- Department of Biology, Trent University, Peterborough, ON, Canada
The COVID-19 pandemic profoundly affected research in ecology and evolution, with lockdowns resulting in the suspension of most research programs and creating gaps in many ecological datasets. Likewise, monitoring efforts directed either at tracking trends in natural systems or documenting the environmental impacts of anthropogenic activities were largely curtailed. In addition, lockdowns have affected human activity in natural environments in ways that impact the systems under investigation, rendering many widely used approaches for handling missing data (e.g., available case analysis, mean substitution) inadequate. Failure to properly address missing data will lead to bias and weak inference. Researchers and environmental monitors must ensure that lost data are handled robustly by diagnosing patterns and mechanisms of missingness and applying appropriate tools like multiple imputation, full-information maximum likelihood, or Bayesian approaches. The pandemic has altered many aspects of society and it is timely that we critically reassess how we treat missing data in ecological research and environmental monitoring, and plan future data collection to ensure robust inference when faced with missing data. These efforts will help ensure the integrity of inference derived from datasets spanning the COVID-19 lockdown and beyond.
Introduction
In ecology and environmental monitoring, studies conducted on free-living organisms or natural environments often span multiple field seasons. While lockdowns related to the COVID-19 pandemic have been necessary to ensure public safety, studies worldwide are now punctuated with missing data due to travel restrictions, laboratory closures, or suspended authorizations (Pennisi, 2020). Likewise, standard environmental monitoring programs relevant to public health and tracking impacts of industrial activity also have been curtailed or suspended (McIntosh, 2020; Patterson et al., 2020; Viglione, 2020; Conservation International, 2021). Restrictions on data collection in ecology have spanned more than a year and may continue to impact data collection for some time. Data have been (and continue to be) lost due to the pandemic. These losses will be globally pervasive, affecting an array of species, systems, and research or monitoring objectives. The community of data collectors and analysts have a responsibility to ensure that datasets with missing data are handled correctly to maintain the integrity of inferences derived from them. In practical terms, this requires improved commitment to employ appropriate statistical tools for dealing with data gaps, while also ensuring that future studies and data collections are robust to missing data.
Challenges Imposed by Missing Data
Generally, data gaps affect estimated values, sample variability, detectable effect size, and statistical power, thereby challenging our ability to make robust inferences (Maxwell et al., 2008; Button et al., 2013a,b). In the context of the COVID-19 pandemic, the unexpectedness, extent, and synchronicity of data loss among ecology data sets is unprecedented. For example, studies tracking population size of endangered species will be missing critical data on population status, productivity, and survival. Cohorts or individuals being tracked through time will suffer from interrupted timelines. Active field experiments or studies requiring time-sensitive comparisons (e.g., Before-After-Control-Impact studies) will lack response measurements. Environmental monitoring programs could miss critical early warnings of contamination or industrial exceedance of approved standards. Long-term monitoring programs aimed at documenting trends or variability in species abundance or contaminant levels through time will be faced with uncertainty in their assessments and forecasts. Harvest quotas may need to be set without key data required to establish reliable sustainable yield targets. Beyond the sheer extent of data lost, lockdowns have also affected many of the systems under investigation, thereby impacting how data gaps should be addressed. The current and future extent of analytical challenges imposed by the pandemic therefore presents an important opportunity for statistical development and awareness.
Several widely used approaches for handling missing data can introduce bias or fail to effectively leverage existing data (Schafer and Graham, 2002; Nakagawa and Freckleton, 2008). Such approaches include: (1) Ignoring data gaps through listwise or pairwise deletion (i.e., complete-case and available-case analysis); (2) replacing missing data with an average value (i.e., mean substitution); and (3) interpolating missing data through loess regression or smoothing splines (McKnight et al., 2007; Enders, 2010; Table 1 and Figure 1). These approaches can compromise data integrity by exacerbating the effects of small sample size on statistical power (listwise deletion), artificially reducing sample variance (mean substitution, smoothing), biasing parameter estimates (listwise and pairwise deletion, smoothing), or synthesizing missing data based on spurious assumptions about data structure or distribution (smoothing) (Schafer and Graham, 2002; Nakagawa and Freckleton, 2008; Little and Rubin, 2019). Although such approaches may still have occasional utility (e.g., see Hughes et al., 2019), our goal is to motivate the uptake of more robust alternatives, where appropriate.
