- 1School of Geographic Sciences, Nantong University, Nantong, China
- 2Institute of Environment and Sustainable Development in Agriculture, Chinese Academy of Agricultural Sciences, Beijing, China
- 3Theoretical Ecology, Institute of Biology, Freie Universität Berlin, Berlin, Germany
- 4Department of Resource Management, Tangshan Normal University, Tangshan, China
- 5Lhasa National Ecological Research Station, Key Laboratory of Ecosystem Network Observation and Modelling, Institute of Geographic Sciences and Natural Resources Research, Chinese Academy of Sciences, Beijing, China
Alpine grasslands on the Tibetan Plateau, being vulnerable to environmental and anthropogenic changes, have experienced dramatic climate change and intensive livestock grazing during the last half-century. Climate change, coupled with grazing activities, has profoundly altered alpine grassland function and structure and resulted in vast grassland degradation. To restore degraded grasslands, the Central Government of China has implemented the Ecological Security Barrier Protection and Construction Project since 2008 across the Tibetan Autonomous Region. However, the relative effect of climate change and grazing activities on the variation in alpine grassland productivity is still under debate. In this study, we quantified how aboveground net primary production (ANPP) varied before (2000–2008) and after (2009–2017) starting the project across different alpine grasslands and how much variance in ANPP could be attributed to climate change and grazing disturbance, in terms of temperature, precipitation, solar radiation, and grazing intensity. Our results revealed that Tibet’s climate got warmer and wetter, and grazing intensity decreased after starting the project. Mean ANPP increased at approximately 81% of the sites, on average from 27.0 g C m–2 during 2000–2008 to 28.4 g C m–2 during 2009–2017. The ANPP positively correlated with annual temperature and precipitation, but negatively with grazing intensity for both periods. Random forest modeling indicated that grazing intensity (14.5%) had a much lower influence in controlling the dynamics of grassland ANPP than precipitation (29.0%), suggesting that precipitation variability was the key factor for alpine grassland ANPP increase across Tibet.
Introduction
Climate change and human activities are primary drivers for changes in terrestrial ecosystems globally (Haberl et al., 2007; Chen et al., 2013; Tong et al., 2018), especially for unprecedented changes in ecosystem service and function (Knight and Harrison, 2012; Seiferling et al., 2014; Erb et al., 2018). A meta-analysis by Lin et al. (2010) found that climate warming can promote global vegetation productivity. Precipitation can also accelerate or decelerate vegetation growth in most terrestrial ecosystems, depending on its frequency and timing (Wu et al., 2011). Meanwhile, human activities can affect ecosystem response and feedback to climate change, via biomass utilization, biofuel consumption, land use, and land cover change (Haberl et al., 2007; Kröel-Dulay et al., 2015). For example, human disturbances might push an intact ecosystem away from its successional trajectory, alter species assembly, and turnover, and make it much more vulnerable to climate change than before (Kröel-Dulay et al., 2015).
Aboveground net primary production (ANPP) is a critical ecosystem service in maintaining global carbon balance (Ruimy et al., 1999; Zhang et al., 2009), often used to forecast ecological consequences under anthropogenic and climatic perturbations. A better understanding of how ecosystem ANPP responds to climate change and human activities can help mitigate environmental damages and optimize ecosystem management (Zhou et al., 2018). A considerable volume of literature tried to identify and quantify the relative influences of climate change and human activities on ecosystem productivity with various methods (Paudel and Andersen, 2010; Erb et al., 2018; Li L. et al., 2018), such as manipulative experiments, traditional statistical analysis, and residuals-trend modeling (Li L. et al., 2018). Recently, random forest modeling, with high accuracy and robust efficiency, is increasingly being used to quantify predictors’ relative importance (Gill et al., 2017; Huang and Xia, 2019). The random forest modeling can also account for interactions and non-linear relationships between predictors, obstacles (the overfitting problems) and deal with data noises (Heung et al., 2014). Therefore, scientists often recommended random forest models for processing high-dimensional and -correlated datasets (Breiman, 2001).
Due to high elevations, alpine grasslands widely distributed on the Tibetan Plateau are susceptible to climate change and human activities, as vegetation in Arctic and Antarctic (Yao et al., 2012; Yang et al., 2017; Li et al., 2020). Climate change, combined with human activities, has significantly reshaped the structure and function of the Tibetan alpine grasslands (Wang et al., 2005), and resulted in about 0.4 × 106 km2 grassland degradation in the1990s, which accounted for 33% of total grassland area on this plateau (Long et al., 2009). Therefore, rational utilization, restoration, and conservation of alpine grasslands under changing climate and anthropogenic disturbances have attracted increasing attention since 2000 (Harris, 2010; Yu et al., 2012; Dong et al., 2020). The central government of China initiated several ecological projects, like the “Ecological Security Barrier Protection and Construction Project” started since 2008, and the “Compensation and Rewards to Herders for Natural Grassland Conservation” since 2010, to promote the recovery and restoration of degraded alpine grasslands on the Tibetan Plateau (Wang et al., 2017). The implementation of these projects has eased the pressure of human disturbances (mainly referring to livestock grazing hereafter) (Fan et al., 2015) and also increased the alpine grassland productivity (Chen et al., 2014; Xu et al., 2016). However, the relative contributions of climate change and human activities to alpine grassland productivity are still unclear. Moreover, it is pertinent to examine the extent to which ecological projects and policies can affect alpine grassland productivity.
To fill these gaps in current research, we collected historical records of climatic variables and the Normalized difference vegetation index (NDVI) data before (2000–2008) and after (2009–2017) the starting of the “Ecological Security Barrier Protection and Construction Project.” These data were mainly used to simulate the changes in alpine grassland productivity during the two subperiods. We also conducted field measurements of aboveground biomass between fenced and grazed pastures in different alpine grassland types to validate the models’ outputs. By comparing the difference in alpine grassland productivity, it is possible to clarify the effects of the “Ecological Security Barrier Protection and Construction Project.” We also collected the livestock numbers from statistic yearbooks of 2000–2017 to quantify grazing intensity over time and across space. Thus, it is also possible to quantify how much the variance in alpine grassland productivity can be explained by the corresponding climatic (refers to temperature, precipitation, and solar radiation) and anthropogenic (refers to grazing intensity) factors.
Materials and Methods
Study Area
The Tibetan Autonomous Region (hereafter Tibet) is located in the southwest of China (Figure 1), covering about 1.22 million km2. With an average elevation of over 4,000 m above sea level, Tibet has a cold (mean annual temperature from 2000 to 2017 was –1.1°C) and dry (mean annual precipitation from 2000 to 2017 was 307.8 mm) climate. This climate shaped various sensitive and vulnerable ecosystems. Alpine grasslands mainly distribute in northwestern places at high elevations, covary, and coevolve well with zonal climates (Wu et al., 2013). From southeast to northwest, grassland types vary from humid alpine meadow (AM) dominated by Kobresia pygmaea to semiarid alpine steppe (AS) dominated by Stipa purpurea, and to arid alpine desert-steppe (ADS) co-dominated by S. purpurea and S. glareosa.
Data Collection
Normalized difference vegetation index (NDVI) is a remote sensing technique widely used in the regional ecosystem monitoring and evaluation. This study used the moderate-resolution imaging spectroradiometer (MODIS) Version 6 NDVI (MOD13A3) from 2000 to 2017, with 1 km spatial resolution and 1 month time interval. NDIV data were downloaded from the National Aeronautics and Space Administration agency1. The monthly NDVI data were developed using the Maximum Value Composition method (MVC) and calibrated for geometrical and atmospheric effects and cloud contamination. Grassland distribution referred to the China Vegetation Atlas with a scale of 1:1,000,000 (Chinese Academy of Sciences, 2001), from the Resource and Environment Data Cloud Platform2.
Daily meteorological data from 2000 and 2017 were collected from the National Meteorological Information Center (NMIC), China Meteorological Administration (CMA)3. Daily meteorological records were aggregated at the monthly level. Then, we interpolated monthly precipitation, temperature, and sunshine duration into raster surfaces with a 1 km spatial resolution using the ANUSPLIN 4.3 (Hutchinson, 2004). According to Allen et al. (1998), solar radiation was calculated based on geographical position and sunshine duration. It has been examined that the grid climatic surfaces match well with field observations (Chen et al., 2014; Tao et al., 2015).
In 2009, a 1,200 km transect was established across the northern Tibetan Plateau. This transect crosses exceptionally arid, semiarid, to semi-humid alpine continental climates from west to east, encompassing three primary grassland type: alpine meadow, alpine steppe and alpine desert-steppe. From 2009 to 2015, we collected aboveground biomass (AGB, g/m2) field-measured during peak growing season (late July to early August) along with this transect, between fenced and open (grazed) alpine grasslands. In total, we measured AGB at 224 sites in open alpine grasslands and 138 sites in fenced ones (Figure 1). At each sampling site, five 0.5 m × 0.5 m quadrats were laid at 20 m intervals along a 100 m random transect line. Plant aboveground materials were dried at 65°C for 48 h and then weighed for AGB measurements. For grassland in Tibet, the most sampled species sprout annually in early May and senesce in late September with peak biomass generally between late July and early August. Thus, the field-measured AGB can surrogate for yearly ANPP. Finally, the dry matter was converted to carbon, assuming a carbon content of 45% (Lieth and Whittaker, 1978).
