is to weaken the benefits of evolving resistance or evasion of therapy. We see analogies to education and public policy realms. Campbell's law states that the more a quantitative social marker is used for social decision making, the more it becomes subject to corruption that distorts the very social process it is intended to monitor (Campbell, 1979). For example, standardized testing can provide valuable information on student performace, but only when teaching is aimed at general competence. However, these quantitative measures soon became goals of the teaching process, subject to corruption that can actively degrade learning (Campbell, 1979). Schools and teachers face immense pressures to produce high test scores, particularly when tied to funding and bonuses (Nichols and Berliner, 2007), leading to “teaching to the test” (Popham, 2001) and elimination of subjects like social studies, music, foreign languages, and art from curricula (Byrd and Varga, 2018). These high stakes tests promote cheating, as discussed in the last subsection (Nichols and Berliner, 2007), and high-priced preparatory classes taken by students from more privileged backgrounds (Alon, 2009; Buchmann et al., 2010). Alternatives include more individualized assessments like portfolios (Kamenetz, 2015). We propose that the way that we assess cancers and choose treatments might be susceptible to Campbell's law. What if cancers start “growing to the test” and conceal their true size or state because it evades our treatment, almost the same way that cancer can evade immune responses?
If thought of narrowly as bribery, corruption provides a poor model for cancer. However, we argue that corruption is less about transfer of resources and more about breaking the communication system and disrupting the reliability of communication. In this sense, corruption is a violation of public trust (Wedel, 2012), the trust in signal integrity that any complex system relies on for coordination.
Evolutionary ecologists have identified five mechanisms that maintain the integrity of signaling systems: reduction of conflict of interest, costly signaling, saturating benefits, enforcement, and physical constraints. Each of these is paralleled in the body, and thus must be degraded by a surviving cancer. We propose examples of each of these mechanisms in ecology and in the body, and how they can be subverted. This approach provides an alternative view of the hallmarks of cancer.
We think this view proposes several directions for therapy, all of course building on prior work and ideas. First, rather than focusing on a single corrupted signal, we could use comprehensive approaches to recognize cancer through disrupted signaling (Krakauer and Pagel, 1996). Potentially dangerous lying, for example, can be recognized through the “too many details” that liars pile on to convince themselves and others (De Becker and Stechschulte, 1997). Cancer cells do not send off a carefully orchestrated set of consistent signals, but a welter of chemical noise that could be recognized through its very incoherence (Sur et al., 2019). From this recognition, we might be able to find ways to treat the resulting corruption of the communication network. The disappointing performance of VEGF inhibitors in effectively controlling cancer as monotherapy (Comunanza and Bussolino, 2017), for example, could reflect the challenge of placing such signal-disrupting therapies in the full context of the network.
By viewing cancer therapy as a public policy problem, we propose two main ways to combat corruption in our body: punishing corruption directly (the stick), changing the incentive structure so corrupt behavior is not favored (the carrot). As punishment, we focus on treatment as a form of taxation, showing how the very specificity and effectiveness of targeted therapies might make them subject to escape: the cancer equivalent of tax evasion. We advocate for careful consideration of the evolvability of the cancer when deciding whether to administer traditional chemotherapy or targeted therapies. In the case of altering the incentive structure, we describe how oncogene-induced senescence and traditional chemotherapy can change the incentives for cancer cells to proliferate rapidly. We highlight how a sucker's gambit therapeutic strategy can combine these two approaches, tempting cancers with a carrot and then slamming them with a stick to promote maximal therapeutic efficacy.
Cancer treatment might benefit from other lessons from the challenges of fighting corruption in the economic and political spheres. Wedel (Wedel, 2012) describes the history of the anticorruption movement that emerged at the end of the cold war and found expression in Wolfensohn's speech to the World Bank in 1996. Of its four central assumptions, we find it remarkable that three (except for the focus on public rather than private sector corruption) have close parallels with cancer treatment, and propose that questioning these assumptions might provide new guidance for treatment.
1. Corruption happens to “the Other.” Anticorruption efforts generally focus on distant nations with very different cultures from the centers of economic power. Not only do those of us fortunate enough to not have faced cancer tend to think “it can't happen to me,” but one can imagine that the body itself sees an incipient cancer as happening elsewhere, rather than permeating the entire system. Treatment that revives the control mechanisms throughout the body, including sites of potential metastases, could stop the spread of cancers.
2. Corruption is about bribery to individuals, often at lower levels, rather than the system, and is illegal. Cancer treatment focuses on cells within the tumor itself, and on ways that cancers “break the rules” rather than on how they reshape the body at all levels. Like much corruption, such as campaign contributions, what cancers do is perfectly legal, and focusing on consequences and mechanisms of corruption could be more effective than a limited set of broken rules.
3. Corruption can be measured. This simple assumption reflects the famous saying by business management guru Peter Drucker, “If you can't measure it, you can't improve it.” Indices, often derived from polls of business and political leaders with their own biases, make corruption easy to publicize in the media and compare across countries. As we have seen, indices are subject to Campbell's Law, effectively corrupting the evaluation mechanism itself. Treatments based on indices like specific biomarkers are subject to the same logic, favoring tumors that evade the evaluation mechanism itself (Staňková et al., 2019). More flexible cancer treatments can anticipate the evasion that can emerge when we use a specific marker to trigger treatment.
