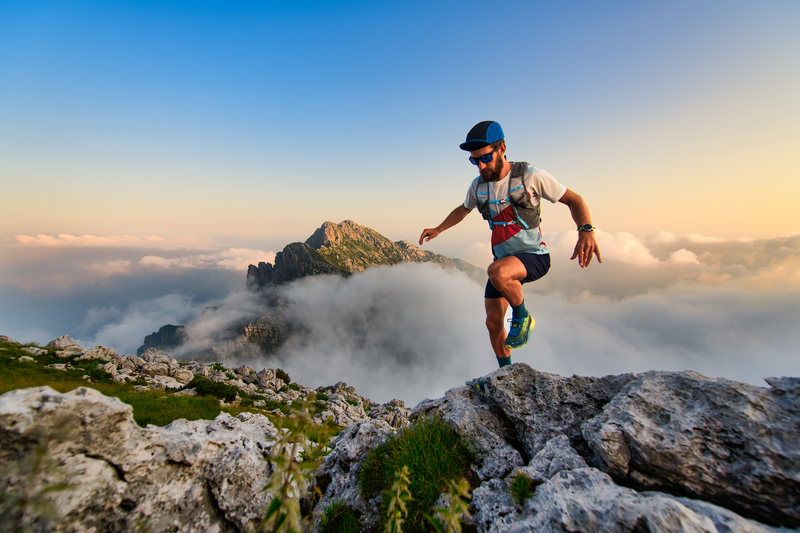
95% of researchers rate our articles as excellent or good
Learn more about the work of our research integrity team to safeguard the quality of each article we publish.
Find out more
ORIGINAL RESEARCH article
Front. Ecol. Evol. , 10 May 2021
Sec. Conservation and Restoration Ecology
Volume 9 - 2021 | https://doi.org/10.3389/fevo.2021.667949
This article is part of the Research Topic The Decline of Wild Bees: Causes and Consequences View all 8 articles
Bumblebees are important pollinators for many wild plants and crops. However, the bumblebee populations are seriously declining in many parts of the world. Hence, the bumblebee conservation strategy should be urgently addressed, and the species distribution modeling approach can effectively evaluate the potentially suitable areas for their conservation. Here, one of the most abundant and endemic species of bumblebee in China, Bombus pyrosoma, was selected to assess current and future climates’ influence on its distribution with MaxEnt. Nine high-resolution bioclimatic/environmental variables with high contribution rates and low correlations were used. Four of the nine bioclimatic/environmental variables, min temperature of the coldest month (bio_06), annual mean temperature (bio_01), precipitation of wettest month (bio_13) and radiation of warmest quarter (bio_26), were found to be the most critical factors influencing the distribution of B. pyrosoma. The modeling results showed that the areas with high and moderate suitability for B. pyrosoma covered 141,858 and 186,198 km2 under the current climate conditions. More than 85% of the sampling sites in 2019 were found to be suitable under the current scenario. Under the future A1B and A2 scenarios in 2050 and 2100, the areas with low and moderate suitability for B. pyrosoma increased. However, alarmingly, the high suitability areas decreased under the future A1B and A2 scenarios in 2050 and 2100. Furthermore, regions covering seven provinces of northern China were the most crucial for developing nature reserves for B. pyrosoma, with the following order of suitable areas: Gansu, Shanxi, Ningxia, Qinghai, Shaanxi, Hebei and Beijing. Our study highlights the impact of future climate changes on the distribution of B. pyrosoma, and conservation strategies should mitigate the threats posed by environmental changes, particularly in the current high suitability areas.
As important pollinators of many wild plants and crops, bumblebees are essential for maintaining the ecological balance and agricultural food production worldwide (Frankie et al., 2009; Arbetman et al., 2017; Bartomeus and Dicks, 2019). However, the distribution and abundance of bumblebees have decreased significantly due to habitat loss, environmental pollution, pathogens and pesticides, and non-native species invasion (Potts et al., 2010; Goulson et al., 2015; Cameron and Sadd, 2020). It has been reported that some bumblebee species have already exhibited apparent declines in Europe and North America, while the related information is limited in Asia (Cameron et al., 2011; Colla et al., 2012; Kerr et al., 2015). China has approximately 50% of the world’s bumblebee species, representing the highest number of bumblebee species of all countries in the world (Huang and An, 2018; Naeem et al., 2018). As China’s terrain and climate are diverse, it is difficult to obtain detailed distribution information for bumblebee species and monitor their population dynamics (Peng et al., 2009; An et al., 2011). Therefore, evaluating the distribution of bumblebees in China is vital to their conservation, which will contribute to the sustainable development of ecosystem management and agricultural food production (Williams et al., 2012; Naeem et al., 2020).
The bumblebee Bombus pyrosoma Morawitz (Hymenoptera, Apidae) is an endemic and abundant species in China (An et al., 2011). This species is distributed from western Liaoning Province to eastern Qinghai Province and has been abundantly reported in Shanxi, Hebei and Gansu Provinces (An et al., 2008; Wu et al., 2009; Ma et al., 2011). Varieties of natural and agricultural plants have been visited by this species, such as Helianthus annuus L., Leonurus sibiricus L., and Medicago sativa L. (Ma et al., 2011). Due to the advantages of large colonies that are easy to rear, this species has become a potential candidate species for artificial rearing (Peng et al., 2009). Although the geographic distribution and differentiation of B. pyrosoma have been explored, detailed distribution models and information on future climate influences are still lacking (Ma et al., 2011; Naeem et al., 2018; Liu et al., 2020).