The livestock number for each county was collected from the “Statistical Yearbooks” of 2000–2017. The numbers of sheep, goats, and large herbivore animals (mainly referring to yaks, donkeys, and horses) were finally calculated as standardized sheep units (SSU), by following the algorithms of Fan et al. (2010) that one sheep equals to one SSU and one large herbivore to four SSUs.
Calculation of Grassland ANPP
The Carnegie-Ames-Stanford Approach (CASA) model was driven by satellite-observed NDVI and climate (e.g., temperature, precipitation, and radiation) and other factors, such as land-use change. The NDVI changes can reflect human harvest from plant material. NPP is mainly determined by two variables, absorbed photosynthetically active radiation (APAR) and the light-use efficiency (LUE) in the CASA model,
where ANPP(x,t) represents plant growth at spatial location x and time t (g C m–2), and PAR(x, t), and FPAR(x, t) are total solar radiation (MJ m–2) and the fraction of the incoming PAR intercepted by plant incident at spatial location x and time. ε^* is the maximum possible light energy conversion efficiency and was set uniformly at 0.56 g C MJ–1 (Zhang et al., 2013). Tε 1(x, t), Tε 2 (x, t) are the effects of temperature to ε^*, and Wε (x, t) accounts for effects of water stress, also at location x and time t. ANPP can be inferred by the ratio between BNPP and ANPP (R), around 0.587 for alpine grasslands on the Tibetan Plateau predicted by Wu et al. (2010).
Calculation of Grazing Intensity
Grazing intensity (GI) was quantified by the ratio between actual livestock carrying capacity (Ca) and theoretical livestock carrying capacity (Ct):
The actual livestock carrying capacity for each county was determined as follows:
where Cn is the number of livestock inventory in a given year, Ch is the number of livestock sold in a given year. A is the available grassland area (ha).
The theoretical livestock carrying capacity for each grassland pixel was calculated as follows:
where Y is grassland yield; U is the utilization rate of herbage (%), estimated as 70%; C is the proportion of the area available for pasture (%), estimated as 0.84 (Fan et al., 2010; Cao et al., 2020); H is the proportion of edible forage (%), estimated as 0.76, 0.69, and 0.76 for alpine meadow, alpine steppe and alpine desert-steppe, respectively, according to observed data in North Tibet; S is the daily feed intake per SSU, set as 1.33 kg (Fan et al., 2010); G is grazing days (d), set as 365.
In our study, we regarded yield (Y) as equivalent to the potential aboveground biomass (AGBp), which was not grazed by herbivores. Grassland ANPPp can be estimated according to the terrestrial ecosystem model (TEM).
TEM is one of the process-based ecosystem models driven by spatially referenced information on vegetation type, climate, elevation, soils, and water availability to calculate the monthly carbon and nitrogen fluxes and pool sizes of terrestrial ecosystems. TEM can only be applied in a mature and undisturbed ecosystem without considering the effects of land use. TEM NPP (NPPp) was calculated by the difference between gross primary productivity (GPP) and autotrophic respiration (Ra) in the monthly time step. Ra is considered as the sum of maintenance respiration (Rm) and growth respiration (Rg). Monthly GPP is driven by several factors and is calculated as:
where Cmax represents the maximum rate of C assimilation by plants in 1 month (g C m–2 month–1). The function of f(PAR), f(T), and f(NA) accounts for the effects of photosynthetically active radiation, temperature and relative nutrient availability, respectively, on GPP. f(LEAF) is the leaf area relative to the maximum annual leaf area and depends on monthly estimated evapotranspiration. f(CO2, H2O) is the interactive effects of atmospheric CO2 concentrations and moisture availability to GPP. In this study, the value of Cmax was set as 949.6 g C m–2 month–1 for alpine meadow, 617.9 g C m–2 month–1 for alpine steppe and 251.2 g C m–2 month–1 for desert steppe (Chen et al., 2014).
Statistical Analysis
The differences in climatic variables, grazing intensity, and alpine grassland ANPP before and after the implementation of the “Ecological Security Barrier Protection and Construction Project in Tibet” were calculated by the following formula:
where V represents each of mean annual temperature (MAT), annual total precipitation (AP), annual total radiation (AR), GI, and ANPP, respectively. We first calculated the zonal mean of each variable at each county in Tibet. Then, we employed a random forest (RF) regression analysis to identify the relative importance of the four variables on the variation in grassland ANPP (Huang and Xia, 2019). In the RF model, the response variable was the differences in the mean ANPP between the two periods, and the predictors were the differences in the mean values of MAT, AP, AR, and GI. The importance of each predictor variable is defined as the percentage increase in the mean square error (%IncMSE) between observations and predictions, and the decrease is averaged over all the trees to produce the final estimation for importance (Delgado-Baquerizo et al., 2017; Huang and Xia, 2019). High%IncMSE values represent high importance for each predictor. The RF model was run using R 4.0.2 with randomForest_4.6-124.
Results
Validation of Grassland ANPP and Potential Aboveground Biomass
To assure the accuracy of the ANPP and AGBp, observed ANPP data in open grazed grasslands and observed AGB data in fenced grasslands were used to validate the simulated ANPP and AGBp, respectively. The results showed that both simulated ANPP and AGBp matched well with observed records. The simulated ANPP can explain 80% of the variance in observed ANPP of open alpine grasslands (Figure 2A), and the simulated AGBp can explain 78% of the variance in observed AGBp of fenced alpine grasslands (Figure 2B).
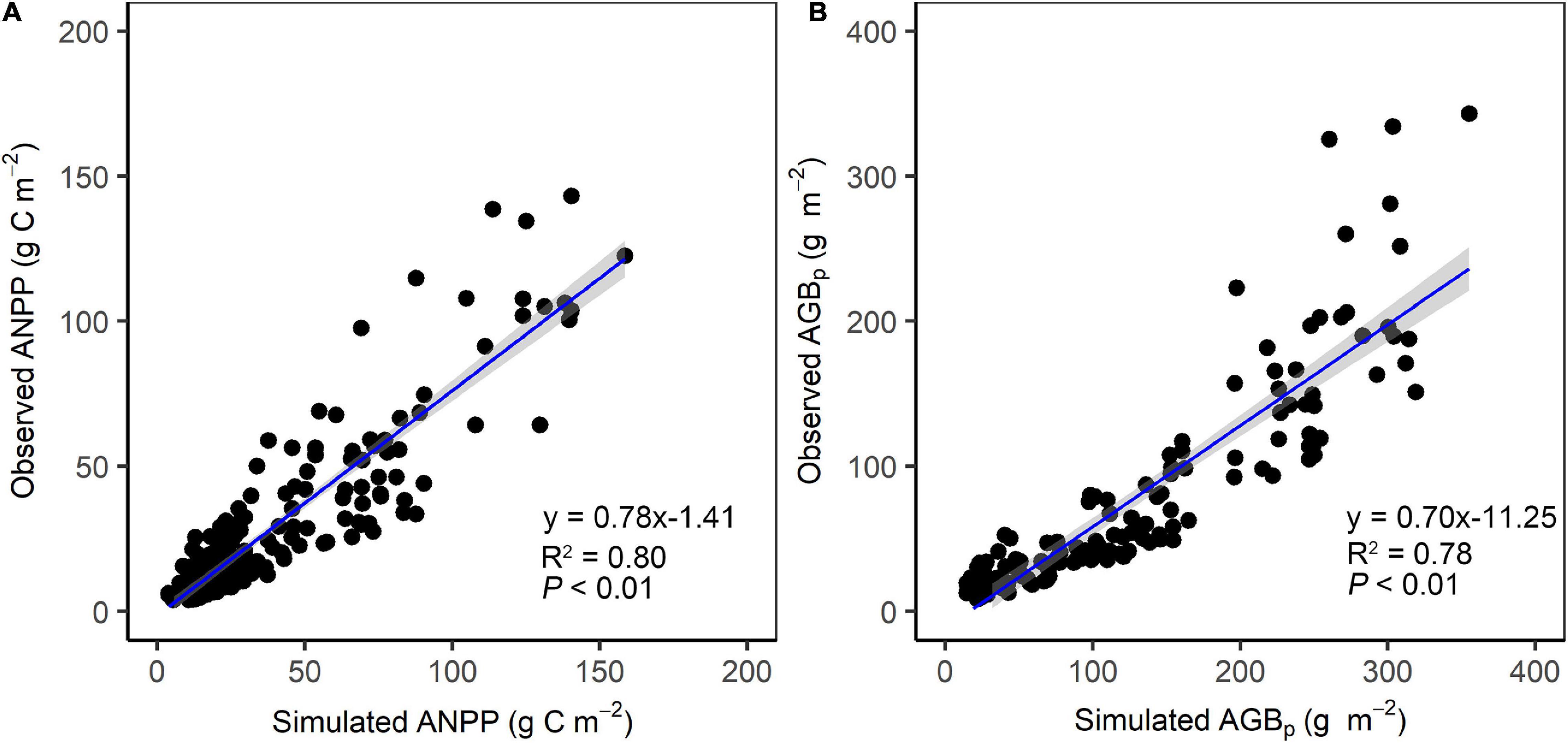
Figure 2. Comparisons (A) between simulated and observed ANPP and (B) between simulated and observed AGBp.
Dynamics of Climatic Variables and Grazing Intensity Between Two Subperiods
Both MAT and AP showed a non-significant increasing trend in the period of before (2000–2008) and after the implementation (2009–2017) of the “Ecological Security Barrier Protection and Construction Project.” MAT trend was faster, but AP trend was slower in 2000–2008, compared to those in 2009–2017 (Figures 3A,B). AR trend was similar to the GI trend in the two periods, showing an initial non-significant increasing trend and then a significant decreasing trend (Figures 3C,D).
