- 1Lab BOREA, Team Ecofunc, Normandie Université, Université de Caen Normandie, CNRS, MNHN, IRD, SU, UA CS 14032, Caen, France
- 2GEOMAR Helmholtz Centre for Ocean Research Kiel, Kiel, Germany
- 3France Energies Marines ITE-EMR, Plouzané, France
- 4IRD, Université de Brest, CNRS, Ifremer, LEMAR, Plouzane, France
- 5Department of Marine Sciences, University of Gothenburg, Göteborg, Sweden
- 6CNRS, INRA, IRD, Institute of Ecology and Environmental Sciences–Paris, Sorbonne Université, Université Paris Est Créteil, Université Paris Diderot, Paris, France
- 7UNICAEN, UNIROUEN, Laboratoire Morphodynamique Continentale et Côtière, M2C Normandie Université, CNRS UMR 6143 M2C, Caen, France
- 8LIttoral ENvironnement et Sociétés (LIENSs), UMR 7266, CNRS, La Rochelle University, Bâtiment ILE, La Rochelle, France
Introduction
The 2020’s will probably stand out as the decade when offshore wind farms (OWF) were developed worldwide (GWEC, 2020) as a response toward sustainability goals. Today, global OWF capacity represents 29.1 GW, which in end of 2019 accounted for 5% of total global wind capacity (GWEC, 2020). Since the world's first offshore wind turbine was installed in Denmark in 1991, Europe has taken the lead in offshore wind development. By 2019, 5,047 offshore wind turbines were installed along the European coasts, corresponding to 110 OWF producing 22.1 GW (WindEurope, 2019). OFW developments are often located on densely populated coastlines and races questions about human-environment interactions, spatial planning, and cumulated impacts with other human activities, fisheries in particular (Berkenhagen et al., 2010).
OWF constructions have consequences on the marine ecosystems and dwellers of coastal areas, which depend on the complex network of ecological, socio-economic and political variables. Energy is indeed a vital issue for our future and requires the development of interdisciplinary research to interpret and drive the choices and responses to the climate challenge faced by our society (Labussière and Nadaï, 2015). A better understanding of the functioning and evolutionary trajectory of social-ecological systems (SES) following the choices in the energy sector is very useful for the governance (Mazé, 2020). SES provides the conceptual framework for the analysis of these intertwined social and natural systems. Berkes and Folke (1998) developed the ideas around SES in a context of resilience, even if this notion was already present in other fields (e.g., epidemiological context, Cherkasskii, 1988). They underlined the need to balance the “social” and “ecological” subsystems when considering them together, and to connect them through non-linear ecological and knowledge of human dynamics.
Studying SES using systems science brings highly valuable answers to numerous questions concerning their interactions and their role in either transmitting or attenuating perturbations. These are theoretical as well as practical questions useful for decision. Several authors have proposed the development of SES models to understand the resilience, vulnerability, and adaptability of these systems (Young et al., 2006), or to answer management questions within a context of resilience-based decision (e.g., Anthony et al., 2013). They emphasize the need of an approach that tackles explicitly the structure of the interactions between the social and ecological components (Walker et al., 2006).
Qualitative models facilitate the study of complex systems without being hampered by the need of accurately measure behavior and relationships between variables (Justus, 2006). Based on loop analysis as developed in the 1970's by Richard Levins (Levins, 1974, 1975; Lane and Levins, 1977), SES can be modeled as signed, directed graphs (signed digraph), where each relationship is described as 0, +, or –, according to the direct effect from one variable to another. The aim of this opinion paper is to emphasize the uniqueness of SES that stem from OWF, and to address how some challenges typically encountered when modeling SES linked with OWF can be resolved through Levin's loop analysis.
The Sudden Emergence of a New Social-Ecological System
Marine energy production systems implementation causes social transformations at large scale and on local territories, and changes in the environmental biophysics. These infrastructures can thus be perceived as either opportunities or threats to the social and ecological environment. When the project to build a new OWF appears, new interactions establishes in the SESs. These interactions evolve through time and form different networks during the different steps of the procedure. In the beginning, some interactions will concern the influence on decisions about whether and where to build the OWF. After authorization and before construction, when the OWF is an object of imagination, fears may appear, e.g., linked with the scenery modification, the loss of living for fishermen, or the threats to the environment and marine life. During the construction and exploitation, new networks are organized, eventually with novel actors entering the SES. This evolution leads to the sudden emergence of new SES, linking actors and the marine ecosystem at each time step. The modifications also concern the relationships within the ecosystem, with impacts and disturbances during the building and exploitation phases. The exploitation modifies the habitat and consequently engenders food webs with new characteristics, e.g., due to the reef effect (Raoux et al., 2017). During operation, the closure to fisheries in the surrounding of the OWF may mimic a marine protected area and, due to spillover mechanisms, generate a reserve effect (Raoux et al., 2019; Halouani et al., 2020).
