- 1Laboratório de Ciências Ambientais (LCA), Programa de Pós-Graduação em Ecologia e Recursos Naturais, Centro de Biociências e Biotecnologia, Universidade Estadual do Norte Fluminense, Campos dos Goytacazes, Brazil
- 2Programa de Pós-Graduação em Modelagem em Ciências da Terra e do Ambiente, Departamento de Ciências Biológicas, Universidade Estadual de Feira de Santana, Feira de Santana, Brazil
- 3Laboratório de Ecologia Espacial e Conservação (LEEC), Departamento de Biodiversidade, Instituto de Biociências, Universidade Estadual Paulista, Rio Claro, Brazil
Human activities have modified the landscape composition. The changes in the landscape structure can be evaluated by metrics, which are influenced, among other factors, by the number of cover classes used for the landscape classification (thematic resolution). In high thematic resolutions, landscape covers that can influence biological responses are identified and detailed. In low thematic resolutions, this detail level is lower because it aggregates different landscape covers in a few classes. However, how the thematic resolution influences our ability to understand landscape structure on biodiversity is poorly explored, particularly for pollinators. Here we asked how thematic resolution affects the explanatory power of landscape composition on explaining Euglossini bees (richness and abundance) within 15 landscapes composed mainly of coffee and pasture. To address this issue, we quantified the association between five attributes of the euglossine bee community and landscape composition: landscape cover classes (%) and landscape heterogeneity. Moreover, we also evaluated how the thematic resolution influences bee responses to landscape structure. We found a strong and positive influence of landscape heterogeneity in low thematic resolutions (i.e., few cover classes on maps) over the richness and rare species abundance. We also observed that- in addition to the forest cover in the landscape- the pasture cover (%) quantified in high thematic resolution positively influenced the total abundance and abundance of common and intermediate species. Our study highlights the importance of maintaining compositional heterogeneity for the orchid bee community in agroecosystems, and forest cover for the biological requirements and conservation of these pollinators. Moreover, the use of different thematic resolutions showed how specific types of landscape covers influence the euglossine community attributes. This can highlight the species preferences for habitats and landscape covers. Thus, we call the attention of landscape ecologists to the importance of the definition of thematic resolution, as our ability to quantify the association between biological responses and landscape structure may be influenced by the number of classes used when building thematic maps.
Introduction
The changes in land use may lead to habitat loss and fragmentation. These processes are the main threat to biodiversity, ecosystem functions, and several ecosystem services in the world (Fahrig, 2003; Haddad et al., 2015; Duarte et al., 2018). Human activities, as urbanization and agriculture, have modified the landscape structure and habitat quality across space and time (Fischer and Lindenmayer, 2007; Haddad et al., 2015). These changes reduce patch size and proximity, scattering habitats into anthropogenic matrices (Neel et al., 2004; Haddad et al., 2015). Moreover, forest fragmentation as a process (sensu Fahrig, 2003) has consequences related to the increase of the edge effect, with changes in microclimate conditions, thus influencing habitat quality and species persistence at the landscape level (Bender and Fahrig, 2005; Prevedello and Vieira, 2010; Martello et al., 2016).
The landscape changes influence the landscape composition (i.e., number and area of the patches). The use of landscape metrics is crucial for quantifying these elements and inferring the functional landscape heterogeneity, i.e., the diversity of landscape cover based on the functionality of each cover type for a given biological group (Fahrig et al., 2011). However, landscape metrics estimates are influenced by grain size and thematic resolution (Castilla et al., 2009; Liang et al., 2013). Grain size refers to the level of spatial resolution used to describe a data set (Allen and Hoekstra, 1991; Turner and Gardner, 2015), while thematic resolution, the central subject of our study, refers to the number of cover classes used in mapping for the landscape classification (Lechner and Rhodes, 2016).
The choice of thematic resolution in a study is based on the species requirements (e.g., available habitats, nesting) and on which patches might contain these requirements (Fahrig et al., 2011). Since the patches number increases with the number of classes (Castilla et al., 2009; Liang et al., 2013), the decision to include, exclude or aggregate patches in cover classes implies several ways of quantifying spatial patterns in the same landscape (Li and Wu, 2004; Bailey et al., 2007; Castilla et al., 2009; Marshall et al., 2020). Lower thematic resolutions with a small number of cover classes have a more straightforward classification and may aggregate different functional covers, suppressing the necessary level of details to explain biological variables from landscape attributes (Lawler et al., 2004; Marshall et al., 2020). Higher thematic resolutions, on the other hand, result in more detailed mapping of landscape composition and functional covers (e.g., habitat specialists) (Kendall et al., 2011; Liang et al., 2013), increasing the probability to detect associations between biological attributes (e.g., species richness) and landscape structure (Qiu et al., 2019; Marshall et al., 2020). For these reasons, increasing or decreasing the number of cover classes can modify landscapes’ functional heterogeneity quantification (Li and Wu, 2004; Lechner and Rhodes, 2016). Thus, our ability of understanding spatio-temporal effects of landscape structure on biodiversity depends on the choice of a thematic resolution that represents the landscape elements that have the most significant explanatory power for ecological responses (Buyantuyev and Wu, 2007; Kendall et al., 2011; Qiu et al., 2019).