Due to the vast area and diverse geographical environment, it is difficult to conduct a large-scale evaluation of bumblebee distribution by traditional methods (Williams et al., 2017). Species distribution models (SDMs) have become a prominent tool to predict and evaluate species distributions (Pearson et al., 2007; Elith and Leathwick, 2009; Xu et al., 2012). Different types of SDMs are developed according to the ecological niche, among which maximum entropy (MaxEnt) is widely used (Elith et al., 2006; Wang et al., 2017). MaxEnt acquires the maximum entropy of species distribution and constructs an SDM to predict species’ potential distribution based on the species distribution coordinates and environmental variables (Araujo et al., 2005; Phillips et al., 2006). MaxEnt is an effective tool for bumblebee prediction and evaluation in the EU, America, and Asia (Penado et al., 2016; Naeem et al., 2018; Naeem et al., 2019; Krechemer et al., 2020). In particular, the potential distribution of the invasive bumblebee species B. terrestris was researched in South America, Japan and China (Kadoya et al., 2009; Marshall et al., 2018; Naeem et al., 2018; Sirois-Delisle and Kerr, 2018; Aizen et al., 2019). Hence, MaxEnt will be used here to evaluate the distribution and conservation assessment of an endemic and abundant bumblebee, B. pyrosoma.
Species distribution modeling will help to improve the precision of conservation (Ma and Sun, 2018). Here, we aim to predict the distribution of B. pyrosoma and evaluate the effects of environmental variables on the habitat changes of this species. The selected current and future climatic variables will be used to assess the influence on the distribution of this species. The prediction and evaluation of the B. pyrosoma distribution will help to improve bumblebee conservation strategies.
The bumblebee species B. pyrosoma is an endemic species in China. A total of 6317 specimens of B. pyrosoma from 1905 to 2016 were collected from the museum of the Institute of Apicultural Research, Chinese Academy of Agriculture Science and the Institute of Zoology, Chinese Academy of Sciences. The specimens were recorded from seventeen provinces (Beijing, Chongqing, Gansu, Guizhou, Hebei, Henan, Hubei, Inner Mongolia, Liaoning, Ningxia, Qinghai, Shaanxi, Shanxi, Sichuan, Tianjin, Tibet, and Yunnan). Furthermore, in 2019, we collected 2119 B. pyrosoma individuals across its historical distribution in China. Specimens were sampled, and their location information was acquired with a handheld GPS (Garmin cs60).
Hence, the sampling sites were divided into three groups: historical samples (1905∼2000), current samples (2000∼2016) and test samples (2019). The adjacent modeled sample sites were thinned where the distance was less than 30 km (Supplementary Figures 1, 2). Data thinning was done by the spThin R package (0.2.0 Version) (de Oliveira et al., 2014; Aiello-Lammens et al., 2015; Santana et al., 2019).
Twenty-three bioclimatic/environmental variables, including nineteen bioclimatic variables at a 2.5 min resolution (∼5 km) and four environmental variables for the current period (1970–2000), were downloaded from the WorldClim-Global climate dataset1 (Santana et al., 2019; Supplementary Table 1). The four environmental variables, related to bumblebee distribution, were growing degree days (30 arc min (∼50 km), Atlas of the Biosphere, The Nelson Institute Center for Sustainability and the Global Environment, University of Wisconsin-Madison)2, rainy_days (5 arc min (∼10 km), Climate Change Agriculture And Food Security, CCAFS)3, global reference evapotranspiration [Global-ET0, 30 arc sec (∼1 km), Consultative Group for International Agriculture Research (CGIAR)]4, and radiation of warmest quarter (bio_26) (10 arc min (∼20 km), Global climatologies for bioclimatic modeling, CliMond)5 (Williams et al., 2014). The conversion tool in ArcGIS software was used to adjust the resolution of these four environmental variables similar to those of the 19 bioclimatic layers.
Future climatic data for 2050 and 2100 were downloaded from CliMond (Kriticos et al., 2012) based on the global climate model (GCM), CSIRO-Mk3.0 (Australia) with the Intergovernmental Panel on Climate Change (IPCC) IV SRES scenarios (A1B and A2) (Kriticos et al., 2014). The A1B scenario describes the balance between fossil and non-fossil resource use (Paterson et al., 2015). However, the A2 scenario depicts a changing world with high population growth but slow economic development and technological change (Paterson et al., 2015).
In the case of multicollinearity among those 23 bioclimatic/environmental variables, the Pearson correlation coefficient was used to select only those with low correlations (Wang et al., 2019). The Pearson correlation coefficient r of pairwise bioclimatic/environmental variables was calculated with the R project (Version 3.6.2). According to the contribution percentage, only those variables with a pairwise correlation efficiency less than 0.8 were retained. However, in the case of a correlation coefficient greater than 0.8, the variable with the highest contribution was selected (Casey et al., 2015; Naeem et al., 2018; Thapa et al., 2018; Mwakapeje et al., 2019).
The maximum entropy model was used to evaluate the potential distribution of the species. First, we conducted an initial model with the thinned sample records and twenty-three bioclimatic/environmental variables with MaxEnt (Version 3.4.1)6. In the initial model, we set “Random Test Percentage” to 25 and chose “Make pictures of predictions” and “Do Jackknife to measure variable importance.” The rest of the model settings were the defaults. Second, the relevant variables were assessed using the abovementioned method in section Bioclimatic/Environmental Variables. Third, the distribution of B. pyrosoma was simulated with the thinned sample records and filtered environmental variables. Response curves were chosen, and the rest of the model settings were consistent with the initial model.
All the bioclimatic/environmental layers were converted into ASCII files from raster grid format using ArcToolbox 2.0 in ArcGIS (Brown et al., 2017). The species distribution data were also converted into comma-separated value (CSV) format in Excel. The predicted distributions of B. pyrosoma were assessed and reclassified into different categories. Furthermore, the distribution area was assessed in km2 with zonal statistical analysis in ArcGIS.