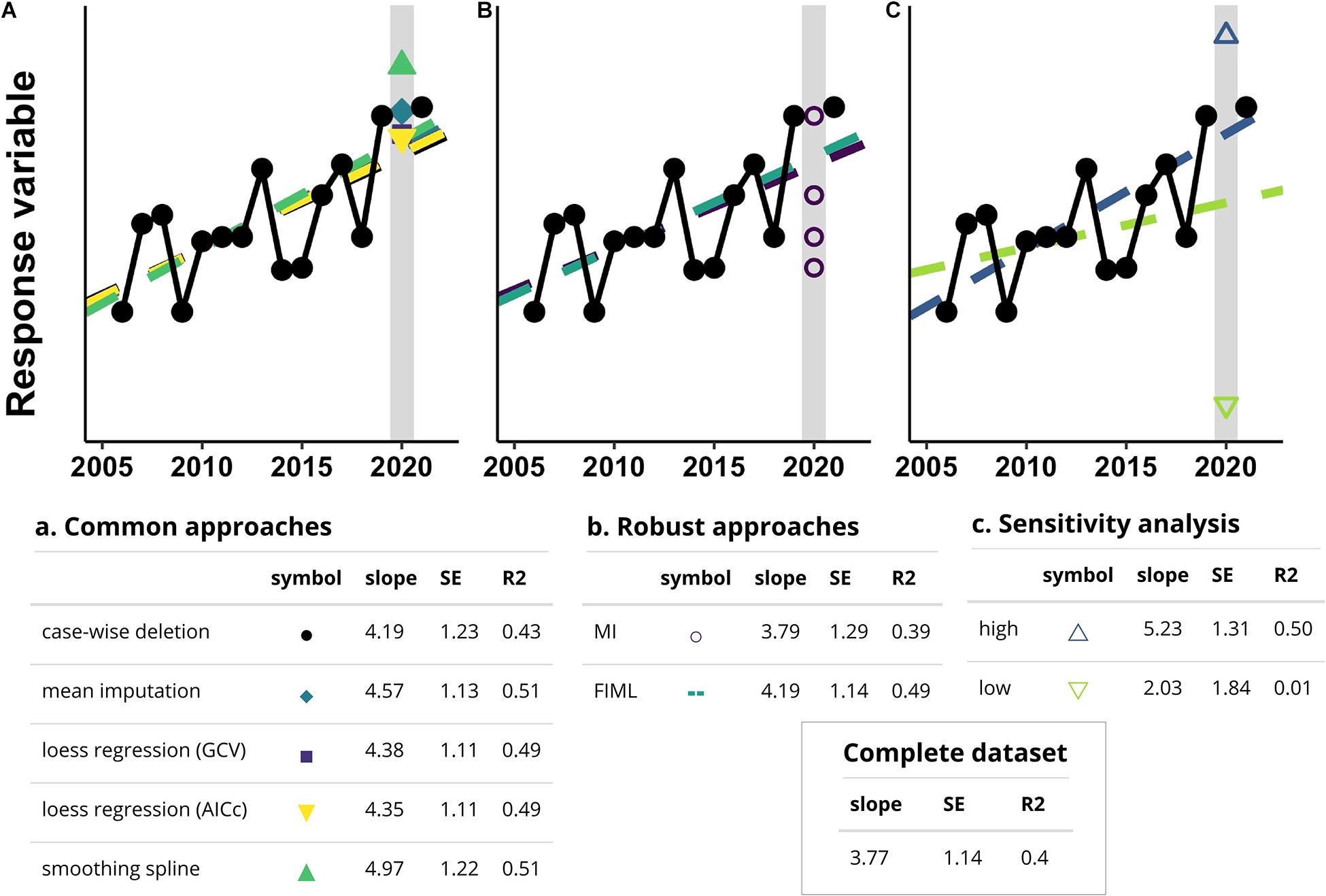
Figure 1. Simulated results from the analysis of a hypothetical dataset based on missing data from the pandemic lockdown. The annual time series has a simple linear trend, where a single data point representing the year 2020 is missing (highlighted by the gray bar). Parameter estimates (slope), and measures of variance (SE) and fit (R2) for the complete dataset are shown in the box for comparison. (A) Common approaches for dealing with missing data yield biased parameter estimates, often underestimate variance, and overestimate fit, as can be seen by the comparison to the complete dataset. (B) For data that are missing at random (MAR) or missing completely at random (MCAR), using multiple imputation (MI) or maximum likelihood-based approaches (e.g., full information maximum likelihood—FIML) help minimize these issues by accounting for uncertainty in the missing data. (C) If data are missing not at random (MNAR), best/worst case sensitivity analyses (e.g., via an evaluation using extreme values as depicted here) can be used to evaluate the effects that the full range of potential values might have on subsequent analyses. Source code available here: https://github.com/JenileeGobin/confronting-missing-data.