These approaches to corruption depend on the complexity of the signaling system. Ants in small colonies with individual foragers require and have fewer layers of control than those with extensive division of labor, just as smaller organisms like mice require and have fewer layers of cancer control than humans and elephants. We expect all aspects of control, ranging from costs, benefits, and enforcement to the modular structure of networks to also differ. It is possible that the challenges of extrapolating treatments from mice to humans are due as much to differences in these structural aspects as they are to differences in molecular details.
A different sort of modification of network functioning emerges with aging. The simultaneous degradation of signaling and of the full set of control systems likely causes the rapid increase of most cancers with age (DeGregori, 2018), and our treatments need to reflect this slow corruption of the integrity of the system. The danger of corruption in increasingly entrenched bureaucracies could reflect a similar process. As institutions develop into ever more complicated structures, corruption itself may become more unequal because only the privileged and well-connected can even figure out how to be corrupt.
In the long run, these general ideas need to be made concrete with mathematical models that build on the literature of corruption (Rose-Ackerman, 1975) and take a more comprehensive view of cancer that includes resources, signaling, and enforcement mechanisms in a framework that generates unexpected novelty (Adler and Gordon, 2019). When these models are linked to specific cancers, mechanisms, and treatments, they can be used to propose improved approaches to therapy that seek to restore balance to the whole patient.
AB and FA contributed to the conceptualization, writing, simulations, and editing of this paper. Both authors contributed to the article and approved the submitted version.
AB acknowledges support by the National Science Foundation Graduate Research Fellowship Program under Grant No. 1746051. FA acknowledges support from NIH-CSBC: U54: Combating subclonal evolution of resistant cancer phenotypes (U54 CA209978).
Any opinions, findings, and conclusions or recommendations expressed in this material are those of the author and do not necessarily reflect the views of the National Science Foundation.
The authors declare that the research was conducted in the absence of any commercial or financial relationships that could be construed as a potential conflict of interest.
The authors thank Elizabeth Fedak and members of sLaM for useful insights and citations.
Adler, F. R., and Gordon, D. M. (2019). Cancer ecology and evolution: positive interactions and system vulnerability. Curr. Opin. Syst. Biol. 17, 1–7. doi: 10.1016/j.coisb.2019.09.001
Alberts, B., Johnson, A. D., Lewis, J., Morgan, D., Raff, M., Roberts, K., et al. (2014). Molecular Biology of the Cell, 6th Edn. New York, NY: W. W. Norton & Company.
Alon, S. (2009). The evolution of class inequality in higher education. Am. Sociol. Rev. 74, 731–755. doi: 10.1177/000312240907400503
Baeriswyl, V., and Christofori, G. (2009). The angiogenic switch in carcinogenesis. Semin. Cancer Biol. 19, 329–337. doi: 10.1016/j.semcancer.2009.05.003
Barnum, K. J., and O'Connell, M. J. (2014). Cell cycle regulation by checkpoints. Methods Mol. Biol. 1170, 29–40. doi: 10.1007/978-1-4939-0888-2_2
Bedikian, A. Y., Millward, M., Pehamberger, H., Conry, R., Gore, M., Trefzer, U., et al. (2006). BCL-2 antisense (oblimersen sodium) plus dacarbazine in patients with advanced melanoma: the oblimersen melanoma study group. J. Clin. Oncol. 24, 4738–4745. doi: 10.1200/JCO.2006.06.0483
Boisvert, A.-M. L., Dent, P., and Quraishi, O. B. (2014). Corruption in canada: definitions and enforcement. Res. Brief 48, 1–3.
Borcan, O., Lindahl, M., and Mitrut, A. (2017). Fighting corruption in education: what works and who benefits? Am. Econ. J. Econ. Policy 9, 180–209. doi: 10.1257/pol.20150074
Bowling, D. L., Garcia, M., Dunn, J. C., Ruprecht, R., Stewart, A., Frommolt, K. H., et al. (2017). Body size and vocalization in primates and carnivores. Sci. Rep. 7, 1–11. doi: 10.1038/srep41070
Buchmann, C., Condron, D. J., and Roscigno, V. J. (2010). Shadow education, american style: test preparation, the SAT and college enrollment. Social Forces 89, 435–461. doi: 10.1353/sof.2010.0105
Buschinger, A. (2009). Social parasitism among ants: a review (hymenoptera: Formicidae). Myrmecol. News 12, 219–235.