The MaxEnt software is used to perform the Jackknife test to determine the relative importance and contribution of different variables in the distribution of species. Three approaches are used for this analysis: (1) Models created using all variables except excluding one, (2) models created using one variable, and all the other variables were excluded, (3) models created using all variables (Phillips et al., 2006; Santana et al., 2019). The response curves were calculated to determine the influence of climate change on the distribution of B. pyrosoma. These curves show how each environmental variable have an impact on the prediction of the MaxEnt model. Similarly, those response curves also show how the logistic prediction is changed with the environmental variable’s change (Phillips et al., 2006). The receiver operating characteristic (ROC) curve and the area under the curve (AUC) values were used to evaluate the accuracy of the species distribution model (Wang et al., 2019). The theoretical value of AUC set between 0.5 and 1. When the value of AUC is close to 1, the prediction accuracy of the model is high. The model classification index was as follows: “failure” is 0.50 < AUC < 0.60, “poor” is 0.60 < AUC < 0.70, “fair” is 0.70 < AUC < 0.80, “good” is 0.80 < AUC < 0.90, and “excellent” is 0.90 < AUC < 1.00 (Araujo et al., 2005; Wang et al., 2017). The distribution classification was divided into four categories: (I) unsuitable (0–0.50), (II) low suitability (0.50–0.75), (III) moderate suitability (0.75–0.90), and (IV) high suitability (0.90–1.00).
We compared the historical, current, and future distribution models with the sampling sites of B. pyrosoma in 2019. The distribution modeling accuracy and fitting degree between the models of different periods and all sampling sites were also assessed. We combined the current distribution of B. pyrosoma with the four future potential distributions in 2050 and 2100 (A1B and A2 scenarios). The raster calculator was used to calculate the suitable overlapping areas (Naeem et al., 2018; Zhang et al., 2018). The distribution area and proportion of different categories of habitat suitability were determined and assigned to China’s corresponding administrative division. The suitable area of each province was sorted to indicate the priority of conservation.
Pearson correlation of pairwise variables showed that the coefficients between 28 pairs of the 23 bioclimatic/environmental variables were greater than 0.8. Twenty-three bioclimatic/environmental variables were sorted according to the initial model’s percentage contribution (Table 1). Nine variables were selected based on the Pearson correlation coefficient and contribution rate. The selected variables were annual mean temperature (bio_01), mean diurnal range {bio_02 [mean of monthly (max temp-min temp)]}, isothermality [bio_03 (bio_02/bio_07) (× 100)], maximum temperature of warmest month (bio_05), minimum temperature of coldest month (bio_06), annual precipitation (bio_12) precipitation of wettest month (bio_13), precipitation seasonality [bio_15 (coefficient of variation)], and radiation of warmest quarter (bio_26). The value of the Pearson correlation coefficient between every two variables was < 0.8, and the cumulative contribution rate of the nine remaining variables was 100% (Figure 1A). The curve of omission on the training samples exhibited a similar trend as that on the test samples. The AUC values of the training data and test data were 0.952 and 0.941, respectively, in the current distribution model (Figures 1B,C). Therefore, the precision of the current distribution model was excellent according to the model evaluation index.
Table 1. Percent contributions and permutation importance of the bioclimatic/environmental variables.
Figure 1. Bioclimatic/environmental variable correlation analysis and model evaluation. (A) Correlation analysis of the independent variables. (B) Change in receiver operating characteristic (ROC) curve. (C) Change in area under the curve (AUC). The symbols of “***”, “**,” and “*” represent significance level of p-value “0–0.001,” “0.001–0.01,” and “0.01–0.05”.
Jackknife analysis showed that the minimum temperature of the coldest month (bio_06), precipitation of the wettest month (bio_13), annual mean temperature (bio_01) and annual precipitation (bio_12) were the most important environmental variables that affected the distribution of B. pyrosoma, with a training gain of more than 0.6 (Figure 2A). The radiation of the warmest quarter (bio_26) and the maximum temperature of the warmest month (bio_05) were also important bioclimatic/environmental variables, with training gains of more than 0.45. The contribution of the last three variables (bio_02, bio_03, bio_15) was more than 0.14.
Figure 2. Contribution and response curves of selected bioclimatic/environmental variables. (A) Jackknife test of variable importance in the distribution of B. pyrosoma. (B) Response curves of the nine variables.
The response curve thresholds of the nine bioclimatic/environmental variables were obtained (Figure 2B). Where the presence probability was more than 0.5, the bioclimatic/environmental variable threshold values were as follows: min temperature of the coldest month (bio_06) ranged from −16.78 to −4.10°C, precipitation of wettest month (bio_13) ranged from 81.33 to 151.65 mm, annual mean temperature (bio_01) ranged from 3.16 to 11.57°C, annual precipitation (bio_12) ranged from 353.75 to 764.47 mm, radiation of warmest quarter (bio_26) ranged from 181.00 to 208.00 W/m2, the maximum temperature of the warmest month (bio_05) ranged from 17.91 to 29.36°C, the mean diurnal range (bio_02) ranged from 8.95 to 13.07°C, isothermality (bio_03) ranged from 26.79 to 34.25, and precipitation seasonality (bio_15) ranged from 70.10 to 123.44.