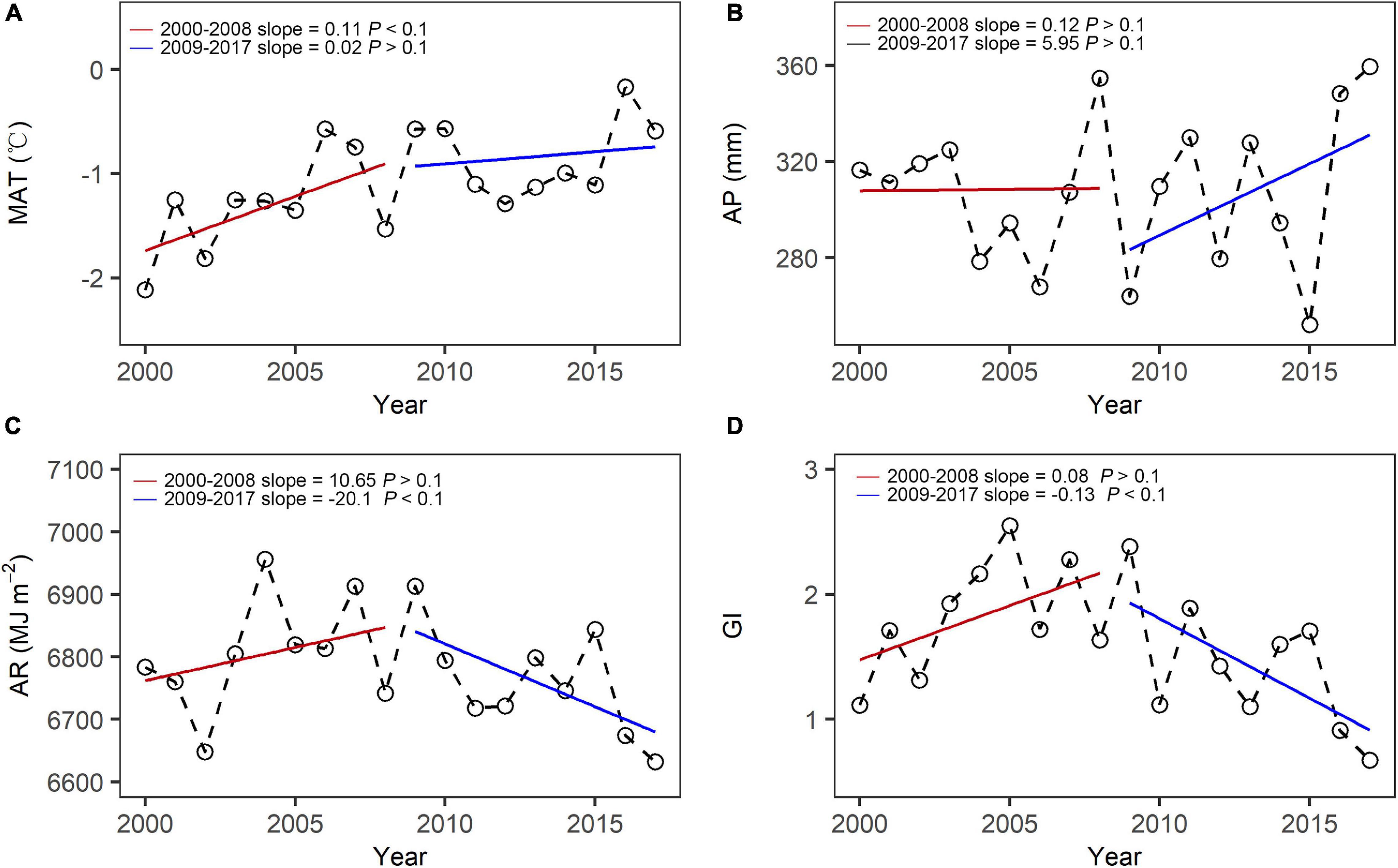
Figure 3. Inter-annual dynamics of (A) MAT, (B) AP, (C) AR, and (D) GI before and after the implementation of the “Ecological Security Barrier Protection and Construction Project.”
The changes in climatic variables and grazing intensity in Tibet were non-uniform across space (Figure 4). Warming was evident across the whole plateau, especially in northern Tibet, where MAT increased by 0.6°C (Figure 4A). Considerable increase and decrease in AP also occurred in northern and western Tibet (ΔAP > 20 mm) and in central and eastern regions, respectively (Figure 4B). In contrast, AR was dimming (negative ΔAR) in northern and western Tibet but lighting (positive ΔAR) in eastern areas (Figure 4C). Regarding grazing intensity, it increased in the middle Tibet after the implementation of the “Ecological Security Barrier Protection and Construction Project” and decreased in the edge counties of the Tibetan Autonomous Region (Figure 4D).
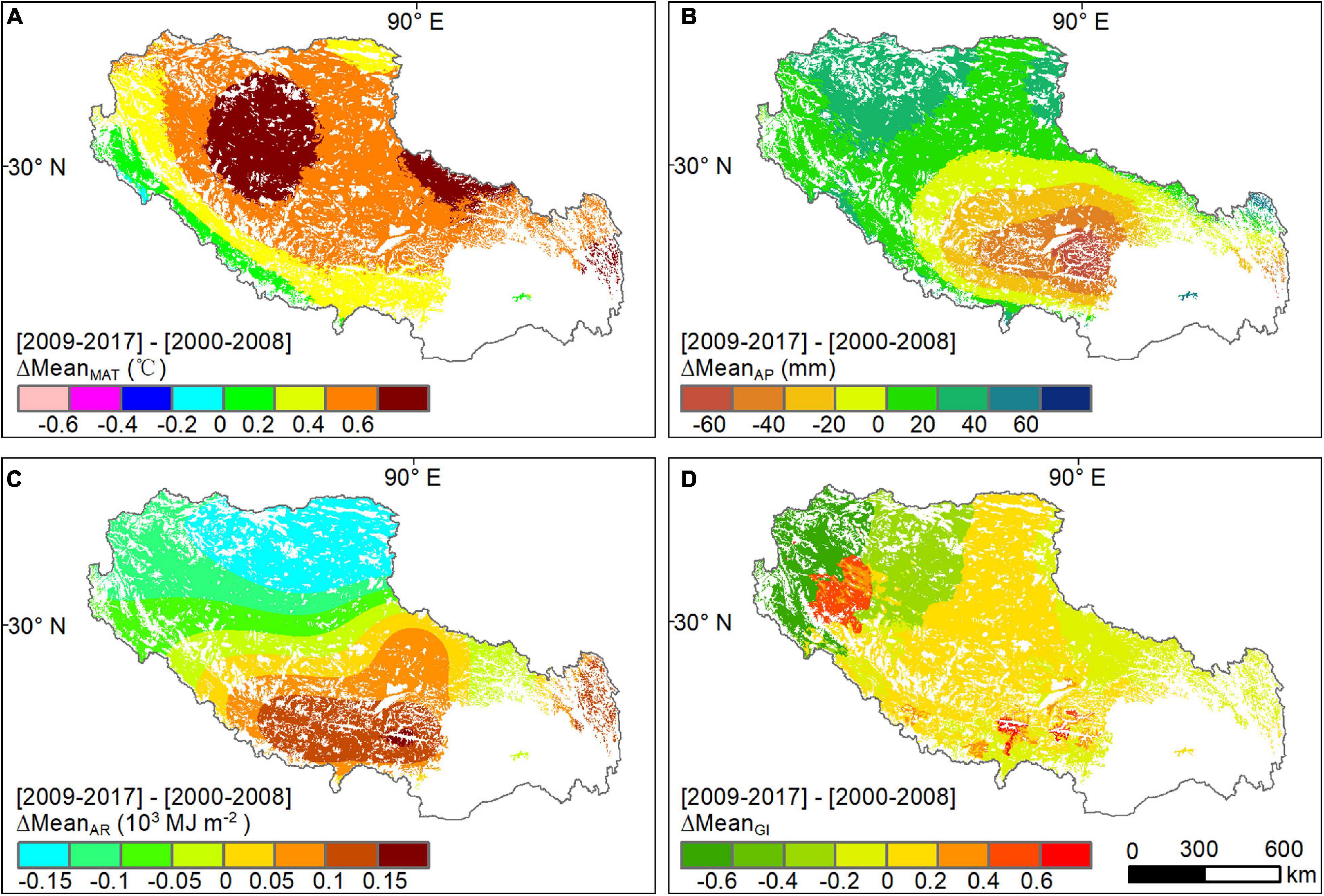
Figure 4. Distribution of the mean-difference in (A) MAT, (B) AP, (C) AR, and (D) GI between before and after the implementation of the “Ecological Security Barrier Protection and Construction Project.”
Dynamics of Grassland Productivity Between Two Subperiods
Grassland ANPP in Tibet decreased from southeast to northwest with grassland types, from alpine meadow, alpine steppe to alpine desert steppe (Figures 5A,B). Mean ANPP for all grassland types (28.4 g C m–2) during 2009–2017 increased by 5.2%, compared to 27.0 g C m–2 during 2000–2008. ANPP increased at approximately 81% of grassland pixels (Figure 5C). Mean ANPP significantly increased by 2.41 g C m–2 (ΔMeanANPP) in alpine meadows, followed by alpine desert steppes (ΔMeanANPP = 0.93 g C m–2) and alpine steppes (ΔMeanANPP = 0.88 g C m–2), respectively (Figure 6A). ANPP decreased in the remaining 19% of grassland pixels, mainly in central Tibet (Figure 5C).
