- 1Institute for Applied Ecosystem Studies, Northern Research Station, United States Department of Agriculture Forest Service, Rhinelander, WI, United States
- 2Department of Ecology and Evolutionary Biology, University of Toronto, Toronto, ON, Canada
Disturbances, both natural and anthropogenic, affect the configuration, composition, and function of forested ecosystems. Complex system behaviors emerge from the interactions between disturbance regimes, the vegetation response to those disturbances, and their interplay with multiple drivers (climate, topography, land use, etc.) across spatial and temporal scales. Here, we summarize conceptual advances and empirical approaches to disturbance interaction investigation, and used those insights to evaluate and categorize 146 landscape modeling studies emerging from a systematic review of the literature published since 2010. Recent conceptual advances include formal disaggregation of disturbances into their constituent components, embedding disturbance processes into system dynamics, and clarifying terminology for interaction factors, types, and ecosystem responses. Empirical studies investigating disturbance interactions now span a wide range of approaches, including (most recently) advanced statistical methods applied to an expanding set of spatial and temporal datasets. Concurrent development in spatially-explicit landscape models, informed by these empirical insights, integrate the interactions among natural and anthropogenic disturbances by coupling these processes to account for disturbance stochasticity, disturbance within and across scales, and non-linear landscape responses to climate change. Still, trade-offs between model elegance and complexity remain. We developed an index for the degree of process integration (i.e., balance of static vs. dynamic components) within a given disturbance agent and applied it to the studies from our systematic review. Contemporary model applications in this line of research have applied a wide range process integration, depending on the specific question, but also limited in part by data and knowledge. Non-linear “threshold” behavior and cross-scaled interactions remain a frontier in temperate, boreal, and alpine regions of North America and Europe, while even simplistic studies are lacking from other regions of the globe (e.g., subtropical and tropical biomes). Understanding and planning for uncertainty in system behavior—including disturbance interactions—is paramount at a time of accelerated anthropogenic change. While progress in landscape modeling studies in this area is evident, work remains to increase model transparency and confidence, especially for understudied regions and processes. Moving forward, a multi-dimensional approach is recommended to address the uncertainties of complex human-ecological dynamics.
Introduction
Reciprocal interactions between disturbances and forested landscapes have been a cornerstone of landscape ecological research and modeling for decades (Baker, 1989; Mladenoff and Baker, 1999; Seidl et al., 2011; Perera et al., 2015). A current frontier in this line of research is the spatially explicit investigation of disturbance interactions across spatial scales (Buma, 2015; Burton et al., 2020; Canelles et al., 2021). Within forested ecosystems, natural disturbances, anthropogenic disturbances, and climatic and anthropogenic drivers interact across a range of spatial scales to shape forested landscapes in term of patterns, processes, and functions (Figure 1) (Turner, 2010). Modeling disturbance interactions can be particularly challenging when and where the cumulative effects of such interactions, including non-linear and threshold behavior, result in catastrophic mega disturbance (Millar and Stephenson, 2015). Hence, a combination of empirical and modeling studies is needed to understand forest ecosystem dynamics that emerge from the interactions of multiple disturbances as well as biophysical and demographic drivers within forested landscapes (Fraterrigo and Rusak, 2008; Johnstone et al., 2016; Davis et al., 2018).
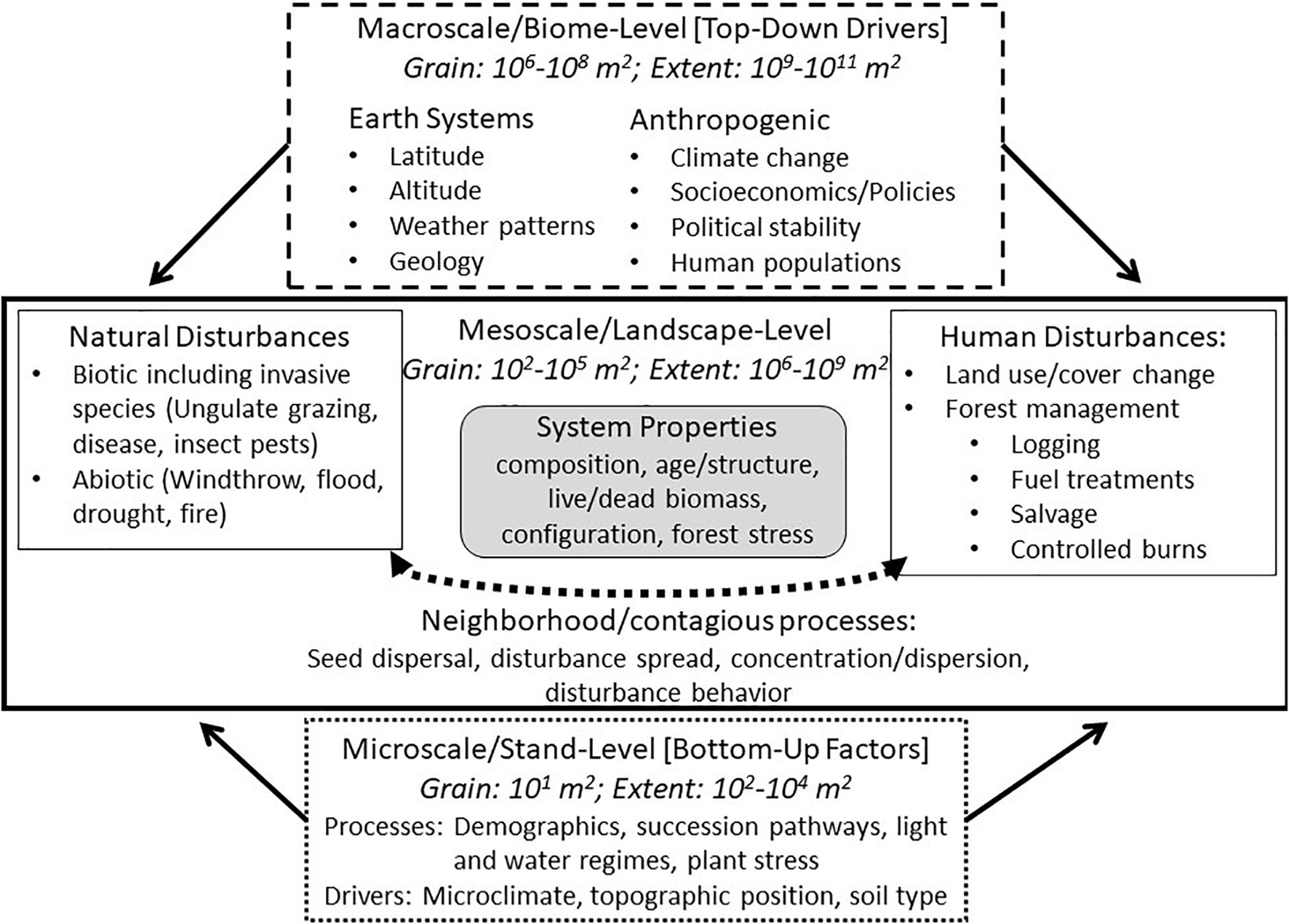
Figure 1. Disturbances at the mesoscale (landscape-level) are affected processes and drivers (bottom-up) at the microscale (stand-level) and higher-order drivers (top-down) at the macroscale (biome-level). Landscape disturbances interact in space and time with reciprocal feedback evident at the landscape level (double-arrow dotted lines). Cross-scale interactions occur where either both bottom-up processes and/or top-down drivers amplify or attenuate disturbance processes within landscapes via threshold behavior in time and space.
Natural disturbances (e.g., wildfire and insect outbreaks) have traditionally been investigated separately within different sub-disciplines of ecology (fire ecology and entomology, respectively) and further separated from the effects of human disturbances, focusing therefore on the properties of “disturbance regimes” (patch size distributions, severity, frequency or rotation length; Figure 2) and with an emphasis on stochasticity. Despite the apparent stochastic nature of natural disturbances, disturbance regimes generally emerge from feedbacks between internal system processes and external drivers across scales in time and space (Peters et al., 2011). Comparatively, anthropogenic disturbances (e.g., harvesting; Figure 2) have been traditionally viewed as deterministic (i.e., under human control). Yet, human systems are subject to analogous uncertainty and surprise caused by economics, social pressures, and political change that directly impact our ability to implement harvest and other land use plans at the temporal scale of forest rotations (Messier et al., 2019). Interactions among natural disturbances, human disturbances, and vegetation responses to those disturbances further influence system predictability. Proactive and adaptive management practices that embrace system uncertainty are therefore needed to respond to emerging “surprises” in disturbance regimes (Foley, 2005; Peters et al., 2011; Parrott and Meyer, 2012; Allen et al., 2014).
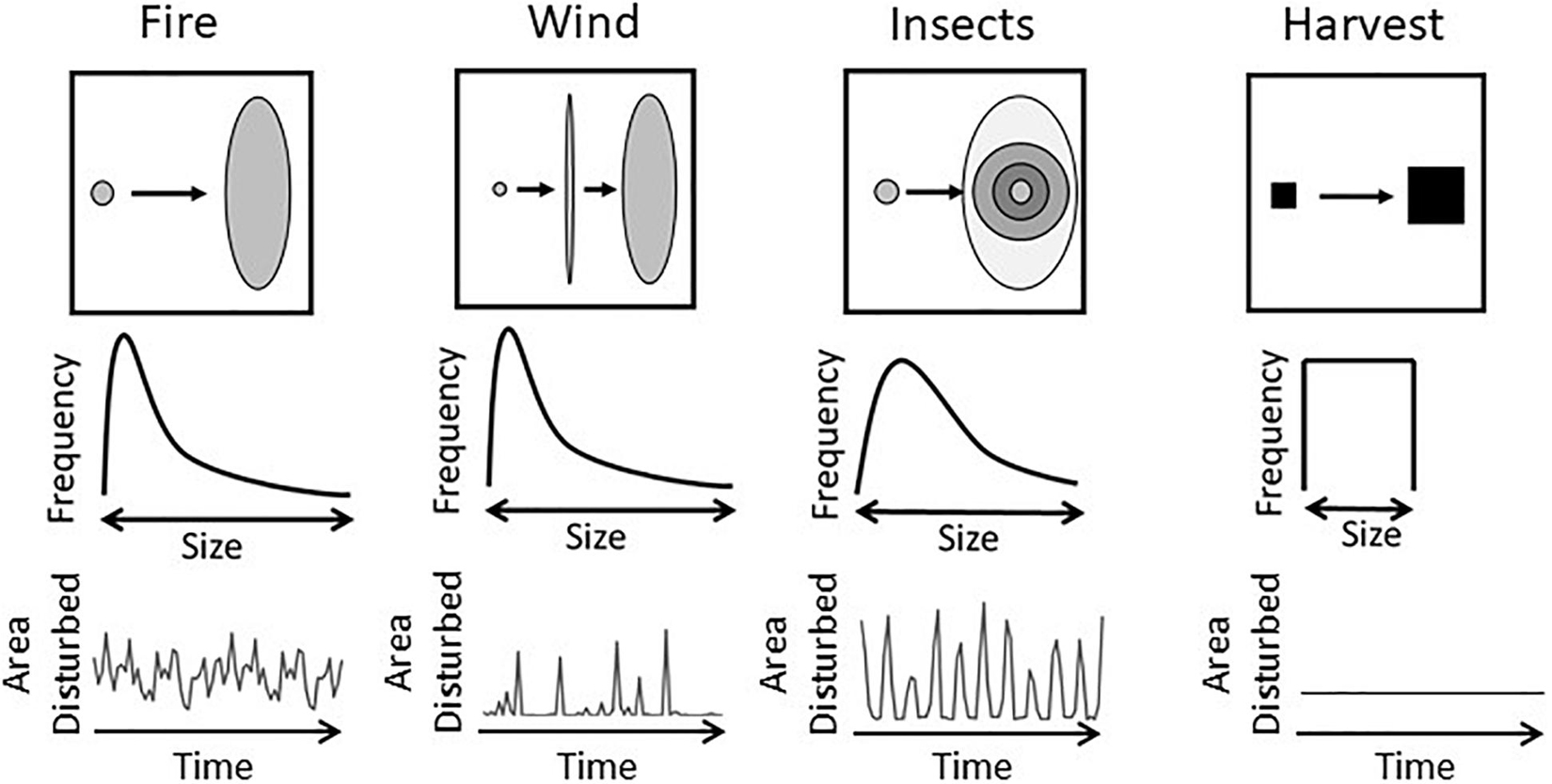
Figure 2. Disturbance regimes are traditionally characterized according to size distributions, shapes (e.g., wind), degrees of intensity (e.g., grayscale under insects), and temporal patterns.
Several conceptual advances have been proposed to help disentangle the emergent properties of disturbance interactions (Foley, 2005; Fraterrigo and Rusak, 2008; Turner, 2010; Peters et al., 2011; Buma, 2015; Messier et al., 2016; Kane et al., 2017; Davis et al., 2018; Ratajczak et al., 2018). These conceptual advances underscore the interplay between pattern and process in natural disturbance dynamics, deterministic and stochastic elements of anthropogenic disturbance, and uncertainty due to climate change that need to be accounted for in modeling frameworks of processes and disturbances across scales (Keane et al., 2015; Urban et al., 2016). Here, we review the conceptual advances and empirical approaches that help disentangle the apparent complexity of disturbance interactions. We further conducted a systematic review of forest landscape simulation modeling studies including more than one disturbance type published since 2010. A class of models known as forest landscape models (FLMs) dominated this field of study. We therefore overview the general design of FLMs, showing how recent developments have shifted from statistically-based disturbance regimes (i.e., static) to process-based methods where disturbance regimes and ecosystem responses are emergent behaviors (i.e., dynamic), and further expanded the ability to choose the degree of system feedbacks depending on the question at hand (Seidl et al., 2011; Keane et al., 2015; Perera et al., 2015). Studies were then categorized according to the specific disturbance interaction questions investigated (as clarified by recent conceptual advances) and the relative balance of static to dynamic model components across disturbance types. We argue for minimum standards in documentation – particularly as model complexity increases – for increased transparency and confidence in model results. We conclude highlighting the current limitations, frontiers, and directions in the understanding and modeling of disturbance interactions at landscape levels.
Conceptual Advances
Disturbances act upon the components of an ecosystem in a way that changes the structure defining the system (Pickett and White, 1985; Rykiel, 1985; Lindenmayer et al., 2017; Newman, 2019). Within forested ecosystems, a disturbance typically disrupts the functioning of its dominant life form (trees) via physical or chemical damage impacting growth and survival. The effects of disturbance can range from “pulse” disturbances that are concentrated in space and time and lead to abrupt change (Jentsch and White, 2019) to “press” disturbances that are diffuse in space and time and lead to cumulative system stress (Bender et al., 1984). The intensity of a disturbance is measured in terms of force, energy, or analogous quantity (e.g., density of insects), while the severity of the disturbance is the consequence of that disturbance intensity on the state of the system (Keeley, 2009). Diversity in life history traits among tree species and other life forms mediate the relationship between disturbance intensity and severity via adaptation (Noble and Slatyer, 1980). Defining characteristics of a disturbance also depend on the scale of observation (Allen and Hoekstra, 2015). For example, a disturbance may be locally abrupt and severe (e.g., killing individual trees) but broadly diffuse and mild if killed trees are thinly dispersed across a large area. Once disturbed, forest system traits such as crown closure, height, and composition can take a long time to recover via the processes of recolonization, growth, and succession, respectively. Disturbances therefore affect landscape spatial heterogeneity including tree-species composition, age structure, and configuration (e.g., James et al., 2011b; Sturtevant et al., 2014).
Disturbance Interaction Process and Terminology
The opportunity for disturbance interaction occurs when one event follows another. Kane et al. (2017) observed that the likelihood of such occurrence depends on both the frequency and size of each disturbance, where opportunities for disturbance interactions increase by chance alone as their respective frequency and size increase. The nature of the specific interaction may be determined by a range of factors such as the disturbance mechanisms at play, the precise sequence, extent, and arrangement of the overlap, and the time since the previous disturbance (Kane et al., 2017; Figure 3). Interactions may include one or more types defined by the constituent components of the disturbance event—its incidence/extent, its intensity, and (or) its effects (Kane et al., 2017). Any of these components may be positively or negatively affected, or otherwise unaffected by the previous event. The nature of the interaction can also take multiple forms—for example the effect of one disturbance on another, or the combined effect of two disturbances on an ecosystem property or function.
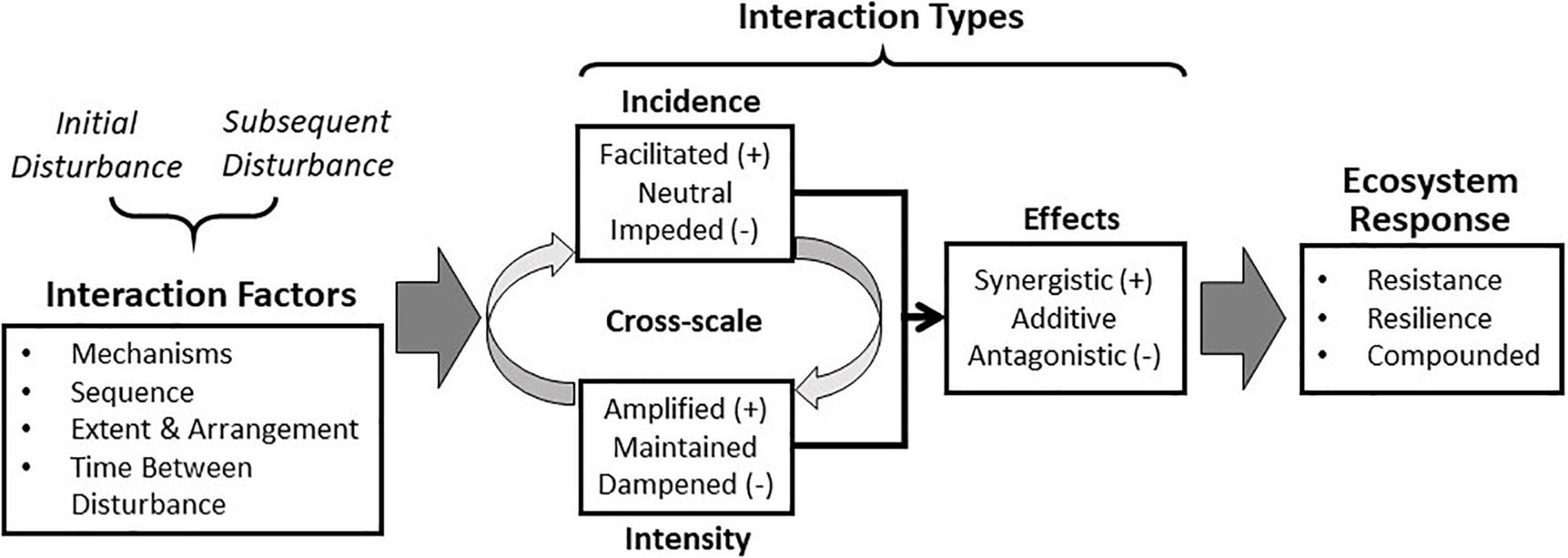
Figure 3. The factors and processes specific to disturbance interactions. A series of interaction factors define the degree and strength of the disturbance interaction, which may manifest through one or more interaction types any of which may be positive, negative, or neutral. Feedback between interaction types (and also drivers; Figures 1, 5) may manifest as cross-scale interactions. Ultimately, the effects of the disturbance interaction determine the ecosystem response (Figure 5). Reprinted from Publication: Forest Ecology and Management Vol 405, Characterizing interactions between fire and other disturbances and their impacts on tree mortality in western U.S., p188–199, 2017, with permission from Elsevier.
From a disturbance-interaction standpoint, the legacy from one disturbance becomes the new system state for the subsequent disturbance (Peterson, 2002). A “linked” disturbance interaction occurs when the legacy of one disturbance is a critical driver for another, affecting the likelihood, extent, or severity of the second disturbance (Buma, 2015). As elaborated by Kane et al. (2017), the specific components underlying the interaction are important to the nature of that interaction. For example, logging disturbance does not generally affect either the incidence (frequency and extent) or intensity of windstorms. In this regard, a wind event following logging disturbance might be considered a “random co-occurrence” (sensu Burton et al., 2020). However, the severity (i.e., effects) of a given wind event can be strongly linked to logging practices – for example, taller trees (generally older) tend to be more susceptible to wind damage than shorter (generally younger) trees, hard edges created by specific logging practices can increase the susceptibility of trees along that edge, and tree species with different rooting habits or wood densities can have differing responses to winds of a given intensity (Quine et al., 1995). Hence, patterns of wind effects may well be linked to the legacy of logging practices. Conversely, if salvage logging is a commonly applied practice, then the incidence and extent of salvage logging will be linked to the disturbance patterns created by wind events.
The occurrence, relative strength, direction, and duration of linked disturbance interactions are mediated by both internal ecosystem processes and external drivers. For example, insect disturbance leading to tree mortality can create pulses of heavy fuels that can set the stage for higher severity fire once the new fuels become combustible, but the period over which that legacy persists as a factor affecting fire depends on fuel decomposition rates (Fleming et al., 2002; but see Section “Empirical Approaches, Challenging Long-standing Dogma”). In some boreal systems, harvesting can transition highly flammable conifer forests to fire-resistant deciduous forest (Carleton and Maclellan, 1994; Cumming, 2001)—a legacy mediated by internal ecosystem recovery processes. Opportunities for disturbances to interact at a given location are therefore sensitive to the timing and sequence of when and where a given disturbance event overlaps a subsequent one, contingent on any relevant time-lag effects (Burton et al., 2020). For example, while tree-killing disturbances can influence soil stability underlying the likelihood of landslide disturbances, this linked disturbance interaction is constrained by topography (i.e., slope angle and position) (Carabella et al., 2019).
