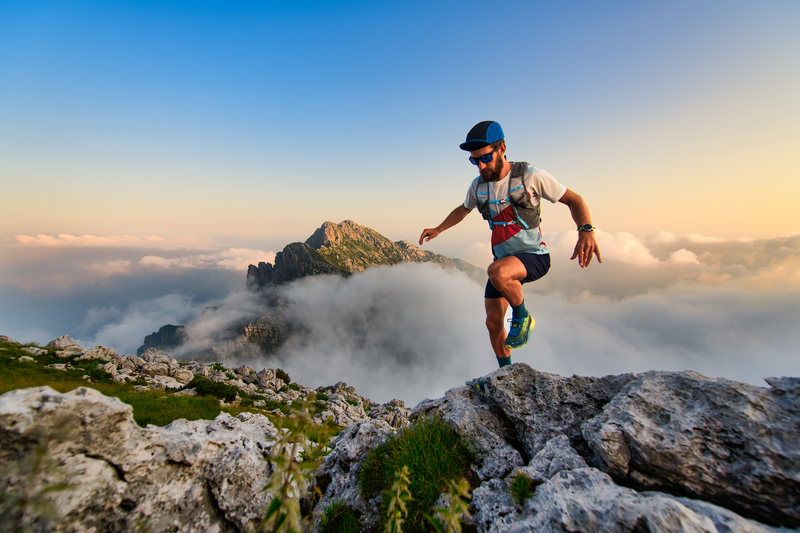
94% of researchers rate our articles as excellent or good
Learn more about the work of our research integrity team to safeguard the quality of each article we publish.
Find out more
ORIGINAL RESEARCH article
Front. Ecol. Evol. , 16 April 2021
Sec. Conservation and Restoration Ecology
Volume 9 - 2021 | https://doi.org/10.3389/fevo.2021.643285
This article is part of the Research Topic Impacts of Habitat Transformation on Species, Biodiversity and Ecosystems in Asia View all 13 articles
While the negative impacts of dam construction on downstream river stretches and riparian forests are well studied, the status of wildlife presence and persistence in upstream reservoir deltas is virtually unknown. We investigated the drivers of terrestrial mammal occupancy and persistence along riparian forests of Koyna reservoir in western India 55 years after its construction. We adopted a catchment-wide field design grounded in the river continuum concept and sampled different stream orders within the reservoir. Camera traps, nested in an occupancy modeling framework, were deployed across 72 riparian sites and replicated for four seasons across all stream types. We recorded a total of nineteen species of terrestrial mammals during the study period. Multi-season occupancy models revealed three key patterns of mammal persistence: (a) ungulates were more frequently photo-captured in riparian forests; gaur and wild pig had the highest proportions of the total sampled area (0.84 ± 0.12 SE; 0.77 ± 0.07 SE, respectively); (b) small-sized ungulates were more vulnerable to local extinction than large-bodied ungulates; extinction probability was highest for barking deer (0.59 ± 0.07) and lowest for sambar (0.15 ± 0.07); and (c) distance from stream played major roles in determining mammal detection. Riparian forests are fundamentally important to ecosystem functioning and biodiversity conservation, and using the data from this study, managers can plan to sustain high mammal persistence along riparian forests at Koyna reservoir or similar Indian reserves. Further, our robust sampling approach, grounded in the terrestrial-riverine continuum concept, can be applied globally to understand species assemblages, aiding in multi-landscape and wildlife management planning.
The river continuum concept was the first unified hypothesis proposing that rivers and associated watersheds should be viewed as a continuum to understand the complete structure and functioning of a river (Vannote et al., 1980). Like river systems, riparian forests form their own continuum running along rivers and streams, from headwaters to perennial rivers, and hence riparian vegetation is also likely to conform to the river continuum concept. Riparian forests as ecotones protect riverbanks from erosion resulting in bank stability (Pinay et al., 2018), drive organic matter and nutrients into streams (Vannote et al., 1980), moderate temperature extremes in river environments (Dugdale et al., 2018), and are predicted to function as hotspots for climate change adaptation (Seavy et al., 2009; Capon et al., 2013). Hence, riparian forests are key constituents of terrestrial-aquatic continua and reflect the functional status of an entire catchment. Large animal ecology in riparian forests has been long studied and was probably first highlighted in mainstream conservation science by the pioneering studies of Naiman (1988); Pastor et al. (1988), and Naiman and Rogers (1997). These studies summarized how large ungulates influence riparian system dynamics primarily by their foraging behavior. There have been few recent studies highlighting the importance of riparian forests for forest mammals, but the riverine-continuum concept is slowly gaining traction across various ecosystems, especially with important works of Santos et al. (2011) and Zimbres et al. (2018). In India, there have been frequent general discussions about dam effects on biodiversity, but detailed empirical studies on the dynamics and distribution of forest mammals within riparian forests remain virtually non-existent. In regards to riparian forests, studies have mainly focused on riparian obligate species like otters (Umapathy and Durairaj, 1995; Hussain and Choudhury, 1997; Anoop and Hussain, 2006a,b; Perinchery et al., 2011; Prakash et al., 2012; Raha and Hussain, 2016), or certain large mammals in floodplains/wetland-dependent species, such as Asiatic buffalo and rhinocerous (Chatterjee and Bhattacharyya, 2021). Chatterjee and Bhattacharyya (2021) found that even though wetland-dependent mammals have been studied, there remains a large knowledge gap in regards to these species ecology and conservation.