Mechanisms of Missingness and Their Implications
It is tempting to assume that because a stochastic process led to the COVID-19 pandemic, data missingness is only problematic insofar as it reduces statistical power due to smaller sample size. Yet, there is an important distinction between scenarios where lockdowns are likely to have only affected data collection vs. those where lockdowns not only prohibited data collection but also directly or indirectly influenced the processes under investigation. In fact, we have already seen several indications that COVID-19 lockdowns altered human behavior in ways that could translate to unforeseen responses in natural environments. These include improvements in air quality, increased use of urban areas by wildlife, as well as possible increases in wildlife poaching and resource extraction (Buckley, 2020; Gardner, 2020; Haggert, 2020). Clearly, additional care must be taken to treat data missingness appropriately when this coincides with acute changes in the system of interest. For environmental monitoring in particular, assuming that industry behavior was unchanged during the lockdown when little/no monitoring and oversight were undertaken seems unwise (Gray and Shimshack, 2011). More generally, failing to critically examine patterns of missing data to infer mechanisms of missingness will promote poor statistical treatment and thereby impact inferences drawn from those datasets.
Statisticians recognize three “mechanisms of missingness”: Missing completely at random, missing at random, and missing not at random (McKnight et al., 2007; Enders, 2010; Little and Rubin, 2019). The extent to which inadequate treatment of missing data leads to faulty inference, and how these gaps may be corrected, depends on the specific mechanism of missingness that has resulted in the missing data. For example, listwise deletion or complete-case analysis are only valid when data are “missing completely at random” (MCAR), meaning that there are no detectable patterns of missingness across any variables in the dataset (McKnight et al., 2007; Nakagawa and Freckleton, 2008). Assuming that data are MCAR means that researchers assume that data missingness is independent of both observed and unobserved data. This assumption is rarely tested despite this being relatively straightforward to do with current statistical techniques (McKnight et al., 2007; Nakagawa and Freckleton, 2008). One approach for evaluating whether data are MCAR, proposed by Little (1988), tests for differences between the means of different missingness patterns across the entire dataset and produces a single χ2 statistic (see also McKnight et al., 2007; Enders, 2010). This test can be implemented using the mcar_test function from the “naniar” package in R (Tierney et al., 2021) but may be sensitive to departures from normality and small sample size (Little, 1988). Researchers can also create a binary dummy variable representing missing/not missing for the variable of interest, then examine whether missingness is related to the values of other variables in the dataset (McKnight et al., 2007; Nakagawa, 2015). The R package “mice” also provides tools for visualizing missing data to better understand patterns (van Buuren and Groothuis-Oudshoorn, 2011).
MCAR is a very strong assumption and rarely valid for empirical datasets, especially those derived from fieldwork in ecology where observer error, natural variation, collinearity, and serial autocorrelation can be substantive (Nakagawa and Freckleton, 2008; Nakagawa, 2015; Little and Rubin, 2019). Instead, data may be “missing at random” (MAR), where data missingness depends on one or more observed variables. In such cases, patterns of missingness within a specific dataset are associated with other observed variables and do not depend on the value of the missing observations themselves (Schafer and Graham, 2002; Dong and Peng, 2013). If pandemic restrictions only limited data collection, and were unlikely to affect the system itself, such data would be MAR so long as missingness in the response variable depends on other explanatory variables that were observed/recorded. A third possibility is that data are “missing not at random” (MNAR), which occurs when the probability of missingness depends on the value of the missing observation, or on an unobserved variable (Schafer and Graham, 2002; Nakagawa and Freckleton, 2008; Dong and Peng, 2013). Specifically, if missing data resulting from a lockdown coincides with acute changes in the system under investigation and/or prohibited the collection of suitable auxiliary data, such data would be MNAR. These acute changes would include those imposed by the researcher (e.g., as part of a Before-After Control-Impact experiment), or those arising as a direct/indirect effect of the lockdowns themselves (e.g., increased use of urban areas by wildlife). Other plausible scenarios where data may be MNAR as a result of lockdowns include changes in emissions or wildlife harvest due to reduced human activity or lapses in oversight during the lockdown period.
