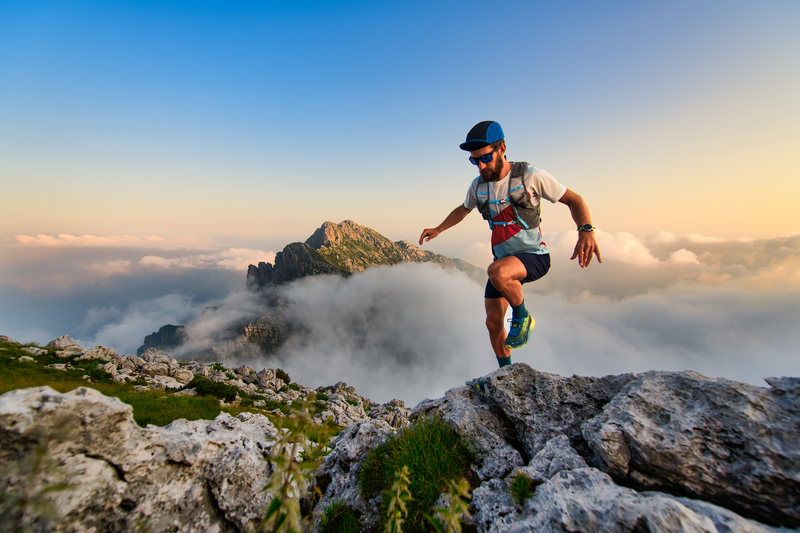
95% of researchers rate our articles as excellent or good
Learn more about the work of our research integrity team to safeguard the quality of each article we publish.
Find out more
REVIEW article
Front. Ecol. Evol. , 04 March 2021
Sec. Behavioral and Evolutionary Ecology
Volume 9 - 2021 | https://doi.org/10.3389/fevo.2021.633947
This article is part of the Research Topic Cognition and Adaptation to Urban Environments View all 6 articles
Explaining how animals respond to an increasingly urbanised world is a major challenge for evolutionary biologists. Urban environments often present animals with novel problems that differ from those encountered in their evolutionary past. To navigate these rapidly changing habitats successfully, animals may need to adjust their behaviour flexibly over relatively short timescales. These behavioural changes, in turn, may be facilitated by an ability to acquire, store, and process information from the environment. The question of how cognitive abilities allow animals to avoid threats and exploit resources (or constrain their ability to do so) is attracting increasing research interest, with a growing number of studies investigating cognitive and behavioural differences between urban-dwelling animals and their non-urban counterparts. In this review we consider why such differences might arise, focusing on the informational challenges faced by animals living in urban environments, and how different cognitive abilities can assist in overcoming these challenges. We focus largely on birds, as avian taxa have been the subject of most research to date, but discuss work in other species where relevant. We also address the potential consequences of cognitive variation at the individual and species level. For instance, do urban environments select for, or influence the development of, particular cognitive abilities? Are individuals or species with particular cognitive phenotypes more likely to become established in urban habitats? How do other factors, such as social behaviour and individual personality, interact with cognition to influence behaviour in urban environments? The aim of this review is to synthesise current knowledge and identify key avenues for future research, in order to improve our understanding of the ecological and evolutionary consequences of urbanisation.
In our increasingly urbanised world, more animals face the challenges of city life than ever before. Urban-dwelling animals must deal with a range of environmental changes that vary in their spatial and temporal scale in order to exploit anthropogenic foods, find suitable breeding sites, and deal with pollution, people and traffic (Legagneux and Ducatez, 2013; Potvin, 2017; Goumas et al., 2020b). While some of these challenges may apply to animals in any novel environment, the scale and rate of environmental change associated with urban ecosystems is unprecedented (Shochat et al., 2006; Alberti et al., 2017). The effects of urbanisation on wildlife populations vary greatly: while urbanisation has been linked to population declines and local extinctions, some species appear to thrive in urban areas (McKinney, 2006; Lowry et al., 2013). Explaining why some animals adapt to urban environments successfully, while others do not, is a major challenge for ecologists and evolutionary biologists (Sih et al., 2011; Sol et al., 2013). Alongside ecological and life history factors, behavioural plasticity plays a key role in facilitating exploitation of novel environments, including cities, by allowing animals to take advantage of new opportunities and respond appropriately to new threats (Wright et al., 2010; Sol et al., 2013; Ducatez et al., 2020b). Cognitive abilities that determine animals’ ability to acquire, store, and process information from the urban environment are likely to influence the potential for behavioural adjustments. The role of cognition in facilitating or inhibiting adaptation to novel environments has attracted increasing research attention in recent years (Sol et al., 2020; Szabo et al., 2020). In the context of urbanisation, a growing number of studies compare urban-rural differences in cognitive performance and neural architecture (Sol et al., 2005, 2013; Snell-Rood and Wick, 2013; Griffin et al., 2017a). To date, these studies paint a complex picture, highlighting substantial variation within and between species, and much remains to be learned about the potential ecological and evolutionary consequences of cognitive variation in novel environments more generally (Szabo et al., 2020) and in urban environments specifically (Sol et al., 2020). In this review, we argue that our ability to identify the importance of cognition in facilitating city life would be greatly enhanced by identifying the specific informational challenges faced by urban-dwelling animals, how these informational challenges differ from those of the non-urban or ancestral environment, and how specific cognitive processes facilitate (or constrain) the ability of animals to overcome these challenges. First, we discuss the importance of quantifying the informational challenges that animals might face, and the insights that may be gained by comparing the statistical properties of urban habitats with other novel, non-urban habitats. We make specific predictions as to how features of the urban environment may influence the costs and benefits of information gathering, which may aid researchers in formulating testable hypotheses. We then consider the proximate mechanisms driving urban-rural differences in cognitive performance within and between species, and their wider implications. We conclude by outlining promising avenues for future research that offer insights into the effects of urbanisation on evolutionary processes.
When compared to species’ ancestral environments, urban environments are likely to present distinct informational challenges. Like any animal in an evolutionarily novel environment, urban-dwelling animals must solve a similar set of problems including finding food, shelter and breeding sites, and avoiding danger. Gathering information from the environment reduces uncertainty (Dall et al., 2005), and it is therefore expected that cognitive mechanisms that facilitate the acquisition and use of information for decision-making are favoured where they allow animals to flexibly adjust their behaviour to novel ecological conditions (Sol et al., 2013; Szabo et al., 2020). However, the specific informational challenges encountered in novel environments, and the role of cognition in overcoming these challenges, depends on how the new environment differs from the ancestral environment. Due to their artificial nature and rapid expansion, urban environments have the potential to generate a high degree of mismatch between current conditions and the conditions animals previously experienced over their lifetime and/or evolutionary history (Shochat et al., 2006; Sih et al., 2011; Sol et al., 2020). Urban habitats expose animals to a suite of novel stimuli and information sources including artificial foods and structures, people, pets, and traffic (Legagneux and Ducatez, 2013; Sol et al., 2013; Goumas et al., 2020b), where ecological changes may occur more frequently and over shorter timescales than in non-urban habitats (Shochat et al., 2006; Alberti et al., 2017). While it is somewhat intuitive to infer that exposure to more evolutionarily novel stimuli may result in a greater uncertainty and consequently a greater need for information processing, it is still unclear as to how these multiple sources of information interact to generate the exact informational challenges that urban animals face (Szulkin et al., 2020). Empirical and theoretical studies are often based on generalisations about how urban and rural environments differ, and few studies attempt to quantify these differences directly (Griffin et al., 2017a). Quantifying relevant environmental differences between habitats is a necessary step to identifying the informational challenges faced by animals in novel environments, and for urban wildlife in particular. This information will assist in formulating specific, testable predictions regarding the importance of cognitive abilities in reducing uncertainty around environmental variability.
Habitats differ in terms of their harshness (e.g., food availability and predation risk), complexity, and predictability. However, studies of urban-rural differences can often be contradictory when considering the abundance and distribution of food sources, levels of competition and predation pressure, and the complexity and variability of stimuli in the environment (reviewed in Griffin et al., 2017a). For instance, some studies assume that in urban environments, food is more abundant but unpredictable (e.g., Tryjanowski et al., 2016), while other studies consider the distribution of urban food sources to be more stable in space and time compared to non-urban food sources (e.g., Miranda et al., 2013; Kozlovsky et al., 2017). While these assumptions may be based on the natural history of the species in question, studies rarely attempt to quantify urban-rural habitat differences directly by measuring the statistical properties of the environment (including the mean, variance, and autocorrelation of relevant environmental variables). This is a necessary first step, as the distribution of fitness-relevant stimuli in space and time, and the predictability with which these stimuli are encountered, partly determines the value of information-gathering to predict future conditions (Frankenhuis et al., 2019; Young et al., 2020). Quantifying the statistical properties of the environment is not straightforward, but researchers could begin by identifying the fitness-relevant resources and threats for urban animals, and how these differ from those available in non-urban habitats (Figure 1). Determining the spatial and temporal distribution of fitness-relevant stimuli provides a measure of mean environmental harshness, which may then be compared with similar values obtained from ancestral habitats or other environments of interest (e.g., other novel environments). Comparatively less harsh environments may still present a challenge, if the availability of resources fluctuates unpredictably (Young et al., 2020). Thus, it is also important to calculate the variance in environmental variables of interest over time (Frankenhuis et al., 2019; Young et al., 2020). Is the distribution of fitness-relevant resources more variable, or less variable, compared to non-urban environments? Even in variable environments, current conditions may accurately indicate future conditions if there is high autocorrelation between current and future resource distributions. For example, if the distribution of a resource follows trends or varies seasonally, individuals may still be able to predict future resource availability with some degree of accuracy (Janmaat et al., 2006; Young et al., 2020). Secondly, the timescale over which resources fluctuate has an important influence on the value of information-gathering, depending on whether the statistical properties of the environment (the mean, variance, and autocorrelation of relevant resources/stimuli) varies between generations, or over the lifetime of an individual. The more frequently and abruptly these fluctuations occur, the greater the need for animals to update their knowledge on the statistical properties of the environment (Frankenhuis et al., 2019; Young et al., 2020). Animals can update their knowledge by sampling relevant cues in the environment, provided these cues act as reliable indicators of current and/or future conditions (Frankenhuis et al., 2019). Figure 1 outlines how this framework may be applied to predict the selective pressures acting on specific cognitive abilities that allow animals to identify and learn about relevant stimuli and reduce uncertainty. We focus on two examples that may be relevant to urban-dwelling animals (namely remembering the locations of anthropogenic food sources, and avoiding potentially dangerous encounters with humans), but this framework could be applied to any challenge facing animals in any environment. Indeed, it is vital that the informational challenges associated with urban living are compared to those associated with non-urban habitats, in order to predict differences in selection pressures acting on cognitive traits. It would be particularly interesting to compare the informational challenges of urban habitats to other novel, but non-urban habitats, in order to determine whether particular cognitive abilities confer benefits across contexts (Sol et al., 2020; Szabo et al., 2020).
Figure 1. Two examples of how information on the statistical properties of the urban environment may be used to formulate hypotheses and predictions regarding the importance of cognitive processes in allowing wildlife to cope with the challenges of city life. (A) Do urban environments favour enhanced spatial memory of food sources? (B) Do urban environments favour individual discrimination of dangerous people?
The informational challenge presented by the urban environment will differ depending on the species in question, its evolutionary history, and the resources it requires to survive and reproduce. The statistical properties of the urban environment should therefore be quantified at a relevant spatial and temporal scale, based on detailed knowledge of the natural and life history of the study species (Frankenhuis et al., 2019). Furthermore, cities themselves are not identical; urban areas vary in their size, population density, geography and infrastructure. Landscape heterogeneity within cities influences the distribution of resources and stimuli encountered by urban wildlife, with consequences for ecological and evolutionary processes (Johnson and Munshi-South, 2017; Schell et al., 2020). Moreover, the informational challenge faced by urban species may depend on invasion stage: behavioural traits and cognitive phenotypes that are advantageous in the early stages of urban invasion may be disadvantageous once a population becomes established (Wright et al., 2010; Chapple et al., 2012; Griffin et al., 2017a). For example, attraction to novelty, rapid learning and innovation may facilitate colonisation of urban habitats, but once urban populations become established, these potentially costly behaviours may only persist if favoured by selection, or if non-urban individuals continue to disperse to the area (Griffin et al., 2017a). Human attitudes may also determine the informational challenge faced by urban-dwelling animals. For animals considered to be pests or “nuisance” species, exploiting the opportunities offered by urban habitats carries a risk that may not be experienced by other species that are tolerated or encouraged by humans (Barrett et al., 2018; Goumas et al., 2020b). With a deeper understanding of the likely informational challenges presented by urban environments, we can begin to formulate hypotheses as to how cognitive processes assist in overcoming these challenges.
Gathering information from the environment allows individuals to update predictions regarding current and future environmental conditions, provided that reliable cues are available (Dall et al., 2005; Frankenhuis et al., 2019). Responding appropriately to stimuli is a three-step process. Animals must: (i) perceive the cue and categorise it correctly; (ii) integrate information about the cue with their previous experience; and (iii) respond appropriately (Sih et al., 2011; Greggor et al., 2014). When animals encounter novel stimuli, cognitive mechanisms mediate information processing at each of these stages. Specifically, how individuals respond to stimuli depends on their perception and categorisation of novelty, the specificity of cue recognition, the ability to retrieve memories of prior experience acquired through learning and to apply learned information in new contexts. Consequently, it is expected that individuals or species possessing cognitive abilities that allow them to track and respond flexibly to new information may be more successful in novel or variable environments (Dridi and Lehmann, 2016). This idea is formally captured in the “Cognitive Buffer Hypothesis,” which posits that large brains confer domain-general cognitive abilities that underpin the capacity for behavioural flexibility and innovation favoured in variable environments (Sol, 2009; Sayol et al., 2016; but see Poirier et al., 2020). The Cognitive Buffer Hypothesis has received some support from comparative studies of brain size (e.g., Sol et al., 2005; Sayol et al., 2016), although findings are mixed (Evans et al., 2011; Dale et al., 2015; Ashton et al., 2018b), and it is not always clear how differences in brain size translate to behaviour (Healy and Rowe, 2007; Ducatez et al., 2020b). Comparative studies also suggest that while cognitive abilities may allow some species to thrive in urban environments, other species may thrive due to life history rather than cognitive characteristics (Sayol et al., 2020). Empirical studies have sought to quantify variation in cognitive performance, using inter- and intraspecific comparisons to investigate how animals in novel environments (including urban habitats) differ from those in environments closer to the “ancestral” state. However, the majority of these studies focus on measures of innovation propensity and problem-solving performance (e.g., Griffin and Diquelou, 2015; Ducatez et al., 2017; Preiszner et al., 2017; Chow et al., 2018). While often treated as cognitive processes in themselves, seemingly innovative or problem-solving behaviours are difficult to interpret and are likely to represent the outcome of several different psychological processes, the relative contributions of which remain unclear (Thornton et al., 2014). Moreover, problem-solving and innovative behaviours may reflect physical characteristics such as strength, motor diversity, persistence and motivation, rather than cognitive ability per se (Thornton and Samson, 2012; Griffin and Guez, 2014; van Horik and Madden, 2016). Indeed, many of the empirical studies investigating the cognitive performance of urban exploiters or invasive species have found that persistence, motor diversity or general activity levels best predict performance in the task (e.g., Bezzina et al., 2014; Griffin and Diquelou, 2015; Daniels et al., 2019). Furthermore, studies have yet to establish a direct link between innovation or problem-solving abilities and fitness of animals in urban or otherwise novel environments (Szabo et al., 2020), although the fitness value of these abilities has been investigated in “natural” habitats (e.g., Cole et al., 2012). Given that the cognitive bases of problem-solving and innovation are poorly understood and likely to differ substantially between species and contexts (Thornton and Samson, 2012; Thornton et al., 2014; van Horik and Madden, 2016), there is a need for experimental studies to unpack the cognitive processes involved in innovation and problem-solving, quantify variation in performance in specific cognitive processes such as neophobia, associative learning and generalisation, and investigate the fitness consequences of this variation (Thornton et al., 2014). Quantifying the specific informational challenges faced by urban animals, and how these challenges compare to non-urban contexts, will aid in formulating hypotheses as to the role of specific cognitive processes in reducing uncertainty (Figure 1). In the following sections, we review existing empirical evidence for the role of cognitive processes including classification, recognition, learning, and memory in facilitating behavioural adjustments. These cognitive processes are not mutually exclusive, and we focus on those that have received most research attention. We also focus primarily on studies carried out in urban environments, where the majority of research to date has been conducted on birds (although studies of invasive species in non-urban habitats consider other taxonomic groups, including reptiles, and invertebrates). While birds provide tractable study systems for investigating questions related to urban cognition, studies in a more diverse range of taxa are needed to determine the generality of the observed patterns.