The current distribution of B. pyrosoma shows that the suitable habitat is concentrated from central to northern China (Figure 3). The high suitability areas were located in eastern Qinghai, southern Gansu and southern Ningxia, parts of Shaanxi and Shanxi provinces. Moderate suitability was located in eastern Qinghai, southern Gansu, southern Ningxia, northern and southern Shaanxi, and parts of Shanxi, Hebei, Beijing, Inner Mongolia, Hubei, Hunan, and Chongqing provinces. The low suitability areas were located in eastern Inner Mongolia, western Liaoning, southern Hebei, parts of Shanxi, north and south of Shaanxi, southern Ningxia and southern Gansu, eastern Qinghai, parts of Sichuan, Chongqing, Hunan and Hubei provinces. According to the percentage of the distinct categories of suitability, the high, moderate, and low suitability areas represented 1.48% (141,858 km2), 1.95% (186,198 km2), and 2.72% (259,878 km2) of the total area of China, respectively.
Figure 3. The current potential distribution of B. pyrosoma predicted by MaxEnt based on the values of area under the curve (AUC). Low suitability (AUC = 0.50–0.75), moderate suitability (AUC = 0.75–0.90), high suitability (AUC = 0.90–1.00), and total suitability (AUC = 0.50–1.00).
The dynamics of the suitable habitat for B. pyrosoma were obtained (Figure 4). The total suitability area trend decreased, then increased, and then slightly decreased from the 1900s to 2100s. The suitability area of the current distribution decreased by 189,375 km2 and increased by 150,365 km2 compared to the historical distribution. Under the A1B scenario, the low suitability area increased by 95,400 km2 in 2050 and 80,400 km2 in 2100, and the moderate suitability area increased by 12,135 km2 in 2050 5,191 km2 in 2100 compared with the current suitability area. However, the high suitability area decreased by 44,636 km2 in 2050 and 36,580 km2 in 2100. Under the A2 scenario, the low suitable area increased by 93,178 km2 in 2050 and 83,178 km2 in 2100, and the moderate suitable area increased by 13,524 km2 in 2050 and 12,413 km2 in 2100 compared with the current suitability area. However, the high suitability area decreased by 42,414 km2 in 2050 and 34,636 km2 in 2100.
Figure 4. Variations in the predicted areas of the past (1905–2000), current (2000–2016) and future (2050 and 2100 based on CSIRO-Mk3.0 global climate model with A1B and A2 scenarios) distributions.
The overlapping suitability areas were also calculated by overlapping the current and future (2050 and 2100 A1B and A2 scenarios) potential distributions of B. pyrosoma (Figure 5). The total suitable area was 200,278 km2, which accounted for 2.10% of China’s total area. The areas of high, moderate, and low suitability were 42,778, 53,889, and 103,611 km2, respectively. Seven provinces were identified as important for the conservation of B. pyrosoma (Table 2). Gansu Province inhabited the most extensive distribution of high suitability areas, with 26,389 km2, which is more than six percent of the total area of the province. The second-largest high suitability area was 8,056 km2 (5.05%) in Shanxi. The Ningxia Hui Autonomous Region was the third most important area, with 4,722 km2 (8.97%). The highly suitable areas in Qinghai covered 2,222 km2 (0.31%), while Shaanxi covered 1,389 km2 (0.68%). Although there were no high suitability areas in Hebei and Beijing, the percentages of moderate and low suitability were large, covering 39,722 and 3,611 km2, respectively.
Figure 5. Suitability overlaying the current (2000–2016) and future (2050 and 2100 A1B and A2 SRES scenarios) potential distributions of B. pyrosoma. (A) High suitability over current and future potential distributions. (B) Moderate suitability over current and future potential distributions. (C) Low suitability over current and future potential distributions.
Table 2. Overlapping area (km2) and percentage (%) of B. pyrosoma distribution area in China and seven provinces.
Comparing sample records in 2019 and the modeled habitat showed a better fitness of the current and future habitat models than the historical habitat model. There were 37 sampling sites in 2019 (37.76% of all sampling sites) located in the unsuitable areas, 21 sampling sites (21.43%) in the low suitability areas, 20 sampling sites (20.41%) in the moderate suitability areas and 20 sampling sites (20.41%) in the high suitability areas under the historical habitat model (Figure 6). Under the current habitat model, there were 14 sampling sites (14.29%) located in the unsuitable areas, 19 sampling sites (19.39%) in the low suitability areas, 31 sampling sites (31.63%) in the moderate suitability areas and 34 sampling sites (34.69%) in the high suitability areas. Under the future habitat model, the fitness of the models was similar in all four scenarios (2050 and 2100 A1B and A2). Specifically, 5–8 sampling sites (5.10–8.16%) were located in the unsuitable areas, 18–24 sampling sites (18.37–24.49%) were located in the low suitability areas, 25–26 sampling sites (25.51–26.02%) were located in the moderate suitability areas, and 25–28 sampling sites (25.51–28.57%) were located in the high suitability areas.
Figure 6. The fitting degree of 2019 sample sites and predicted distribution in different time periods. (A) The overlap between 2019 sample sites and historical distribution (1905–2000). (B) The overlap between 2019 sample sites and current distribution (2000–2016). (C) The overlap between 2019 sample sites and future distribution (2050 A1B SRES scenarios). (D) The overlap between 2019 sample sites and future distribution (2050 A2 SRES scenarios). (E) The overlap between 2019 sample sites and future distribution (2100 A1B SRES scenarios). (F) The overlap between 2019 sample sites and future distribution (2100 A2 SRES scenarios).
Bumblebees contribute significantly to maintaining the natural ecological balance and crop production (Brown, 2011; Cameron and Sadd, 2020). To conserve these important pollinators, their potential distribution has often been evaluated by modeling tools (Elith et al., 2006; Naeem et al., 2018). In this study, the current distribution of the bumblebee B. pyrosoma was modeled based on historical sample records and validated with samples collected in 2019. Furthermore, the potential distribution of B. pyrosoma was simulated under future climate scenarios. The potential distribution and future suitability dynamics of B. pyrosoma will help to develop a conservation strategy.