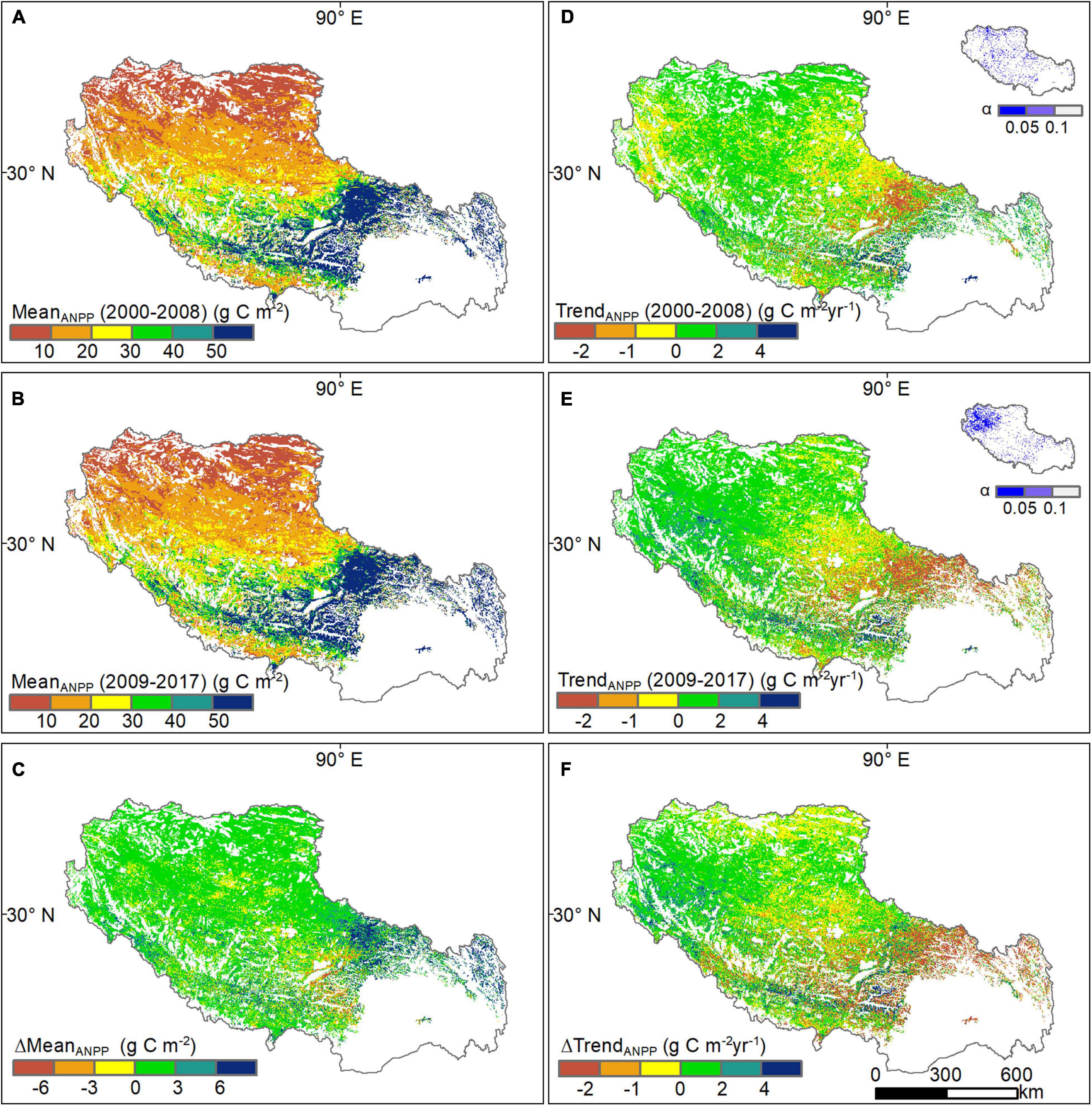
Figure 5. Changes in grassland ANPP across Tibet. (A,B) The average of grassland ANPP in the two subperiods, before (2000-2008) and after (2009–2017) starting the “Ecological Security Barrier Protection and Construction Project.” (C) The mean-difference of grassland ANPP between the two subperiods. (D,E) The changing trend in grassland ANPP in the two subperiods. (F) The trend-difference in grassland ANPP between the two subperiods.
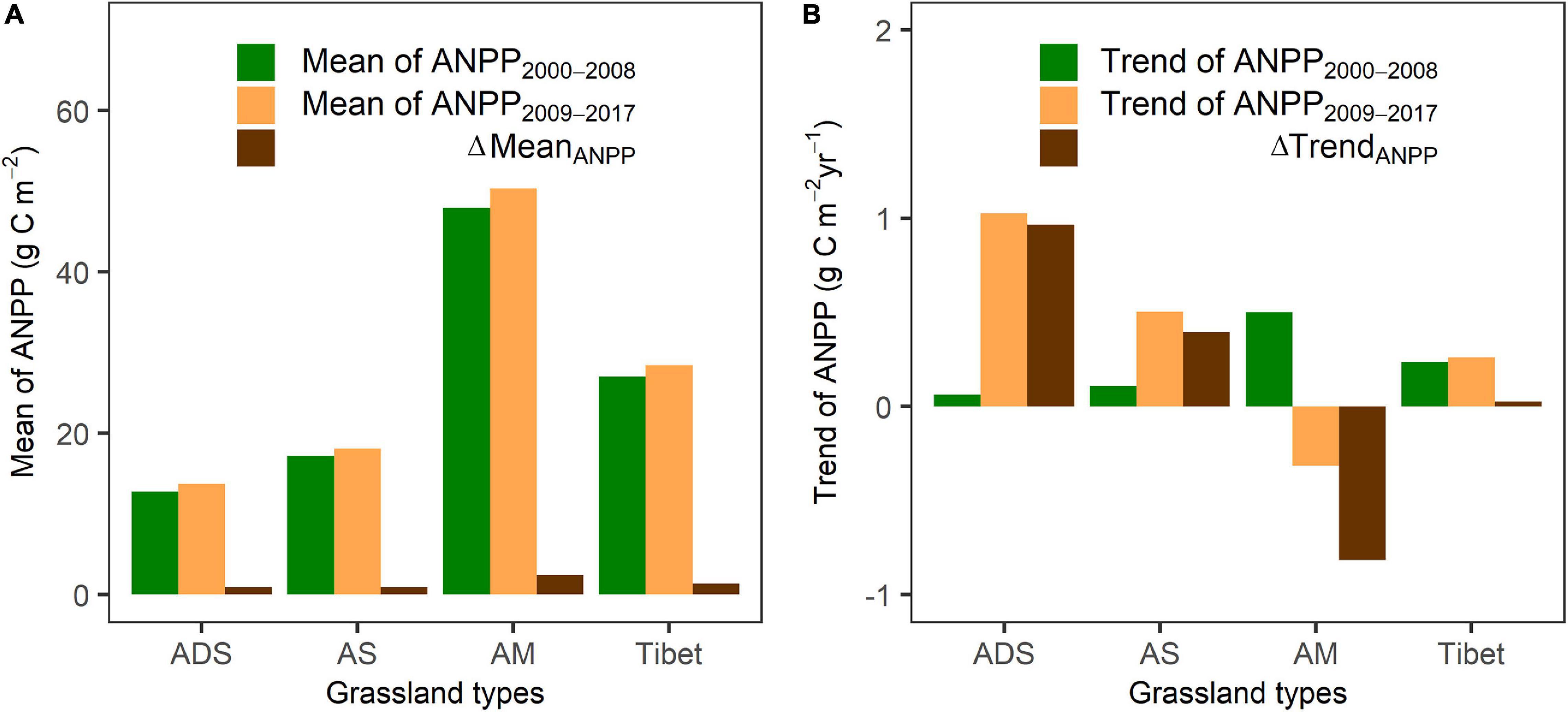
Figure 6. The (A) mean and (B) trend of grassland ANPP in the two subperiods, before (2000–2008) and after (2009–2017) staring the “Ecological Security Barrier Protection and Construction Project” for each grassland type in Tibet.
ANPP decreased during 2000–2008 for 54.2% of grasslands in central Tibet (Figure 5D). However, ANPP mainly increased during 2009–2017 in western and southern Tibet (Figure 5E). The ANPP trend during 2009–2017 (0.26 g C m–2 year–1) for all grasslands increased by 13.0% (Figures 5F, 6B), compared with that during 2000–2008 (0.23 g C m–2 year–1). Especially, alpine desert steppes increased most evidently (1.03 g C m–2 year–1), followed by alpine steppes (0.50 g C m–2 year–1). The ANPP of alpine meadows reversed from an increasing trend (0.50 g C m–2 year–1) during 2000–2008 to a decreasing trend (–0.31 g C m–2 year–1) during 2009–2017 (Figure 6B).
Effects of Climate and Grazing Intensity on Grassland ANPP Changes
The correlation coefficients between ANPP and three climatic variables (MAT, AP, and AR) and grazing intensity (GI) were presented in Figure 7. The ANPP had weak positive correlation with MAT (r = 0.20), AP (r = 0.13), and AR (r = 0.06) and weak negative correlation with GI (r = –0.12) during 2000–2008. The four variables had only explained 32% of the variance in ANPP during 2000–2008 (Table 1). The correlation coefficients between ANPP and the four variables changed during 2009–2017. MAT (r = 0.23) and AP (r = 0.42) had more positive effects on ANPP while AR (r = –0.12) and GI (r = –0.39) had more negative effects during 2009–2017, compared to 2000–2008. The four variables explained 72% of the variance in ANPP during 2009–2017, among which AP became the dominant factor and explained 60% of the variance in ANPP (Table 1).