Individuals’ responses to stimuli firstly depend on how the stimulus is recognised or classified, allowing animals to discriminate between cues and associate them with previous experience. Repeated exposure to stimuli creates opportunities for learned categorisation, particularly for stimuli associated with reward (e.g., food) or danger (e.g., predators), and facilitates risk assessment. The exact mechanisms by which cues are recognised varies between species and contexts, depending on the value of classifying cues to different levels of specificity. For example, category-level discrimination has been widely studied in wild animals in the context of social cognition, and many species appear to discriminate between familiar and unfamiliar conspecifics, or between kin and non-kin (Tibbetts and Dale, 2007). Classifying cues in this way may allow urban-dwelling animals to quickly identify which food items are safe to eat, and which foraging locations and people are best avoided. Indeed, urban birds appear to discriminate between litter and other novel objects, suggesting a learned categorisation of litter objects that are associated with food (Greggor et al., 2016a). Another study demonstrated that jackdaws (Corvus monedula), a successful urban exploiter, appear to incorporate expectations of animacy in their assessment of risk: encountering inanimate objects that move triggers wariness and further information-gathering (Greggor et al., 2018).
Categorisation mechanisms also allow animals to assess risk during encounters with humans (Bates et al., 2007; Papworth et al., 2013; McComb et al., 2014; Goumas et al., 2020b). In urban environments, animals are likely to encounter humans more frequently than in rural areas, and only some of these people may pose a threat. It may therefore benefit urban-dwelling animals to categorise “dangerous” people based on shared cues or behaviour, such as gaze direction (e.g., Eason et al., 2006; Carter et al., 2008; Clucas et al., 2013; Bateman and Fleming, 2014; Goumas et al., 2019). Urban animals may also use human behavioural cues in deciding when to interact with potential food items. For example, urban herring gulls (Larus argentatus) preferentially interact with potential food items that have been handled by people (Goumas et al., 2020a). In cases where humans vary in their level of threat, it may be useful to recognise potentially dangerous people at an individual level. Experiments on urban-dwelling species including northern mockingbirds (Mimus polyglottos), American crows (Corvus brachyrhynchos), and jackdaws (C. monedula) show that these birds discriminate between individual people and recognise those that have posed a threat in the past (Levey et al., 2009; Marzluff et al., 2010; Davidson et al., 2015). However, it is not clear to what extent individual-level discrimination of humans is based on “true” individual recognition of a person, where an individual’s cues are integrated with previously acquired information about the unique features of that individual (Tibbetts and Dale, 2007; Proops and McComb, 2012; Wiley, 2013). Instead, animals may be categorising people on the basis of shared cues, or be able to discriminate one person from another but not one among many.
While these examples suggest that the ability to classify stimuli and generalise to future encounters may be useful in urban habitats, it is currently unclear whether urban environments especially favour these abilities. Indeed, the ability to classify cues indicating food or danger is likely to be useful in any environment (e.g., Lee et al., 2016). The specific demands on classification and recognition therefore depend on how the urban environment differs from organisms’ ancestral environment, in terms of the number and diversity of potential stimuli, how frequently these stimuli are encountered, and how reliably they predict events or outcomes. For example, if the urban environment presents species or individuals with a more diverse and/or variable range of stimuli, where stimuli share salient features that reliably indicate food or danger, we might expect that individuals or species that are able to accurately classify relevant cues, and generalise to novel cues on the basis of similar features, will be better able to survive and reproduce. On the other hand, if the relevant cues in animals’ ancestral habitats are similarly diverse, or if the same cues are present in both urban and non-urban habitats, the urban environment may not exert a strong informational challenge by comparison. Quantifying the range of stimuli available to animals in both urban and non-urban habitats, and how frequently stimuli are encountered, is therefore essential to formulating hypotheses as to the importance of these cognitive mechanisms in allowing animals to cope with urban environments. To this end, further research identifying the exact cues used by urban wildlife to categorise people, food and other stimuli, and the specificity of this recognition, would be valuable in understanding how animals learn to exploit anthropogenic resources and avoid danger (Goumas et al., 2020b). For example, some domesticated species attend to human emotional signals during interactions (Müller et al., 2015; Proops et al., 2018) and horses (Equus caballus) have been shown to use this information in future encounters with the person (Proops et al., 2018). Human emotional signals may provide valuable information for urban animals, depending on the reliability of these signals and the frequency with which they are encountered. This possibility has yet to be examined and presents an interesting avenue for future research. In addition, the majority of studies investigating discrimination and recognition abilities in urban-dwelling animals have focused on assessing risk from humans themselves, and further work is needed to examine how classification and recognition processes shape responses to cues in other contexts (such as finding food, shelter, and potential breeding sites).
How animals respond to stimuli is influenced by the extent to which cues differ from those encountered previously. How individuals respond to novelty is important when exploring novel habitats, encountering new foods, and innovating new foraging techniques (Greenberg and Mettke-Hoffman, 2001). Neophobia (avoidance of novelty) and neophilia (attraction to novelty) are considered separate traits, and may work simultaneously to influence individuals’ responses to stimuli (Greenberg and Mettke-Hoffman, 2001); both processes are mediated by cognitive mechanisms including classification, learning, and memory (Greggor et al., 2015). Whether urban environments favour neophobic or neophilic tendencies is under debate: on the one hand, the complexity of urban habitats may favour neophilic behaviour that facilitates the exploitation of novel resources; on the other, neophobia is likely to be useful in avoiding potential threats. Indeed, urban habitats may favour initial neophobia when new stimuli are first encountered, followed by novelty-seeking behaviours after a period of habituation (Greenberg and Mettke-Hoffman, 2001; Griffin et al., 2017a). This complex relationship may explain the mixed findings of empirical studies (reviewed in Griffin et al., 2017a): while some studies find that urban individuals or species are less neophobic than those in rural areas (Greggor et al., 2016a; Ducatez et al., 2017), others find that urban individuals are more neophobic (Audet et al., 2016). Studies of neophilia have shown similar mixed findings (e.g., Miranda et al., 2013; Tryjanowski et al., 2016). While this variation may be partly due to methodological and contextual differences, the findings suggest that the separate payoffs of neophobia and neophilia differ according to species ecology, and highlight the need to examine the exact informational challenges associated with urban environments for the species in question. For instance, the degree of habitat and dietary generalism may influence responses to novel stimuli when the environment becomes urbanised; while specialist species may struggle to cope if their preferred niche is no longer available, generalists may be more likely to interact with and learn about new stimuli by generalising from previous experience (Sol et al., 2013). Differences in socio-ecology may be particularly relevant, if social information facilitates the safe exploration of new resources (e.g., Greggor et al., 2016a, b). It has also been suggested that different novelty responses may be favoured at different stages in the establishment process (Griffin et al., 2017a). In the early stages of urban invasion, attraction to novelty may allow individuals to exploit novel foods; over time, especially if urban invaders become targets for persecution (e.g., pigeons, rats; Barrett et al., 2018), avoidance of novelty may enhance survival (Griffin et al., 2017a). The plasticity of neophobia and neophilia over time and across contexts, and the role of learning in shaping these behaviours, is a key area for future research.
The cognitive processes underlying responses to novelty may also play an important role in innovation. For instance, innovating solutions to a novel problem may involve categorisation of novel stimuli, behavioural inhibition, operant conditioning, and generalisation of solutions from one problem to another (Hauser, 2003). While it is possible that variation in cognitive performance within and between species influences innovation tendencies, there is currently limited evidence to support that innovation is cognitively demanding (Thornton and Samson, 2012). Studies of innovation and problem solving typically rely on either tallies of anecdotal reports, or presentations of a single “novel problem” with a binary outcome. In order to determine how cognitive abilities contribute to variation in innovation propensity, experimental studies are needed to unpack the cognitive components underlying innovative behaviours. This may be achieved by presenting animals with a series of novel problems over several trials, in order to examine the role of instrumental learning, inhibitory control and rule generalisation. For instance, in a series of experimental foraging tasks, meerkats (Suricata suricatta) learned to inhibit their tendency to try to access rewards through the transparent sides of the apparatus, but did not then generalise this rule when presented with new tasks (Thornton and Samson, 2012). An important focus for future research should be to understand the role of inhibition in urban animals’ responses to novel problems, the extent to which urban animals can generalise solutions to future problems, and how these inhibition and generalisation abilities compare to their non-urban counterparts.
It is possible that the nature of spatial information that animals are required to process differs between urban and non-urban habitats (Barrett et al., 2018). For example, spatial memory may be involved in navigating spatially complex urban environments (Maguire et al., 2000; Barrett et al., 2018), and may assist in remembering the locations of food resources (Griffin et al., 2017b; Barrett et al., 2018). Urban infrastructure fragments patches of natural vegetation (McKinney, 2002); depending on the resource requirements of the species in question, and the distribution of these resources, urban animals may need to travel frequently between different habitat patches. On the other hand, if food resources are more abundant in urban areas, or if urban areas represent a more homogenous habitat, the selective pressure on spatial memory may be reduced compared to rural environments where animals may be required to remember specific locations or routes (Kozlovsky et al., 2017; Thompson and Morand-Ferron, 2019). The benefits of spatial memory in urban environments is currently poorly understood, having received comparatively little research attention. The majority of empirical studies to date have tended to compare habitat differences in the spatial abilities of chickadees (Poecile gambeli and Poecile atricapilla), highly specialised scatter-hoarding birds. Studies of chickadee populations along elevation and climatic gradients suggest that harsh environmental conditions select for ecologically-relevant spatial memory abilities (Pravosudov and Clayton, 2002; Freas et al., 2012; Roth et al., 2012) by increasing survival (Sonnenberg et al., 2019). Applying these findings in the context of urban environments again raises the question of whether urban habitats can be considered less harsh than non-urban habitats, reducing the need to remember the locations of cached food; or whether urban habitats represent a harsher environment exerting strong selective pressure on spatial memory. Thompson and Morand-Ferron (2019) found no evidence for variation in caching intensity or spatial memory performance in black-capped chickadees (P. atricapilla) captured along an urban-rural gradient. A common garden experiment by Kozlovsky et al. (2017) also showed that urban and rural mountain chickadees (P. gambeli) did not differ in their caching intensity or spatial memory acquisition, although chickadees from urban areas showed higher retention of spatial information, which suggests that this ability may be useful in remembering the locations of urban food sources. While these studies provide an excellent starting point for the study of spatial memory in urban environments, there is a need to examine the benefits of spatial memory in other species with less specialised foraging ecologies, and in other behavioural contexts such as navigation. Identifying the relevant cues that animals use when dealing with spatial information may yield interesting insights: for example, do the cues that animals attend to as landmarks in natural habitats resemble those that they may encounter in cities? How does the spatial and temporal distribution of food patches differ between urban and non-urban habitats? Do cities represent a more or less homogenous environment? Generating predictions and interpreting behavioural differences between urban and non-urban animals is made more difficult when relying on assumptions about the homogeneity and predictability of urban environments (Griffin et al., 2017a), and studies seeking to quantify environmental differences between urban and non-urban habitats would help in formulating specific hypotheses as to the value of specific cognitive abilities in urban-dwelling animals (Johnson and Munshi-South, 2017; Szulkin et al., 2020).
Episodic memory, the retention and integration of information about what happened in a specific previous event, and when and where it happened (Shettleworth, 2010), may also be useful in allowing urban-dwelling animals to remember predictable events such as rubbish collections (Griffin et al., 2017b; Barrett et al., 2018). Again, whether urban environments favour enhanced episodic memory abilities will depend on the resources required by a given species or individual, and how the spatial and temporal variability of these resources differs from non-urban habitats (Figure 1). While laboratory studies of various species provide growing evidence of episodic-like memory in non-human animals (e.g., Clayton and Dickinson, 1998; Roberts, 2006), studies of wild animals are currently restricted to primates (e.g., Janmaat et al., 2006; Janson, 2016; Trapanese et al., 2019). There is currently no empirical research on the role of episodic memory in the context of urban adaptation (Barrett et al., 2018), although these abilities have been identified in species that also happen to be urban exploiters, such as corvids, and rats (Clayton and Dickinson, 1998; Roberts, 2006; Barrett et al., 2018). The role of episodic memory in enhancing the ability of wildlife to exploit urban habitats is therefore an exciting avenue for future research.
The mechanisms by which animals learn range from non-associative mechanisms such as habituation and sensitisation (whereby responses to stimuli gradually decrease or increase over time, respectively, as a result of repeated exposure); to associative mechanisms including classical and operant conditioning (whereby associations are formed between stimuli, or the outcomes of behaviour; Shettleworth, 2010). These domain-general learning mechanisms are widespread across taxa and underpin behavioural flexibility, innovation and problem-solving (although variation in these behaviours may reflect non-cognitive factors; see Griffin and Guez, 2014; van Horik and Madden, 2016). Learning plays a key role in allowing animals to adapt to changing environments (Brown, 2012): repeated exposure to cues leads animals to tolerate benign cues (habituation) and avoid aversive stimuli (sensitisation; Blumstein, 2016); and associative learning allows animals to flexibly adjust their behaviour in response to opportunities and threats. For example, habituation to human presence may explain why urban wildlife exhibit shorter flight initiation distances than those in rural habitats (Samia et al., 2015; Vincze et al., 2016), although these patterns may also reflect reduced investment in vigilance behaviour (risk allocation; Rodriguez-Prieto et al., 2009; Uchida et al., 2019). While both of these mechanisms are predicted to produce similar outcomes, and may not be mutually exclusive, they can be distinguished by investigating whether increased tolerance extends to unfamiliar threats, and the degree to which tolerance levels vary temporally with the frequency of human disturbance (Rodriguez-Prieto et al., 2009). Learning may also allow urban-dwelling animals to take advantage of novel foraging opportunities provided by humans. For example, long-tailed macaques (Macaca fascicularis) at the Uluwatu Temple in Bali, Indonesia frequently steal inedible items from visitors, and use these items as bartering tokens in exchange for food rewards, the quality of which are based on the value of the stolen item (Leca et al., 2021). Observational and experimental data suggest that this behaviour is learned, with individuals becoming more successful in their bartering interactions with age (although this may be partly due to non-cognitive factors such as physical strength, which influence individuals’ ability to steal higher-value items). Older macaques also showed evidence of payoff maximisation by actively seeking more or higher-quality food rewards in exchange for higher-value items (Leca et al., 2021).
