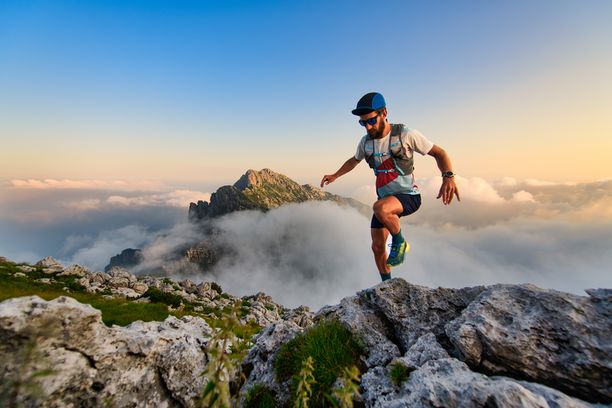
94% of researchers rate our articles as excellent or good
Learn more about the work of our research integrity team to safeguard the quality of each article we publish.
Find out more
ORIGINAL RESEARCH article
Front. Ecol. Evol., 08 March 2021
Sec. Population, Community, and Ecosystem Dynamics
Volume 9 - 2021 | https://doi.org/10.3389/fevo.2021.630944
This article is part of the Research TopicNew Perspectives and Emerging Directions in Predator–Prey Functional Response Research: Hommage to C.S. Holling (1930–2019)View all 18 articles
The functional response is at the core of any predator-prey interactions as it establishes the link between trophic levels. The use of inaccurate functional response can profoundly affect the outcomes of population and community models. Yet most functional responses are evaluated using phenomenological models which often fail to discriminate among functional response shapes and cannot identify the proximate mechanisms regulating predator acquisition rates. Using a combination of behavioral, demographic, and experimental data collected over 20 years, we develop a mechanistic model based on species traits and behavior to assess the functional response of a generalist mammalian predator, the arctic fox (Vulpes lagopus), to various tundra prey species (lemmings and the nests of geese, passerines, and sandpipers). Predator acquisition rates derived from the mechanistic model were consistent with field observations. Although acquisition rates slightly decrease at high goose nest and lemming densities, none of our simulations resulted in a saturating response in all prey species. Our results highlight the importance of predator searching components in predator-prey interactions, especially predator speed, while predator acquisition rates were not limited by handling processes. By combining theory with field observations, our study provides support that the predator acquisition rate is not systematically limited at the highest prey densities observed in a natural system. Our study also illustrates how mechanistic models based on empirical estimates of the main components of predation can generate functional response shapes specific to the range of prey densities observed in the wild. Such models are needed to fully untangle proximate drivers of predator-prey population dynamics and to improve our understanding of predator-mediated interactions in natural communities.
A long-standing problem in ecology is to measure how the acquisition rate of a predator varies with prey availability, namely the functional response. Functional response shapes are typically categorized as linear (type I), hyperbolic (type II), or sigmoidal (type III; Holling 1959b,a). This classification is commonly used by ecologists when incorporating predation into population and community models (Turchin and Hanski, 1997; Fryxell et al., 2007; Serrouya et al., 2015), and type II is the most widely applied model (Rall et al., 2012). The shape of the functional response can have major consequences on the outcomes of population and community models. For instance, a type III promotes stability or coexistence whereas a type II destabilizes predator-prey dynamics (Murdoch, 1973; Sinclair et al., 1998). Describing the functional response of pairwise trophic interactions is also important to understand higher-order interactions. For instance, the shape of the functional response alone can profoundly change predictions about the outcome of predator-mediated trophic interactions (Abrams et al., 1998; Holt and Bonsall, 2017).
Although functional responses are at the core of predator-prey theory (Solomon, 1949), most empirical research on functional responses has been conducted under controlled laboratory or field enclosure conditions (96%, n = 116 studies, reviewed by Pawar et al. 2012) where prey density is manipulated, predator consumption is recorded, and the functional response models are compared through statistical analysis (referred to as phenomenological models). These approaches are not well-suited for predators with relatively large home ranges and may fail to reproduce foraging conditions encountered in the wild. Determining the shape of functional responses in natural systems is however often limited by a combination of factors, including small sample sizes, a relatively narrow gradient of observed prey densities, the difficulty to observe predator-prey interactions directly, or the difficulty to estimate predator and prey numbers (Gilg et al., 2006; Therrien et al., 2014; Suryawanshi et al., 2017; Ellis et al., 2019). The large variability around predator acquisition rates observed in the wild can also prevent us from discriminating among functional response shapes, and hence limit our ability to accurately model predator-prey interactions in complex and natural ecosystems (O'Donoghue et al., 1998; Vucetich et al., 2002; Chan et al., 2017). Moreover, phenomenological models fail to identify the proximate mechanisms regulating predator acquisition rates. In this context, a mechanistically grounded approach to derive functional response is appealing.
Derivation of functional responses based on measurable features of species behavior (e.g., speed, attack and success probability) provides several advantages. Compared with phenomenological models, mechanistic models (1) allow assessing the shape of the functional response based on behavioral attributes of the predator, (2) are based on parameters with a direct biological interpretation, and hence have the potential to reinforce links between theory and data (Connolly et al., 2017). The number of mechanistic models of predator-prey interactions is growing, and most of them aim to predict trophic links based on species traits, especially body size (Gravel et al., 2013; Ho et al., 2019; Portalier et al., 2019). Mechanistic models of functional response further allow the integration of predator-prey pairs to describe trophic links, which can improve our ability to model complex ecological interactions. Despite their utility, mechanistic models based on the main components of predation in a natural system are, to our knowledge, virtually non-existent in vertebrate predators.
Using a combination of behavioral, demographic, and experimental data collected over 20 years in a natural system, we develop a mechanistic model to assess the functional response of a generalist mammalian predator to various prey species (4 predator-prey pairs). The originality of our approach is to assess functional response (i) by breaking down the components of predation (searching, chasing, capturing, and handling prey) and (ii) by using field experiments and detailed behavioral observations to parameterize each component included in the mechanistic model. We focused on the derivation of functional response of predator-prey pairs. We evaluated the coherence of our models using data from a long-term field study that estimated prey densities and predator acquisition rates. We also performed sensitivity analyses to identify the main proximate drivers of change in predator acquisition rates. Finally, we modeled the potential effects of density dependence in components of predation on the shape of the functional responses within the range of prey densities observed in the field.