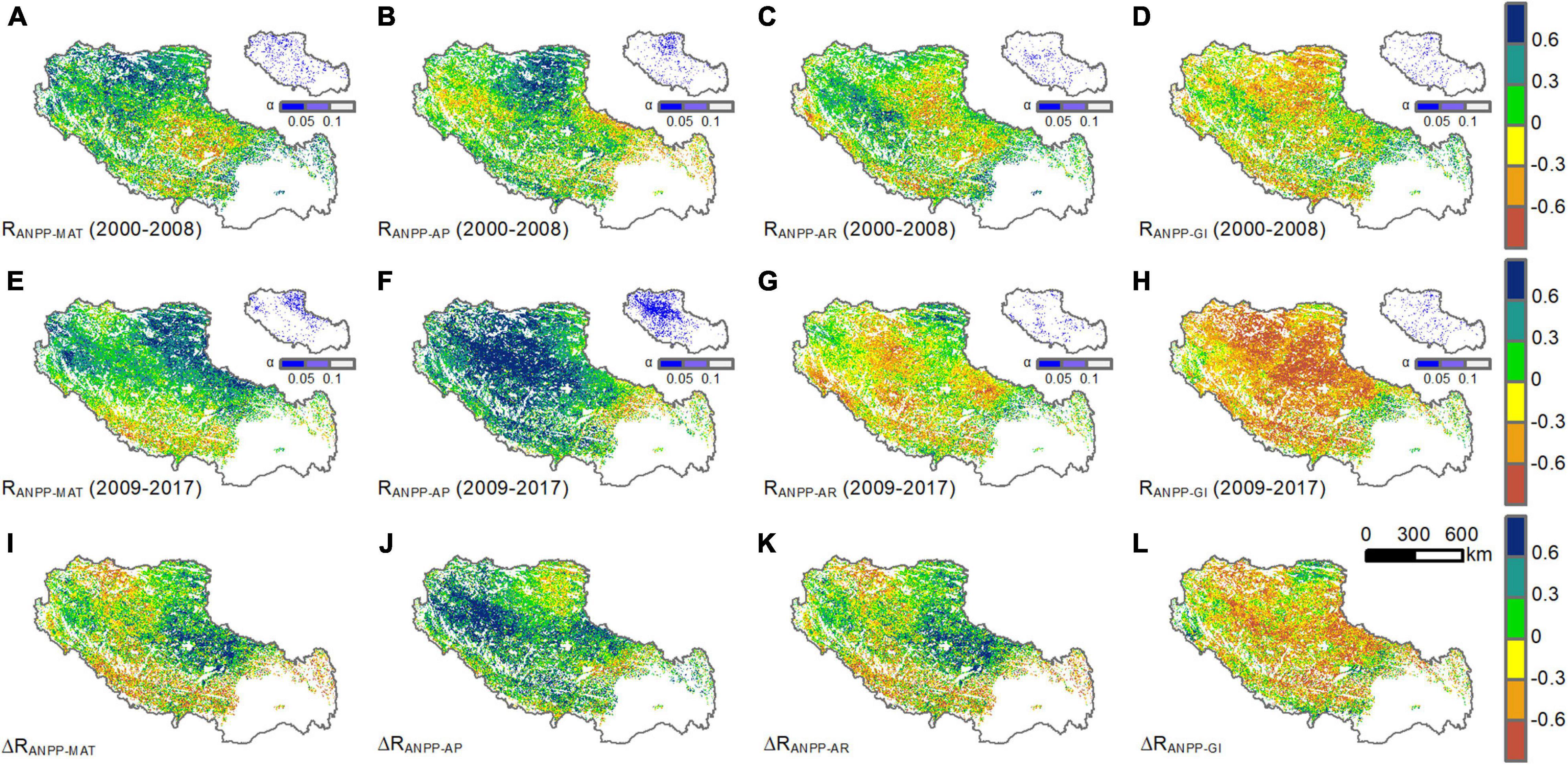
Figure 7. Spatial patterns of the correlation coefficients (R) of grassland ANPP with MAT, AP AR, and GI during (A–D) 2000–2008, (E–H) 2009–2017, and (I–L) the difference in the corresponding correlation coefficients (ΔR) between the two subperiods, before and after the starting “Ecological Security Barrier Protection and Construction Project.”
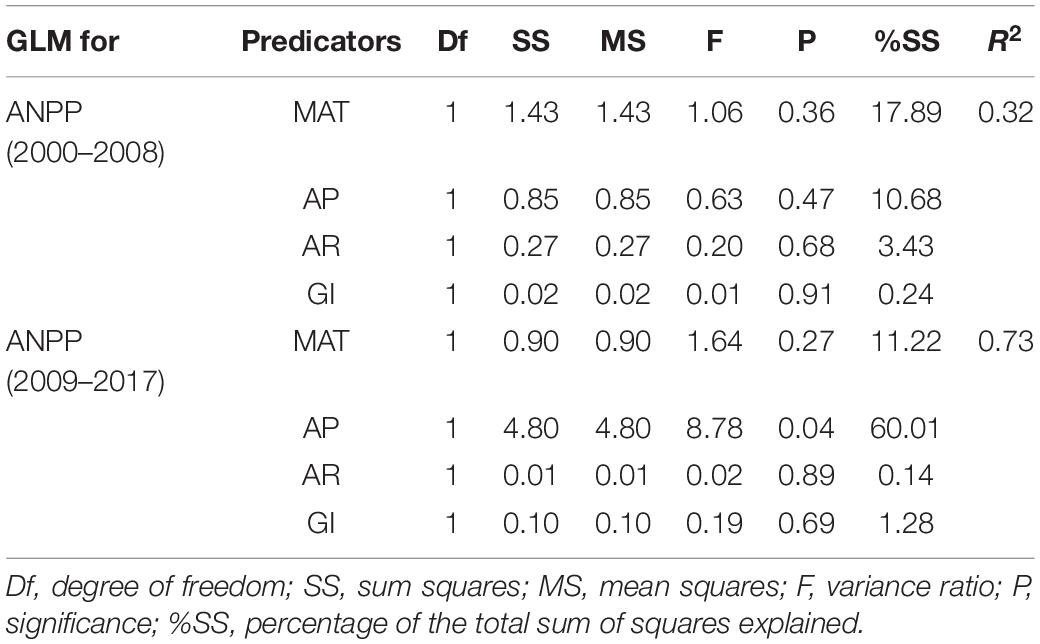
Table 1. Summary of general linear models (GLMs) that included MAT, AP, AR, and GI as predictors for grassland ANPP in the two periods, before and after starting the “Ecological Security Barrier Protection and Construction Project” in Tibet.
RF model was used to quantify the relative contributions of the four responsible variables (ΔMeanMAT, ΔMeanAP, ΔMeanAR, and ΔMeanGI) with respect to the differences in mean ANPP (ΔMeanANPP) between the two subperiods. ΔMeanAP was the primary driver of ΔMeanANPP with% IncMSE of 29.0%, followed by ΔMeanMAT (%IncMSE = 19.9%), ΔMeanAR (%IncMSE = 16.2%) and ΔMeanGI (%IncMSE = 14.5%), respectively (Figure 8).