Diagnosing the mechanism of missingness (sensu Little and Rubin, 1987, 2019) is an important step to guide subsequent data analysis and should be prioritized for all datasets with missing data (Schafer and Graham, 2002; McKnight et al., 2007). It would be risky, especially for regulators, to assume that missing data owing to the lockdown are MCAR. In fact, even when diagnostic tests identify missing data as MCAR, it is generally safer to presume MAR when dealing with missing values in ecological studies (Nakagawa and Freckleton, 2008; Nakagawa, 2015, but see Chen and Ibrahim, 2013). In so doing the researcher acknowledges that, in practice, MAR is a more realistic assumption and that missing data may be MAR or MNAR even when tests fail to detect departures from MCAR (Nakagawa and Freckleton, 2011; Nakagawa, 2015). When data are MAR, bias in parameter estimates can be avoided by leveraging information about the non-random associations between data missingness and other observed variables in the dataset, but this is not the case when data are MNAR (Schafer and Graham, 2002; Little and Rubin, 2019). Differentiating between MAR or MNAR is difficult, in part because it relies on fundamentally untestable assumptions related to unobserved data, however, a number of conceptual, numerical, and graphical methods can be helpful (see McKnight et al., 2007; Enders, 2010). Detectable patterns of missingness that cannot be explained by other variables in the dataset also indicate MNAR. Ultimately, researchers must rely primarily on their understanding of the system, and a correct interpretation of missingness mechanisms, to determine whether data are likely to be MAR or MNAR (Bhaskaran and Smeeth, 2014; Nakagawa, 2015) before moving on to determine how to handle gaps in their datasets.
Remedies for Missing Data
Once the mechanism of missingness has been determined, researchers should adopt statistically robust procedures for dealing with missing data, of which there are several options that differ in their statistical philosophy, complexity, and underlying assumptions (Table 1). Here, we focus on the most relevant and straightforward approaches that should meet the needs of the majority of professionals in ecology (Nakagawa and Freckleton, 2008; Nakagawa, 2015). When missing data are MAR, multiple imputation (MI) and full information maximum likelihood (FIML) are reliable options (Nakagawa and Freckleton, 2008; Jakobsen et al., 2017; Little and Rubin, 2019; Figure 1). In MI, missing data are replaced with a set of random draws from the observed data distribution, and this process is repeated to create several new full datasets. Each full dataset is then analyzed separately, and the results are combined (McKnight et al., 2007; Enders, 2010). The number of imputations required depends in part on how much data are missing. As few as 3–20 imputed datasets may be sufficient to generate unbiased estimates (Dong and Peng, 2013; Jakobsen et al., 2017), but 30–40 imputations are often required (Nakagawa, 2017; Nakagawa and de Villemereuil, 2019). FIML does not replace missing values, but instead uses observed data distributions to infer the characteristics of the complete dataset. The approach uses an iterative process of implicit replacement and analysis with feedbacks, most commonly using the expectation–maximization algorithm (Enders, 2010; Dong and Peng, 2013; Little and Rubin, 2019). Whereas FIML permits the use of likelihood ratio tests for comparing nested models and generally yields smaller standard errors, MI can deal with categorical variables (Dong and Peng, 2013, see also Allison, 2005). Both MI and FIML maintain statistical power, preserve patterns of natural variability in data, and generally lead to unbiased estimates when assumptions are met and data are MAR (Donders et al., 2006; Nakagawa and Freckleton, 2008). Reporting guidelines for missing data analysis can be found in Enders (2010) and van Buuren (2018).
The above approaches may not be helpful when the proportion of missing data are large (e.g., ∼40%), only missing from the dependent variable and suitable auxiliary variables are not identified, or when the assumptions of MNAR are plausible (Dong and Peng, 2013; Jakobsen et al., 2017). Importantly, even when < 5% of data are missing it may not be “ignorable” (i.e., MCAR or MAR). When missing data is “non-ignorable” (i.e., MNAR), analysis requires that the researcher make specific assumptions about how the unobserved data are missing and build a model to fill in missing values. Methods for dealing with MNAR include selection models and pattern-mixture models (see Enders, 2010; Little and Rubin, 2019). Assumptions about unobserved data are fundamentally untestable, and these models will perform poorly if the assumptions are incorrect. Supplementary analyses (e.g., worst/best case sensitivity analyses) should be employed to quantify the potential impacts of missing data on key outcomes (Enders, 2010; Jakobsen et al., 2017), and efforts should be made to clearly articulate uncertainly in the outcome. Given that pure forms of MCAR, MAR, and MNAR are unlikely in ecological datasets, employing MAR approaches (e.g., multiple imputation) may still be helpful when dealing with datasets where the mechanism of missingness is, in part, MNAR, because it will help mitigate problems caused by the MAR component (Nakagawa, 2015; van Ginkel et al., 2020).