A novel avenue for riparian ecology research opened up very recently with studies of Datry et al. (2014, 2016, 2017a, 2017b), which stressed the ecology of dry and intermittent rivers, as perennial river systems have received the majority of research attention historically. Following the research on fluvial dynamics of perennial and temporary rivers, Sánchez-Montoya et al. (2016) studied dry streams as corridors for large mammals using an innovative animal footprint method. These studies have all contributed to the development of the terrestrial riverine continuum concept, but they have also all been conducted largely in free-flowing rivers and associated riparian forests. Within altered habitats, especially in upstream hydropower reservoirs, research on forest mammals in riparian forests is still lacking. These altered habitats are interesting especially because the riparian forests experience high levels of flooding and drought owing to uneven rainfall patterns. Unlike natural watersheds, the water level in reservoirs is operated by dam authorities which alters the normal hydrological cycle of the river (Alho, 2011). Species occupancy patterns in an environment of such dynamic water level fluctuations is key to provide insights into how species persist within these reservoirs. With this background in the river continuum framework, we aimed to identify the drivers of mammal assembly and persistence in a human-altered watershed, a dammed river which now forms the Koyna Wildlife Sanctuary in the northern Western Ghats.
Study Area: The Koyna Hydroelectric Project is the largest completed hydropower station in India with a total capacity of 1960 MW. It is comprised of four dams, the largest of which, the Koyna Dam, was completed in 1963, forming the Shivsagar Reservoir (Bokil, 1999). The reservoir is now protected as Koyna Wildlife Sanctuary (hereafter referred to as “Koyna”). Koyna forms an important corridor between Mahabaleshwar-Panchgani Ecologically Sensitive Zone in the north and Chandoli National Park in the south; Koyna and the Chandoli National Park together form the Sahyadri Tiger Reserve. With seven land cover types (Jelil et al., 2020; Figure 1), Koyna covers 423.55 km2 and the vegetation in the sanctuary is classified as southern tropical evergreen forest and southern moist deciduous forest (Champion and Seth, 1968). Red clay is the main soil type. The mean annual rainfall in Koyna is ∼ 5,000 mm, which falls predominantly from June–September (Joglekar et al., 2015). Koyna was declared a wildlife sanctuary in 1985, and private forests owned by villagers before resettlement now persist as grasslands, scrub and moist deciduous forests (Joglekar et al., 2015). Joglekar et al. (2015) reported that the relative inaccessibility and undulating terrain supports some of the few remaining undisturbed tall evergreen forests in the northern Western Ghats, and hosts large mammals such as the common leopard (Panther pardus), dhole (Cuon alpinus), sloth bear (Melursus ursinus), Indian gaur (Bos gaurus), and sambar (Rusa unicolor). The last confirmed record of tiger (Panthera tigris) from Chandoli National Park was in 2018 (Jelil et al., 2020), but the species has not been recorded in Koyna since 2007.
Figure 1. Map of study area detailing the field survey design and the camera trap locations. In each of three sections of the river we sampled four kinds of stream types: perennial, intermittent, ephemeral and headwater streams.
Field Study Design: We used stream types as sampling strata with the river stretch divided into three sections (Table 1). In each section, we assessed riparian forests associated with a perennial order, two intermittent orders, four ephemeral orders and eight headwater orders (Figures 1, 2). We sampled four locations in both the perennial and intermittent order and eight locations in both ephemeral and headwaters orders. In total, we sampled 24 locations in each of the three sections resulting in 72 total sampled locations. The riparian buffer was set at 1 km in either direction of the stream edge in the perennial habitat, at 500 m in the intermittent, 200 m in ephemeral and 100 m in headwater streams. The study was carried out from April 2018 to March 2020 encompassing four seasons (two summer and winter seasons, i.e., summer 2018, winter 2018, summer 2019, and winter 2019). Summer season data collection was conducted from April to July and winter data collection from November to February. This amounted to c. 480 days of survey effort in the 2 year period.
Table 1. Description of riparian habitat complexes/habitat types selected for the study and the rationale/basis for selecting the habitat types.
Camera Trapping Surveys: The camera traps were deployed up to 1 km from the waterway for the perennial stream-type, up to 500 m for the intermittent stream-type, up to 200 m for the ephemeral stream type, and up to 100 m for headwater stream type. Mean values for the distance of the camera-trap site to the waterway shown in Table 1. At each of the 72 sites, a single Cuddeback white flash (C1 model) camera trap was deployed by affixing it at a height of c. 60–70 cm to suitable trees. These were set to take consecutive images (set 5 s apart) when triggered. Cameras were checked regularly in field after deployment to limit the missing survey replicates. The mean of the trapping days in summer 2018 was 24.4 (±0.62 SE) days, winter 2018 was 39.89 (±1.75 SE), summer 2019 was 40.97 (±0.89 SE) days and winter 2019 was 35.05 (±1.73 SE) days. The trapping effort in summer 2018 was lower because initially we had fewer number of camera traps available for this study, and hence, we had to cover the 72 sites in two rounds. However, during the next sessions, more camera traps were at our disposal and all 72 cameras could be deployed at once. In any case, the different number of camera traps days is likely to increase confidence intervals and thereby means that any differences in effects due to variable effort are conservative.
Riparian Habitat Assessment: To test habitat factors influencing species occupancy, circular vegetation plots were established in all the 72 riparian buffers for collection of fine-scale habitat data. Keeping the camera trap location as the center, the number of trees and fallen logs were assessed within 10 m radius plots covering 314 m2. Percent canopy cover, elevation, the adjacent stream type and the distance to stream edge were recorded from the center of the plot. We set the radius of the plot to be short to conserve time in the field.
Data Analyses: Images retrieved from the camera traps were identified to species level excluding random captures of birds, and data were sorted into species-specific folders for all sites by season. Images were considered to be independent when they were at least 30 min apart (Linkie and Ridout, 2011; Rovero and Zimmerman, 2016; Allen et al., 2018, 2020).