Butler, N. E., Magrath, R. D., and Peters, R. A. (2017). Lack of alarm calls in a gregarious bird: models and videos of predators prompt alarm responses but no alarm calls by zebra finches. Behav. Ecol. Sociobiol. 71, 1–13. doi: 10.1007/s00265-017-2343-z
Byrd, M., and Varga, B. A. (2018). The manifestation of Campbell's law: consequences of eliminating of social studies from the curriculum. Soc. Stud. 109, 27–33. doi: 10.1080/00377996.2017.1406322
Campbell, D. T. (1979). Assessing the impact of planned social change. Eval. Prog. Plann. 2, 67–90. doi: 10.1016/0149-7189(79)90048-X
Campisi, J., Gray, H. E., Pardee, A. B., Dean, M., and Sonenshein, G. E. (1984). Cell-cycle control of c-myc but not c-ras expression is lost following chemical transformation. Cell 36, 241–247. doi: 10.1016/0092-8674(84)90217-4
Carter, G. G., and Wilkinson, G. S. (2013). Food sharing in vampire bats: reciprocal help predicts donations more than relatedness or harassment. Proc. R. Soc. B Biol. Sci. 280:20122573. doi: 10.1098/rspb.2012.2573
Catakovic, K., Klieser, E., Neureiter, D., and Geisberger, R. (2017). T cell exhaustion: from pathophysiological basics to tumor immunotherapy. Cell Commun. Signal. 15, 1–16. doi: 10.1186/s12964-016-0160-z
Cha, J. H., Chan, L. C., Li, C. W., Hsu, J. L., and Hung, M. C. (2019). Mechanisms controlling PD-L1 expression in cancer. Mol. Cell 76, 359–370. doi: 10.1016/j.molcel.2019.09.030
Cheney, D. L., and Seyfarth, R. M. (1988). Assessment of meaning and the detection of unreliable signals by vervet monkeys. Anim. Behav. 36, 477–486. doi: 10.1016/S0003-3472(88)80018-6
Cheng, H., Wang, Z., Fu, L., and Xu, T. (2019). Macrophage polarization in the development and progression of ovarian cancers: an overview. Front. Oncol. 9:421. doi: 10.3389/fonc.2019.00421
Clark, R., and Kimbrough, S. O. (2017). Social structure, opportunistic punishment and the evolution of honest signaling. PLoS ONE 12:e0188249. doi: 10.1371/journal.pone.0188249
Clutton-Brock, T. H., and Albon, S. D. (1979). The roaring of red deer and the evolution of honest advertisement. Behaviour 69, 145–170. doi: 10.1163/156853979X00449
Comunanza, V., and Bussolino, F. (2017). Therapy for cancer: strategy of combining anti-angiogenic and target therapies. Front. Cell Dev. Biol. 5:101. doi: 10.3389/fcell.2017.00101
Cotton, P. A., Kacelnik, A., and Wright, J. (1996). Chick begging as a signal: are nestlings honest? Behav. Ecol. 7, 178–182. doi: 10.1093/beheco/7.2.178
De Waal, F., and Waal, F. B. (2007). Chimpanzee Politics: Power and Sex Among Apes. London: JHU Press.
Dearborn, D. C. (1998). Begging behavior and food acquisition by brown-headed cowbird nestlings. Behav. Ecol. Sociobiol. 43, 259–270. doi: 10.1007/s002650050490
DeGregori, J. (2018). Adaptive Oncogenesis: A New Understanding of How Cancer Evolves Inside US. Cambridge, MA: Harvard University Press. doi: 10.4159/9780674985964
Detto, T., Jennions, M. D., and Backwell, P. R. (2010). When and why do territorial coalitions occur? Experimental evidence from a fiddler crab. Am. Nat. 175, E119–E125. doi: 10.1086/651588
Duijn, P. A., Kashirin, V., and Sloot, P. M. (2014). The relative ineffectiveness of criminal network disruption. Sci. Rep. 4, 1–15. doi: 10.1038/srep04238
Ellis, L. M., and Hicklin, D. J. (2009). Resistance to targeted therapies: refining anticancer therapy in the era of molecular oncology. Clin. Cancer Res. 15, 7471–7478. doi: 10.1158/1078-0432.CCR-09-1070
Elmore, S. (2007). Apoptosis: a review of programmed cell death. Toxicol. Pathol. 35, 495–516. doi: 10.1080/01926230701320337
Evans, C., and Evans, L. (2002). “Sceptical hens: receivers contrain deceptive food calling by male fowl,” in Animal Behavior Society Meeting (Boise, ID).