The landscape heterogeneity is an essential predictor of biodiversity and positively influences biological attributes such as animal richness and abundance, besides ecological interactions as plant-pollinator (Fahrig et al., 2011; Stein et al., 2014; Moreira et al., 2015, 2018). There is a higher diversity of landscape covers in more heterogeneous landscapes, which may be the niche or complementary habitats for different species (Dunning et al., 1992; Fahrig et al., 2011; Boscolo et al., 2017). The positive effect of landscape heterogeneity has been reported for different biological groups, including bees (Moreira et al., 2015; Boscolo et al., 2017; Coutinho et al., 2020). The higher bee diversity in heterogeneous landscapes has been linked to a high resource diversity essential for feeding, nesting, and maintaining populations in the landscape (Moreira et al., 2017; Nery et al., 2018). However, estimating the effects of landscape heterogeneity on bees depends on choosing a thematic resolution that can distinguish necessary habitats and meaningful landscape covers for the different bee species (Kallioniemi et al., 2017; Marshall et al., 2020).
Bees are essential for the maintaining of the ecosystem’s functioning and services through pollination. However, they have been critically affected by landscape degradation and simplification processes (Garibaldi et al., 2011; Viana et al., 2012). The neotropical Euglossini bees constitute an important model for understanding the impact of landscape changes (Brosi, 2009; Cândido et al., 2018). Besides the high dependence on forest environments (Powell and Powell, 1987; Nemésio and Silveira, 2010), euglossine males are easily sampled with aromatic baits (Dodson et al., 1969; Roubik and Hanson, 2004). This tribe comprises about 240 species distributed in five genera (Moure et al., 2012), with a higher diversity reported for the tropical rain forests of South America (Roubik and Hanson, 2004; Nemésio, 2009). Previous studies already showed that changes in the structure of the Euglossini bee community are associated with changes in the landscape (e.g., Powell and Powell, 1987; Brosi, 2009; Cândido et al., 2018; Rocha-Filho et al., 2020). The increase in isolation between forest patches influenced the decline in the species richness of these bees (Powell and Powell, 1987), while the total abundance and richness seem to depend on variables such as shape, size (ha) and isolation (m) of the patches (Brosi, 2009; Nemésio and Silveira, 2010). In addition to forest cover, euglossine bees can be positively influenced by the landscape compositional heterogeneity (Opedal et al., 2020), including different anthropogenic surrounding matrix types (i.e., pasture, agriculture, forestry, or urban areas) (Aguiar et al., 2015; Brito et al., 2017; Cândido et al., 2018). Some species with remarkable environmental plasticity may use these environments to obtain floral resources, as nectar and perfumes (Briggs et al., 2013; Aguiar et al., 2015). Therefore, analyzing non-forest covers is essential to evaluate the euglossine community’s responses to the landscape composition and the importance of such environments for these pollinators.
The Euglossini bee communities are characterized by a few dominant species and many rare species (Aguiar and Gaglianone, 2008; Cândido et al., 2018; Rocha-Filho et al., 2020). The dominant euglossine species are habitat generalists and have wide occurrence and tolerance to landscape changes (Aguiar and Gaglianone, 2008; Silva and Marco, 2014; Aguiar et al., 2015). In contrast, rare species are generally restricted to more preserved areas (Tonhasca et al., 2002; Ramalho et al., 2009), resulting in a high sensitivity of these species to landscape disturbances. It is important to consider that the responses of species, communities, or species assemblages to landscape structure at different scales of effects (i.e., the extent of analysis in which a landscape attribute has a high influence on the biological response) can be divergent (Boscolo and Metzger, 2009; Fahrig, 2013; Gestich et al., 2018). An alternative to measuring the scale of effect of a community is to focus on which species were sampled because the species have different life histories, which influence their responses to landscape changes (Fischer and Lindenmayer, 2007; Fahrig, 2013; Hanski, 2015). Therefore, defining the proper spatial extents (i.e., scales of effect) when assessing ecological processes is challenging but is an essential step when considering landscape-based effects.