In many cases MI and FIML will be appropriate, however fully Bayesian approaches are among the most powerful for dealing with missing data. Here, missing data are treated like any other unknown random variable that is represented by a probability distribution (i.e., the posterior distribution) and can be obtained by assigning priors to the unknown parameters. When data are MCAR or MAR, missingness does not depend on the unobserved response variable, meaning that any inference would be equivalent to that from a model for the observed data alone (Rubin, 1976; Seaman et al., 2013). However, construction of a joint model that accounts for the mechanism of missingness is required when data are MNAR, and this can take a variety of forms (e.g., selection, pattern mixture, shared parameter models) (Little and Rubin, 2019). This process may still involve making strong and unverifiable assumptions. The strengths of this approach are that: (1) Assumptions are made clear and explicit through the specification of priors as part of the model, and (2) missing data can be accommodated within a Bayesian framework and applied to both missing response and covariate data distributions without a need for new techniques for inference (Ibrahim et al., 2002, 2005). Understanding that Bayesian statistics are not yet widespread among ecologists, whichever approach is selected must be appropriate given the extent and type of data missingness.
The pandemic presents a unique situation where research and monitoring activities that vary widely in their goals and data collection techniques have all been affected by the same problem; the solutions to which may ultimately take similar forms. Missing data theory is an active area of research, and a growing number of techniques are available which offer useful or promising routes for robust inference in the face of missing data. It is not possible for us to review them all here, although we provide a summary of the most prominent approaches in Table 1. For additional coverage of the topic, we direct readers to McKnight et al. (2007), Daniels and Hogan (2008), Enders (2010); Nakagawa (2015), and Little and Rubin (2019).
Avoiding and Planning for Missing Data
The pandemic should prompt researchers and monitors to consider how they might mitigate the missing data problem in the future. Indeed, in specific cases the lockdown may motivate development and use of automated or remote data collection techniques that limit reliance on human site attendance. Many of these tools are already available and are rapidly becoming less costly (e.g., Wickert, 2014). However, validation of many techniques is still forthcoming, some data collection simply cannot be automated, and prohibitive costs of automation may preclude widespread adoption. Nonetheless, researchers can prepare for missing data at the study design and data collection stage by identifying variables most likely to suffer from data loss, as well as auxiliary variables that can be collected more reliably. These adjustments will ensure that even with missingness, data will meet the assumption of MAR rather than being relegated to MNAR (Nakagawa, 2015; Noble and Nakagawa, 2018). Automating the collection of auxiliary variables may increase its availability for dealing with missing data. Researchers interested in this approach should explore Two-method Measurement Design and Planned Missing Data Designs, both of which are active areas of research (Enders, 2010; Nakagawa, 2015; Noble and Nakagawa, 2018). Once lockdowns are rescinded and data collection has fully resumed, we may also see an increase in the use of backcasting methods which predict unobserved values that might have existed in the years where data collection was on hold, given the observed data (e.g., Le et al., 2008; Saghafian et al., 2018; Contreras-Reyes and Idrovo-Aguirre, 2020). These methods are distinct from others discussed in this paper and could serve as an effective alternative in data restoration for robust inference in the future.
Discussion
Missing data are now prevalent across virtually all longitudinal datasets in ecology, and similar gaps could arise in the future. COVID-19 lockdowns should therefore motivate researchers and environmental monitors to critically reassess how data collection is planned and missing data are dealt with. Failing to do so will surely lead to future instances of weak or faulty inference, with potentially severe impacts on academic research, environmental monitoring and management. The statistical tools discussed here are not new, but novel approaches continue to become available (Table 1). Multiple imputation and other model-based methods already have R libraries available and will be appropriate for many missing data scenarios encountered in ecology (Nakagawa and Freckleton, 2008; Nakagawa, 2015). More broadly, missing data theory and corrective measures should be covered more extensively in graduate statistics training in ecology and environmental science. We will also benefit from efforts to make approaches for handling missing data more accessible (e.g., via development of R packages and tutorials), and greater collaboration with statisticians. In light of the ongoing global environmental crisis it is increasingly evident that longitudinal data in ecology will play a critical role in understanding, anticipating, and possibly mitigating environmental changes. To meet these challenges, we have a responsibility to employ procedures that ensure reliable insight from our research and monitoring efforts.