Certain disturbances are more sensitive to forest composition or age structure than others, and this sensitivity will determine the relative strength of the feedback between vegetation, the specific disturbance, and (by extension) linked interactions with other disturbances. For instance, most outbreaking insects are limited to a few host tree species, leading to relatively strong feedbacks between vegetation and insect disturbance impacts across all three interaction types (incidence, intensity, and effects; Figure 3) (Ohmart, 1989; Hennigar et al., 2008; Bentz, 2019). When landscape spatial pattern is shaped by the cumulative effects of one disturbance (e.g., logging; Sturtevant et al., 2014), the consequent spatial pattern can either enhance or limit subsequent disturbances in terms of their intensity, duration, and size (e.g., insect outbreak; Robert et al., 2018). Such disturbance “legacies” often accumulate across space and time, producing “landscape memory” (Peterson, 2002) with important consequences for future disturbance regimes and consequent landscape dynamics (Foster et al., 1998; Buma and Wessman, 2011).
“Looped disturbances” form a subset of linked disturbance interactions in the form of recurrent disturbances with feedbacks that create a metastability of the system (Burton et al., 2020). Classic looped disturbances are often associated as a component of disturbance regimes supporting a systems’ historic range of variability (HRV; Landres et al., 1999). For example, boreal conifers with fire-dependent life-history traits (e.g., serotiny) are both adapted and conducive to infrequent but extensive crown-fire events. Likewise, many temperate pine systems once dominant across much of the southern United States (Hanberry et al., 2020) are adapted (e.g., thick bark, high canopy) and conducive (e.g., grassy flammable understory) to frequent surface fire events (Figure 4). Looped disturbances of the same type can occur wherever life history traits of the primary vegetation are adapted to given type of disturbance so that it replicates itself – such as the build-up of fir regeneration in the understory that is released by the periodic defoliation by eastern spruce budworm (Choristoneura fumiferana Clemens) in the North American boreal forest (Baskerville, 1975). More complex looped disturbances across disturbance types are also possible when those types help reinforce each other in sequence (as illustrated by Burton et al., 2020).
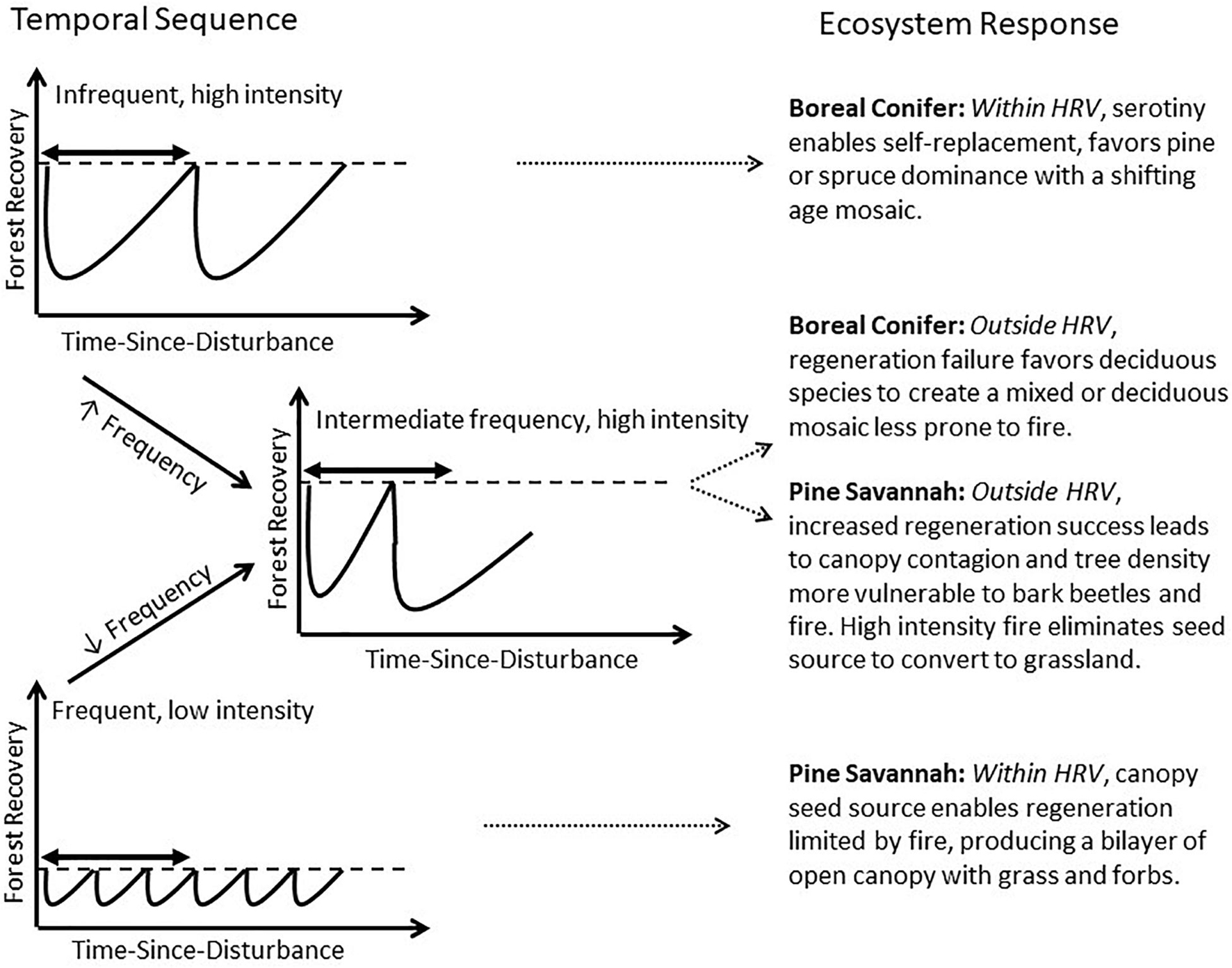
Figure 4. Compound disturbances are those affecting system recovery (i.e., resilience), where the Historic Range of Variability (HRV) serves as the context for ecosystem resilience. By example, serotinous boreal conifers are adapted to high intensity crown fire but require sufficient time to develop a canopy seed bank. Non-serotinous pines often require frequent fire to dampen regeneration to maintain open savannah structure. Fire frequencies intermediate to these extremes can consume the seed producing potential in either system, leading to a conversion to new systems (see text for references).
“Compound” disturbance interactions occur when the combination of two or more disturbances have a multiplicative effect over the state of the system (Buma and Wessman, 2011), often via the recovery processes affecting ecosystem resilience (Buma, 2015; Coop et al., 2020). In the above example for boreal conifers, two fires in rapid succession can burn the tree regeneration of a serotinous species before they have sufficient time to produce new seeds—dramatically delaying subsequent system recovery (Figure 4). Indeed, starkly different vegetation communities resulted from different combinations and sequences of fire and wind in Minnesota as the life history strategies of different tree species were affected quite differently by each disturbance type and their compound disturbance effects (Johnstone et al., 2016). By contrast, regeneration failure due to more frequent fire is a feature of pine savannah (Figure 4) (Hanberry et al., 2020). Decreasing fire frequency enhances regeneration success, leading to increased stem density and canopy connectivity that make the systems more susceptible to catastrophic disturbances such as bark beetles, crown fire, or both in combination (Negrón and Fettig, 2014; Clarke et al., 2016). The consequence of the restriction of a fundamental structuring agent in this example (frequent surface fires) is therefore a bifurcation of the system to the extremes: either closed canopy forest or grassland (Figure 4). Analogous patterns have been observed in many regions (e.g., western North America; Coop et al., 2020). The potential for compound disturbance is likely increasing as climate change can directly and indirectly affect disturbance processes (Soranno et al., 2014; Becknell et al., 2015), human land use intensification is disrupting natural disturbance regimes on a global scale (Foley, 2005), and increasing mobility and transportation increases novel introductions of pests and disease (Liebhold et al., 2017). Such broad-scaled changes can have non-linear “cascading effects” resulting in disturbance regime shifts and transforming the system to alternative states (Buma and Wessman, 2011; Buma, 2015; Johnstone et al., 2016; Ramsfield et al., 2016; Burton et al., 2020).
The effects of the disturbances and their interactions depends on a larger context of system dynamics. This larger context includes (1) external drivers operating at regional scales [e.g., biome constraints (Burton et al., 2020), anthropogenic pressure (Turner, 2010)] (Figure 1), (2) states of the system (e.g., vegetation composition, age structure, soil conditions, etc.) that determine the behavior and effects of (3) disturbance mechanisms (e.g., defoliation, stem breakage, and combustion) that in turn determine the new state of the system (Peters et al., 2011; Figure 5). The new state is considered the legacy of the disturbance, upon which internal ecosystem processes (e.g., recolonization, growth, and succession) enable subsequent system recovery (Peterson, 2002, Johnstone et al., 2016). The type of ecosystem response, as defined by Kane et al. (2017; Figure 3), depends upon how the disturbance legacy relates to the internal processes of the system. If the essential structure (i.e., tree density, size, and composition) remains effectively intact, the ecosystem response is one of resistance. If the system requires a longer recovery period but remains within the natural variability of the broader ecosystem, the ecosystem response to the disturbance interaction is one of resilience. If the recovering system falls outside that natural variability the disturbances compounded to result in an alternate system state (Figure 5). External drivers such as climate and climate change interact with disturbances via three pathways (Seidl et al., 2017) – directly via its effect on a disturbance property such as incidence or intensity, indirectly via effects on internal processes that modify system properties that in turn affect the disturbance process, and through a secondary interaction with another disturbance process that in turn affects the focal disturbance (i.e., the focus of this paper).
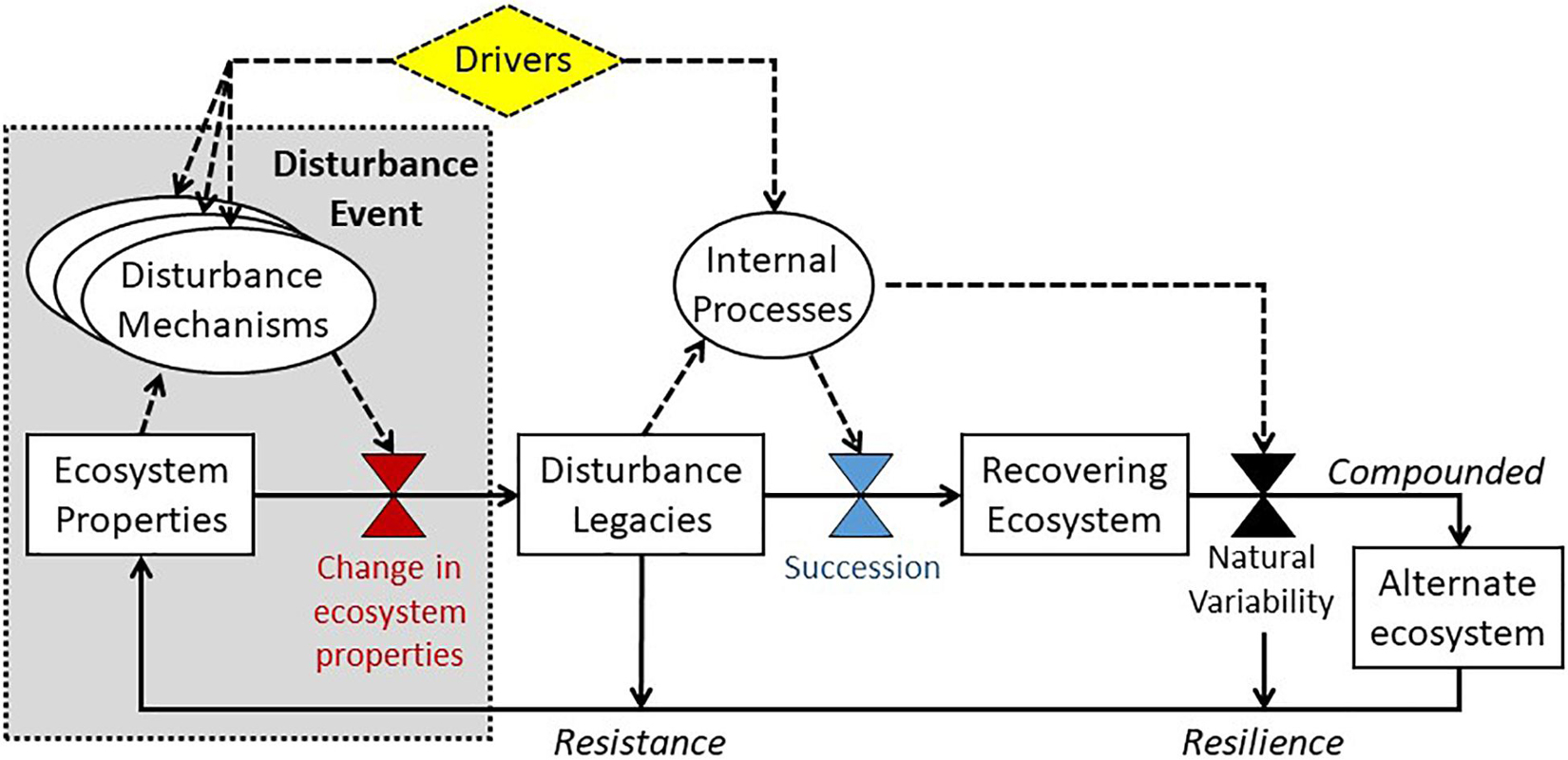
Figure 5. Disaggregating disturbance mechanisms, drivers, and internal processes affecting ecosystem response to disturbance interactions. Drivers (yellow) refer to the microscale and macroscale factors affecting mesoscale dynamics in Figure 1. Hourglass symbols represent system changes that can either be abrupt (red – rapid disturbance effects), gradual in time (blue – succession), or gradual in space and time (black – natural variability in local system states). Depending on the degree of change in system properties, the legacies that remain, and the recovery processes, the mesoscale ecosystem response can be one of resistance, resilience, or (if overlapping disturbances compound to impede recovery processes) transformation to an alternative system. (Adapted from Peters et al., 2011).
Cross-Scale Interactions
To understand processes affecting multiple disturbances and their interactions, integration of processes and disturbances operating at more than one scale is needed (O’Neill et al., 1996; Wu and David, 2002; Peters et al., 2007; Soranno et al., 2014) (Figure 1). Living and non-living elements of a system tend to interact most strongly with other elements that have characteristic process rates and spatial scales similar to their own (Allen and Hoekstra, 2015), leading to more closely-linked feedbacks within a single domain of scale (Messier et al., 2016). For example, at tree and stand-levels, life history traits of tree species affect demographic (mortality and recruitment), ecosystem (nutrient cycling), and disturbance (e.g., flammability, plant defense, etc.) processes that can include neighborhood effects that may also be self-reinforcing (Frelich and Reich, 1999). Nonetheless, the statistical properties of disturbance regimes (i.e., Figure 2) are not defined by processes at any one scale, but rather they emerge from the interactions of processes across scales (Figures 1, 5). So while compartmentalizing system dynamics into scale-specific components is a useful way to disentangle system complexity (Allen and Hoekstra, 2015), cumulative changes at one scale can either amplify or attenuate system behaviors across scales—a phenomenon known as a “cross-scale interaction” (Peters et al., 2004, 2007; Figure 3). For example, when local fuels become interconnected across landscapes to the point that firestorms (fire events that create their own weather) are possible, then discontinuities that previously served as fuel breaks may become irrelevant (Peters et al., 2004).
Cross-scale interactions often underlie dramatic change as a system shifts from a strong negative effect to a strong positive one. More precisely, different processes operating at different scales can work synergistically to amplify disturbances across scales. For example, tree-killing bark beetles subject to strong, non-linear and density-dependent feedbacks (e.g., Raffa et al., 2008) are often linked to other disturbance types. Under low-density endemic situations, individual healthy trees easily defend against attacking beetles. Outbreaks are generally triggered by drivers that enable beetle populations to grow sufficiently to overwhelm the resources of healthy trees. In Europe, windstorm events provide a pulse of food resources in the form of weakened or fresh-killed trees (i.e., a disturbance legacy) that enables European spruce bark beetle (Ips typographus L.) populations to increase to the point they can overcome adjacent healthy tree defenses, such that a strong negative feedback (strong defense) changes to a strong positive feedback (ample food) (Kausrud et al., 2012). Such an effect can be further amplified if wind events overlap periods of drought, where trees are already stressed and defenses weakened (Kausrud et al., 2012). Climate warming in central Europe serves as a common driver increasing the frequency and intensity of windstorms, the frequency and intensity of drought events, and decreasing generation time of I. typographus to dramatically increase population growth potential (Senf and Seidl, 2018, Figure 6). Climate-driven synergies underlying explosive outbreaks of this insect have been further exacerbated by a long history of spruce plantation establishment in the region (Jansen et al., 2017), leading to relatively continuous food resources (Seidl et al., 2016b, Figure 6). While this example appears like a “perfect storm” event, it is a pattern replicated across many forest insect systems across the globe, particularly in bark beetle systems subject to similarly strong non-linear feedback processes (Burton et al., 2020; Kneeshaw et al., 2021).
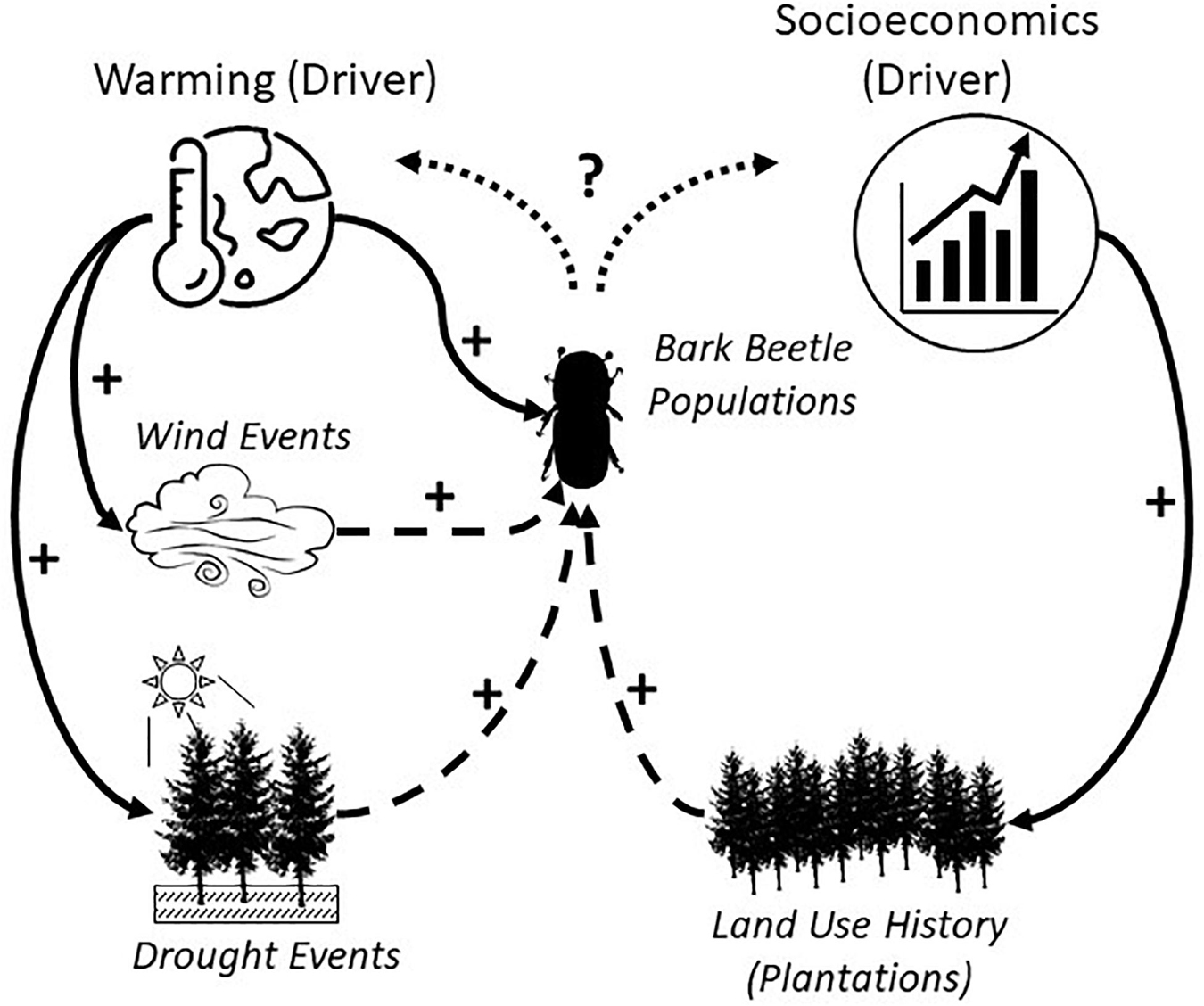
Figure 6. Direct and indirect interactions of disturbances and drivers contributing to the amplification of a disturbance agent, illustrated using the European spruce beetle example from central Europe (see Section “Cross-Scale Interactions”).
Insights From Conceptual Advances
Current conceptual advances in disturbance interaction investigation include formal disaggregation of disturbances into their constituent components, embedding the disturbance process into system dynamics affected by both external drivers and internal processes (Figure 5), and explicitly recognizing the interaction factors, types, and ecosystem responses (Figure 3). While early modeling investigations of disturbance interactions focused only simple binary interactions (Figure 7; Mladenoff et al., 1996), the desire to include more processes structuring landscapes has spurred development and application of more complicated and complex models including many interacting disturbances having direct and indirect effects (Figure 7) – a theme we will return to in Section “Modeling Approaches.” Furthermore, under the Anthropocene, socio-economic drivers are crucial to include as well in forest disturbance models (e.g., Figure 6).
