- 1Veterinary Services Department, Kenya Wildlife Service, Nairobi, Kenya
- 2IGAD Climate Prediction and Applications Centre, Nairobi, Kenya
- 3Department of Clinical Microbiology, Umeå University, Umeå, Sweden
- 4Institute of Primate Research, National Museums of Kenya, Nairobi, Kenya
Anthrax, an acute disease of homeotherms caused by soil-borne Bacillus anthracis is implicated in dramatic declines in wildlife mainly in sub-Saharan Africa. Anthrax outbreaks are often localized in space and time. Therefore, understanding predictors of the spatial and temporal occurrence of anthrax in wildlife areas is useful in supporting early warning and improved response and targeting measures to reduce the impact of epizootic risk on populations. Spatial localization of anthrax is hypothesized to be driven by edaphic factors, while the temporal outbreaks are thought to be driven by extreme weather events including temperature, humidity, rainfall, and drought. Here, we test the role of select edaphic factors and normalized difference vegetation index (NDVI) metrics driven by vegetation structure and climate variability on the spatial and temporal patterns of wildlife mortality from anthrax in key wildlife areas in Kenya over a 20-year period, from 2000 to 2019. There was a positive association between the number of anthrax outbreaks and the total number of months anthrax was reported during the study period and the nitrogen and organic carbon content of the soil in each wildlife area. The monthly occurrence (timing) of anthrax in Lake Nakuru (with the most intense outbreaks) was positively related to the previous month’s spatial heterogeneity in NDVI and monthly NDVI deviation from 20-year monthly means. Generalized linear models revealed that the number of months anthrax was reported in a year (intensity) was positively related to spatial heterogeneity in NDVI, total organic carbon and cation exchange capacity of the soil. These results, examined in the light of experimental studies on anthrax persistence and amplification in the soil enlighten on mechanisms by which these factors are driving anthrax outbreaks and spatial localization.
Introduction
Anthrax is an acute, febrile disease of homeotherms, caused by the gram-positive, non-motile, spore-forming, soil-borne bacteria; Bacillus anthracis and Bacillus cereus biovar anthracis (Koch, 1937; Hoffmaster et al., 2004; Leendertz et al., 2006b). The disease has a global distribution (Schild et al., 2006; Turnbull, 2008; Revich et al., 2012; Barro et al., 2016; Chen et al., 2016; Hoffmann et al., 2017; New et al., 2017), and infects a wide range of vertebrate species (Mollel, 1977; Lindeque, 1998; Leendertz et al., 2006a; Clegg et al., 2007; Beyer and Turnbull, 2009). Anthrax epizootics have caused significant declines in wildlife species by 10–30% in most outbreaks and by 80–90% in severe outbreaks (Creel et al., 1995; Hugh-Jones and De Vos, 2002; Leendertz et al., 2006a; Clegg et al., 2007; Muoria et al., 2007; Kaitho et al., 2013; Salb et al., 2014; Hoffmann et al., 2017).
Bacillus anthracis is endemic in many parts of the world with an epizootic transmission cycle (van Ness, 1971; Hugh-Jones and Blackburn, 2009). Areas that B. anthracis is deemed endemic to are characterized by high content of soil calcium, pH (pH ≥ 6.1), moisture, and organic carbon and nitrogen (Joyner et al., 2010; Chikerema et al., 2013; Kracalik et al., 2017; Steenkamp et al., 2018; Romero-Alvarez et al., 2020) and occur at low-lying topography (Dragon and Rennie, 1995; Munang’andu et al., 2012). B. anthracis consists of clade A and clade B among others, but clade A thrives in soil with wider ranges in pH and calcium whereas anthrax clade B is more common in soil rich in calcium and high pH (Smith et al., 2000). Regarding topology, seasonally flooded plains and low lying areas are known to be prone to anthrax outbreaks (Dragon and Rennie, 1995; Munang’andu et al., 2012).
Anthrax outbreaks are driven by a synergy of intrinsic and extrinsic factors linked to both the host and the pathogen. The occurrence and severity of anthrax are highly variable across seasons and years and appear to be driven by mean temperature, and precipitation in the temperate regions while in the tropics, precipitation is a dominant factor (Joyner et al., 2010; Chikerema et al., 2013; Kracalik et al., 2017; Steenkamp et al., 2018; Romero-Alvarez et al., 2020). Most anthrax epizootics in endemic tropical regions occur in the dry season and it is speculated that exposure to hot weather and subsequent heat stress and malnutrition from forage scarcity play a role in decreasing host resistance to infections (Hugh-Jones and Blackburn, 2009). However, this hypothesis does not address cases in which anthrax mortalities occur in the wet season or where the disease begins at the end of the rains or beginning of dry seasons. Nevertheless, recent evidence suggest that anthrax outbreaks are driven by extreme weather events (Hampson et al., 2011; Anttila et al., 2015) that may be caused by El Nino and La Nina and associated ENSO phenomenon. Similar timing of anthrax outbreaks has been observed in temperate and arctic climates, where outbreaks are associated with hot-dry summers preceded by heavy spring rain (Blackburn and Goodin, 2013; Maksimović et al., 2017). Extreme weather events may induce anthrax epizootics because precipitation and temperature are known to modulate ecological dynamics of soil-dwelling microbes known experimentally to amplify or inhibit B. anthracis multiplication in the soil (Clarholm, 1981; Rutherford and Juma, 1992; Anderson, 2000; Ritz and Young, 2011; Dey et al., 2012).
Another driver of temporal patterns of anthrax epizootics is vegetation as measured through NDVI. A few studies have confirmed a positive relationship between NDVI and anthrax outbreaks (Kracalik et al., 2017; Steenkamp et al., 2018) or variance in NDVI and occurrence of anthrax (Chikerema et al., 2013). Plant growth is experimentally known to enhance the proliferation of B. anthracis (Saile and Koehler, 2006; Ganz et al., 2014) and influence the dynamics of organisms such as Acanthamoeba (Rodriguez-Zaragoza et al., 2005; Fernández, 2015), which are speculated to amplify anthrax presence in the soil based on experimental results (Dey et al., 2012).
In this study, we examined the influence of edaphic factors particularly total organic carbon, total nitrogen, pH, total carbonate equivalent, cation exchange capacity, gypsum content and available water capacity on the frequency and duration of anthrax outbreaks. We also examined the influence of normalized difference vegetation index (NDVI) metrics - surrogates for climate variability, vegetation structure, plant diversity, plant growth or phenology and plant cover – on the monthly occurrence (timing) and the number of months of anthrax when anthrax mortality was reported each year (intensity) in selected wildlife areas in Kenya. Lastly, we combined both edaphic variables and NDVI metrics as predictors of anthrax mortality in wildlife in multilevel model analyses.
Materials and Methods
Wildlife Areas: Sizes, Climate, and Vegetation
The focal wildlife areas selected are those with endangered species, such as Rothschilds giraffe (Giraffa camelopardalis rothschildi Lydekker), black rhinoceros (Diceros bicornis Linn.) and white rhinoceros (Ceratotherium simum, Burchell) where veterinary investigations on causes of mortality are prioritized and likely to be reported. These areas included Hell’s Gate National Park (HGNP), Lake Nakuru National Park (LNNP), Nairobi (NNP), Tsavo East National Park (TENP), Tsavo West National Park (TWNP), Sibiloi National Park (SNP) and Mwea National Reserve (MNR) (Figure 1). Areal coverage, climate, and elevation for each of these protected areas are summarized in Table 1.
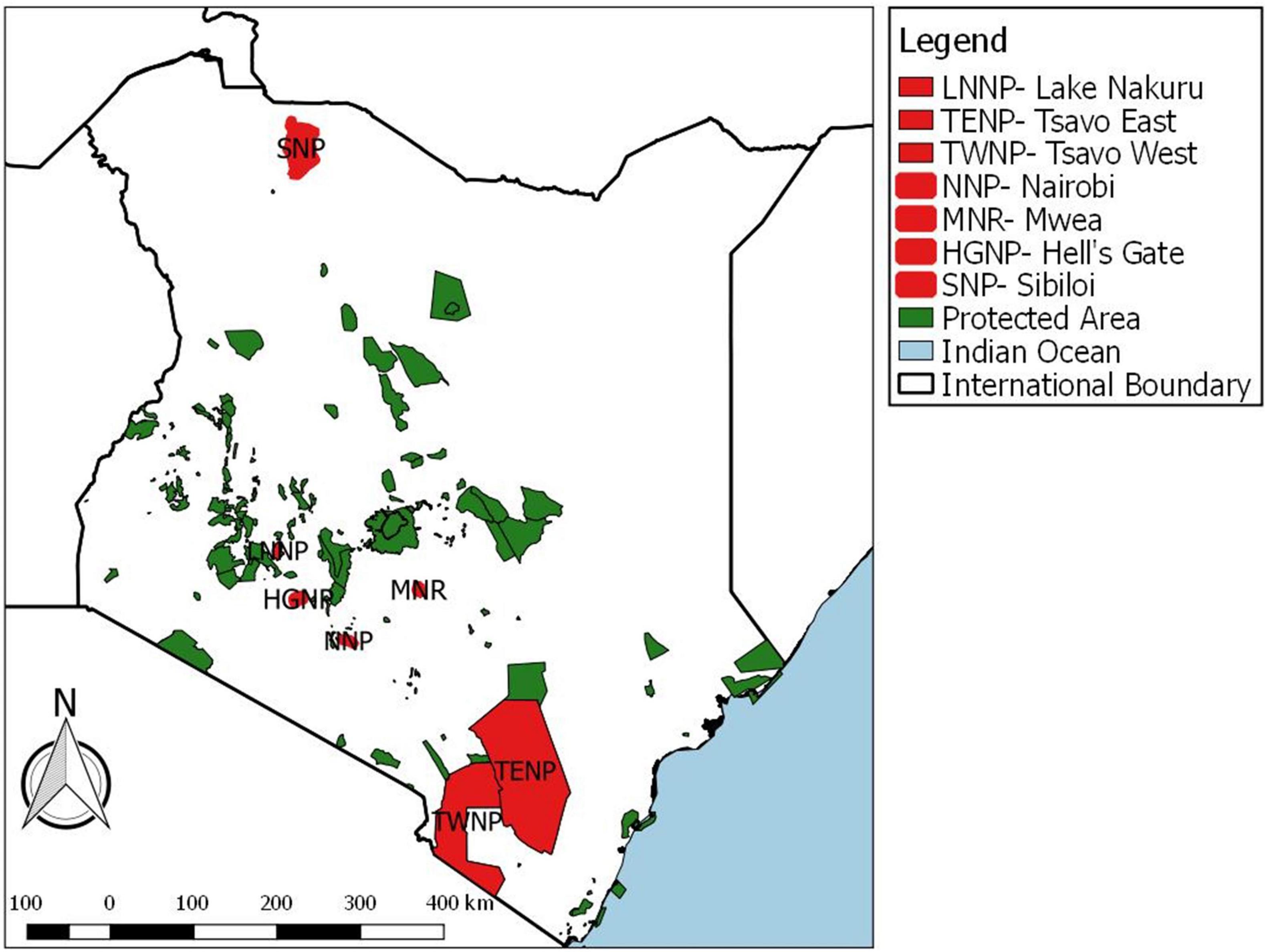
Figure 1. The spatial distribution of Kenya’s protected wildlife areas (NP, National Park; NR, National Reserve) showing focal wildlife areas examined for anthrax outbreaks. Focal wildlife areas are indicated in red and other wildlife areas are indicated by green.