Overall, the question of whether urban environments favour rapid learning has received mixed empirical support. For example, while urban house sparrows (Passer domesticus) learn to tolerate human presence more quickly than those in rural areas (Vincze et al., 2016), Audet et al. (2016) found no significant differences between urban and non-urban Barbados bullfinches (Loxigilla barbadensis) in discrimination or reversal learning speed. In fact, urban mynas (Acridotheres tristis) were slower to acquire a learned discrimination than individuals tested in rural areas, as urban birds spent longer sampling relationships between cues and their outcomes (Federspiel et al., 2017). Similar inter- and intra-specific studies focusing on invasive species have shown similarly mixed findings (Roudez et al., 2008; Bezzina et al., 2014; Kang et al., 2018). This suggests that novel and/or urban environments may not always favour rapid learning as is frequently assumed (Griffin et al., 2017a). In theory, rapid learning should be favoured in complex environments that vary predictably over an individual’s lifetime (Dunlap and Stephens, 2009); the extent to which this applies to urban environments is currently unknown as the complexity, variability and predictability of stimuli and events are rarely quantified. Studies investigating learning in urban environments should therefore determine how the informational demands on species vary between urban and non-urban environments, and ideally, how learning performance influences survival and/or reproductive success in different habitats. Moreover, learning may not always be adaptive: the metabolic costs of learning may generate trade-offs with other traits (Snell-Rood, 2013), and individuals that rapidly learn to exploit anthropogenic resources and habituate to deterrents may come into conflict with humans (Barrett et al., 2018). Understanding the cognitive mechanisms underlying behaviour is therefore crucial to mitigating these conflicts, and may be used to develop effective management strategies (Greggor et al., 2014).
When individual learning is costly, animals may be able to gather information more efficiently via social learning (Dall et al., 2005). Social learning may occur through several different processes, including stimulus enhancement (where an individual is more likely to interact with a stimulus after observing another interacting with the same stimulus) and local enhancement (where individuals are exposed to learning opportunities as a result of visiting locations where others are present; Heyes, 1994; Hoppitt and Laland, 2008). Individuals may also learn via observational conditioning, altering their future behaviour as a result of observing another’s behavioural response to a stimulus (Heyes, 1994; Hoppitt and Laland, 2008). Other social learning processes include imitation (copying of others’ motor actions; Hoppitt and Laland, 2008); and teaching, whereby demonstrators modify their behaviour in order to invoke a change of behaviour in others (Caro and Hauser, 1992; Thornton and Raihani, 2008).
Distinguishing between these processes often presents an experimental challenge, but regardless of the process by which social learning occurs, it is often likely to facilitate foraging decisions, breeding site selection, and predator avoidance. Social learning is hypothesised to be particularly useful in novel or variable environments (Laland, 2004; Brown, 2012), but the exact benefits of social learning will depend on how the statistical properties of the novel environment compare to the ancestral environment (Frankenhuis et al., 2019), and the relative payoffs of individual learning. For example, social learning can facilitate the rapid spread of novel information or behaviours through populations; but may also hinder the emergence of adaptive behaviours if individuals favour outdated social information over the innovation of new solutions (Barrett et al., 2019). While few studies have explicitly investigated the importance of social learning in urban environments, recent empirical work has provided some insight into social learning dynamics in urban species. For example, jackdaws (C. monedula) are more likely to consume a novel food item or approach novel stimuli after seeing a conspecific do so (Greggor et al., 2016a, b). Although the exact processes underpinning this effect are unclear in this case, these findings imply that social facilitation allows these successful urban exploiters to incorporate new foods into their diet. Social transmission experiments by Aplin et al. (2015) demonstrate that wild parids (Parus major) learn novel foraging techniques from each other, suggesting that social learning may also play an important role in allowing these birds to exploit anthropogenic food sources, such as famously opening milk bottles left on doorsteps (Fisher and Hinde, 1949; Aplin et al., 2013). However, there is an urgent need to examine how variation in social learning tendencies influence individual fitness in urban environments, identify the exact learning processes involved, and investigate the role of social structure in determining the transmission of social information.
Social learning may be particularly useful in facilitating antipredator behaviour, allowing individuals to learn about danger without the need for individual encounters with predators that are potentially costly (Griffin, 2004). Social learning is therefore likely to be useful for urban-dwelling species encountering novel threats, including humans. While a number of studies have demonstrated that animals learn socially about novel predators (Griffin, 2004), only a handful of studies to date have examined the importance of social learning in allowing animals to avoid threats encountered in urban environments. For example, common mynas (A. tristis) appear to use social cues to infer danger associated with pest control measures, showing higher wariness of a location after seeing a conspecific being captured in that location (Griffin and Boyce, 2009; Diquelou and Griffin, 2019). Studies of urban corvids also provide evidence of social learning: American crows (C. brachyrhynchos) will gather to mob individual people seen capturing crows; this behaviour persists in future encounters with the person, and appears to spread to other birds not present at the initial capture event (Cornell et al., 2012). A study of wild jackdaws (C. monedula) identified a potential mechanism by which fear of “dangerous” humans may spread through groups. In this study, individual jackdaws modified their behavioural response to an unfamiliar person, depending on whether they had previously heard conspecific alarm calls associated with that person (Lee et al., 2019). In this way, social learning may lead to the emergence of cultural traditions, with individuals in a particular area exhibiting similar preferences for anthropogenic food sources, or similar antipredator responses to locally relevant dangers (Aplin, 2018). A growing body of work provides evidence of animal culture, including in urban environments; however, as yet, no study has demonstrated how culturally-acquired behaviours enhance fitness in the wild (Aplin, 2018).
Some species also use social learning to make breeding decisions. For example, birds have been shown to use social information in deciding where and when to breed (Nocera et al., 2006; Seppänen and Forsman, 2007; Seppänen et al., 2011) and in their choice of nesting material (Guillette et al., 2016). As yet, there has been little work investigating the role of social learning in influencing breeding decisions in urban contexts. The built environment provides an abundance of potential breeding sites for urban wildlife, and an abundance of anthropogenic material with which to build nests. Indeed, the use of anthropogenic materials in nest building has been shown to have fitness consequences (Suárez-Rodríguez et al., 2013, 2017). For example, the use of smoked cigarette butts as nest lining material by birds may reduce the ectoparasite load of nests; on the other hand, they may also be toxic to chicks and adult birds (Suárez-Rodríguez et al., 2013, 2017). Further research investigating the cognitive mechanisms that influence the breeding decisions of urban wildlife would therefore be both interesting and timely.
If socially-acquired information is inaccurate or out of date, social learning may not always be beneficial (Dall et al., 2005). Social learning is therefore predicted to be most valuable in situations where individual learning is costly or unreliable (Laland, 2004; Kendal et al., 2005). Variation in the relative payoffs of social learning generates learning strategies and biases that influence how, when, and from whom individuals learn (Laland, 2004; Rendell et al., 2011). While social learning strategies and biases have been widely studied in various taxa (Coussi-Korbel and Fragaszy, 1995; Laland, 2004; van de Waal et al., 2010; Brown and Laland, 2011; Greggor et al., 2017), the role of social learning strategies in influencing the uptake of anthropogenic food or the development of antipredator behaviour in urban wildlife has yet to be examined. Furthermore, even if socially-acquired information or behaviours benefit individuals in the short-term, they may be detrimental if these behavioural changes carry long-term costs (Mazur and Seher, 2008; Semeniuk and Rothley, 2008; Donaldson et al., 2012; Greggor et al., 2014). In this way, anthropogenic activity may both promote the emergence of new socially-acquired behaviours, and modify existing behaviours (Gruber et al., 2019). Identifying the social learning dynamics at play can therefore help to reduce conflict and effectively manage urban wildlife populations (Greggor et al., 2014; Barrett et al., 2018).
Researchers of animal cognition have long been interested in the extent to which non-human animals perceive cause-effect relationships. An understanding of the causal contingencies between stimuli and events is hypothesised to be useful in a wide range of contexts. For example, perceiving relationships between objects may allow animals to infer their stability on surfaces, anticipate the exertion required to interact with them (Jelbert et al., 2019), and possibly allow animals to use objects in the environment to exploit inaccessible food (Rutz et al., 2010). Despite attracting a great deal of research interest, the study of causal understanding in non-human animals is highly controversial (Penn et al., 2008); often, the extent to which observed behaviours require causal understanding is unclear, and the potential fitness consequences have yet to be investigated. However, some laboratory studies suggest that a few species may demonstrate causal reasoning in certain contexts: for instance, rats (Rattus norvegicus) infer the correct motor action required to bring about previously observed events (Blaisdell et al., 2006); great apes use visual and auditory cues to infer the location of hidden food (Bräuer et al., 2006); jays (Garrulus glandarius); and rooks (Corvus frugilegus) perceive support relationships between inanimate objects (Bird and Emery, 2010; Davidson et al., 2017); and New Caledonian crows (Corvus moneduloides) appear to infer the weight of objects based on their movement in the breeze (Jelbert et al., 2019). Understanding the functional properties of objects and their contingencies has also been argued to facilitate the development of object-oriented behaviours such as tool use (Taylor et al., 2009; Gumert and Malaivijitnond, 2013; St Clair and Rutz, 2013). However, tool use does not necessarily require causal understanding (Teschke et al., 2011, 2013), and whether causal understanding plays a role in any form of animal tool use is contested (Biro et al., 2013; Teschke et al., 2013). Moreover, little is known about how ideas about object relationships develop over time (Meulman et al., 2013; Greggor et al., 2018) and are generalised across contexts (Davidson et al., 2017). Ontogenetic and life history factors may play an important role by determining the time available for skill development, as well as opportunities for individual and/or social learning (Humle et al., 2009; Sargeant and Mann, 2009; Fragaszy et al., 2013; Meulman et al., 2013).
If causal learning and other physical cognition abilities assist animals in interacting with objects in the environment, these abilities may be beneficial for urban animals that must learn about novel stimuli and the relationships between them. However, examples in urban contexts are currently scant, and the role of causal learning in facilitating urban living remains speculative. Another interesting question concerns whether animals perceive humans as causal agents. A causal understanding of the actions of humans may allow animals to attend to relevant cues and generalise “rules” across contexts, helping to generate appropriate behaviour more efficiently than would be achieved through other mechanisms (e.g., associative learning). Experiments in New Caledonian crows (C. moneduloides) and New Zealand robins (Petroica longipes) have investigated the possibility that humans are perceived as causal agents by measuring birds’ latency to feed at locations where humans were either capable or incapable of disrupting foraging (Taylor et al., 2012; Garland and Low, 2016). However, methodological considerations have cast doubt on the reliability of the findings of these studies (Dymond et al., 2013; Boogert et al., 2013a). As the evidence for causal learning in non-human animals is currently limited, empirical studies conducted in urban animals have the potential to contribute knowledge to this hotly-debated area of the field, as well as shed light on how causal learning abilities allow animals to navigate the challenges of urban environments.
In cases where urban and non-urban animals differ in the way they process information or perform particular cognitive tasks, the next question is: how do these differences arise? Firstly, individuals may develop relevant cognitive skills as a result of moving to an urban area (behavioural plasticity). Equally, individuals with particular cognitive skills may be more likely to select urban habitats in the first place (non-random sorting). Thirdly, selection pressures in urban environments may favour individuals exhibiting particular cognitive skills that enhance survival and/or reproductive success (Figure 2; Sol et al., 2013). These three hypotheses are not mutually exclusive, and distinguishing between them presents a theoretical and empirical challenge. The picture is further complicated by the possibility that different cognitive abilities are useful at different stages of urban invasion: cognitive skills that are useful when animals first disperse to an urban habitat may become detrimental once the population becomes established, with this change occurring over several generations or within the lifetime of an individual (Griffin et al., 2017a). Whether urban species invest in cognitive solutions may also depend on their life history. The value of learning, for example, is hypothesised to be higher for long-lived species that have more opportunities to adjust their behaviour according to environmental conditions, whereas for shorter-lived species the time and metabolic costs of learning may outweigh the benefits (Maspons et al., 2019). Other aspects of species ecology are also likely to influence the probability of animals settling in urban habitats, and the payoffs of investing in information gathering and its associated neural architecture. For instance, urban development may better support generalist species that are able to exploit more commonly-found resources (Evans et al., 2011). Migratory tendency may also play a role: while long-distance migrants possess cognitive adaptations to deal with predictable variation in the environment, their relatively low behavioural flexibility may mean that these individuals may be at a disadvantage when faced with the rapid and unpredictable changes brought about by human activity (Mettke-Hofmann, 2017; but see Evans et al., 2011). As highlighted in previous sections, a growing body of work provides important insights into urban-rural differences in behaviour and cognitive performance, but further work is needed to determine how these differences arise. We argue that by identifying the specific cognitive mechanisms underlying the observed behavioural differences, we can better understand how cognitive variation influences fitness in urban environments. Once the relevant cognitive process(es) have been established, we could build on this knowledge to examine how cognitive abilities develop over individual lifetimes in urban and rural habitats; the extent to which cognitive performance is heritable; and the fitness consequences of cognitive variation.
Figure 2. Factors influencing cognitive variation in urban and non-urban habitats, and their consequences for ecological and evolutionary processes.
While urban environments may favour enhanced learning and problem-solving abilities in theory, the costs associated with urban life may also impair cognitive performance (Griffin et al., 2017b). For example, diet has an important influence on cognitive development (e.g., Pravosudov et al., 2005; Kitaysky et al., 2006) and studies of urban birds suggest that nutritional constraints may partly explain the general trend for lower reproductive success in urban habitats (Sumasgutner et al., 2014; Biard et al., 2017; Meyrier et al., 2017; Seress et al., 2020). Taken together, these findings imply that poor-quality food in urban habitats could impair the cognitive development of growing chicks (e.g., Arnold et al., 2007), but this possibility has yet to be tested explicitly. Urban diets could also affect cognitive development indirectly through its effects on the gut microbiome (Davidson et al., 2018): indeed, recent experimental evidence suggests a role for gut microbiome variation in mediating problem-solving performance in wild great tits (P. major; Davidson et al., 2020). Secondly, the prevalence and diversity of parasites may differ between urban and rural habitats, with potential implications for cognitive performance. A recent review by Ducatez et al. (2020a) outlines the potential feedbacks between parasitism and host cognition: through its effects on behaviour, cognition may increase or decrease an individual’s exposure to parasites (e.g., the exploitation of novel food sources may expose hosts to new parasites; hosts may develop innovative behaviours that reduce parasite load), and parasite infection may have a direct influence on host cognitive performance. However, evidence to date remains largely correlative, and studies have yet to demonstrate a direct role for cognition in influencing host exposure or susceptibility to parasites. In addition, feedbacks between cognition and parasitism may be mediated by other traits such as sociality, life history and immunocompetence. Distinguishing between these causal and non-causal links will be necessary to fully understand the interactions between parasites and cognition (Ducatez et al., 2020a).