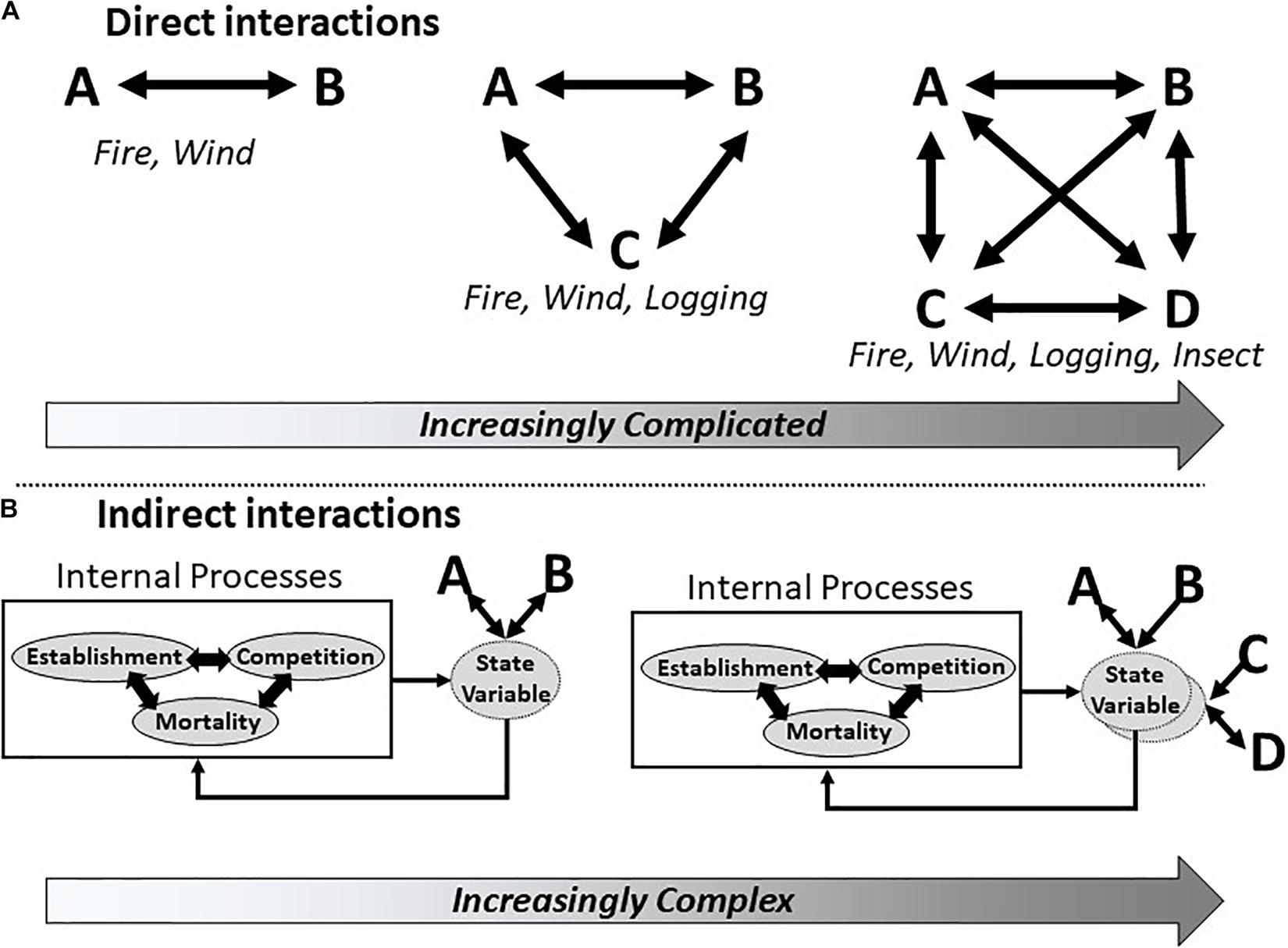
Figure 7. Challenges and approaches to simulating multiple disturbance interactions. When disturbance interactions are simulated as direct (A), the number of interactions can multiply quickly to become increasing complicated, especially if the interactions are bidirectional (i.e., double-arrows). Indirect interactions are those mediated through ecosystem state variables, such as composition or fuel (B). Depending on model design, it may be more elegant (i.e., fewer interactions) to explicitly model the indirect processes affecting disturbance processes, but such models may also be more interdependent and therefore more complex.
Importantly, the same drivers affecting disturbance mechanisms may also influence the internal processes affecting ecosystem response (Figure 5). The sum total of the drivers’ effects on disturbances, internal processes, and their interactions can lead to changes in system properties and system behaviors that can be either gradual or abrupt, depending on the direction and form of the effect (Ratajczak et al., 2018). Empirical disturbance interaction studies (see Section “Empirical Approaches”) should therefore take care to define the nature and form of the interaction explicitly, while models (see Section “Modeling Approaches”) should effectively capture the form, direction, and magnitude of interaction processes, including accounting for processes that transcend spatiotemporal scales.
Empirical Approaches
In the last decade, there have been several excellent papers reviewing interactions among disturbances. For example, Kleinman et al. (2019) reviewed compound disturbance interactions reported in nearly 300 journal articles, finding that such studies tended to be concentrated in North America, and focused primarily on fire, wind, and salvage logging. Salvage logging interactions have been more extensively reviewed by Leverkus et al. (2018) and Thorn et al. (2018), demonstrating a concentration of such studies in North America and Europe. Kane et al. (2017) reviewed fire interactions with other disturbance types concentrating on studies from the western United States (Figure 3). Kolb et al. (2016) reviewed the responses of biotic disturbances (insects, disease, and parasitic plants) to drought in the United States. Emerging from their review was a series of observed trendlines between different classes of insects and disease and their impact response to drought severity. Canelles et al.’s (2021) systematic review focused on disturbance interactions between insect disturbance and other forest disturbance types, and included a synthesis revealing different processes underlying those interactions. Seidl et al. (2017) performed a meta-analysis of the direct, indirect, and interaction effects of climate change on disturbance processes. Among the commonalities of these different synthesis articles is the geographic concentration of studies primarily in North America and Europe, an emphasis on certain disturbance types, with important knowledge gaps in mass-movement disturbances such as avalanches and landslides, meteorological events beyond wind and drought (e.g., ice storms), and disease. The reviews further noted an emphasis on the amplifying effects of disturbance interactions, with fewer empirical examples of the buffering effects of such interactions. While most of these reviews contained landscape modeling studies, they were not the primary focus of the research. Here, we present different empirical approaches to investigating disturbance interactions as a fundamental source of knowledge underlying the conceptualization and implementation of disturbance interactions within landscape models (Table 1).
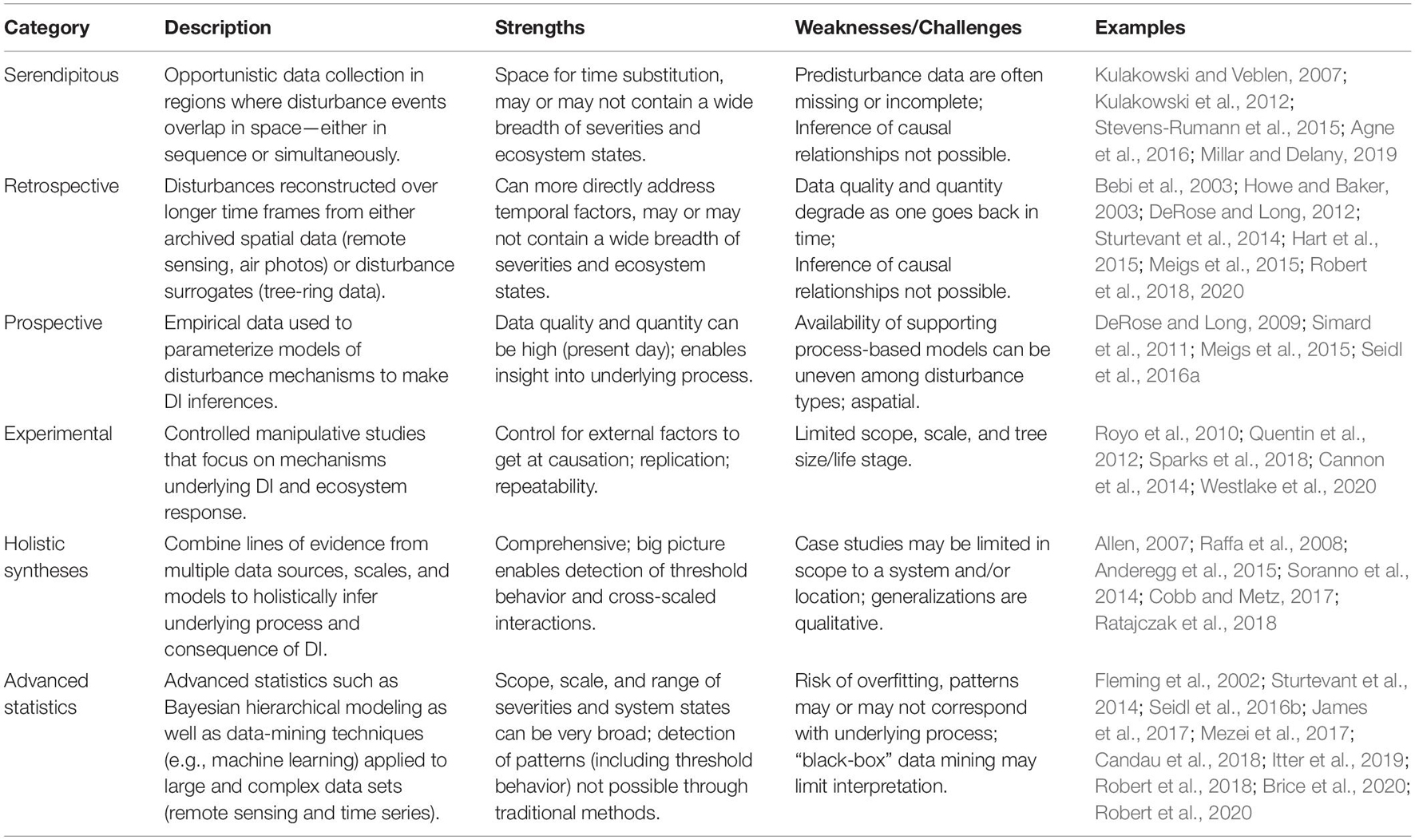
Table 1. Strengths and weaknesses of alternative empirical approaches for investigating disturbance interactions (DI).
Serendipitous Studies
The study of disturbance interactions in forested ecosystems has unique challenges because it is nearly impossible to manipulate for the overlap of two (or more) disturbances in the field. Consequently, there are many serendipitous correlational studies in the disturbance interaction literature. For example, the extensive bark beetle epidemics affecting western North America in the last two decades, coincident with both widespread drought (Raffa et al., 2008), and highly active fire seasons (Hart et al., 2015) enabled a broad range of opportunistic disturbance-interaction studies (Kulakowski and Veblen, 2007; Kulakowski et al., 2012; Stevens-Rumann et al., 2015; Agne et al., 2016; Millar and Delany, 2019), including the widespread study of salvage logging and its effect on ecosystem response and recovery (Leverkus et al., 2018). Such studies are often descriptive in nature, documenting patterns rather than understanding the processes producing them. Nonetheless, as the Anthropocene increasingly enhances disturbances under climate change, such disturbance overlap may become increasingly frequent (Seidl et al., 2016b; Burton et al., 2020; Kim et al., 2020).
Retrogressive and Prospective Studies
One common approach for investigating disturbance interactions is “retrogressive” (sensu Simard et al., 2011), where investigators reconstruct disturbances over a longer period of time, using a variety of data sources such as archived satellite imagery, air photos, dendrochronology, and disturbance records (e.g., Bebi et al., 2003; Howe and Baker, 2003; DeRose and Long, 2012; Sturtevant et al., 2014; Hart et al., 2015; Meigs et al., 2015; Robert et al., 2018, 2020). A challenge facing retrogressive approaches is that the quality of data defining underlying drivers, such as forest composition or age structure, generally degrades the further back in time one investigates (McKenzie and Perera, 2015; Robert et al., 2020) (Table 1). Conversely, empirical data defining a given disturbance legacy (e.g., fuel) are often used to drive models to infer future disturbance behavior (e.g., flame length) (DeRose and Long, 2009; Simard et al., 2011; Meigs et al., 2015; Seidl et al., 2016a). Such “prospective” studies, including those referenced above, typically apply models at the plot or stand scale as their method to infer consequent system behavior.
Experimental
A number of recent manipulative experiments have focused on disturbance mechanisms and their interactions on a variety of ecosystem responses. For example, Royo et al. (2010) examined the interactive effects of canopy gaps, ungulate browsing pressure, and fire via girdling, fencing, and prescribed fire, respectively. The authors found substantial synergistic effects leading to greater herbaceous diversity under the combined disturbances relative to any one disturbance alone. Quentin et al. (2012) applied a combination of irrigation and mechanical defoliation to investigate the interactive effects of water supply and leaf consumption on eucalyptus (Eucalyptus globulus Labill) plantations in Tasmania. Sparks et al. (2018) investigated the interactive effects of drought stress and fire on western larch (Larix occidentalis Nutt.) seedlings and found that severely drought-stressed seedlings had higher survival following fire relative to moderately drought-stressed seedlings, in part due to the physiological response to drought. Cannon et al. (2014) simulated windthrow disturbance by the static winching of trees to investigate consequent fire behavior, burn intensities, and consequent effects of those intensities related to elevated fuels on vegetation composition. Such studies can support the development of more process-based models enabling the simulation of disturbance interactions based on first principles (Figures 3, 5). The challenges in their application relate to their inherent limitations, such as the extrapolation of disturbance or stressor responses across tree life stages (Máliš et al., 2016; Dayrell et al., 2018), or the scale or intensity of experimental vs. natural disturbances (Cannon et al., 2014) (Table 1). Indeed, it is often the spatial dynamics of disturbances and their interactions that simulation models are designed to investigate, precisely because empirical measurement of such processes are difficult to quantify explicitly in space. Nonetheless, experimental studies can inform even spatial processes, such as the spatial concentration of ungulate herbivory associated with burned locations, and the cascading effects on plant species diversity across space (Westlake et al., 2020).
Holistic Syntheses
Despite the value of process identification and quantification, a whole systems approach is often necessary to fully understand the nature and behavior of disturbance interactions. For example, Anderegg et al. (2015) offered a data-driven conceptual model to capture the processes underlying interactions between temperature, precipitation, tree stress, and the different physiological, chemical, and ecological constraints influencing herbivory by bark beetles and defoliators that can lead to widespread mortality under climate change. Likewise, Cobb and Metz (2017) adapted the heuristic “disease triangle”, traditionally applied to forest and plant pathology, to understand the landscape ecology of tree diseases. The disease triangle consists of the pathogen, its host, and the environment conducive to the infection of the host by the pathogen. From the perspective of disturbance interactions, past disturbance events from various agents might contribute to the “environment” point of the triangle that can either facilitate or impede infection rates. Such syntheses can serve as the foundation for process-based landscape simulation models.
Case study syntheses further enable the investigation of disturbance interactions from a more holistic systems perspective. Evidence for the relative strength of specific interactions are built from diverse data sources—often at widely different scales—and gaps in knowledge may be addressed through a combination of prospective modeling and speculation based on systems and scaling theory (Peters et al., 2007). For example, Allen (2007) investigated cross-scaled interactions among drought, bark beetles, fire, and erosion in northern New Mexico based on a conceptual model that included non-linear dynamics that could either amplify or ameliorate the propagation of the different disturbances at play. Synthesized data sources spanned seven distinct studies ranging in spatiotemporal scale from weekly dendrometer (i.e., physiology) measurements to regional maps of inter-annual forest dieback developed via remote sensing. Such syntheses may be uniquely poised to address the sharp non-linearities associated with cross-scaled disturbance interactions that can lead to fundamental disturbance regime shifts. However, broader inference from such case studies will remain limited until the greater body of underlying theory can be rigorously tested (Allen et al., 2014) (Table 1).
Advanced Statistics
Analysis of ecological data in recent decades has shifted from the use of analytically tractable statistical methods constrained by parametric assumptions, such as ordinary least-squared regression and analysis of variance, to more advanced but computationally intensive methods such as mixed effects models, Bayesian inference, and machine learning techniques (Touchon and McCoy, 2016; Holmes and Huber, 2018). Such recent methods are better able to address the complexities of ecological datasets that are rapidly accumulating, including data collected via remote sensing and other automated sensors, as well as time-series data from long-term monitoring and experiments.
The combination of advanced statistical techniques and rich data resources enabled the modeling of underlying processes, legacy interactions, and driver roles related to disturbance interactions in time and space. For example, For example, Mezei et al. (2017) examined temporal patterns in disturbance rates of the European spruce beetle as a function of wind disturbance, prior beetle disturbance, and climatic variables affecting brood frequency. The authors used generalized additive models to allow detection of non-linear relationships, and applied multi-model inference (Burnham et al., 2011) to select from a set of candidate models reflecting plausible alternative hypotheses of underlying processes. Hierarchical and hidden process modeling better accommodate the variability and uncertainties inherent in ecological research (Gimenez et al., 2014), as well as non-linear responses due to the interactions among disturbances and climatic drivers (Brice et al., 2020), leading to more complete understanding of complex phenomena like disturbance interactions. As such, Itter et al. (2019) applied Bayesian hierarchical modeling to tree ring data in eastern and western regions of boreal Canada to evaluate the separate and combined impacts of tree defoliation and drought on tree growth patterns. They found a synergistic effect of defoliation plus drought on the “ecological memory” of the disturbances in terms of their temporal recovery period. Thus, advanced statistical modeling techniques can be applied to complex datasets to determine the relative importance of disturbance types and factors that affect the state of forest ecosystems.
Similarly, machine learning techniques are often more appropriate to modeling complex and non-linear relationships (Olden et al., 2008). For example, Candau et al. (2018) applied random forest methods to define spatial domains in central Canada where fire and eastern spruce budworm defoliation were likely to interact, based on compositional (i.e., host) and climatic gradients (i.e., moisture). Deep learning methodologies such as convolutional neural networks have been applied to extract useful patterns from complex data, such as imagery and LiDAR, although their application has focused on extracting patterns rather than understanding the processes underlying those patterns (Brodrick et al., 2019). Machine learning techniques are both diverse and evolving, and do have limitations such as propensity to over fit models and (particularly in the case of neural nets) lower transparency in the model-building process (Olden et al., 2008; Liu et al., 2018). Nonetheless, such methods may detect relationships or domains of scale that are poorly understood and worth more explicit attention.
Challenging Long-Standing Dogma
Regardless of the empirical approach, a critical role of empirical studies is to challenge long-standing assumptions of disturbance interactions that often find their way into landscape models. For example, the persistent dogma of “insect disturbance increases fuel load and therefore fire risk”—has been confronted with conflicting empirical evidence. Analysis of spruce eastern budworm outbreaks suggested that they increased forest fire probability in the first 20 years post-outbreak (Fleming et al., 2002), while Meigs et al. (2015) found that fire probability was lower after a western spruce budworm (Choristoneura freemani Razowski) event. These two studies were similar in that they were retrospective studies that evaluated whether the area burned increased during periods of budworm outbreaks, using a range of lag periods (i.e., time since outbreak). By contrast, James et al. (2017) investigated a similar question for spruce budworm in a similar geographic area to that of Fleming et al. (2002) but included weather drivers as covariates in the analyses. They found that the magnitude of defoliation effects was lower than those associated with weather. Likewise, a literature review of bark beetle-fire interactions in western North America concluded the effects of mountain pine beetle (Dendroctonus ponderosae Hopkins) disturbance on fire occurrence and severity were ambivalent (Simard et al., 2008). A subsequent study applied prospective modeling to focus on a particular fire process (i.e., probability of active crown fire; Simard et al., 2011). The authors considered the changes in fuel characteristics with time since disturbance (i.e., dead needles in canopy, deposited needles, fallen trees, and understory development), weather characteristics affecting fire behavior (wind speed and moisture) and interactions between the disturbance legacy and weather drivers (e.g., micrometeorology below canopy). As in Simard et al. (2011), James et al. (2017) found that the potential amplifying effects of the fuel disturbance legacy from the outbreak were constrained by fire weather conditions. Hicke et al. (2012) found that categorizing studies according to a conceptual model of expected lags in different fuel components as they related to different stages of bark beetle outbreaks led to more consistency in results and consequent fire implications across studies.
Insights From Empirical Studies
Empirical study of processes and drivers governing disturbance interactions are prerequisites of plausible models that simulate their dynamics. The different empirical approaches to the investigation of such interactions each have their role to play in the development and application of such models. Empirical study should justify when and where the added complexity of disturbance interactions is warranted, and confront persistent dogma based on perception rather than data. While interest in this line of research has increased in recent decades, disturbance interactions remain complex and challenging to quantify. Recent conceptual advances (see Section “Conceptual Advances”) are helping to refine the questions and terminology to channel empirical research, while advances in statistical methods, coupled with increasingly detailed data sources, provide additional tools to probe the characteristically messy data for useful insights. As evidenced by a series of recent reviews, research in this area is active but also unevenly distributed across geography, biomes, and disturbance types. By extension, such deficiencies limit the scope of landscape modeling in this line of research.
Modeling Approaches
The application of landscape models with multiple disturbances has exploded with the availability of advanced landscape models and increasing accessibility to computing power. We systematically reviewed the literature using the online search engine ISI Web of Science. Our initial review (access date August 15, 2020) applied the keywords “forest∗”, “landscape∗,” “simulation∗,” and “disturbance∗”; where we searched abstracts for landscape simulation studies including least two simultaneous disturbance agents. Landscape simulation studies were identified as those falling within the range of spatial scales falling within the landscape to regional extent (i.e., 5.0 × 101 − 5.0 × 107 ha) defined by Seidl et al. (2017), with model designs that allowed for spatial interactions among finer-scaled entities (i.e., grid cells or polygons). We further limited our review to papers from 2010 forward to focus on the most recent studies with the latest technology. Our initial search yielded 81 papers, however, we found it missed a number of relevant studies. We therefore repeated the literature review of the same search engine, using the names of the modeling software identified in the first review as keywords. This second review, with a final access date of June 06, 2021, identified an additional 66 papers meeting the above criteria (total = 146). The full details of the systematic review and the resulting citation list are provided in Supplementary Materials.