Finver, S. N., Nishikura, K., Finger, L. R., Haluska, F. G., Finan, J., Nowell, P. C., et al. (1988). Sequence analysis of the MYC oncogene involved in the t(8;14)(q24;q11) chromosome translocation in a human leukemia T-cell line indicates that putative regulatory regions are not altered. Proc. Natl. Acad. Sci. U.S.A. 85, 3052–3056. doi: 10.1073/pnas.85.9.3052
Fisman, R., and Golden, M. (2017). How to fight corruption. Science 356, 803–804. doi: 10.1126/science.aan0815
Fuchs, Y., and Steller, H. (2011). Programmed cell death in animal development and disease. Cell 147, 742–758. doi: 10.1016/j.cell.2011.10.033
Garcia, R., Bowman, T. L., Niu, G., Yu, H., Minton, S., Muro-Cacho, C. A., et al. (2001). Constitutive activation of Stat3 by the Src and JAK tyrosine kinases participates in growth regulation of human breast carcinoma cells. Oncogene 20, 2499–2513. doi: 10.1038/sj.onc.1204349
Gatenby, R. A., Brown, J., and Vincent, T. (2009). Lessons from applied ecology: cancer control using an evolutionary double bind. Cancer Res. 69, 7499–7502. doi: 10.1158/0008-5472.CAN-09-1354
Geiselhardt, S. F., Peschke, K., and Nagel, P. (2007). A review of myrmecophily in ant nest beetles (coleoptera: Carabidae: Paussinae): linking early observations with recent findings. Naturwissenschaften 94, 871–894. doi: 10.1007/s00114-007-0271-x
Glaudas, X., Rice, S. E., Clark, R. W., and Alexander, G. J. (2020). The intensity of sexual selection, body size and reproductive success in a mating system with male-male combat: is bigger better? Oikos 129, 998–1011. doi: 10.1111/oik.07223
Gorgoulis, V. G., and Halazonetis, T. D. (2010). Oncogene-induced senescence: The bright and dark side of the response. Curr. Opin. Cell Biol. 22, 816–827. doi: 10.1016/j.ceb.2010.07.013
Grafen, A. (1990). Biological signals as handicaps. J. Theoret. Biol. 144, 517–546. doi: 10.1016/S0022-5193(05)80088-8
Griffiths, J. G., and Bonser, S. P. (2013). Is sex advantageous in adverse environments? A test of the abandon-ship hypothesis. Am. Nat. 182, 718–725. doi: 10.1086/673476
Hanahan, D., and Weinberg, R. A. (2011). Hallmarks of cancer: the next generation. Cell 144, 646–674. doi: 10.1016/j.cell.2011.02.013
Hare, J. F., and Atkins, B. A. (2001). The squirrel that cried wolf: Reliability detection by juvenile Richardson's ground squirrels (Spermophilus richardsonii). Behav. Ecol. Sociobiol. 51, 108–112. doi: 10.1007/s002650100414
Hasselquist, D. (1994). Male attractiveness, mating tactics and realized fitness in the polygynous great reed warbler (Ph.D. thesis). Lund University, Lund, Sweden.
Hoi-Leitner, M., Nechtelberger, H., and Hoi, H. (1995). Song rate as a signal for nest site quality in blackcaps (Sylvia atricapilla). Behav. Ecol. Sociobiol. 37, 399–405. doi: 10.1007/s002650050207
Hoshino, A., Costa-Silva, B., Shen, T. L., Rodrigues, G., Hashimoto, A., Tesic Mark, M., et al. (2015). Tumour exosome integrins determine organotropic metastasis. Nature 527, 329–335. doi: 10.1038/nature15756
Hough, K. P., Chanda, D., Duncan, S. R., Thannickal, V. J., and Deshane, J. S. (2017). Exosomes in immunoregulation of chronic lung diseases. Allergy 72, 534–544. doi: 10.1111/all.13086
Hua, Y., and Bergers, G. (2019). Tumors vs. chronic wounds: an immune cell's perspective. Front. Immunol. 10:2178. doi: 10.3389/fimmu.2019.02178
Janeway, C. A., Travers, P., Walport, M., and Shlomchik, M. J. (2001). “Immunological memory,” in Immunobiology: The Immune System in Health and Disease, 5th Edn, eds C. A. Janeway, Jr., and P. Travers (New York, NY: Garland Science).
Jiang, G., Wei, Z. P., Pei, D. S., Xin, Y., Liu, Y. Q., and Zheng, J. N. (2011). A novel approach to overcome temozolomide resistance in glioma and melanoma: inactivation of MGMT by gene therapy, volume 406. Biochem. Biophys. Res. Commun. 406, 311–314. doi: 10.1016/j.bbrc.2011.02.042
Jiang, Y., Li, Y., and Zhu, B. (2015). T-cell exhaustion in the tumor microenvironment. Cell Death Dis. 6:e1792. doi: 10.1038/cddis.2015.162
Johnstone, R. (1977). “The evolution of animal signals,” in Behavioral Ecology, eds J. Krebs and N. Davies (Oxford: Blackwell), 155–178.