The mechanistic model was developed for the arctic fox (Vulpes lagopus), a generalist predator of the tundra ecosystem, using highly detailed empirical observations from a long-term ecological monitoring program in the Arctic (Gauthier et al., 2013). This system offers several benefits to study predator-prey interactions among vertebrates, including a relatively simple food web, an open landscape and the continuous summer daylight allowing direct behavioral observations. The arctic fox is an active hunting predator that travels extensive daily distances within its territory in summer (Poulin et al., 2021). Lemmings and birds (mostly eggs and juveniles) are the main components of the summer diet of arctic foxes in most tundra ecosystems (Angerbjörn et al., 1999; Giroux et al., 2012). Lemmings exhibit population cycles with peak density every 3–5 years (Fauteux et al., 2015), and the arctic fox predation pressure on tundra ground-nesting birds is typically released at high lemming density (Summers et al., 1998; Bêty et al., 2002; McKinnon et al., 2014). Surprisingly, the exact mechanisms driving this well-known short-term apparent mutualism between lemmings and birds are still unclear, but they likely involve fox functional responses (Summers et al., 1998; Bêty et al., 2002).
A few studies attempted to quantify the functional responses of arctic fox using phenomenological models (Angerbjörn et al., 1999; Eide et al., 2005; Gilg et al., 2006). Relatively low sample sizes reduced the ability of previous studies to fully distinguish between different shapes of functional responses. Moreover, the hoarding behavior of arctic foxes was not considered in previous estimations of functional responses (Angerbjörn et al., 1999; Eide et al., 2005; Gilg et al., 2006). Like many other animals (Vander Wall, 1990), arctic foxes can predate more prey than they consume on the short-term, and such behavior can strongly increase prey acquisition rates, e.g., foxes foraging in goose colonies can hoard between 40 and 97% of eggs acquired during the bird nesting period (Samelius and Alisauskas, 2000; Careau et al., 2008). Although type III functional responses were previously used to model fox-prey population dynamics (Gilg et al., 2003, 2009), food hoarding may substantially reduce handling time and could therefore make the shape of the functional response linear or slightly convex (Oksanen et al., 1985).
During the summer, the southwest plain of Bylot Island, Nunavut, Canada (73° N; 80° W) harbors a large greater snow goose colony (Anser caerulescens atlanticus; ~20,000 pairs). Insectivorous migratory birds are also nesting in the study area and include the lapland longspur (Calcarius lapponicus), a passerine, and several species of shorebirds (primarily Calidris spp. and Pluvialis spp.). Two species of small mammals are present, the brown (Lemmus trimucronatus) and collared (Dicrostonyx groenlandicus) lemmings. The brown lemming has high-amplitude cycles of abundance with a 3–5-year periodicity, whereas the collared has low-amplitude cycles (Gruyer et al., 2008). The mammalian predator guild is dominated by the arctic fox and the ermine (Mustela erminea). The arctic fox is the main nest predator of geese (Bêty et al., 2002; Lecomte et al., 2008), sandpipers (McKinnon and Bêty, 2009; Royer-Boutin, 2015), and passerines (Royer-Boutin, 2015). Additional details on plant communities and general landscape can be found in Gauthier et al. (2013).
The model was parameterized and evaluated using data from Bylot Island, where foxes and their prey have been monitored since 1993. We observed foraging foxes using binoculars and spotting scopes (20 × 60x) from one or two blinds located in the middle of the goose colony during 10 summers between 1996 and 2019.
We used the Holling disk equation as a starting point to build the mechanistic model of functional response (Holling, 1959a) inspired by the general formalism of Pawar et al. (2012). Predation was broken down into four different components, which are searching, chasing, capturing, and handling of a prey item by a predator. Acquisition rate of a prey item (species i) by a predator [f(i)], namely the functional response, takes the following form:
where αi is the capture efficiency (km2/h), Ni the prey density (number of prey/km2), and hi the handling time of prey (h/prey). Capture efficiency is obtained by the product of predator speed (s; km/h), reaction distance (di; km), detection (zi), and attack probability (ki) of the prey by the predator, and the success probability (pi) of an attack (Table 1):
The combination of the time spent chasing the prey once encountered () and the time spent manipulating the prey once subdued (Tmi) define an overall prey handling time (hi):
The time spent manipulating includes the time spent eating or hoarding the prey item.
αi depends only on prey density, and we assumed that prey are randomly distributed. Satiety was not considered as a potential mechanism limiting acquisition rate. Indeed, foxes can predate more prey than they consume on the short-term; e.g., about 4% (n = 128) and 48% (n = 98) of predated eggs and lemmings are immediately eaten, respectively (Careau et al., 2007). Predator interference was not incorporated in the model as foxes rarely encounter and interact with other individuals while foraging within their summer territory (49 interactions, which represents 0.9% of the time over 118 h of direct observations of foxes foraging in the study area). The full model derivation is provided in Supplementary Material (section 1.1).
The general model of functional response (Equation 1) allows for a continuum between a linear (type 1) and a concave (type 2) functional response shape as a linear response can be elicited when handling time is negligible. In order to allow the mechanistic model to extend to a sigmoidal shape (type 3), we added density dependence in capture efficiency components that were expected to vary with prey density (i.e., reaction distance and detection and attack probabilities; see below).
We adapted the general model (Equation 1) to each prey species based on their traits and anti-predator behavior (Figure 1). The specific models for each prey species are provided in Supplementary Table S2.
Figure 1. Conceptual mechanistic model of functional response of arctic fox to each prey species: attended (A) or unattended (B) goose nests, lemmings (C), and passerine and sandpiper nests (D). Predation was divided into four main components: search, detect, attack, and handle (which includes eating and hoarding). Arrows illustrate the probability that the predator reaches the next component. When there is no parameter indicated beside the arrow, the probability is 1. Parameters are as follows: d is the reaction distance, s the predator speed, z the detection probability, k the attack probability, p the success probability, Pc the probability of complete nest predation, TC the time spent chasing, and Tm the time spent manipulating the prey.