We note that our review methodology had consequences for the final set of papers included in the review. Landscape simulation studies that included more than one disturbance agent were included regardless of whether the investigation of disturbance interactions was stated as an explicit objective. In reality, investigators include multiple disturbance types for a wide diversity of questions that fall along a spectrum of explicit to implicit attention to the interactions of those disturbances within the simulations. Conversely, we did not include studies focused on a single disturbance agent, such as the fire frequency interactions illustrated in Figure 4. Doing so would retain all landscape simulation studies that simulated single disturbance regimes. However, we did retain studies investigating interactions between different agents of the same type, for example, between multiple insect species, or between wildfire and prescribed burning practices. Further, certain classes of models were excluded that have relevance to the broader question of disturbance interactions, but were not landscape models per se. Prospective modeling studies (Table 1) of disturbance interaction were commonly investigated using plot or stand level models (e.g., Simard et al., 2011). Many such studies covered a large geographic extent (e.g., Jain et al., 2020), but could not account for contiguous spatial processes fundamental to landscape ecology. Similarly, aspatial forest optimization models aggregate local dynamics across broad spatial units without spatial interaction within or between units. This is also the case for dynamic global vegetation models (DGVMs) that aggregate disturbance processes within course-resolution cells without any spatial interactions within or between cells. We also identified a couple fine-resolution land surface model applications that lacked spatial processes. All such aspatial model applications were excluded from our review. It is also possible that the initial use of the term “landscape” may have neglected models from some disciplines (e.g., hydrology) that use alternative terms (i.e., watershed, basin, etc.) to define their geographic extent.
Human land management was included in most of the disturbance interaction studies surveyed (79%), though only 7 studies included land use/land cover (LULC) change as a disturbance agent. By comparison, the most frequently investigated natural disturbance type from our review was fire (65%), followed by wind (38%) and insects (37%). Less frequently investigated disturbance types included water balance (drought or flooding; 15%), mammalian browsing/grazing (10%), and mass movement (i.e., erosion, debris flows, landslides, avalanche; 7%). Only two interaction studies included a simulated disease, perhaps due to a lack of empirical studies (Cobb and Metz, 2017).
Consistent with past reviews of disturbance interactions more generally (see Section “Empirical Approaches”), studies identified by our systematic review were unevenly distributed across continents and biomes (Table 2). Nearly two thirds of the studies were from North America, primarily from the United States and Canada, including nearly all of the boreal examples. Close to a quarter of the studies were from Europe, focused on temperate and alpine systems, but also including some Mediterranean examples. Studies from Asia accounted for about 10 percent of the total, distributed a little more evenly across biomes, including several studies from China. We found only 3 studies from Australia, one from South America, and none from Africa. Clearly there are underrepresented regions of the world within this field of research, most notably within tropical and subtropical systems. However, some of this uneven distribution may be attributed to our search methodology. We suspect the inclusion of the keyword “savanna∗” would have expanded the geographic scope a bit further. Searching on formal model names, while clearly essential in terms of the numbers of studies identified, may have biased the results to regions of the world where those models are applied. Custom models implemented in more generic software such as R were identified within the first search, but not the second name-specific search; this method may have also missed studies from under-represented regions. Nonetheless, our systematic review represents a strong cross-section of the landscape modeling investigations of disturbance interactions.
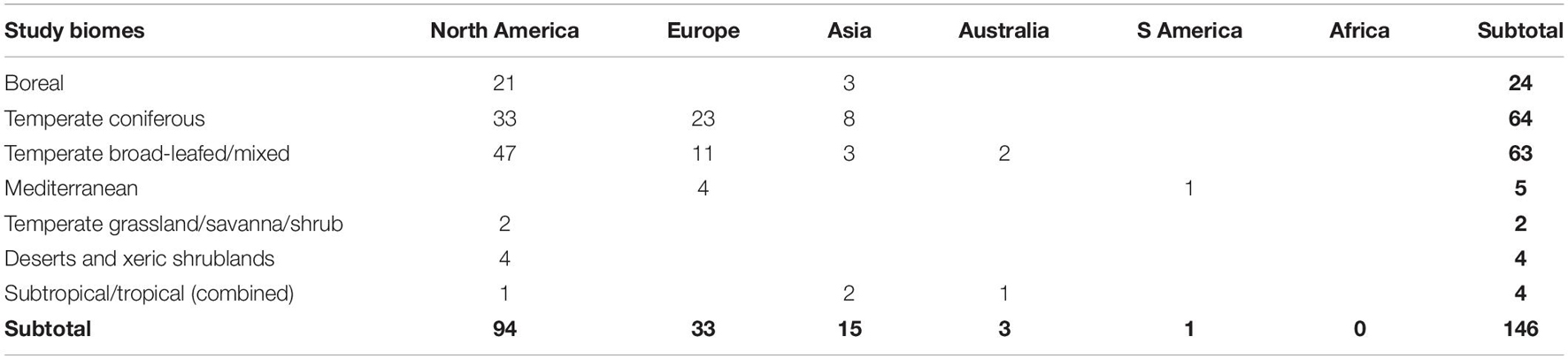
Table 2. Geographical distribution of disturbance interactions studies covered by the systematic review (Supplementary Materials).
Forest Landscape Models
A specific class of models known as forest landscape models (FLMs) dominated the literature of landscape disturbance interactions, representing 92% of studies from our systematic review (Table 3). FLMs were first developed in the late 20th century (Baker, 1989; Mladenoff and Baker, 1999; Gustafson, 2013) and have greatly diversified since then (He and Mladenoff, 1999; Schumacher et al., 2004; Lischke et al., 2006; Scheller and Mladenoff, 2007; Keane et al., 2011; Seidl et al., 2012; Wang et al., 2014, among others). Landscape modeling software (Fall and Fall, 2001; e.g., Bolte et al., 2007) further enabled the rapid development of customized FLMs. Despite this diversification, a common FLM structure has emerged integrating vegetation dynamics (succession, growth, mortality, senescence, etc.) with spatially-explicit disturbances affecting those dynamics. In some fashion, both vegetation and disturbance dynamics may be influenced from the “bottom up” by biophysical drivers such as soil characteristics and terrain, and from the “top down” by climatic drivers such as precipitation and temperature patterns, and in some cases atmospheric conditions (e.g., CO2 concentrations, flux of photosynthetically active radiation). Model development during the last two decades improved the architecture of the models, in terms of modular design and scaling methodologies, the relative balance between phenomenological patterns and simulated processes, and the diversity of disturbance agents that can affect system dynamics. Active development and widespread application of FLMs precipitated several reviews in the last decade (Seidl et al., 2011; Keane et al., 2013, 2015; Shifley et al., 2017).
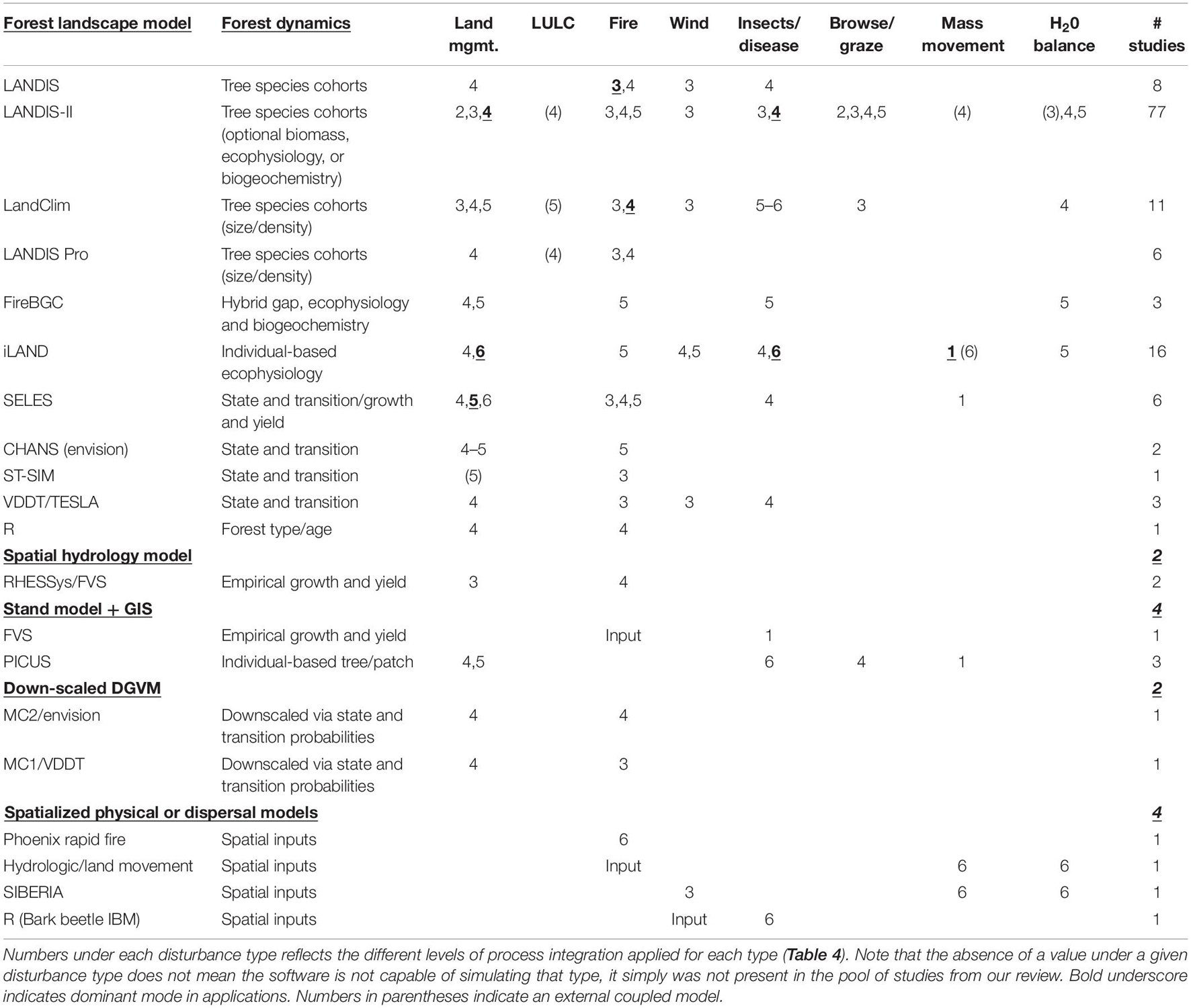
Table 3. Summary of the software applied to address disturbance interactions from the systematic review (Supplementary Materials).
Disturbance processes simulated within FLMs and other landscape models share common elements of initiation, spread or contagion, intensity, and effects, where opportunities for interaction can occur within each of these processes (Figure 3 and Table 3). Direct interactions between disturbances are often approximated through some surrogate for underlying process. For example, time since fire may serve as a proxy the process of fuel build-up. Indeed, Mladenoff et al. (1996) extended this approximation to enable one of the first formal disturbance interactions within an FLM, where a recent wind event increases the intensity of a subsequent fire event. Such logic becomes increasingly complicated, however, as one simulates multiple overlapping disturbances or other disturbance types, such as harvesting or insects (Figure 7). To what degree does the surrogate (in this case, either time since fire or time since disturbance) approximate the process (fuel accumulation) when multiple disturbances are at play? There is the potential for compounding error as additional direct disturbance interactions are added (Figure 7). This issue relates to any of the interaction types that can occur within a system (Figure 3). The complexity of state and transition models, for example, multiply with the number of disturbance processes that are included, because transition probabilities must be specified for every combination of possible disturbance sequences (James et al., 2011a; Daniel et al., 2017).
The solution to the increasing intractability of “direct” interactions via surrogates is to model the underlying process, such as fuel dynamics, as a state variable. Here, the interaction becomes indirectly mediated by the internal processes underlying the dynamics of the state variable, that in some cases, may be more elegantly modeled than attempting to keep track of overlapping surrogate variables (Figure 7). Such methods have other benefits, such as allowing for external drivers such as climate to further mediate the interaction (Figure 5), and to model the lagged effects appropriate for a given process and location (Burton et al., 2020). Simulation of multiple interacting disturbances is likely related to the decline of the state and transition model approach within FLMs in favor of tracking individual tree species that can respond individualistically to a wider range of disturbance types and their various combinations. Only 10 percent of the studies in our review applied the state and transition model approach (Table 3). A more recent innovation in FLMs is the simulation of tree stress via carbon balance (e.g., Keane et al., 2011) or even more explicitly in the form of non-structural carbohydrate reserves (Seidl et al., 2012; DeBruijn et al., 2014). Such innovations enable trees to respond to the accumulation of multiple stressors that include both disturbances and environmental drivers.
A related model design choice relates to trade-offs in “static” versus “dynamic” disturbance regimes. A static disturbance regime is one parameterized according to its statistical properties (e.g., rotation, event size distribution, intensity distribution, etc.; Figure 2). The focus of such applications is typically the response of the system to a given disturbance regime, without feedback to the regime itself. A fully dynamic disturbance regime is one where the statistical properties of the regime are an emergent property of the system dynamics (Perera et al., 2015). In practice, any of the individual components of a given disturbance type (i.e., Figure 3) can be simulated as either static or dynamic. Most contemporary FLMs simulate disturbance effects dynamically, where mortality is contingent on the relative susceptibility of forest types, tree species, age classes, or some combination of these variables. Many introduce stochastic elements that may be related to process (e.g., likelihood of insect damage or fire ignitions related to vegetation characteristics). Some models offer different options where the user can specify the relative balance of static vs. dynamic components (e.g., Sturtevant et al., 2009; Keane et al., 2011; DeJager et al., 2017).
We developed a classification scheme to address the degree of process integration (i.e., balance of static vs. dynamic components) within a specific disturbance agent within a given landscape disturbance application (Table 4), and then applied that scheme to the different disturbance agents simulated across the studies identified by our systematic review (Table 3). While the classification scheme was both logical and readily applied given proper documentation – we found this supporting documentation was sometimes lacking within individual studies. Many referenced earlier model applications, or original model publications, requiring more extensive review to fully understand the nature of the disturbance interactions simulated. Other applications required assumptions on our part where the documentation was insufficient, including vague descriptions of the specific model options applied, or references to model code requiring literacy of the underlying programming language. Nonetheless, we were able to document ranges of process integration across disturbance types and model software platforms (Table 3), including dominant modes of process integration, and instances where more detailed process integration was possible via the coupling of models (see Section “Coupled Models”). We found that while the trend in disturbance modeling is toward more explicit modeling of disturbance processes and their emergent effects on system dynamics and landscape structure (Gustafson, 2013; Keane et al., 2015), static disturbance regimes are still commonly applied. The relative balance of static vs. dynamic components in application was also unevenly distributed across different disturbance types and model approaches – including non-FLM applications (Table 3).
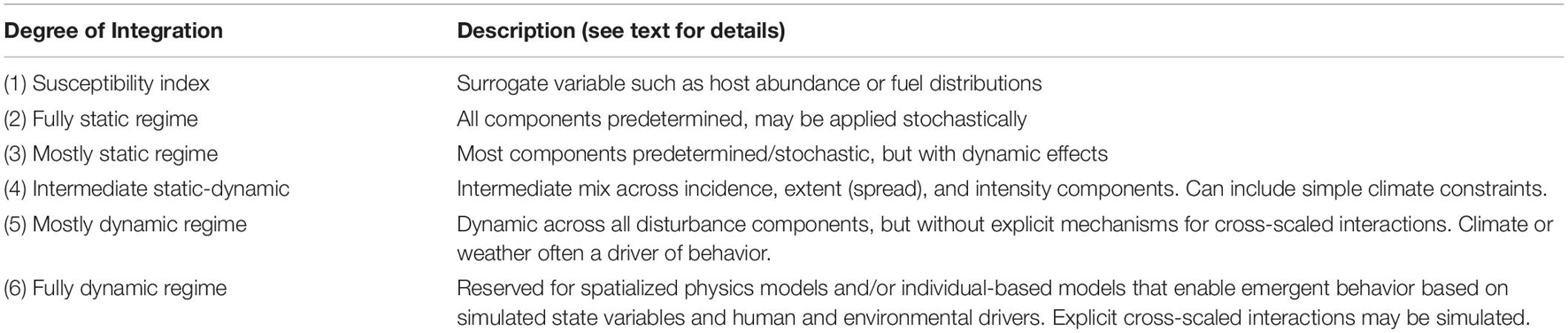
Table 4. An index reflecting the relative degree of process integration applied for a given disturbance type and more complete description, with index values corresponding to the numbers applied within disturbance type columns in Table 3.
Some contemporary FLMs are better described as model frameworks, where different trade-offs in process vs. surrogates, static vs. dynamic disturbance regimes, and the number and types of disturbance mechanisms simulated may be customized according to both the question addressed and the available supporting data. The most frequently applied model software from our review was LANDIS-II, an FLM model framework accounting for 59% of all studies (Table 3). A case study pair of applications of this model in north central Minnesota (United States) illustrates the framework approach (Lucash et al., 2017, 2018) (Figure 8). The authors applied a highly mechanistic succession option (Scheller et al., 2011a) where tree-species cohorts respond individualistically to different disturbance types and compete for above and belowground resources (light, water, nitrogen) via a set of life history traits and parameters. The first study focused on how the combination of climate change and forest management affected forest resilience following wind events (i.e., forest recovery rates), applying a factorial experiment that crossed climate scenarios with forest management scenarios (Lucash et al., 2017). The second study focused on the degree to which multiple disturbance regimes affected tree mortality rates (i.e., effects). Results of the latter supported antagonistic interactions, where total tree mortality caused by multiple disturbance regimes applied simultaneously was less than the sum of the mortality from each of the respective disturbance types applied independently. Closer examination of the simulation methods reveals a combination of process integration methods were applied to different disturbance types (Lucash et al., 2018). Wind disturbance was simulated as “mostly static” regimes with characteristic size, shape, and rotations of three classes of wind patterns (microburst, tornado, and derecho), each with species and age-specific impact patterns. Such static regimes are consistent with the lack of feedback between vegetation and wind event frequency or intensity, but cannot address spatial factors such as the effect of forest edges on tree susceptibility to windthrow (see Section “Disturbance Interaction Process and Terminology”). Fire regimes were simulated as mostly dynamic functions of the vegetation, fuel dynamics, and climate-related fire weather. Limitations of this approach in terms of emergent fire behavior are shared across virtually all FLMs: rules governing fire spread do not account for critical feedbacks between fire behavior and weather that fundamentally change those rules (such as long-distance dispersion of fire embers) (see Section “Cross-Scale Interactions”). Recent wind or insect disturbance could modify fuel attributes as direct interactions (Figure 7). The three different insect disturbance agents included intermediate blends of dynamic and static regime elements, where the extent of each disturbance was directly related to availability of vulnerable host, but the frequency and intensity of outbreaks was predefined. The exception was the patterns of mortality in oak (Quercus spp.) species by the two-lined chestnut borer (Agrilus bilineatus Weber) that occurred in instances where forest tent caterpillar (Malacosoma disstria Hübner) defoliation overlapped in time with drought events, reflecting a surrogate for tree stress defined by direct interactions with defoliation and climate, respectively (Figure 8).
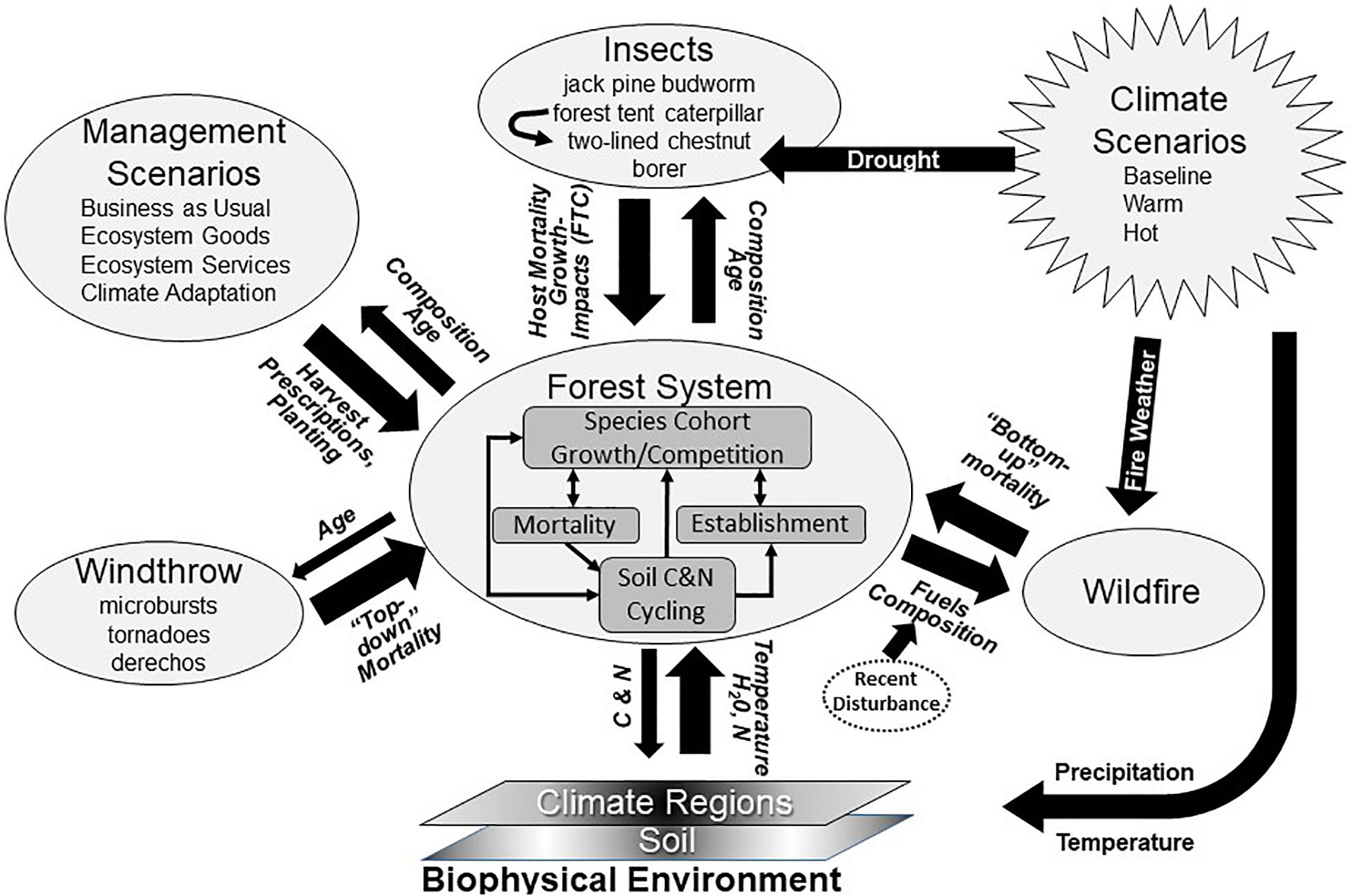
Figure 8. Example modeling study investigating multiple disturbance interactions, where disturbance interactions are primarily indirect via site-level state variables such as composition, age structure, and fuel loading. Climate drivers directly affect just small subsets of disturbances directly, but indirectly affect state variables that can lead to emergent system behavior as a function of two scenario factors (i.e., climate and management). (Adapted from Lucash et al., 2018).