Nine bioclimatic/environmental variables were selected in this study, and they are slightly different from the variables selected in previous studies (Casey et al., 2015; Naeem et al., 2019; Krechemer et al., 2020). The difference in the variable selection may be due to the different bumblebee species and their sampling sites. Certain variables play an important role in the distribution of certain bumblebees (Casey et al., 2015; Naeem et al., 2018). Currently, the minimum temperature of the coldest month (bio_06), precipitation of the wettest month (bio_13) and annual mean temperature (bio_01) made significant contributions to the model. Such conditions are present in the meadow of Wutai Mountain and Genting Mountain in North China, which also have grasslands with abundant flowers (An et al., 2008; Richardson et al., 2018; Naeem et al., 2019). Other than bioclimatic/environmental conditions, the abundance of foraging plants together with the cold environment and humidity will benefit bumblebees (Table 1). Most bumblebees are concentrated in temperate and subfrigid zones in the Northern Hemisphere, and these species are suitable for cold and humid climates (Penado et al., 2016; Streinzer et al., 2019).
Furthermore, the contribution of radiation in the warmest quarter (bio_26) cannot be ignored. When it was omitted, the radiation of the warmest quarter was the environmental variable that decreased the gain the most; therefore, this variable had the most information that was not available in the other variables (Figure 2A). The results are consistent with those of Williams’s study since extreme values reduce food-plant pollen and nectar production (Williams et al., 2014).
The modeled distribution of B. pyrosoma was mainly distributed in the north of the Qinling Mountains and south of the Inner Mongolia Plateau in northern China (An et al., 2011; Ma et al., 2011; Naeem et al., 2018). The suitable areas (high and moderate suitability collectively) covered 328,605 km2 (3.43%) under the current climate scenario, 297,361 km2 (3.09%) in 2050 on average, and 301,250 km2 (3.13%) in 2100 on average. The suitable area found in our current study was smaller than that found in a previous study (844,057 km2) (Naeem et al., 2018). The difference may be due to the use of thinned species records with different distances in our study. We thinned the records by distances of at least 30 km, whereas previously, the samples were thinned by distances of less than 10 km. Additionally, different environmental variables may lead to different model results. We used nine bioclimatic and environmental variables, while Naeem et al. (2019) used eight variables, five of which were the same in our study. The remaining three, temperature seasonality, precipitation of driest month and elevation, were different variables.
A1B and A2 are two different scenarios for the future climate. The future projection years of 2050 and 2100 were selected to provide a reasonable snapshot of two time periods to represent the near future and distant future. Under these two scenarios, the suitable habitat of B. pyrosoma will increase in the future. However, the high suitability area will decrease under both future scenarios (Figure 4). These trends were consistent with those found in the previous study. However, the fluctuations in the previous study were stronger than those found in ours. This difference may be due to the climatic scenarios and the sampling size. Naeem et al. (2019) used different climatic scenarios with two levels of representative concentration pathways (R) (2050s-RCP2.6, 2050s-RCP8.5, 2070s-RCP2.6, and 2070s-RCP8.5) from the Coupled Model Intercomparison Project Phase 5 (CMIP5) in the 5th assessment report of the IPCC. Besides, more than 700 sampling sites in our study were one hundred more sampling sites than in the previous study (Naeem et al., 2019). Furthermore, the B. pyrosoma population dynamics are similar to those in other reports of bumblebees, who also suffer from an existential crisis in the future (Kerr et al., 2015; Sirois-Delisle and Kerr, 2018).
Finally, the suitable areas for B. pyrosoma will extend in the future compared with the current distribution. However, high suitability areas will be lost, which cannot be neglected. The shrinkage in the high suitability area may be caused by future climate changes, agrochemicals, parasites and pathogenic factors (Goulson et al., 2015; Bartomeus and Dicks, 2019). In addition, it is expected that the interaction between climate change and land use, including agricultural lands, developed land, cropland, wetlands, or in the form of crop and grazing lands will exacerbate species range losses (Newbold, 2018; Sirois-Delisle and Kerr, 2018). We should also pay attention to the influence of those factors on the distribution of the species. In this study, the overlaid potential distribution under the current and four future climate scenarios improves the accuracy of conservation area identification. According to the overlaying habitat, conservation of B. pyrosoma should be established in the following administrative divisions: Gansu, Shanxi, Ningxia Qinghai, Shaanxi, Hebei, and Beijing provinces (Table 2). Gansu, Shanxi, and Ningxia provinces have the most significant proportions of high suitability areas, accounting for more than 5% of the province’s total area. These areas are recommended to focus on for primary conservation.
Our study evaluated the consistency between the distribution models of B. pysoroma and the resampling in 2019. More than 85% of the resampling sites were located in potentially suitable areas, and more than 40% of the resampling sites were highly suitable. More than 70% of the resampling sites were situated in future predicted distribution areas. Furthermore, we optimized the bioclimatic/environmental variables and thinned the sample sites to improve modeling accuracy. Through the data screening, modeling verification and analyzing previous studies, the species distribution model established by MaxEnt software was reliable (Elith et al., 2006; Naeem et al., 2018; Mwakapeje et al., 2019; Naeem et al., 2019).
In conclusion, the potential distribution of an endemic and abundant bumblebee species in China, B. pyrosoma, was modeled under the past, current, 2050 and 2100 climates (A1B and A2 scenarios). The temperature, precipitation and radiation of the warmest quarter were recognized as the most significant bioclimatic/environmental variables that affect bumblebees’ distribution. With climate change, the main potential distribution areas may increase, while the high suitability areas will decrease. Therefore, it is essential to develop a conservation strategy according to the suitability dynamics for bumblebees. Bioclimatic/environmental factors, potential distribution and conservation strategies should be combined to protect bumblebees in Asia.