For goose nests, the first modification was to add a component for complete and partial nest predation. This modification was necessary since a successful attack by the predator does not always result in complete clutch predation (Bêty et al., 2002), which can affect manipulation time and, ultimately, acquisition rates. The second modification was to split the general model into two components. A first component models acquisition rate of goose nests when the female is incubating or when one protecting adult is at <10 m from the nest (attended nest; Figure 1A). A second component models acquisition rate of goose nests during incubation recesses when both adults are at >10 m from the nest (unattended nest; Figure 1B). As geese can actively protect their nests against arctic foxes, their presence at the nest strongly influences fox foraging behavior (Samelius and Alisauskas, 2001; Bêty et al., 2002). This anti-predator behavior translates into changes in capture efficiency components. Thus, parameter values of capture rates were estimated separately for goose nests that were attended or unattended (Supplementary Table S1). When a nest is attended by a highly conspicuous snow goose, we assumed that nest detection probability is 1 within d (Figure 1A). For unattended nests, we used a detection probability function obtained from an artificial nests experiment (Supplementary Figure S1). Sometimes, unattended nests can be protected if parents detect a fox during an incubation recess and return quickly to their nest. Like attended nests, we thus estimated success probability (p) and complete clutch predation probability (Pc) for unattended nests (Figure 1B). The third and last modification was to introduce the nest attendance probability (w). We estimated this parameter by combining information on the average time spent on the nest by females and on the average distance between females and their nest during the goose incubation period (Reed et al. 1995; Poussart et al. 2000; see Supplementary Material, section 1.2).
The general model (Equation 1) was simplified for lemmings as we assumed that an attack is systematically initiated by the fox once a lemming is detected within d (Figure 1C). Sandpipers and passerines exhibit a variety of antipredator behaviors (such as distraction displays) to avoid nest detection by the predator (Smith and Edwards, 2018). However, sandpipers and passerines cannot protect their nest once detected by a fox. Thus, we assumed that once a nest is detected, it is always predated (attack probability is 1 and no chasing time is included in the model; Figure 1D).
We incorporated density dependence into the goose and the lemming models within the range of densities observed in our study system. For each parameter in which density dependence was incorporated, the minimum and the maximum parameter values were associated, respectively, with the minimum and the maximum prey density to calculate the slope and the intercept of the density-dependence relationship. In the goose model, we modified attack and success probabilities for attended nests, and reaction distance and detection probability for unattended nests. In the lemming model, we added density dependence in reaction distance, detection, and success probabilities. The rationale behind these additions is that predators may form search images for abundant prey, which can increase their ability to detect them (Nams, 1997; Ishii and Shimada, 2010). As predators could also increase their attack rate and success as prey density increases, we added density dependence in attack and success probabilities. We did not incorporate density dependence into the passerine and sandpiper nest models as the range of nest densities observed in our study system is likely too low to influence fox behavior (maximum of 12 nests/km2 compared to a maximum of 926 goose nests and 414 lemmings per km2). See Supplementary Material (section 1.3) for more details on the incorporation of density dependence.
The model was implemented in R v. 3.6.0 (R Core Team, 2019).
The model was parameterized mostly using data from Bylot Island but also from the literature when data were missing. Parameters were derived from field experiments using artificial nests or estimated using arctic fox GPS tracking data and direct observations of foraging foxes (Supplementary Table S1). See Supplementary Material (section 1.2) for a detailed description of the method used to extract each parameter.
Predator acquisition rates at different prey densities were assessed in the field annually using two independent methods. These data did not allow validation of the shape of the functional responses, but they provided a way to evaluate the performance of the mechanistic model in estimating prey acquisition rates at the various prey densities observed in our study system.
First, we obtained goose eggs and lemming acquisition rates by conducting direct observations of foraging foxes for 10 summers between 1996 and 2019 during the goose incubation period (details on behavioral observations can be found in Bêty et al., 2002; Careau et al., 2008). For each year, the acquisition rate was calculated as the total number of prey acquired (goose eggs or lemmings) divided by the total length of the observation bouts of individual foxes. The acquisition rate of a clutch of eggs was estimated by dividing the acquisition rate of goose eggs by the annual average clutch size. For the years where information was available, we also calculated the acquisition rate for attended and unattended nests. We estimated annual goose nest density either by visual counts of the nests located in the observation zone (range: 0.5–3 km2) during the incubation period (1996–1999, 2019) or over a fixed 0.2 km2 plot within the intensively monitored core area of the goose colony (2004–2005, 2015–2016). We estimated lemming density annually with snap traps from 1994 to 2009 and with live traps from 2004 to 2019 (see Fauteux et al., 2018 for methods). We summed the density estimate of brown and collared lemming.
Second, we obtained passerine and sandpiper nest acquisition rates by monitoring annually (2005–2013) the fate of passerine and sandpiper nests (Gauthier et al., 2013; McKinnon et al., 2014). Nest density was estimated as the number of passerine and sandpiper nests found in a 8 km2 plot systematically searched throughout the breeding season. We estimated acquisition rate of nest content (eggs or chicks) by using the daily survival rate of nests (dsr), the total number of nests found in the study plot (Ntot), the number of foxes foraging in the plot (Nfox), and the proportion of nests predated by foxes (Pfox). Since foxes establish territorial pairs on Bylot (Rioux et al., 2017), we assumed that 2 foxes were foraging in the study plot. We also considered that foxes were responsible for 100% (n = 19) and 81% (n = 25) of the failed sandpiper and passerine nests, respectively, as indicated by camera monitoring (McKinnon and Bêty, 2009; Royer-Boutin, 2015). An estimation of the acquisition rate is obtained by:
The daily nest survival rate was modeled using the logistic exposure method (Shaffer, 2004). Additional details on daily nest survival rate calculations and nest monitoring methods can be found in Royer-Boutin (2015). Density estimates for all prey species were standardized as the number of nests per km2.
We quantified how uncertainty in parameter values affected estimation of predator acquisition rates by using the Latin hypercube sampling technique (an efficient implementation of the Monte Carlo methods; Marino et al., 2008). This analysis allowed us to investigate the uncertainty in the model output generated by the uncertainty and variability in parameter inputs. Each parameter was represented by a probability distribution (uniform or normal truncated) based on the distribution of empirical data (Supplementary Table S1). For some parameters, the biological information was limited, so we assigned a uniform distribution allowing for a large range bounded by minimum and maximum values. Latin hypercube sampling was then applied to each distribution (N = 1,000 iterations). This method involved dividing a probability distribution into N equal probability intervals that were then sampled without replacement, resulting in N iterations of the model using each combination of parameters values. This method allowed us to explore the entire range of each parameter and most of them encompass various environmental conditions (e.g., weather conditions, prey availability). We computed the median, the 90th, 95th, and 99th percentiles of the model output by using the empirical cumulative distribution.
We also conducted a local sensitivity analysis to identify key parameters of the mechanistic models within the range of prey densities observed in our study system. We modified each parameter value by ±100% while holding others constant, and we assessed how this variation affected the predator acquisition rate (expressed as % of change).