Finally, cognitive performance may be impaired through exposure to pollutants in urban environments, including light, noise (Potvin, 2017), and heavy metals (Burger and Gochfeld, 1993; Burger, 1998; Zhong et al., 2010). For example, laboratory experiments have shown that living in a constant-light environment impairs song acquisition in zebra finches (Taeniopygia guttata), as well as performance in spatial and colour association tasks (Jha and Kumar, 2017). Similar experiments in house crows (Corvus splendens) have identified that constant-light conditions inhibit performance in a spatial memory task by reducing neuronal activation in relevant areas of the brain (Taufique et al., 2019). In contrast, a study of peafowl (Pavo cristatus) found no effect of artificial night-time light on problem-solving performance, but as discussed by the authors of the study, subjects were only exposed to artificial night-time light over a short period and longer-term studies are needed before reliable conclusions can be drawn (Yorzinski et al., 2017). Light and noise pollution may impair cognitive function through several potential mechanisms, including disrupting sleep patterns and the circadian rhythms governing cognitive performance (Krishnan and Lyons, 2015; Yorzinski et al., 2017; Aulsebrook et al., 2020; Connelly et al., 2020). While studies have demonstrated the negative effects of disrupted sleep on cognitive function (Alkadhi et al., 2013; Vorster and Born, 2015), the extent to which urban animals experience sleep disruption, and the consequences for cognitive performance, are currently unknown. Noise pollution may also affect performance in cognitive tasks by reducing the ability of urban animals to perceive and attend to acoustic signals, and thereby influence opportunities for learning (Potvin, 2017). If noise pollution in urban environments reduces the efficacy of acoustic signals, animals may fail to respond appropriately to relevant stimuli in future encounters, simply through lack of experience. It has been suggested that noise may also impair cognitive performance through its effects on the stress response; either directly if noise is perceived as a stressor (Afarinesh et al., 2018) or indirectly (e.g., through nutritional deficits arising from disruption of parental care in early life; Schroeder et al., 2012; Potvin and MacDougall-Shackleton, 2015). While potential interactions between noise pollution, stress and cognitive performance are intriguing, more work is required before these links can be established (Potvin, 2017). In turn, cognitive processes can help overcome the detrimental effects of noise by facilitating behavioural adjustments, promoting adaptation to noisy environments (Halfwerk and Slabbekoorn, 2009; Potvin and Mulder, 2013), and/or aiding spatial or temporal avoidance of noisy areas (Fuller et al., 2007; Arroyo-Solís et al., 2013). The role of cognition in facilitating responses to noise and other pollutants is an area of growing empirical interest, and much remains to be explored. To this end, identifying the specific cognitive mechanisms involved would be a useful starting point; and further research is needed on the complex feedbacks between cognition and ecology (including food availability, parasitism, and pollution), in order to fully understand the cognitive challenges of life in urban environments.
Explaining differences in cognitive performance between urban and non-urban habitats is not straightforward, as environmental conditions and individual-level factors generate within-population variation. Firstly, seasonal alterations in behaviour may influence the stimuli that individuals encounter and opportunities for learning. For example, jackdaws (C. monedula) are more likely to join a conspecific in foraging next to a potentially threatening object during the breeding season when parental care demands are high (Greggor et al., 2016b). Seasonal variation in selection pressures may also bias the processing of fitness-relevant information: female starlings (Sturnus vulgaris) exhibit differences in neuronal activity in response to sexual and non-sexual signals depending on the time of year (Cousillas et al., 2013). Secondly, environmental conditions during the early life of urban animals may influence subsequent learning dynamics. Elevated levels of developmental stress appear to alter social learning processes in later life (e.g., Boogert et al., 2013b; Farine et al., 2015); interestingly, these differences may not arise as a result of impaired cognitive development, but rather plasticity in how individuals choose to gather social information (Farine et al., 2015; Wascher et al., 2018). For instance, early life stress causes zebra finch chicks to switch from learning from parents to learning from non-kin (Farine et al., 2015).
As a final, and related point, understanding the impacts of urbanisation on cognitive and behavioural phenotypes will require a greater focus on the social environment. It is increasingly clear that the social environment determines opportunities for social learning, and may contribute to generating cognitive variation. In turn, individual variation in cognitive ability and knowledge has the potential to determine social network position, the formation of social relationships, and access to information and learning opportunities (Wascher et al., 2018; Kulahci and Quinn, 2019). Although social learning occurs in non-social species (Wilkinson et al., 2010), highly social species have greater access to social information (Greggor et al., 2017). Therefore, rapid social transmission of urban-adapted behaviours may be more likely to occur in species that have a high propensity for social learning in other contexts. Social information transmission is also influenced by the size and structure of social groups that individuals have access to Coussi-Korbel and Fragaszy (1995); Laland (2004); Chapman et al. (2008); Ashton et al. (2019). The effects of the social environment may also extend beyond social learning to other cognitive processes: current research suggests that individuals living in larger groups may perform better in domain-general cognitive tasks such as discrimination learning and spatial memory (e.g., Ashton et al., 2018a; Langley et al., 2018). While the mechanisms generating this effect are not well understood (see Ashton et al., 2018b), it may be that larger groups generate cognitive challenges, such as the need to track multiple relationships, and/or provide a greater diversity of individuals to learn from. Larger groups may also allow animals to reduce vigilance and engage more with learning opportunities (Roberts, 1995), and increased competition could favour innovation of alternative behavioural strategies (Thornton and Samson, 2012; see Langley et al., 2018 for a discussion). Examining the extent to which urbanisation modifies the size and structure of social groups, and the subsequent implications for information transmission and cognitive development, is a clear priority for future research.
At an individual level, factors including sex, age and personality generate learning biases that influence learning performance and attention to stimuli. Several studies have shown that juveniles generally perform less well in cognitive tasks compared to adults (Ono et al., 2002; Thornton and Lukas, 2012; Ushitani et al., 2016; Ashton et al., 2018a), and variation between males and females may arise if the cognitive ability in question has different fitness consequences for each sex (Vallortigara, 1996; Laland and Reader, 1999; Jonasson, 2005; Cauchoix et al., 2018). Personality (consistent inter-individual differences in behaviour) plays an important role in determining responses to risk (Bell et al., 2009; Sih et al., 2012), and is therefore likely to influence the stimuli that individuals encounter and learn about (Sih and Del Giudice, 2012). The interaction between cognition and personality is complex (Dougherty and Guillette, 2018), but initial empirical studies suggest that personality may play an important role in influencing cognitive performance in urban environments (Barnett et al., 2013; Miranda et al., 2013; Wat et al., 2020). When investigating cognitive performance in urban-dwelling animals, studies should take into account the effects of season, social environment and individual differences in generating within-population variation in information processing, by quantifying the repeatability of behaviour over time and across contexts (Cauchoix et al., 2018).
Variation in cognitive phenotypes, both within and between species, has implications for the evolution of behaviour in urban environments. Human activity drives phenotypic changes in urban wildlife through disturbance, habitat modification and altering biotic interactions (Alberti et al., 2017). These phenotypic changes affect physiology and morphology (Hendry et al., 2008; Johnson and Munshi-South, 2017), as well as behaviour (e.g., Miranda et al., 2013; Mueller et al., 2013; Van Dongen et al., 2015). Despite this growing interest in genetic adaptation to urban environments, it is an emerging field (Johnson and Munshi-South, 2017) and as yet, no research has investigated the potential for cognitive adaptation (Sol et al., 2020). This is an exciting area for future work, as cognitive processes may allow populations to shift toward adaptive peaks and potentially reduce the speed of genetic adaptation (Price et al., 2003; Griffin et al., 2017b; Sol et al., 2020).
As well as its effects on genetic evolution, animal cognition has implications for the evolution of culture. Social learning dynamics can influence the emergence of culturally-transmitted traditions, where traits or behaviours are shared by animals living in the same geographic area (Whiten et al., 2017). Cultures have been identified in a range of non-human animals to date, including insects, mammals, and birds (Whiten et al., 2017). Culture evolves in a manner analogous to genetic evolution, and shares common core principles: cultural information is transmitted between individuals; variation arises as a result of innovation and copying errors; and natural and sexual selection act on culturally-acquired behaviours (Mesoudi et al., 2004; Aplin, 2018). Functional behaviours that are culturally acquired may enhance fitness (e.g., Slagsvold and Wiebe, 2011; Aplin et al., 2015), although direct empirical evidence for these fitness consequences is currently limited (Aplin, 2018). Moreover, socially-acquired behaviours have the capacity to alter aspects of organisms’ physical and social environment, create new selective forces and relax or intensify existing selection pressures (Whitehead et al., 2019). In variable environments, cultural transmission of behaviour can facilitate rapid adaptation where genetic evolution might occur more slowly, or facilitate a shift from one adaptive peak to another. On the other hand, if environmental conditions change slowly over time, social transmission of behaviour can erode phenotypic differences between individuals and slow the pace of genetic evolution (Borenstein et al., 2006; Whitehead et al., 2019). If socially-acquired behaviours fail to track environmental variability, reliance on social learning may also lead to the spread of maladaptive behaviours and reduce rates of behavioural innovation (Barrett et al., 2019). The interplay between genetic and cultural evolution is relatively unexplored, but in some cases culturally-acquired behaviours may influence the evolution of morphological and physiological adaptations (Foote et al., 2016). For example, it has been suggested that dietary traditions of killer whales (Orcinus orca) may have favoured the evolution of digestive adaptations for dealing with different types of prey (Foote et al., 2016; Whitehead et al., 2019). From the point of view of this review, of particular interest is the potential feedback between culture and the evolution of cognition (van Schaik and Burkart, 2011; Lotem et al., 2017). For instance, some authors suggest that a reliance on social learning may drive concurrent changes in brain size and neural architecture that facilitate the acquisition and processing of cultural information (Forss et al., 2016; Street et al., 2017). While recent studies have identified a growing number of cultural behaviours in urban-dwelling animals (e.g., Cornell et al., 2012; Aplin et al., 2015), much remains to be learned about cultural transmission in urban environments, how culturally-acquired behaviours enhance fitness, and facilitate morphological, physiological, and cognitive adaptation.
Urbanisation is a relatively recent phenomenon in evolutionary time, but has the potential to increase or reduce gene flow between populations or bring together formerly isolated species. In theory, this could lead to population divergence over time (Bull and Maron, 2016; Alberti et al., 2017; Johnson and Munshi-South, 2017) and the emergence of new species if populations become reproductively isolated (e.g., Byrne and Nichols, 1999). Cognitive processes such as learning can play a role in promoting or inhibiting reproductive isolation between populations under certain ecological conditions (Verzijden et al., 2012; Whitehead et al., 2019). For example, social learning of mate preferences and sexual displays has the potential to enhance or limit the probability of two populations becoming reproductively isolated (Grant and Grant, 2010; Verzijden et al., 2012; Alberti et al., 2017). In an urban context, this may occur if traits or behaviours beneficial in urban environments form a component of mate choice, or if selection on learning in other behavioural contexts is carried over to mate selection (Verzijden et al., 2012). This process could also occur indirectly, via effects on demography. Urban environments alter the social and ecological conditions under which mate choice occurs, influencing encounter rates between males and females and opportunities for learning (Verzijden et al., 2012). Likewise, if urban animals must adjust their sexual displays to cope with disturbance (such as birds adjusting their vocalisations to reduce the impacts of traffic noise; Halfwerk and Slabbekoorn, 2009; Potvin, 2017), this may influence mate choice and/or dispersal to and from cities, with potential consequences for gene flow. Whilst intriguing, there is currently limited evidence for the role of learning in speciation (Sorenson et al., 2003; Lipshutz et al., 2017; Lamichhaney et al., 2018), and its importance in the context of urbanisation has yet to investigated.
If urban-rural differences in cognitive performance (and the resulting behavioural differences) are heritable and affect fitness, this may have knock-on effects at a wider ecosystem level (Alberti et al., 2017). Behavioural changes have the potential to affect population dynamics and community composition, influencing predator-prey relationships, host-parasite relationships, and competitive interactions (Schlesinger et al., 2008; Tuomainen and Candolin, 2011; Pirotta et al., 2018). For example, cultural learning in one species may drive genetic evolution in other species (reviewed in Whitehead et al., 2019). For instance, socially-learned foraging preferences in parids alter the selection pressures on prey species, favouring aposematism over crypsis (Thorogood et al., 2018). Of particular relevance in urban environments is the influence of human culture on the genetic evolution of non-human species. As already discussed, human attitudes toward urban wildlife, which are likely to reflect culturally transmitted social norms, may influence the selection pressures acting on animal behaviour and cognition (Barrett et al., 2018). These selection pressures may also extend to other traits: for example, the tendency of humans to feed urban birds, a culturally-acquired behaviour, appears to have altered selection pressures on bill morphology in house finches (Carpodacus mexicanus; Badyaev et al., 2008). These community-level changes may, in turn, influence ecosystem processes including nutrient cycling and primary productivity (Matthews et al., 2011). Further research is needed to determine how selection on cognitive processes in urban environments influences species interactions and community structure, and the wider effects at the ecosystem level.
Understanding how cognitive abilities allow animals to navigate novel environments, and urban environments in particular, would be greatly enhanced by identifying the specific psychological mechanisms involved in generating behavioural changes. Urbanisation exposes wildlife to widespread, rapid ecological change that is evolutionarily unprecedented (Shochat et al., 2006; Sol et al., 2020). While cognitive abilities play a role in generating appropriate behavioural responses, the importance of cognition will vary depending on the degree of environmental mismatch. Is life in the city more cognitively demanding than life in more “natural” environments, or other types of novel habitat? Quantifying the specific informational challenges faced by urban-dwelling animals, and how these challenges differ from those faced by their non-urban counterparts, is key to determining the fitness relevance of such abilities, and would provide vital information from which to develop testable hypotheses and predictions. Quantifying informational challenges is not straightforward, but valuable insights may be gained from measuring the statistical properties of the environment and may shed light on the current lack of agreement between studies (see Frankenhuis et al., 2019; Young et al., 2020 for a discussion). Theoretical models would be very useful to generate predictions regarding the selective pressures acting on different cognitive abilities under different resource distributions. Empirical studies would be required to validate these models, and could begin by quantifying the distribution of fitness-relevant stimuli in time and space, and the rate at which animals encounter these stimuli in different habitats. Field studies in cognitive ecology rarely gather this information, and we recognise that it is an ambitious task. However, advancements in remote sensing, data logging and other technologies allow ecologists to map the distribution of resources at fine spatial and temporal scales (Szulkin et al., 2020). By collaborating across disciplines and sharing data, there is great potential to further investigate the role of information use in evolutionary processes.