Occupancy Modeling Framework: We constructed species-specific multi-season occupancy models (MacKenzie et al., 2002, 2006) using PRESENCE 12.6 (Hines, 2006). We modeled occupancy of species that had at least 20 independent captures across all four seasons. We arranged our camera trap data into 7 day occasions (weekly replicates) to record detection and non-detection to create detection history matrices for each site. A detection was coded as (1), non-detection was coded as (0) and a missing survey was coded as (.), which in our case would mean that a camera stopped working during the deployed time. We finally compiled our detection history matrices with four replicates for summer 2018, seven for winter 2018, summer 2019 and winter 2019. Hence, we had 25 replicates across all four seasons, i.e., we had 25 × 7 days = 175 occasions for each of the 72 sites covering all four seasons.
For all multi-season models, the model parameterization was fixed to initial occupancy, local colonization, extinction and detection. The parameters used in multi-season models were:
ψ: occupancy probability (probability that the area is occupied by the species)
pi: detection probability (probability of detection species in survey i, given the species is present)
γi: colonization probability (probability of unoccupied site being colonized between seasons i and i + 1)
εi: extinction probability (probability of occupied site going extinct between seasons i and i + 1)
Predictor Variables: The riparian habitat covariates were selected a priori because of their likely importance in driving wildlife occupancy along streams (Table 2). To minimize model overfitting, which often risks the inclusion of spurious variables (Burnham and Anderson, 2002), we tested for pair-wise correlations between covariates using Pearson’s correlation analyses. This was done using cor function and plotted using corrplot function in the corrplot 0.84 (Wei and Simko, 2017) package in R 4.0.0 (R Core Team, 2020). Correlation threshold was fixed at r ≥ 0.7 (Dornmann et al., 2013; Supplementary Code 1 and Supplementary Figure 1) and when correlation between two variables was higher than 0.7, we removed one of the two covariates. Since cameras were set at specific distances from stream edge at each of the stream types, we sensed a possible correlation of habitat type with distance to stream. However, since habitat type was a categorical variable it was not possible to test for correlation using the above method, since that only works with continuous variables. Regression models allow to test for this by using square root of the R2 value as a surrogate that can be treated similarly to correlation. This works for a regression model considering a continuous variable (in our case distance from stream edge) as dependent variable and a categorical variable (stream type) as independent variable. Again, the correlation threshold was fixed at 0.7 (Supplementary Code 2).
Simultaneously, we ran a principal component analysis (PCA) to test for multicollinearity in addition to the pair-wise correlation analysis. We ran the PCA using the prcomp function in the factoextra 1.0.7 package (Kassambara and Mundt, 2020) in R (Supplementary Code 3, Supplementary Figure 2, and Supplementary Table 1).
Model Selection: For model selection, χ2 goodness-of-fit test (MacKenzie and Bailey, 2004) using 999 parametric bootstraps was run to estimate overdispersion parameter (Burnham and Anderson, 2002). This was done keeping in mind the caveat described by Burnham and Anderson (2002) that estimating for every model would make the correct use of model selection criteria tricky, hence they recommend that the global model should be used as the basis for estimating a single variance inflation factor . We evaluated model fit with program PRESENCE by using the “assess model fit” function, while creating the design matrix of the global model. Finally, to account for overdispersion (where > 1) indicating a lack of fit, the model selection was done using quasi AIC (QAIC), and model parameters were adjusted by multiplying the standard errors by a factor of √ (Burnham and Anderson, 2002). The QAIC was computed using the following formula:
where,
log Like = log likelihood of the model
= dispersion parameter from the global model
k = number of parameters in the model
The estimates of occupancy (ψ), seasonal colonization (γ), local extinction (ε), and detection probability (p) were obtained through the null models of each species. Graphs were created using ggplot2 (Wickham, 2016) and ggpubr 0.4.0 (Kassambara, 2020) R packages.
Our camera trap efforts accounted for 10, 021 trap nights across all four seasons—1757 trap nights in summer 2018, 2872 in winter 2018, 2868 in summer 2019 and 2524 in winter 2019. We photo-captured 19 species of terrestrial mammals (Table 3 and Figure 3). Our camera capture threshold criterion of at least 20 independent captures was fulfilled by 10 species in summer 2018, 10 in winter 2018, six in summer 2019 and 10 in winter 2019. Only five species fulfilled this criterion across all four seasons and hence multi-season models were run for these five species—gaur (Bos gaurus), wild pig (Sus scrofa), sambar (Rusa unicolor), barking deer (Muntiacus muntjak), and porcupine (Hyxtrix indica).
Table 3. List of all species photo-captured during the study period from riparian forests of Koyna Wildlife Sanctuary.
Figure 3. Select camera trap images of highly ‘phot-captured’ mammals during the study. (A) ‘gaur’ Bos gaurus; (B) wild pig Sus scrofa; (C) sambar Rusa unicolor; (D) sloth bear Melursus ursinus; (E) barking deer Muntiacus ‘muntjac’; (F) leopard Panthera pardus; (G) porcupine Hystrix indica; (H) small Indian civet ‘Vivericula’ indica; (I) common palm civet Paradoxurus ‘hermaphrodites’.
Final Set of Predictor Variables: The pairwise correlation test showed that distance from stream edge and type of stream/habitat had high correlation (0.94) (Supplementary Code 2) and hence stream type was removed from the final variable set. Further, distance from stream, number of fallen logs, and percent understory cover were removed from the occupancy, colonization and extinction models because the test for multi-collinearity (PCA) demonstrated these covariates to have low contribution in the overall dataset. However, we retained distance from stream as a covariate to model species detection probabilities. The final list of covariates after both correlation and multi-collinearity analyses were used as occupancy, seasonal colonization, local extinction and detection probability covariates in the occupancy models (Table 4).