From 1996 to 2019, we observed foraging foxes in the goose colony for 124 h. Average goose nest density was 409 nests/km2 (range: 100–926 nests/km2) and lemming density was 193 ind./km2 (range: 11–414 ind./km2; Supplementary Table S3). Based on direct observations of foraging foxes, average acquisition rates were 0.61 nest/fox/h (range: 0.19–1.82 nest/fox/h) for goose nests and 0.94 ind./fox/h (range: 0–2.85 ind./fox/h) for lemmings (Supplementary Table S3). The majority of eggs acquired (67%) were from unattended goose nests, while 33% were from attended nests (n = 218). Average passerine nest density was 7.7 nests/km2 (range: 6.1–12.3 nests/km2), and sandpiper density was 2.5 nests/km2 (range: 1.0–5.9 nests/km2; Supplementary Table S4). Based on nest monitoring, average acquisition rates were 0.10 nest/fox/h (range: 0.03–0.28 nest/fox/h) and 0.04 nest/fox/h (range: 0.002–0.169 nest/fox/h) for passerine and sandpiper nests, respectively (Supplementary Table S4).
The uncertainty analysis revealed that varying simultaneously all parameters used in the mechanistic model generated considerable variation in fox acquisition rates (Figure 2). Nonetheless, no parameter combinations resulted in a saturating functional response for all prey species within the range of prey densities observed in our study system: the acquisition rate at maximal prey density was below the saturation point in all simulations (see histograms in Figure 2, Supplementary Figure S5). Based on the value of the parameters estimated within the observed prey densities, acquisition rate at saturation was 8 nests/fox/h for goose nests, 17 ind./fox/h for lemmings, 166 nests/fox/h for passerine, and 26 nests/fox/h sandpiper nests. Depending on the prey species, most or all fox acquisition rates observed in the field fell within the 99th percentile of the values derived from the mechanistic models (Figure 2). The highest acquisition rates observed in the field were also much below the estimated saturation point in all prey species (Figure 2). As the goose nest model was split for attended and unattended goose nests, we also computed acquisition rates separately for each of these situations. Goose nest acquisition rate derived from the mechanistic model was higher for unattended nests than attended nests, which is consistent with empirical estimations (Supplementary Figure S6). Although most (66%) field estimates of acquisition rates fell within the 95th percentiles of the model output for unattented goose nests, all values were under the model median at nest densities above 200 nests/km2 (Supplementary Figure S6). This may indicate a slight overestimation of the proportion of unattended nests at relatively high densities.
Figure 2. Functional response of arctic fox to density of goose nests (A), lemmings (B), passerine nests (C), and sandpiper nests (D). Black lines represent the median of the mechanistic model and the color bands represent the 90th, 95th, and 99th percentiles based on 1,000 simulations. Empirical data are represented by red and yellow dots respectively. Histograms in the inset show the distributions of acquisition rate at saturation for each simulation. Horizontal error bars in (A) indicate the range of nest density during the incubation period. Vertical errors bars in (A,B) represent standard errors calculated using bootstrapping. Errors bars in (C,D) represent 95% confidence intervals from daily survival rate estimates.
Sensitivity analyses indicated that predator speed was an influential parameter of the functional response of all prey species (Figure 3). Goose nest acquisition rate was generally more affected by parameters associated with unattended nests than attended nests (Figure 3A). The magnitude of change in goose nest acquisition rate related to the changes in manipulation time increased slightly with nest density. Lemming acquisition rate was not affected by chasing and manipulation time, whereas detection distance, and detection and success probability had an influence equivalent to predator speed (Figure 3B). Similarly, functional response models of passerine and sandpiper nests were not sensitive to change in manipulation time, whereas detection distance and detection probability had an influence equivalent to predator speed (Figure 3C).
Figure 3. Sensitivity of predator acquisition rates to changes in parameter values of the mechanistic models used to assess the functional response of arctic fox to goose nests [at 100 (A1), and 1,000 nests/km2 (A2)], to lemmings at 250 ind./km2 (B) and to passerine and sandpiper nests at 10 nests/km2 (C). Sensitivity analyses are presented at intermediate densities for lemmings and passerine and sandpiper nests, since the results were very similar within the range of densities observed in our study system.
Adding density dependence into the goose and the lemming models had relatively minor effects on acquisition rates derived for low to moderate densities observed in our study system. The shape of the functional response changed slightly between models without or with density dependence in capture efficiency components (allowing for a gradient between type I and type III). At high densities, acquisition rates remained much below saturation points, and a maximum difference of 1.4 nests/fox/h at 1,000 goose nests/km2 and 2.1 lemmings/fox/h at 450 lemmings/km2 were found between models (Figure 4).
Figure 4. Functional response models of arctic fox to goose nests (A) and lemmings (B) with and without density dependence on capture efficiency components, within the range of densities observed in the field. Black lines represent the median of the mechanistic model and the color bands represent the 95th percentiles based on 1,000 simulations.
Benefiting from a combination of behavioral, demographic, and experimental data collected over the past 20 years, we developed a mechanistic model of arctic fox functional response to four prey species. Our model derives the shape of the functional response of each predator-prey pair along a gradient from linear to sigmoidal. Predator acquisition rates derived from the mechanistic model were consistent with field observations, and the main proximate mechanisms driving predator acquisition rates were also identified. In all prey species, predator speed was an influential parameter, while handling time had a limited influence on acquisition rates. Although type III functional responses were previously used to model fox-prey population dynamics (Gilg et al., 2003, 2009), our simulations indicate that predator acquisition rate was not systematically limited at the highest prey densities observed in our study system. Our model allows for a mechanistic interpretation of the functional response of predator-prey pair and could be extended to more complex modules involving multiple predators and prey species.