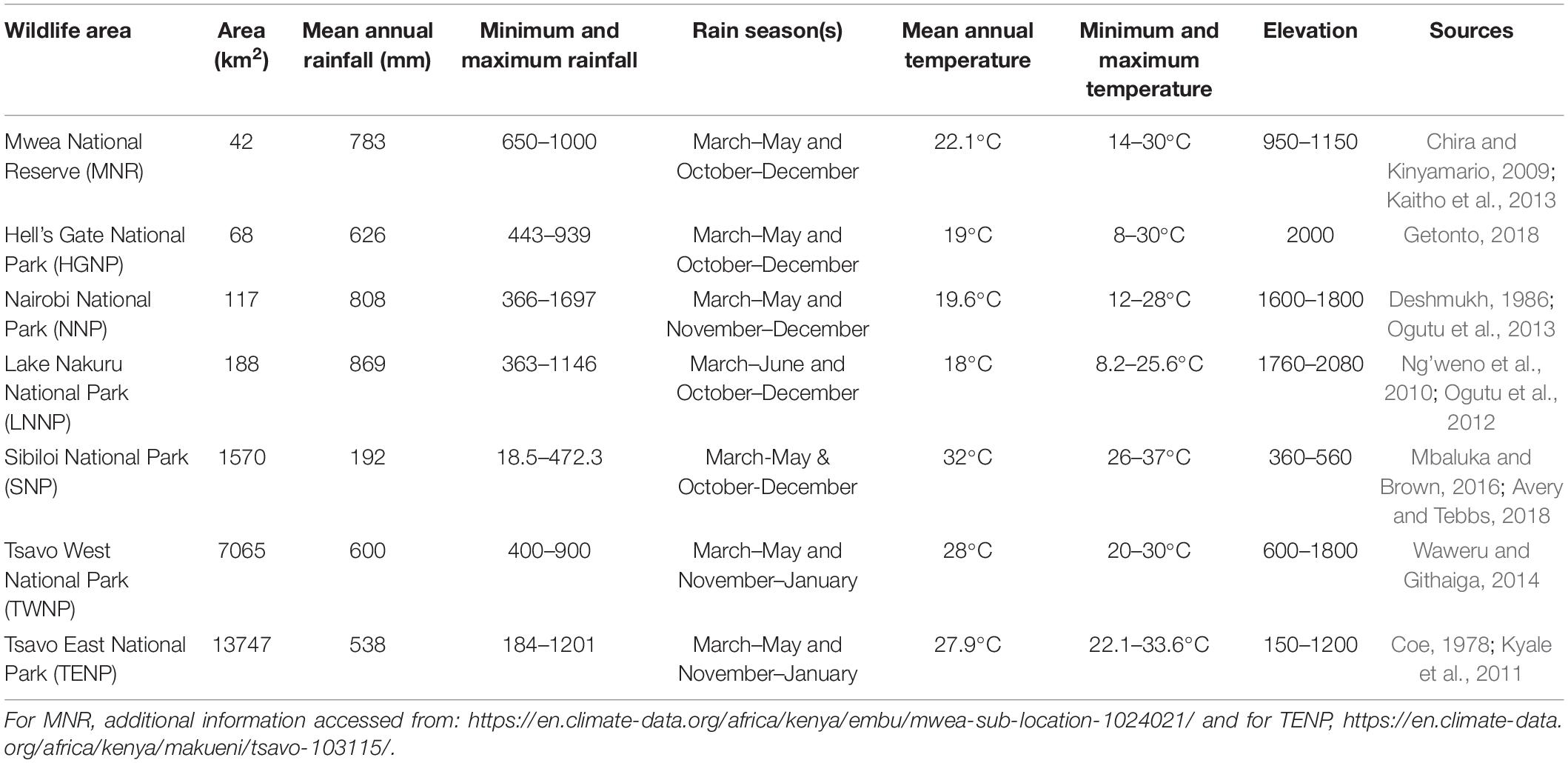
Table 1. Areal coverage, climatic and topographic variables of focal wildlife areas in Kenya examined.
The vegetation of LNNP consists of wooded and bushed grassland. The dominant grasses include Cynodon niemfluencis, Chloris gayana, Sporobolus spicatus, and Themeda triandra while bushlands and woodlands are dominated by closed stands of Euphorbia candelabrum and Acacia xanthophloea. NNP has a vegetation composed of lightly wooded grasslands on the plains to the east and dry forest in the upland areas to the west. The wooded grasslands are dominated by Acacia drepanolobium trees, and Themeda triandra, Pennisetum mezianum, Bothriochloa insculpta, and Digitaria macroblepharu grasses whereas the highland dry forest is dominated with Olea africana, Croton dichogamus, Brachylaena hutchinsii, and Calodendrum (Boutton et al., 1988). The vegetation of HGNP is predominantly open to dense scrubland, and open grassland. Scrubland is dominated by Acacia drepanolobium and Tarchonanthus camphoratus, while grasslands are dominated by Themeda triandra (Lovart and Lucherin, 1992). MNR has three vegetation types including bushlands dominated by Acacia mellifera, Commiphora africana, Grewia bicolor and Acacia ataxacantha, woodlands dominated by A. mellifera and C. africana and wooded grasslands covered by mainly under Terminalia brownie trees (Chira and Kinyamario, 2009). SNP has an arid vegetation dominated by grassland and dwarf shrubland, with riparian forest and riparian woodland at the shores (Mbaluka and Brown, 2016). The Vegetation of TWNP is dominated by Acacia–Commiphora bushland and Hyphaene compressa woodlands in the north and open grassy plains in the south (Wato et al., 2006). TENP has a semi-arid vegetation consisting mainly of bushland–grassland savannah and Acacia–Commiphora woodlands interspersed with Premna sp., Bauhinia sp. and Sericocomorpsis sp., Delonix elata and Melia volkensii trees in open plains (Kyale et al., 2011).
The Occurrence of Anthrax in Focal Wildlife Areas in Kenya
We extracted data on months when anthrax outbreaks occurred from disease surveillance reports at the Kenya Wildlife Service as well as published literature for seven wildlife areas in Kenya for the period 2000 to 2019 (Kaitho et al., 2013; Muturi et al., 2018). An Anthrax outbreak was defined as an occurrence of wildlife deaths more than what would normally be expected in a defined geographical area within a span of less than 2 weeks that warranted a disease investigation. We used only data from reports in which anthrax was determined by clinical examination of fresh carcasses and through microscopic examination of bacilli in blood-stained smears (Examples: Muoria et al., 2007; Aminu et al., 2020). Typically, thin blood smears were prepared in the field, stained with polychrome methylene blue and examined under a light microscope. The presence of blue square-ended rods, usually in short chains, surrounded by a pinkish-red capsule was used to diagnose the presence of B. anthracis (M’Fadyean, 1903). For each outbreak, we extracted the months, and records of wildlife species affected. The number of animals that died from each outbreak were opportunistically counted following confirmation of positive cases. There were no systematic surveys to determine detection probability and to objectively estimate the number of carcasses. The number of animals reported are therefore unreliable as estimates of the magnitude of outbreaks and are lower than the true number of animals that died. More generally, wildlife cases are usually underestimates compared to human or livestock anthrax cases.
Normalized Difference Vegetation Index Variables
Normalized difference vegetation index is a measure of differential reflectance and absorbance of the light spectra by vegetation and depends on the phenology and density of vegetation. NDVI is emerging as an important predictor of anthrax outbreaks as it integrates soil moisture, plant phenology, spatial variability in vegetation structure, species composition, topography, and variability in rainfall (e.g., Norris and Blackburn, 2019). The NDVI data used for this study covered January 2000 to December 2019 and was sourced from satellite images at the MODIS NDVI, a 16-day, 250-m resolution database1. Shape files of the study areas were obtained from the http://datasets.wri.org. The zonal statistic tool in ArcGIS 10.1 (ESRI, 2012) was used to calculate the mean, minimum, maximum, and standard deviation of NDVI values based on the number of 250-m pixels within each polygon and two time periods within each month. From these statistics, the spatial variation in NDVI, a metric which is positively correlated with plant diversity, vegetation structure and vegetation coverage was obtained (Gould, 2000; Fairbanks and McGwire, 2004; Oldeland et al., 2010; Rocchini et al., 2010; Pau et al., 2012), whereas temporal variability is correlated with climatic variability particularly precipitation and temperature that drive plant phenology (Hawinkel et al., 2016; Kalisa et al., 2019). Estimates of mean monthly NDVI and monthly standard deviation of NDVI across pixels for each wildlife area (spatial NDVI heterogeneity) were used as independent covariates. In addition, we calculated monthly NDVI deviation from long-term monthly average over a 20-year period and temporal heterogeneity in NDVI or the standard deviation from the annual average.
Edaphic Variables
The soil data were obtained from the ISRIC- an International World Soil Reference and Information Centre2. We extracted the following soil variables using data from soil classification maps for our focal areas; soil type, soil type specific mass fraction (g/kg––1) of total organic carbon, total nitrogen and alkalinity or pH, a measure of hydrogen ions [H+] concentration expressed on a negative logarithmic scale of the hydrogen ion concentration [H+] in water from SOTER-based soil parameter estimates (SOTWIS) for Kenya3. Further, we extracted soil-type specific average; total carbonate equivalent (g/kg–1), cation exchange capacity (cmolc/kg–1), gypsum content (g/kg–1), and available water capacity (vol.% –33 to –1500 kPa, USDA standards). We clipped soil data to each wildlife area shape file polygon. The area of coverage of each soil type was calculated using ArcGIS 10.1 software (ESRI, 2012). To obtain a single measure for each soil variable above for each wildlife area, the value for each soil type was multiplied by the areal coverage of that soil type and summed across soil types and divided by total wildlife area.
Statistical Analyses
First, we examined the influence of edaphic factors [total organic carbon (g/kg–1), total nitrogen (g/kg–1), pH, lime, or total carbonate equivalent (g/kg–1), cation exchange capacity (cmolc/kg–1), gypsum content (g/kg–1), and available water capacity] on anthrax outbreaks across protected areas. The total number of months anthrax was reported and the number of outbreaks for each wildlife area during the study period were used as dependent variables in a Poisson regression using a generalized linear model (GLM) framework. We performed univariate and multivariate relationships among dependent and independent variables. In a multivariate GLM, we selected the most important variables using AIC implemented in the MuMIn package (Barton and Barton, 2015).
Second, we examined the influence of NDVI metrics on anthrax outbreaks at the monthly level and at the annual level. To identify the possible drivers of the monthly timing of anthrax, we used data for Lake Nakuru National Park for which there were many months when anthrax was reported. We compared this with monthly timing of anthrax outbreaks for all wildlife areas. The presence of anthrax cases reported in a month was used as a binary dependent variable and monthly NDVI metrics, including monthly NDVI, spatial NDVI heterogeneity, monthly NDVI deviation from a 20-year mean were used as dependent variables. Additionally, we used lagged NDVI variables above (values from the previous month) as independent variables. We performed both univariate and multivariate relationships using a logistic regression in a GLM framework for Lake Nakuru NP and a generalized mixed model (GLMM) framework for all wildlife areas combined. For GLMM, wildlife area ID was entered as a random effect and edaphic variables were also incorporated in a set of multivariate analyses. The best temporal predictors for monthly timing of anthrax in Lake Nakuru and all wildlife areas combined were selected using the AIC implemented in the MuMIn package in R software for statistical computing (Barton and Barton, 2015).
At the annual level, we used the number of months that anthrax deaths were recorded each year in a wildlife area as a dependent variable. We made this aggregation for two reasons: (1) to reduce data sparseness and enhance model stability and convergence and (2) it is a better proxy for intensity or size of outbreak in wildlife where mortality estimates are not reliable indicator of intensity, but the duration of an outbreak is a better indicator of the intensity. As independent variables, we obtained annual means for spatial NDVI heterogeneity, NDVI, and monthly deviations from 20-year means for each month and the annual standard deviation of NDVI for annual heterogeneity. We used a Poisson regression under a generalized linear mixed model framework (GLMM) with a log link function for statistical analysis with the number of months in a year when anthrax occurred as a dependent variable. Wildlife area ID was incorporated into the model as a random effect. All GLMMs were performed using the glmmTMB package in the R software for statistical computing (Brooks et al., 2017). Conditional effects of covariates were visualized using the R package ggeffects (Lüdecke, 2018). We also tested the effect of edaphic factors, total organic carbon (g/kg–1), total nitrogen (g/kg–1), pH, lime, or total carbonate equivalent (g/kg–1), cation exchange capacity (cmolc/kg–1), gypsum content (g/kg–1), and available water capacity and annual NDVI metrics as independent covariates and number of months anthrax is reported in a year as a dependent variable.
In all multivariate analyses undertaken above, we tested for collinearity as it can lead to unstable parameter estimates and inaccurate variances which affects confidence intervals, hypothesis tests and model selection in a multivariable model (Shen and Gao, 2008). To address this, we took the following steps. First, we checked for multicollinearity among covariate using the performance package in R (Lüdecke et al., 2021) and confirmed that multicollinearity was pervasive (Supplementary Table 1). Second, we performed univariate analyses with a single covariate using maximum likelihood estimation and ranked the importance of our covariates using Akaike Information Criteria (AIC). Thirdly, we used the MuMIn package (Barton and Barton, 2015) to compare all possible permutation of independent variables and selected the best model based on AIC.
All AIC model selection details are provided in the Supplementary Material 1.