Joly, M. (2017). Corruption: the shortcut to disaster. Sustain. Product. Consump. 10, 133–156. doi: 10.1016/j.spc.2016.09.005
Kalluri, R., and LeBleu, V. S. (2020). The biology, function, and biomedical applications of exosomes. Science 367:6478. doi: 10.1126/science.aau6977
Kamenetz, A. (2015). A review of: The Test: Why our schools are obsessed with standardized testing—but you don't have to be. Wisdom in Educ. 6:5. Available online at: https://scholarworks.lib.csusb.edu/wie/vol6/iss1/5
Kareva, I. (2015). Cancer ecology: niche construction, keystone species, ecological succession, and ergodic theory. Biol. Theory 10, 283–288. doi: 10.1007/s13752-015-0226-y
Kastan, M. B., and Bartek, J. (2004). Cell-cycle checkpoints and cancer. Nature 432, 316–323. doi: 10.1038/nature03097
Kilner, R. M., Noble, D. G., and Davies, N. B. (1999). Signals of need in parent-offspring communication and their exploitation by the common cuckoo. Nature 397, 667–672. doi: 10.1038/17746
Kleiven, O. T., Larsson, P., Hobæk, A., and Hobaek, A. (1992). Sexual reproduction in Daphnia magna requires three stimuli. Oikos 65:197. doi: 10.2307/3545010
Kolokoltsov, V. N., and Malafeyev, O. A. (2017). Mean-field-game model of corruption. Dyn. Games Appl. 7, 34–47. doi: 10.1007/s13235-015-0175-x
Korolev, K. S., Xavier, J. B., and Gore, J. (2014). Turning ecology and evolution against cancer. Nat. Rev. Cancer 14, 371–380. doi: 10.1038/nrc3712
Krakauer, D. C., and Pagel, M. (1996). Selection by somatic signals: the advertisement of phenotypic state through costly intercellular signals. Philos. Trans. Biol. Sci. 351, 647–658. doi: 10.1098/rstb.1996.0062
Kumar, H., Kawai, T., and Akira, S. (2011). Pathogen recognition by the innate immune system. Int. Rev. Immunol. 30, 16–34. doi: 10.3109/08830185.2010.529976
Lage, H., Christmann, M., Kern, M. A., Dietel, M., Pick, M., Kaina, B., et al. (1999). Expression of DNA repair proteins hMSH2, hMSH6, hMLH1, O6- methylguanine-DNA methyltransferase and N-methylpurine-DNA glycosylase in melanoma cells with acquired drug resistance. Int. J. Cancer 80, 744–750. doi: 10.1002/(SICI)1097-0215(19990301)80:5<744::AID-IJC19>3.0.CO;2-5
Lederberg, J. (1999). JBS Haldane (1949) on infectious disease and evolution. Genetics 153, 1–3. doi: 10.1093/genetics/153.1.1
Lenoir, A., d'Ettorre, P., Errard, C., and Hefetz, A. (2001). Chemical ecology and social parasitism in ants. Annu. Rev. Entomol. 46, 573–599. doi: 10.1146/annurev.ento.46.1.573
Lev, D. C., Ruiz, M., Mills, L., McGary, E. C., Price, J. E., and Bar-Eli, M. (2003). Dacarbazine causes transcriptional up-regulation of interleukin 8 and vascular endothelial growth factor in melanoma cells: a possible escape mechanism from chemotherapy. Mol. Cancer Therapeut. 2, 753–763.
Levin, M., and Tsirik, M. (1998). Corruption as an object of mathematical modeling. Matekon 34, 16–46.
Levine, E. M., Becker, Y., Boone, C. W., and Eagle, H. (1965). Contact inhibition, macromolecular synthesis, and polyribosomes in cultured human diploid fibroblasts. Proc. Natl. Acad. Sci. U.S.A. 53, 350–356. doi: 10.1073/pnas.53.2.350
Li, X.-B., Zhang, Z.-R., Schluesener, H. J., and Xu, S.-Q. (2006). Role of exosomes in immune regulation. J. Cell. Mol. Med. 10, 364–375. doi: 10.1111/j.1582-4934.2006.tb00405.x
Liberti, M. V., and Locasale, J. W. (2016). The Warburg effect: how does it benefit cancer cells? Trends Biochem. Sci. 41, 211–218. doi: 10.1016/j.tibs.2015.12.001
Liu, X. l., Ding, J., and Meng, L. h. (2018). Oncogene-induced senescence: a double edged sword in cancer. Acta Pharmacol. Sin. 39, 1553–1558. doi: 10.1038/aps.2017.198
Luna-Pla, I., and Nicolás-Carlock, J. R. (2020). Corruption and complexity: a scientific framework for the analysis of corruption networks. Appl. Netw. Sci. 5, 1–18. doi: 10.1007/s41109-020-00258-2
Madan, E., Gogna, R., and Moreno, E. (2018). Cell competition in development: information from flies and vertebrates. Curr. Opin. Cell Biol. 55, 150–157. doi: 10.1016/j.ceb.2018.08.002
Madan, E., Pelham, C. J., Nagane, M., Parker, T. M., Canas-Marques, r., Fazio, K., et al. (2019). Flower isoforms promote competitive growth in cancer. Nature. 572, 260–264. doi: 10.1038/s41586-019-1429-3
Manica, L. T., Maia, R., Dias, A., Podos, J., and Macedo, R. H. (2014). Vocal output predicts territory quality in a neotropical songbird. Behav. Process. 109, 21–26. doi: 10.1016/j.beproc.2014.07.004
Martincorena, I., Roshan, A., Gerstung, M., Ellis, P., Van Loo, P., McLaren, S., et al. (2015). High burden and pervasive positive selection of somatic mutations in normal human skin. Science 348, 880–886. doi: 10.1126/science.aaa6806
Maverakis, E., Kim, K., Shimoda, M., Gershwin, M. E., Patel, F., Wilken, R., et al. (2015). Glycans in the immune system and The Altered Glycan theory of autoimmunity: a critical review. J. Autoimmun. 57, 1–13. doi: 10.1016/j.jaut.2014.12.002