The original contributions presented in the study are included in the article/Supplementary Material, further inquiries can be directed to the corresponding author/s.
XH, JLiu, GD, and JLi: conceptualization. XH, JLiu, MN, and JLi: methodology. XH, FM, and MN: software. XH, JLiu, GD, and JA: data curation. XH and JH: writing – original draft. XH, JH, and GD: visualization. JH and FM: supervision, funding. XH, JLiu, GD, MN, JLi, FM, JH, and JA: writing – reviewing and editing. All authors contributed to the article and approved the submitted version.
This project was financially supported in part by the biodiversity investigation, observation and assessment program of the Ministry of Ecology and Environment of China, the Agricultural Science and Technology Innovation Program (CAAS-ASTIP-2021-IAR) and the China Agriculture Research System (CARS-44).
The authors declare that the research was conducted in the absence of any commercial or financial relationships that could be construed as a potential conflict of interest.
The reviewer, GP, declared a past co-authorship with several of the authors, MN, JH, GD, to the handling editor.
We thank Yaning Zhang, Biao Wang, Yong Zhang, Yanjie Liu, Qi Zhang, Guangshou Zhang, and Shiwen Zhang for their help with sample collection.
The Supplementary Material for this article can be found online at: https://www.frontiersin.org/articles/10.3389/fevo.2021.667949/full#supplementary-material
Aiello-Lammens, M. E., Boria, R. A., Radosavljevic, A., Vilela, B., and Anderson, R. P. (2015). spThin: an R package for spatial thinning of species occurrence records for use in ecological niche models. Ecography 38, 541–545. doi: 10.1111/ecog.01132
Aizen, M. A., Smith-Ramírez, C., Morales, C. L., Vieli, L., Sáez, A., Barahona-Segovia, R. M., et al. (2019). Coordinated species importation policies are needed to reduce serious invasions globally: The case of alien bumblebees in South America. J. Appl. Ecol. 56, 100–106. doi: 10.1111/1365-2664.13121
An, J., Huang, J., Dong, J., and Zhou, B. (2011). Isolation and characterization of microsatellite markers from Bombus pyrosoma (Hymenoptera, Apidae), a bumblebee species endemic to China. Acta Entomol. Sin. 54, 1423–1432.
An, J., Jian, Y., Huang, J., Shao, Y., Wu, J., Li, J. L., et al. (2008). Bombus fauna (Hymenoptera, Apidae) in Shanxi, China. Acta Zootaxonomica Sin. 33, 80–88.
Araujo, M. B., Pearson, R. G., Thuiller, W., and Erhard, M. (2005). Validation of species-climate impact models under climate change. Glob. Change Biol. 11, 1504–1513. doi: 10.1111/j.1365-2486.2005.01000.x
Arbetman, M. P., Gleiser, G., Morales, C. L., Williams, P., and Aizen, M. A. (2017). Global decline of bumblebees is phylogenetically structured and inversely related to species range size and pathogen incidence. Proc. R. Soc. B Biol. Sci. 284:20170204. doi: 10.1098/rspb.2017.0204
Bartomeus, I., and Dicks, L. V. (2019). The need for coordinated transdisciplinary research infrastructures for pollinator conservation and crop pollination resilience. Environ. Res. Lett. 14:045017. doi: 10.1088/1748-9326/ab0cb5
Brown, J. L., Bennett, J. R., and French, C. M. (2017). SDMtoolbox 2.0: the next generation Python-based GIS toolkit for landscape genetic, biogeographic and species distribution model analyses. PeerJ 5:e4095. doi: 10.7717/peerj.4095
Brown, M. J. (2011). Conservation: The trouble with bumblebees. Nature 469, 169–170. doi: 10.1038/469169a
Cameron, S. A., Lozier, J. D., Strange, J. P., Koch, J. B., Cordes, N., Solter, L. F., et al. (2011). Patterns of widespread decline in North American bumble bees. Proc. Natl. Acad. Sci. U.S.A. 108, 662–667. doi: 10.1073/pnas.1014743108
Cameron, S. A., and Sadd, B. M. (2020). Global trends in bumble bee health. Annu. Rev. Entomol. 65, 209–232. doi: 10.1146/annurev-ento-011118-111847
Casey, L. M., Rebelo, H., Rotheray, E., and Goulson, D. (2015). Evidence for habitat and climatic specializations driving the long-term distribution trends of UK and Irish bumblebees. Divers. Distrib. 21, 864–875. doi: 10.1111/ddi.12344
Colla, S. R., Gadallah, F., Richardson, L., Wagner, D., and Gall, L. (2012). Assessing declines of North American bumble bees (Bombus spp.) using museum specimens. Biodivers. Conserv. 21, 3585–3595. doi: 10.1007/s10531-012-0383-2
de Oliveira, G., Rangel, T. F., Lima-Ribeiro, M. S., Terribile, L. C., and Diniz-Filho, J. A. F. (2014). Evaluating, partitioning, and mapping the spatial autocorrelation component in ecological niche modeling: a new approach based on environmentally equidistant records. Ecography 37, 637–647. doi: 10.1111/j.1600-0587.2013.00564.x
Elith, J., Graham, C. H., Anderson, R. P., Dudík, M., Ferrier, S., Guisan, A., et al. (2006). Novel methods improve prediction of species’ distributions from occurrence data. Ecography 29, 129–151. doi: 10.1111/j.2006.0906-7590.04596.x
Elith, J., and Leathwick, J. R. (2009). Species distribution models: ecological explanation and prediction across space and time. Annu. Rev. Ecol. Evol. Syst. 40, 677–697. doi: 10.1146/annurev.ecolsys.110308.120159
Frankie, G. W., Rizzardi, M., Vinson, S. B., and Griswold, T. L. (2009). Decline in bee diversity and abundance from 1972-2004 on a flowering leguminous tree, Andira inermis in Costa Rica at the interface of disturbed dry forest and the urban environment. J. Kansas Entomol. Soc. 82, 1–20. doi: 10.2317/JKES708.23.1
Goulson, D., Nicholls, E., Botias, C., and Rotheray, E. L. (2015). Bee declines driven by combined stress from parasites, pesticides, and lack of flowers. Science 347:1255957. doi: 10.1126/science.1255957
Huang, J., and An, J. (2018). Species diversity, pollination application and strategy for conservation of the bumblebees of China. Biodivers. Sci. 26, 486–497. doi: 10.17520/biods.2018068
Kadoya, T., Ishii, H. S., Kikuchi, R., Suda, S., and Washitani, I. (2009). Using monitoring data gathered by volunteers to predict the potential distribution of the invasive alien bumblebee Bombus terrestris. Biol. Conserv. 142, 1011–1017.