In this study, we aimed to evaluate how thematic resolution influences our ability to assess the influence of landscape composition on the euglossine bee species. Moreover, we also aimed to understand how species sensitivity to landscape disturbance can be influenced by landscape structure quantified in different thematic resolutions. For this, species dominance and occurrence frequency in the community were used as a proxy of species sensitivity. Specifically, we evaluated the association between five attributes of the euglossine bee community (richness, total abundance, abundance of common, intermediate, and rare species) with compositional metrics [cover (%) of landscape classes and landscape heterogeneity]. We hypothesized that the landscape composition in high thematic resolutions result in a higher explanatory power on bee community attributes (Figure 1). We also hypothesized that the euglossine community is mainly influenced by forest cover followed by spatial heterogeneity and non-forest covers (Figure 1). Given the dependence of Euglossini bees on forest environments (Roubik and Hanson, 2004), we expected: (a) the richness, total abundance, and species group abundance (common, intermediate, and rare) increase with the forest cover (%) (Figure 1A). Given the positive influence of heterogeneity on biodiversity, particularly on insects (Fahrig et al., 2011; Boscolo et al., 2017; Coutinho et al., 2020), we expected: (b) the richness and rare species abundance increase with heterogeneity but decreases when heterogeneity is high (Figure 1B), and (c) total abundance, abundance of common and intermediate species increases with heterogeneity (Figure 1B). Since non-forest environments are less permeable to some Euglossini species and negatively correlated with richness and abundance (Briggs et al., 2013; Aguiar et al., 2015), we also expect (d) a negative effect of non-forest covers on the five attributes of the Euglossini community (Figure 