Cognitive ecologists should also seek to identify the relevant features of stimuli utilised by urban animals, the extent to which these stimuli share characteristics with stimuli in non-urban environments, and the perceptual or cognitive processes that constrain or enable animals to generalise from their past experience of similar stimuli. The stimuli used by urban animals, and the relevant spatial and temporal scales over which these stimuli vary, will differ between species depending on ecological and life history factors. These studies should therefore be carried out on a wide range of taxa, with hypotheses and predictions grounded in the natural history of the species in question. This is particularly important given that studies of urban cognition currently show a strong taxonomic bias toward birds; further studies in other urban invaders, such as foxes, rats, raccoons and primates, are urgently needed (e.g., Daniels et al., 2019; Leca et al., 2021). Moreover, further study is needed within urban populations to explore how and why individuals vary in their cognitive performance. This requires ecologically-relevant tests of cognitive performance, repeated within and between individuals and populations (Boogert et al., 2018; Cauchoix et al., 2018). To this end, focusing on clearly defined cognitive processes may avoid some of the difficulties in interpreting apparently innovative or problem-solving behaviours (Thornton et al., 2014; van Horik and Madden, 2016; Shaw and Schmelz, 2017). Experimental studies may also allow researchers to tease apart the cognitive components of innovation and problem-solving (e.g., Thornton and Samson, 2012). Accompanying data on survival and reproductive success are required to understand the fitness consequences of cognitive variation.
Identifying the mechanisms underlying urban-rural variation in behaviour may be achieved through longitudinal studies investigating how cognitive performance changes over the lifetime of the individual, and the heritability of cognitive performance. At the population level, investigating how cognitive abilities vary over time is important to distinguish between the potential mechanisms underlying urban-rural differences in cognitive processing (plasticity, non-random sorting, and selection) and the value of cognitive abilities at various stages in the establishment process (Griffin et al., 2017a). More work is also needed to investigate how urban environments impair cognition, as well as select for particular cognitive abilities; and the knock-on effects for populations, communities and the urban ecosystem (Box 1). Long-term field studies, carried out on multiple species across multiple habitats would be especially valuable. Again, this is an ambitious task, but many such studies already exist around the world. Collaborating and pooling data would provide valuable insights into cognitive evolution, generate new questions and provide possibilities for answering other questions in behavioural and urban ecology.
BOX 1 | Outstanding questions for future research.
• What are the informational demands associated with living in urban environments?
• How do these informational challenges compare to those encountered in non-urban habitats, and how to they vary with species ecology?
• How do cognitive processes including responses to novelty, classification, recognition, learning and memory allow animals to overcome the informational challenges associated with urban environments?
• How and why do individuals vary in their cognitive abilities, and how does this variation influence survival and reproductive success in urban environments?
• How does this variation arise (e.g., through plasticity, non-random sorting and/or natural selection)?
• How do urban environments impair cognitive function and development?
• Does urbanisation influence the size and structure of social groups, and what are the knock-on effects for cognitive and behavioural phenotypes?
• How does cognition influence the rate of genetic evolution in urban environments?
• How does cognition influence the establishment of cultural traditions in urban environments, and do these traditions evolve over time?
• How do genetic and cultural processes interact to influence the rate of urban adaptation?
• Does cognition play a role in driving speciation in urban environments?
• How do cognitively-driven behavioural changes influence wider processes in urban ecosystems?
VL wrote the manuscript with input and guidance from AT. Both authors contributed to the article and approved the submitted version.
VL was supported by a Ph.D. studentship from the Natural Environment Research Council (NE/L002434/1). AT was funded by a BBSRC David Phillips Fellowship (B/H021817/2) and the Human Frontiers Science Program (Grant No. RG0049/2017).
The authors declare that the research was conducted in the absence of any commercial or financial relationships that could be construed as a potential conflict of interest.
We would like to thank the Editor and two reviewers for their helpful and constructive feedback on the manuscript.
Afarinesh, M. R., Akhtardanesh, B., Haghpanah, T., Golshan, F., Meftahi, G. H., Ghanbarpour, N., et al. (2018). Urban traffic noise pollution disturbs spatial learning and memory and increases anxiety-like behavior in adult male rats. Physiol. Pharmacol. 22, 146–154.
Alberti, M., Marzluff, J., and Hunt, V. M. (2017). Urban driven phenotypic changes: empirical observations and theoretical implications for eco-evolutionary feedback. Philos. Trans. R. Soc. B Biol. Sci. 372:29. doi: 10.1098/rstb.2016.0029
Alkadhi, K., Zagaar, M., Alhaider, I., Salim, S., and Aleisa, A. (2013). Neurobiological consequences of sleep deprivation. Curr. Neuropharmacol. 11, 231–249. doi: 10.2174/1570159x11311030001
Aplin, L. M. (2018). Culture and cultural evolution in birds: a review of the evidence. Anim. Behav. 147, 179–187. doi: 10.1016/j.anbehav.2018.05.001
Aplin, L. M., Farine, D. R., Morand-Ferron, J., Cockburn, A., Thornton, A., and Sheldon, B. C. (2015). Experimentally induced innovations lead to persistent culture via conformity in wild birds. Nature 518, 538–541. doi: 10.1038/nature13998
Aplin, L. M., Sheldon, B. C., and Morand-Ferron, J. (2013). Milk bottles revisited: social learning and individual variation in the blue tit, Cyanistes caeruleus. Anim. Behav. 85, 1225–1232. doi: 10.1016/j.anbehav.2013.03.009
Arnold, K. E., Ramsay, S. L., Donaldson, C., and Adam, A. (2007). Parental prey selection affects risk-taking behaviour and spatial learning in avian offspring. Proc. R. Soc. B 274, 2563–2569. doi: 10.1098/rspb.2007.0687
Arroyo-Solís, A., Castillo, J. M., Figueroa, E., López-Sánchez, J. L., and Slabbekoorn, H. (2013). Experimental evidence for an impact of anthropogenic noise on dawn chorus timing in urban birds. J. Avian Biol. 44, 288–296. doi: 10.1111/j.1600-048X.2012.05796.x
Ashton, B. J., Ridley, A. R., Edwards, E. K., and Thornton, A. (2018a). Cognitive performance is linked to group size and affects fitness in Australian magpies. Nature 554, 364–367. doi: 10.1038/nature25503
Ashton, B. J., Thornton, A., and Ridley, A. R. (2018b). An intraspecific appraisal of the social intelligence hypothesis. Philos. Trans. R. Soc. B 373:20170288.
Ashton, B. J., Thornton, A., and Ridley, A. R. (2019). Larger group sizes facilitate the emergence and spread of innovations in a group-living bird. Anim. Behav. 158, 1–7. doi: 10.1016/j.anbehav.2019.10.004
Audet, J. N., Ducatez, S., and Lefebvre, L. (2016). The town bird and the country bird: problem solving and immunocompetence vary with urbanization. Behav. Ecol. 27, 637–644. doi: 10.1093/beheco/arv201
Aulsebrook, A. E., Connelly, F., Johnsson, R. D., Jones, T. M., Mulder, R. A., Hall, M. L., et al. (2020). White and amber light at night disrupt sleep physiology in birds. Curr. Biol. 30, 3657–3663.e5. doi: 10.1016/j.cub.2020.06.085
Badyaev, A. V., Young, R. L., Oh, K. P., and Addison, C. (2008). Evolution on a local scale: Developmental, functional, and genetic bases of divergence in bill form and associated changes in song structure between adjacent habitats. Evolution 62, 1951–1964. doi: 10.1111/j.1558-5646.2008.00428.x
Barnett, C., Salter, M., Chevallier, C., Robertson, N., Berard, O., and Burns, K. C. (2013). The ability of north island robins to discriminate between humans is related to their behavioural type. PLoS One 8:e064487. doi: 10.1371/journal.pone.0064487
Barrett, B., Zepeda, E., Pollack, L., Munson, A., and Sih, A. (2019). Counter-culture: does social learning help or hinder adaptive response to human-induced rapid environmental change? Front. Ecol. Evol. 7:183. doi: 10.3389/fevo.2019.00183
Barrett, L. P., Stanton, L., and Benson-Amram, S. (2018). The cognition of ‘nuisance’ species. Anim. Behav. 147, 167–177. doi: 10.1016/j.anbehav.2018.05.005
Bateman, P. W., and Fleming, P. A. (2014). Does human pedestrian behaviour influence risk assessment in a successful mammal urban adapter? J. Zool. 294, 93–98. doi: 10.1111/jzo.12156
Bates, L. A., Sayialel, K. N., Njiraini, N. W., Moss, C. J., Poole, J. H., and Byrne, R. W. (2007). Elephants classify human ethnic groups by odor and garment color. Curr. Biol. 17, 1938–1942. doi: 10.1016/j.cub.2007.09.060
Bell, A. M., Hankison, S. J., and Laskowski, K. L. (2009). The repeatability of behaviour: a meta-analysis. Anim. Behav. 77, 771–783. doi: 10.1016/j.anbehav.2008.12.022
Bezzina, C. N., Amiel, J. J., and Shine, R. (2014). Does invasion success reflect superior cognitive ability? A case study of two congeneric lizard species (Lampropholis, Scincidae). PLoS One 9:e0086271. doi: 10.1371/journal.pone.0086271
Biard, C., Brischoux, F., Meillère, A., Michaud, B., Nivière, M., Ruault, S., et al. (2017). Growing in cities: an urban penalty for wild birds? A study of phenotypic differences between urban and rural great tit chicks (Parus major). Front. Ecol. Evol. 5:79. doi: 10.3389/fevo.2017.00079
Bird, C. D., and Emery, N. J. (2010). Rooks perceive support relations similar to six-month-old babies. Proc. R. Soc. B Biol. Sci. 277, 147–151. doi: 10.1098/rspb.2009.1456
Biro, D., Haslam, M., and Rutz, C. (2013). Tool use as adaptation. Philos. Trans. R. Soc. B Biol. Sci. 368:408. doi: 10.1098/rstb.2012.0408
Blaisdell, A. P., Sawa, K., Leising, K. J., and Waldmann, M. R. (2006). Causal reasoning in rats. Science 311, 1020–1022. doi: 10.1126/science.1121872
Blumstein, D. T. (2016). Habituation and sensitization: new thoughts about old ideas. Anim. Behav. 120, 255–262. doi: 10.1016/j.anbehav.2016.05.012
Boogert, N. J., Arbilly, M., Muth, F., and Seed, A. M. (2013a). Do crows reason about causes or agents? The devil is in the controls. Proc. Natl. Acad. Sci. U.S.A. 110:E273. doi: 10.1073/pnas.1219664110
Boogert, N. J., Zimmer, C., and Spencer, K. A. (2013b). Pre- and post-natal stress have opposing effects on social information use. Biol. Lett. 9:20121088. doi: 10.1098/rsbl.2012.1088
Boogert, N. J., Madden, J. R., Morand-Ferron, J., and Thornton, A. (2018). Measuring and understanding individual differences in cognition. Philos. Trans. R. Soc. B 373:20170280. doi: 10.1098/rstb.2017.0280
Borenstein, E., Meilijson, I., and Ruppin, E. (2006). The effect of phenotypic plasticity on evolution in multipeaked fitness landscapes. J. Evol. Biol. 19, 1555–1570. doi: 10.1111/j.1420-9101.2006.01125.x
Bräuer, J., Kaminski, J., Riedel, J., Call, J., and Tomasello, M. (2006). Making inferences about the location of hidden food: social dog, causal ape. J. Comp. Psychol. 120, 38–47. doi: 10.1037/0735-7036.120.1.38
Brown, C. (2012). “Experience and learning in changing environments,” in Behavioural Responses to a Changing World: Mechanisms and Consequences, eds U. Candolin and B. B. M. Wong (Oxford: Oxford University Press), doi: 10.1093/acprof:osobl/9780199602568.003.0004
Brown, C., and Laland, K. (2011). “Social learning in fishes,” in Fish Cognition and Behavior, eds C. Brown, K. Laland, and J. Krause (Hoboken, NJ: Wiley), 240–257. doi: 10.1002/9781444342536.ch11
Bull, J. W., and Maron, M. (2016). How humans drive speciation as well as extinction. Proc. R. Soc. B Biol. Sci. 283:600. doi: 10.1098/rspb.2016.0600
Burger, J. (1998). Effects of lead on sibling recognition in young herring gulls. Toxicol. Sci. 43, 155–160. doi: 10.1006/toxs.1998.2451
Burger, J., and Gochfeld, M. (1993). Lead and behavioral development in young herring gulls: effects of timing of exposure on individual recognition. Toxicol. Sci. 21, 187–195. doi: 10.1093/toxsci/21.2.187
Byrne, K., and Nichols, R. A. (1999). Culex pipiens in London underground tunnels: differentiation between surface and subterranean populations. Heredity 82, 7–15. doi: 10.1038/sj.hdy.6884120
Caro, T., and Hauser, M. D. (1992). Is there teaching in nonhuman animals? Q. Rev. Biol. 67, 151–174.
Carter, J., Lyons, N. J., Cole, H. L., and Goldsmith, A. R. (2008). Subtle cues of predation risk: starlings respond to a predator’s direction of eye-gaze. Proc. R. Soc. B Biol. Sci. 275, 1709–1715. doi: 10.1098/rspb.2008.0095
Cauchoix, M., Chow, P. K. Y., van Horik, J. O., Atance, C. M., Barbeau, E. J., Barragan-Jason, G., et al. (2018). The repeatability of cognitive performance. Philos. Trans. R. Soc. B 373:20170281. doi: 10.1111/ddi.12749
Chapman, B. B., Ward, A. J. W., and Krause, J. (2008). Schooling and learning: early social environment predicts social learning ability in the guppy, Poecilia reticulata. Anim. Behav. 76, 923–929. doi: 10.1016/j.anbehav.2008.03.022
Chapple, D. G., Simmonds, S. M., and Wong, B. B. M. (2012). Can behavioral and personality traits influence the success of unintentional species introductions? Trends Ecol. Evol. 27, 57–64. doi: 10.1016/j.tree.2011.09.010
Chow, P. K. Y., Lurz, P. W. W., and Lea, S. E. G. (2018). A battle of wits? Problem-solving abilities in invasive eastern grey squirrels and native Eurasian red squirrels. Anim. Behav. 137, 11–20. doi: 10.1016/j.anbehav.2017.12.022
Clayton, N. S., and Dickinson, A. (1998). Episodic-like memory during cache recovery by scrub jays. Nature 395, 272–274.