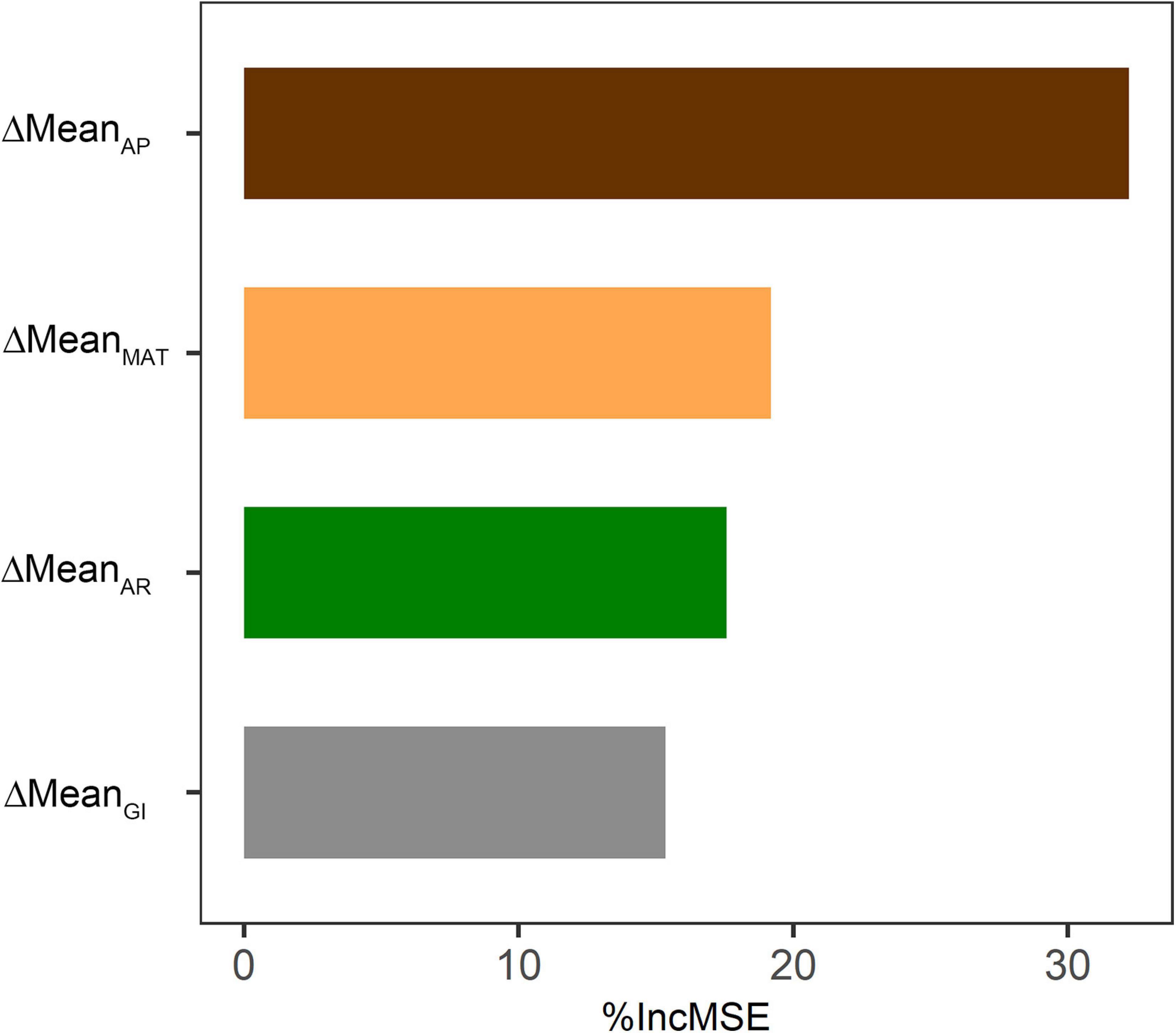
Figure 8. Relative contribution of the differences in means of the four variables (ΔMeanMAT, ΔMeanAP, ΔMeanAR, and ΔMeanGI) to the differences in mean ANPP (ΔMeanANPP) between before and after starting the “Ecological Security Barrier Protection and Construction Project” in RF model. The values were denoted by the percentage increase of mean squared error (%IncMSE).
Discussion
Quantifying the key ecosystems’ dynamics controlling factors is crucial for ecological management and adaptation (Li L. et al., 2018; Wei et al., 2020). Alpine grasslands in Tibet are one of the most vulnerable biomes to human activities and climate change in the world. However, it is still a question of debate how and at what extent different drivers contribute to grassland productivity change on this plateau. In this study, we first used the CASA model to quantify the alpine grassland ANPP in Tibet from 2000 to 2017. Then, we investigated the changes in grassland ANPP before (2000–2008) and after (2009–2017) the implementation of the “Ecological Security Barrier Protection and Construction Project.” Finally, an RF model was used to quantify the relative importance of temperature, precipitation, radiation, and grazing intensity to the dynamics of grassland ANPP.
Increased ANPP Due to More Favorable Climate
We found that ANPP after the implementation of the project increased to approximately 81% of alpine grasslands in Tibet. The mean values of grassland ANPP increased from 27.0 g C m–2 before to 28.4 g C m–2 after the project. This finding was consistent with Wang et al. (2017) and Huang et al. (2018) who also examined that vegetation coverage and forage supply capacity in Tibet increased slightly after the start of the project. Correlation analysis revealed that this increase in ANPP might be attributed to more favorable climatic conditions and the decrease in the grazing intensity after the project. Further, the climate in Tibet became warmer and wetter after the project’s start, which positively affected the grassland ANPP. We also found that the increased precipitation had a higher effect on the f grassland ANPP than temperature. Nonetheless, this finding is not in agreement with Zhu et al. (2016) who concluded that alpine plants are sensitive to temperature as the average growing season temperature is lower than the optimum air temperature of vegetation productivity in global alpine regions (Huang et al., 2019). However, the finding is in line with long-term in situ monitoring, manipulative experiments, satellite remote sensing, and model simulations on this plateau. For example, Wu et al. (2016) found that growing season precipitation explained the most variance of alpine grassland species richness and aboveground biomass across the northern Tibetan Plateau. Fu et al. (2018) reported that increased precipitation has more strong effects on plant production in alpine meadows than experimental warming in Tibet. A potential explanation is that colder habitats have long shaped alpine vegetation’s functional traits to adapt to the low temperatures and large diurnal temperature ranges (Elmendorf et al., 2012; Shi et al., 2014). This might be due to the reason that arctic and alpine plants have excellent resistance to short-term temperature fluctuations (Theurillat and Guisan, 2001; Elmendorf et al., 2012).
Increased ANPP Due to Weakening Grazing Intensity
Human activities were significantly intense across the Tibetan Plateau before the implementation of the project. For example, the population reached upto 3.12 million in the Tibetan Autonomous Region of China, in 2013, which is twice as in 1965; meanwhile, the livestock number also increased from 9.74 million in 1958 to 23 million in the early twenty-first century (Fan et al., 2015). This study found that grazing intensity was negatively correlated with ANPP, suggesting that grazing activities can mediate the interannual ANPP change. After the start of the project, grazing intensity decreased significantly (Figure 3D), owing to the increase in the potential grassland productivity and the reduction in the livestock (Supplementary Figure S1). This significant decrease in the grazing intensity had a positive effect on grassland ANPP. Grazing and human-induced land use/cover change are the two most significant human disturbances for alpine grassland ANPP in Tibet, and the former is supposed to be the dominant one (Arthur et al., 2008; Harris, 2010; Chen et al., 2014). The complicated process of grazing, such as forage selection, herbivores and their trampling (Parsons and Dumont, 2003; Paruelo et al., 2008), directly and indirectly, can modify grassland productivity (Chen et al., 2007; Xiong et al., 2014; Wang and Wesche, 2016). Meanwhile, grassland productivity is highly dependent on grazing and its intensity. The prevailing view is that moderate grazing promotes plant growth due to compensatory growth while overgrazing reduces vegetation productivity (de Mazancourt et al., 1998; Schuman et al., 1999; Luo et al., 2012). At the end of the twentieth century, the stocking rates of livestock in Tibet were high in most of its counties (Fan et al., 2015). Overgrazing affected the native species diversity and ecosystem stability in Tibet and altered the structure and function of grassland ecosystems, induced C losses and grassland degradation (Zhou et al., 2017; Zhang et al., 2019). The Chinese government continuously formulates policies to limit grazing throughout the project, such as grazing exclusion, conversion of grazing land to grass and grazing withdrawal programs. This decline in grazing intensity after the start of the project elevated grassland productivity. Thus, we concluded that the check on the livestock numbers was a useful tool for the restoration of grassland in Tibet.
Climate Variability Dominated the ANPP Dynamic
The RF model revealed that the grazing intensity is far less critical than climatic variables in controlling grassland ANPP. This signifies that climate change is the primary driver for the sustainability of alpine grassland ecosystems on the Tibetan Plateau (Huang et al., 2016; Lehnert et al., 2016; Xu et al., 2016). Although the intensity of human activities on the Tibetan Plateau is increasing rapidly, however, the impact of human activities on ecosystems is less than other regions of the world (Venter et al., 2016; Li S. et al., 2018). Grazing activities have not altered the dominant role of climate change for grassland variation in Tibet. However, we must highlight that, as suggested by previous studies, the biochemical cycle and their feedbacks to climate change would get more complicated under human disturbance (Kröel-Dulay et al., 2015; Peters et al., 2019). For example, in our study, we found that the relationships between climatic variables and grassland ANPP were more robust before the project’s start. Those changes in correlation coefficients might cause by the changes in grazing intensity. Thus, the mechanisms of how plants respond to the coupled effects of climatic and anthropogenic stresses should be explored in the future.
Conclusion
This study used 9 years of datasets before and after the implementation of Ecological Security Barrier Protection and Construction Project, including meteorological conditions, grazing intensity, to explore the combined effects of climate change and grazing activities on the dynamics of grassland ANPP in Tibet. We found an increase in grassland ANPP after the project’s start. Precipitation was the dominant factor in controlling the observed alpine grassland changes during the study period. Furthermore, the weak grazing intensity after the project promoted grassland productivity. Thus, we suggested that the check on the livestock numbers has a positive effect on the restoration of degraded grasslands in Tibet.
Data Availability Statement
The original contributions presented in the study are included in the article/Supplementary Material, further inquiries can be directed to the corresponding author/s.
Author Contributions
JW and ML conceptualized this study and led the writing. ML collected and analyzed the data. YF, BN, YH, and XZ interpreted the results and revised the text. All authors contributed to this work and approved the final manuscript before submission.
Funding
The study was supported by the Second Tibetan Plateau Scientific Expedition and Research (STEP) program (2019QZKK1002) and the Strategic Priority Research Program of the Chinese Academy of Sciences (XDA19050502). JW was funded by a 2 years scholarship from the Alexander von Humboldt Foundation from 2017 to 2019 and supported by the Young Talent Scientist Program of the Chinese Academy of Agricultural Sciences since December 2019.
Conflict of Interest
The authors declare that the research was conducted in the absence of any commercial or financial relationships that could be construed as a potential conflict of interest.
Acknowledgments
We acknowledge the support of all co-authors for their constructive and helpful comments and organization of this study.