1C). We used euglossine males as our ecological model because (a) they have high flight capacity and respond to landscape degradation (Janzen, 1971; Brosi, 2009; Cândido et al., 2018), (b) they have requirements of nectar and floral perfumes that can be found in several habitats types and landscape cover, resulting in plant pollination in fragmented ecosystems (Roubik and Hanson, 2004; Rocha-Filho et al., 2012; Aguiar et al., 2015), and (c) they can indirectly indicate the abundance of Euglossini females (Opedal et al., 2016).
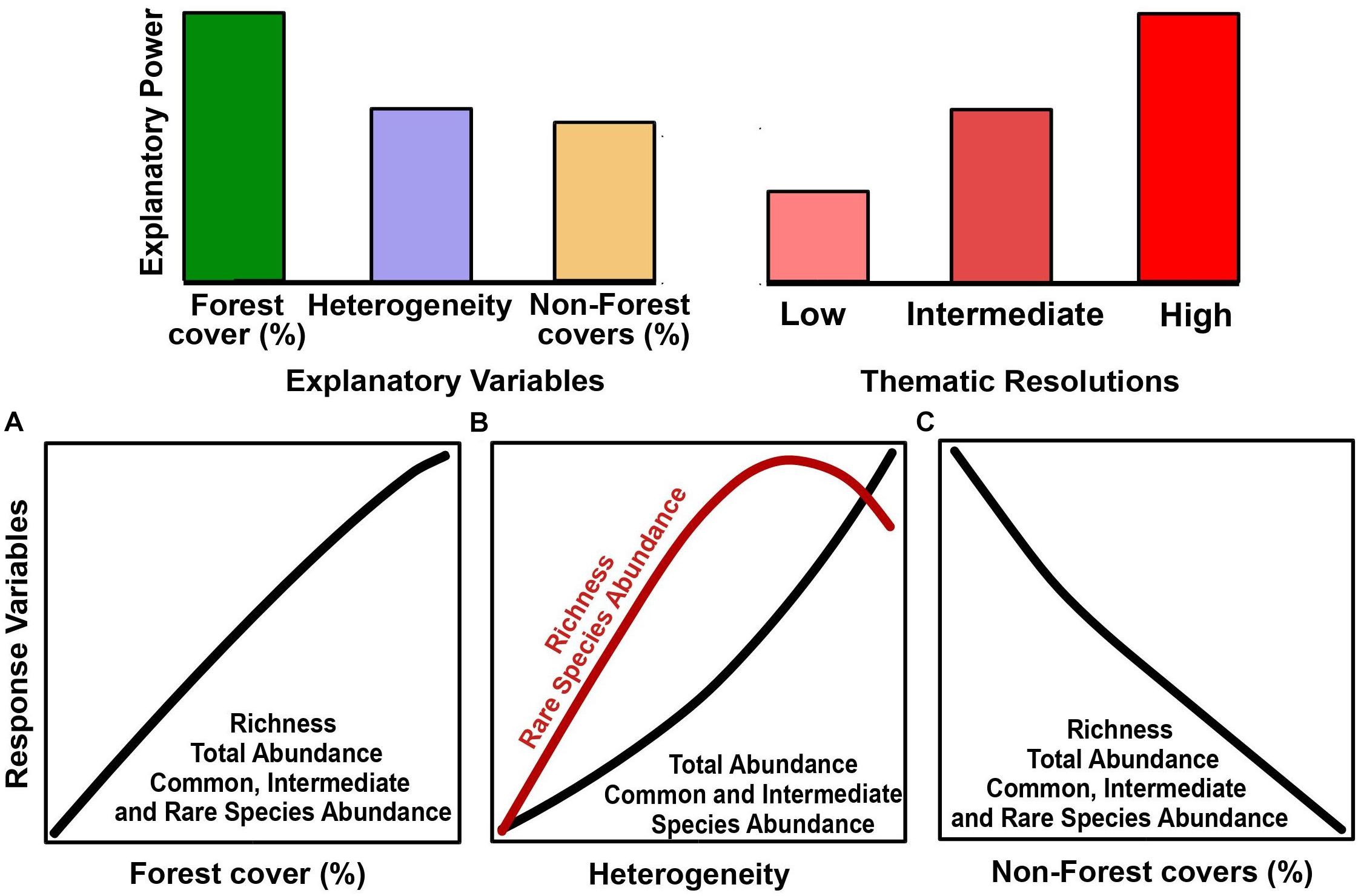
Figure 1. Hypotheses and predictions of the effects of explanatory variables [forest cover (A), heterogeneity (B), and non-forest covers (C)], and thematic resolution (low, intermediate, and high) on the parameters of the Euglossini bee community (richness, total abundance, common species abundance, intermediate species abundance, and rare species abundance).
Materials and Methods
Study Area
We carried out this study in 15 landscapes (L01-L15) in the Southeast of Brazil, located in Rio de Janeiro, Espírito Santo, and Minas Gerais states (Figure 2 and Supplementary Table 1). The region has a subtropical altitude climate of the Cwa, and Cwb types, with hot and rainy summer and cold and dry winter. This area was originally covered by phytophysiognomies of the semideciduous seasonal forest and dense montane and submontane forest, characteristics of the Atlantic Forest (Lumbreras et al., 2004; Garbin et al., 2017). The fragmentation process in the region occurred mainly during the economic coffee cycle in the nineteenth century. Coffee crops (Coffea arabica L., and Coffea canephora P.) occur mainly in many small farms, between 1 and 3 ha, which together span large areas (Figure 2). We selected the landscapes along a gradient of forest cover (10–66%).
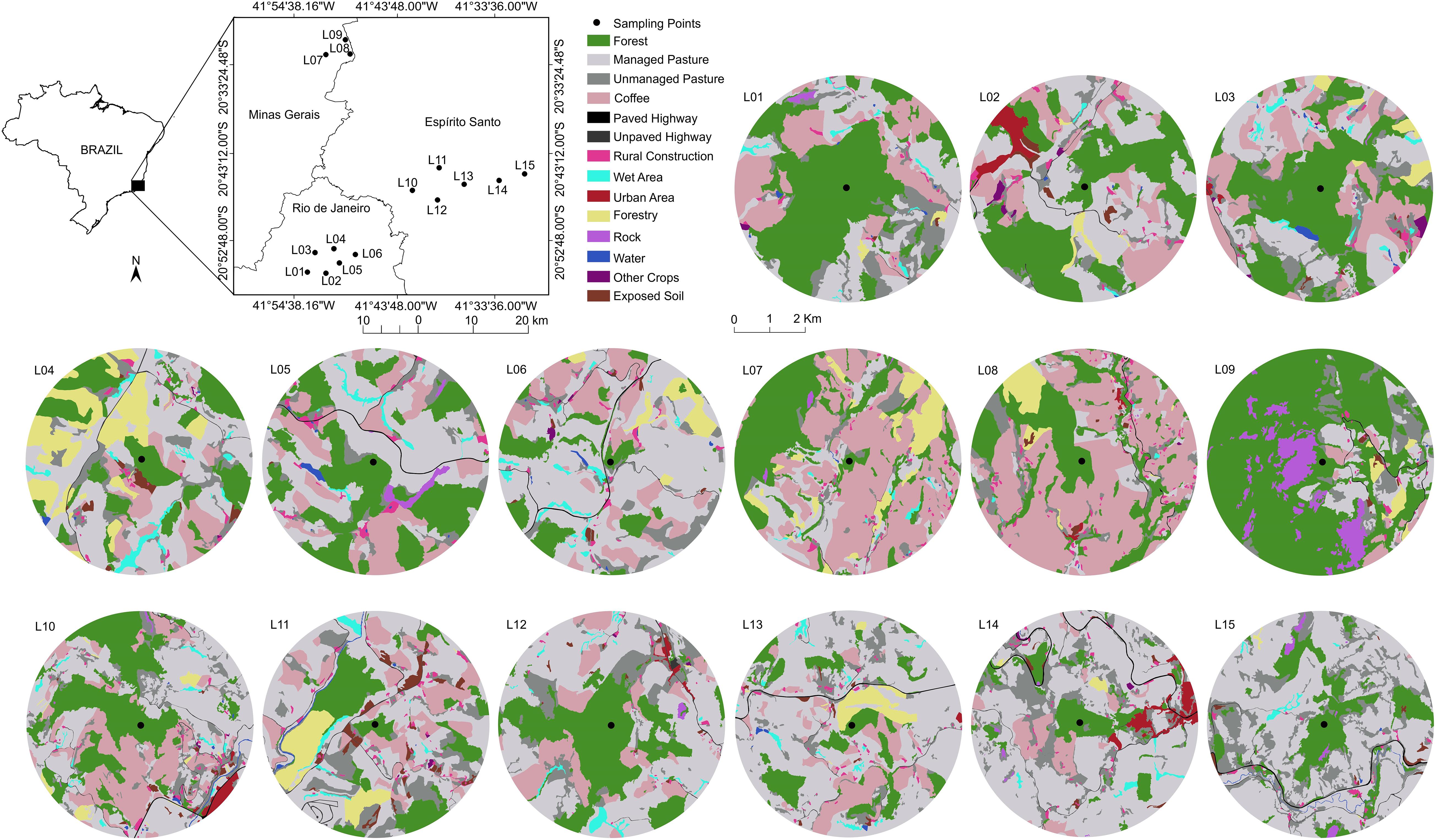
Figure 2. Geographic location and composition of the 15 landscapes in 14-class thematic resolution used for sampling Euglossini bees in the Southeast of Brazil.