Model Selection: We detected evidence of overdispersion for two species viz., gaur ( = 2.83) and sambar ( = 2.05) (Table 4). No model overdispersion was detected for barking deer ( = 0.03), wild pig ( = 0.88) and porcupine ( = 0.85) (Supplementary Table 2). Top ranking models for each species (ΔQAIC ≤ 2) were considered which accounted for 83 and 95% of the QAIC model weight for sambar and gaur, respectively. For barking deer, porcupine and wild pig, top ranking models (ΔAIC ≤ 2) accounted for 67, 81, and 93% of AIC model weights, respectively.
Occupancy modeling results (occupancy, colonization, extinction and detection probability parameter estimates with standard error SE are reported within parentheses):
Porcupine (0.43 ± 0.01) had the highest detection probability followed by wild pig (0.37 ± 0.02), sambar (0.31 ± 0.01), barking deer (0.29 ± 0.02), and gaur (0.27 ± 0.03). Indian gaur (0.84 ± 0.12) had the highest proportion of occupied sites followed by wild pig (0.77 ± 0.07), porcupine (0.65 ± 0.07), sambar (0.49 ± 0.10), and barking deer (0.49 ± 0.08). Sambar (0.63 ± 0.10) had the highest probability to colonize unoccupied sites between seasons, followed by wild pig (0.62 ± 0.08), gaur (0.57 ± 0.15), porcupine (0.47 ± 0.06), and barking deer (0.24 ± 0.05). Barking deer (0.59 ± 0.07) had the highest probability to go extinct from a previously occupied site between seasons, followed by porcupine (0.43 ± 0.05), gaur (0.39 ± 0.11), wild pig (0.32 ± 0.04), and sambar (0.15 ± 0.07) (Figure 4).
Figure 4. Probability estimates of occupancy, colonization, extinction and detection of all the five species.
Predictors of species occupancy, detection, local colonization and extinction between seasons (β estimates with standard error SE are reported within parentheses):
Barking Deer: Barking deer occupancy was positively affected by elevation (0.09 ± 0.01) and number of trees (0.05 ± 0.02), and negatively by canopy cover (−0.06 ± 0.01). Its colonization probability was positively affected by canopy cover (0.03 ± 0.01), and negatively by elevation (−0.04 ± 0.01) and number of trees (−0.01 ± 0.02). Its extinction probability was negatively affected by elevation (−0.05 ± 0.01), canopy cover (−0.01 ± 0.01), and number of trees (−0.05 ± 0.02). Its detection was higher at sites near to streams (−0.01 ± 0.01) (Figures 5, 6 and Supplementary Tables 3–6).
Figure 5. Factors affecting species (A) occupancy, (B) colonization, and (C) extinction probabilities of common mammal species. The number of trees also showed statistically significant relationships to these probabilities, similar to those of canopy openness shown here (Supplementary Tables 4–6).
Porcupine: Porcupine occupancy was positively affected by canopy cover (0.01 ± 0.01), number of trees (0.03 ± 0.01), and negatively by elevation (−0.01 ± 0.01). Its colonization probability was positively affected by canopy cover (0.02 ± 0.01), number of trees (0.01 ± 0.01) and negatively by elevation (−0.03 ± 0.01). Its detection was higher closer to streams (−0.001 ± 0.001) and it was also affected by season. None of the covariates considered could explain porcupine extinction probability (Figures 5, 6 and Supplementary Tables 3–6).
Gaur: Gaur detection (−0.02 ± 0.01) were higher at sites near streams. Its detection was also affected by season. Its occupancy, colonization and extinction probabilities were not explained by any of the covariates considered in the occupancy models (Figure 6 and Supplementary Tables 3–6).
Sambar: Sambar occupancy, colonization and extinction probability were not influenced by any of covariates. However, sites near to streams (−0.001 ± 0.001) and season affected its detection probability (Figure 6 and Supplementary Tables 3–6).
Wild Pig: Wild pig extinction probability was positively affected by elevation (0.02 ± 0.01), and negatively by canopy cover (−0.02 ± 0.01), number of trees (−0.06 ± 0.08). Its detection was higher near streams (−0.01 ± 0.01), and was also affected by season. No factors could explain its occupancy and colonization (Figures 5, 6; Supplementary Tables 3–6).
Three key patterns emerge from our study. Firstly, ungulates were the most frequently photo-captured mammals in camera traps, with higher occupancy probability in riparian forests. Apart from ungulates, small mammals were also captured, however, large carnivores which included leopard Panthera pardus, dhole Cuon alpinus and sloth bear Melursus ursinus had low captures rates (Table 3). Pioneering studies by Naiman (1988); Pastor et al. (1988), and Naiman and Rogers (1997) found substantial evidence of large ungulates shaping structure of riparian forests in temperate ecosystems by selective browsing, dispersing seeds, and thereby affecting riparian plant community and ultimately modifying channel morphology (Naiman and Rogers, 1997). A similar kind of ungulate dominance in terms of high occupancy and persistence was found in riparian forests in Koyna.