Results
Anthrax Outbreaks, Edaphic Factors and Normalized Difference Vegetation Index Variables in Focal Wildlife Areas
Fourteen anthrax outbreaks were recorded during the study period from 2000 to 2019 in six of the seven focal wildlife areas. The average number of months per outbreak was 1.92 ± 0.97. The longest outbreaks lasted 3–4 months but most outbreaks did not last more than a month (Table 2). LNNP and NNP recorded the highest number of outbreaks at five outbreaks each, during the years examined (Table 2). TWNP, HGNP, SNP, and MNR experienced a single outbreak each while TENP did not experience an outbreak during the years examined. However, anthrax is known to have occurred in TENP and TWNP in 1996. Most anthrax outbreaks (71% or 10/14) occurred at the beginning of the dry season or end of the wet season. In terms of years, 2006 had a very widespread occurrence of anthrax outbreaks, occurring in four of seven wildlife areas considered in this study (Table 2). Several species suffered anthrax mortality. The dominant species documented in terms of frequency and numbers are the African buffalo (Syncerus caffer Sparrman), Thomson’s gazelle (Eudorcas thomsonii Günther), Rothchild’s giraffe (Giraffa camelopardalis rothschildi Lydekker), and common zebra (Equus quagga burchellii Gray). Others documented include white rhinoceros (Ceratotherium simum, Burchell), black rhinoceros (Diceros bicornis Linn.), and lesser kudu (Tragelaphus imberbis Blyth) (Table 2).
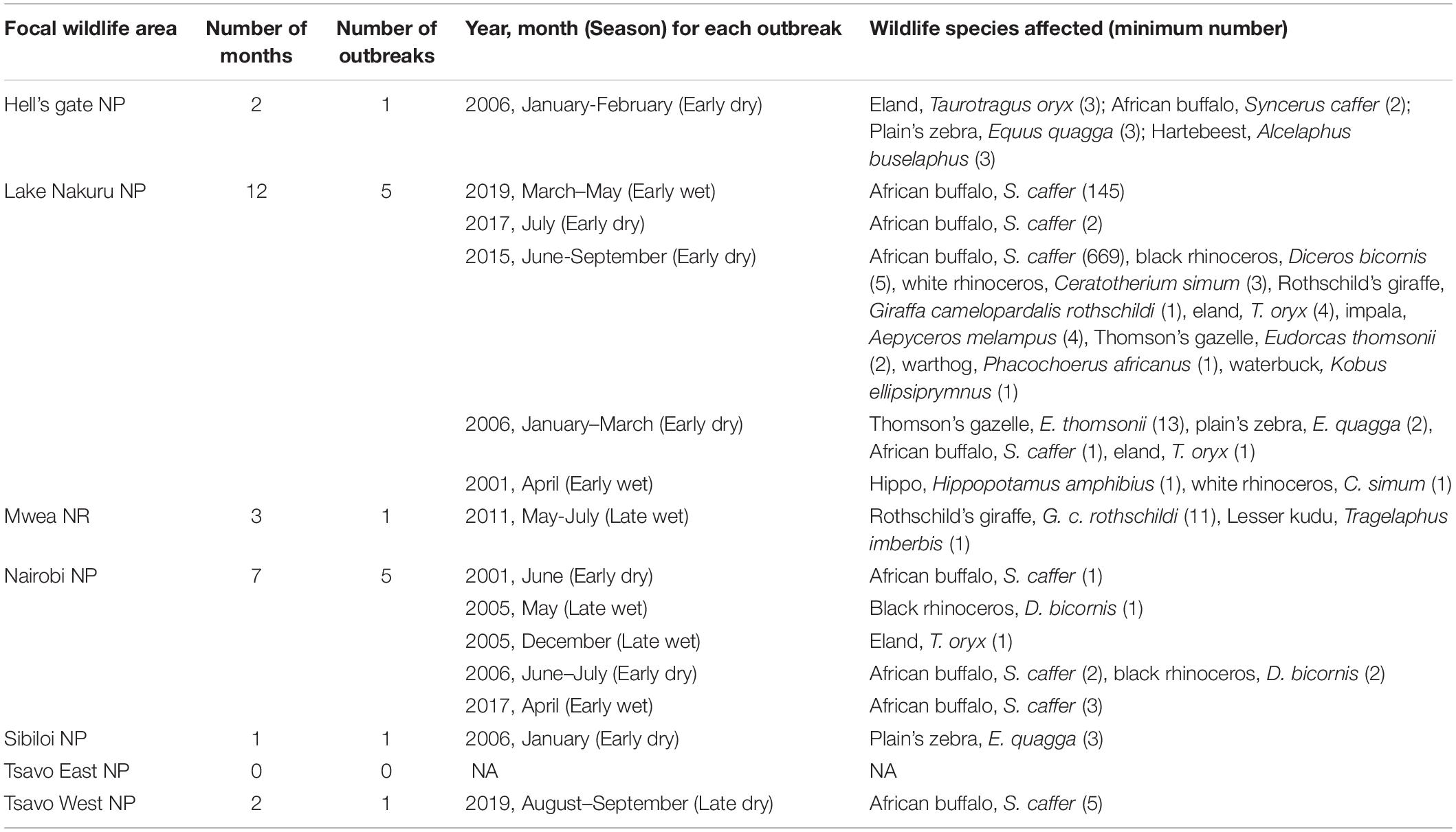
Table 2. Anthrax outbreaks from 2000 to 2019 in selected wildlife areas (NP, National Park and NR, National Reserve) in Kenya.
The average soil pH across most wildlife areas was greater than 6.0 except for TWNP (Table 3). The average organic carbon content across all wildlife areas ranged from 6.44 g/kg of soil in HGNP to 18.68 g/kg of soil in LNNP while Nitrogen content ranged from 0.78 g/kg of soil in SNP to 1.58 g/kg of soil in LNNP (Table 3). Lime content measured as total carbonate equivalent was highest in HGNP and SNP at 79.14 and 55.73 respectively and lowest in MNR and TWNP (Table 3). Cation exchange capacity (cmolc/kg–1), and available water capacity were highest in HGNP and lowest in TENP and TWNP respectively.
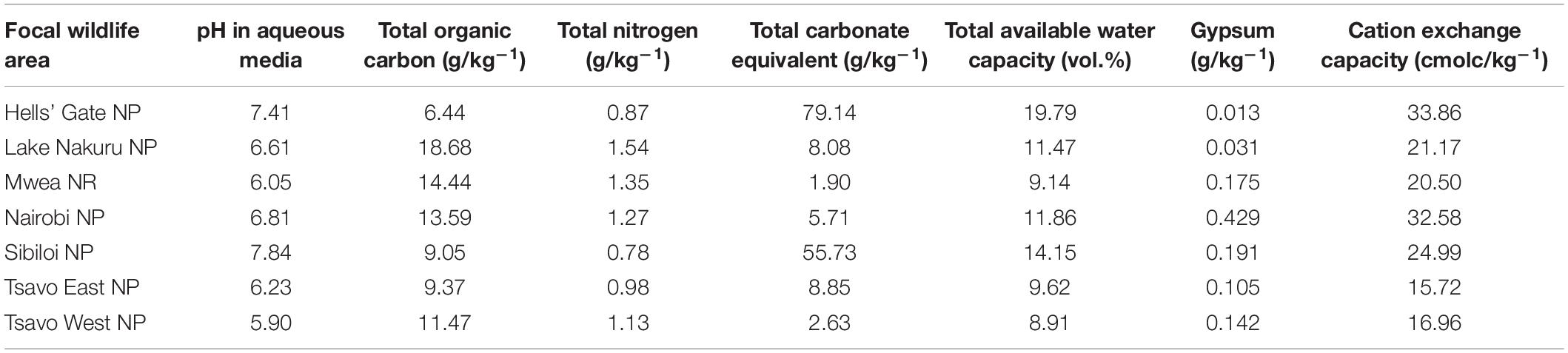
Table 3. Average values of selected edaphic covariates of the focal wildlife areas (NP, National Park; NR, National Reserve) in Kenya.
The wildlife areas in Kenya were typically dry with mean monthly NDVI varying from 0.574 in LNNP to a low of 0.162 in SNP over 20 years (Table 4). The mean of monthly standard deviation was highest in LNNP at 0.117 and lowest in NNP at 0.047 and SNP at 0.042 (Table 4).
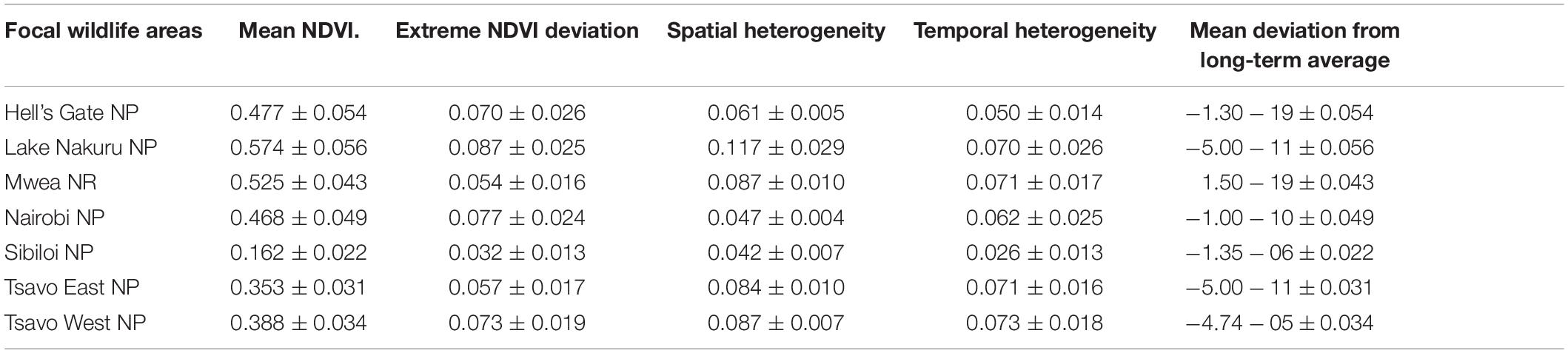
Table 4. Average values of NDVI metrics across focal wildlife areas (NP, National Park; NR, National Reserve) in Kenya from 2000–2019.
Variables Influencing Spatial Variation in Anthrax Outbreaks in Focal Wildlife Areas
Univariate GLM models revealed that the total number of recorded anthrax outbreaks in each wildlife area was positively influenced by total organic soil carbon and total organic nitrogen (Table 5 and Figures 2A,B). Similarly, the number of cumulative month when anthrax outbreaks were reported for each wildlife area was also positively influenced by total organic carbon and organic nitrogen in the soil (Table 5 and Figures 2C,D). All other edaphic variables were not statistically significant (Table 5). No multivariate model was selected as the most parsimonious in multivariate model selection of the edaphic factors affecting both the total number of months and number of anthrax outbreaks in wildlife areas. Instead, total soil organic carbon was the best predictor, but nitrogen was also supported (Number of months ΔAIC = 0.28, Number of outbreaks; ΔAIC = 0.38). Total soil organic carbon and total nitrogen were highly correlated (r = 0.95, t = 6.98, df = 5, P = 0.0009, Supplementary Table 1) when the number of months (VIF = 22.78) and number of outbreaks (VIF = 18.36) were used as dependent variables.