Analyzing the dynamics of these new SES requires considering social and ecological variables together, linked by interactions of various types and currencies. They may be consumption or influence processes. The former is a typical predator-prey relationship within food webs. Influence may have different nature and range from economic relationships to regulatory processes. Understanding the dynamics of SESs also requires considering sentimental aspects and perception. This aspect includes, e.g., what people value, how they perceive the environment and ecosystems, how they appropriate and accept a change. Including these connections is central for understanding how acceptance of the OFW development can affect the SES dynamics.
As different ends of the impact spectrum could emerge at any step of the OWF life-cycle, the SES is continuously self-adapted and self-adjusted to improve its stability (Latour, 2005). Then, loop analysis allows calculating the local stability in terms of understanding eco-social feedbacks to the arrival of a new OWF, which can modify the dynamics of interactions in and between each subsystem. The system may then be considered for trade-off calculations, where a new balance may be found between the need of conserving some components of the marine ecosystem (defining what is most valued), and the need of exploiting marine resources and territory.
The Advantage of Loop Analysis
When constructing a qualitative model, the first step is to identify all relevant actors (variables). Through good knowledge of the system, the modeler has to characterize the direct positive or negative links between these variables, without needing to quantify the intensity. Good practice is also maintaining a good balance between the social and ecological components, with comparable numbers of variables in the two constitutive sub-systems (Berkes and Folke, 1998).
An n × n Jacobian matrix summarizes all direct interactions among the n variables of the SES. Loop analysis applies only to Jacobian matrices in the small neighborhood of local equilibrium (Justus, 2006), a condition verified if and only if the real part of all eigenvalues is negative (Lyapunov, 1992). Levins (1974) reformulated the stability criterion in terms suitable to loop analysis applications. It requires negative feedback at each level, and stronger feedback at lower levels than higher ones. In general, the inclusion of negative self-loops (i.e., negative diagonal elements in the Jacobian matrix) contributes to system's stability (Dambacher and Ramos-Jiliberto, 2007). However, this condition may impose constraints to SES modeling (e.g., negative self-loops are not compatible with symbionts; Justus, 2005). Upon verification of local stability, loop analysis may develop in the direction of either local stability analysis or press perturbation analysis. Local stability analysis investigates how the system reacts to small perturbations of finite duration, while press perturbation analysis determines how the system responds to perturbations of indefinite duration (Schmitz, 1997).
Local stability requires every variable to return to its initial status after perturbation. Locally stable systems always display negative overall feedback and their resistance to external perturbation increases with the magnitude of the overall feedback (Levins, 1998). Changes in variables' self-dynamics and modifications of the digraph architecture may alter the local stability. Hence, the comparison between the overall feedback of the reference and alternative models may inform about the impact that various management scenarios have on the sustainability of the entire SES (Ortiz and Levins, 2011; Ortiz et al., 2013).
Press perturbation analysis allows understanding how perturbations affect the variable equilibrium in the SES. A press perturbation in the OWF SES might represent expected social or ecological changes. The estimated probability of change indicates how the different social and ecological variables are likely to react (negatively, positively or neutral), and may be represented as heatmaps (Figure 1D). These heatmaps highlight system's reactions, and may help to identify the emergence of trade-offs, synergies, and conflicts among the variables.