Data Availability Statement
The datasets presented in this study can be found in online repositories. The names of the repository/repositories and accession number(s) can be found below: https://github.com/JenileeGobin/confronting-missing-data.
Author Contributions
TH, JG, and DM contributed equally to the study conception. TH wrote the initial manuscript draft, with JG and DM contributing significantly to the manuscript writing. All authors contributed to the article and approved the submitted version.
Funding
This research was supported by the NSERC Discovery program and Canada Research Chairs program.
Conflict of Interest
The authors declare that the research was conducted in the absence of any commercial or financial relationships that could be construed as a potential conflict of interest.
Publisher’s Note
All claims expressed in this article are solely those of the authors and do not necessarily represent those of their affiliated organizations, or those of the publisher, the editors and the reviewers. Any product that may be evaluated in this article, or claim that may be made by its manufacturer, is not guaranteed or endorsed by the publisher.
Acknowledgments
We thank the W.S. Burr, B.K. Sandercock, and reviewers for their critical feedback and constructive comments on earlier versions of this manuscript.
References
Allison, P. D. (2005). Imputation of categorical variables with PROC MI. SUGI 30 Proc. 2005 113–130, 1–14.
Bhaskaran, K., and Smeeth, L. (2014). What is the difference between missing completely at random and missing at random? Int. J. Epidemiol. 43, 1336–1339. doi: 10.1093/ije/dyu080
Buckley, R. (2020). Conservation implications of COVID19: effects via tourism and extractive industries. Biol. Conserv. 247:108640. doi: 10.1016/j.biocon.2020.108640
Button, K. S., Ioannidis, J. P. A., Mokrysz, C., Nosek, B. A., Flint, J., Robinson, E. S. J., et al. (2013a). Confidence and precision increase with high statistical power. Nat. Rev. Neurosci. 14:585. doi: 10.1038/nrn3475-c4
Button, K. S., Ioannidis, J. P. A., Mokrysz, C., Nosek, B. A., Flint, J., Robinson, E. S. J., et al. (2013b). Power failure: why small sample size undermines the reliability of neuroscience. Nat. Rev. Neurosci. 14, 365–376. doi: 10.1038/nrn3475
Chen, Q., and Ibrahim, J. G. (2013). A note on the relationships between multiple imputation, maximum likelihood and fully Bayesian methods for missing responses in linear regression models. Stat. Interface 6, 315–324. doi: 10.4310/sii.2013.v6.n3.a2
Conservation International (2021). Global Conservation Rollbacks Tracker. Available online at: https://www.conservation.org/projects/global-conservation-rollbacks-tracker (accessed February 18, 2021).
Contreras-Reyes, J., and Idrovo-Aguirre, B. J. (2020). Backcasting and forecasting time series using detrended cross-correlation analysis. Physica A 560:125109. doi: 10.1016/j.physa.2020.125109
Daniels, M. J., and Hogan, J. W. (2008). Missing Data in Longitudinal Studies: Strategies for Bayesian Modelling and Sensitivity Analysis. Boca Ratan, FL: Chapman & Hall/CRC, Taylor & Francis Group.
Donders, A. R. T., van der Heijden, G. J. M. G., Stijnen, T., and Moons, K. G. M. (2006). Review: a gentle introduction to imputation of missing values. J. Clin. Epidemiol. 59, 1087–1091. doi: 10.1016/j.jclinepi.2006.01.014
Dong, Y., and Peng, C.-Y. J. (2013). Principled missing data methods for researchers. SpringerPlus 2:222. doi: 10.1186/2193-1801-2-222
Gardner, C. (2020). Nature’s Comeback? No, the Coronavirus Pandemic Threatens the World’s Wildlife. Available online at: https://theconversation.com/natures-comeback-no-the-coronavirus-pandemic-threatens-the-worlds-wildlife-136209 (accessed April 14, 2020).
Gray, W. B., and Shimshack, J. P. (2011). The effectiveness of environmental monitoring and enforcement: a review of the empirical evidence. Rev. Environ. Econ. Policy 5, 3–24. doi: 10.1093/reep/req017
Haggert, A. (2020). From Air Pollution to Wildlife Sightings: How COVID-19 is Changing Our World. Canadian Geographic. Available online at: https://theconversation.com/natures-comeback-no-the-coronavirus-pandemic-threatens-the-worlds-wildlife-136209 (accessed April 10, 2020).