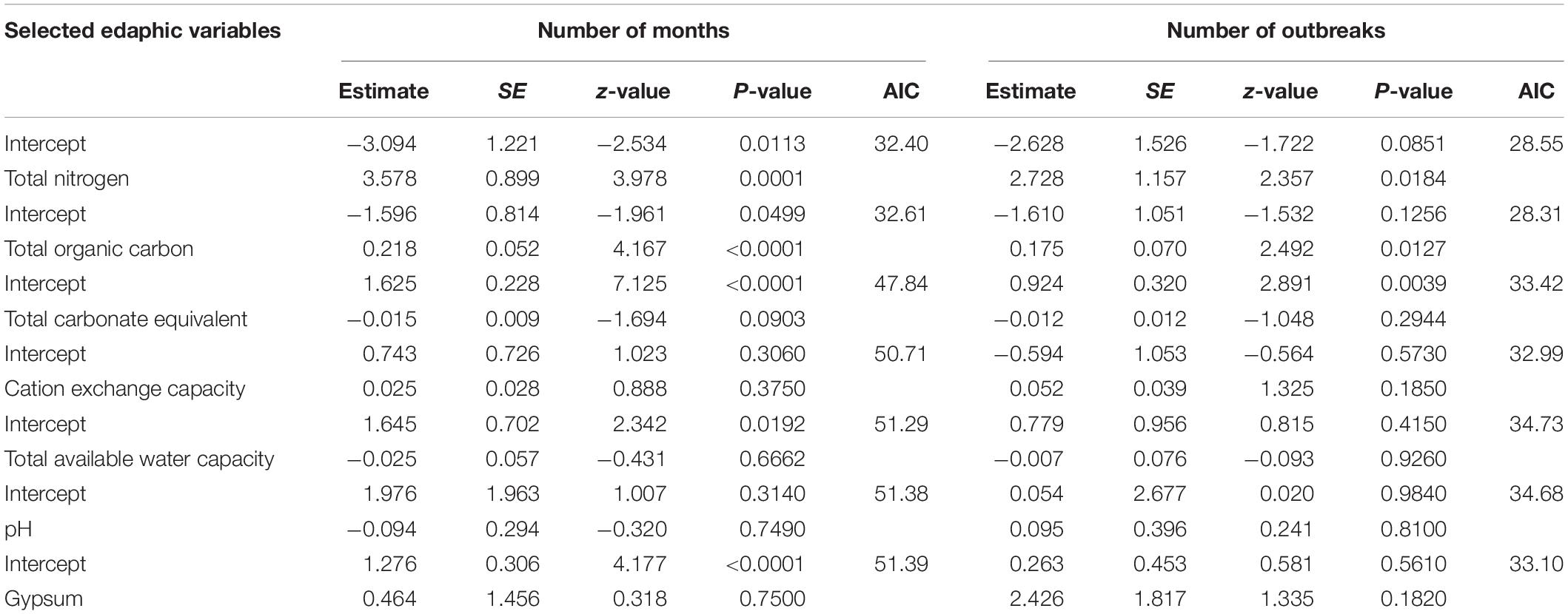
Table 5. The influence of selected edaphic variables on the number of months and the number of anthrax outbreaks in focal wildlife areas of Kenya 2000–2019.
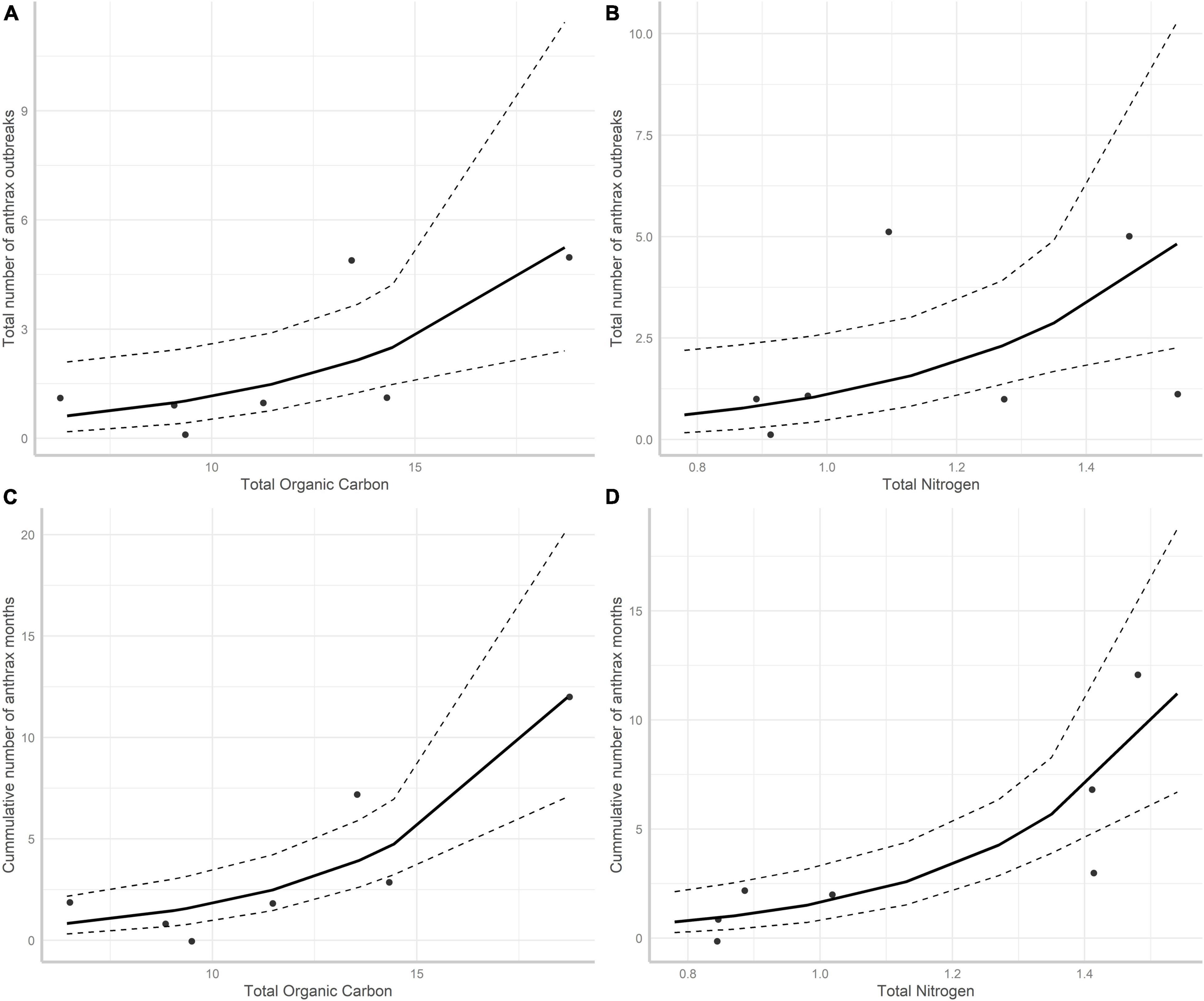
Figure 2. The relationships between total organic carbon (g/kg–1), total nitrogen (g/kg–1), with the total number of anthrax outbreaks (A,B) and cumulative total months anthrax mortality was recorded (C,D) in Kenya’s wildlife focal areas. The predicted values and their confidence intervals were obtained from a Poisson GLM analysis.
Factors Influencing Monthly Timing of Anthrax Outbreaks in Lake Nakuru National Park and All Focal Wildlife Areas
Univariate GLM analyses revealed that monthly occurrence (timing) of anthrax mortality in LNNP was positively associated with spatial heterogeneity in NDVI from the current and previous months (Table 6 and Figure 2). Monthly NDVI, and monthly NDVI deviations from 20-year mean for each month both from the current and previous months did not influence the occurrence of anthrax mortality in LNNP (Table 6). However, in a multivariable GLM analyses, both spatial NDVI heterogeneity and monthly NDVI deviations from a 20-year mean for each month from the previous months were included as important predictors of the monthly timing of anthrax mortality in LNNP (Table 6 and Figures 3A,B).
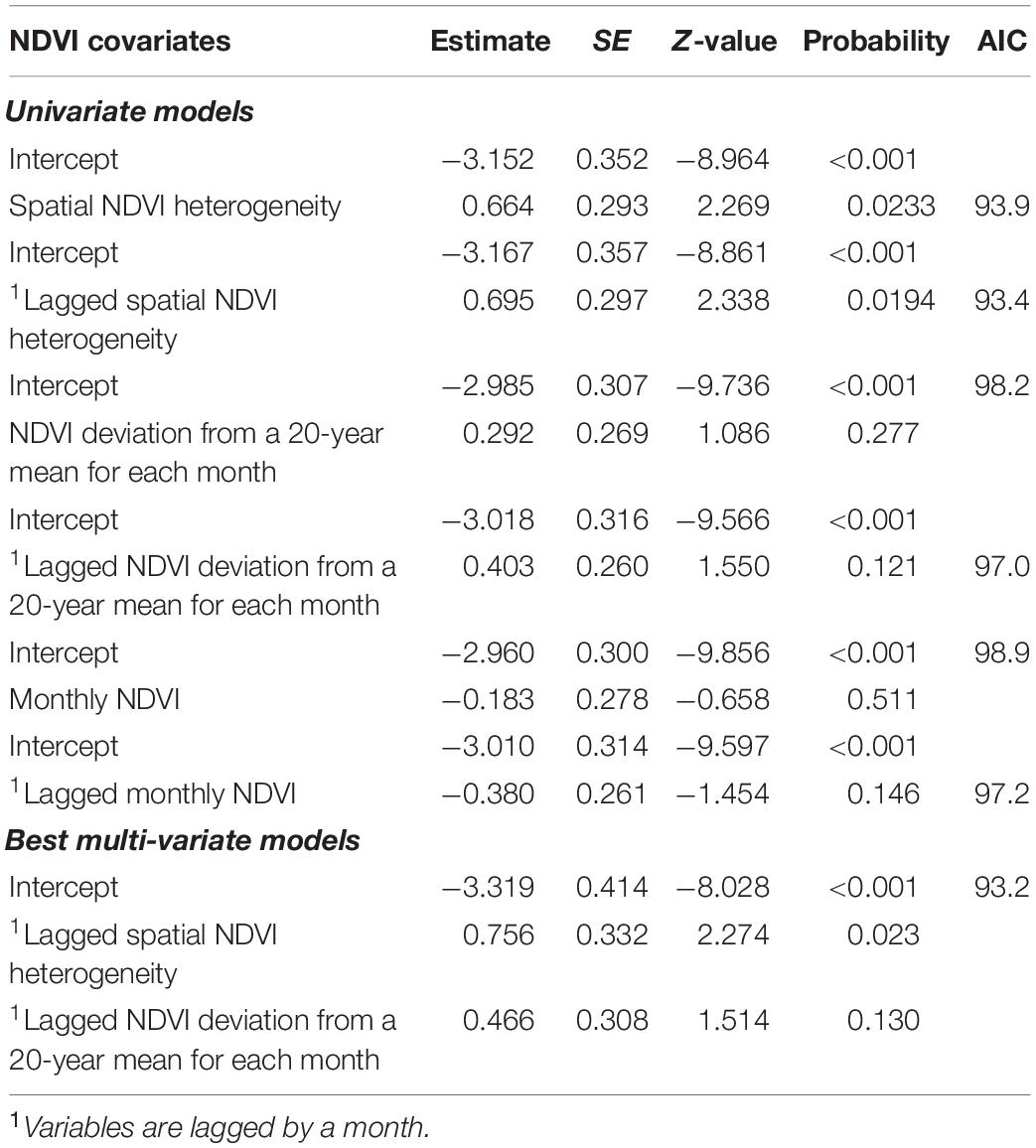
Table 6. Univariate and multivariate logistic models showing the influence of NDVI metrics on the monthly occurrence of anthrax outbreaks in wildlife from LNNP, Kenya
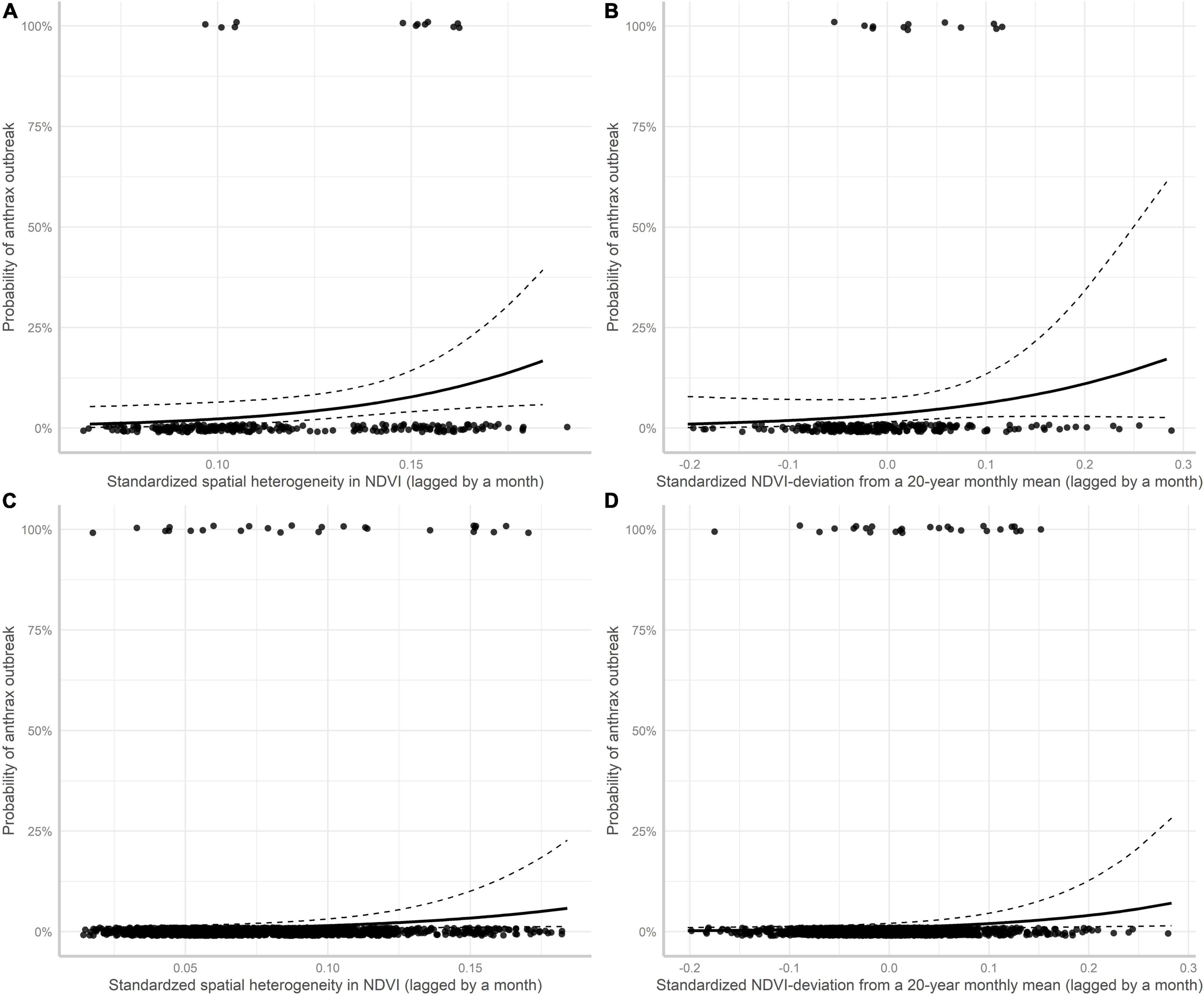
Figure 3. The influence of NDVI metrics on the probability of monthly anthrax mortality in Lake Nakuru NP (A,B) and combined focal wildlife areas (C,D). The predicted values and their confidence intervals are from a GLM (A,B), and GLMM (C,D) binomial regressions respectively.