Supplementary Material
The Supplementary Material for this article can be found online at: https://www.frontiersin.org/articles/10.3389/fevo.2021.631024/full#supplementary-material
Supplementary Figure 1 | The dynamics of livestock number and potential grassland net primary production (NPPp) in Tibet from 2000 to 2017.
Footnotes
- ^ https://lpdaac.usgs.gov/get_data/data_pool
- ^ http://www.resdc.cn/data.aspx?DATAID=122
- ^ http://www.cma.gov.cn/
- ^ https://cran.rproject.org/web/packages/randomForest
References
Allen, R., Pereira, L., Raes, D., and Smith, M. (1998). Crop Evapotranspiration-Guidelines for Computing Crop Water Requirements-FAO Irrigation and Drainage Paper 56. Rome: FAO.
Arthur, A. D., Pech, R. P., Davey, C., Jiebu, O., Yanming, Z., and Hui, L. (2008). Livestock grazing, plateau pikas and the conservation of avian biodiversity on the Tibetan plateau. Biol. Conserv. 141, 1972–1981. doi: 10.1016/j.biocon.2008.05.010
Cao, Y., Wu, J., Zhang, X., Niu, B., and He, Y. (2020). Comparison of methods for evaluating the forage-livestock balance of alpine grasslands on the Northern Tibetan Plateau. J. Resour. Ecol. 11:272. doi: 10.5814/j.issn.1674-764x.2020.03.004s
Chen, B., Zhang, X., Tao, J., Wu, J., Wang, J., Shi, P., et al. (2014). The impact of climate change and anthropogenic activities on alpine grassland over the Qinghai-Tibet Plateau. Agric. For. Meteorol. 18, 11–18. doi: 10.1016/j.agrformet.2014.01.002
Chen, H., Zhu, Q., Peng, C., Wu, N., Wang, Y., Fang, X., et al. (2013). The impacts of climate change and human activities on biogeochemical cycles on the Qinghai-Tibetan Plateau. Glob. Chang. Biol. 19, 2940–2955. doi: 10.1111/gcb.12277
Chen, Y., Lee, G., Lee, P., and Oikawa, T. (2007). Model analysis of grazing effect on above-ground biomass and above-ground net primary production of a Mongolian grassland ecosystem. J. Hydrol. 333, 155–164. doi: 10.1016/j.jhydrol.2006.07.019
de Mazancourt, C., Loreau, M., and Abbadie, L. (1998). Grazing optimization and nutrient cycling: when do herbivores enhance plant production? Ecology 79, 2242–2252. doi: 10.1890/0012-96581998079
Delgado-Baquerizo, M., Eldridge, D. J., Maestre, F. T., Karunaratne, S. B., Trivedi, P., Reich, P. B., et al. (2017). Climate legacies drive global soil carbon stocks in terrestrial ecosystems. Sci. Adv. 3:7. doi: 10.1126/sciadv.1602008
Dong, S., Shang, Z., Gao, J., and Boone, R. (2020). Enhancing sustainability of grassland ecosystems through ecological restoration and grazing management in an era of climate change on Qinghai-Tibetan Plateau. Agric. Ecosyst. Environ. 287:106684. doi: 10.1016/j.agee.2019.106684
Elmendorf, S. C., Henry, G. H. R., Hollister, R. D., Björk, R. G., Boulanger-Lapointe, N., Cooper, E. J., et al. (2012). Plot-scale evidence of tundra vegetation change and links to recent summer warming. Nat. Clim. Chang. 2, 453–457. doi: 10.1038/nclimate1465
Erb, K. H., Kastner, T., Plutzar, C., Bais, A. L. S., Carvalhais, N., Fetzel, T., et al. (2018). Unexpectedly large impact of forest management and grazing on global vegetation biomass. Nature 553, 73–76. doi: 10.1038/nature25138
Fan, J., Niu, Y., Chen, D., Sun, W., Xu, Y., and Wang, C. (2015). The effects of human activities on the ecological environment of Tibet over the past half century. Chin. Sci. Bull. 60, 3057–3066. doi: 10.1360/n972014-01311
Fan, J., Shao, Q., Liu, J., Wang, J., Harris, W., Chen, Z., et al. (2010). Assessment of effects of climate change and grazing activity on grassland yield in the Three Rivers Headwaters Region of Qinghai-Tibet Plateau, China. Environ. Monit. Assess. 170, 571–584. doi: 10.1007/s10661-009-1258-1
Fu, G., Shen, Z., and Zhang, X. (2018). Increased precipitation has stronger effects on plant production of an alpine meadow than does experimental warming in the Northern Tibetan Plateau. Agric. For. Meteorol. 249, 11–21. doi: 10.1016/j.agrformet.2017.11.017
Gill, D. A., Mascia, M. B., Ahmadia, G. N., Glew, L., Lester, S. E., Barnes, M., et al. (2017). Capacity shortfalls hinder the performance of marine protected areas globally. Nature 543, 665–669. doi: 10.1038/nature21708
Haberl, H., Erb, K. H., Krausmann, F., Gaube, V., Bondeau, A., Plutzar, C., et al. (2007). Quantifying and mapping the human appropriation of net primary production in earth’s terrestrial ecosystems. Proc. Natl. Acad. Sci. U.S.A. 104, 12942–12945. doi: 10.1073/pnas.0704243104
Harris, R. B. (2010). Rangeland degradation on the Qinghai-Tibetan plateau: a review of the evidence of its magnitude and causes. J. Arid Environ. 74, 1–12. doi: 10.1016/j.jaridenv.2009.06.014
Heung, B., Bulmer, C. E., and Schmidt, M. G. (2014). Predictive soil parent material mapping at a regional-scale: a random forest approach. Geoderma 214–215, 141–154. doi: 10.1016/j.geoderma.2013.09.016
Huang, K., and Xia, J. (2019). High ecosystem stability of evergreen broadleaf forests under severe droughts. Glob. Chang. Biol. 25, 3494–3503. doi: 10.1111/gcb.14748
Huang, K., Zhang, Y., Zhu, J., Liu, Y., Zu, J., and Zhang, J. (2016). The influences of climate change and human activities on vegetation dynamics in the Qinghai-Tibet Plateau. Remote Sens. 8:876. doi: 10.3390/rs8100876
Huang, L., Cao, W., Xu, X., Fan, J., and Wang, J. (2018). The ecological effects of ecological security barrier protection and construction project in Tibet Plateau (in Chinese). J. Nat. Resour. 33, 398–411. doi: 10.11849/zrzyxb.20170116
Huang, M., Piao, S., Ciais, P., Peñuelas, J., Wang, X., Keenan, T. F., et al. (2019). Air temperature optima of vegetation productivity across global biomes. Nat. Ecol. Evol. 3, 772–779. doi: 10.1038/s41559-019-0838-x
Hutchinson, M. (2004). Anusplin Version 4.3. Centre for Resource and Environmental Studies. Canberra: The Australian National University.
Knight, J., and Harrison, S. (2012). The impacts of climate change on terrestrial Earth surface systems. Nat. Clim. Chang. 3:24. doi: 10.1038/nclimate1660
Kröel-Dulay, G., Ransijn, J., Schmidt, I. K., Beier, C., De Angelis, P., de Dato, G., et al. (2015). Increased sensitivity to climate change in disturbed ecosystems. Nat. Commun. 6:6682. doi: 10.1038/ncomms7682
Lehnert, L. W., Wesche, K., Trachte, K., Reudenbach, C., and Bendix, J. (2016). Climate variability rather than overstocking causes recent large scale cover changes of Tibetan pastures. Sci. Rep. 6:24367. doi: 10.1038/srep24367
Li, L., Zhang, Y., Liu, L., Wu, J., Li, S., Zhang, H., et al. (2018). Current challenges in distinguishing climatic and anthropogenic contributions to alpine grassland variation on the Tibetan Plateau. Ecol. Evol. 8, 5949–5963. doi: 10.1002/ece3.4099
Li, S., Wu, J., Gong, J., and Li, S. (2018). Human footprint in Tibet: assessing the spatial layout and effectiveness of nature reserves. Sci. Total Environ. 621, 18–29. doi: 10.1016/j.scitotenv.2017.11.216
Li, M., Zhang, X., He, Y., Niu, B., and Wu, J. (2020). Assessment of the vulnerability of alpine grasslands on the Qinghai-Tibetan Plateau. PeerJ 8:e8513. doi: 10.7717/peerj.8513
Lieth, H., and Whittaker, R. H. (1978). Primary Productivity of the Biosphere. New York, NY: Springer.
Lin, D., Xia, J., and Wan, S. (2010). Climate warming and biomass accumulation of terrestrial plants: a meta-analysis. New Phytol. 188, 187–198. doi: 10.1111/j.1469-8137.2010.03347.x
Long, R., Shang, Z., Guo, X., and Ding, L. (2009). “Qinghai–Tibetan plateau rangelands,” in Rangeland Degradation and Recovery in China’s Pastoral Lands, eds V. R. Squires, X. Lu, Q. Lu, T. Wang, and Y. Yang (Wallingford: CABI), 184–196.