Clucas, B., Marzluff, J. M., Mackovjak, D., and Palmquist, I. (2013). Do American crows pay attention to human gaze and facial expressions? Ethology 119, 296–302. doi: 10.1111/eth.12064
Cole, E. F., Morand-Ferron, J., Hinks, A. E., and Quinn, J. L. (2012). Cognitive ability influences reproductive life history variation in the wild. Curr. Biol. 22, 1808–1812. doi: 10.1016/j.cub.2012.07.051
Connelly, F., Johnsson, R. D., Aulsebrook, A. E., Mulder, R. A., Hall, M. L., Vyssotski, A. L., et al. (2020). Urban noise restricts, fragments, and lightens sleep in Australian magpies. Environ. Pollut. 267:115484. doi: 10.1016/j.envpol.2020.115484
Cornell, H. N., Marzluff, J. M., and Pecoraro, S. (2012). Social learning spreads knowledge about dangerous humans among American crows. Proc. R. Soc. B 279, 499–508. doi: 10.1098/rspb.2011.0957
Cousillas, H., George, I., Alcaix, S., Henry, L., Richard, J.-P., and Hausberger, M. (2013). Seasonal female brain plasticity in processing social vs. sexual vocal signals. Eur. J. Neurosci. 37, 728–734. doi: 10.1111/ejn.12089
Coussi-Korbel, S., and Fragaszy, D. M. (1995). On the relation between social dynamics and social learning. Anim. Behav. 50, 1441–1453. doi: 10.1016/0003-3472(95)80001-8
Dale, S., Lifjeld, J. T., and Rowe, M. (2015). Commonness and ecology, but not bigger brains, predict urban living in birds. BMC Ecol. 15:12. doi: 10.1186/s12898-015-0044-x
Dall, S. R. X., Giraldeau, L.-A., Olsson, O., McNamara, J. M., and Stephens, D. W. (2005). Information and its use by animals in evolutionary ecology. Trends Ecol. Evol. 20, 187–193. doi: 10.1016/j.tree.2005.01.010
Daniels, S. E., Fanelli, R. E., Gilbert, A., and Benson-Amram, S. (2019). Behavioral flexibility of a generalist carnivore. Anim. Cogn. 22, 387–396. doi: 10.1007/s10071-019-01252-7
Davidson, G., Miller, R., Loissel, E., Cheke, L. G., and Clayton, N. S. (2017). The development of support intuitions and object causality in juvenile Eurasian jays (Garrulus glandarius). Sci. Rep. 7, 1–11. doi: 10.1038/srep40062
Davidson, G. L., Clayton, N. S., and Thornton, A. (2015). Wild jackdaws, Corvus monedula, recognize individual humans and may respond to gaze direction with defensive behaviour. Anim. Behav. 108, 17–24. doi: 10.1016/j.anbehav.2015.07.010
Davidson, G. L., Cooke, A. C., Johnson, C. N., and Quinn, J. L. (2018). The gut microbiome as a driver of individual variation in cognition and functional behaviour. Philos. Trans. R. Soc. B Biol. Sci. 373:286. doi: 10.1098/rstb.2017.0286
Davidson, G. L., Wiley, N., Cooke, A. C., Johnson, C. N., Fouhy, F., Reichert, M. S., et al. (2020). Diet induces parallel changes to the gut microbiota and problem solving performance in a wild bird. bioRxiv [Preprint], doi: 10.1101/827741
Diquelou, M. C., and Griffin, A. S. (2019). It’s a trap! invasive common mynas learn socially about control-related cues. Behav. Ecol. 30, 1314–1323. doi: 10.1093/beheco/arz079
Donaldson, R., Finn, H., Bejder, L., Lusseau, D., and Calver, M. (2012). The social side of human-wildlife interaction: wildlife can learn harmful behaviours from each other. Anim. Conserv. 15, 427–435. doi: 10.1111/j.1469-1795.2012.00548.x
Dougherty, L. R., and Guillette, L. M. (2018). Linking personality and cognition: a meta-analysis. Philos. Trans. R. Soc. B 373:20170282. doi: 10.1098/rstb.2017.0282
Dridi, S., and Lehmann, L. (2016). Environmental complexity favors the evolution of learning. Behav. Ecol. 27, 842–850. doi: 10.1093/beheco/arv184
Ducatez, S., Audet, J. N., Rodriguez, J. R., Kayello, L., and Lefebvre, L. (2017). Innovativeness and the effects of urbanization on risk-taking behaviors in wild Barbados birds. Anim. Cogn. 20, 33–42. doi: 10.1007/s10071-016-1007-0
Ducatez, S., Lefebvre, L., Sayol, F., Audet, J. N., and Sol, D. (2020a). Host cognition and parasitism in birds: a review of the main mechanisms. Front. Ecol. Evol. 8:102. doi: 10.3389/fevo.2020.00102
Ducatez, S., Sol, D., Sayol, F., and Lefebvre, L. (2020b). Behavioural plasticity is associated with reduced extinction risk in birds. Nat. Ecol. Evol. 4, 788–793. doi: 10.1038/s41559-020-1168-8
Dunlap, A. S., and Stephens, D. W. (2009). Components of change in the evolution of learning and unlearned preference. Proc. R. Soc. B Biol. Sci. 276, 3201–3208. doi: 10.1098/rspb.2009.0602
Dymond, S., Haselgrove, M., and McGregor, A. (2013). Clever crows or unbalanced birds? Proc. Natl. Acad. Sci. U.S.A. 110:E336. doi: 10.1073/pnas.1218931110
Eason, P. K., Sherman, P. T., Rankin, O., and Coleman, B. (2006). Factors affecting flight initiation distance in american robins. J. Wildl. Manag. 70, 1796–1800.
Evans, K. L., Chamberlain, D. E., Hatchwell, B. J., Gregory, R. D., and Gaston, K. J. (2011). What makes an urban bird? Glob. Chang. Biol. 17, 32–44. doi: 10.1111/j.1365-2486.2010.02247.x
Farine, D. R., Spencer, K. A., and Boogert, N. J. (2015). Early-life stress triggers juvenile zebra finches to switch social learning strategies. Curr. Biol. 25, 2184–2188. doi: 10.1016/j.cub.2015.06.071
Federspiel, I. G., Garland, A., Guez, D., Bugnyar, T., Healy, S. D., Güntürkün, O., et al. (2017). Adjusting foraging strategies: a comparison of rural and urban common mynas (Acridotheres tristis). Anim. Cogn. 20, 65–74. doi: 10.1007/s10071-016-1045-7
Fisher, J., and Hinde, R. A. (1949). The opening of milk bottles by birds. Br. Birds 42, 347–357. doi: 10.1038/1691006a0
Foote, A. D., Vijay, N., Ávila-Arcos, M. C., Baird, R. W., Durban, J. W., Fumagalli, M., et al. (2016). Genome-culture coevolution promotes rapid divergence of killer whale ecotypes. Nat. Commun. 7:11693. doi: 10.1038/ncomms11693
Forss, S. I. F., Willems, E., Call, J., and van Schaik, C. P. (2016). Cognitive differences between Orang-Utan species: a test of the cultural intelligence hypothesis. Sci. Rep. 6, 1–12. doi: 10.1038/srep30516
Fragaszy, D. M., Biro, D., Eshchar, Y., Humle, T., Izar, P., Resende, B., et al. (2013). The fourth dimension of tool use: temporally enduring artefacts aid primates learning to use tools. Philos. Trans. R. Soc. B Biol. Sci. 368:20120410. doi: 10.1098/rstb.2012.0410
Frankenhuis, W. E., Nettle, D., and Dall, S. R. X. (2019). A case for environmental statistics of early-life effects. Philos. Trans. R. Soc. B Biol. Sci. 374:20180110. doi: 10.1098/rstb.2018.0110
Freas, C. A., LaDage, L. D., Roth, T. C., and Pravosudov, V. V. (2012). Elevation-related differences in memory and the hippocampus in mountain chickadees, Poecile gambeli. Anim. Behav. 84, 121–127. doi: 10.1016/j.anbehav.2012.04.018
Fuller, R. A., Warren, P. H., and Gaston, K. J. (2007). Daytime noise predicts nocturnal singing in urban robins. Biol. Lett. 3, 368–370. doi: 10.1098/rsbl.2007.0134
Garland, A., and Low, J. (2016). Reasoning about “capability”: wild robins respond to limb visibility in humans. Behav. Sci. 6:15. doi: 10.3390/bs6030015
Goumas, M., Boogert, N. J., and Kelley, L. A. (2020a). Urban herring gulls use human behavioural cues to locate food. R. Soc. Open Sci. 7:1959. doi: 10.1098/rsos.191959
Goumas, M., Lee, V. E., Kelley, L. A., and Thornton, A. (2020b). The role of animal cognition in human-wildlife interactions. Front. Psychol. 11:589978. doi: 10.3389/fpsyg.2020.589978
Goumas, M., Burns, I., Kelley, L. A., and Boogert, N. J. (2019). Herring gulls respond to human gaze direction. Biol. Lett. 15, 20190405. doi: 10.1098/rsbl.2019.0405
Grant, B. R., and Grant, P. R. (2010). Songs of Darwin’s finches diverge when a new species enters the community. Proc. Natl. Acad. Sci. U.S.A. 107, 20156–20163. doi: 10.1073/pnas.1015115107
Greenberg, R., and Mettke-Hoffman, C. (2001). “Ecological aspects of neophobia and neophilia in birds,” in Current Ornithology, eds V. Nolan Jr. and C. F. Thompson (New York, NY: Plenum Publishers), 119–178.
Greggor, A. L., Clayton, N. S., Fulford, A. J. C., and Thornton, A. (2016a). Street smart: faster approach towards litter in urban areas by highly neophobic corvids and less fearful birds. Anim. Behav. 117, 123–133. doi: 10.1016/j.anbehav.2016.03.029
Greggor, A. L., McIvor, G., Clayton, N. S., and Thornton, A. (2016b). Contagious risk taking: social information and context influence wild jackdaws’ responses to novelty and risk. Sci. Rep. 6:27764. doi: 10.1038/srep27764
Greggor, A. L., Clayton, N. S., Phalan, B., and Thornton, A. (2014). Comparative cognition for conservationists. Trends Ecol. Evol. 29, 489–495. doi: 10.1016/j.tree.2014.06.004
Greggor, A. L., McIvor, G. E., Clayton, N. S., and Thornton, A. (2018). Wild jackdaws are wary of objects that violate expectations of animacy. R. Soc. Open Sci. 5:181070. doi: 10.1098/rsos.181070
Greggor, A. L., Thornton, A., and Clayton, N. S. (2015). Neophobia is not only avoidance; improving neophobia tests by combining cognition and ecology. Curr. Opin. Behav. Sci. 6, 82–89. doi: 10.1016/j.cobeha.2015.10.007
Greggor, A. L., Thornton, A., and Clayton, N. S. (2017). Harnessing learning biases is essential for applying social learning in conservation. Behav. Ecol. Sociobiol. 71:16. doi: 10.1007/s00265-016-2238-4
Griffin, A. S. (2004). Social learning about predators: a review and prospectus. Learn. Behav. 32, 131–140. doi: 10.3758/BF03196014
Griffin, A. S., and Boyce, H. M. (2009). Indian mynahs, Acridotheres tristis, learn about dangerous places by observing the fate of others. Anim. Behav. 78, 79–84. doi: 10.1016/j.anbehav.2009.03.012
Griffin, A. S., and Diquelou, M. C. (2015). Innovative problem solving in birds: a cross-species comparison of two highly successful passerines. Anim. Behav. 100, 84–94. doi: 10.1016/j.anbehav.2014.11.012
Griffin, A. S., and Guez, D. (2014). Innovation and problem solving: a review of common mechanisms. Behav. Proces. 109, 121–134. doi: 10.1016/j.beproc.2014.08.027
Griffin, A. S., Netto, K., and Peneaux, C. (2017a). Neophilia, innovation and learning in an urbanized world: a critical evaluation of mixed findings. Curr. Opin. Behav. Sci. 16, 15–22. doi: 10.1016/j.cobeha.2017.01.004
Griffin, A. S., Tebbich, S., and Bugnyar, T. (2017b). Animal cognition in a human-dominated world. Anim. Cogn. 20, 1–6. doi: 10.1007/s10071-016-1051-9
Gruber, T., Luncz, L., Mörchen, J., Schuppli, C., Kendal, R. L., and Hockings, K. (2019). Cultural change in animals: a flexible behavioural adaptation to human disturbance. Palgrave Commun. 5, 1–9. doi: 10.1057/s41599-019-0271-4
Guillette, L. M., Scott, A. C. Y. Y., and Healy, S. D. (2016). Social learning in nest-building birds: a role for familiarity. Proc. R. Soc. B 283:20152685. doi: 10.1098/rspb.2015.2685
Gumert, M. D., and Malaivijitnond, S. (2013). Long-tailed macaques select mass of stone tools according to food type. Philos. Trans. R. Soc. B Biol. Sci. 368:20120413. doi: 10.1098/rstb.2012.0413
Halfwerk, W., and Slabbekoorn, H. (2009). A behavioural mechanism explaining noise-dependent frequency use in urban birdsong. Anim. Behav. 78, 1301–1307. doi: 10.1016/j.anbehav.2009.09.015
Hauser, M. D. (2003). “To innovate or not to innovate? That is the question,” in Animal Innovation, eds S. M. Reader and K. N. Laland (Oxford: Oxford University Press), 329–338. doi: 10.1093/acprof
Healy, S. D., and Rowe, C. (2007). A critique of comparative studies of brain size. Proc. R. Soc. B Biol. Sci. 274, 453–464. doi: 10.1098/rspb.2006.3748
Hendry, A. P., Farrugia, T. J., and Kinnison, M. T. (2008). Human influences on rates of phenotypic change in wild animal populations. Mol. Ecol. 17, 20–29. doi: 10.1111/j.1365-294X.2007.03428.x
Heyes, C. M. (1994). Social learning in animals: categories and mechanisms. Biol. Rev. 69, 207–231. doi: 10.1111/j.1469-185X.1994.tb01506.x
Hoppitt, W. J. E., and Laland, K. N. (2008). Social processes influencing learning in animals: a review of the evidence. Adv. Study Behav. 38, 105–165. doi: 10.1016/S0065-3454(08)00003-X
Humle, T., Snowdon, C. T., and Matsuzawa, T. (2009). Social influences on ant-dipping acquisition in the wild chimpanzees (Pan troglodytes verus) of Bossou, Guinea, West Africa. Anim. Cogn. 12, 37–48. doi: 10.1007/s10071-009-0272-6
Janmaat, K. R. L., Byrne, R. W., and Zuberbühler, K. (2006). Primates take weather into account when Searching for Fruits. Curr. Biol. 16, 1232–1237. doi: 10.1016/j.cub.2006.04.031
Janson, C. H. (2016). Capuchins, space, time and memory: an experimental test of what-where-when memory in wild monkeys. Proc. R. Soc. B Biol. Sci. 283:20161432. doi: 10.1098/rspb.2016.1432
Jelbert, S. A., Miller, R., Schiestl, M., Boeckle, M., Cheke, L. G., Gray, R. D., et al. (2019). New Caledonian crows infer the weight of objects from observing their movements in a breeze. Proc. R. Soc. B Biol. Sci. 286:20182332. doi: 10.1098/rspb.2018.2332
Jha, N. A., and Kumar, V. (2017). Effect of no-night light environment on behaviour, learning performance and personality in zebra finches. Anim. Behav. 132, 29–47. doi: 10.1016/j.anbehav.2017.07.017
Johnson, M. T. J., and Munshi-South, J. (2017). Evolution of life in urban environments. Science 358:aam8327. doi: 10.1126/science.aam8327
Jonasson, Z. (2005). Meta-analysis of sex differences in rodent models of learning and memory: a review of behavioral and biological data. Neurosci. Biobehav. Rev. 28, 811–825. doi: 10.1016/j.neubiorev.2004.10.006
Kang, F., Goulet, C. T., and Chapple, D. G. (2018). The impact of urbanization on learning ability in an invasive lizard. Biol. J. Linn. Soc. 123, 55–62. doi: 10.1093/biolinnean/blx131
Kendal, R. L., Coolen, I., van Bergen, Y., and Laland, K. N. (2005). Trade-offs in the adaptive use of social and asocial learning. Adv. Study Behav. 35, 333–379. doi: 10.1016/S0065-3454(05)35008-X
Kitaysky, A. S., Kitaiskaia, E. V., Piatt, J. F., and Wingfield, J. C. (2006). A mechanistic link between chick diet and decline in seabirds? Proc. R. Soc. B Biol. Sci. 273, 445–450. doi: 10.1098/rspb.2005.3351
Kozlovsky, D. Y., Weissgerber, E. A., and Pravosudov, V. V. (2017). What makes specialized food-caching mountain chickadees successful city slickers? Proc. R. Soc. B Biol. Sci. 284, 25–27. doi: 10.1098/rspb.2016.2613
Krishnan, H. C., and Lyons, L. C. (2015). Synchrony and desynchrony in circadian clocks: impacts on learning and memory. Learn. Mem. 22, 426–437. doi: 10.1101/lm.038877.115
Kulahci, I. G., and Quinn, J. L. (2019). Dynamic relationships between information transmission and social connections. Trends Ecol. Evol. 34, 545–554. doi: 10.1016/j.tree.2019.02.007
Laland, K. N., and Reader, S. M. (1999). Foraging innovation in the guppy. Anim. Behav. 57, 331–340. doi: 10.1006/anbe.1998.0967
Lamichhaney, S., Han, F., Webster, M. T., Andersson, L., Grant, B. R., and Grant, P. R. (2018). Rapid hybrid speciation in Darwin’s finches. Science 359:172. doi: 10.1126/science.359.6372.172-d
Langley, E. J. G., van Horik, J. O., Whiteside, M. A., and Madden, J. R. (2018). Individuals in larger groups are more successful on spatial discrimination tasks. Anim. Behav. 142, 87–93. doi: 10.1016/j.anbehav.2018.05.020
Leca, J.-B., Gunst, N., Gardiner, M., and Wandia, I. N. (2021). Acquisition of object-robbing and object/food-bartering behaviours: a culturally maintained token economy in free-ranging long-tailed macaques. Philos. Trans. R. Soc. B Biol. Sci. 376:20190677. doi: 10.1098/rstb.2019.0677
Lee, V. E., Régli, N., McIvor, G. E., and Thornton, A. (2019). Social learning about dangerous people by wild jackdaws. R. Soc. Open Sci. 6:191031. doi: 10.1098/rsos.191031
Lee, W. Y., Han, Y.-D., Lee, S., Jablonski, P. G., Jung, J.-W., and Kim, J.-H. (2016). Antarctic skuas recognize individual humans. Anim. Cogn. 19, 861–865. doi: 10.1007/s10071-016-0970-9
Legagneux, P., and Ducatez, S. (2013). European birds adjust their flight initiation distance to road speed limits. Biol. Lett. 9:20130417. doi: 10.1098/rsbl.2013.0417
Levey, D. J., Londoño, G. A., Ungvari-Martin, J., Hiersoux, M. R., Jankowski, J. E., Poulsen, J. R., et al. (2009). Urban mockingbirds quickly learn to identify individual humans. Proc. Natl. Acad. Sci. U.S.A. 106, 8959–8962. doi: 10.1073/pnas.0811422106
Lipshutz, S. E., Overcast, I. A., Hickerson, M. J., Brumfield, R. T., and Derryberry, E. P. (2017). Behavioural response to song and genetic divergence in two subspecies of white-crowned sparrows (Zonotrichia leucophrys). Mol. Ecol. 26, 3011–3027. doi: 10.1111/mec.14002
Lotem, A., Halpern, J. Y., Edelman, S., and Kolodny, O. (2017). The evolution of cognitive mechanisms in response to cultural innovations. Proc. Natl. Acad. Sci. U.S.A. 114, 7915–7922. doi: 10.1073/pnas.1620742114
Lowry, H., Lill, A., and Wong, B. B. M. (2013). Behavioural responses of wildlife to urban environments. Biol. Rev. 88, 537–549. doi: 10.1111/brv.12012
Maguire, E. A., Gadian, D. G., Johnsrude, I. S., Good, C. D., Ashburner, J., Frackowiak, R. S. J., et al. (2000). Navigation-related structural change in the hippocampi of taxi drivers. Proc. Natl. Acad. Sci. U.S.A. 97, 4398–4403.