Our results add to a growing body of research indicating that predators may not become systematically satiated or saturated at the highest densities of prey observed in nature (Novak, 2010; Chan et al., 2017; Preston et al., 2018). Holling's functional response models (type II and III), which are commonly used in population dynamics models (Turchin and Hanski, 1997; Gervasi et al., 2012; Serrouya et al., 2015), predict that predator acquisition rates should eventually saturate at high prey densities. Based on mechanistic models, which allowed us to vary simultaneously all components of predation, we found no evidence of arctic fox saturation at the highest prey densities observed in a natural system. Several factors may explain this result. First, the hoarding behavior of arctic foxes may substantially reduce handling time by limiting the constraints associated with digestion and satiety, which can make the functional response shape linear or slightly convex even at high prey densities (Oksanen et al., 1985). Second, while predator acquisition rates must theoretically become constrained by handling and/or digestion at high prey densities, the prey densities required to reach a saturation point could be rarely observed in natural systems. Indeed, empirical support for saturating functional response in the wild is relatively rare and comes mostly from controlled laboratory experiments in which the range of prey densities may exceed the range observed in nature [99% of all type II functional response were derived from controlled laboratory experiments (n = 61 studies); reviewed by Rall et al., 2012]. Such an issue can be avoided when mechanistic approaches are used to derive functional responses. One particularity of our system is the presence of a large goose colony where prey density can be quite high (up to ~900 nests/km2). Interestingly, even in this context, we found no evidence of predator saturation.
Historically, a categorical approach was adopted by ecologists to define functional responses. A linear functional response was traditionally attributed to filter feeders (Jeschke et al., 2004), a hyperbolic shape (type II) to invertebrates and a sigmoidal shape (type III) to vertebrate predators (Holling 1965, but see Hassell et al. 1977). Although, this categorization has some heuristic value in introductory texts and can be useful in some aspects of research where categorization is necessary, types I, II, and III should be considered simply as particular cases along a continuum. Instead of using a priori shapes to describe functional responses, our study illustrates how mechanistic models can generate functions linking prey density and predator acquisition rates that are specific, and hence more relevant, to the range of densities observed in a food web. Considering the strong effect of functional responses on the outcome of predator-prey models (Abrams et al., 1998; Sinclair et al., 1998), such specific functions should improve our ability to adequately simulate and quantify the strength of species interactions in natural communities.
We did not incorporate predator dependence in the functional response model, despite a growing body of studies indicating that some mechanisms (e.g., facilitation, interference) are likely to occur in functional responses (Novak et al., 2017). However, arctic foxes maintained summer territories (averaging 9.6 km2) with low overlap (Grenier-Potvin and Berteaux, submitted manuscript), which prevents potential interference within territories. We are thus confident that variation in predator density should not affect our main conclusions. Nonetheless, the mechanistic model could be extended to more complex predator-prey systems, including predator interference.
Habitat characteristics could affect several parameters of the mechanistic model, hence the functional response shape and magnitude could be modulated by the structural complexity of the landscape (Toscano and Griffen, 2013; Barrios-O'Neill et al., 2015). For instance, the detection distance of a nest by arctic foxes could be lower in dense vegetation (Flemming et al., 2016), the attack probability could be lower for nests located in wetlands and islets only accessible by swimming (Lecomte et al., 2008; Gauthier et al., 2015), and the success probability of an attack could be modulated by the presence of complex networks of lemming tunnels offering refuges. Exploration of the effects of structural complexity on functional responses remains rare (but see Lipcius and Hines 1986; Toscano and Griffen 2013; Barrios-O'Neill et al. 2015), and more empirical research is needed to integrate these sources of variation in mechanistic models.
The outputs of the mechanistic model were generally consistent with field observations. However, adding more complexity could improve its performance and our ability to identify the main drivers of predator acquisition rates. For instance, group defense and mutual vigilance are additional factors that may reduce predator acquisition rates at high prey density (Clark and Robertson, 1979). Although there is no evidence of group defense in geese (Bêty et al., 2001), the snow goose could benefit from the vigilance and early warning provided by neighbors nesting nearby (Samelius and Alisauskas, 2001). Beyond a threshold of goose nest density, such anti-predator behavior could reduce the proportion of unattended nests with increasing nest densities. Nest attendance probability was an influential parameter of the goose model and mutual vigilance may partly explain why acquisition rates observed in the field at moderate-high nest densities were under the model median (Figure 2A).
One mechanism often advanced for explaining the apparent mutualism between two prey sharing a common predator is predator saturation or satiation (Holt, 1977; Abrams and Matsuda, 1996). Our results showed that the arctic fox doesn't reach saturation at the highest lemming densities observed in our study system. This suggests that the underlying mechanism for the short-term positive effect of high lemming density on arctic bird reproductive success (Bêty et al., 2002; Blomqvist et al., 2002) is likely not predator satiation nor saturation. Instead, the apparent mutualism between birds and lemmings could arise from changes in other components of the functional response. For instance, the attack probability of an attended goose nest could be inversely dependent of lemming density, or daily distance traveled by the predator (speed) could be dependent of lemming density. As indicated by our sensitivity analyses, attack probability was not a strong driver of prey acquisition rates while predator speed was an influential parameter in all prey species. Hence, lemming-induced changes in predator speed through changes in predator activity budget (e.g., due to predator reproductive status or hunger level) could be an alternative hypothesis explaining the apparent mutualism between lemmings and arctic birds. Density dependent changes in components of the functional response have been observed in other systems and can generate nonlinearities in the functional response (Hassell et al., 1977; Abrams, 1982). The integration of all prey species into a mechanistic multi-species functional response model is the next step to fully identify the main proximate drivers of indirect interactions in natural communities.
Previous studies of functional responses typically tried to discriminate between predetermined shapes of functional responses. Our study illustrates how mechanistic models based on empirical estimates of the main components of predation can generate functional responses specific to a range of prey densities relevant to a given food web. Such mechanistically derived functional responses are needed to untangle proximate drivers of predator-prey population dynamics and to improve our understanding of predator-mediated interactions in natural communities. Although it would be unrealistic to resolve every pairwise interaction within ecological networks, our mechanistic model provides a starting point for studying higher-order effects such as indirect interactions that can emerge among prey species.
The original contributions presented in the study are included in the article/Supplementary Material, further inquiries can be directed to the corresponding author/s.
The animal study was reviewed and approved by Capture techniques and immobilization procedures were approved by the UQAR Animal Care Committee (CPA-64-16-169 R2).
AB, DG, and JB conceived the study. AB, DG, DB, GG, JC, VC, NL, C-CJ, PR-B, and JB collected the data. AB, PR-B, and JC analyzed the data. AB wrote the manuscript with contribution from all authors. All authors contributed to the article and approved the submitted version.
The research relied on the logistic assistance of the Polar Continental Shelf Program (Natural Resources Canada) and of Sirmilik National Park of Canada. The research was funded by (alphabetical order): Arctic Goose Joint Venture, the Canada Foundation for Innovation, the Canada Research Chairs Program, the Canadian Wildlife Service, the Fonds de recherche du Québec-Nature et technologies, the International Polar Year program of Indian and Northern Affairs Canada, the Natural Sciences and Engineering Research Council of Canada, the ArcticNet Network of Centers of Excellence, the Northern Ecosystem Initiative Program (Environment Canada), the Northern Scientific Training Program, the Nunavut Wildlife Management Board, Polar Knowledge Canada, Université du Québec à Rimouski, Université Laval and the W. Garfield Weston Foundation.