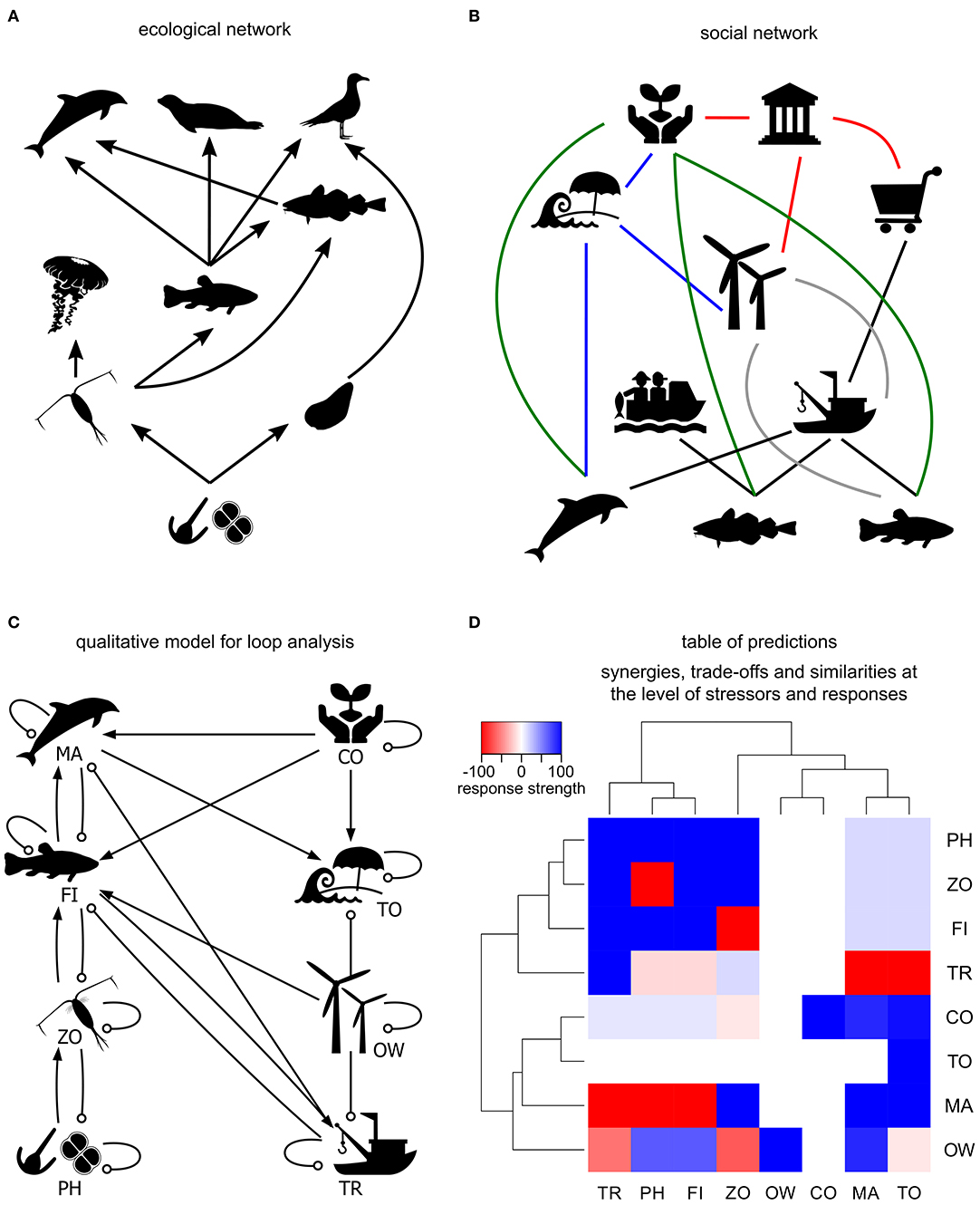
Figure 1. From ecological and social networks to predictions of SES dynamics with loop analysis. The ecological network (A) depicts trophic interactions in a hypothetical food web (arrows indicate energy flow direction). The social network (B) shows a toy model with biomass flows (black) and governmental (red) relationships together with conservation (green), marine protection (gray) and perception (blue). Animals in the social network represent the interface through which social actors directly interact with the ecosystem. A selection of variables from the ecological and social systems allows building the qualitative model of the SES (C) with positive (arrow-headed) and negative (circle-headed) relationships (i.e., digraph). The table of predictions (D) derived from the digraph describes expected changes in column variables triggered by positive press perturbations on row variables. The clustering along the rows and the columns accounts for similarities in the impacts generated and the responses, respectively. Codes: PH, phytoplankton; ZO, zooplankton; FI, forage fishes; MA, marine mammals; TR, trawl fisheries; CO, conservation; TO, tourism; OW, offshore wind farms.
Sensitivity analyses are also recommended. These will identify variables and links that are more sensitive to a certain type of perturbation, and thus more likely to be affected by a change. The assessment of responses to press perturbations, the summary of all paths linking variables and the application of sensitivity analysis enable to understand how a SES will respond to known or expected changes, and might help taking informed decisions along with planning adaptive management strategies.