Questions and Approaches Across Disturbance Types
We summarized the class of explicit disturbance interaction questions addressed across the different disturbance types (Table 5), using the conceptual advances summarized in Figures 3, 5. Frequencies of studies across questions and disturbance types were limited to those who either quantified the effect via their experimental design, or explicitly defined the nature of the simulated interaction within their methods. “Ecosystem response” was the one exception (see below). Linked disturbance interactions were specified according to which disturbance types were affected by another type. While looped disturbance types of the same disturbance (e.g., the effect of fire frequency on future fire likelihood) were not included in Table 5, instances where disturbance of a given type included multiple agents of that type (e.g., more than one insect agent) were included in the tabulation. Linked disturbances generally related to questions of disturbance resistance (Buma, 2015), but some studies aggregated responses across multiple disturbance types. In these cases, we tallied the study under “resistance (general)” (Table 5). Articles addressing resilience interaction questions were defined as studies that quantified the rates of return to a reference condition, shifts to alternative vegetation states, direct comparisons with HRV, or some combination of these responses. Climate-mediated disturbance questions refer to those studies where a given disturbance type was either modified to be consistent with future climate, or dynamically linked to climate. In the case of land management, climate-mediated disturbance involved some form of intentional climate adaption, such as assisted migration via planting. Cross-scaled disturbance frequencies refer to those studies that quantified cross-scaled interactions via their experimental design. Ecosystem response frequencies refer to those studies quantifying state variables other than those expressly linked to the disturbance interaction questions above (e.g., compositional trends). We note that most studies, whether self-identified as disturbance interaction studies or not, have an interest in capturing system dynamics under multiple interacting disturbance regimes. Frequencies of ecosystem responses in Table 5 therefore reflect the number of studies that included ecosystem responses regardless of whether the specific effect of a given disturbance type could be quantified in those response variables via the experimental design.
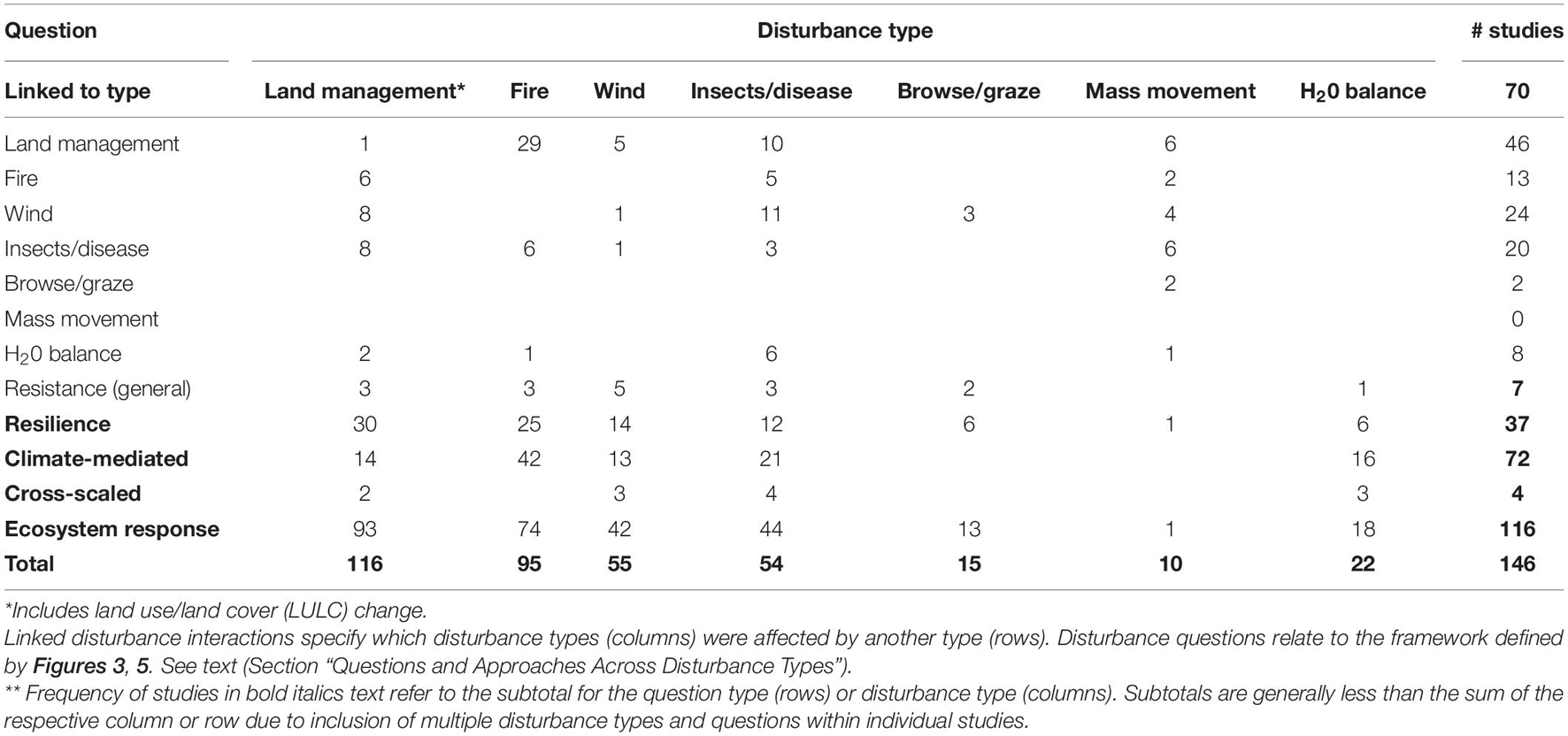
Table 5. Frequency of explicit disturbance interaction questions addressed by studies in the systematic review (Supplementary Materials).
The most commonly investigated linked disturbance was fire (Table 5), with most investigations focused on how land management actions such as fuel treatments or logging affected fire severity or extent. Far fewer studies investigated fire as linked to insect disturbance. Insect disturbance was investigated as linked to a broad range of disturbance types in half the studies for which it was included. Wind was less often investigated as linked to other disturbances, with the largest exceptions from studies in central Europe. Studies including the major disturbance types (management, fire, wind, and insects) investigated questions related to system resilience in roughly a quarter of their respective cases. For less-studied disturbance types, questions addressed varied widely, though the sample size was low. Drought was primarily investigated as a climate-mediated disturbance (16 of 22 studies) and related questions of forest resilience (6 of 22 studies). Six of 15 studies including mammalian browse investigated questions of ecosystem resilience, often related to the loss of forest cover. Almost all of the studies including mass movement disturbances focused on linkages to other disturbance types that either removed forest cover or otherwise destabilized soil in steep terrain.
Half of the studies from our review included some form of climate mediation of disturbance regimes (Table 5). Of those, 61 studies evaluated the degree to which forest management scenarios could mitigate the less desirable effects of climate change, such as elevated mortality (i.e., resistance) or transition to alternative system states (i.e., resilience). For example, Cadieux et al. (2020) investigated how projected climate change interacted with logging and fire disturbance to affect bird species habitat in the boreal forests of Alberta, Canada. In this case, the focus was on the vegetation response rather than on disturbance processes. They therefore applied mostly static fire and drought regimes parameterized differently for each climate scenario, based on empirical studies (Boulanger et al., 2014; Chen et al., 2016, respectively). The combined effects of climate change in combination with the associated elevated fire and drought mortality resulted in transitions from conifer-dominated forests to either deciduous forest or open habitats, with important implications for bird habitat. How this change to alternative forest states might interact with the fire regime itself could not be quantified using this approach. Keane et al. (2019) explicitly focused on emergent fire dynamics related to climate change, and how such behavior might be mitigated via either fire suppression or fuel treatment rates. In this study, they used simulations under historic climate and without human intervention to define the multivariate HRV for three contrasting landscapes in the northern Rocky Mountains (United States). This approach, combined with their process-based modeling framework (Keane et al., 2011), enabled them to draw inferences with regards to tipping points in system behavior that indicated loss of system resilience relative to the benchmark HRV, and the degree to which human action might affect system resilience in the face of climate change.
The relative balance of static to dynamic process in disturbance agents define the degree to which reciprocal interactions among those agents may be investigated, and therefore must be specified carefully with regard to the questions asked and addressed. For example, Chen et al. (2011) investigated the effects of larch caterpillar (Dendrolimus superans Butler) defoliation of different intensities on fire regime. However, key assumptions governing the fire regime, such as whether fire size and rotation was an input or an output of the model, were not provided, making it impossible to evaluate their results regarding insect effects on response variables such as fire frequency. Sturtevant et al. (2012) investigated reciprocal interactions between spruce budworm and fire disturbance and demonstrated that budworm defoliation disturbance reduced live ladder fuels, and therefore decreased fire extent over long time scales (centuries), despite increasing fire spread and intensity at shorter (decadal) scales. Specifically, the authors examined how an intermediate static-dynamic insect disturbance regime affected a mostly dynamic fire regime. While they could directly address the question of budworm effects on fire regimes, they could not fully address the reciprocal question (i.e., how does fire influence the insect disturbance regime?). Temperli et al. (2015) coupled a spatially-explicit bark beetle model with the LandClim FLM (Schumacher et al., 2004) to investigate interactions among spruce bark beetles (Dendroctonus rufipennis Kirby), fire, wind, and climate. In this case, wind disturbance was simulated as a mostly static regime, fire was intermediate (i.e., ignitions and size distributions were static inputs, but spread and effects were dynamic), drought stress was linked to climate inputs, and beetles responded dynamically to vegetation, drought, host abundance, and windthrown host resources. Analogous to Sturtevant et al. (2012), the authors could address the effects of wind and fire on bark beetle disturbance behavior, but not the reverse. They found that climate change-driven drought events would promote beetle outbreaks, but the combination of the two mortality agents progressively reduced long-term susceptibility to bark beetle outbreaks. As a final example, Seidl and Rammer (2017) simulated emergent dynamics of bark beetles responding to wind disturbance and climate change in the Austrian Alps, concluding that climate change amplified the outbreaks initiated by wind events, in large part by shifting the optimal development rates of the beetles to higher elevations where its spruce host was more concentrated. Since the authors applied disturbance agents that each responded dynamically to both climate drivers and ecosystem state variables, they could make inferences about reciprocal interactions among disturbance agents, as well as the mediating role of climate.
Some of the constraints on the types of disturbance interaction questions that may be addressed via landscape modeling are imposed by the design constraints of the selected model software (e.g., Table 3). Nonetheless, model designs are ultimately constrained by supporting knowledge and data. In the insect examples above, there is greater consensus on the landscape factors controlling bark beetle dynamics than there are for defoliator dynamics (Kneeshaw et al., 2021). Indeed, bark beetles were included in all four of the disturbance interaction studies that explicitly addressed cross-scaled interactions (Table 5). Of those, one approximated non-linear responses of bark beetles to host abundance via percolation theory (Seidl et al., 2016a). The remaining three explicitly modeled emergence and spread of bark beetle agents as they interacted with environmental drivers such as temperature, food resources including both pulses provided by windthrow and host concentrations impacted in part by drought stress (Temperli et al., 2015; Dobor et al., 2020; Honkaniemi et al., 2020) (see Figure 6). While a few model designs may have allowed for cross-scale interactions in other studies (Table 3), they were not explicitly quantified so their importance cannot be evaluated.
Modeling Anthropogenic Forest Disturbance
Forest management was frequently investigated as a linked factor affecting other disturbance types, however, the reverse (effects of natural disturbance on land management) was far less common (Table 5). Eleven of the studies linking management to other disturbances included explicit salvage logging and/or sanitary logging, representing post-disturbance harvest of felled trees and preventative treatments to reduce insect impacts, respectively. Given the quantity of empirical studies investigating salvage logging, it is somewhat surprising this process is less investigated via landscape modeling.
FLMs most often model forest management practices as partially dynamic functions of landscape conditions (Table 3). Harvest locations are often selected via ranking algorithms such as oldest harvested first or biased toward species or forest types of interest (Gustafson et al., 2000). Harvest intensity and effects can likewise be defined to reflect a wide range of management prescriptions. However, the rates (i.e., extent) of any given treatment is generally predefined within scenarios. By contrast, forest planning models represent a different class of models that approach the question of how much to harvest dynamically based on the temporal changes and sometimes spatial distribution in the timber resource (Pukkala, 2013). Disturbance interaction questions from a forest optimization point of view often revolve around accounting for natural disturbances that can add uncertainty to the optimization process (Savage et al., 2011). There is a well-developed literature on spatial forest planning models that focus on the drivers of timber management such as growth and yield, access, road-building, and especially cost-benefit trade-offs that FLMs generally lack (Baskent and Keles, 2005; De Pellegrin Llorente et al., 2017). Nonetheless, such approaches have struggled with the stochastic and contiguous nature of natural disturbances, and therefore either simplistically account for such processes as aspatial rates of disturbance or ignore them altogether (De Pellegrin Llorente et al., 2017). Occasionally these divergent approaches have been blended to account for both human and ecological drivers of disturbance interactions. For example, forest optimization models have been used to define harvest rates for scenarios implemented in an FLM (e.g., Daniel et al., 2017). Raulier et al. (2014) used the SELES modeling platform to apply an iterative harvest optimization process – one that included spatially contagious processes such as road building and salvage logging where existing roads enabled rapid access. They used the model to simulate reciprocal interactions between fire and timber harvest to better quantify risk to timber supply under climate change in boreal Canada.
More recent methods for integration of socioeconomic drivers that contribute to both reciprocal interactions with forest landscape systems include demand and allocation models and also agent-based models (An, 2012; Egli et al., 2019). Developers recently incorporated agent-based logging and salvage logging within the iLAND model (Rammer and Seidl, 2015). Such development enabled simulation experiments examining reciprocal interactions among wind, European bark beetle, and forestry practices in the face of climate warming (e.g., Dobor et al., 2020) as illustrated in Figure 6, and account for the studies linking land management to wind and insect disturbance in Table 5. Agent-based forest management approaches are now being implemented in other FLM platforms (Spies et al., 2017; Sotnik et al., 2021). Land-use intensification and other anthropogenic disturbance activities have profound effects on landscape legacies and disturbance regime shifts (Watson et al., 2014). An emerging hotspot of research activity therefore lies in the investigation of reciprocal interactions between human activities and ecosystem processes—falling broadly under the scope of coupled human and natural systems (Wimberly et al., 2015). Anthropogenic and natural disturbance interactions might be modeled using integrated modeling platforms (Verburg and Overmars, 2009), where the combined effects of disturbance events and climate change can be explored via experimental designs that include either contrasting or a range of climatic and land-use intensification scenarios (Becknell et al., 2015). While six studies from our review used outputs from human land use change models to drive land cover change within FLMs (e.g., Duveneck and Thompson, 2019), none coupled land used change dynamically with forest harvest or natural disturbances to investigate their reciprocal interactions. One study applied an FLM to estimate forest productivity under alternative climate change scenarios and coupled those outputs with that of yield models from other economic sectors (agriculture and livestock) to drive an economic land allocation model to simulate land conversions under the twin drivers of climate change and human incentives (Briner et al., 2012). This example represents the closest approximation to the complete system portrayed in Figure 1 of any included in our review.
Coupled Models
As illustrated by the coupling of FLMs with land cover change models in the previous section, some authors of articles in our review extended the capabilities of a given model by coupling them with one or more additional models. Different models may be linked via a “loose coupling,” where the output of one model is used as input for another (i.e., the most common form, see Section “Modeling Anthropogenic Forest Disturbance”), or “tight coupling”, where the dynamics of one model interact with the dynamics of another (Sturtevant et al., 2007). Loose couplings of models are widely used as a scaling technique, for example by deriving the input parameters of a coarse-scaled model from a finer-scaled process model (Urban et al., 1999; Xu et al., 2012; Boulanger et al., 2018; Huang et al., 2018) or down-scaling vegetation or disturbance behavior from coarse-scaled models such as DGVMs (Halofsky et al., 2014; Turner et al., 2015) (Table 3). FLM outputs are also commonly used as inputs for wildlife habitat or viability models that may be considered a dimension of ecosystem response to disturbance interactions (e.g., Longru et al., 2010; Scheller et al., 2011b; Nitschke et al., 2020).
Apart from human factors such land cover change or forest optimization, use of coupled models to address disturbance interactions centered on better representation of hydrologic and mass movement processes currently lacking within FLMs (Table 3). Research by Saksa et al. (2020a, b) coupled an ecohydrology model with a stand growth and yield model (for vegetation dynamics) and a fire behavior model to evaluate the integrated effects of fuel treatment patterns on fire risk and hydrologic outputs from small watersheds of the Sierra Nevada Mountains in the western United States. A planar surface model was used to define the flood disturbance regime for an FLM application to a Mississippi floodplain system in the central United States (DeJager et al., 2019). The combination of forest cover, disturbances, and impervious surface outputs from a coupled land cover change and FLM model system were used to project flood risk via a simple hydrologic model (Thompson et al., 2016). Scheidl et al. (2020) used the projections of an FLM to drive a physically-based spatial hydrologic model coupled with a slope-stability model to evaluate the integrated effects of climate change, forest management, and natural disturbance processes on shallow landslide frequency within the eastern Alps of Austria.
Hybrid Empirical-Simulation Modeling
Hybrid empirical-modeling studies leverage the synthetic knowledge within process models to relate data representing measurable system components to processes underlying disturbance interactions that are either difficult or impossible to measure directly. For example, McGuire and Youberg (2019) empirically investigated the effects of repeat burns on both soil water infiltration rates and the occurrence of debris flow disturbances in dry montane forests of southeastern Arizona, United States. They then coupled remote sensing and terrain inputs with a high-resolution hydrologic model to demonstrate that differences in soil infiltration between once and twice burned soils were sufficient enough to affect threshold rain intensities necessary to generate debris flows. Similarly, Hancock et al. (2012) measured windthrown trees and consequent erosion patterns following a cyclone impacting northern Australia. They then used this data within the geomorphic landform model SIBERIA (Willgoose et al., 1991) to investigate ecohydrological controls (including windthrow patterns) on long-term erosion and catchment evolution. Økland et al. (2016) investigated a critical transition in bark beetle outbreak dynamics where beetle populations move from trees injured or killed by wind events to attack healthy trees (i.e., windfall-driven to patch-driven dynamics). They compared alternative models representing different hypothesized drivers within an individual-based model framework and found that the model driven by beetle aggregation was the most consistent with their empirical data on the spatial progression of an outbreak in Tatra National Park in Slovakia. Analogous hybrid modeling by Seidl and Rammer (2017) now serves as the foundation for the individual-based model of beetle disturbance contained within the iLAND FLM.
Model Transparency, Uncertainty, and Confidence
A primary challenge to conducting a systematic and consistent review of landscape models featuring disturbance interactions was the lack of standards by which to classify the implementation of a given disturbance agent in terms of its relative degree of process integration - or for that matter, documentation standards for FLMs more generally. There is an increasing call for standardization of methods in analogous classes of models, including agent-based model designs (Grimm et al., 2010) and species distribution models (Zurell et al., 2020). Table 6 represents a partial example of how documentation standards relevant to disturbance interactions can convey the nature of the interactions being simulated and investigated. Minimum standards should include the disturbance interaction type simulated (Figure 3), the response variable quantifying its effect, and the dynamic components of a given disturbance agent that dictate which interaction types are justifiably investigated. This documentation would facilitate a rapid classification – like that in Table 4 – so that the nature of the simulated interaction is transparent to the reader. Other essential documentation should include a clearly articulated study design and basic information such as the spatial and temporal grain and extent of simulated processes (Table 6). The latter is coming increasingly important to clarify as the temporal and spatial resolution (grain) has become more specific to individual processes as model designs become more refined (Table 6). Additional relevant documentation includes the level at which disturbance effects are applied (patch/cell, age class, species, cohort, tree, etc.), the spatial implementation of the disturbance agents (spread, dispersion, etc.), and how the various disturbance agents are interspersed in time. All such documentation should be specific to the model application at hand, rather than the potential capabilities of a given model software, as this was sometimes conflated in the articles we reviewed. Outputs from spatial models are complex enough without confusing artifacts of modeling assumptions with emergent behavior based on underlying process.