The authors declare that the research was conducted in the absence of any commercial or financial relationships that could be construed as a potential conflict of interest.
We are especially grateful to the many people who helped us with field work over many years, the Mittimatalik Hunters and Trappers Organization and Park Canada's staff for their assistance. We sincerely thank both reviewers for their constructive and relevant comments. The article was published on BioRxiv (Beardsell et al., 2020).
The Supplementary Material for this article can be found online at: https://www.frontiersin.org/articles/10.3389/fevo.2021.630944/full#supplementary-material
Abrams, P. A. (1982). Functional responses of optimal foragers. Am. Nat. 120, 382–390. doi: 10.1086/283996
Abrams, P. A., Holt, R. D., and Roth, J. D. (1998). Apparent competition or apparent mutualism? Shared predation when population cycle. Ecology 79, 201–212. doi: 10.1890/0012-9658(1998)079[0201:ACOAMS]2.0.CO;2
Abrams, P. A., and Matsuda, H. (1996). Positive indirect effects between prey species that share predators. Ecology 77, 610–616. doi: 10.2307/2265634
Angerbjörn, A., Tannerfeldt, M., and Erlinge, S. (1999). Predator-prey relationships: arctic foxes and lemmings. J. Anim. Ecol. 68, 34–49. doi: 10.1046/j.1365-2656.1999.00258.x
Bêty, J., Gauthier, G., Giroux, J.-F., and Korpimäki, E. (2001). Are goose nesting success and lemming cycles linked? Interplay between nest density and predators. Oikos 93, 388–400. doi: 10.1034/j.1600-0706.2001.930304.x
Bêty, J., Gauthier, G., Korpimäki, E., and Giroux, J.-F. (2002). Shared predators and indirect trophic interactions: lemming cycles and arctic-nesting geese. J. Anim. Ecol. 71, 88–98. doi: 10.1046/j.0021-8790.2001.00581.x
Barrios-O'Neill, D., Dick, J. T., Emmerson, M. C., Ricciardi, A., and MacIsaac, H. J. (2015). Predator-free space, functional responses and biological invasions. Funct. Ecol. 29, 377–384. doi: 10.1111/1365-2435.12347
Beardsell, A., Gravel, D., Berteaux, D., Gauthier, G., Clermont, J., Careau, V., et al. (2020). Derivation of predator functional responses using a mechanistic approach in a natural system. bioRxiv 47. doi: 10.1101/2020.11.04.368670
Blomqvist, S., Holmgren, N., Akesson, S., Hedenström, A., and Pettersson, J. (2002). Indirect effects of lemming cycles on sandpiper dynamics: 50 years of counts from southern Sweden. Oecologia 133, 146–158. doi: 10.1007/s00442-002-1017-2
Careau, V., Giroux, J.-F., and Berteaux, D. (2007). Cache and carry: hoarding behavior of arctic fox. Behav. Ecol. Sociobiol. 62, 87–96. doi: 10.1007/s00265-007-0441-z
Careau, V., Lecomte, N., Bêty, J., Giroux, J.-F., Gauthier, G., and Berteaux, D. (2008). Hoarding of pulsed resources: temporal variations in egg-caching by arctic fox. Ecoscience 15, 268–276. doi: 10.2980/15-2-3097
Chan, K., Boutin, S., Hossie, T. J., Krebs, C. J., O'Donoghue, M., and Murray, D. L. (2017). Improving the assessment of predator functional responses by considering alternate prey and predator interactions. Ecology 98, 1787–1796. doi: 10.1002/ecy.1828
Clark, K. L., and Robertson, R. J. (1979). Spatial and temporal multi-species nesting aggregations in birds as anti-parasite and anti-predator defenses. Behav. Ecol. Sociobiol. 5, 359–371. doi: 10.1007/BF00292524
Connolly, S. R., Keith, S. A., Colwell, R. K., and Rahbek, C. (2017). Process, mechanism, and modeling in macroecology. Trends Ecol. Evol. 32, 835–844. doi: 10.1016/j.tree.2017.08.011
Eide, N. E., Eid, P. M., Prestrud, P., and Swenson, J. E. (2005). Dietary responses of arctic foxes Alopex lagopus to changing prey availability across an Arctic landscape. Wildl. Biol. 11, 109–121. doi: 10.2981/0909-6396(2005)11[109:DROAFA]2.0.CO;2
Ellis, K., Larsen, R. T., and Koons, D. N. (2019). The importance of functional responses among competing predators for avian nesting success. Funct. Ecol. 34, 252–264. doi: 10.1111/1365-2435.13460
Fauteux, D., Gauthier, G., and Berteaux, D. (2015). Seasonal demography of a cyclic lemming population in the Canadian Arctic. J. Anim. Ecol. 84, 1412–1422. doi: 10.1111/1365-2656.12385
Fauteux, D., Gauthier, G., Mazerolle, M. J., Coallier, N., Bêty, J., and Berteaux, D. (2018). Evaluation of invasive and non-invasive methods to monitor rodent abundance in the Arctic. Ecosphere 9, 1–18. doi: 10.1002/ecs2.2124
Flemming, S. A., Calvert, A., Nol, E., and Smith, P. A. (2016). Do hyperabundant Arctic-nesting geese pose a problem for sympatric species? Environm. Rev. 10, 1–10. doi: 10.1139/er-2016-0007
Fryxell, J. M., Mosser, A., Sinclair, A. R. E., and Packer, C. (2007). Group formation stabilizes predator? prey dynamics. Nature 449, 1041–1043. doi: 10.1038/nature06177
Gauthier, G., Bêty, J., Cadieux, M.-C., Legagneux, P., Doiron, M., Chevallier, C., et al. (2013). Long-term monitoring at multiple trophic levels suggests heterogeneity in responses to climate change in the Canadian Arctic tundra. Philos. Trans. R. Soc. Lond. B Biol. Sci. 368:1624. doi: 10.1098/rstb.2012.0482
Gauthier, G., Legagneux, P., Valiquette, M.-A., Cadieux, M.-C., and Therrien, J.-F. (2015). Diet and reproductive success of an Arctic generalist predator: Interplay between variations in prey abundance, nest site location, and intraguild predation. Auk 132, 735–747. doi: 10.1642/AUK-14-273.1
Gervasi, V., Nilsen, E. B., Sand, H., Panzacchi, M., Rauset, G. R., Pedersen, H. C., et al. (2012). Predicting the potential demographic impact of predators on their prey: a comparative analysis of two carnivore?ungulate systems in Scandinavia. J. Anim. Ecol. 81, 443–454. doi: 10.1111/j.1365-2656.2011.01928.x
Gilg, O., Hanski, I., and Sittler, B. (2003). Cyclic dynamics in a simple vertebrate predator-prey community. Science 302, 866–868. doi: 10.1126/science.1087509