Secondly, we observed an ungulate body size effect on colonization and extinction probabilities, in that the smallest ungulate, barking deer (20–30 kg), had highest extinction probability (0.59 ± 0.07) and lowest colonization probability (0.24 ± 0.05), while the much larger sambar (100–350 kg) had highest colonization probability (0.63 ± 0.10) and lowest extinction probability (0.15 ± 0.07) (Figure 4 and Supplementary Table 7). This indicates that smaller ungulates are more vulnerable to vacate previously occupied sites (local extinction) than larger ungulates, perhaps due to resource competition. In a larger landscape context, however, the opposite of this pattern has been recorded, i.e., large herbivores are more vulnerable to extinction through large-scale anthropogenic factors (Ripple et al., 2015). However, at local scales, more empirical studies are needed to understand this pattern further. Body size has been successfully used to explain ungulate niche differentiation with regards to food requirements and predator sensitivity (Veldhuis et al., 2019). Previous studies in parts of Asia show that smaller ungulate species persist more widely than larger species (Karanth, 2016; Phumanee et al., 2020). Whereas muntjac and wild pig occurred at more sites than sambar and gaur in Thailand (Phumanee et al., 2020), gaur and pig were the least and most wide-ranging species in Karnataka Western Ghats landscape (Karanth, 2016). Contrastingly, Lamichhane et al. (2020) found that barking deer had lowest site occupancy in comparison to other species in Shuklaphanta National Park, Nepal. Hence, there exists much variation in ungulate occupancy patterns in regards to body size, perhaps influenced by local habitat, environmental factors and anthropogenic pressure. We found that large ungulates occur at a higher number of sites, and that gaur and pig both had higher rates of occupancy, in contrast to Karanth (2016) study.
Thirdly, distance from stream edge was a dominant predictor of mammal detection probabilities (Figure 6 and Supplementary Table 3). As distance from stream increased, we observed a drop in the probabilities suggesting that ungulates in riparian forests congregate near streams. It is now well established that rivers, riparian forests and adjacent upland forests are part of a single large contiguous system composed of different smaller units of landscape. Hence viewing riparian forests as part of river continuum framework is essential. Among other riparian habitat factors, elevation was an important feature that influenced species persistence across seasons conforming with the previous occupancy study by Karanth (2016). Canopy cover and number of trees also affected species occupancy, colonization and extinction in our study, as we had hypothesized (Table 2).
Testing the role of riparian forests, especially in regards to stream proximity, is important to understand how climate change will affect ungulate communities (Speakman and Król, 2010; Fuller et al., 2014; Shreshtha et al., 2014; Veldhuis et al., 2019), because increasing land temperatures, changing rainfall regimes and habitat fragmentation increase the risk of regional extinctions (Ripple et al., 2015). The integration of food and water requirements, predation risk and thermoregulation constraints yields a multi-dimensional framework that generates testable predictions to understand ungulate assemblages (Veldhuis et al., 2019). Our work adds to this framework by documenting persistence of forest mammals in riparian forests. Our testing focused on habitat cover and proximity to water and this offers insights into mammal persistence in an altered habitat regime.
Mammal Persistence in Koyna Reservoir: Nilsson and Dynesius (1994) report two major impacts of dams to be the permanent inundation of vast areas of land and disruption of the seasonal flood regime along the river. Other local disturbances due to dam construction may be highly variable globally; however, Alho (2011) generally describes what land mammals face when a river dam is constructed. The prolonged noise produced by machinery, light, presence of workers and other activities during the period of construction disturb wild animals, which will then try to escape to adjacent habitats. Large dams take a long time to complete—Koyna Dam construction began in 1954 and was completed in 1963. The formation of the reservoir displaces resident animals to nearby areas where higher densities of individuals of the same species are already resident. This phenomenon is termed as the reservoir’s extended effect (Sá, 1995; Alho, 2011). This renders free ranging individuals without fixed home ranges more vulnerable and they eventually submit to resident individuals in disputes/competition over natural resources. The result is that animals displaced by the effects of reservoir will die or move to more vulnerable areas. However, once a reservoir is complete and a substantial amount of time has passed, mammals adapt to their surroundings. In our case, 55 years have passed since the Koyna Dam was completed. The landscape has since experienced numerous changes in terms of land and river structure as well the socio-political context. Many villages in the valley have been relocated, firstly when the dam was being constructed and secondly when the area was notified as a wildlife sanctuary in 1985. Tigers also experienced local extinction from Koyna landscape with the last confirmed tiger record from Koyna in 2007. With this study, we know the present status of wildlife in the reservoir, acknowledging that severe changes in mammal community may have taken place which went predominantly undocumented. However, this may be treated as a baseline study on mammal ecology in reference to the Koyna Hydropower Dam five decades after its construction.
Management Implications: Our findings have high relevance for management of riparian forests and accordingly how species can be managed within a reservoir. Habitat variables that contribute to species-specific occupancy and long-term well-being were identified which can be prioritized in management plans. By conserving these factors, wildlife authorities can increase long term species persistence and strategically attempt to limit seasonal and local species extinctions. In addition to highlighting species-habitat relationship patterns of mammals utilizing riparian forests, the information generated in this study provides a strong empirical basis for developing catchment-wide and multi-species strategies for conservation management. Management strategies that have focused only on one key aspect and have simplified riverscapes have inevitably failed. Multi-landscape planning that encompasses streams, rivers and adjacent riparian forests which go beyond conventional planning of a single landscape unit, have had overarching benefits (Naiman and Rogers, 1997; Hermosa et al., 2012; Adams et al., 2014). We implemented a novel field design to study riparian forest use across an entire catchment. This approach employs a robust sampling design by incorporating riparian forests adjacent to all stream types of a catchment which presents a better understanding of mammalian occupancy along different stream types. Following this design, researchers can study species assemblages and help management agencies to efficiently draft plans that manage multiple species rather than focusing on only one charismatic species.
The original contributions presented in the study are included in the article/Supplementary Material, further inquiries can be directed to the corresponding author.
The animal study was reviewed and approved by the Wildlife Institute of India. There was no handling of animals. All surveys were non-invasive in nature (camera traps). All necessary codes of conduct were followed and surveys were permitted by national (Wildlife Institute of India/National Tiger Conservation Authority) and state (Maharashtra Forest Department) authorities.