Moreover, multivariate GLMM analyses and model selection on anthrax mortality in all focal wildlife areas in Kenya revealed that monthly NDVI deviation from 20-year mean for each month and spatial NDVI heterogeneity both from the previous month positively influenced monthly occurrence of mortality from anthrax (Table 7 and Figures 3C,D). Combined models including edaphic variables and NDVI metrics indicated that the previous month’s spatial NDVI heterogeneity, and NDVI deviation from long-term average, total organic carbon and cation exchange capacity influenced the monthly occurrence of wildlife mortality in wildlife focal areas (Table 8).
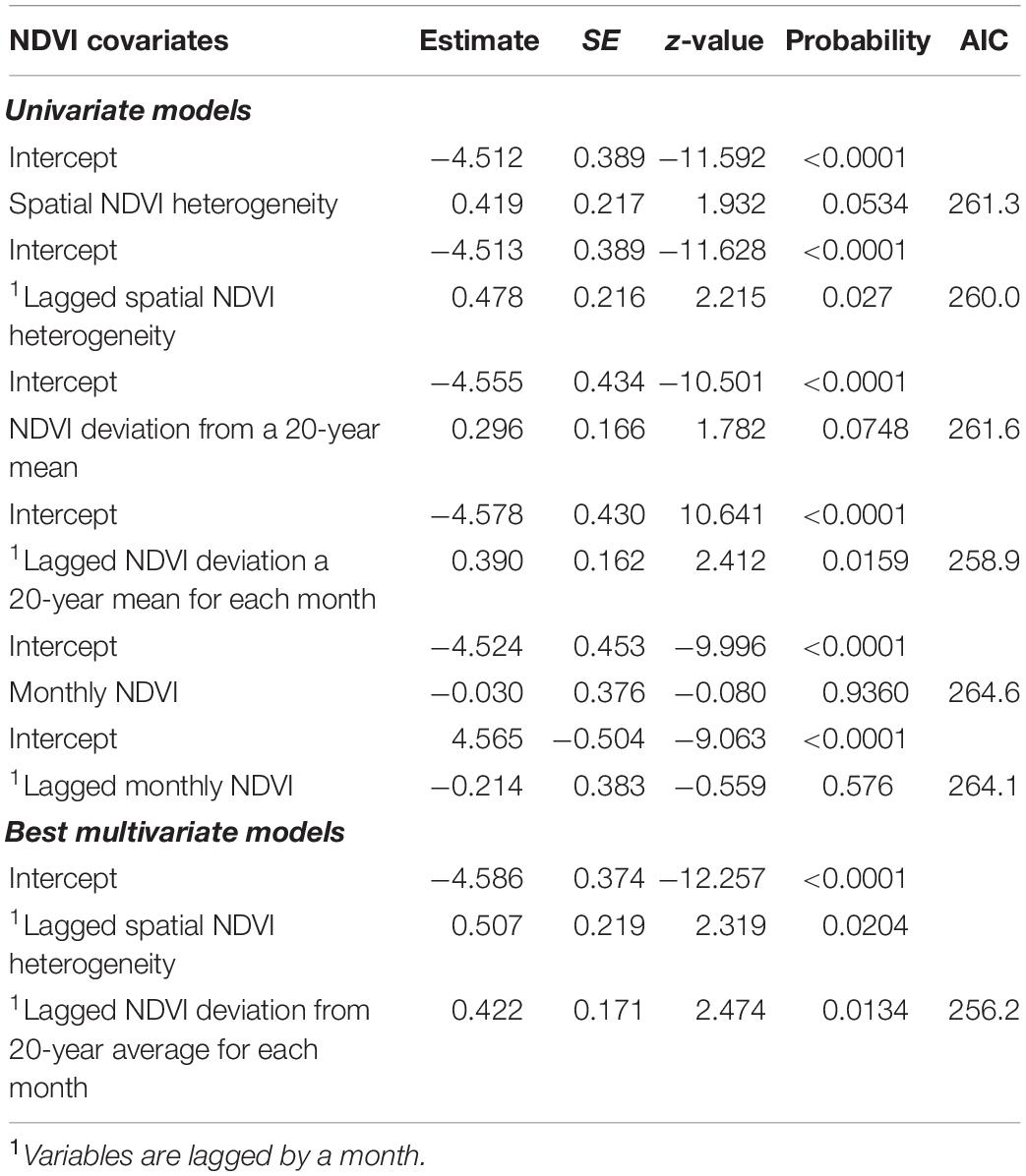
Table 7. Univariate and the best multivariate NDVI models from GLMM analyses showing their influence on monthly occurrence of anthrax in focal wildlife area, Kenya.
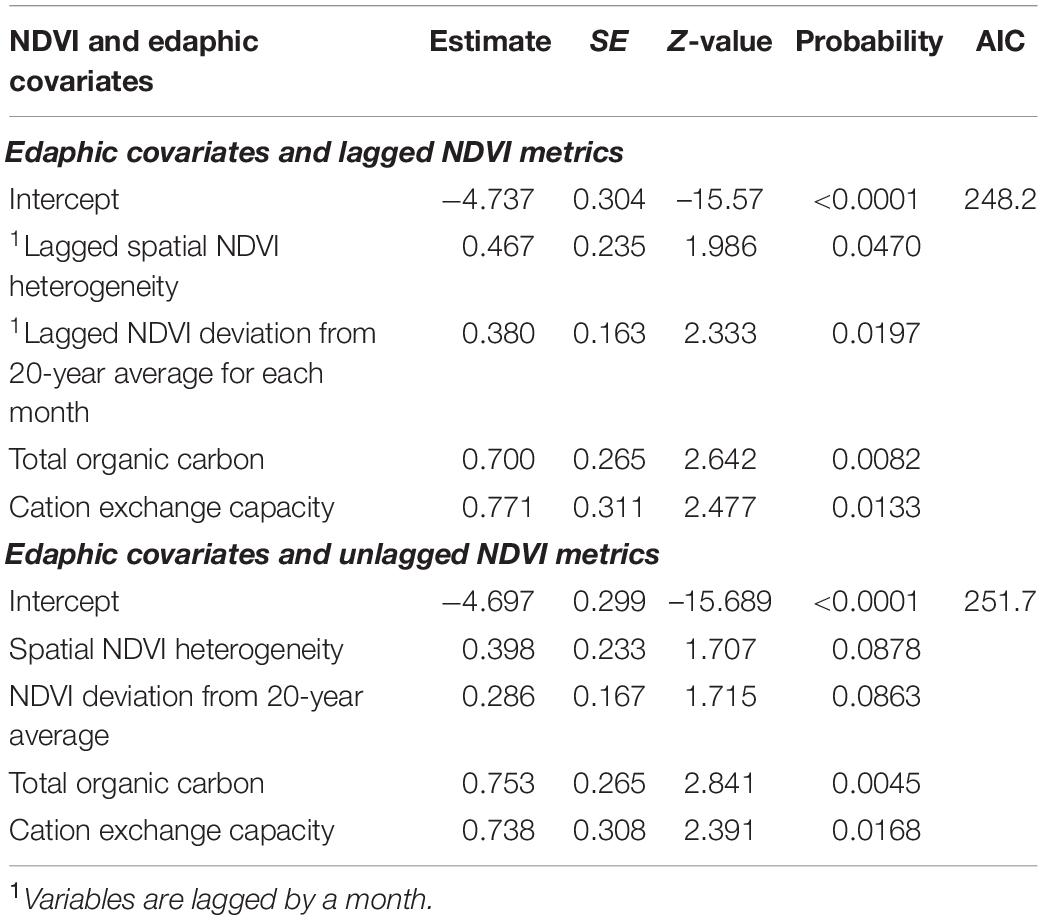
Table 8. Best multivariate logistic model combining edaphic and NDVI-metric predictors of the monthly occurrence of anthrax in wildlife focal areas showing lagged and unlagged NDVI metrics.
Influence of Normalized Difference Vegetation Index-Metrics and Edaphic Variables on the Annual Intensity of Anthrax in Focal Wildlife Areas
Univariate GLMM analyses revealed statistically significant positive relationship between the number of months when anthrax mortality was reported per year and mean spatial heterogeneity in NDVI among NDVI metrics (Table 9). There was no multivariate model more parsimonious than the best univariate model. Among edaphic factors, total organic carbon in the soils and cation exchange capacity were the most parsimonious predictors (Table 10). Alkalinity or pH, gypsum, available water capacity and total carbonate equivalent were not significantly correlated with occurrence and duration of anthrax outbreaks (Table 10).
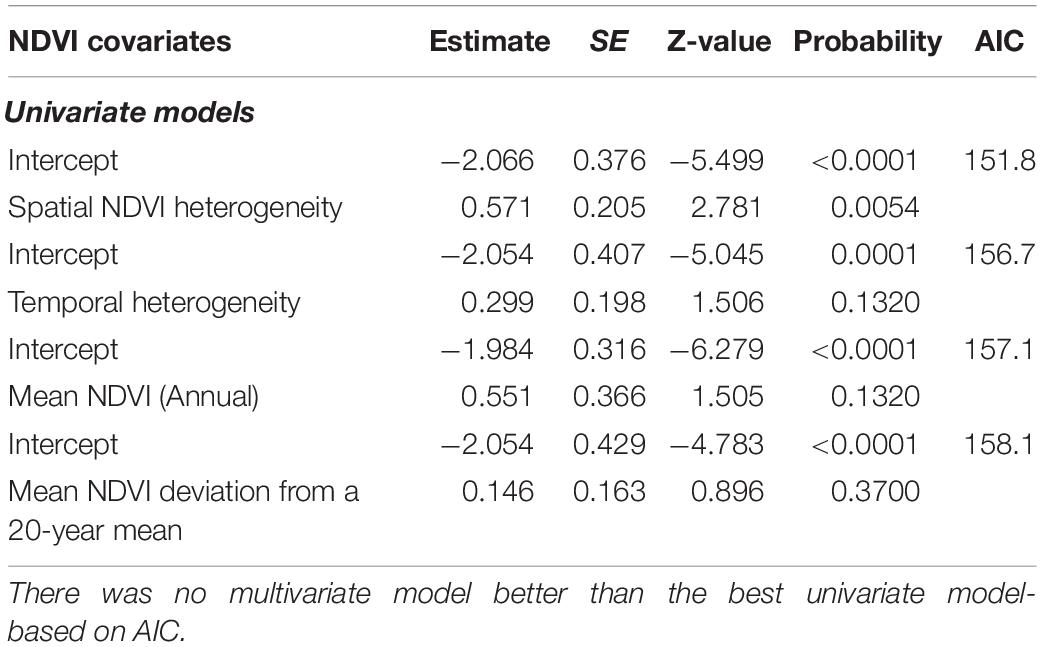
Table 9. Univariate NDVI-metric Poisson models and their influence on the number of months in a year (intensity) anthrax was reported from wildlife areas in Kenya.