Gilg, O., Sittler, B., and Hanski, I. (2009). Climate change and cyclic predator-prey population dynamics in the high Arctic. Glob. Change Biol. 15, 2634–2652. doi: 10.1111/j.1365-2486.2009.01927.x
Gilg, O., Sittler, B., Sabard, B., Hurstel, A., Sané, R., Delattre, P., and Hanski, I. (2006). Functional and numerical responses of four lemming predators in high arctic Greenland. Oikos 113, 193–216. doi: 10.1111/j.2006.0030-1299.14125.x
Giroux, M.-A., Berteaux, D., Lecomte, N., Gauthier, G., Szor, G., and Bêty, J. (2012). Benefiting from a migratory prey: spatio-temporal patterns in allochthonous subsidization of an arctic predator. J. Anim. Ecol. 81, 533–542. doi: 10.1111/j.1365-2656.2011.01944.x
Gravel, D., Poisot, T., Albouy, C., Velez, L., and Mouillot, D. (2013). Inferring food web structure from predator-prey body size relationships. Methods Ecol. Evol. 4, 1083–1090. doi: 10.1111/2041-210X.12103
Gruyer, N., Gauthier, G., and Berteaux, D. (2008). Cyclic dynamics of sympatric lemming populations on Bylot Island, Nunavut, Canada. Can. J. Zool. 86, 910–917. doi: 10.1139/Z08-059
Hassell, M. P., Lawton, J. H., and Beddington, J. R. (1977). Sigmoid functional responses by invertebrate predators and parasitoids. J. Anim. Ecol. 46, 249–262. doi: 10.2307/3959
Ho, H., Tylianakis, J. M., Zheng, J. X., and Pawar, S. (2019). Predation risk influences food? web structure by constraining species diet choice. Ecol. Lett. 22, 1734–1745. doi: 10.1111/ele.13334
Holling, C. S. (1959a). Some characteristics of simple types of predation and parasitism. Can. Entomol. 385–398. doi: 10.4039/Ent91385-7
Holling, C. S. (1959b). The components of predation as revealed by a study of small-mammal predation of the European pine sawfly. Can. Entomol. 91, 293–320. doi: 10.4039/Ent91293-5
Holling, C. S. (1965). The functional response of predators to prey density and its role in mimicry and population regulation. Memoirs Entomol. Soc. Can. 97, 5–60. doi: 10.4039/entm9745fv
Holt, R. D. (1977). Predation, apparent competition, and the structure of prey communities. Theor. Popul. Biol. 12, 197–229. doi: 10.1016/0040-5809(77)90042-9
Holt, R. D., and Bonsall, M. (2017). Apparent competition. Annu. Rev. Ecol. Evol. Syst. 48, 447–471. doi: 10.1146/annurev-ecolsys-110316-022628
Ishii, Y., and Shimada, M. (2010). The effect of learning and search images on predator-prey interactions. Popul. Ecol. 52, 27–35. doi: 10.1007/s10144-009-0185-x
Jeschke, J. M., Kopp, M., and Tollrian, R. (2004). Consumer-food systems: why type I functional responses are exclusive to filter feeders. Biol. Rev. Camb. Philos. Soc. 79, 337–349. doi: 10.1017/S1464793103006286
Lecomte, N., Careau, V., Gauthier, G., and Giroux, J. F. (2008). Predator behaviour and predation risk in the heterogeneous Arctic environment. J. Anim. Ecol. 77, 439–447. doi: 10.1111/j.1365-2656.2008.01354.x
Lipcius, R. N., and Hines, A. H. (1986). Variable functional responses of a marine predator in dissimilar homogeneous microhabitats. Ecology 67, 1361–1371. doi: 10.2307/1938692
Marino, S., Hogue, I. B., Ray, C. J., and Kirschner, D. E. (2008). A methodology for performing global uncertainty and sensitivity analysis in systems biology. J. Theor. Biol. 254, 178–196. doi: 10.1016/j.jtbi.2008.04.011
McKinnon, L., and Bêty, J. (2009). Effect of camera monitoring on survival rates of High-Arctic shorebird nests. J. Field Ornithol. 80, 280–288. doi: 10.1111/j.1557-9263.2009.00231.x
McKinnon, L., Berteaux, D., and Bêty, J. (2014). Predator-mediated interactions between lemmings and shorebirds: a test of the alternative prey hypothesis. Auk 131, 619–628. doi: 10.1642/AUK-13-154.1
Nams, V. O. (1997). Density-dependent predation by skunks using olfactory search images. Oecologia 110, 440–448. doi: 10.1007/s004420050179
Novak, M. (2010). Estimating interaction strengths in nature: experimental support for an observational approach. Ecology 91, 2394–2405. doi: 10.1890/09-0275.1
Novak, M., Wolf, C., Coblentz, K. E., and Shepard, I. D. (2017). Quantifying predator dependence in the functional response of generalist predators. Ecol. Lett. 20, 761–769. doi: 10.1111/ele.12777
O'Donoghue, M., Boutin, S., Krebs, C. J., Zuleta, G., Murray, D. L., and Hofer, E. J. (1998). Functional responses of coyotes and lynx to the snowshoe hare cycle. Ecology 79, 1193–1208. doi: 10.2307/176736
Oksanen, T., Oksanen, L., and Fretwell, P. T. (1985). Surplus killing in the hunting strategy of small predators. Am. Nat. 126, 328–346. doi: 10.1086/284420
Pawar, S., Dell, A. I., and Van M. Savage (2012). Dimensionality of consumer search space drives trophic interaction strengths. Nature 486, 485–489. doi: 10.1038/nature11131
Portalier, S. M., Fussmann, G. F., Loreau, M., and Cherif, M. (2019). The mechanics of predator? prey interactions: first principles of physics predict predator?prey size ratios. Funct. Ecol. 33, 323–334. doi: 10.1111/1365-2435.13254
Poulin, M.-P., Clermont, J., and Berteaux, D. (2021). Extensive daily movement rates measured in territorial arctic foxes. Ecol. Evol 1–12. doi: 10.1002/ece3.7165
Poussart, C., Larochelle, J., and Gauthier, G. (2000). The thermal regime of eggs during laying and incubation in greater snow geese. Condor 102, 292–300. doi: 10.1093/condor/102.2.292
Preston, D. L., Henderson, J. S., Falke, L. P., Segui, L. M., Layden, T. J., and Novak, M. (2018). What drives interaction strengths in complex food webs? A test with feeding rates of a generalist stream predator. Ecology 99, 1591–1601. doi: 10.1002/ecy.2387
R Core Team (2019). R: A Language and Environment for Statistical Computing. Vienna: R Foundation for Statistical Computing.