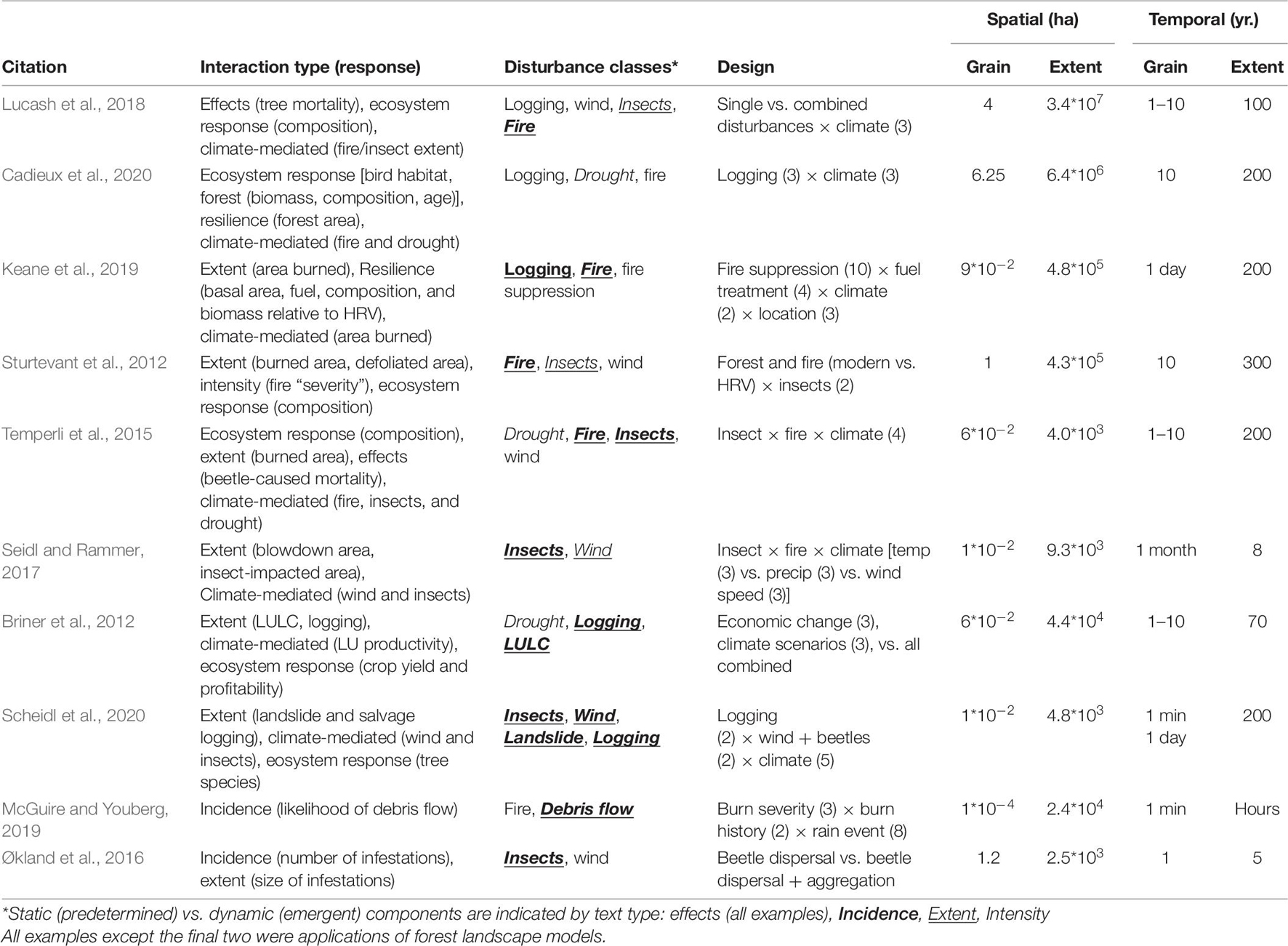
Table 6. Representative studies reviewed in the “Modeling Approaches” Section, including disturbance interaction type(s), response variable(s), disturbance classes, characterization of disturbance components*, study design, and scale.
We do not assert that complex models are better than simple models. Rather, that one should account for the processes underlying the question being investigated to the extent that data and knowledge can support them. The contemporary argument for design of models based on first principles is that such models should make more robust predictions under the increasingly novel conditions of changing climate, atmospheric composition, and disturbance agents (Cuddington et al., 2013; Gustafson, 2013). We note also that many landscape modeling studies did not explicitly investigate disturbance interactions per se, but rather included multiple disturbance agents to simply account for all the relevant structuring processes within their forest system. Regardless, it is important to acknowledge and account for the cumulative effects of model error propagation as the number of interacting components increase and from one scale to another (Rastetter et al., 1992). To be more explicit about the relative importance of multiple processes and errors, Cressie et al. (2009) promoted the use of hierarchical statistical modeling that allows the quantification of the relative importance of uncertainty stemming from the data model (i.e., data error), the process model (i.e., model specification error), and the parameter model (i.e., parameter estimation error). There are, however, multiple additional sources of uncertainties—ranging from the data, models, processes, knowledge of the processes, and climate scenarios—that will affect the precision and accuracy of model projections.
To quantify the relative importance of these various types of uncertainty, one could compare the outcomes of several models (Cheaib et al., 2012). A recent comparison of four “state of the art” FLMs demonstrated wide divergence in model outcomes, in large part due to design choices affecting vegetation response to disturbance (Petter et al., 2020). This result suggests a certain degree of humility when reporting the results of landscape models – particularly where interactions by multiple disturbance agents may compound error associated with cumulative vegetative responses.
Scheller (2018) suggested that parameterization and “model confidence” are twin challenges facing contemporary landscape modeling studies. The increased burden of input parameter specification that generally comes with replacement of simple statistical relationships with more mechanistic detail may potentially undermine the perceived robustness of process-based models if those parameters are either poorly understood or quantified (McKenzie et al., 2019). Relevant knowledge acquisition necessary for parameter estimation will require multidisciplinary research crossing different domains in scale, integrating experimental research, monitoring of earth systems, advanced statistical analyses, and next generation models assembled from these insights to determine process-based responses to future climatic conditions. Knowledge gaps may further require more novel hybrid modeling frameworks (e.g., Talluto et al., 2016) that integrate process-based and statistical models to include both bottom-up processes and drivers and top-down drivers and account explicitly for feedbacks across scales (Figure 1). Such integrative models require knowledge about ecological responses based on targeted experiments in the field and carefully designed data analysis of existent data (Poisot et al., 2016).
Model confidence refers to the degree to which independent readers trust the utility of the model results – in this regard independent validation data remains a fundamental issue underlying confidence in FLMs (Shifley et al., 2017; Scheller, 2018). Validation issues may be particularly acute in the area of disturbance interactions given system sensitivity to the timing and order of disturbance mechanisms and the individualistic responses of the vegetation. While there were examples within our review that evaluated their results using empirical observations (Henne et al., 2013; Karam et al., 2013; Schwörer et al., 2014; Thom et al., 2018), these examples were rare. Validation of model components in combination with targeted uncertainty and sensitivity analyses may also improve model confidence (Cressie et al., 2009; Xu et al., 2012; Scheller, 2018; McKenzie et al., 2019). More specifically, model uncertainty refers to the degree to which model inputs are known as well as their associated measurement error, whereas sensitivity analyses focuses on the degree to which model inputs have influence on the model outputs (McKenzie et al., 2019). Guidance on effective uncertainty and sensitivity analyses can be found across disciplines for simulation modeling more generally (e.g., Saltelli et al., 2006; Wagener and Pianosi, 2019).
Synthesis: New Insights
We found a broad spectrum of questions related to disturbance interactions in forested landscapes have been addressed by an equally diverse range of modeling approaches (Tables 3, 6). Notably, it is the more intractable of these questions that are also the most challenging to quantify empirically, and these require the support of simulation models to investigate. Integration of recent conceptual advances (i.e., linked disturbances, compound disturbances, cross-scaled interactions, and cascading effects) often require more detailed processes to simulate. Among the most important are non-deterministic tree-species competitive interactions and disturbance responses via life history traits, explicit modeling of stand structural characteristics, physiological traits of tree species that enable dynamic responses to climate and biophysical variables, and various integrations of these factors (Keane et al., 2011; Scheller et al., 2011a; Seidl et al., 2012; DeBruijn et al., 2014; Wang et al., 2014). Depending on the questions asked, modeling disturbances require ecological detail necessary to effectively capture their incidence, extent, intensity, and/or effects (see examples within Section “Questions and Approaches Across Disturbance Types”). We are approaching a point where some of the most process-based disturbance models, such as physical fire models, individual-based insect models, and agent-based human disturbances are possible either within FLMs or coupled model architectures (Rammer and Seidl, 2015; Bentley and Penman, 2017; Seidl and Rammer, 2017). Nonetheless, trade-offs between model elegance and complexity remain (McKenzie and Perera, 2015). For example, the parameter demands of highly complex models may exceed the data available to parameterize them (Scheller, 2018) (see also Section “Model Transparency, Uncertainty, and Confidence”). We also observe that the most mechanistic examples reviewed here were comparatively limited in the extent at which they were applied (e.g., thousands versus millions of ha, years versus centuries), illustrating practical trade-offs between ecological detail and scope remain despite advances in computing power (Table 6).
The study of disturbance interactions through landscape modeling reflects the asymmetry in systems and disturbance agents of the empirical studies (see Section “Empirical Approaches”). Studies were concentrated primarily and temperate, boreal, and alpine regions of North America and Europe (Table 2) and were almost absent from the southern hemisphere. Perhaps it is no coincidence that the prevalent disturbance regimes of subtropical and tropical regions (e.g., mass movement, hurricane/cyclones, mammalian grazers; Burton et al., 2020) were less commonly investigated in the context of disturbance interactions (Table 5). We found that human disturbance – by far the most commonly simulated disturbance type – was primarily simulated as a driver rather than an interactive component of the simulations. Cross-scale interactions remain an important concern that are particularly challenging to model and quantify. Each of these deficiencies suggests fruitful areas of future research. Finally, we echo the plea made by previous authors to improve model transparency – perhaps via documentation standards such as that developed for other classes of models.
Future Directions
Our review demonstrates substantial progress in the study of disturbance interactions necessary to model their effects on forested landscapes. We now have far greater clarity in the conceptual underpinnings of disturbance interactions, a wealth of empirical studies to draw from, and more advanced model frameworks capable of simulating multiple disturbance types across a range of complexities, relative to what was available at the turn of the millennium when such questions were gaining traction. Questions asked by ecologists are becoming increasingly more ambitious, in part due to the expanding data resources and tools available, and in part due to urgently expanding needs for reliable forecasts of natural and managed ecosystems under increasing anthropogenic stressors. Multiple disturbances and drivers as well as their interactions increasingly insert uncertainty and surprise as forested systems adjust to novel conditions (Urban et al., 2016), complicating our ability to project their behavior. It is therefore paramount to unravel the cumulative effects of multiple disturbances and factors (e.g., edaphic, environmental, and climatic) on vegetation change, with the understanding that as the number of interactions increases, so does the uncertainty associated with ecosystem responses. Such complexity underscores the need to include both conceptual advances and empirical approaches in a review that is ultimately about effective modeling of forest landscape disturbance interactions. As so eloquently stated by Seidl (2017), “to model or not to model, that is no longer the question…”. Conceptual understanding, empirical study, and simulation modeling should continually reinforce one another if we are to unravel the complexities of disturbance interactions in time and space.
The “art” of modeling landscape disturbance interactions calls for balanced approaches and scales: while macroscale, empirical models are used to approximate processes, the integration of micro- and mesoscales mechanisms into process-based models is the way to adapt and plan for the novel conditions of the “Anthropocene” (Bodner et al., 2020). The simulation studies cited in this review demonstrate a broad range of modeling approaches that reflect the diversity of ways investigators balance trade-offs in scale, scope, and ecological complexity when modeling disturbance interactions (e.g., Tables 3, 6). Given the importance of transparency to model confidence (Scheller, 2018), it is critical that modelers be explicit about basic assumptions and methods. We note that many landscape simulation studies in the literature cite the potential capabilities of a given modeling platform while glossing over the specifics of the options they applied. We further caution against loading simulation studies with multiple disturbance types simply because a given model platform allows it. Careful consideration of how the disturbances interact within the context of the model design and the questions being addressed are recommended to avoid compounding model artifacts that can produce misleading results. Furthermore, computing technology may enable modeling of processes that lack the empirical studies necessary to parameterize them—hence the need for a multi-dimensional research agenda.
Turner (2010) emphasized the importance of explicitly accounting for human-induced changes and societal land-use intensification demands, as well as societal responses to disturbance regime shifts and rapid land cover changes. The effects of landscape change resulting from disturbance regime shifts can be investigated by comparing alternative scenarios of plausible system states (Titeux et al., 2016; Urban et al., 2016). By contrast, the more human-focused modeling studies tend to focus on a single ecological response variable (Egli et al., 2019). Blending the state-of-the-art in coupled human natural systems modeling and FLMs remains a current frontier, leveraging the advances in each respective discipline (e.g., Briner et al., 2012).
To sum, modeling disturbance interactions across scales epitomizes the broader challenges plaguing ecological research more generally. The dynamic nature of earth systems makes a strong argument for process-based modeling grounded in a mechanistic understanding of the dynamics of individual system components (Cuddington et al., 2013; Gustafson, 2013). Yet the compounding uncertainty of the interactions between system components—including disturbance processes—that can be both non-linear and scale-dependent lie at the heart of the intractability of complex human-ecological systems (Turner, 2010). Clarity in concepts (knowledge), an empirical foundation (data and analysis), and model designs (synthesis and software) will ultimately enhance understanding of complex systems, but the choice of which processes to model explicitly and which processes to aggregate remains a fundamental challenge of our time.
Author Contributions
BS and M-JF jointly developed the theme and content of the manuscript. BS led the literature review and the writing of the manuscript. M-JF provided literature review support, helped shape the flow of ideas, and edited several versions of the manuscript through its current form. Both authors contributed to the article and approved the submitted version.
Funding
This work was supported by NSERC Discovery Grant #5134 and a Canada Research Chair to M-JF.
Conflict of Interest
The authors declare that the research was conducted in the absence of any commercial or financial relationships that could be construed as a potential conflict of interest.
Publisher’s Note
All claims expressed in this article are solely those of the authors and do not necessarily represent those of their affiliated organizations, or those of the publisher, the editors and the reviewers. Any product that may be evaluated in this article, or claim that may be made by its manufacturer, is not guaranteed or endorsed by the publisher.
Acknowledgments
We thank Kathleen Quigley, Gordon Reese, Eric Gustafson, Megan Davis, and three reviewers for helpful critiques of earlier versions of the manuscript.
Supplementary Material
The Supplementary Material for this article can be found online at: https://www.frontiersin.org/articles/10.3389/fevo.2021.653647/full#supplementary-material
References
Agne, M. C., Woolley, T., and Fitzgerald, S. (2016). Fire severity and cumulative disturbance effects in the post-mountain pine beetle lodgepole pine forests of the Pole Creek Fire. For. Ecol. Manage. 366, 73–86. doi: 10.1016/j.foreco.2016.02.004
Allen, C. D. (2007). Interactions across spatial scales among forest dieback, fire, and erosion in northern New Mexico landscapes. Ecosystems 10, 797–808. doi: 10.1007/s10021-007-9057-4
Allen, C. R., Angeler, D. G., Garmestani, A. S., Gunderson, L. H., and Holling, C. S. (2014). Panarchy: theory and application. Ecosystems 17, 578–589. doi: 10.1007/s10021-013-9744-2
Allen, T. F. H., and Hoekstra, T. W. (2015). Toward a Unified Ecology, 2nd Edn. New York NY: Columbia University Press.
An, L. (2012). Modeling human decisions in coupled human and natural systems: review of agent-based models. Ecol. Modell. 229, 25–36. doi: 10.1016/j.ecolmodel.2011.07.010
Anderegg, W. R. L., Hicke, J. A., Fisher, R. A., Allen, C. D., Aukema, J., Bentz, B., et al. (2015). Tree mortality from drought, insects, and their interactions in a changing climate. New Phytol. 208, 674–683. doi: 10.1111/nph.13477
Baker, W. L. (1989). A review of models of landscape change. Landsc. Ecol. 2, 111–133. doi: 10.1007/BF00137155
Baskent, E. Z., and Keles, S. (2005). Spatial forest planning: a review. Ecol. Modell. 188, 145–173. doi: 10.1016/j.ecolmodel.2005.01.059
Bebi, P., Kulakowski, D., and Veblen, T. T. (2003). Interactions between fire and spruce beetles in a subalpine Rocky Mountain forest landscape. Ecology 84, 362–371.
Becknell, J. M., Desai, A. R., Dietze, M. C., Schultz, C. A., Starr, G., Duffy, P. A., et al. (2015). Assessing interactions among changing climate, management, and disturbance in forests: a macrosystems approach. Bioscience 65, 263–274. doi: 10.1093/biosci/biu234
Bender, E. A., Case, T. J., and Gilpin, M. E. (1984). Perturbation experiments in community ecology: theory and practice. Ecology 65, 1–13. doi: 10.2307/1939452
Bentley, P. D., and Penman, T. D. (2017). Is there an inherent conflict in managing fire for people and conservation? Int. J. Wildl. Fire 26, 455. doi: 10.1071/WF16150
Bentz, B. (2019). “Advances in understanding and managing insect pests of forest trees,” in Achieving Sustainable Management of Boreal and Temperate Forests, ed. J. A. Stanturf (Cambridge: Burleigh Dodds Science Publishing), 1–70. doi: 10.19103/AS.2019.0057.19
Bodner, K., Fortin, M., and Molnár, P. K. (2020). Making predictive modelling ART: accurate, reliable, and transparent. Ecosphere 11:e03160. doi: 10.1002/ecs2.3160
Bolte, J. P., Hulse, D. W., Gregory, S. V., and Smith, C. (2007). Modeling biocomplexity – actors, landscapes and alternative futures. Environ. Model. Softw. 22, 570–579. doi: 10.1016/j.envsoft.2005.12.033
Boulanger, Y., Gauthier, S., and Burton, P. J. (2014). A refinement of models projecting future Canadian fire regimes using homogeneous fire regime zones. Can. J. For. Res. 44, 365–376. doi: 10.1139/cjfr-2013-0372
Boulanger, Y., Taylor, A. R., Price, D. T., Cyr, D., and Sainte-Marie, G. (2018). Stand-level drivers most important in determining boreal forest response to climate change. J. Ecol. 106, 977–990. doi: 10.1111/1365-2745.12892
Brice, M., Vissault, S., Vieira, W., Gravel, D., Legendre, P., and Fortin, M. (2020). Moderate disturbances accelerate forest transition dynamics under climate change in the temperate–boreal ecotone of eastern North America. Glob. Chang. Biol. 26, 4418–4435. doi: 10.1111/gcb.15143
Briner, S., Elkin, C., Huber, R., and Grêt-Regamey, A. (2012). Assessing the impacts of economic and climate changes on land-use in mountain regions: a spatial dynamic modeling approach. Agric. Ecosyst. Environ. 149, 50–63. doi: 10.1016/j.agee.2011.12.011
Brodrick, P. G., Davies, A. B., and Asner, G. P. (2019). Uncovering ecological patterns with convolutional neural networks. Trends Ecol. Evol. 34, 734–745. doi: 10.1016/j.tree.2019.03.006
Buma, B. (2015). Disturbance interactions: characterization, prediction, and the potential for cascading effects. Ecosphere 6, 1–15. doi: 10.1890/ES15-00058.1
Buma, B., and Wessman, C. A. (2011). Disturbance interactions can impact resilience mechanisms of forests. Ecosphere 2:art64. doi: 10.1890/ES11-00038.1
Burnham, K. P., Anderson, D. R., and Huyvaert, K. P. (2011). AIC model selection and multimodel inference in behavioral ecology: some background, observations, and comparisons. Behav. Ecol. Sociobiol. 65, 23–35. doi: 10.1007/s00265-010-1029-6
Burton, P. J., Jentsch, A., and Walker, L. R. (2020). The ecology of disturbance interactions. Bioscience 70, 854–870. doi: 10.1093/biosci/biaa088
Cadieux, P., Boulanger, Y., Cyr, D., Taylor, A. R., Price, D. T., Sólymos, P., et al. (2020). Projected effects of climate change on boreal bird community accentuated by anthropogenic disturbances in western boreal forest, Canada. Divers. Distrib. 26, 668–682. doi: 10.1111/ddi.13057
Candau, J. N., Fleming, R. A., and Wang, X. (2018). Ecoregional patterns of spruce budworm—Wildfire interactions in central Canada’s forests. Forests 9:137. doi: 10.3390/f9030137
Canelles, Q., Aquilué, N., James, P. M. A., Lawler, J., and Brotons, L. (2021). Global review on interactions between insect pests and other forest disturbances. Landsc. Ecol. 36, 945–972. doi: 10.1007/s10980-021-01209-7
Cannon, J. B., O’Brien, J. J., Loudermilk, E. L., Dickinson, M. B., and Peterson, C. J. (2014). The influence of experimental wind disturbance on forest fuels and fire characteristics. For. Ecol. Manage. 330, 294–303. doi: 10.1016/j.foreco.2014.07.021
Carabella, C., Miccadei, E., Paglia, G., and Sciarra, N. (2019). Post-wildfire landslide hazard assessment: the case of the 2017 Montagna Del Morrone Fire (Central Apennines, Italy). Geosciences 9:175. doi: 10.3390/geosciences9040175
Carleton, T. J., and Maclellan, P. (1994). Woody vegetation responses to fire versus clear-cutting logging: a comparative survey in the central Canadian boreal forest. Écoscience 1, 141–152. doi: 10.1080/11956860.1994.11682238
Cheaib, A., Badeau, V., Boe, J., Chuine, I., Delire, C., Dufrêne, E., et al. (2012). Climate change impacts on tree ranges: model intercomparison facilitates understanding and quantification of uncertainty. Ecol. Lett. 15, 533–544. doi: 10.1111/j.1461-0248.2012.01764.x
Chen, H., Hu, Y., Chang, Y., Bu, R., Li, Y., and Liu, M. (2011). Simulating impact of larch caterpillar (Dendrolimus superans) on fire regime and forest landscape in Da Hinggan Mountains, Northeast China. Chinese Geogr. Sci. 21, 575–586. doi: 10.1007/s11769-011-0494-9
Chen, H. Y. H., Luo, Y., Reich, P. B., Searle, E. B., and Biswas, S. R. (2016). Climate change-associated trends in net biomass change are age dependent in western boreal forests of Canada. Ecol. Lett. 19, 1150–1158. doi: 10.1111/ele.12653
Clarke, S. R., Riggins, J. J., and Stephen, F. M. (2016). Forest management and southern pine beetle outbreaks: a historical perspective. For. Sci. 62, 166–180. doi: 10.5849/forsci.15-071
Cobb, R., and Metz, M. (2017). Tree diseases as a cause and consequence of interacting forest disturbances. Forests 8:147. doi: 10.3390/f8050147
Coop, J. D., Parks, S. A., Stevens-Rumann, C. S., Crausbay, S. D., Higuera, P. E., Hurteau, M. D., et al. (2020). Wildfire-driven forest conversion in Western North American landscapes. Bioscience 70, 659–673. doi: 10.1093/biosci/biaa061
Cressie, N., Calder, C. A., Clark, J. S., Ver Hoef, J. M., and Wikle, C. K. (2009). Accounting for uncertainty in ecological analysis: the strengths and limitations of hierarchical statistical modeling. Ecol. Appl. 19, 553–570. doi: 10.1890/07-0744.1
Cuddington, K., Fortin, M.-J., Gerber, L. R., Hastings, A., Liebhold, A., O’Connor, M., et al. (2013). Process-based models are required to manage ecological systems in a changing world. Ecosphere 4:art20. doi: 10.1890/ES12-00178.1
Cumming, S. G. (2001). Forest type and wildfire in the Alberta boreal mixedwood: what do fires burn? Ecol. Appl. 11, 97–110.