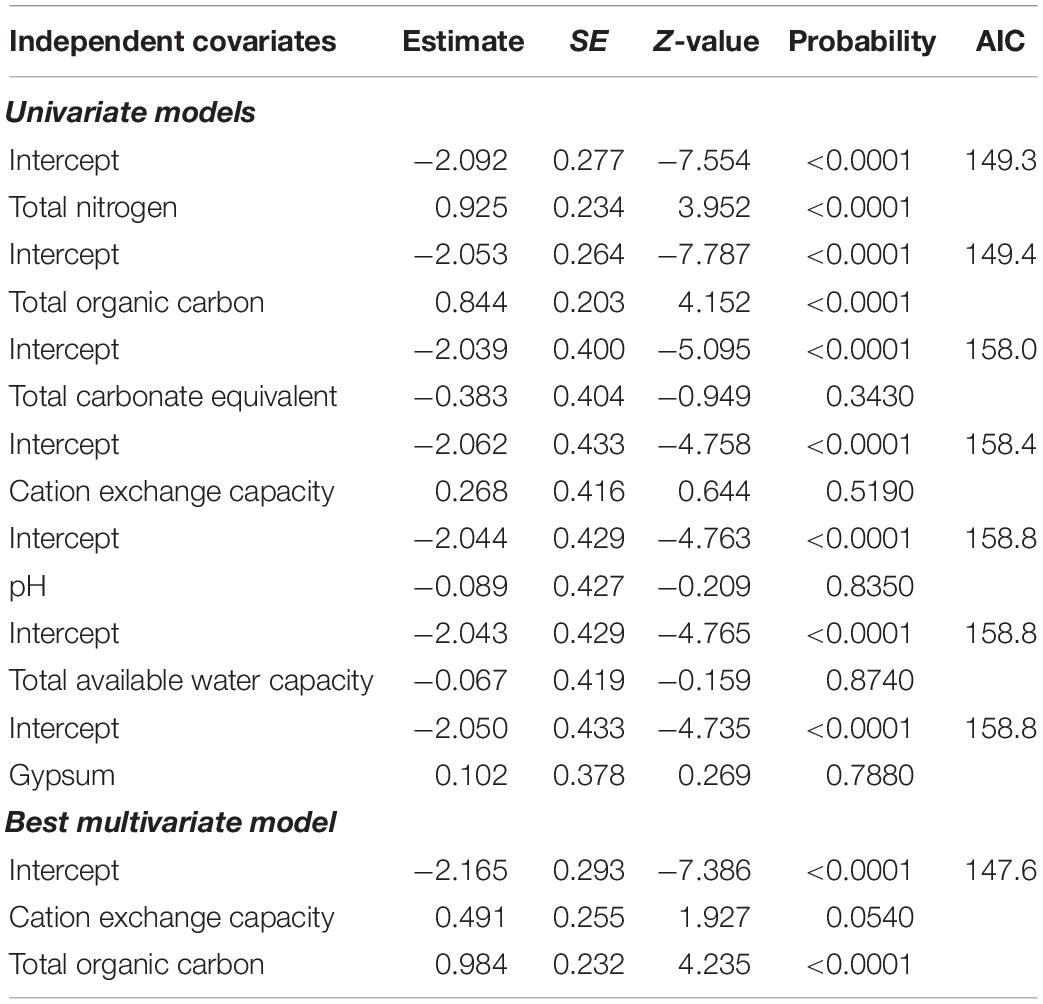
Table 10. Univariate models and the best multivariate model for the influence of edaphic factors on the number of months anthrax mortality occurred in a year in focal wildlife areas.
Multivariable GLMM analyses and model selection of combined edaphic variables and NDVI metrics, indicated that spatial NDVI heterogeneity, total organic carbon and cation exchange capacity were the best predictors of the number of months anthrax mortality was recorded in a year (Table 11).
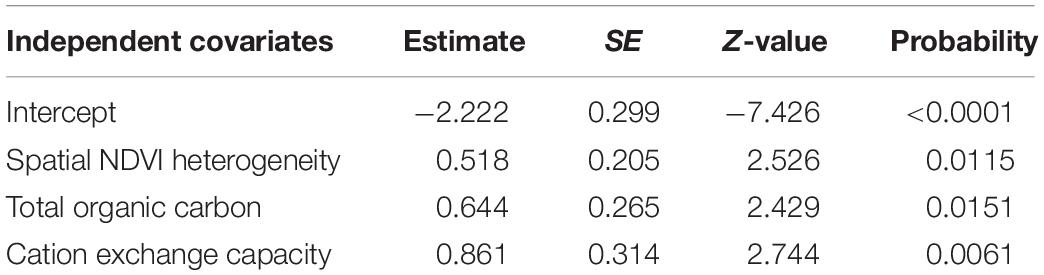
Table 11. The best multivariate GLMM model for the influence of edaphic variables and NDVI metrics on the number of months an anthrax outbreak was recorded in a year in Kenya’s focal wildlife areas.
Discussion
In this study, we found a statistically significant positive relationship between monthly occurrence of anthrax (timing) and monthly NDVI deviation from a 20-year mean for each calendar month. These findings suggest that rainfall fluctuation is an important driver of anthrax outbreaks in endemic areas as rainfall has a major influence on NDVI variability in East Africa (Kalisa et al., 2019; Dagnachew et al., 2020). Specifically, anthrax mortality in wildlife was associated with above average rainfall as inferred from a positive relationship between anthrax occurrence and the previous month’s NDVI deviations from each month 20-year mean. These results are consistent with empirical data showing that anthrax outbreaks occur following heavy rains (Hampson et al., 2011; Maksimović et al., 2017; Rume et al., 2020) and in locations with high NDVI variability (Chikerema et al., 2013). For example, studies in the Serengeti Ecosystem observed an association between anthrax outbreaks in wildlife with cumulative extremes in weather conditions; two outbreaks followed heavy rains, while two other outbreaks occurred during prolonged dry periods (Hampson et al., 2011). Anthrax niche model for Zimbabwe indicated that large variance in NDVI was positively correlated with anthrax occurrence in some locations in that country (Chikerema et al., 2013).
However, anthrax outbreaks are often associated with extreme weather events but the mechanism by which this enhances outbreaks is unclear. One possible hypothesis is that extreme weather events modulate multiple environmental factors as well as ecological dynamics of soil dwelling organisms known to amplify or inhibit anthrax multiplication in the soil. Experimental analyses have shown that protists particularly amoebas contribute to the persistence and amplification of B. anthracis in natural environments (Dey et al., 2012). These studies have revealed that spores germinate within phagosomes of amoebas, where vegetative bacilli multiply and sporulate in the extracellular milieu following the demise of amoeba. Interestingly, the most important factor driving protists abundance is precipitation (Anderson, 2000). Specifically, precipitation after a prolonged drought has been shown to cause a 20-fold increase in the abundance of naked amoebae few days after rainfall (Clarholm, 1981). This is because protists essentially depend on the water layer connecting soil pores to move, feed and multiply (Rutherford and Juma, 1992; Ritz and Young, 2011) but when soils are moist, there is strong chemical warfare between abundant bacteria and protist grazers (Matz and Kjelleberg, 2005; Bonkowski and Clarholm, 2012; Jousset, 2012) and drought inactivates this inhibitory effect (Foissner, 1987). In support, most of the anthrax outbreaks we observed occurred at the beginning of the dry season, when conditions may be favorable for protists.
Monthly timing of anthrax in LNNP and all focal wildlife areas or the number of months in a year when there was an anthrax mortality were positively related to the spatial heterogeneity in NDVI. This result suggests that diversity and heterogeneity in vegetation cover or species richness has an important influence on anthrax outbreaks. Spatial and temporal heterogeneity in vegetation cover can influence the distribution and migratory behavior of herbivores (Winnie et al., 2008; Peters et al., 2017; Duparc et al., 2020), enhancing aggregation in greener habitats. Such aggregations can lead to overgrazing of rich patches and an increased risk of anthrax infection (Gainer, 1987). For example, Turner et al. (2014) found improved vegetation vigor on zebra carcass sites and persistence of Bacillus anthracis on grasses for up to 2 years after death, thereby demonstrating that animal carcasses enhance local vegetation heterogeneity and attract grazing hosts to B. anthracis hotspots and thus increasing transmission risk and potential for anthrax outbreaks. In dry areas, presence of certain species of shrubs was found to be correlated with high densities of Acanthamoeba (Rodriguez-Zaragoza et al., 2005), protist species speculated to amplify anthrax presence in the soil based on experimental results (Dey et al., 2012). Similarly, areas covered with vegetation are known to be rich in protists compared to areas devoid of vegetation (Fernández, 2015). A more direct association between vegetation and B. anthracis proliferation has been demonstrated experimentally using simple grass plant-soil model system, that have revealed that B. anthracis can survive as a saprophyte outside of a mammalian host, germinating and growing characteristic long filaments on and around plant roots (Saile and Koehler, 2006). Another experiment using a local, virulent strain of B. anthracis in an enclosure within a grassland savanna, revealed that B. anthracis increased the rate of establishment of a native grass (Enneapogon desvauxii) by 50% and that grass seeds exposed to blood attained heights that were 45% taller than controls (Ganz et al., 2014). Interactions between B. anthracis and plants may result in increased host grazing and subsequently increased transmission to hosts. These studies agree with observations that anthrax outbreaks are positively correlated with NDVI, a measurement of vegetation growth vigor. For example in temperate and arctic climates, anthrax epizootics are associated with hot-dry summers preceded by heavy spring rain (Blackburn and Goodin, 2013). Monitoring of annual trajectories of vegetation indices has revealed that early green-up in the spring in contrast to later and less intense spring green-up signals the occurrence of anthrax epizootic in the approaching summer.
We observed a statistically significant positive association between the number of anthrax outbreaks, or the total number of months anthrax was during the study period and the nitrogen, organic carbon content and cation exchange capacity of the soil. These results confirm previous findings that the geographical localization of anthrax outbreaks are associated with specific soil factors, specifically organic matter content (Hugh-Jones and Blackburn, 2009). Soil cation exchange capacity, CEC, is a measure of the soil’s storage capacity for nutrients and is estimated from the sum of exchangeable major cations (Ca2+, Mg2+, K+, Na+) expressed in centimoles of positive charge per kilogram of soil [cmol(+)/kg]. Soil organic matter and clay content correlate with measures of soil cation exchange capacity, CEC (Peverill et al., 1999; Liang et al., 2006), because cations are attracted to the negatively charged sites in clay minerals and organic matter. Soils with high organic carbon content or high CEC content contribute to greater soil microbial abundance and diversity (Stotzky, 1966; Drenovsky et al., 2004; Docherty et al., 2015). Although pH is known to influence B. anthracis persistence, we did not find any influence perhaps because nearly all focal wildlife areas had pH > 6.1. Locations with alkaline pH (≥6.1), high soil moisture, and organic matter are conducive for the persistence and proliferation of B. anthracis spores, through repeated cycles of spore germination, vegetative cell outgrowth, and re-sporulation which can cause an overall increase in spore numbers and probability of an anthrax outbreak (West et al., 1985). Further support comes from a study in Badin district of Pakistan, which found a positive correlation between anthrax prevalence in soil with percent organic matter and calcium contents of soil with prevalence of B. anthracis (Mari et al., 2017). Similarly, a study in Minnesota indicate that soil calcium and magnesium concentrations, soil pH, and sand content are the most important properties for predicting soil suitability for B. anthracis (Nath and Dere, 2016). Although we found a spatial association of anthrax outbreaks with edaphic factors, we cannot entirely rule out the influence of non-edaphic spatial effects.
Empirical studies on anthrax outbreaks and their association with population dynamics of soil microbiota, plant diversity, and vegetation growth with climate variability and edaphic factors are urgently needed. This will enhance our understanding of the environmental ecology of Bacillus anthracis and will provide a more accurate and refined variables for the prediction of anthrax outbreaks in wildlife areas.