He, Y., Yucel, R., and Raghunathan, T. E. (2011). A functional multiple imputation approach to incomplete longitudinal data. Stat. Med. 30, 1137–1156. doi: 10.1002/sim.4201
Hughes, R. A., Heron, J., Sterne, J. A. C., and Tilling, K. (2019). Accounting for missing data in statistical analyses: multiple imputation is not always the answer. Int. J. Epidemiol. 48, 1294–1304. doi: 10.1093/ije/dyz032
Ibrahim, J. G., Chen, M.-H., and Lipstitz, S. R. (2002). Bayesian methods for generalized linear models with covariates missing at random. Can. J. Stat. 30, 55–78. doi: 10.2307/3315865
Ibrahim, J. G., Chen, M.-H., Lipstitz, S. R., and Herring, A. (2005). Missing-data methods for generalized linear models. J. Am. Stat. Assoc. 100, 332–346.
Jakobsen, J. C., Gluud, C., Wetterslev, J., and Winkle, P. (2017). When and how should multiple imputation be used for handling missing data in randomised clinical trials – a practical guide with flowcharts. BMC Med. Res. Methodol. 17:162. doi: 10.1186/s12874-017-0442-1
Le, N., Sun, L., and Zidek, J. V. (2008). Spatial prediction and temporal backcasting for environmental fields having monotone data patterns. Can. J. Stats. 29, 529–554. doi: 10.2307/3316006
Lepot, M., Aubin, J.-B., and Clemens, F. H. L. R. (2017). Interpolation in time series: an introductive overview of existing methods, their performance criteria and uncertainty assessment. Water 9:796. doi: 10.3390/w9100796
Li, L., Shen, C., Li, X., and Robins, J. M. (2013). On weighting approaches for missing data. Stat. Methods Med. Res. 22, 14–30. doi: 10.1177/0962280211403597
Little, R. J. A. (1988). A test of missing completely at random for multivariate data with missing values. J. Amer. Statist. Assoc. 83, 1198–1202. doi: 10.1080/01621459.1988.10478722
Little, T. D., and Rubin, D. B. (1987). Statistical Analysis with Missing Data. Hoboken, NJ: John Wiley & Sons, Inc.
Little, T. D., and Rubin, D. B. (2019). Statistical Analysis with Missing Data, 3rd Edn. Hoboken, NJ: John Wiley & Sons, Inc.
Mansournia, M. A., and Altman, D. G. (2016). Inverse probability weighting. BMJ 352:i189. doi: 10.1136/bmj.i189
Maxwell, S. E., Kelley, K., and Rausch, J. R. (2008). Sample size planning for statistical power and accuracy in parameter estimation. Annu. Rev. Psychol. 59, 537–563. doi: 10.1146/annurev.psych.59.103006.093735
McIntosh, E. (2020). Here’s Every Environmental proTection in Canada that Has Been Suspended, Delayed and Cancelled During COVID-19. Canada’s National Observer. Available online at: https://www.nationalobserver.com/2020/06/03/news/heres-every-environmental-protection-canada-has-been-suspended-delayed-and-cancelled (accessed June 3, 2020).
McKnight, P. E., McKnight, K. M., Sidani, S., and Figueredo, A. J. (2007). Missing Data: A Gentle Introduction. New York, NY: Guilford Press.
Nakagawa, S. (2015). “Missing data: mechanisms, methods, and messages,” in Ecological Statistics: Contemporary Theory and Application, eds G. A. Fox, S. Negrete-Yankelevich, and V. J. Sosa (Oxford: Oxford University Press), 81–105.
Nakagawa, S. (2017). “Missing data: mechanisms, methods, and messages,” in Ecological Statistics: Contemporary Theory and Application, eds G. A. Fox, S. Negrete-Yankelevich, and V. J. Sosa (Oxford: Oxford University Press), 81–105.