Luo, G., Han, Q., Zhou, D., Li, L., Chen, X., Li, Y., et al. (2012). Moderate grazing can promote aboveground primary production of grassland under water stress. Ecol. Complex. 11, 126–136. doi: 10.1016/j.ecocom.2012.04.004
Parsons, A. J., and Dumont, B. (2003). Spatial heterogeneity and grazing processes. Anim. Res. 52, 161–179. doi: 10.1051/animres:2003013
Paruelo, J. M., Pütz, S., Weber, G., Bertiller, M., Golluscio, R. A., Aguiar, M. R., et al. (2008). Long-term dynamics of a semiarid grass steppe under stochastic climate and different grazing regimes: a simulation analysis. J. Arid Environ. 72, 2211–2231. doi: 10.1016/j.jaridenv.2008.07.010
Paudel, K. P., and Andersen, P. (2010). Assessing rangeland degradation using multi temporal satellite images and grazing pressure surface model in Upper Mustang, Trans Himalaya, Nepal. Remote Sens. Environ. 114, 1845–1855. doi: 10.1016/j.rse.2010.03.011
Peters, M. K., Hemp, A., Appelhans, T., Becker, J. N., Behler, C., Classen, A., et al. (2019). Climate–land-use interactions shape tropical mountain biodiversity and ecosystem functions. Nature 568, 88–92. doi: 10.1038/s41586-019-1048-z
Ruimy, A., Kergoat, L., Bondeau, A., and Intercomparison, T. P. O. T. P. N. M. (1999). Comparing global models of terrestrial net primary productivity (NPP), analysis of differences in light absorption and light-use efficiency. Glob. Chang. Biol. 5, 56–64. doi: 10.1046/j.1365-2486.1999.00007.x
Schuman, G. E., Reeder, J. D., Manley, J. T., Hart, R. H., and Manley, W. A. (1999). Impact of grazing management on the carbon and nitrogen balance of a mixed-grass Rangeland. Ecol. Appl. 9, 65–71. doi: 10.2307/2641168
Seiferling, I., Proulx, R., and Wirth, C. (2014). Disentangling the environmental-heterogeneity–species-diversity relationship along a gradient of human footprint. Ecology 95, 2084–2095. doi: 10.1890/13-1344.1
Shi, Y., Wang, Y., Ma, Y., Ma, W., Liang, C., Flynn, D. F. B., et al. (2014). Field-based observations of regional-scale, temporal variation in net primary production in Tibetan alpine grasslands. Biogeosciences 11, 16843–16878. doi: 10.5194/bg-11-2003-2014
Tao, J., Zhang, Y., Dong, J., Fu, Y., Zhu, J., Zhang, G., et al. (2015). Elevation-dependent relationships between climate change and grassland vegetation variation across the Qinghai-Xizang Plateau. Int. J. Climatol. 35, 1638–1647. doi: 10.1002/joc.4082
Theurillat, J. P., and Guisan, A. (2001). Potential impact of climate change on vegetation in the european alps: a review. Clim. Chang. 50, 77–109. doi: 10.1023/A:1010632015572
Tong, X., Brandt, M., Yue, Y., Horion, S., Wang, K., Keersmaecker, W. D., et al. (2018). Increased vegetation growth and carbon stock in China karst via ecological engineering. Nat. Sustain. 1, 44–50. doi: 10.1038/s41893-017-0004-x
Venter, O., Sanderson, E. W., Magrach, A., Allan, J. R., Beher, J., Jones, K. R., et al. (2016). Sixteen years of change in the global terrestrial human footprint and implications for biodiversity conservation. Nat. Commun. 7:12558. doi: 10.1038/ncomms12558
Wang, X., Cheng, G., Zhao, T., Zhang, X., Zhu, L., and Huang, L. (2017). Assessment on protection and construction of ecological safety shelter for Tibet (in Chinese). Bull. Chin. Acad. Sci. 32, 29–34. doi: 10.16418/j.issn.1000-3045.2017.01.004
Wang, Y., and Wesche, K. (2016). Vegetation and soil responses to livestock grazing in Central Asian grasslands: a review of Chinese literature. Biodivers. Conserv. 25, 2401–2420. doi: 10.1007/s10531-015-1034-1
Wang, Y. B., Wang, G. X., Shen, Y. P., and Wang, Y. L. (2005). Degradation of the eco-environmental system in alpine meadow on the Tibetan Plateau. J. Glaciol. Geocryol. 337, 517–522.
Wei, D., Zhao, H., Zhang, J., Qi, Y., and Wang, X. (2020). Human activities alter response of alpine grasslands on Tibetan Plateau to climate change. J. Environ. Manag. 262:110335. doi: 10.1016/j.jenvman.2020.110335
Wu, J., Wurst, S., and Zhang, X. (2016). Plant functional trait diversity regulates the nonlinear response of productivity to regional climate change in Tibetan alpine grasslands. Sci. Rep. 6:35649. doi: 10.1038/srep35649
Wu, J., Zhang, X., Shen, Z., Shi, P., Xu, X., and Li, X. (2013). Grazing-exclusion effects on aboveground biomass and water-use efficiency of alpine Grasslands on the Northern Tibetan Plateau. Rangeland Ecol. Manag. 66, 454–461. doi: 10.2111/REM-D-12-00051.1
Wu, Y., Tan, H., Deng, Y., Wu, J., Xu, X., Wang, Y., et al. (2010). Partitioning pattern of carbon flux in a Kobresia grassland on the Qinghai-Tibetan Plateau revealed by field 13C pulse-labeling. Glob. Chang. Biol. 16, 2322–2333. doi: 10.1111/j.1365-2486.2009.02069.x
Wu, Z., Dijkstra, P., Koch, G., Penuelas, J., and Hungate, B. A. (2011). Responses of terrestrial ecosystems to temperature and precipitation change: a meta-analysis of experimental manipulation. Glob. Chang. Biol. 17, 927–942. doi: 10.1111/j.1365-2486.2010.02302.x
Xiong, D., Shi, P., Sun, Y., Wu, J., and Zhang, X. (2014). Effects of grazing exclusion on plant productivity and soil carbon, nitrogen storage in alpine meadows in northern Tibet, China. Chin. Geogr. Sci. 24, 488–498. doi: 10.1007/s11769-014-0697-y
Xu, H., Wang, X., and Zhang, X. (2016). Alpine grasslands response to climatic factors and anthropogenic activities on the Tibetan Plateau from 2000 to 2012. Ecol. Eng. 92, 251–259. doi: 10.1016/j.ecoleng.2016.04.005
Yang, G., Peng, C., Chen, H., Dong, F., Wu, N., Yang, Y., et al. (2017). Qinghai–Tibetan Plateau peatland sustainable utilization under anthropogenic disturbances and climate change. Ecosyst. Health Sust. 3:e01263. doi: 10.1002/ehs2.1263
Yao, T., Thompson, L. G., Mosbrugger, V., Zhang, F., Ma, Y., Luo, T., et al. (2012). Third Pole Environment (TPE). Environ. Dev. 3, 52–64. doi: 10.1016/j.envdev.2012.04.002
Yu, C., Zhang, Y., Claus, H., Zeng, R., Zhang, X., and Wang, J. (2012). Ecological and environmental issues faced by a developing Tibet. Environ. Sci. Technol. 46, 1979–1980. doi: 10.1021/es2047188
Zhang, Y., Qi, W., Zhou, C., Ding, M., Liu, L., Gao, J., et al. (2013). Spatial and temporal variability in the net primary production (NPP) of alpine grassland on Tibetan Plateau from 1982 to 2009 (in Chinese). Acta Geogr. Sin. 68, 1197–1211. doi: 10.11821/dlxb201309004
Zhang, Y., Xu, M., Chen, H., and Adams, J. (2009). Global pattern of NPP to GPP ratio derived from MODIS data: effects of ecosystem type, geographical location and climate. Glob. Ecol. Biogeogr. 18, 280–290. doi: 10.1111/j.1466-8238.2008.00442.x
Zhang, Y., Zhu, Y., Li, J., and Chen, Y. (2019). Current status and future directions of the Tibetan Plateau ecosystem research. Sci. Bull. 64:428. doi: 10.1016/j.scib.2019.03.009
Zhou, G., Zhou, X., He, Y., Shao, J., Hu, Z., Liu, R., et al. (2017). Grazing intensity significantly affects belowground carbon and nitrogen cycling in grassland ecosystems: a meta-analysis. Glob. Chang. Biol. 23, 1167–1179. doi: 10.1111/gcb.13431
Zhou, X., Yamaguchi, Y., and Arjasakusuma, S. (2018). Distinguishing the vegetation dynamics induced by anthropogenic factors using vegetation optical depth and AVHRR NDVI: a cross-border study on the Mongolian Plateau. Sci. Total Environ. 616–617, 730–743. doi: 10.1016/j.scitotenv.2017.10.253
Keywords: climate change, grassland degradation, human activities, NPP, Tibetan Plateau
Citation: Li M, Wu J, Feng Y, Niu B, He Y and Zhang X (2021) Climate Variability Rather Than Livestock Grazing Dominates Changes in Alpine Grassland Productivity Across Tibet. Front. Ecol. Evol. 9:631024. doi: 10.3389/fevo.2021.631024
Received: 19 November 2020; Accepted: 12 February 2021;
Published: 08 March 2021.
Edited by:
Zoltán Bátori, University of Szeged, HungaryReviewed by:
Chengcheng Gang, Chinese Academy of Sciences, ChinaGyörgy Kröel-Dulay, Hungarian Academy of Science, Hungary
Copyright © 2021 Li, Wu, Feng, Niu, He and Zhang. This is an open-access article distributed under the terms of the Creative Commons Attribution License (CC BY). The use, distribution or reproduction in other forums is permitted, provided the original author(s) and the copyright owner(s) are credited and that the original publication in this journal is cited, in accordance with accepted academic practice. No use, distribution or reproduction is permitted which does not comply with these terms.
*Correspondence: Jianshuang Wu, d3VqaWFuc2h1YW5nQGNhYXMuY24=