Several studies have analyzed the impact of OWF construction on species. However, a recent review concluded that we lack knowledge on how OWF operations modify the functioning of ecosystems, and addressing this knowledge-gap will require hypothesis-driven research combined with ecological modeling (Dannheim et al., 2020). Recent works have focused on independent perturbation effects on ecosystems (Raoux et al., 2018). Nevertheless, the awareness that marine ecosystems are threatened by multiple anthropogenic and natural perturbations calls for the need of understanding how these perturbations interact to influence ecosystem functioning and stability. From a social perspective, there is a knowledge lack on how OWF projects change actors' positions in the governance of marine ecosystems. Although we have some understanding on how local populations may perceive OWF projects (Ladenburg, 2010; Haggett, 2011), we have less knowledge on how these perceptions influence the SES dynamics, and how the perception can be altered through, e.g., social compensations. Qualitative mathematical modeling is a powerful approach to project and analyse different management options, and investigate the impact of multiple anthropogenic stressors on a system (Raoux et al., 2018). Its strength lies in its rapid analysis of the system structure and dynamics, which facilitates the comparison of alternative system structures (Dambacher et al., 2002). In this context, qualitative models have been used to analyse and predict the impact of multiple cumulative pressure changes associated to an OWF in the Bay of Seine (Raoux et al., 2018) and to explore the responses of an OWF SES under different hypothetical social compensation strategies (Haraldsson et al., 2020).
A social-ecological modeling approach is not new to OWF studies. For instance, it has taken the view of the stakeholders (Château et al., 2012), considered the social and emotional view of OWF (Bidwell, 2013), or adopted the ecosystem perspective (Raoux et al., 2019). Often, these SES characterize one of the subsystems in more detail, while the other subsystem is represented by one or a few variables, and the intricate interactions taking place within the two networks are thereby ignored. The decision about network resolution depends on the purpose of the SES model and the question to address. However, added complexity in both social and ecological system through a “balanced view” is crucial for capturing unpredictable indirect effects and feedbacks within and between domains (Haraldsson et al., 2020), independently of the viewpoint, and it can have impact on our overall understanding of the SES.
Adding complexity in both social and ecological domains comes with clear methodological challenges, e.g., (1) the immense effort required to collect data from both social and ecological networks, (2) the difficulty of combining these data, which are often measured in different currencies and on different scales. Loop analysis can circumvent these constraints as it models the structure and dynamics of a system without including quantitative data, and it has been proposed as a suitable tool when aiming at adding complexity in both domains of the SES (Haraldsson et al., 2020). Instead of tedious sampling programs, time and effort should be dedicated to developing and comparing alternative system structures based on different views or hypotheses about how the system works. Like in any modeling exercise, we will not have the answer from one ultimate model, but it is by comparing and understanding multiple model structures that we can draw meaningful conclusions.
Concluding Remarks
The main advantage of loop analysis relies in its power of addressing general but realistic questions in the context of heterogeneous networks as the OWF associated SES. The main outcomes are:
• The possibility to consider concurrent press perturbations and the generation of weighted predictions (Dambacher et al., 2002; Bodini et al., 2018).
• The quick testing of various management strategies following combinations of press perturbations (i.e., basic screening of options for adaptive management).
• The identification of parts of the system that behave in synchronous way (i.e., similarities of responses deduced from the table of predictions).
• The summary of antagonistic goals and the classification of press perturbations according to their similarities and potentially synergistic effects.
These outputs provide a good basis for communications, which may ease the acceptance of OWFs due to simple and unambiguous description of benefits and impacts. They also facilitate the participation of stakeholders in the definition and testing of alternative scenarios.
Author Contributions
MS and MT created Figure 1. All authors have made a substantial, direct and intellectual contribution to the work, and approved it for publication.
Funding
This work was part of the project APPEAL (Socio-ecosystemic approach to the impact of floating wind farms) which benefited from France Energies Marines and State financing managed by the National Research Agency under the Investments for the Future program bearing the reference ANR- 10-IED-0006-25. NN was also funded by Fondation de France, within the project Sensitroph about actors' sensitivity to marine ecosystems.
Conflict of Interest
The authors declare that the research was conducted in the absence of any commercial or financial relationships that could be construed as a potential conflict of interest.
Abbreviations
OWF, offshore wind farms; SES, social-ecological system.