Bee Sampling
We sampled bees on each landscape for 3 days in the rainy season (November 2019 to March 2020) and 2 days in the dry season (August to September 2019, July 2020). We chose a greater sampling effort in the rainy season because the euglossine bee species show a higher activity during this season (Roubik and Hanson, 2004). Some species also have been associated with the rainy season (Roubik and Hanson, 2004).
A sampling point was selected within a forest fragment in each of the 15 landscapes (Figure 2). The minimum distance from the fragment edge was 200 m, and the minimum distance between each sampling point was 2,500 m. We chose forest fragments from secondary successional stages for sampling bees. We used five bait traps to attract euglossine males at each sampling point. These traps were built with polyethylene terephthalate- PET bottles (Aguiar and Gaglianone, 2008), containing one of the five baits soaked in cotton (methyl cinnamate, eugenol, eucalyptol, methyl salicylate, and vanillin). These scents are widely used to sample Euglossini bees (Aguiar and Gaglianone, 2008, 2012; Aguiar et al., 2015; Rocha-Filho et al., 2020). The traps were hung in the vegetation, at the height of 1.5 m from the ground, and a minimum distance of 2.0 m between each other (Aguiar and Gaglianone, 2008). We distributed the traps randomly in each landscape on sunny days, early in the morning (06:00–08:00 h), and removed them in the afternoon (03:00–05:00 h). This passive sampling method allows standardization and also sampling in several areas simultaneously. When necessary, the sampled individuals were killed in a chamber with ethyl acetate for taxonomic identification. We deposited the specimens dry in the entomological collection of the Ecologia Experimental sector of the Laboratório de Ciências Ambientais- LCA, Universidade Estadual do Norte Fluminense Darcy Ribeiro- UENF.