Maynard-Smith, J. (1991). Honest signalling: the Philip Sidney game. Anim. Behav. 42, 1034–1035. doi: 10.1016/S0003-3472(05)80161-7
Maziveyi, M., Dong, S., Baranwal, S., Mehrnezhad, A., Rathinam, R., Huckaba, T. M., et al. (2019). Exosomes from nischarin-expressing cells reduce breast cancer cell motility and tumor growth. Cancer Res. 79, 2152–2166. doi: 10.1158/0008-5472.CAN-18-0842
Merino, M. M., Rhiner, C., Lopez-Gay, J. M., Buechel, D., Hauert, B., and Moreno, E. (2015). Elimination of unfit cells maintains tissue health and prolongs lifespan. Cell 160, 461–476. doi: 10.1016/j.cell.2014.12.017
Merino, M. M., Rhiner, C., Portela, M., and Moreno, E. (2013). “Fitness fingerprints” mediate physiological culling of unwanted neurons in Drosophila. Curr. Biol. 23, 1300–1309. doi: 10.1016/j.cub.2013.05.053
Merlo, L. M., Pepper, J. W., Reid, B. J., and Maley, C. C. (2006). Cancer as an evolutionary and ecological process. Nat. Rev. Cancer 6, 924–935. doi: 10.1038/nrc2013
Milojkovic, D., and Apperley, J. F. (2009). Mechanisms of resistance to imatinib and second-generation tyrosine inhibitors in chronic myeloid leukemia. Clin. Cancer Res. 15, 7519–7527. doi: 10.1158/1078-0432.CCR-09-1068
Mitchell, S. E., Read, A. F., and Little, T. J. (2004). The effect of a pathogen epidemic on the genetic structure and reproductive strategy of the crustacean Daphnia magna. Ecol. Lett. 7, 848–858. doi: 10.1111/j.1461-0248.2004.00639.x
Møller, A. P. (2010). False alarm calls as a means of resource usurpation in the great tit parus major. Ethology 79, 25–30. doi: 10.1111/j.1439-0310.1988.tb00697.x
Monnin, T., and Ratnieks, F. L. (2001). Policing in queenless ponerine ants. Behav. Ecol. Sociobiol. 50, 97–108. doi: 10.1007/s002650100351
Moreno, E., Fernandez-Marrero, Y., Meyer, P., and Rhiner, C. (2015). Brain regeneration in Drosophila involves comparison of neuronal fitness. Curr. Biol. 25, 955–963. doi: 10.1016/j.cub.2015.02.014
Morton, E. S. (1977). On the occurrence and significance of motivation-structural rules in some bird and mammal sounds. Am. Nat. 111, 855–869. doi: 10.1086/283219
Nagel, D., Vincendeau, M., Eitelhuber, A. C., and Krappmann, D. (2014). Mechanisms and consequences of constitutive NF-κB activation in B-cell lymphoid malignancies. Oncogene 33, 5655–5665. doi: 10.1038/onc.2013.565
Nekovee, M., and Pinto, J. (2019). Modeling the impact of organization structure and whistle-blowers on intra-organizational corruption contagion. Phys. A Stat. Mech. Appl. 522, 339–349. doi: 10.1016/j.physa.2019.01.140
Nichols, S. L., and Berliner, D. C. (2007). “The pressure to cheat in a high-stakes testing environment,” in Psychology of Academic Cheating, eds E. M. Anderman and T. B. Murdock (Amsterdam: Elsevier Inc.), 289–311. doi: 10.1016/B978-012372541-7/50016-4
Ombrato, L., Nolan, E., Kurelac, I., Mavousian, A., Louise Bridgeman, V., Heinze, I., et al. (2019). Metastatic-niche labelling reveals parenchymal cells with stem features. Nature 572, 603–608. doi: 10.1038/s41586-019-1487-6
Otte, D. (1974). Effects and functions in the evolution of signaling systems. Annu. Rev. Ecol. Syst. 5, 385–417. doi: 10.1146/annurev.es.05.110174.002125
Park, J. E., Tan, H. S., Datta, A., Lai, R. C., Zhang, H., Meng, W., et al. (2010). Hypoxic tumor cell modulates its microenvironment to enhance angiogenic and metastatic potential by secretion of proteins and exosomes. Mol. Cell. Proteom. 9, 1085–1099. doi: 10.1074/mcp.M900381-MCP200
Pavel, M., Renna, M., Park, S. J., Menzies, F. M., Ricketts, T., Füllgrabe, J., et al. (2018). Contact inhibition controls cell survival and proliferation via YAP/TAZ-autophagy axis. Nat. Commun. 9, 1–18. doi: 10.1038/s41467-018-05388-x
Pienta, K. J., Hammarlund, E. U., Brown, J. S., Amend, S. R., and Axelrod, R. M. (2021). Cancer recurrence and lethality are enabled by enhanced survival and reversible cell cycle arrest of polyaneuploid cells. Proc. Natl. Acad. Sci. U.S.A. 118:e2020838118. doi: 10.1073/pnas.2020838118
Pienta, K. J., McGregor, N., Axelrod, R., and Axelrod, D. E. (2008). Ecological therapy for cancer: defining tumors using an ecosystem paradigm suggests new opportunities for novel cancer treatments. Transl. Oncol. 1, 158–164. doi: 10.1593/tlo.08178
Pohlmann, P. R., Mayer, I. A., and Mernaugh, R. (2009). Resistance to trastuzumab in breast cancer. Clin. Cancer Res. 15, 7479–7491. doi: 10.1158/1078-0432.CCR-09-0636
Ribatti, D., Nico, B., Crivellato, E., Roccaro, A. M., and Vacca, A. (2007). The history of the angiogenic switch concept. Leukemia 21, 44–52. doi: 10.1038/sj.leu.2404402
Rose-Ackerman, S. (1975). The economics of corruption. J. Public Econ. 4, 187–203. doi: 10.1016/0047-2727(75)90017-1
Ryan, M. J., Fox, J. H., Wilczynski, W., and Rand, A. S. (1990). Sexual selection, sensory systems and sensory exploitation. Oxford Surv. Evol. Biol. 7, 157–195.