Daniel, C. J., Ter-Mikaelian, M. T., Wotton, B. M., Rayfield, B., and Fortin, M.-J. (2017). Incorporating uncertainty into forest management planning: timber harvest, wildfire and climate change in the boreal forest. For. Ecol. Manage. 400, 542–554. doi: 10.1016/j.foreco.2017.06.039
Davis, K. T., Higuera, P. E., and Sala, A. (2018). Anticipating fire-mediated impacts of climate change using a demographic framework. Funct. Ecol. 32, 1729–1745. doi: 10.1111/1365-2435.13132
Dayrell, R. L. C., Arruda, A. J., Pierce, S., Negreiros, D., Meyer, P. B., Lambers, H., et al. (2018). Ontogenetic shifts in plant ecological strategies. Funct. Ecol. 32, 2730–2741. doi: 10.1111/1365-2435.13221
De Pellegrin Llorente, I., Hoganson, H. M., Carson, M. T., and Windmuller-Campione, M. (2017). Recognizing spatial considerations in forest management planning. Curr. For. Reports 3, 308–316. doi: 10.1007/s40725-017-0068-x
DeBruijn, A., Gustafson, E. J., Sturtevant, B. R., Foster, J. R., Miranda, B. R., Lichti, N. I., et al. (2014). Toward more robust projections of forest landscape dynamics under novel environmental conditions: embedding PnET within LANDIS-II. Ecol. Modell. 287, 44–57. doi: 10.1016/j.ecolmodel.2014.05.004
DeJager, N. R., Drohan, P. J., Miranda, B. M., Sturtevant, B. R., Stout, S. L., Royo, A. A., et al. (2017). Simulating ungulate herbivory across forest landscapes: a browsing extension for LANDIS-II. Ecol. Modell. 350, 11–29. doi: 10.1016/j.ecolmodel.2017.01.014
DeJager, N. R., Van Appledorn, M., Fox, T. J., Rohweder, J. J., Guyon, L. J., Meier, A. R., et al. (2019). Spatially explicit modelling of floodplain forest succession: interactions among flood inundation, forest successional processes, and other disturbances in the Upper Mississippi River floodplain. USA. Ecol. Modell. 405, 15–32. doi: 10.1016/j.ecolmodel.2019.05.002
DeRose, R. J., and Long, J. N. (2009). Wildfire and spruce beetle outbreak: simulation of interacting disturbances in the central Rocky Mountains. Écoscience 16, 28–38. doi: 10.2980/16-1-3160
DeRose, R. J., and Long, J. N. (2012). Drought-driven disturbance history characterizes a southern Rocky Mountain subalpine forest. Can. J. For. Res. 42, 1649–1660. doi: 10.1139/x2012-102
Dobor, L., Hlásny, T., Rammer, W., Zimová, S., Barka, I., and Seidl, R. (2020). Is salvage logging effectively dampening bark beetle outbreaks and preserving forest carbon stocks? J. Appl. Ecol. 57, 67–76. doi: 10.1111/1365-2664.13518
Duveneck, M. J., and Thompson, J. R. (2019). Social and biophysical determinants of future forest conditions in New England: effects of a modern land-use regime. Glob. Environ. Chang. 55, 115–129. doi: 10.1016/j.gloenvcha.2019.01.009
Egli, L., Weise, H., Radchuk, V., Seppelt, R., and Grimm, V. (2019). Exploring resilience with agent-based models: State of the art, knowledge gaps and recommendations for coping with multidimensionality. Ecol. Complex. 40:100718. doi: 10.1016/j.ecocom.2018.06.008
Fall, A., and Fall, J. (2001). A domain-specific language for models of landscape dynamics. Ecol. Modell. 141, 1–18. doi: 10.1016/S0304-3800(01)00334-9
Fleming, R. A., Candau, J. N., and McAlpine, R. S. (2002). Landscape-scale analysis of interactions between insect defoliation and forest fire in central Canada. Clim. Change 55, 251–272. doi: 10.1023/A:1020299422491
Foley, J. A. (2005). Global consequences of land use. Science 309, 570–574. doi: 10.1126/science.1111772
Foster, D. R., Knight, D. H., and Franklin, J. F. (1998). Landscape patterns and legacies resulting from large, infrequent forest disturbances. Ecosystems 1, 497–510. doi: 10.1007/s100219900046
Fraterrigo, J. M., and Rusak, J. A. (2008). Disturbance-driven changes in the variability of ecological patterns and processes. Ecol. Lett. 11, 756–770. doi: 10.1111/j.1461-0248.2008.01191.x
Frelich, L. E., and Reich, P. B. (1999). Neighborhood effects, disturbance severity, and community stability in forests. Ecosystems 2, 151–166. doi: 10.1007/s100219900066
Gimenez, O., Buckland, S. T., Morgan, B. J. T., Bez, N., Bertrand, S., Choquet, R., et al. (2014). Statistical ecology comes of age. Biol. Lett. 10:20140698. doi: 10.1098/rsbl.2014.0698
Grimm, V., Berger, U., DeAngelis, D. L., Polhill, J. G., Giske, J., and Railsback, S. F. (2010). The ODD protocol: a review and first update. Ecol. Modell. 221, 2760–2768. doi: 10.1016/j.ecolmodel.2010.08.019
Gustafson, E. J. (2013). When relationships estimated in the past cannot be used to predict the future: using mechanistic models to predict landscape ecological dynamics in a changing world. Landsc. Ecol. 28, 1429–1437. doi: 10.1007/s10980-013-9927-4
Gustafson, E. J., Shifley, S. R., Mladenoff, D. J., Nimerfro, K. K., and He, H. S. (2000). Spatial simulation of forest succession and timber harvesting using LANDIS. Can. J. For. Res. 30, 32–43. doi: 10.1139/x99-188
Halofsky, J. S., Halofsky, J. E., Burcsu, T., and Hemstrom, M. A. (2014). Dry forest resilience varies under simulated climate-management scenarios in a central Oregon, USA landscape. Ecol. Appl. 24, 1908–1925. doi: 10.1890/13-1653.1
Hanberry, B. B., Bragg, D. C., and Alexander, H. D. (2020). Open forest ecosystems: an excluded state. For. Ecol. Manage. 472:118256. doi: 10.1016/j.foreco.2020.118256
Hancock, G. R., Evans, K. G., McDonnell, J., and Hopp, L. (2012). Ecohydrological controls on soil erosion and landscape evolution. Ecohydrology 5, 478–490. doi: 10.1002/eco.241
Hart, S. J., Schoennagel, T., Veblen, T. T., and Chapman, T. B. (2015). Area burned in the western United States is unaffected by recent mountain pine beetle outbreaks. Proc. Natl. Acad. Sci.U.S.A. 112, 4375–4380. doi: 10.1073/pnas.1424037112
He, H. S., and Mladenoff, D. J. (1999). Spatially explicit and stochastic simulation of forest−landscape fire disturbance and succession. Ecology 80, 81–99.
Henne, P. D., Elkin, C., Colombaroli, D., Samartin, S., Bugmann, H., Heiri, O., et al. (2013). Impacts of changing climate and land use on vegetation dynamics in a Mediterranean ecosystem: insights from paleoecology and dynamic modeling. Landsc. Ecol. 28, 819–833. doi: 10.1007/s10980-012-9782-8
Hennigar, C. R., MacLean, D. A., Quiring, D. T., and Kershaw, J. A. J. (2008). Differences in spruce budworm defoliation among balsam fir and white, red, and black spruce. For. Sci. 54, 158–166.
Hicke, J. A., Johnson, M. C., Hayes, J. L., and Preisler, H. K. (2012). Effects of bark beetle-caused tree mortality on wildfire. For. Ecol. Manage. 271, 81–90. doi: 10.1016/j.foreco.2012.02.005
Holmes, S., and Huber, W. (2018). Modern Statistics for Modern Biology. Cambridge: Cambridge University Press.
Honkaniemi, J., Rammer, W., and Seidl, R. (2020). Norway spruce at the trailing edge: the effect of landscape configuration and composition on climate resilience. Landsc. Ecol. 35, 591–606. doi: 10.1007/s10980-019-00964-y
Howe, E., and Baker, W. L. (2003). Landscape heterogeneity and disturbance interactions in a subalpine watershed in northern Colorado. USA. Ann. Assoc. Am. Geogr. 93, 797–813. doi: 10.1111/j.1467-8306.2003.09304002.x
Huang, C., He, H. S., Liang, Y., Wu, Z., Hawbaker, T. J., Gong, P., et al. (2018). Long-term effects of fire and harvest on carbon stocks of boreal forests in northeastern China. Ann. For. Sci. 75:42. doi: 10.1007/s13595-018-0722-x
Itter, M. S., D’Orangeville, L., Dawson, A., Kneeshaw, D., Duchesne, L., and Finley, A. O. (2019). Boreal tree growth exhibits decadal-scale ecological memory to drought and insect defoliation, but no negative response to their interaction. J. Ecol. 107, 1288–1301. doi: 10.1111/1365-2745.13087
Jain, T. B., Fried, J. S., and Loreno, S. M. (2020). Simulating the effectiveness of improvement cuts and commercial thinning to enhance fire resistance in West Coast dry mixed conifer forests. For. Sci. 66, 157–177. doi: 10.1093/forsci/fxz071
James, P. M. A., Sturtevant, B. R., Townsend, P., Wolter, P., and Fortin, M.-J. (2011b). Two-dimensional wavelet analysis of spruce budworm host basal area in the Border Lakes landscape. Ecol. Appl. 21, 2197–2209. doi: 10.1890/09-1876.1
James, P. M. A., Robert, L.-E., Wotton, B. M., Martell, D. L., and Fleming, R. A. (2017). Lagged cumulative spruce budworm defoliation affects the risk of fire ignition in Ontario. Can. Ecol. Appl. 27, 532–544. doi: 10.1002/eap.1463
James, P. M. A., Fortin, M.-J., Sturtevant, B. R., Fall, A., and Kneeshaw, D. (2011a). Modelling spatial interactions among fire, spruce budworm, and logging in the boreal forest. Ecosystems 14, 60–75. doi: 10.1007/s10021-010-9395-5
Jansen, S., Konrad, H., and Geburek, T. (2017). The extent of historic translocation of Norway spruce forest reproductive material in Europe. Ann. For. Sci. 74, 1–17. doi: 10.1007/s13595-017-0644-z
Jentsch, A., and White, P. (2019). A theory of pulse dynamics and disturbance in ecology. Ecology 100:e02734. doi: 10.1002/ecy.2734
Johnstone, J. F., Allen, C. D., Franklin, J. F., Frelich, L. E., Harvey, B. J., Higuera, P. E., et al. (2016). Changing disturbance regimes, ecological memory, and forest resilience. Front. Ecol. Environ. 14:369–378. doi: 10.1002/fee.1311
Kane, J. M., Varner, J. M., Metz, M. R., and van Mantgem, P. J. (2017). Characterizing interactions between fire and other disturbances and their impacts on tree mortality in western U.S. Forests. For. Ecol. Manage. 405, 188–199. doi: 10.1016/j.foreco.2017.09.037
Karam, S. L., Weisberg, P. J., Scheller, R. M., Johnson, D. W., and Miller, W. W. (2013). Development and evaluation of a nutrient cycling extension for the LANDIS-II landscape simulation model. Ecol. Modell. 250, 45–57. doi: 10.1016/j.ecolmodel.2012.10.016
Kausrud, K., Økland, B., Skarpaas, O., Grégoire, J.-C., Erbilgin, N., and Stenseth, N. C. (2012). Population dynamics in changing environments: the case of an eruptive forest pest species. Biol. Rev. 87, 34–51. doi: 10.1111/j.1469-185X.2011.00183.x
Keane, R. E., Cary, G. J., Flannigan, M. D., Parsons, R. A., Davies, I. D., King, K. J., et al. (2013). Exploring the role of fire, succession, climate, and weather on landscape dynamics using comparative modeling. Ecol. Modell. 266, 172–186. doi: 10.1016/j.ecolmodel.2013.06.020
Keane, R. E., Gray, K., Davis, B., Holsinger, L. M., and Loehman, R. (2019). Evaluating ecological resilience across wildfire suppression levels under climate and fuel treatment scenarios using landscape simulation modelling. Int. J. Wildl. Fire 28:533. doi: 10.1071/WF19015
Keane, R. E., Loehman, R. A., and Holsinger, L. M. (2011). The FireBGCv2 Landscape Fire And Succession Model: A Research Simulation Platform For Exploring Fire And Vegetation Dynamics General Technical Report RMRS-GTR-255. Fort Collins: U.S. Department of Agriculture, Forest Service, Rocky Mountain Research Station, doi: 10.2737/RMRS-GTR-255
Keane, R. E., McKenzie, D., Falk, D. A., Smithwick, E. A. H., Miller, C., and Kellogg, L.-K. B. (2015). Representing climate, disturbance, and vegetation interactions in landscape models. Ecol. Modell. 309–310, 33–47. doi: 10.1016/j.ecolmodel.2015.04.009
Keeley, J. E. (2009). Fire intensity, fire severity and burn severity: a brief review and suggested usage. Int. J. Wildl. Fire 18:116. doi: 10.1071/WF07049
Kim, D., Millington, A. C., and Lafon, C. W. (2020). Disturbance after disturbance: combined effects of two successive hurricanes on forest community structure. Ann. Am. Assoc. Geogr. 110, 571–585. doi: 10.1080/24694452.2019.1654844
Kleinman, J. S., Goode, J. D., Fries, A. C., and Hart, J. L. (2019). Ecological consequences of compound disturbances in forest ecosystems: a systematic review. Ecosphere 10:e02962. doi: 10.1002/ecs2.2962
Kneeshaw, D. D., Sturtevant, B. R., DeGrandpé, L., Doblas-Miranda, E., James, P. M. A., Tardif, D., et al. (2021). The vision of managing for pest-resistant landscapes: realistic or utopic? Curr. For. Rep. 7, 97–113. doi: 10.1007/s40725-021-00140-z
Kolb, T. E., Fettig, C. J., Ayres, M. P., Bentz, B. J., Hicke, J. A., Mathiasen, R., et al. (2016). Observed and anticipated impacts of drought on forest insects and diseases in the United States. For. Ecol. Manage. 380, 321–334. doi: 10.1016/j.foreco.2016.04.051
Kulakowski, D., Jarvis, D., Veblen, T. T., and Smith, J. (2012). Stand-replacing fires reduce susceptibility of lodgepole pine to mountain pine beetle outbreaks in Colorado. J. Biogeogr. 39, 2052–2060. doi: 10.1111/j.1365-2699.2012.02748.x
Kulakowski, D., and Veblen, T. T. (2007). Effect of prior disturbances on the extent and severity of wildfire in Colorado subalpine forests. Ecology 88, 759–769. doi: 10.1890/06-0124
Landres, P. B., Morgan, P., and Swanson, F. J. (1999). Overview of the use of natural variability concepts in managing ecological systems. Ecol. Appl. 9, 1179–1188.
Leverkus, A. B., Lindenmayer, D. B., Thorn, S., and Gustafsson, L. (2018). Salvage logging in the world’s forests: interactions between natural disturbance and logging need recognition. Glob. Ecol. Biogeogr. 27, 1140–1154. doi: 10.1111/geb.12772
Liebhold, A. M., Brockerhoff, E. G., Kalisz, S., Nuñez, M. A., Wardle, D. A., and Wingfield, M. J. (2017). Biological invasions in forest ecosystems. Biol. Invasions 19, 3437–3458. doi: 10.1007/s10530-017-1458-5
Lindenmayer, D., Thorn, S., and Banks, S. (2017). Please do not disturb ecosystems further. Nat. Ecol. Evol. 1:0031. doi: 10.1038/s41559-016-0031
Lischke, H., Zimmermann, N. E., Bolliger, J., Rickebusch, S., and Löffler, T. J. (2006). TreeMig: a forest-landscape model for simulating spatio-temporal patterns from stand to landscape scale. Ecol. Modell. 199, 409–420. doi: 10.1016/j.ecolmodel.2005.11.046
Liu, Z., Peng, C., Work, T., Candau, J.-N., DesRochers, A., and Kneeshaw, D. (2018). Application of machine-learning methods in forest ecology: recent progress and future challenges. Environ. Rev. 26, 339–350. doi: 10.1139/er-2018-0034
Longru, J., He, H. S., Yufei, Z., Rencang, B., and Keping, S. (2010). Assessing the effects of management alternatives on habitat suitability in a forested landscape of Northeastern China. Environ. Manage. 45, 1191–1200. doi: 10.1007/s00267-010-9473-7
Lucash, M. S., Scheller, R. M., Gustafson, E. J., and Sturtevant, B. R. (2017). Spatial resilience of forested landscapes under climate change and management. Landsc. Ecol. 32, 953–969. doi: 10.1007/s10980-017-0501-3
Lucash, M. S., Scheller, R. M., Sturtevant, B. R., Gustafson, E. J., Kretchun, A. M., and Foster, J. R. (2018). More than the sum of its parts: how disturbance interactions shape forest dynamics under climate change. Ecosphere 9:e02293. doi: 10.1002/ecs2.2293
Máliš, F., Kopecký, M., Petřík, P., Vladovič, J., Merganič, J., and Vida, T. (2016). Life stage, not climate change, explains observed tree range shifts. Glob. Chang. Biol. 22, 1904–1914. doi: 10.1111/gcb.13210
McGuire, L. A., and Youberg, A. M. (2019). Impacts of successive wildfire on soil hydraulic properties: implications for debris flow hazards and system resilience. Earth Surf. Process. Landforms 44, 2236–2250. doi: 10.1002/esp.4632
McKenzie, D., and Perera, A. H. (2015). “Modeling wildfire regimes in forest landscapes: abstracting a complex reality,” in Simulation Modeling of Forest Landscape Disturbances, eds A. H. Perera, B. R. Sturtevant, and L. J. Buse (Cham: Springer International Publishing), 73–92. doi: 10.1007/978-3-319-19809-5_4
McKenzie, P. F., Duveneck, M. J., Morreale, L. L., and Thompson, J. R. (2019). Local and global parameter sensitivity within an ecophysiologically based forest landscape model. Environ. Model. Softw. 117, 1–13. doi: 10.1016/j.envsoft.2019.03.002
Meigs, G. W., Campbell, J. L., Zald, H. S. J., Bailey, J. D., Shaw, D. C., and Kennedy, R. E. (2015). Does wildfire likelihood increase following insect outbreaks in conifer forests? Ecosphere 6:art118. doi: 10.1890/ES15-00037.1
Messier, C., Bauhus, J., Doyon, F., Maure, F., Sousa-Silva, R., Nolet, P., et al. (2019). The functional complex network approach to foster forest resilience to global changes. For. Ecosyst. 6:21. doi: 10.1186/s40663-019-0166-2
Messier, C., Puettmann, K., Filotas, E., and Coates, D. (2016). Dealing with non-linearity and uncertainty in forest management. Curr. For. Reports 2, 150–161. doi: 10.1007/s40725-016-0036-x
Mezei, P., Jakuš, R., Pennerstorfer, J., Havašová, M., Škvarenina, J., Ferenčík, J., et al. (2017). Storms, temperature maxima and the Eurasian spruce bark beetle Ips typographus—An infernal trio in Norway spruce forests of the Central European High Tatra Mountains. Agric. For. Meteorol. 242, 85–95. doi: 10.1016/j.agrformet.2017.04.004
Millar, C. I., and Delany, D. L. (2019). Interaction between mountain pine beetle-caused tree mortality and fire behavior in subalpine whitebark pine forests, eastern Sierra Nevada. CA; Retrospective observations. For. Ecol. Manage. 447, 195–202. doi: 10.1016/j.foreco.2019.05.052
Millar, C. I., and Stephenson, N. L. (2015). Temperate forest health in an era of emerging megadisturbance. Science 349, 823–826. doi: 10.1126/science.aaa9933
Mladenoff, D., Host, G., Boeder, J., and Crow, T. (1996). “A spatial model of forest landscape disturbance, succession and management,” in GIS and Environmental Modeling: Progress and Research Issues, eds M. F. Goodchild, L. T. Steyaert, B. O. Parks, C. Johnston, D. Maidment, M. Crane, et al. (Ft. Collins, CO: GIS World Books), 175–179.
Mladenoff, D. J., and Baker, W. L. eds (1999). Spatial Modeling of Forest Landscape Change: Approaches And Applications. Cambridge: Cambridge University Press.