Landscape Delimitation and Land Cover Classification
The landscapes were delimited by buffers with a radius of 1,500 m from the centroid of bee sampling locations. We mapped the land cover classes of each landscape using high-resolution satellite images (1-m resolution) available in ArcGis software on a 1:2,500 scale. The mapping was made by generating vector polygons in ArcGIS, followed by visual classification. We used a thematic resolution of 14 land cover classes for mapping (Figure 2). The biological importance of each of the 14 classes for euglossine bees is shown in Table 1. The vector maps was converted into raster files (5-m resolution). Because we aimed to quantify the effect of thematic resolution on our ability to explain bee responses, we used the R raster package (Hijmans, 2020) and aggregated the 14 land cover classes onto lower thematic resolutions maps: 12, 6, and 3 classes. For the thematic resolution with 3 classes, we generated two different classifications- see 3 and 3.1 in Table 2.
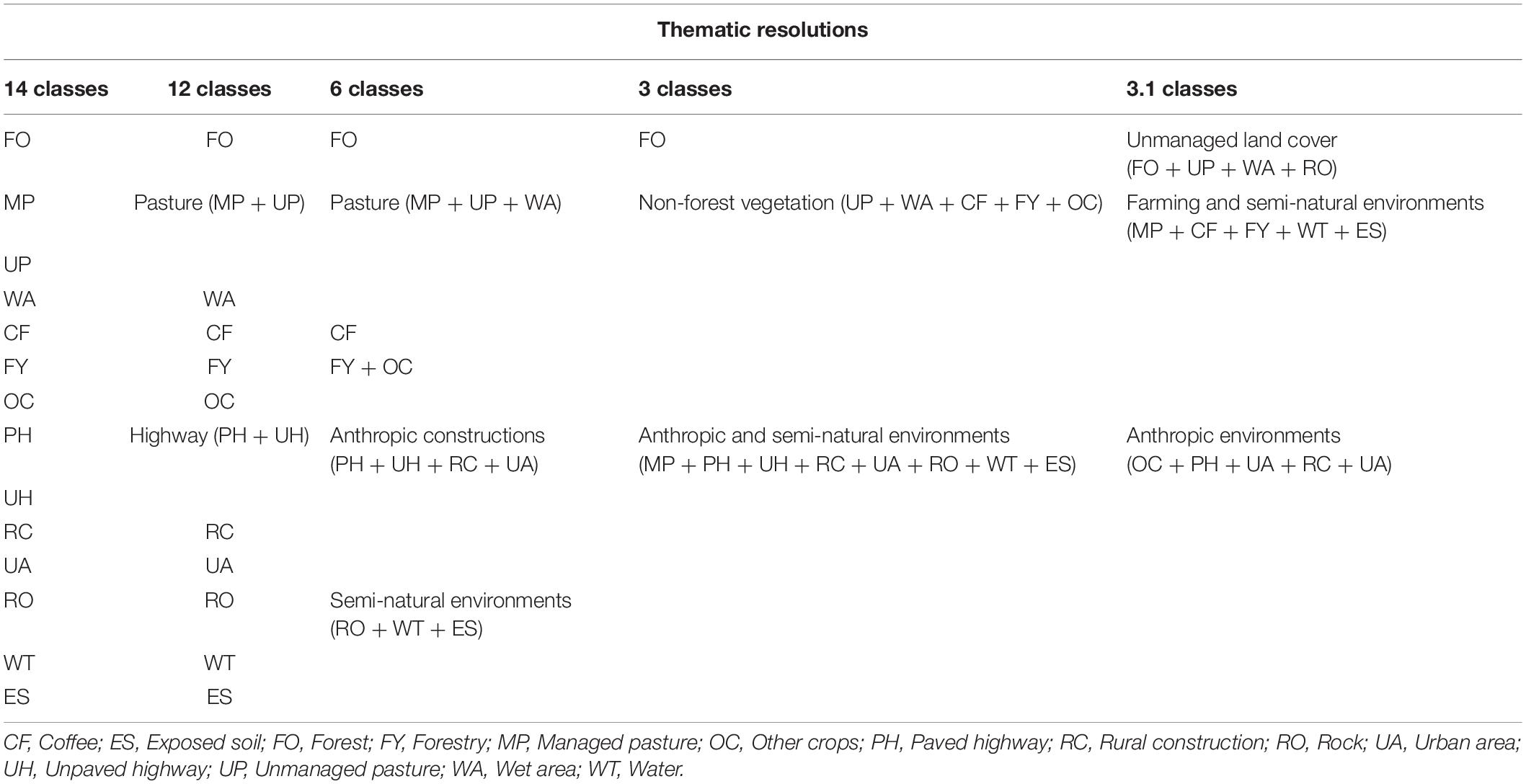
Table 2. Number and arrangement of classes used to classify the landscape in five thematic resolutions.