RK, CB, and SJ: study design. SJ, AG, and NG: data collection. SJ: data analysis and writing. RK, MH: supervision, assistance with data analysis and writing. All authors contributed to the article and approved the submitted version.
This work was supported by the Sahyadri Tiger Conservation Foundation and National Tiger Conservation Authority (NTCA).
The authors declare that the research was conducted in the absence of any commercial or financial relationships that could be construed as a potential conflict of interest.
The study was part of a larger project entitled “Tiger Recovery Strategy and Long-term Monitoring in Sahyadri Tiger Reserve, Maharashtra.” We thank the funding agencies for generous support. We also thank the Director and Dean of the Wildlife Institute of India. We thank the frontline staff of Koyna for support and cooperation during the field surveys. SJ especially thanks the boatman Mr. Ramesh Zendekar for guidance and support while accessing remote parts of Koyna Wildlife Sanctuary. Finally, we thank two reviewers for their suggestions and advice, which substantially helped to improve the quality of the paper.
The Supplementary Material for this article can be found online at: https://www.frontiersin.org/articles/10.3389/fevo.2021.643285/full#supplementary-material
Adams, V. M., Álvarez, J. G., Carwardine, J., Cattarino, L., Hermosa, V., Kennard, M. J., et al. (2014). Planning across freshwater and terrestrial realms: cobenefits and tradeoffs between conservation actions. Conserv. Lett. 7, 425–440. doi: 10.1111/conl.12080
Alho, C. J. R. (2011). Environmental effects of hydropower reservoirs on wild mammals and freshwater turtles in Amazonia: a review. Oecol. Austr. 15, 593–604. doi: 10.4257/oeco.2011.1503.11
Allen, M. L., Peterson, B., and Krofel, M. (2018). No respect for apex carnivores: distribution and activity patterns of honey badgers in the Serengeti. Mammalian Biol. 89, 90–94. doi: 10.1016/j.mambio.2018.01.001
Allen, M. L., Sibarani, M. C., Utoyo, L., and Krofel, M. (2020). Terrestrial mammal community richness and temporal overlap between tigers and other carnivores in Bukit Barisan Selatan National Park, Sumatra. Animal Biodiv. Conserv. 43, 97–107. doi: 10.32800/abc.2020.43.0097
Anoop, K. R., and Hussain, S. A. (2006a). Food and feeding habits of smooth-coated otters (Lutra perspicillata) and their significance to the fish population of Kerala. India. J. Zool. 266, 15–23. doi: 10.1017/s0952836905006540
Anoop, K. R., and Hussain, S. A. (2006b). Factors affecting habitat selection by smooth-coated otters (Lutra perspicillata) in Kerala, India. J. Zool. 263, 417–423. doi: 10.1017/s0952836904005461
Bokil, M. S. (1999). People in protected areas: Koyna sanctuary in Maharashtra. Econ. Political Weekly. 34, 293–299.
Burnham, K. P., and Anderson, D. R. (2002). Model Selection and Miltimodel Inference: A Practical Information-Theoretic Approach. New York, NY: Springer.
Capon, S. J., Chambers, L. E., Mac Nally, R., Naiman, R. J., Davies, P., Marshall, N., et al. (2013). Riparian ecosystems in the 21st century: hotspots for climate change adaptation? Ecosystems 16, 359–381. doi: 10.1007/s10021-013-9656-1
Champion, H. G., and Seth, S. K. (1968). Revised Survey of the Forest Types of India. New Delhi: Government of India Publications.
Chatterjee, A., and Bhattacharyya, S. (2021). Assessing the threats facing wetland mammals in india using an evidence-based conservation approach. Mammal Rev. doi: 10.1111/mam.12242
Datry, T., Larned, S. T., and Tockner, K. (2014). Intermittent rivers: a challenge for freshwater ecology. BioScience 64, 229–235. doi: 10.1093/biosci/bit027
Datry, T., Pella, H., Leigh, C., Bonada, N., and Huguney, B. (2016). A landscape approach to advance intermittent river ecology. Freshw. Biol. 61, 1200–1213. doi: 10.1111/fwb.12645
Datry, T., Singer, G., Sauquet, E., Jorda-Capdevilla, D., Von Schiller, D., Subbington, R., et al. (2017a). Science and management of intermittent rivers and ephemeral streams (SMIRES). Res. Ideas Outcomes 3, e21774. doi: 10.3897/rio.3.e21774
Datry, T., Bonada, N., and Boulton, A. (2017b). Intermittent Rivers and Ephemeral Streams Ecology and Management. Cambridge, MA: Academic Press.
Dornmann, C. F., Elith, J., Bacher, S., Buchmann, C., Carl, G., Carré, G., et al. (2013). Collinearity: a review of methods to deal with it and a simulation study evaluating their performance. Ecography 36, 027–046. doi: 10.1111/j.1600-0587.2012.07348.x
Duckworth, J. W., and Hedges, S. (1998). A Review of the Status of Tiger, Asian Elephant, Gaur and Banteng in Vietnam, Lao, Camobodia and Yunnan (China), with Recommendations for Future Conservation Action. Hanoi: WWF Indochina Programme.
Duckworth, J. W., Salter, R. E., and Khounbline, K. (1999). Wildlife in Lao PDR: 1999 Status Report. Vientiane: IUCN.
Dugdale, S. J., Malcolm, I. A., Kantola, K., and Hannah, D. M. (2018). Stream temperature under contrasting riparian forest cover: understanding thermal dynamics and heat exchange processes. Sci. Total Environ. 610– 611, 1375–1389. doi: 10.1016/j.scitotenv.2017.08.198
Fuller, A., Hetem, R. S., Maloney, S. K., and Mitchell, D. (2014). Adaptation to heat and water shortage in large, arid-zone mammals. Physiology 29, 159–167. doi: 10.1152/physiol.00049.2013
Greiser Johns, A. (2000). Pu Mat: A Biodiversity Survey of a Vietnamese Protected Area. Social Forestry and Nature Conservation in Nghe An Province. Vietnam: SFNC Project, Vinh, Nghe An Province.