Kerr, J. T., Pindar, A., Galpern, P., Packer, L., Potts, S. G., Roberts, S. M., et al. (2015). Climate change impacts on bumblebees converge across continents. Science 349, 177–180. doi: 10.1126/science.aaa7031
Krechemer, F. D. S., Marchioro, C. A., and Butt, N. (2020). Past, present and future distributions of bumblebees in South America: identifying priority species and areas for conservation. Appl. Ecol. 57, 1829–1839. doi: 10.1111/1365-2664.13650
Kriticos, D. J., Jarošik, V., and Ota, N. (2014). Extending the suite of bioclim variables: a proposed registry system and case study using principal components analysis. Methods Ecol. Evol. 9, 956–960. doi: 10.1111/2041-210X.12244
Kriticos, D. J., Webber, B. L., Leriche, A., Ota, N., Macadam, I., Bathols, J., et al. (2012). CliMond: global high-resolution historical and future scenario climate surfaces for bioclimatic modelling. Methods Ecol. Evol. 3, 53–64. doi: 10.1111/j.2041-210X.2011.00134.x
Liu, Y., Zhao, H., Luo, Q., Yang, Y., Zhang, G., Zhou, Z., et al. (2020). De novo transcriptomic and metabolomic analyses reveal the ecological adaptation of high-altitude Bombus pyrosoma. Insects 11:631. doi: 10.3390/insects11090631
Ma, B., and Sun, J. (2018). Predicting the distribution of Stipa purpurea across the Tibetan Plateau via the MaxEnt model. BMC Ecol. 18:10. doi: 10.1186/s12898-018-0165-0
Ma, W., Qi, H., Zhang, Y., Liu, Y., and Shao, Y. (2011). The biological observation to Bombus pyrosome Morawitz in Shanxi province. J. Shanxi Agric. Sci. 39, 477–479.
Marshall, L., Biesmeijer, J. C., Rasmont, P., Vereecken, N. J., Dvorak, L., Fitzpatrick, U., et al. (2018). The interplay of climate and land use change affects the distribution of EU bumblebees. Glob. Change Biol. 24, 101–116. doi: 10.1111/gcb.13867
Mwakapeje, E. R., Ndimuligo, S. A., Mosomtai, G., Ayebare, S., Nyakarahuka, L., Nonga, H. E., et al. (2019). Ecological niche modeling as a tool for prediction of the potential geographic distribution of Bacillus anthracis spores in Tanzania. Int. J. Infect. Dis. 79, 142–151. doi: 10.1016/j.ijid.2018.11.367
Naeem, M., Huang, J., Zhang, S., Luo, S., Liu, Y., Zhang, H., et al. (2020). Diagnostic indicators of wild pollinators for biodiversity monitoring in long-term conservation. Sci. Total Environ. 708:135231. doi: 10.1016/j.scitotenv.2019.135231
Naeem, M., Liu, M., Huang, J., Ding, G., Potapov, G., Jung, C., et al. (2019). Vulnerability of East Asian bumblebee species to future climate and land cover changes. Agric. Ecosyst. Environ. 277, 11–20. doi: 10.1016/j.agee.2019.03.002
Naeem, M., Yuan, X., Huang, J., and An, J. (2018). Habitat suitability for the invasion of Bombus terrestris in East Asian countries: A case study of spatial overlap with local Chinese bumblebees. Sci. Rep. 8:11035. doi: 10.1038/s41598-018-29414-6
Newbold, T. (2018). Future effects of climate and land-use change on terrestrial vertebrate community diversity under different scenarios. Proc. R. Soc. B Biol. Sci. 1881:20180792.
Paterson, R. R. M., Kumar, L., Taylor, S., and Lima, N. (2015). Future climate effects on suitability for growth of oil palms in Malaysia and Indonesia. Sci. Rep. 5:11457. doi: 10.1038/srep14457
Pearson, R. G., Raxworthy, C. J., Nakamura, M., and Peterson, A. T. (2007). Predicting species distributions from small numbers of occurrence records: a test case using cryptic geckos in Madagascar. J. Biogeogr. 34, 102–117. doi: 10.1111/j.1365-2699.2006.01594.x
Penado, A., Rebelo, H., Goulson, D., Didham, R., and Brady, S. (2016). Spatial distribution modelling reveals climatically suitable areas for bumblebees in undersampled parts of the Iberian Peninsula. Insect Conserv. Diver. 9, 391–401. doi: 10.1111/icad.12190
Peng, W., Huang, J., Wu, J., and An, J. (2009). Geographic distribution and bionomics of six bumblebee species in North China. Chinese Bull. Entomol. 46, 115–168.