Nakagawa, S., and de Villemereuil, P. (2019). A general method for simultaneously accounting for phylogenetic and species sampling uncertainty via Rubin’s rules in comparative analysis. Syst. Biol. 68, 632–641. doi: 10.1093/sysbio/syy089
Nakagawa, S., and Freckleton, R. P. (2008). Missing inaction: the dangers of ignoring missing data. Trends Ecol. Evol. 23, 592–596. doi: 10.1016/j.tree.2008.06.014
Nakagawa, S., and Freckleton, R. P. (2011). Model averaging, missing data and multiple imputation: a case study for behavioural ecology. Behav. Ecol. Sociobiol. 65, 103–116. doi: 10.1007/s00265-010-1044-7
Noble, D. W. A., and Nakagawa, S. (2018). Planned missing data design: stronger inferences, increased research efficiency and improved animal welfare in ecology and evolution. BioRXiv [preprint] doi: 10.1101/247064
Patterson, J. E., Devine, B., and Mordecai, G. (2020). Rolling Back Canadian Environmental reguLations During Coronavirus is Short-Sighted. The Conversation. Available online at: https://theconversation.com/rolling-back-canadian-environmental-regulations-during-coronavirus-is-short-sighted-139636 (accessed June 14, 2020).
Pennisi, E. (2020). Pandemic carves gaps in long-term field projects. Science 368, 220–221. doi: 10.1126/science.368.6488.220
Perkins, N. J., Cole, S. R., and Harel, O. (2018). Principled approaches to missing data in epidemiologic studies. Am. J. Epidemiol. 187, 568–575. doi: 10.1093/aje/kwx348
Rubin, D. B. (1976). Inference and missing data. Biometrika 63, 581–592. doi: 10.1093/biomet/63.3.581
Saghafian, B., Aghbalaghi, S. G., and Nasseri, M. (2018). Backcasting long-term climate data: evaluation of hypothesis. Theoret. Appl. Climatol. 132, 717–726. doi: 10.1007/s00704-017-2113-x
Schafer, J. L., and Graham, J. W. (2002). Missing data: our view of the state of the art. Psychol. Methods 7, 147–177. doi: 10.1037/1082-989x.7.2.147
Seaman, S., Galati, J., Jackson, D., and Carlin, J. (2013). What is meant by “Missing at Random”? Stat. Sci. 28, 257–268.
Seaman, S. R., and White, I. R. (2013). Review of inverse probability weighting for dealing with missing data. Stat. Methods Med. Res. 22, 278–295. doi: 10.1177/0962280210395740
Tanner, M. A., and Wong, W. H. (1987). The calculation of posterior distributions by data augmentation. J. Am. Stat. Assoc. 82, 528–540.
Tierney, N., Cook, D., McBain, M., Fay, C., O-Hara-Wild, M., Hester, J., et al. (2021). Naniar: Data Structures, Summaries, and Visualisations for Missing Data. Version 0.6.1. Available online at: https://cran.r-project.org/package=naniar (accessed May 14, 2021).
van Buuren, S., and Groothuis-Oudshoorn, K. (2011). mice: multivariate imputation by chained equations in R. J. Stat. Softw. 45, 1–67.
van Ginkel, J. R., Linting, M., Rippe, R. C. A., and van der Voort, A. (2020). Rebutting existing misconceptions about multiple imputation as a method for handling missing data. J. Pers. Assess. 102, 297–308. doi: 10.1080/00223891.2018.1530680
Viglione, G. (2020). How COVID-19 Could Ruin Weather Forecasts and Climate Records. Nature. Available online at: https://www.nature.com/articles/d41586-020-00924-6/ (April 13, 2020).
Keywords: data missingness, data analysis, imputation, missingness mechanisms, data gap, full information maximum likelihood, Bayesian, COVID-19
Citation: Hossie TJ, Gobin J and Murray DL (2021) Confronting Missing Ecological Data in the Age of Pandemic Lockdown. Front. Ecol. Evol. 9:669477. doi: 10.3389/fevo.2021.669477
Received: 18 February 2021; Accepted: 26 July 2021;
Published: 19 August 2021.
Edited by:
Alex Perkins, University of Notre Dame, United StatesReviewed by:
Hannah Worthington, University of St Andrews, United KingdomJohanna Staerk, University of Southern Denmark, Denmark
Copyright © 2021 Hossie, Gobin and Murray. This is an open-access article distributed under the terms of the Creative Commons Attribution License (CC BY). The use, distribution or reproduction in other forums is permitted, provided the original author(s) and the copyright owner(s) are credited and that the original publication in this journal is cited, in accordance with accepted academic practice. No use, distribution or reproduction is permitted which does not comply with these terms.
*Correspondence: Thomas J. Hossie, dGhvc3NpZUB0cmVudHUuY2E=
†ORCID: Thomas J. Hossie, orcid.org/0000-0001-7777-4379; Jenilee Gobin, orcid.org/0000-0003-4411-2533; Dennis L. Murray, orcid.org/0000-0002-5710-042X