Hermosa, V., Kennard, M. J., and Linke, S. (2012). Integrating multidirectional connectivity requirements in systematic conservation planning for freshwater systems. Divers. Distrib. 18, 448–458. doi: 10.1111/j.1472-4642.2011.00879.x
Hines, J. E. (2006). PRESENCE – Software to Estimate Patch Occupancy and Related Parameters. USGS – PWRC. Available online at: http://www.mbr-pwrc.usgs.gov/software/presence.html
Hussain, S. A., and Choudhury, B. C. (1997). Distribution and status of the smooth-coated otter Lutra perspicillata in National Chambal Wildlife Sanctuary, India. Biol. Conserv. 80, 199–206. doi: 10.1016/s0006-3207(96)00033-x
Jelil, S. N., Gaykar, A., Girkar, N., Vyas, V., Ben, V. C., and Ramesh, K. (2020). Recent record of tiger from Sahaydri Tiger Reserve, India. CATnews 71, 16–17.
Joglekar, A., Tadwalkar, M., Mhaskar, M., Chavan, B., Ganeshaiah, K. N., and Patwardhan, A. (2015). Tree species composition in Koyna Wildlife Sanctuary, Northern Western Ghats of India. Curr. Sci. 108, 1688–1693.
Johnsingh, A. J. T., Qureshi, Q., Goyal, S. P., Rawat, G. S., Ramesh, K., David, A., et al. (2004). Conservation Status of Tiger and Associated Species in the Terai-Arc Landscape, India. Dehradun: Wildlife Institute of India, 110.
Karanth, K. K. (2016). Wildlife in the matrix: Spatio-temporal patterns of herbivore occurrence in Karnataka, India. Environ. Manage. 57, 189–206. doi: 10.1007/s00267-015-0595-9
Kassambara, A. (2020). ggpubr: ‘ggplot2’ Based Publication Ready Plots. R Package Version 0.4.0. Vienna: R Foundation for Statistical Computing.
Kassambara, A., and Mundt, F. (2020). factoextra: Extract and Visualize the Results of Multivariate Data Analyses. R Package Version 1.0.7. Vienna: R Foundation for Statistical Computing.
Lamichhane, S., Khanal, G., Karki, J. B., Aryal, C., and Acharya, S. (2020). Natural and anthropogenic correlates of habitat use by wild ungulates in Shuklaphanta National Park, Nepal. Global Ecol. Conserv. 24:e01338. doi: 10.1016/j.gecco.2020.e01338
Linkie, M., and Ridout, M. S. (2011). Assessing tiger-prey interactions in Sumatran rainforests. J. Zool. 284, 224–229. doi: 10.1111/j.1469-7998.2011.00801.x
MacKenzie, D. I., Nichols, J. D., Lachman, G. B., Droege, S., Royle, J. A., and Langtimm, C. A. (2002). Estimating site occupancy rates when detection probabilities are less than one. Ecology 83, 2248–2255. doi: 10.1890/0012-9658(2002)083[2248:esorwd]2.0.co;2
MacKenzie, D. I., and Bailey, L. L. (2004). Assessing the fit of site-occupancy models. J. Agricul. Biol. Environ. Stat. 9, 300–318. doi: 10.1198/108571104x3361
MacKenzie, D. I., Nichols, J. D., Royle, J. A., Pollock, K. H., Hines, J. E., and Bailey, L. L. (2006). Occupancy Estimation and Modelling: Inferring Patterns and Dynamics of Species Occurrence. San Diego, CA: Elsevier.
Naiman, R. J., and Rogers, K. H. (1997). Large animals and system-level characteristics in river corridors. BioScience 47, 521–529. doi: 10.2307/1313120
Naiman, R. J. (1988). Animal influences on ecosystem dynamics. BioScience 38, 750–752. doi: 10.2307/1310783
Nilsson, C., and Dynesius, M. (1994). Ecological effects of river regulation on mammals and birds: a review. Regul. Rivers Res. Manage. 9, 45–53. doi: 10.1002/rrr.3450090105
Pastor, J., Naiman, R. J., Dewey, B., and McInnes, P. (1988). Moose, microbes and the boreal forest. BioScience 38, 770–777. doi: 10.2307/1310786
Phumanee, W., Steinmetz, R., Phoonjampa, R., Bejraburnin, T., Grainer, M., and Savini, T. (2020). Occupancy-based monitoring of ungulate prey species in Thailand indicates population stability, but limited recovery. Ecosphere 11:e03208. doi: 10.1002/ecs2.3208
Pinay, G., Bernal, S., Abbott, B. W., Lupon, A., Marti, E., Sabater, F., et al. (2018). Riparian corridors: a new conceptual framework for assessing nitrogen buffering across biomes. Front. Environ. Sci. 6:47. doi: 10.3389/fenvs.2018.00047
Prakash, N., Mudappa, D., Shankar Raman, T. R., and Kumar, A. (2012). Conservation of the Asian small-clawed otter (Aonyx cinereus) in human-modified landscapes, Western Ghats, India. Trop. Sci. Conserv. 5, 67–78. doi: 10.1177/194008291200500107
Raha, A., and Hussain, S. A. (2016). Factors affecting habitat selection by three sympatric otter species in the southern Western Ghats, India. Acta Ecol. Sinica 36, 45–49. doi: 10.1016/j.chnaes.2015.12.002
R Core Team (2020). R: A Language and Environment for Statistical Computing. Vienna: R Foundation for Statistical Computing.