Phillips, S. J., Anderson, R. P., and Schapire, R. E. (2006). Maximum entropy modeling of species geographic distributions. Ecol. Model. 190, 231–259. doi: 10.1016/j.ecolmodel.2005.03.026
Potts, S. G., Biesmeijer, J. C., Kremen, C., Neumann, P., Schweiger, O., and Kunin, W. E. (2010). Global pollinator declines: trends, impacts and drivers. Trends Ecol. Evol. 25, 345–353. doi: 10.1016/j.tree.2010.01.007
Richardson, L. L., McFarland, K. P., Zahendra, S., and Hardy, S. (2018). Bumble bee (Bombus) distribution and diversity in Vermont, USA: a century of change. J. Insect Conserv. 23, 45–62. doi: 10.1007/s10841-018-0113-5
Santana, P. J., Kumar, L., Da, S. R., Pereira, J. L., and Picanco, M. C. (2019). Assessing the impact of climate change on the worldwide distribution of Dalbulus maidis (DeLong) using MaxEnt. Pest Manag. Sci. 75, 2706–2715. doi: 10.1002/ps.5379
Sirois-Delisle, C., and Kerr, J. T. (2018). Climate change-driven range losses among bumblebee species are poised to accelerate. Sci. Rep. 8:14464. doi: 10.1038/s41598-018-32665-y
Streinzer, M., Chakravorty, J., Neumayer, J., Megu, K., Narah, J., Schmitt, T., et al. (2019). Species composition and elevational distribution of bumble bees (Hymenoptera, Apidae, Bombus latreille) in the East Himalaya, Arunachal Pradesh, India. ZooKeys 851, 71–89. doi: 10.3897/zookeys.851.32956
Thapa, A., Wu, R., Hu, Y., Nie, Y., Singh, P. B., Khatiwada, J. R., et al. (2018). Predicting the potential distribution of the endangered red panda across its entire range using MaxEnt modeling. Ecol. Evol. 8, 10542–10554. doi: 10.1002/ece3.4526
Wang, R., Yang, H., Luo, W., Wang, M., Lu, X., Huang, T., et al. (2019). Predicting the potential distribution of the Asian citrus psyllid, Diaphorina citri (Kuwayama), in China using the MaxEnt model. PeerJ 7:e7323. doi: 10.7717/peerj.7323
Wang, Y. Q., Ma, J. F., Li, X. Q., Wang, Y. F., Cao, S., Xie, A. T., et al. (2017). The distribution of Athetis lepigone and prediction of its potential distribution based on GARP and MaxEnt. J. Appl. Entomol. 141, 431–440. doi: 10.1111/jen.12347
Williams, P., Huang, J., and An, J. (2017). Bear wasps of the Middle Kingdom: a decade of discovering China’s bumblebees. Antenna 41, 21–24.
Williams, P. H., An, J., Brown, M. J., Carolan, J. C., Goulson, D., Huang, J., et al. (2012). Cryptic bumblebee species: consequences for conservation and the trade in greenhouse pollinators. PLoS One 7:e32992. doi: 10.1371/journal.pone.0032992
Williams, P. H., Bystriakova, N., Huang, J., Miao, Z., and An, J. (2014). Bumblebees, climate and glaciers across the Tibetan plateau (Apidae:Bombus latreille). Syst. Biodivers. 13, 164–181. doi: 10.1080/14772000.2014.982228
Wu, J., An, J., Yao, J., Huang, J., and Feng, X. (2009). Bombus fauna (Hymenoptera, Apidae) in Hebei, China. Acta Zootaxonomica Sin. 34, 87–97.
Xu, Z., Zhao, C., and Feng, Z. (2012). Species distribution models to estimate the deforested area of Picea crassifolia in arid region recently protected: qilian Mts. National natural reserve (China). Pol. J. Ecol. 60, 515–524. doi: 10.3354/meps09902
Keywords: pollinator, bumblebee, Bombus pyrosoma, modeling, MaxEnt
Citation: Hu X, Liu J, Ding G, Naeem M, Li J, Ma F, Huang J and An J (2021) An Evaluation of Habitat Uses and Their Implications for the Conservation of the Chinese Bumblebee Bombus pyrosoma (Hymenoptera: Apidae). Front. Ecol. Evol. 9:667949. doi: 10.3389/fevo.2021.667949
Received: 15 February 2021; Accepted: 16 April 2021;
Published: 10 May 2021.
Edited by:
Michael Hrncir, University of São Paulo, BrazilReviewed by:
Jonathan Berenguer Koch, University of Hawaii at Hilo, United StatesCopyright © 2021 Hu, Liu, Ding, Naeem, Li, Ma, Huang and An. This is an open-access article distributed under the terms of the Creative Commons Attribution License (CC BY). The use, distribution or reproduction in other forums is permitted, provided the original author(s) and the copyright owner(s) are credited and that the original publication in this journal is cited, in accordance with accepted academic practice. No use, distribution or reproduction is permitted which does not comply with these terms.
*Correspondence: Fangzhou Ma, bWZ6QG5pZXMub3Jn; Jiaxing Huang, aHVhbmdqaWF4aW5nQGNhYXMuY24=
Disclaimer: All claims expressed in this article are solely those of the authors and do not necessarily represent those of their affiliated organizations, or those of the publisher, the editors and the reviewers. Any product that may be evaluated in this article or claim that may be made by its manufacturer is not guaranteed or endorsed by the publisher.
Research integrity at Frontiers
Learn more about the work of our research integrity team to safeguard the quality of each article we publish.