We downgrade the thematic resolution based on land use and management similarities, and the class functionality for the bees. Thus, we aggregated classes that could be perceived and used similarly by euglossine species. We first aggregated subtype classes into single classes, as expected to have a similar effect on the explanatory power. Thus, in the 12-class thematic resolution, we only aggregated the pasture and highway subtypes into two unique classes (“Pasture” and “Highway”). In the 6-class thematic resolution, we also considered the different levels of human activities over the landscape. Four covers resulted of human activities were aggregated in “Anthropic constructions”. We aggregated as “Semi-natural environments” three covers distributed naturally in the landscape but may be subject to anthropic changes (Table 1). Likewise, the class “Pasture” aggregated three cover classes, including managed and unmanaged pasture, and “wet areas” that are spatially associated (Table 1 and Figure 2). We aggregated “Forestry and other crops” as a single cover class. However, we kept “Coffee” as a single cover class in this resolution because of its high proportion in the landscape and potential influence on the Euglossini communities (see Table 1). In the 3-class thematic resolution, we aggregated all covers with arboreal-shrub vegetation (Table 2) that can provide floral resources for euglossine bee species in “Non-forest vegetation” (Table 1). Likewise, we aggregated all covers with open but non-arboreal vegetations or occupied by humans in “Anthropic and semi-natural environments” since their abiotic conditions could be less friendly to euglossine bees (Table 1). Finally, in the 3.1-class thematic resolution, we aggregated all covers that could be fundamental or complementary habitats for Euglossini bees in “Unmanaged land cover” (Tables 1, 2). We aggregated in “Farming and semi-natural environments” covers with high management intensity or open environments that could present less suitable environmental conditions for euglossine bees (Tables 1, 2). In the last class (“Anthropic environments”), we aggregated covers including anthropic constructions or those spatially associated (“Other crops”) (Tables 1, 2).
Landscape Metrics
We used the lsm function of the R landscapemetrics package (Hesselbarth et al., 2019) to calculate two landscape metrics for the five thematic resolutions: (a) cover (%) of classes in the landscape (PLAND = percentage that each class occupies regarding the landscape area), and (b) landscape heterogeneity (SHDI = Shannon diversity index calculates the proportion and diversity of classes in the landscape) (McGarigal, 2015). The forest is an essential habitat for the Euglossini species requirements (e.g., nectar, pollen, resin, floral perfumes, nesting sites) (Roubik and Hanson, 2004; Rocha-Filho et al., 2012). The landscape heterogeneity represents the environmental diversity that Euglossini species can use and also influence the community composition of Euglossini (Aguiar et al., 2015; Opedal et al., 2020). The cover of classes also allows measuring non-forest covers in the landscape (e.g., pastures, crops) that may represent complementary habitats for euglossine bees (Briggs et al., 2013; Aguiar et al., 2015). Moreover, it is known that cover (%) of classes and heterogeneity are good predictors of Euglossini bee communities (Cândido et al., 2018; Opedal et al., 2020). We calculated these landscape metrics at different spatial scales: 500, 750, 1,000, and 1,500 m. As we have an interest in quantifying the relative contribution of forest cover (%) and heterogeneity on explaining bee responses, we calculated the Pearson’s correlation between these metrics for all the spatial scales and all the thematic resolution to identify which spatial scale presents the lower correlations. Therefore, we evaluated the low (r < 0.5) and non-significant (p > 0.05) Pearson’s correlation between the forest cover and heterogeneity in the five thematic resolutions (14, 12, 6, 3, and 3.1 classes). After critical analysis and based on previous studies (Moreira et al., 2017; Cândido et al., 2018), we decided to use only the metrics calculated at the 1000 m spatial scale for all the thematic resolutions (see Supplementary Table 2).
Euglossini Bee Response Variables
Five aspects of euglossine bee community structure were used as response variables: richness, total abundance, common species abundance, intermediate species abundance, and rare species abundance. We quantified abundances using occurrence frequency (OF) and dominance (D) of each species in the community, in which OF = number of samples with species i/number of total samples ∗ 100, and D = abundance of species i/total abundance ∗ 100. When OF ≥ 50%, the species were classified as very frequent (vf), if OF < 50% and ≥ 5%, the species were frequent (f), and OF < 25%, the species were low frequent (lf) (Bodenheimer, 1955). If D ≥ 5%, the species were categorized as dominant (d), D < 5% and ≥ 2.5% the species were accessory (a), and D < 2.5% the species were occasional (o) (Bodenheimer, 1955). The OF and D values were combined, allowing the categorization of the three groups of species: Ct = vf + d = common species, Ct = lf + o = rare species; Ct = other combinations = intermediate species (Palma, 1975; Aguiar and Gaglianone, 2012).