References
Anthony, K. R. N., Dambacher, J. M., Walshe, T., and Beeden, R. (2013). A Framework for Understanding Cumulative Impacts, Supporting Environmental Decisions and Informing Resilience-based Management of the Great Barrier Reef World Heritage Area. Townsville, QLD; Hobart, TAS: Australian Institute of Marine Science, NERPDecisions Hub, University of Melbourne and Great Barrier Reef Marine Park Authority; CSIRO. Available online at: http://hdl.handle.net/11017/2850
Berkenhagen, J., Döring, R., Fock, H. O., Kloppmann, M. H., Pedersen, S. A., and Schulze, T. (2010). Decision bias in marine spatial planning of offshore wind farms: problems of singular versus cumulative assessments of economic impacts on fisheries. Mar. Policy 34, 733–736. doi: 10.1016/j.marpol.2009.12.004
Berkes, F., and Folke, C. (1998). Linking Social and Ecological Systems: Management Practices and Social Mechanisms for Building Resilience. New York, NY: Cambridge University Press.
Bidwell, D. (2013). The role of values in public beliefs and attitudes towards commercial wind energy. Energy Policy 58, 189–199. doi: 10.1016/j.enpol.2013.03.010
Bodini, A., Rocchi, M., and Scotti, M. (2018). Insights into the ecology of the Black Sea through the qualitative loop analysis of the community structure. Limnol. Oceanogr. 63, 968–984. doi: 10.1002/lno.10713
Château, P. A., Chang, Y. C., Chen, H., and Ko, T. T. (2012). Building a stakeholder's vision of an offshore wind-farm project: a group modeling approach. Sci. Total Environ. 420, 43–53. doi: 10.1016/j.scitotenv.2012.01.031
Cherkasskii, B. L. (1988). The system of the epidemic process. J. Hyg. Epidemiol. Microbiol. Immunol. 32, 321–328.
Dambacher, J. M., Li, H. W., and Rossignol, P. A. (2002). Relevance of community structure in assessing indeterminacy of ecological predictions. Ecology 83, 1372–1385. doi: 10.1890/0012-9658
Dambacher, J. M., and Ramos-Jiliberto, R. (2007). Understanding and predicting effects of modified interactions through a qualitative analysis of community structure. Q. Rev. Biol. 82, 227–250. doi: 10.1086/519966
Dannheim, J., Bergström, L., Birchenough, S. N., Brzana, R., Boon, A. R., et al. (2020). Benthic effects of offshore renewables: identification of knowledge gaps and urgently needed research. ICES J. Mar. Sci. 77, 1092–1108. doi: 10.1093/icesjms/fsz018
GWEC (2020). Global Wind 2019 Report. Global Wind Energy Council. Available online at: https://gwec.net/global-wind-report-2019/
Haggett, C. (2011). Understanding public responses to offshore wind power. Energy Policy 39, 503–510. doi: 10.1016/j.enpol.2010.10.014
Halouani, G., Villanueva, C. M., Raoux, A., Dauvin, J. C., Ben Rais Lasram, F., Foucher, E., et al. (2020). A spatial food web model to investigate potential spillover effects of a fishery closure in an offshore wind farm. J. Mar. Syst. 212:103434. doi: 10.1016/j.jmarsys.2020.103434
Haraldsson, M., Raoux, A., Riera, F., Hay, J., Dambacher, J. M., and Niquil, N. (2020). How to model social-ecological systems?–A case study on the effects of a future offshore wind farm on the local society and ecosystem, and whether social compensation matters. Mar. Policy 119:104031. doi: 10.1016/j.marpol.2020.104031
Justus, J. (2005). Qualitative scientific modeling and loop analysis. Philos. Sci. 72, 1272–1286. doi: 10.1086/508099
Justus, J. (2006). Loop analysis and qualitative modeling: limitations and merits. Biol. Philos. 21, 647–666. doi: 10.1007/s10539-006-9050-x
Labussière, O., and Nadaï, A. (2015). L'énergie des Sciences Sociales. Paris: Alliance Athena. Available online at: http://books.openedition.org/allianceathena/203
Ladenburg, J. (2010). Attitudes towards offshore wind farms—the role of beach visits on attitude and demographic and attitude relations. Energy Policy 38, 1297–1304. doi: 10.1016/j.enpol.2009.11.005
Lane, P., and Levins, R. (1977). The dynamics of aquatic systems II. The effects of nutrient enrichment on model plankton communities. Limnol. Oceanogr. 22, 454–471.
Latour, B. (2005). Reassembling the Social. An Introduction to Actor-Network-Theory. London: Oxford University Press.
Levins, R. (1974). Discussion paper: the qualitative analysis of partially specified systems. Ann. N. Y. Acad. Sci. 231, 123–138.