Ripple, W. J., Newsome, T. M., Wolf, C., Dirzo, R., Everatt, K. T., Galetti, M., et al. (2015). Collapse of the world’s largest herbivores. Sci. Adv. 1:e1400103. doi: 10.1126/sciadv.1400103
Rovero, F., and Zimmerman, F. (2016). Camera Trapping for Wildlife Research, 1st Edition. Exeter: Pelagic Publishing.
Sá, R. M. L. (1995). Effects of the Samuel Hydroelectric Dam on Mammal and Bird Communities in A Heterogenous Amazonian Lowland Forest. 140. [doctoral dissertation]. University of Florida, Gainesville, FL.
Sánchez-Montoya, M. M., Moleón, M., Sánchez-Zapata, J. A., and Tockner, K. (2016). Dry riverbeds: corridors for terrestrial vertebrates. Ecosphere 7:e01508. doi: 10.1002/ecs2.1508
Santos, M. J., Matos, H. M., Palomares, F., and Santos-Reis, M. (2011). Factors affecting mammalian carnivore use of riparian ecosystems in Mediterranean climates. J. Mammal. 92, 1060–1069. doi: 10.1644/10-mamm-a-009.1
Schaller, G. B. (1967). The Deet and the Tiger. A Study of Wildlife in India. Chicago, IL: University of Chicago Press.
Seavy, N. E., Gardali, T., Golet, G. H., Griggs, T., Howell, C. A., Kesley, R., et al. (2009). Why climate change makes riparian restoration more important than ever: recommendations for practice and research. Ecol. Restor. 27, 330–338. doi: 10.3368/er.27.3.330
Shreshtha, A. K., van Wieren, S. E., van Langevelde, F., Fuller, A., Hetem, R. S., Meyer, L., et al. (2014). Larger antelopes are sensitive to heat stress throughout all seasons but smaller antelopes only during summer in an African semi-arid environment. Int. J. Biometeorol. 58, 41–49. doi: 10.1007/s00484-012-0622-y
Speakman, J. R., and Król, E. (2010). Maximal heat dissipation capacity and hyperthermia risk: neglected key factors in the ecology of endotherms. J. Animal Ecol. 79, 726–746.
Timmins, R. J., and Ou, R. (2001). The Importance of Phnom Prich Wildlife Sanctuary and Adjacent Areas for the Conservation of Tigers and Other Key Species. Hanoi: WWF Indochina Programme.
Timmins, R., Kawanishi, K., Giman, B., Lynam, A., Chan, B., Steinmetz, R., et al. (2015). Rusa Unicolor. The IUCN Red List of Threatened Species 2015. Gland: IUCN.
Timmins, R. J., Duckworth, J. W., and Hedges, S. (2016). Muntiacus Muntjak. The IUCN Red List of Threatened Species 2016. Gland: IUCN.
Umapathy, G., and Durairaj, G. (1995). Preliminary studies on the feeding ecology of the otter Lutra lutra at Pitchavaram, East Coast of India. IUCN Otter Special. Group Bull. 11, 24–26.
Perinchery, A., Jathanna, D., and Kumar, A. (2011). Factors determining occupancy and habitat use by Asian small-clawed otters in the Western Ghats, India. J. Mammal. 92, 796–802. doi: 10.1644/10-mamm-a-323.1
Vannote, R. L., Minshall, G. W., Cummins, K. W., Sedell, J. R., and Cushing, C. E. (1980). River continuum concept. Can. J. Fish. Aquat. Sci. 37, 130–137. doi: 10.1139/f80-017
Veldhuis, M. P., Kihwle, E. S., Cromsigt, J. P. G. M., Ogutu, J. O., Hopcraft, J. G. C., Owen-Smith, N., et al. (2019). Large herbivore assemblages in a changing climate: incorporating water dependence and thermoregulation. Ecol. Lett. 22, 1536–1546. doi: 10.1111/ele.13350
Wei, T., and Simko, V. (2017). R Package “Corrplot”: Visualization of A Correlation Matrix (Version 0.84). Vienna: R Foundation for Statistical Computing.
Keywords: dammed river, occupancy modeling, colonization, extinction, reservoir biodiversity, river continuum
Citation: Jelil SN, Gaykar A, Girkar N, Ben C, Hayward MW and Krishnamurthy R (2021) Mammal Persistence Along Riparian Forests in Western India Within a Hydropower Reservoir 55 Years Post Construction. Front. Ecol. Evol. 9:643285. doi: 10.3389/fevo.2021.643285
Received: 17 December 2020; Accepted: 26 March 2021;
Published: 16 April 2021.
Edited by:
Eben Goodale, Guangxi University, ChinaReviewed by:
Riddhika Ramesh (Kalle), Salim Ali Center for Ornithology and Natural History, IndiaCopyright © 2021 Jelil, Gaykar, Girkar, Ben, Hayward and Krishnamurthy. This is an open-access article distributed under the terms of the Creative Commons Attribution License (CC BY). The use, distribution or reproduction in other forums is permitted, provided the original author(s) and the copyright owner(s) are credited and that the original publication in this journal is cited, in accordance with accepted academic practice. No use, distribution or reproduction is permitted which does not comply with these terms.
*Correspondence: Ramesh Krishnamurthy, cmFtZXNoQHdpaS5nb3YuaW4=
Disclaimer: All claims expressed in this article are solely those of the authors and do not necessarily represent those of their affiliated organizations, or those of the publisher, the editors and the reviewers. Any product that may be evaluated in this article or claim that may be made by its manufacturer is not guaranteed or endorsed by the publisher.
Research integrity at Frontiers
Learn more about the work of our research integrity team to safeguard the quality of each article we publish.