Rall, B. C., Brose, U., Hartvig, M., Kalinkat, G., Schwarzmüller, F., Vucic-Pestic, O., et al. (2012). Universal temperature and body-mass scaling of feeding rates. Philos. Trans. R. Soc. B Biol. Sci. 367, 2923–2934. doi: 10.1098/rstb.2012.0242
Reed, A., Hughes, R. J., and Gauthier, G. (1995). Incubation behavior and body mass of female greater snow geese. Condor 97, 993–1001. doi: 10.2307/1369538
Rioux, M. J., Lai, S., Casajus, N., Bêty, J., and Berteaux, D. (2017). Winter home range fidelity and extraterritorial movements of Arctic fox pairs in the Canadian High Arctic. Polar Res. 1:10. doi: 10.1080/17518369.2017.1316930
Royer-Boutin, P. (2015). Effets des Cycles de Lemmings sur le Succés de Nidification d'oiseaux Différant par Leur Taille Corporelle et Leur Comportement. M.SC., Université du Québecá Rimouski.
Samelius, G., and Alisauskas, R. T. (2000). Foraging patterns of arctic foxes at a large arctic goose colony. Arctic 53, 279–288. doi: 10.14430/arctic858
Samelius, G., and Alisauskas, R. T. (2001). Deterring arctic fox predation: the role of parental nest attendance by lesser snow geese. Can. J. Zool. 79, 861–866. doi: 10.1139/z01-048
Serrouya, R., Wittmann, M. J., McLellan, B. N., Wittmer, H. U., and Boutin, S. (2015). Using predator-prey theory to predict outcomes of broadscale experiments to reduce apparent competition. Am. Nat. 185, 665–679. doi: 10.1086/680510
Shaffer, T. L. (2004). A unified approach to analyzing nest success. Auk 121, 526–540. doi: 10.1642/0004-8038(2004)121[0526:AUATAN]2.0.CO;2
Sinclair, A. R. E., Pech, R. P., Dickman, C. R., Hik, D., Mahon, P., and Newsome, A. E. (1998). Predicting effects of predation on conservation of endangered prey. Conserv. Biol. 12, 564–575. doi: 10.1046/j.1523-1739.1998.97030.x
Smith, P. A. and Edwards, D. B. (2018). Deceptive nest defence in ground-nesting birds and the risk of intermediate strategies. PLoS ONE 13:e205236. doi: 10.1371/journal.pone.0205236
Solomon, M. (1949). The natural control of animal populations. J. Anim. Ecol. 18, 1–35. doi: 10.2307/1578
Summers, R. W., Underhill, L. G., and Syroechkovski, E. E. Jr. (1998). The breeding productivity of dark-bellied brent geese and curlew sandpipers in relation to changes in the numbers of arctic foxes and lemmings on the Taimyr Peninsula, Siberia. Ecography 21, 573–580. doi: 10.1111/j.1600-0587.1998.tb00549.x
Suryawanshi, K. R., Redpath, S. M., Bhatnagar, Y. V., Ramakrishnan, U., Chaturvedi, V., Smout, S. C., et al. (2017). Impact of wild prey availability on livestock predation by snow leopards. R. Soc. Open Sci. 4:1–11. doi: 10.1098/rsos.170026
Therrien, J.-F., Gauthier, G., Korpimäki, E., and Bêty, J. (2014). Predation pressure by avian predators suggests summer limitation of small-mammal populations in the Canadian Arctic. Ecology 95, 56–67. doi: 10.1890/13-0458.1
Toscano, B. J., and Griffen, B. (2013). Predator size interacts with habitat structure to determine the allometric scaling of the functional response. Oikos 122, 454–462. doi: 10.1111/j.1600-0706.2012.20690.x
Turchin, P., and Hanski, I. (1997). An empirically based model for latitudinal gradient in vole population dynamics. Am. Nat. 149, 842–874. doi: 10.1086/286027
Keywords: functional response, predation, trophic interactions, tundra, predator-prey interactions, arctic fox (Vulpes lagopus), arctic
Citation: Beardsell A, Gravel D, Berteaux D, Gauthier G, Clermont J, Careau V, Lecomte N, Juhasz C-C, Royer-Boutin P and Bêty J (2021) Derivation of Predator Functional Responses Using a Mechanistic Approach in a Natural System. Front. Ecol. Evol. 9:630944. doi: 10.3389/fevo.2021.630944
Received: 18 November 2020; Accepted: 11 February 2021;
Published: 08 March 2021.
Edited by:
Dennis Murray, Trent University, CanadaCopyright © 2021 Beardsell, Gravel, Berteaux, Gauthier, Clermont, Careau, Lecomte, Juhasz, Royer-Boutin and Bêty. This is an open-access article distributed under the terms of the Creative Commons Attribution License (CC BY). The use, distribution or reproduction in other forums is permitted, provided the original author(s) and the copyright owner(s) are credited and that the original publication in this journal is cited, in accordance with accepted academic practice. No use, distribution or reproduction is permitted which does not comply with these terms.
*Correspondence: Andréanne Beardsell, YWJlYXJkc2VsbEBob3RtYWlsLmNvbQ==; Joël Bêty, am9lbF9iZXR5QHVxYXIuY2E=
Disclaimer: All claims expressed in this article are solely those of the authors and do not necessarily represent those of their affiliated organizations, or those of the publisher, the editors and the reviewers. Any product that may be evaluated in this article or claim that may be made by its manufacturer is not guaranteed or endorsed by the publisher.
Research integrity at Frontiers
Learn more about the work of our research integrity team to safeguard the quality of each article we publish.