Marzluff, J. M., Walls, J., Cornell, H. N., Withey, J. C., and Craig, D. P. (2010). Lasting recognition of threatening people by wild American crows. Anim. Behav. 79, 699–707. doi: 10.1016/j.anbehav.2009.12.022
Maspons, J., Molowny-Horas, R., and Sol, D. (2019). Behaviour, life history and persistence in novel environments. Philos. Trans. R. Soc. B Biol. Sci. 374:20180056. doi: 10.1098/rstb.2018.0056
Matthews, B., Narwani, A., Hausch, S., Nonaka, E., Peter, H., Yamamichi, M., et al. (2011). Toward an integration of evolutionary biology and ecosystem science. Ecol. Lett. 14, 690–701. doi: 10.1111/j.1461-0248.2011.01627.x
Mazur, R., and Seher, V. (2008). Socially learned foraging behaviour in wild black bears, Ursus americanus. Anim. Behav. 75, 1503–1508. doi: 10.1016/j.anbehav.2007.10.027
McComb, K., Shannon, G., Sayialel, K. N., and Moss, C. (2014). Elephants can determine ethnicity, gender, and age from acoustic cues in human voices. Proc. Natl. Acad. Sci. U.S.A. 111, 5433–5438. doi: 10.1073/pnas.1321543111
McKinney, M. L. (2006). Urbanization as a major cause of biotic homogenization. Biol. Conserv. 127, 247–260. doi: 10.1016/j.biocon.2005.09.005
Mesoudi, A., Whiten, A., and Laland, K. N. (2004). Perspective: is human cultural evolution darwinian? evidence reviewed from the perspective of the origin of species. Evolution 58, 1–11. doi: 10.1554/03-212
Mettke-Hofmann, C. (2017). Avian movements in a modern world: cognitive challenges. Anim. Cogn. 20, 77–86. doi: 10.1007/s10071-016-1006-1
Meulman, E. J. M., Seed, A. M., and Mann, J. (2013). If at first you don’t succeed. Studies of ontogeny shed light on the cognitive demands of habitual tool use. Philos. Trans. R. Soc. B Biol. Sci. 368:20130050. doi: 10.1098/rstb.2013.0050
Meyrier, E., Jenni, L., Bötsch, Y., Strebel, S., Erne, B., and Tablado, Z. (2017). Happy to breed in the city? Urban food resources limit reproductive output in Western Jackdaws. Ecol. Evol. 7, 1363–1374. doi: 10.1002/ece3.2733
Miranda, A. C., Schielzeth, H., Sonntag, T., and Partecke, J. (2013). Urbanization and its effects on personality traits: a result of microevolution or phenotypic plasticity? Glob. Chang. Biol. 19, 2634–2644. doi: 10.1111/gcb.12258
Mueller, J. C., Partecke, J., Hatchwell, B. J., Gaston, K. J., and Evans, K. L. (2013). Candidate gene polymorphisms for behavioural adaptations during urbanization in blackbirds. Mol. Ecol. 22, 3629–3637. doi: 10.1111/mec.12288
Müller, C. A., Schmitt, K., Barber, A. L. A., and Huber, L. (2015). Dogs can discriminate emotional expressions of human faces. Curr. Biol. 25, 601–605. doi: 10.1016/j.cub.2014.12.055
Nocera, J. J., Forbes, G. J., and Giraldeau, L.-A. (2006). Inadvertent social information in breeding site selection of natal dispersing birds. Proc. R. Soc. B 273, 349–355. doi: 10.1098/rspb.2005.3318
Ono, M., Kawai, R., Horikoshi, T., Yasuoka, T., and Sakakibara, M. (2002). Associative learning acquisition and retention depends on developmental stage in Lymnaea stagnalis. Neurobiol. Learn. Mem. 78, 53–64. doi: 10.1006/nlme.2001.4066
Papworth, S., Milner-Gulland, E. J., and Slocombe, K. (2013). Hunted woolly monkeys (Lagothrix poeppigii) show threat-sensitive responses to human presence. PLoS One 8:e0062000. doi: 10.1371/journal.pone.0062000
Penn, D. C., Holyoak, K. J., and Povinelli, D. J. (2008). Darwin’s mistake: explaining the discontinuity between human and nonhuman minds. Behav. Brain Sci. 31, 109–178. doi: 10.1017/S0140525X08003543
Pirotta, E., Booth, C. G., Costa, D. P., Fleishman, E., Kraus, S. D., Lusseau, D., et al. (2018). Understanding the population consequences of disturbance. Ecol. Evol. 8, 9934–9946. doi: 10.1002/ece3.4458
Poirier, M. A., Kozlovsky, D. Y., Morand-Ferron, J., and Careau, V. (2020). How general is cognitive ability in non-human animals? A meta-analytical and multi-level reanalysis approach: not-so general animal intelligence. Proc. R. Soc. B Biol. Sci. 287:1853. doi: 10.1098/rspb.2020.1853rspb20201853
Potvin, D. A. (2017). Coping with a changing soundscape: avoidance, adjustments and adaptations. Anim. Cogn. 20, 9–18. doi: 10.1007/s10071-016-0999-9
Potvin, D. A., and MacDougall-Shackleton, S. A. (2015). Traffic noise affects embryo mortality and nestling growth rates in captive zebra finches. J. Exp. Zool. 323, 722–730. doi: 10.1002/jez.1965
Potvin, D. A., and Mulder, R. A. (2013). Immediate, independent adjustment of call pitch and amplitude in response to varying background noise by silvereyes (Zosterops lateralis). Behav. Ecol. 24, 1363–1368. doi: 10.1093/beheco/art075
Pravosudov, V. V., and Clayton, N. S. (2002). A test of the adaptive specialization hypothesis: population differences in caching, memory, and the hippocampus in black-capped chickadees (Poecile atricapilla). Behav. Neurosci. 116, 515–522. doi: 10.1037/0735-7044.116.4.515
Pravosudov, V. V., Lavenex, P., and Omanska, A. (2005). Nutritional deficits during early development affect hippocampal structure and spatial memory later in life. Behav. Neurosci. 119, 1368–1374. doi: 10.1037/0735-7044.119.5.1368
Preiszner, B., Papp, S., Pipoly, I., Seress, G., Vincze, E., Liker, A., et al. (2017). Problem-solving performance and reproductive success of great tits in urban and forest habitats. Anim. Cogn. 20, 53–63. doi: 10.1007/s10071-016-1008-z
Price, T. D., Qvarnström, A., and Irwin, D. E. (2003). The role of phenotypic plasticity in driving genetic evolution. Proc. R. Soc. B Biol. Sci. 270, 1433–1440. doi: 10.1098/rspb.2003.2372
Proops, L., Grounds, K., Smith, A. V., and McComb, K. (2018). Animals remember previous facial expressions that specific humans have exhibited. Curr. Biol. 28, 1428–1432.e4. doi: 10.1016/j.cub.2018.03.035
Proops, L., and McComb, K. (2012). Cross-modal individual recognition in domestic horses (Equus caballus) extends to familiar humans. Proc. R. Soc. B Biol. Sci. 279, 3131–3138. doi: 10.1098/rspb.2012.0626
Rendell, L. E., Fogarty, L., Hoppitt, W. J. E., Morgan, T. J. H., Webster, M. M., and Laland, K. N. (2011). Cognitive culture: theoretical and empirical insights into social learning strategies. Trends Cogn. Sci. 15, 68–76. doi: 10.1016/j.tics.2010.12.002
Roberts, G. (1995). A real-time response of vigilance behaviour to changes in group size. Anim. Behav. 50, 1371–1374. doi: 10.1016/0003-3472(95)80052-2
Roberts, W. A. (2006). Animal memory: episodic-like memory in rats. Curr. Biol. 16, 601–603. doi: 10.1016/j.cub.2006.07.003
Rodriguez-Prieto, I., Fernández-Juricic, E., Martín, J., and Regis, Y. (2009). Antipredator behavior in blackbirds: habituation complements risk allocation. Behav. Ecol. 20, 371–377. doi: 10.1093/beheco/arn151
Roth, T. C., la Dage, L. D., Freas, C. A., and Pravosudov, V. V. (2012). Variation in memory and the hippocampus across populations from different climates: a common garden approach. Proc. R. Soc. B Biol. Sci. 279, 402–410. doi: 10.1098/rspb.2011.1020
Roudez, R. J., Glover, T., and Weis, J. S. (2008). Learning in an invasive and a native predatory crab. Biol. Invas. 10, 1191–1196. doi: 10.1007/s10530-007-9195-9
Rutz, C., Bluff, L. A., Reed, N., Troscianko, J., Newton, J., Inger, R., et al. (2010). The ecological significance of tool use in New Caledonian crows. Science 329, 1523–1526.
Samia, D. S. M., Nakagawa, S., Nomura, F., Rangel, T. F., and Blumstein, D. T. (2015). Increased tolerance to humans among disturbed wildlife. Nat. Commun. 6, 1–8. doi: 10.1038/ncomms9877
Sargeant, B. L., and Mann, J. (2009). Developmental evidence for foraging traditions in wild bottlenose dolphins. Anim. Behav. 78, 715–721. doi: 10.1016/j.anbehav.2009.05.037
Sayol, F., Maspons, J., Lapiedra, O., Iwaniuk, A. N., Székely, T., and Sol, D. (2016). Environmental variation and the evolution of large brains in birds. Nat. Commun. 7:13971. doi: 10.1038/ncomms13971
Sayol, F., Sol, D., and Pigot, A. L. (2020). Brain size and life history interact to predict urban tolerance in birds. Front. Ecol. Evol. 8:58. doi: 10.3389/fevo.2020.00058
Schell, C. J., Dyson, K., Fuentes, T. L., Des Roches, S., Harris, N. C., Miller, D. S., et al. (2020). The ecological and evolutionary consequences of systemic racism in urban environments. Science 369:eaay4497. doi: 10.1126/science.aay4497
Schlesinger, M. D., Manley, P. N., and Holyoak, M. (2008). Distinguishing stressors acting on land bird communities in an urbanizing environment. Ecology 89, 2302–2314.