Negrón, J. F., and Fettig, C. J. (2014). Mountain pine beetle, a major disturbance agent in us western coniferous forests: a synthesis of the state of knowledge. For. Sci. 60, 409–413. doi: 10.5849/forsci.13-169
Newman, E. A. (2019). Disturbance ecology in the Anthropocene. Front. Ecol. Evol. 7:147. doi: 10.3389/fevo.2019.00147
Nitschke, C. R., Trouvé, R., Lumsden, L. F., Bennett, L. T., Fedrigo, M., Robinson, A. P., et al. (2020). Spatial and temporal dynamics of habitat availability and stability for a critically endangered arboreal marsupial: implications for conservation planning in a fire-prone landscape. Landsc. Ecol. 35, 1553–1570. doi: 10.1007/s10980-020-01036-2
Noble, I. R., and Slatyer, R. O. (1980). The use of vital attributes to predict successional changes in plant communities subject to recurrent disturbances. Vegetatio 43, 5–21. doi: 10.1007/BF00121013
Ohmart, C. P. (1989). Why are there so few tree-killing bark beetles associated with Angiosperms? Oikos 54:242. doi: 10.2307/3565273
Økland, B., Nikolov, C., Krokene, P., and Vakula, J. (2016). Transition from windfall- to patch-driven outbreak dynamics of the spruce bark beetle Ips typographus. For. Ecol. Manage. 363, 63–73. doi: 10.1016/j.foreco.2015.12.007
Olden, J. D., Lawler, J. J., and Poff, N. L. (2008). Machine learning methods without tears: a primer for ecologists. Q. Rev. Biol. 83, 171–193. doi: 10.1086/587826
O’Neill, R. V., Hunsaker, C. T., Timmins, S. P., Jackson, B. L., Jones, K. B., Riitters, K. H., et al. (1996). Scale problems in reporting landscape pattern at the regional scale. Landsc. Ecol. 11, 169–180. doi: 10.1007/BF02447515
Parrott, L., and Meyer, W. S. (2012). Future landscapes: managing within complexity. Front. Ecol. Environ. 10:382–389. doi: 10.1890/110082
Perera, A. H., Sturtevant, B. R., and Buse, L. J. eds (2015). Simulation Modeling of Forest Landscape Disturbances. Cham: Springer International Publishing, doi: 10.1007/978-3-319-19809-5
Peters, D. P. C., Bestelmeyer, B. T., and Turner, M. G. (2007). Cross–scale interactions and changing pattern–process relationships: consequences for system dynamics. Ecosystems 10, 790–796. doi: 10.1007/s10021-007-9055-6
Peters, D. P. C., Lugo, A. E., Chapin, F. S., Pickett, S. T. A., Duniway, M., Rocha, A. V., et al. (2011). Cross-system comparisons elucidate disturbance complexities and generalities. Ecosphere 2:art81. doi: 10.1890/ES11-00115.1
Peters, D. P. C., Pielke, R. A., Bestelmeyer, B. T., Allen, C. D., Munson-McGee, S., and Havstad, K. M. (2004). Cross-scale interactions, nonlinearities, and forecasting catastrophic events. Proc. Natl. Acad. Sci.U.S.A. 101, 15130–15135. doi: 10.1073/pnas.0403822101
Peterson, G. D. (2002). Contagious disturbance, ecological memory, and the emergence of landscape pattern. Ecosystems 5, 329–338. doi: 10.1007/s10021-001-0077-1
Petter, G., Mairota, P., Albrich, K., Bebi, P., Brůna, J., Bugmann, H., et al. (2020). How robust are future projections of forest landscape dynamics? Insights from a systematic comparison of four forest landscape models. Environ. Model. Softw. 134:104844. doi: 10.1016/j.envsoft.2020.104844
Pickett, S. T. A., and White, P. S. eds (1985). The Ecology of Natural Disturbance and Patch Dynamics. Cambridge MA: Academic Press.
Poisot, T., Gravel, D., Leroux, S., Wood, S. A., Fortin, M.-J., Baiser, B., et al. (2016). Synthetic datasets and community tools for the rapid testing of ecological hypotheses. Ecography (Cop.). 39, 402–408. doi: 10.1111/ecog.01941
Pukkala, T. ed (2013). Multi-Objective Forest Planning, Vol. 6. Cham: Springer Science & Business Media.
Quentin, A. G., O’Grady, A. P., Beadle, C. L., Mohammed, C., and Pinkard, E. A. (2012). Interactive effects of water supply and defoliation on photosynthesis, plant water status and growth of Eucalyptus globulus Labill. Tree Physiol. 32, 958–967. doi: 10.1093/treephys/tps066
Quine, C. P., Coutts, M. P., Gardiner, B. A., and Pyatt, D. G. (1995). Forests and wind: management to minimise damage. For. Comm. Bull. 114, 1–27.
Raffa, K. F., Aukema, B. H., Bentz, B. J., Carroll, A. L., Hicke, J. A., Turner, M. G., et al. (2008). Cross-scale drivers of natural disturbances prone to anthropogenic amplification: the dynamics of bark beetle eruptions. Bioscience 58, 501–517. doi: 10.1641/b580607
Rammer, W., and Seidl, R. (2015). Coupling human and natural systems: simulating adaptive management agents in dynamically changing forest landscapes. Glob. Environ. Chang. 35, 475–485. doi: 10.1016/j.gloenvcha.2015.10.003
Ramsfield, T. D., Bentz, B. J., Faccoli, M., Jactel, H., and Brockerhoff, E. G. (2016). Forest health in a changing world: effects of globalization and climate change on forest insect and pathogen impacts. Forestry 89, 245–252. doi: 10.1093/forestry/cpw018
Rastetter, E. B., King, A. W., Cosby, B. J., Hornberger, G. M., O’Neill, R. V., and Hobbie, J. E. (1992). Aggregating fine-scale ecological knowledge to model coarser-scale attributes of ecosystems. Ecol. Appl. 2, 55–70. doi: 10.2307/1941889
Ratajczak, Z., Carpenter, S. R., Ives, A. R., Kucharik, C. J., Ramiadantsoa, T., Stegner, M. A., et al. (2018). Abrupt change in ecological systems: inference and diagnosis. Trends Ecol. Evol. 33, 513–526. doi: 10.1016/j.tree.2018.04.013
Raulier, F., Dhital, N., Racine, P., Tittler, R., and Fall, A. (2014). Increasing resilience of timber supply: how a variable buffer stock of timber can efficiently reduce exposure to shortfalls caused by wildfires. For. Policy Econ. 46, 47–55. doi: 10.1016/j.forpol.2014.06.007
Robert, L., Sturtevant, B. R., Kneeshaw, D., James, P. M. A., Fortin, M., Wolter, P. T., et al. (2020). Forest landscape structure influences the cyclic-eruptive spatial dynamics of forest tent caterpillar outbreaks. Ecosphere 11:e03096. doi: 10.1002/ecs2.3096
Robert, L.-E., Sturtevant, B. R., Cooke, B. J., James, P. M. A., Fortin, M.-J., Townsend, P. A., et al. (2018). Landscape host abundance and configuration regulate periodic outbreak behavior in spruce budworm Choristoneura fumiferana. Ecography (Cop.). 41, 1556–1571. doi: 10.1111/ecog.03553
Royo, A. A., Collins, R., Adams, M. B., Kirschbaum, C., and Carson, W. P. (2010). Pervasive interactions between ungulate browsers and disturbance regimes promote temperate forest herbaceous diversity. Ecology 91, 93–105. doi: 10.1890/08-1680.1
Rykiel, E. J. (1985). Towards a definition of ecological disturbance. Austral Ecol. 10, 361–365. doi: 10.1111/j.1442-9993.1985.tb00897.x
Saksa, P. C., Bales, R. C., Tague, C. L., Battles, J. J., Tobin, B. W., and Conklin, M. H. (2020a). Fuels treatment and wildfire effects on runoff from Sierra Nevada mixed-conifer forests. Ecohydrology 13:e2151. doi: 10.1002/eco.2151
Saksa, P. C., Conklin, M. H., Tague, C. L., and Bales, R. C. (2020b). Hydrologic response of Sierra Nevada mixed-conifer headwater catchments to vegetation treatments and wildfire in a warming climate. Front. For. Glob. Chang. 3:539429. doi: 10.3389/ffgc.2020.539429
Saltelli, A., Ratto, M., Tarantola, S., and Campolongo, F. (2006). Sensitivity analysis practices: strategies for model-based inference. Reliab. Eng. Syst. Saf. 91, 1109–1125. doi: 10.1016/j.ress.2005.11.014
Savage, D. W., Martell, D. L., and Wotton, B. M. (2011). Forest management strategies for dealing with fire-related uncertainty when managing two forest seral stages. Can. J. For. Res. 41, 309–320. doi: 10.1139/X10-212
Scheidl, C., Heiser, M., Kamper, S., Thaler, T., Klebinder, K., Nagl, F., et al. (2020). The influence of climate change and canopy disturbances on landslide susceptibility in headwater catchments. Sci. Total Environ. 742:140588. doi: 10.1016/j.scitotenv.2020.140588
Scheller, R. M. (2018). The challenges of forest modeling given climate change. Landsc. Ecol. 33, 1481–1488. doi: 10.1007/s10980-018-0689-x
Scheller, R. M., Hua, D., Bolstad, P. V., Birdsey, R. A., and Mladenoff, D. J. (2011a). The effects of forest harvest intensity in combination with wind disturbance on carbon dynamics in Lake States Mesic Forests. Ecol. Modell. 222, 144–153. doi: 10.1016/j.ecolmodel.2010.09.009
Scheller, R. M., and Mladenoff, D. J. (2007). An ecological classification of forest landscape simulation models: tools and strategies for understanding broad-scale forested ecosystems. Landsc. Ecol. 22, 491–505. doi: 10.1007/s10980-006-9048-4
Scheller, R. M., Spencer, W. D., Rustigian-Romsos, H., Syphard, A. D., Ward, B. C., and Strittholt, J. R. (2011b). Using stochastic simulation to evaluate competing risks of wildfires and fuels management on an isolated forest carnivore. Landsc. Ecol. 26, 1491–1504. doi: 10.1007/s10980-011-9663-6
Schumacher, S., Bugmann, H., and Mladenoff, D. J. (2004). Improving the formulation of tree growth and succession in a spatially explicit landscape model. Ecol. Modell. 180, 175–194. doi: 10.1016/j.ecolmodel.2003.12.055
Schwörer, C., Henne, P. D., and Tinner, W. (2014). A model-data comparison of Holocene timberline changes in the Swiss Alps reveals past and future drivers of mountain forest dynamics. Glob. Chang. Biol. 20, 1512–1526. doi: 10.1111/gcb.12456
Seidl, R. (2017). To model or not to model, that is no longer the question for ecologists. Ecosystems 20, 222–228. doi: 10.1007/s10021-016-0068-x
Seidl, R., Müller, J., Hothorn, T., Bässler, C., Heurich, M., and Kautz, M. (2016b). Small beetle, large-scale drivers: how regional and landscape factors affect outbreaks of the European spruce bark beetle. J. Appl. Ecol. 53, 530–540. doi: 10.1111/1365-2664.12540
Seidl, R., Fernandes, P. M., Fonseca, T. F., Gillet, F., Jönsson, A. M., Merganičová, K., et al. (2011). Modelling natural disturbances in forest ecosystems: a review. Ecol. Modell. 222, 903–924. doi: 10.1016/j.ecolmodel.2010.09.040
Seidl, R., Donato, D. C., Raffa, K. F., and Turner, M. G. (2016a). Spatial variability in tree regeneration after wildfire delays and dampens future bark beetle outbreaks. Proc. Natl. Acad. Sci. U.S.A. 113, 13075–13080. doi: 10.1073/pnas.1615263113
Seidl, R., and Rammer, W. (2017). Climate change amplifies the interactions between wind and bark beetle disturbances in forest landscapes. Landsc. Ecol. 32, 1485–1498. doi: 10.1007/s10980-016-0396-4
Seidl, R., Rammer, W., Scheller, R. M., and Spies, T. A. (2012). An individual-based process model to simulate landscape-scale forest ecosystem dynamics. Ecol. Modell. 231, 87–100. doi: 10.1016/j.ecolmodel.2012.02.015
Seidl, R., Thom, D., Kautz, M., Martin-Benito, D., Peltoniemi, M., Vacchiano, G., et al. (2017). Forest disturbances under climate change. Nat. Clim. Chang. 7, 395–402. doi: 10.1038/nclimate3303
Senf, C., and Seidl, R. (2018). Natural disturbances are spatially diverse but temporally synchronized across temperate forest landscapes in Europe. Glob. Chang. Biol. 24, 1201–1211. doi: 10.1111/gcb.13897
Shifley, S. R., He, H. S., Lischke, H., Wang, W. J., Jin, W., Gustafson, E. J., et al. (2017). The past and future of modeling forest dynamics: from growth and yield curves to forest landscape models. Landsc. Ecol. 32, 1307–1325. doi: 10.1007/s10980-017-0540-9
Simard, M., Powell, E. N., Griffin, J. M., Raffa, K. F., and Turner, M. G. (2008). Annotated Bibliography For Forest Managers On Fire-Bark Beetle Interactions. Prineville, OR: USFS, Western Wildlands Environmental Threats Assessment Center.
Simard, M., Romme, W. H., Griffin, J. M., and Turner, M. G. (2011). Do mountain pine beetle outbreaks change the probability of active crown fire in lodgepole pine forests? Ecol. Monogr. 81, 3–24. doi: 10.1890/10-1176.1
Soranno, P. A., Cheruvelil, K. S., Bissell, E. G., Bremigan, M. T., Downing, J. A., Fergus, C. E., et al. (2014). Cross-scale interactions: quantifying multi-scaled cause−effect relationships in macrosystems. Front. Ecol. Environ. 12:65–73. doi: 10.1890/120366
Sotnik, G., Cassell, B. A., Duveneck, M. J., and Scheller, R. M. (2021). A new agent-based model provides insight into deep uncertainty faced in simulated forest management. Landsc. Ecol. doi: 10.1007/s10980-021-01324-5
Sparks, A. M., Talhelm, A. F., Feltrin, R. P., Smith, A. M. S., Johnson, D. M., Kolden, C. A., et al. (2018). An experimental assessment of the impact of drought and fire on western larch injury, mortality and recovery. Int. J. Wildl. Fire 27:490. doi: 10.1071/WF18044
Spies, T. A., White, E., Ager, A., Kline, J. D., Bolte, J. P., Platt, E. K., et al. (2017). Using an agent-based model to examine forest management outcomes in a fire-prone landscape in Oregon. USA. Ecol. Soc. 22:art25. doi: 10.5751/ES-08841-220125
Stevens-Rumann, C., Morgan, P., and Hoffman, C. (2015). Bark beetles and wildfires: how does forest recovery change with repeated disturbances in mixed conifer forests? Ecosphere 6:art100. doi: 10.1890/ES14-00443.1
Sturtevant, B. R., Fall, A., Kneeshaw, D. D., Simon, N. P. P., Papaik, M. J., Berninger, K., et al. (2007). A toolkit modeling approach for sustainable forest management planning: achieving balance between science and local needs. Ecol. Soc. 12:26. doi: 10.5751/ES-02102-120207
Sturtevant, B. R., Miranda, B. R., Shinneman, D. J., Gustafson, E. J., and Wolter, P. T. (2012). Comparing modern and presettlement forest dynamics of a subboreal wilderness: does spruce budworm enhance fire risk? Ecol. Appl. 22, 1278–1296. doi: 10.1890/11-0590.1
Sturtevant, B. R., Miranda, B. R., Wolter, P. T., James, P. M. A., Fortin, M.-J., and Townsend, P. A. (2014). Forest recovery patterns in response to divergent disturbance regimes in the Border Lakes region of Minnesota (USA) and Ontario (Canada). For. Ecol. Manage. 313, 199–211. doi: 10.1016/j.foreco.2013.10.039
Sturtevant, B. R., Scheller, R. M., Miranda, B. R., Shinneman, D., and Syphard, A. (2009). Simulating dynamic and mixed-severity fire regimes: a process-based fire extension for LANDIS-II. Ecol. Modell. 220, 3380–3393. doi: 10.1016/j.ecolmodel.2009.07.030
Talluto, M. V., Boulangeat, I., Ameztegui, A., Aubin, I., Berteaux, D., Butler, A., et al. (2016). Cross-scale integration of knowledge for predicting species ranges: a metamodelling framework. Glob. Ecol. Biogeogr. 25, 238–249. doi: 10.1111/geb.12395
Temperli, C., Veblen, T. T., Hart, S. J., Kulakowski, D., and Tepley, A. J. (2015). Interactions among spruce beetle disturbance, climate change and forest dynamics captured by a forest landscape model. Ecosphere 6:art231. doi: 10.1890/ES15-00394.1
Thom, D., Rammer, W., Garstenauer, R., and Seidl, R. (2018). Legacies of past land use have a stronger effect on forest carbon exchange than future climate change in a temperate forest landscape. Biogeosciences 15, 5699–5713. doi: 10.5194/bg-15-5699-2018
Thompson, J. R., Lambert, K. F., Foster, D. R., Broadbent, E. N., Blumstein, M., Almeyda Zambrano, A. M., et al. (2016). The consequences of four land-use scenarios for forest ecosystems and the services they provide. Ecosphere 7:e01469. doi: 10.1002/ecs2.1469
Thorn, S., Bässler, C., Brandl, R., Burton, P. J., Cahall, R., Campbell, J. L., et al. (2018). Impacts of salvage logging on biodiversity: a meta-analysis. J. Appl. Ecol. 55, 279–289. doi: 10.1111/1365-2664.12945
Titeux, N., Henle, K., Mihoub, J.-B., Regos, A., Geijzendorffer, I. R., Cramer, W., et al. (2016). Biodiversity scenarios neglect future land-use changes. Glob. Chang. Biol. 22, 2505–2515. doi: 10.1111/gcb.13272
Touchon, J. C., and McCoy, M. W. (2016). The mismatch between current statistical practice and doctoral training in ecology. Ecosphere 7:e01394. doi: 10.1002/ecs2.1394
Turner, D. P., Conklin, D. R., and Bolte, J. P. (2015). Projected climate change impacts on forest land cover and land use over the Willamette River Basin. Oregon, USA. Clim. Change 133, 335–348. doi: 10.1007/s10584-015-1465-4
Turner, M. G. (2010). Disturbance and landscape dynamics in a changing world. Ecology 91, 2833–2849. doi: 10.1890/10-0097.1
Urban, D. L., Acevedo, M. F., and Garman, S. L. (1999). “Scaling fine-scale processes to large-scale patterns using models derived from models: meta-models,” in Spatial Modeling Of Forest Landscape Change: Approaches And Applications, eds D. J. Mladenoff and W. L. Baker (Cambridge: Cambridge University Press), 70–98.
Urban, M. C., Bocedi, G., Hendry, A. P., Mihoub, J.-B., Peer, G., Singer, A., et al. (2016). Improving the forecast for biodiversity under climate change. Science 353:aad8466. doi: 10.1126/science.aad8466
Verburg, P. H., and Overmars, K. P. (2009). Combining top-down and bottom-up dynamics in land use modeling: exploring the future of abandoned farmlands in Europe with the Dyna-CLUE model. Landsc. Ecol. 24, 1167–1181. doi: 10.1007/s10980-009-9355-7
Wagener, T., and Pianosi, F. (2019). What has Global Sensitivity Analysis ever done for us? A systematic review to support scientific advancement and to inform policy-making in earth system modelling. Earth-Science Rev. 194, 1–18. doi: 10.1016/j.earscirev.2019.04.006
Wang, W. J., He, H. S., Fraser, J. S., Thompson, F. R., Shifley, S. R., and Spetich, M. A. (2014). LANDIS PRO: a landscape model that predicts forest composition and structure changes at regional scales. Ecography (Cop.). 37, 225–229. doi: 10.1111/j.1600-0587.2013.00495.x
Watson, S. J., Luck, G. W., Spooner, P. G., and Watson, D. M. (2014). Land-use change: incorporating the frequency, sequence, time span, and magnitude of changes into ecological research. Front. Ecol. Environ. 12:241–249. doi: 10.1890/130097
Westlake, S. M., Mason, D., Lázaro-Lobo, A., Burr, P., McCollum, J. R., Chance, D., et al. (2020). The magnet effect of fire on herbivores affects plant community structure in a forested system. For. Ecol. Manage. 458:117794. doi: 10.1016/j.foreco.2019.117794
Willgoose, G., Bras, R. L., and Rodriguez-Iturbe, I. (1991). A coupled channel network growth and hillslope evolution model: 1. Theory. Water Resour. Res. 27, 1671–1684. doi: 10.1029/91WR00935
Wimberly, M. C., Sohl, T. L., Liu, Z., and Lamsal, A. (2015). “Simulating forest landscape disturbances as coupled human and natural systems,” in Simulation Modeling of Forest Landscape Disturbances, eds A. H. Perera, B. R. Sturtevant, and L. J. Buse (Cham: Springer International Publishing), 233–261. doi: 10.1007/978-3-319-19809-5_9
Wu, J., and David, J. L. (2002). A spatially explicit hierarchical approach to modeling complex ecological systems: theory and applications. Ecol. Modell. 153, 7–26. doi: 10.1016/S0304-3800(01)00499-9
Xu, C., Gertner, G. Z., and Scheller, R. M. (2012). Importance of colonization and competition in forest landscape response to global climatic change. Clim. Change 110, 53–83. doi: 10.1007/s10584-011-0098-5
Keywords: compound disturbance, linked disturbance, feedback effects, resilience, forest landscape model (FLM), landscape legacy, cross-scale interaction (CSI)
Citation: Sturtevant BR and Fortin M-J (2021) Understanding and Modeling Forest Disturbance Interactions at the Landscape Level. Front. Ecol. Evol. 9:653647. doi: 10.3389/fevo.2021.653647
Received: 14 January 2021; Accepted: 01 September 2021;
Published: 04 October 2021.
Edited by:
Charles K. Lee, University of Waikato, New ZealandReviewed by:
Steve Cumming, Laval University, CanadaSusanne Suvanto, University of Birmingham, United Kingdom
Olalla Díaz-Yáñez, University of Eastern Finland, Finland
Copyright © 2021 Sturtevant and Fortin. This is an open-access article distributed under the terms of the Creative Commons Attribution License (CC BY). The use, distribution or reproduction in other forums is permitted, provided the original author(s) and the copyright owner(s) are credited and that the original publication in this journal is cited, in accordance with accepted academic practice. No use, distribution or reproduction is permitted which does not comply with these terms.
*Correspondence: Brian R. Sturtevant, YnJpYW4uci5zdHVydGV2YW50QHVzZGEuZ292