Our findings support previous research indicating that incidences of anthrax outbreaks are positively correlated with indices of climate variability. These have implications for re-emergence of anthrax under projected current climate change scenarios where climatic extremes in precipitation are predicted for the East African region including Kenya (Case, 2006; CDKN, 2014). Our findings also suggest soil factors can be used for spatial disease risk assessment across wildlife areas in Kenya to guide strategic anthrax surveillance and aid with timely vaccination of endangered species that are vulnerable to anthrax and susceptible to local extinction.
Data Availability Statement
The original contributions presented in the study are included in the article/Supplementary Material, further inquiries can be directed to the corresponding author/s.
Ethics Statement
Ethical review and approval was not required for the animal study because we did not handle animals but used data collected during anthrax surveillance conducted by Kenya Wildlife Service, a government parastatal with the mandate to manage and protect wildlife.
Author Contributions
VO and PC conceived the idea. VO, DN, and EK compiled the anthrax outbreak data. VAO, OL, and PC assembled the soil and NDVI data. All authors contributed equally in drafting, editing, and analysis of the manuscript.
Funding
Data generated was funded by the Kenya Widlife Service.
Conflict of Interest
The authors declare that the research was conducted in the absence of any commercial or financial relationships that could be construed as a potential conflict of interest.
Publisher’s Note
All claims expressed in this article are solely those of the authors and do not necessarily represent those of their affiliated organizations, or those of the publisher, the editors and the reviewers. Any product that may be evaluated in this article, or claim that may be made by its manufacturer, is not guaranteed or endorsed by the publisher.
Acknowledgments
We thank Mary Kageni for processing NDVI and soil data and KWS veterinarians for their reports on anthrax investigation and surveillance which formed the basis of these analyses.
Supplementary Material
The Supplementary Material for this article can be found online at: https://www.frontiersin.org/articles/10.3389/fevo.2021.643334/full#supplementary-material
Footnotes
- ^ http://ivfl-info.boku.ac.at
- ^ https://www.isric.org/projects/world-inventory-soil-emission-potentials-wise
- ^ https://data.isric.org/geonetwork/srv/eng/catalog.search;jsessionid=A3C1451B0F8C364D00CCF9A61ECDC69A#/metadata/4648929a-8031-49cc-9d56-9f3aeff2f8d9
References
Aminu, O. R., Lembo, T., Zadoks, R. N., Biek, R., Lewis, S., Kiwelu, I., et al. (2020). Practical and effective diagnosis of animal anthrax in endemic low-resource settings. PLoS Negl. Trop.Dis. 14:e0008655. doi: 10.1371/journal.pntd.0008655
Anderson, O. R. (2000). Abundance of terrestrial Gymnamoebae at a Northeastern U. S. site: a four-year study, including the El Niiio winter of 1997-1998. J. Eukaryot. Microbiol. 47, 148–155. doi: 10.1111/j.1550-7408.2000.tb00024.x
Anttila, J., Kaitala, V., Laakso, J., and Ruokolainen, L. (2015). Environmental variation generates environmental opportunist pathogen outbreaks. PLoS One 10:e0145511. doi: 10.1371/journal.pone.0145511
Avery, S. T., and Tebbs, E. J. (2018). Lake Turkana, major Omo River developments, associated hydrological cycle change and consequent lake physical and ecological change. J. Great Lakes Res. 44, 1164–1182.
Barro, A. S., Fegan, M., Moloney, B., Porter, K., Muller, J., Warner, S., et al. (2016). Redefining the Australian anthrax belt: modeling the ecological niche and predicting the geographic distribution of Bacillus anthracis. PLoS Negl. Trop. Dis. 10:e0004689. doi: 10.1371/journal.pntd.0004689
Barton, K., and Barton, M. K. (2015). Package ‘mumin’. Version, 1, 18. Vienna: R Foundation for Statistical Computing.
Blackburn, J. K., and Goodin, D. G. (2013). Differentiation of springtime vegetation indices associated with summer anthrax epizootics in west Texas, USA, deer. J. Wildl. Dis. 49, 699–703. doi: 10.7589/2012-10-253
Bonkowski, M., and Clarholm, M. (2012). Stimulation of plant growth through interactions of bacteria and protozoa: testing the auxiliary microbial loop hypothesis. Acta Protozool. 51, 237–247.
Boutton, T. W., Tieszen, L. L., and Imbamba, S. K. (1988). Biomass dynamics of grassland vegetation in Kenya. Afr. J. Ecol. 25, 89–101.
Brooks, M. E., Kristensen, K., van Benthem, K. J., Magnusson, A., Berg, C. W., Nielsen, A., et al. (2017). glmmTMB balances speed and flexibility among packages for zero-inflated generalized linear mixed modeling. R J. 9, 378–400.
Case, M. (2006). Climate Change Impacts on East Africa; A Review of the Scientific Literature. Gland: WWF.
Chen, W.-J., Lai, S.-J., Yang, Y., Liu, K., Li, X.-L., Yao, H.-W., et al. (2016). Mapping the distribution of anthrax in mainland China, 2005–2013. PLoS Negl. Trop. Dis. 10:e0004637. doi: 10.1371/journal.pntd.0004637
Chikerema, S. M., Murwira, A., Matope, G., and Pfukenyi, D. M. (2013). Spatial modelling of Bacillus anthracis ecological niche in Zimbabwe. Prev. Vet. Med. 111, 25–30. doi: 10.1016/j.prevetmed.2013.04.006
Chira, R. M., and Kinyamario, J. (2009). Growth response of woody species to elephant foraging in Mwea National Reserve, Kenya. Afr. J. Ecol. 47, 598–605.
Clarholm, M. (1981). Protozoan grazing of bacteria in soil-impact and importance. Microbial Ecol. 7, 343–350. doi: 10.1007/BF02341429
Clegg, S. B., Turnbull, P. C. B., Foggin, C. M., and Lindeque, P. M. (2007). Massive outbreak of anthrax in wildlife in the Malilangwe Wildlife Reserve, Zimbabwe. Vet. Rec. 160, 113–118. doi: 10.1136/vr.160.4.113
Coe, M. (1978). The decomposition of elephant carcases in the Tsavo (East) National Park, Kenya. J. Arid Environ. 1, 71–86.
Creel, S., Creel, N. M., Mtambo, M. M. A., Batamuzi, E. K., Matovelo, J. A., and Cooper, J. E. (1995). The effects of anthrax on endangered African wild dogs (Lycaon pictus). J. Zool. 235, 199–209.
Dagnachew, M., Kebede, A., Moges, A., and Abebe, A. (2020). Effects of climate variability on normalized difference vegetation index (NDVI) in the Gojeb River Catchment, Omo-Gibe Basin, Ethiopia. Adv. Meteorol. 2020:8263246.
Deshmukh, I. (1986). Primary production of a grassland in Nairobi National Park, Kenya. J. Appl. Ecol. 23, 115–123. doi: 10.1111/gcb.14904
Dey, R., Hoffman, P. S., and Glomski, I. J. (2012). Germination and amplification of anthrax spores by soil-dwelling amoebas. Appl. Environ. Microbiol. 78, 8075–8081. doi: 10.1128/AEM.02034-12
Docherty, K. M., Borton, H. M., Espinosa, N., Gebhardt, M., Gil-Loaiza, J., Gutknecht, J. L., et al. (2015). Key edaphic properties largely explain temporal and geographic variation in soil microbial communities across four biomes. PLoS One 10:e0135352. doi: 10.1371/journal.pone.0135352
Dragon, D. C., and Rennie, R. P. (1995). The ecology of anthrax spores: tough but not invincible. Can. Vet. J. 36, 295–301.
Drenovsky, R., Vo, D., Graham, K., and Scow, K. (2004). Soil water content and organic carbon availability are major determinants of soil microbial community composition. Microbial Ecol. 48, 424–430. doi: 10.1007/s00248-003-1063-2
Duparc, A., Garel, M., Marchand, P., Dubray, D., Maillard, D., and Loison, A. (2020). Through the taste buds of a large herbivore: foodscape modeling contributes to an understanding of forage selection processes. Oikos 129, 170–183.
ESRI (2012). ArcGIS Desktop: Release 10.1 Environmental Systems Research Institute. Redlands, CA: ESRI.
Fairbanks, D. H. K., and McGwire, K. C. (2004). Patterns of floristic richness in vegetation communities of California: regional scale analysis with multi-temporal NDVI. Glob. Ecol. Biogeogr. 13, 221–235.
Fernández, L. D. (2015). Source–sink dynamics shapes the spatial distribution of soil protists in an arid shrubland of northern Chile. J. Arid Environ. 113, 121–125.
Foissner, W. (1987). Soil protozoa: fundamental problems, ecological significance, adaptions in ciliates and testaceans, bioindicators, and guide to the literature. Prog. Protozool. 2, 69–212.
Gainer, R. S. (1987). Epizootiology of anthrax and Nyasa wildebeest in the Selous Game Reserve, Tanzania. J. Wildl. Dis. 23, 175–178. doi: 10.7589/0090-3558-23.1.175
Ganz, H. H., Turner, W. C., Brodie, E. L., Kusters, M., Shi, Y., Sibanda, H., et al. (2014). Interactions between Bacillus anthracis and plants may promote anthrax transmission. PLoS Negl. Trop. Dis. 8:e2903. doi: 10.1371/journal.pntd.0002903
Getonto, J. A. (2018). Impacts of Geothermal Power Station on Avifauna at Hell’s Gate National Park, Nakuru County, Kenya. Nairobi: Kenyatta University.
Gould, W. (2000). Remote sensing of vegetation, plant species richness, and regional biodiversity hotspots. Ecol. Appl. 10, 1861–1870.
Hampson, K., Lembo, T., Bessell, P., Auty, H., Packer, C., Halliday, J., et al. (2011). Predictability of anthrax infection in the Serengeti, Tanzania. J. Appl. Ecol. 48, 1333–1344. doi: 10.1111/j.1365-2664.2011.02030.x
Hawinkel, P., Thiery, W., Lhermitte, S., Swinnen, E., Verbist, B., Van Orshoven, J., et al. (2016). Vegetation response to precipitation variability in East Africa controlled by biogeographical factors. J. Geophys. Res. Biogeosci. 121, 2422–2444.
Hoffmann, C., Zimmermann, F., Biek, R., Kuehl, H., Nowak, K., Mundry, R., et al. (2017). Persistent anthrax as a major driver of wildlife mortality in a tropical rainforest. Nature 548, 82–86. doi: 10.1038/nature23309
Hoffmaster, A. R., Ravel, J., Rasko, D. A., Chapman, G. D., Chute, M. D., Marston, C. K., et al. (2004). Identification of anthrax toxin genes in a Bacillus cereus associated with an illness resembling inhalation anthrax. Proc. Natl. Acad. Sci. 101, 8449–8454. doi: 10.1073/pnas.0402414101
Hugh-Jones, M., and Blackburn, J. K. (2009). The ecology of Bacillus anthracis. Mol. Aspects Med. 30, 356–367.
Jousset, A. (2012). Ecological and evolutive implications of bacterial defences against predators. Environ. Microbiol. 14, 1830–1843. doi: 10.1111/j.1462-2920.2011.02627.x
Joyner, T. A., Lukhnova, L., Pazilov, Y., Temiralyeva, G., Hugh-Jones, M. E., Aikimbayev, A., et al. (2010). Modeling the potential distribution of Bacillus anthracis under multiple climate change scenarios for Kazakhstan. PLoS One 5:e9596. doi: 10.1371/journal.pone.0009596
Kaitho, T., Ndeereh, D., and Ngoru, B. (2013). An outbreak of anthrax in endangered Rothschild’s giraffes in Mwea National Reserve, Kenya. Vet. Med. 4, 45–48.
Kalisa, W., Igbawua, T., Henchiri, M., Ali, S., Zhang, S., Bai, Y., et al. (2019). Assessment of climate impact on vegetation dynamics over East Africa from 1982 to 2015. Sci. Rep. 9:16865. doi: 10.1038/s41598-019-53150-0
Koch, R. (1937). The etiology of anthrax based on the ontogeny of anthrax Bacillus. Med. Class. 2, 787–8821.