Rytkonen, S. O., Orell, M. I., Koivula, K. T., and Welling, P. P. (1997). Song rate correlates with paternal care and survival in willow tits: advertisement of male quality? Behaviour. 134, 891–904. doi: 10.1163/156853997X00214
Sacchi, R. (2002). Features of begging calls reveal general condition and need of food of barn swallow (Hirundo rustica) nestlings. Behav. Ecol. 13, 268–273. doi: 10.1093/beheco/13.2.268
Schietinger, A., and Greenberg, P. D. (2014). Tolerance and exhaustion: defining mechanisms of T cell dysfunction. Trends Immunol. 35, 51–60. doi: 10.1016/j.it.2013.10.001
Schlaepfer, M. A., Runge, M. C., and Sherman, P. W. (2002). Ecological and evolutionary traps. Trends Ecol. Evol. 17, 474–480. doi: 10.1016/S0169-5347(02)02580-6
Schmid, M. C., and Varner, J. A. (2012). Myeloid cells in tumor inflammation. Vasc. Cell 4:14. doi: 10.1186/2045-824X-4-14
Schmidt-Nielsen, K. (1984). Scaling, Why Is Animal Size So Important? New York, NY: Cambridge University Press. doi: 10.1017/CBO9781139167826
Searcy, W. A., and Nowicki, S. (2005). The Evolution of Animal Communication: Reliability and Deception in Signaling Systems. Princeton, NJ: Princeton University Press.
Seliger, B. (2005). Strategies of tumor immune evasion. Biodrugs 19, 347–354. doi: 10.2165/00063030-200519060-00002
Shine, R. (1990). Function and evolution of the frill of the frillneck lizard, Chlamydosaurus kingii (sauria: Agamidae). Biol. J. Linnean Soc. 40, 11–20. doi: 10.1111/j.1095-8312.1990.tb00531.x
Silk, J. B., Kaldor, E., and Boyd, R. (2000). Cheap talk when interests conflict. Anim. Behav. 59, 423–432. doi: 10.1006/anbe.1999.1312
Slamon, D. J., Leyland-Jones, B., Shak, S., Fuchs, H., Paton, V., Bajamonde, A., et al. (2001). Use of chemotherapy plus a monoclonal antibody against HER2 for metastatic breast cancer that overexpresses HER2. N. Engl. J. Med. 344, 783–792. doi: 10.1056/NEJM200103153441101
Staňková, K., Brown, J. S., Dalton, W. S., and Gatenby, R. A. (2019). Optimizing cancer treatment using game theory: a review. JAMA Oncol. 5, 96–103. doi: 10.1001/jamaoncol.2018.3395
Stegelmeier, A. A., van Vloten, J. P., Mould, R. C., Klafuric, E. M., Minott, J. A., Wootton, S. K., et al. (2019). Myeloid cells during viral infections and inflammation. Viruses 11:1. doi: 10.3390/v11020168
Strauss, L., Guarneri, V., Gennari, A., and Sica, A. (2020). Implications of metabolism-driven myeloid dysfunctions in cancer therapy. Cell. Mol. Immunol. 18, 1–13. doi: 10.1038/s41423-020-00556-w
Sur, D. G., Colceriu, M., Sur, G., Aldea, C., Silaghi, C., Samasca, G., et al. (2019). MiRNAs roles in the diagnosis, prognosis and treatment of colorectal cancer. Expert Rev. Proteom. 16, 851–856. doi: 10.1080/14789450.2019.1659732
Swann, J. B., and Smyth, M. J. (2007). Immune surveillance of tumors. J. Clin. Investig. 117, 1137–1146. doi: 10.1172/JCI31405
Tansey, W. P. (2014). Mammalian MYC proteins and cancer. N. J. Sci. 2014:757534. doi: 10.1155/2014/757534
Tawbi, H. A., and Buch, S. C. (2010). Chemotherapy resistance abrogation in metastatic melanoma. Clin. Adv. Hematol. Oncol. 8, 259–266.