Levins, R. (1975). “Problems of signed digraphs in ecological theory” in Ecosystem Analysis and Prediction, ed S. A. Levin (Philadelphia, PA: Society for Industrial and Applied Mathematics), 264–277.
Levins, R. (1998). “Qualitative mathematics for understanding, prediction, and interventions in complex ecosystems,” in Ecosystem Health, eds D. Rapport, R. Costanza, P. Epstein, C. Gaudet, and R. Levins (Oxford: Blackwell Science), 178–204.
Mazé, C. (2020). Le concept de transformation vers la soutenabilité: de la science à l'(in)action publique. Le cas brûlant de la gouvernance des socio-écosystèmes marins et côtiers dans le climat du XXIe siècle. La Rochelle: Habilitation à diriger des recherches (HDR)–Université de la Rochelle.
Ortiz, M., and Levins, R. (2011). Re-stocking practices and illegal fishing in northern Chile (SE Pacific coast): a study case. Oikos 120, 1402–1412. doi: 10.1111/j.1600-0706.2011.19041.x
Ortiz, M., Levins, R., Campos, L., Berrios, F., Campos, F., Jordán, F., et al. (2013). Identifying keystone trophic groups in benthic ecosystems: implications for fisheries management. Ecol. Indic. 25, 133–140. doi: 10.1016/j.ecolind.2012.08.020
Raoux, A., Dambacher, J. M., Pezy, J. P., Mazé, C., Dauvin, J. C., and Niquil, N. (2018). Assessing cumulative socio-ecological impacts of offshore wind farm development in the Bay of Seine (English Channel). Mar. Policy 89, 11–20. doi: 10.1016/j.marpol.2017.12.007
Raoux, A., Lassalle, G., Pezy, J. P., Tecchio, S., Safi, G., Ernande, B., et al. (2019). Measuring sensitivity of two OSPAR indicators for a coastal food web model under offshore wind farm construction. Ecol. Indic. 96, 728–738. doi: 10.1016/j.ecolind.2018.07.014
Raoux, A., Tecchio, S., Pezy, J. P., Lassalle, G., Degraer, S., Wilhelmsson, D., et al. (2017). Benthic and fish aggregation inside an offshore wind farm: which effects on the trophic web functioning? Ecol. Indic. 72, 33–46. doi: 10.1016/j.ecolind.2016.07.037
Schmitz, O. J. (1997). Press perturbations and the predictability of ecological interactions in a food web. Ecology 78, 55–69. doi: 10.1890/0012-9658(1997)078(0055:PPATPO)2.0.CO
Walker, B. H., Anderies, J. M., Kinzig, A. P., and Ryan, P. (2006). Exploring resilience in social-ecological systems through comparative studies and theory development: introduction to the special issue. Ecol. Soc. 11:12. doi: 10.5751/ES-01573-110112
WindEurope (2019). Offshore Wind in Europe—Key Trends and Statistics 2019. Available online at: https://windeurope.org/wp-content/uploads/files/about-wind/statistics/WindEurope-Annual-Offshore-Statistics-2019.pdf
Keywords: social-ecosystem modeling, loop analysis, adaptive management, sensitivity analysis, marine renewable energy
Citation: Niquil N, Scotti M, Fofack-Garcia R, Haraldsson M, Thermes M, Raoux A, Le Loc'h F and Mazé C (2021) The Merits of Loop Analysis for the Qualitative Modeling of Social-Ecological Systems in Presence of Offshore Wind Farms. Front. Ecol. Evol. 9:635798. doi: 10.3389/fevo.2021.635798
Received: 30 November 2020; Accepted: 14 January 2021;
Published: 17 February 2021.
Edited by:
Ferenc Jordan, Hungarian Academy of Science, HungaryReviewed by:
Marco Ortiz, University of Antofagasta, ChileCopyright © 2021 Niquil, Scotti, Fofack-Garcia, Haraldsson, Thermes, Raoux, Le Loc'h and Mazé. This is an open-access article distributed under the terms of the Creative Commons Attribution License (CC BY). The use, distribution or reproduction in other forums is permitted, provided the original author(s) and the copyright owner(s) are credited and that the original publication in this journal is cited, in accordance with accepted academic practice. No use, distribution or reproduction is permitted which does not comply with these terms.
*Correspondence: Nathalie Niquil, nathalie.niquil@unicaen.fr
†ORCID: Marco Scotti orcid.org/0000-0002-0775-6148