Schroeder, J., Nakagawa, S., Cleasby, I. R., and Burke, T. (2012). Passerine birds breeding under chronic noise experience reduced fitness. PLoS One 7:e39200. doi: 10.1371/journal.pone.0039200
Semeniuk, C. A. D., and Rothley, K. D. (2008). Costs of group-living for a normally solitary forager: effects of provisioning tourism on southern stingrays Dasyatis americana. Mar. Ecol. Prog. Ser. 357, 271–282. doi: 10.3354/meps07299
Seppänen, J. T., and Forsman, J. T. (2007). Interspecific social learning: novel preference can be acquired from a competing species. Curr. Biol. 17, 1248–1252. doi: 10.1016/j.cub.2007.06.034
Seppänen, J. T., Forsman, J. T., Mönkkönen, M., Krams, I., and Salmi, T. (2011). New behavioural trait adopted or rejected by observing heterospecific tutor fitness. Proc. R. Soc. B 278, 1736–1741. doi: 10.1098/rspb.2010.1610
Seress, G., Sándor, K., Evans, K. L., and Liker, A. (2020). Food availability limits avian reproduction in the city: an experimental study on great tits Parus major. J. Anim. Ecol. 89, 1570–1580. doi: 10.1111/1365-2656.13211
Shaw, R. C., and Schmelz, M. (2017). Cognitive test batteries in animal cognition research: evaluating the past, present and future of comparative psychometrics. Anim. Cogn. 20, 1003–1018. doi: 10.1007/s10071-017-1135-1
Shochat, E., Warren, P. S., Faeth, S. H., McIntyre, N. E., and Hope, D. (2006). From patterns to emerging processes in mechanistic urban ecology. Trends Ecol. Evol. 21, 186–191. doi: 10.1016/j.tree.2005.11.019
Sih, A., Cote, J., Evans, M., Fogarty, S., and Pruitt, J. (2012). Ecological implications of behavioural syndromes. Ecol. Lett. 15, 278–289. doi: 10.1111/j.1461-0248.2011.01731.x
Sih, A., and Del Giudice, M. (2012). Linking behavioural syndromes and cognition: a behavioural ecology perspective. Philos. Trans. R. Soc. B 367, 2762–2772. doi: 10.1098/rstb.2012.0216
Sih, A., Ferrari, M. C. O., and Harris, D. J. (2011). Evolution and behavioural responses to human-induced rapid environmental change. Evol. Appl. 4, 367–387. doi: 10.1111/j.1752-4571.2010.00166.x
Slagsvold, T., and Wiebe, K. L. (2011). Social learning in birds and its role in shaping a foraging niche. Philos. Trans. R. Soc. B 366, 969–977. doi: 10.1098/rstb.2010.0343
Snell-Rood, E. C. (2013). An overview of the evolutionary causes and consequences of behavioural plasticity. Anim. Behav. 85, 1004–1011. doi: 10.1016/j.anbehav.2012.12.031
Snell-Rood, E. C., and Wick, N. (2013). Anthropogenic environments exert variable selection on cranial capacity in mammals. Proc. R. Soc. B 280:20131384.
Sol, D. (2009). Revisiting the cognitive buffer hypothesis for the evolution of large brains. Biol. Lett. 5, 130–133. doi: 10.1098/rsbl.2008.0621
Sol, D., Duncan, R. P., Blackburn, T. M., Cassey, P., and Lefebvre, L. (2005). Big brains, enhanced cognition, and response of birds to novel environments. Proc. Natl. Acad. Sci. U.S.A. 102, 5460–5465. doi: 10.1073/pnas.0408145102
Sol, D., Lapiedra, O., and Ducatez, S. (2020). “Cognition and adaptation to urban environments,” in Urban Evolutionary Biology, eds M. Szulkin, J. Munshi-South, and A. Charmantier (Oxford: Oxford University Press), 253–267. doi: 10.1093/oso/9780198836841.003.0015
Sol, D., Lapiedra, O., and González-Lagos, C. (2013). Behavioural adjustments for a life in the city. Anim. Behav. 85, 1101–1112. doi: 10.1016/j.anbehav.2013.01.023
Sonnenberg, B. R., Branch, C. L., Pitera, A. M., Bridge, E., and Pravosudov, V. V. (2019). Natural selection and spatial cognition in wild food-caching mountain chickadees. Curr. Biol. 29, 670–676.e3. doi: 10.1016/j.cub.2019.01.006
Sorenson, M. D., Sefc, K. M., and Payne, R. B. (2003). Speciation by host switch in brood parasitic indigobirds. Nature 424, 928–931. doi: 10.1038/nature01863
St Clair, J. J. H., and Rutz, C. (2013). New Caledonian crows attend to multiple functional properties of complex tools. Philos. Trans. R. Soc. B Biol. Sci. 368:20120415. doi: 10.1098/rstb.2012.0415
Street, S. E., Navarrete, A. F., Reader, S. M., and Laland, K. N. (2017). Coevolution of cultural intelligence, extended life history, sociality, and brain size in primates. Proc. Natl. Acad. Sci. U.S.A. 114, 7908–7914. doi: 10.5061/dryad.jb22k75/1
Suárez-Rodríguez, M., López-Rull, I., and Garcia, C. M. Í (2013). Incorporation of cigarette butts into nests reduces nest ectoparasite load in urban birds: new ingredients for an old recipe? Biol. Lett. 9, 10–12. doi: 10.1098/rsbl.2012.0931
Suárez-Rodríguez, M., Montero-Montoya, R. D., and Garcia, C. M. (2017). Anthropogenic nest materials may increase breeding costs for urban birds. Front. Ecol. Evol. 5:4. doi: 10.3389/fevo.2017.00004
Sumasgutner, P., Nemeth, E., Tebb, G., Krenn, H. W., and Gamauf, A. (2014). Hard times in the city-attractive nest sites but insufficient food supply lead to low reproduction rates in a bird of prey. J. Wildl. Rehabil. 34, 17–31. doi: 10.1186/1742-9994-11-48
Szabo, B., Damas-Moreira, I., and Whiting, M. J. (2020). Can cognitive ability give invasive species the means to succeed? A review of the evidence. Front. Ecol. Evol. 8:187. doi: 10.3389/fevo.2020.00187
Szulkin, M., Garroway, C. J., Corsini, M., Kotarba, A. Z., Dominoni, D., Szulkin, M., et al. (2020). How to quantify urbanization when testing for urban evolution? Urban Evol. Biol. 48, 13–33. doi: 10.1093/oso/9780198831.003.0002
Taufique, S. T., Prabhat, A., and Kumar, V. (2019). Light at night affects hippocampal and nidopallial cytoarchitecture: implication for impairment of brain function in diurnal corvids. J. Exp. Zool. Part A Ecol. Integr. Physiol. 331, 149–156. doi: 10.1002/jez.2238
Taylor, A. H., Hunt, G. R., Medina, F. S., and Gray, R. D. (2009). Do new Caledonian crows solve physical problems through causal reasoning? Proc. R. Soc. B Biol. Sci. 276, 247–254. doi: 10.1098/rspb.2008.1107
Taylor, A. H., Miller, R., and Gray, R. D. (2012). New Caledonian crows reason about hidden causal agents. Proc. Natl. Acad. Sci. U.S.A. 109, 16389–16391. doi: 10.1073/pnas.1208724109
Teschke, I., Cartmill, E. A., Stankewitz, S., and Tebbich, S. (2011). Sometimes tool use is not the key: no evidence for cognitive adaptive specializations in tool-using woodpecker finches. Anim. Behav. 82, 945–956. doi: 10.1016/j.anbehav.2011.07.032
Teschke, I., Wascher, C. A. F., Scriba, M. F., von Bayern, A. M. P., Huml, V., Siemers, B., et al. (2013). Did tool-use evolve with enhanced physical cognitive abilities? Philos. Trans. R. Soc. B Biol. Sci. 368:20120418. doi: 10.1098/rstb.2012.0418
Thompson, M. J., and Morand-Ferron, J. (2019). Food caching in city birds: urbanization and exploration do not predict spatial memory in scatter hoarders. Anim. Cogn. 22, 743–756. doi: 10.1007/s10071-019-01271-4
Thornton, A., Isden, J., and Madden, J. R. (2014). Toward wild psychometrics: linking individual cognitive differences to fitness. Behav. Ecol. 25, 1299–1301. doi: 10.1093/beheco/aru095
Thornton, A., and Lukas, D. (2012). Individual variation in cognitive performance: developmental and evolutionary perspectives. Philos. Trans. R. Soc. B 367, 2773–2783. doi: 10.1098/rstb.2012.0214
Thornton, A., and Raihani, N. J. (2008). The evolution of teaching. Anim. Behav. 75, 1823–1836. doi: 10.1111/j.1558-5646.2011.01370.x
Thornton, A., and Samson, J. (2012). Innovative problem solving in wild meerkats. Anim. Behav. 83, 1459–1468. doi: 10.1016/j.anbehav.2012.03.018
Thorogood, R., Kokko, H., and Mappes, J. (2018). Social transmission of avoidance among predators facilitates the spread of novel prey. Nat. Ecol. Evol. 2, 254–261. doi: 10.1038/s41559-017-0418-x
Tibbetts, E. A., and Dale, J. (2007). Individual recognition: it is good to be different. Trends Ecol. Evol. 22, 529–537. doi: 10.1016/j.tree.2007.09.001
Trapanese, C., Meunier, H., and Masi, S. (2019). What, where and when: spatial foraging decisions in primates. Biol. Rev. 94, 483–502. doi: 10.1111/brv.12462
Tryjanowski, P., Møller, A. P., Morelli, F., Biaduñ, W., Brauze, T., Ciach, M., et al. (2016). Urbanization affects neophilia and risk-taking at bird-feeders. Sci. Rep. 6, 1–7. doi: 10.1038/srep28575
Tuomainen, U., and Candolin, U. (2011). Behavioural responses to human-induced environmental change. Biol. Rev. 86, 640–657. doi: 10.1111/j.1469-185X.2010.00164.x
Uchida, K., Suzuki, K. K., Shimamoto, T., Yanagawa, H., and Koizumi, I. (2019). Decreased vigilance or habituation to humans? Mechanisms on increased boldness in urban animals. Behav. Ecol. 30, 1583–1590. doi: 10.1093/beheco/arz117
Ushitani, T., Perry, C. J., Cheng, K., and Barron, A. B. (2016). Accelerated behavioural development changes fine-scale search behaviour and spatial memory in honey bees (Apis mellifera L.). J. Exp. Biol. 219, 412–418. doi: 10.1242/jeb.126920
Vallortigara, G. (1996). Learning of colour and position cues in domestic chicks: males are better at position, females at colour. Behav. Process. 36, 289–296. doi: 10.1016/0376-6357(95)00063-1
van de Waal, E., Renevey, N., Favre, C. M., and Bshary, R. (2010). Selective attention to philopatric models causes directed social learning in wild vervet monkeys. Proc. R. Soc. B 277, 2105–2111. doi: 10.1098/rspb.2009.2260
Van Dongen, W. F. D., Robinson, R. W., Weston, M. A., Mulder, R. A., and Guay, P. J. (2015). Variation at the DRD4 locus is associated with wariness and local site selection in urban black swans. BMC Evol. Biol. 15:253. doi: 10.1186/s12862-015-0533-8
van Horik, J. O., and Madden, J. R. (2016). A problem with problem solving: motivational traits, but not cognition, predict success on novel operant foraging tasks. Anim. Behav. 114, 189–198. doi: 10.1016/j.anbehav.2016.02.006
van Schaik, C. P., and Burkart, J. M. (2011). Social learning and evolution: the cultural intelligence hypothesis. Philos. Trans. R. Soc. B Biol. Sci. 366, 1008–1016. doi: 10.1098/rstb.2010.0304
Verzijden, M. N., Ten Cate, C., Servedio, M. R., Kozak, G. M., Boughman, J. W., and Svensson, E. I (2012). The impact of learning on sexual selection and speciation. Trends Ecol. Evol. 27, 511–519. doi: 10.1016/j.tree.2012.05.007
Vincze, E., Papp, S., Preiszner, B., Seress, G., Bókony, V., and Liker, A. (2016). Habituation to human disturbance is faster in urban than rural house sparrows. Behav. Ecol. 27, 1304–1313. doi: 10.1093/beheco/arw047
Vorster, A. P., and Born, J. (2015). Sleep and memory in mammals, birds and invertebrates. Neurosci. Biobehav. Rev. 50, 103–119. doi: 10.1016/j.neubiorev.2014.09.020
Wascher, C. A. F., Kulahci, I. G., Langley, E. J. G., and Shaw, R. C. (2018). How does cognition shape social relationships? Philos. Trans. R. Soc. B Biol. Sci. 373, 15–18. doi: 10.1098/rstb.2017.0293
Wat, K. K. Y., Banks, P. B., and McArthur, C. (2020). Linking animal personality to problem-solving performance in urban common brushtail possums. Anim. Behav. 162, 35–45. doi: 10.1016/j.anbehav.2020.01.013
Whitehead, H., Laland, K. N., Rendell, L., Thorogood, R., and Whiten, A. (2019). The reach of gene-culture coevolution in animals. Nat. Commun. 10:2405. doi: 10.1038/s41467-019-10293-y
Whiten, A., Ayala, F. J., Feldman, M. W., and Laland, K. N. (2017). The extension of biology through culture. Proc. Natl. Acad. Sci. U.S.A. 114, 7775–7781. doi: 10.1073/pnas.1707630114
Wiley, R. H. (2013). Specificity and multiplicity in the recognition of individuals: implications for the evolution of social behaviour. Biol. Rev. 88, 179–195. doi: 10.1111/j.1469-185X.2012.00246.x
Wilkinson, A., Kuenstner, K., Mueller, J., and Huber, L. (2010). Social learning in a non-social reptile (Geochelone carbonaria). Biol. Lett. 6, 614–616. doi: 10.1098/rsbl.2010.0092
Wright, T. F., Eberhard, J. R., and Avery, M. L. (2010). Behavioral flexibility and species invasions: the adaptive flexibility hypothesis. Ethol. Ecol. Evol. 22, 393–404. doi: 10.1080/03949370.2010.505580
Yorzinski, J. L., Ordonez, K. A., and Chema, K. T. (2017). Does artificial light pollution impair problem-solving success in peafowl? Ethology 123, 854–860. doi: 10.1111/eth.12658
Young, E. S., Frankenhuis, W. E., and Ellis, B. J. (2020). Theory and measurement of environmental unpredictability. Evol. Hum. Behav. 41, 550–556. doi: 10.1016/j.evolhumbehav.2020.08.006
Keywords: urban, cognition, learning, plasticity, adaptation
Citation: Lee VE and Thornton A (2021) Animal Cognition in an Urbanised World. Front. Ecol. Evol. 9:633947. doi: 10.3389/fevo.2021.633947
Received: 26 November 2020; Accepted: 15 February 2021;
Published: 04 March 2021.
Edited by:
Jayna Lynn DeVore, The University of Sydney, AustraliaReviewed by:
Culum Brown, Macquarie University, AustraliaCopyright © 2021 Lee and Thornton. This is an open-access article distributed under the terms of the Creative Commons Attribution License (CC BY). The use, distribution or reproduction in other forums is permitted, provided the original author(s) and the copyright owner(s) are credited and that the original publication in this journal is cited, in accordance with accepted academic practice. No use, distribution or reproduction is permitted which does not comply with these terms.
*Correspondence: Victoria E. Lee, VmljdG9yaWEuTGVlQHNydWMuYWMudWs=
†Present address: Victoria E. Lee, Animal and Veterinary Sciences, Scotland’s Rural College, Midlothian, United Kingdom
Disclaimer: All claims expressed in this article are solely those of the authors and do not necessarily represent those of their affiliated organizations, or those of the publisher, the editors and the reviewers. Any product that may be evaluated in this article or claim that may be made by its manufacturer is not guaranteed or endorsed by the publisher.
Research integrity at Frontiers
Learn more about the work of our research integrity team to safeguard the quality of each article we publish.