Temeles, E. J. (1994). The role of neighbours in territorial systems: when are they “dear enemies?” Anim. Behav. 47, 339–350. doi: 10.1006/anbe.1994.1047
Tibbetts, E. A., and Dale, J. (2004). A socially enforced signal of quality in a paper wasp. Nature 432, 218–222. doi: 10.1038/nature02949
Tibbetts, E. A., and Izzo, A. (2010). Social punishment of dishonest signalers caused by mismatch between signal and behavior. Curr. Biol. 20, 1637–1640. doi: 10.1016/j.cub.2010.07.042
Tsukumo, S. I., and Yasutomo, K. (2018). Regulation of CD8+ T cells and antitumor immunity by Notch signaling. Front. Immunol. 9:101. doi: 10.3389/fimmu.2018.00101
Vander Heiden, M. G., Cantley, L. C., and Thompson, C. B. (2009). Understanding the warburg effect: The metabolic requirements of cell proliferation. Science 324, 1029–1033. doi: 10.1126/science.1160809
Vehrencamp, S. (2000). Handicap, Index, and Conventional Signal Elements of Bird Song. Trondheim: Tapir Academic Press.
Vehrencamp, S. L. (2009). “Animal communication: honesty and deception,” in Encyclopedia of Neuroscience, ed L. R. Squire (Berlin: Academic Press), 397–406. doi: 10.1016/B978-008045046-9.01835-0
Vinay, D. S., Ryan, E. P., Pawelec, G., Talib, W. H., Stagg, J., Elkord, E., et al. (2015). Immune evasion in cancer: mechanistic basis and therapeutic strategies. Semin. Cancer Biol. 35, S185–S198. doi: 10.1016/j.semcancer.2015.03.004
Watson, K. A., and Ritchison, G. (2018). Effect of variation in nestling hunger levels on the begging behaviour of nestlings and the provisioning behaviour of adult American kestrels. Avian Biol. Res. 11, 35–43. doi: 10.3184/175815617X15127411523971
Wedel, J. R. (2012). Rethinking corruption in an age of ambiguity. Annu. Rev. Law Soc. Sci. 8, 453–498. doi: 10.1146/annurev.lawsocsci.093008.131558
Wei, S.-J. (1999). “Corruption in economic development: beneficial grease, minor annoyance, or major obstacle?” in World Bank Policy Research Working Paper. doi: 10.1596/1813-9450-2048
Wilts, B. D., Michielsen, K., De Raedt, H., and Stavenga, D. G. (2014). Sparkling feather reflections of a bird-of-paradise explained by finite-difference time-domain modeling. Proc. Natl. Acad. Sci. U.S.A. 111, 4363–4368. doi: 10.1073/pnas.1323611111
Wolfensohn, J. D. (2005). Voice for the World's Poor: Selected Speeches and Writings of World Bank President James D. Wolfensohn, 1995-2005, Vol. 889. World Bank Publications. doi: 10.1596/0-8213-6156-2
Yom-Tov, Y. (1980). Intraspecific nest parasitism in birds. Biol. Rev. 55, 93–108. doi: 10.1111/j.1469-185X.1980.tb00689.x
Zhang, Z., Liu, S., Zhang, B., Qiao, L., Zhang, Y., and Zhang, Y. (2020). T cell dysfunction and exhaustion in cancer. Front. Cell Dev. Biol. 8:17. doi: 10.3389/fcell.2020.00017
Zhu, H., Blake, S., Kusuma, F. K., Pearson, R. B., Kang, J., and Chan, K. T. (2020). Oncogene-induced senescence: from biology to therapy. Mech. Ageing Dev. 187:111229. doi: 10.1016/j.mad.2020.111229
Keywords: signaling, corruption, deception, cancer, evolution, behavioral ecology, targeted therapy
Citation: Bukkuri A and Adler FR (2021) Viewing Cancer Through the Lens of Corruption: Using Behavioral Ecology to Understand Cancer. Front. Ecol. Evol. 9:678533. doi: 10.3389/fevo.2021.678533
Received: 09 March 2021; Accepted: 16 June 2021;
Published: 08 July 2021.
Edited by:
Etienne Baratchart, Lund University, SwedenReviewed by:
Robert Noble, City University of London, United KingdomCopyright © 2021 Bukkuri and Adler. This is an open-access article distributed under the terms of the Creative Commons Attribution License (CC BY). The use, distribution or reproduction in other forums is permitted, provided the original author(s) and the copyright owner(s) are credited and that the original publication in this journal is cited, in accordance with accepted academic practice. No use, distribution or reproduction is permitted which does not comply with these terms.
*Correspondence: Anuraag Bukkuri, YW51cmFhZy5idWtrdXJpQG1vZmZpdHQub3Jn
Disclaimer: All claims expressed in this article are solely those of the authors and do not necessarily represent those of their affiliated organizations, or those of the publisher, the editors and the reviewers. Any product that may be evaluated in this article or claim that may be made by its manufacturer is not guaranteed or endorsed by the publisher.
Research integrity at Frontiers
Learn more about the work of our research integrity team to safeguard the quality of each article we publish.