Kracalik, I. T., Kenu, E., Ayamdooh, E. N., Allegye-Cudjoe, E., Polkuu, P. N., Frimpong, J. A., et al. (2017). Modeling the environmental suitability of anthrax in Ghana and estimating populations at risk: implications for vaccination and control. PLoS Negl. Trop. Dis. 11:e0005885. doi: 10.1371/journal.pntd.0005885
Kyale, D. M., Ngene, S., and Maingi, J. (2011). Biophysical and human factors determine the distribution of poached elephants in Tsavo East National Park, Kenya. Pachyderm 49, 48–60.
Leendertz, F. H., Lankester, F., Guislain, P., Neel, C., Drori, O., Dupain, J., et al. (2006a). Anthrax in Western and Central African great apes. Am. J. Primatol. 68, 928–933. doi: 10.1002/ajp.20298
Leendertz, F. H., Yumlu, S., Pauli, G., Boesch, C., Couacy-Hymann, E., Vigilant, L., et al. (2006b). A new Bacillus anthracis found in wild chimpanzees and a gorilla from West and Central Africa. PLoS Pathog. 2:e8. doi: 10.1371/journal.ppat.0020008
Liang, B., Lehmann, J., Solomon, D., Kinyangi, J., Grossman, J., O’Neill, B., et al. (2006). Black carbon increases cation exchange capacity in Soils. Soil Sci. Soc. Am. J. 70, 1719–1730.
Lindeque, P. M. (1998). “Anthrax in wild cheetahs in the Etosha National Park,” in Proceedings of the ARC-Ondestepoort, OIE International Congress with WHO-Cosponsorship on Anthrax, Brucellosis, CBPP. Clostridial and Mycobacterial diseases, Kruger National Park.
Lovart, S., and Lucherin, M. (1992). Larger mammals as a tourist attraction: factors influencing their visibility in the Hell’s Gate National Park, Kenya. Hystrix 4, 51–58.
Lüdecke, D. (2018). ggeffects: tidy data frames of marginal effects from regression models. J. Open Source Softw. 3:772.
Lüdecke, D., Ben-Shachar, M. S., Patil, I., Waggoner, P., and Makowski, D. (2021). Performance: an R package for assessment, comparison and testing of statistical models. J. Open Sour. Softw. 6:3139.
Maksimović, Z., Cornwell, M., Semren, O., and Rifatbegović, M. (2017). The apparent role of climate change in a recent anthrax outbreak in cattle. Rev. Sci. Tech. Off. Int. Epiz. 36, 959–963. doi: 10.20506/rst.36.3.2727
Mari, A. S., Kamboh, A. A., Bhutto, K.-U.-R. I, Sahito, A., and Abro, S. H. (2017). Prevalence of Bacillus anthracis spores in soil of district Badin. J. Anim. Health Prod. 5, 79–84.
Matz, C., and Kjelleberg, S. (2005). Off the hook–how bacteria survive protozoan grazing. Trends Microbiol. 13, 302–307. doi: 10.1016/j.tim.2005.05.009
Mbaluka, J. K., and Brown, F. H. (2016). Vegetation of the Koobi fora region northeast of Lake Turkana, Marsabit County, northern Kenya. J. East Afr. Nat. Hist. 105, 21–50.
M’Fadyean, J. (1903). A peculiar staining reaction of the blood of animals dead of anthrax. J. Comp. Pathol. Ther. 16, 35–IN31.
Mollel, C. L. (1977). A probable case of anthrax in a Serengeti lioness. East Afr. Wildl. J. 15:331.
Munang’andu, H. M., Banda, F., Siamudaala, V. M., Munyeme, M., Kasanga, C. J., and Hamududu, B. (2012). The effect of seasonal variation on anthrax epidemiology in the upper Zambezi floodplain of western Zambia. J. Vet. Sci. 13:293. doi: 10.4142/jvs.2012.13.3.293
Muoria, P. K., Muruthi, P., Kariuki, W. K., Hassan, B. A., Mijele, D., and Oguge, N. O. (2007). Anthrax outbreak among Grevy’s zebra (Equus grevyi) in Samburu, Kenya. Afr. J. Ecol. 45, 483–489.
Muturi, M., Gachohi, J., Mwatondo, A., Lekolool, I., Gakuya, F., Bett, A., et al. (2018). Recurrent anthrax outbreaks in humans, livestock, and wildlife in the same locality, Kenya, 2014–2017. Am. J. Trop. Med. Hyg. 99, 833–839. doi: 10.4269/ajtmh.18-0224
Nath, S., and Dere, A. (2016). Soil geochemical parameters influencing the spatial distribution of anthrax in Northwest Minnesota, USA. Appl. Geochem. 74, 144–156.
New, D., Elkin, B., Armstrong, T., and Epp, T. (2017). Anthrax in the Mackenzie wood bison (Bison bison athabascae) population: 2012 anthrax outbreak and historical exposure in nonoutbreak years. J. Wildl. Dis. 53, 769–780. doi: 10.7589/2016-11-257
Ng’weno, C. C., Mwasi, S. M., and Kairu, J. K. (2010). Distribution, density and impact of invasive plants in Lake Nakuru National Park, Kenya. Afr. J. Ecol. 48, 905–913.
Norris, M. H., and Blackburn, J. K. (2019). Linking geospatial and laboratory sciences to define mechanisms behind landscape level drivers of anthrax outbreaks. Int. J. Environ. Res. Public Health 16:3747. doi: 10.3390/ijerph16193747
Ogutu, J. O., Owen-Smith, N., Piepho, H.-P., Kuloba, B., and Edebe, J. (2012). Dynamics of ungulates in relation to climatic and land use changes in an insularized African savanna ecosystem. Biodivers. Conserv. 21, 1033–1053.
Ogutu, J. O., Owen-Smith, N., Piepho, H.-P., Said, M. Y., Kifugo, S. C., Reid, R. S., et al. (2013). Changing wildlife populations in Nairobi National Park and adjoining Athi-Kaputiei Plains: collapse of the migratory wildebeest. Open Conserv. Biol. J. 7, 11–26.
Oldeland, J., Wesuls, D., Rocchini, D., Schmidt, M., and Jürgens, N. (2010). Does using species abundance data improve estimates of species diversity from remotely sensed spectral heterogeneity? Ecol. Indic. 10, 390–396.
Pau, S., Gillespie, T. W., and Wolkovich, E. M. (2012). Dissecting NDVI-species richness relationships in Hawaiian dry forests. J. Biogeogr. 39, 1678–1686.
Peters, W., Hebblewhite, M., Mysterud, A., Spitz, D., Focardi, S., Urbano, F., et al. (2017). Migration in geographic and ecological space by a large herbivore. Ecol. Monogr. 87, 297–320.
Peverill, K., Sparrow, L., and Reuter, D. J. (1999). Soil Analysis: An Interpretation Manual. Clayton, VIC: CSIRO publishing.
Revich, B., Tokarevich, N., and Parkinson, A. J. (2012). Climate change and zoonotic infections in the Russian Arctic. Int. J. Circumpolar Health 71:18792. doi: 10.3402/ijch.v71i0.18792
Ritz, K., and Young, I. (2011). The Architecture and Biology of Soils: Life in Inner Space. Wallingford: Cabi.
Rocchini, D., Balkenhol, N., Carter, G. A., Foody, G. M., Gillespie, T. W., He, K. S., et al. (2010). Remotely sensed spectral heterogeneity as a proxy of species diversity: recent advances and open challenges. Ecol. Inform. 5, 318–329.
Rodriguez-Zaragoza, S., Mayzlish, E., and Steinberger, Y. (2005). Vertical distribution of the free-Living amoeba population in soil under desert shrubs in the Negev Desert, Israel. Appl. Environ. Microbiol. 71, 2053–2060. doi: 10.1128/AEM.71.4.2053-2060.2005
Romero-Alvarez, D., Peterson, A. T., Salzer, J. S., Pittiglio, C., Shadomy, S., Traxler, R., et al. (2020). Potential distributions of Bacillus anthracis and Bacillus cereus biovar anthracis causing anthrax in Africa. PLoS Negl. Trop. Dis. 14:e0008131. doi: 10.1371/journal.pntd.0008131
Rume, F. I., Karim, M. R., Biswas, P. K., Yasmin, M., and Ahsan, C. R. (2020). Climate change and its influence on occurrence and distribution of Anthrax in Bangladesh. Int. J. Infect. Dis. 101:411.
Rutherford, P. M., and Juma, N. G. (1992). Influence of texture on habitable pore space and bacterial-protozoan populations in soil. Biol. Fertil. Soils 12, 221–227.
Saile, E., and Koehler, T. M. (2006). Bacillus anthracis multiplication, persistence, and genetic exchange in the rhizosphere of grass plants. Appl. Environ. Microbiol. 72, 3168–3174. doi: 10.1128/AEM.72.5.3168-3174.2006
Salb, A., Stephen, C., Ribble, C., and Elkin, B. (2014). Descriptive epidemiology of detected anthrax outbreaks in wild wood bison (Bison bison athabascae) in northern Canada, 1962-2008. J. Wildl. Dis. 50, 459–468. doi: 10.7589/2013-04-095
Schild, A. L., Sallis, E. S. V., Soares, M. P., Ladeira, S. R. L., Schramm, R., Priebe, A. P., et al. (2006). Anthrax in cattle in southern Brazil: 1978-2006. Pesqui. Vet. Bras. 26, 243–248.
Shen, J., and Gao, S. (2008). A solution to separation and multicollinearity in multiple logistic regression. J. Data Sci. 6, 515–531.
Smith, K. L., DeVos, V., Bryden, H., Price, L. B., Hugh-Jones, M., and Keim, P. (2000). Bacillus anthracis diversity in Kruger National Park. J. Clin. Microbiol. 38, 3780–3784. doi: 10.1128/JCM.38.10.3780-3784.2000
Steenkamp, P. J., Van Heerden, H., and Van Schalkwyk, O. L. (2018). Ecological suitability modeling for anthrax in the Kruger National Park, South Africa. PLoS One 13:e0191704. doi: 10.1371/journal.pone.0191704
Stotzky, G. (1966). Influence of clay minerals on microorganisms: III. Effect of particle size, cation exchange capacity, and surface area on bacteria. Can. J. Microbiol. 12, 1235–1246. doi: 10.1139/m66-165
Turner, W. C., Kausrud, K. L., Krishnappa, Y. S., Cromsigt, J. P. G. M., Ganz, H. H., Mapaure, I., et al. (2014). Fatal attraction: vegetation responses to nutrient inputs attract herbivores to infectious anthrax carcass sites. Proc. R. Soc. B Biol. Sci. 281:20141785. doi: 10.1098/rspb.2014.1785
Wato, Y. A., Wahungu, G. M., and Okello, M. M. (2006). Correlates of wildlife snaring patterns in Tsavo West National Park, Kenya. Biol. Conserv. 132, 500–509.
Waweru, F., and Githaiga, A. (2014). Resource partitioning by the Black Rhinoceros (Diceros Bicornis), Elephant (Loxodonta Africana) and the Giraffe (Giraffa Camelopardalis) in Tsavo West National Park, Kenya. Int. J. Eng. Res. Technol. 3, 2530–2544.
West, A. W., Burges, H. D., Dixon, T. J., and Wyborn, C. H. (1985). Survival of Bacillus thuringiensis and Bacillus cereus spore inocula in soil: effects of pH, moisture, nutrient availability and indigenous micro-organisms. Soil Biol. Biochem. 17, 657–665.
Keywords: anthrax, wildlife, NDVI, Kenya, spatio-temporal
Citation: Obanda V, Otieno VA, Kingori EM, Ndeereh D, Lwande OW and Chiyo PI (2021) Identifying Edaphic Factors and Normalized Difference Vegetation Index Metrics Driving Wildlife Mortality From Anthrax in Kenya’s Wildlife Areas. Front. Ecol. Evol. 9:643334. doi: 10.3389/fevo.2021.643334
Received: 17 December 2020; Accepted: 29 November 2021;
Published: 16 December 2021.
Edited by:
Alex Perkins, University of Notre Dame, United StatesReviewed by:
Henriette Van Heerden, University of Pretoria, South AfricaYen-Hua Huang, University of Wisconsin–Madison, United States
Copyright © 2021 Obanda, Otieno, Kingori, Ndeereh, Lwande and Chiyo. This is an open-access article distributed under the terms of the Creative Commons Attribution License (CC BY). The use, distribution or reproduction in other forums is permitted, provided the original author(s) and the copyright owner(s) are credited and that the original publication in this journal is cited, in accordance with accepted academic practice. No use, distribution or reproduction is permitted which does not comply with these terms.
*Correspondence: Patrick I. Chiyo, cGNoaXlvQGdtYWlsLmNvbQ==