Data Analysis
Our data analysis comprised two steps. First, we performed exploratory analyses using Generalized Linear Models (GLMs) to find the models in which the landscape attributes best explained the euglossine community in each thematic resolution. In the second step, we made a new GLM selection using only the best models previously selected. In this step, the best models that had the cover (%) of classes or landscape heterogeneity as explanatory variables in each thematic resolution were ranked with each other. Thus, we accessed the landscape attributes with the higher explanatory power on the euglossine community and the thematic resolution (i.e., high or low) in which these attributes were measured. We presented the details of the two steps below.
Step 1
As altitude is a very influential factor in the Euglossini communities (Aguiar and Gaglianone, 2012; Pinto et al., 2019), we first quantified the influence of altitude on species richness and total abundance using linear regressions. Before that, abundance was log-transformed using base 10. The diagnosis of these models was made by the boxcox function of the R MASS package (Venables and Ripley, 2002). Given the observed altitude influence on the euglossine community (Supplementary Figure 1), we removed the altitude’s effect on the response variables by analyzing residuals from linear models, in which the altitude was the explanatory variable (Response Variable ∼ Altitude).
We sought to explore through Generalized Linear Models (GLMs) an association of the residues of the euglossine community attributes with the cover (%) of forest and non-forest classes, and landscape heterogeneity in each thematic resolution. We build models that combined the landscape heterogeneity, cover (%) of forest, pastures, and coffee as explanatory variables in resolutions of 14, 12, and 6 classes (Supplementary Table 3). These three covers represented the largest proportion of the landscape composition (76–98%) in these resolutions. In resolutions of 3 and 3.1 classes, the models combined landscape heterogeneity and the three types of covers as explanatory variables (Supplementary Table 3). We used the residuals of the response variables to associate with the landscape metrics, so the Gaussian distribution was used in the GLMs. We used Akaike’s Information Criterion corrected for small sample size (AICc) (Burnham and Anderson, 2002) to rank the models. The model with the lowest ΔAICc was considered the most explanatory model. Also, models in which ΔAICc < 2.0 and the model weight (wi) > 0.1 were considered equally plausible to explain the patterns. A null model- that assumes no association between the response and explanatory variable- was also considered in the model comparison. We used the Ictab function from the R bbmle package to select the models (Bolker and R Development Core Team, 2020). In this step, we analyzed 255 models in the five thematic resolutions (Supplementary Table 3), of which 59 models were selected for Step 2 (Supplementary Table 4).
Step 2
From the selected models in step 1, we performed another round of model comparison to identify the thematic resolution that better explain the response variables to the landscape attributes (heterogeneity and cover (%) of classes) (Supplementary Table 4). The null model was also included as a competing model in the model selection procedure. The best models were chosen using the same criteria presented above (i.e., ΔAICc < 2.0 and wi > 0.1). We accessed the coefficient of determination (R2) of the “variance-function-based” type of the best GLMs to quantify the variation of the response variable explained by the landscape attributes. For this, we used the rsq function from the R rsq package (Zhang, 2020).
Results
Overview
We sampled 1890 male Euglossini bees, distributed in four genera and 14 species (Supplementary Table 5). Eulaema nigrita Lepeletier (55.1%) and Euglossa cordata (Linnaeus) (25.5%) were the most abundant species in the community. The total abundance in the landscapes ranged from 7 to 391 individuals (126 ± 111), while the richness varied between 4 and 9 species (6.4 ± 1.5).
Common species (S = 4) were sampled in all 15 landscapes (N = 1783 individuals; variation in landscapes 118 ± 110), intermediate species (S = 4) were recorded in 14 landscapes (N = 96 individuals, variation in landscapes 6.4 ± 4.2), while rare species (S = 6) occurred in eight landscapes (N = 11, 0.73 ± 0.79) (Supplementary Table 5).
Best Thematic Resolutions to Explain the Euglossini Community From Landscape Composition
The Euglossini community showed different responses to the landscape attributes in the thematic resolutions. The low thematic resolution detected significant effects of landscape composition on the residual richness (ΔAICc < 2.0 and wi > 0.1). The 3-class resolution showed a positive effect of landscape heterogeneity on the residual richness (R2 = 0.28) (Figure 3A and Table 3). The second plausible model in this resolution combined the positive effect of heterogeneity and negative effect of forest cover on the residual richness (R2 = 0.37) (Figure 3B and Table 3).
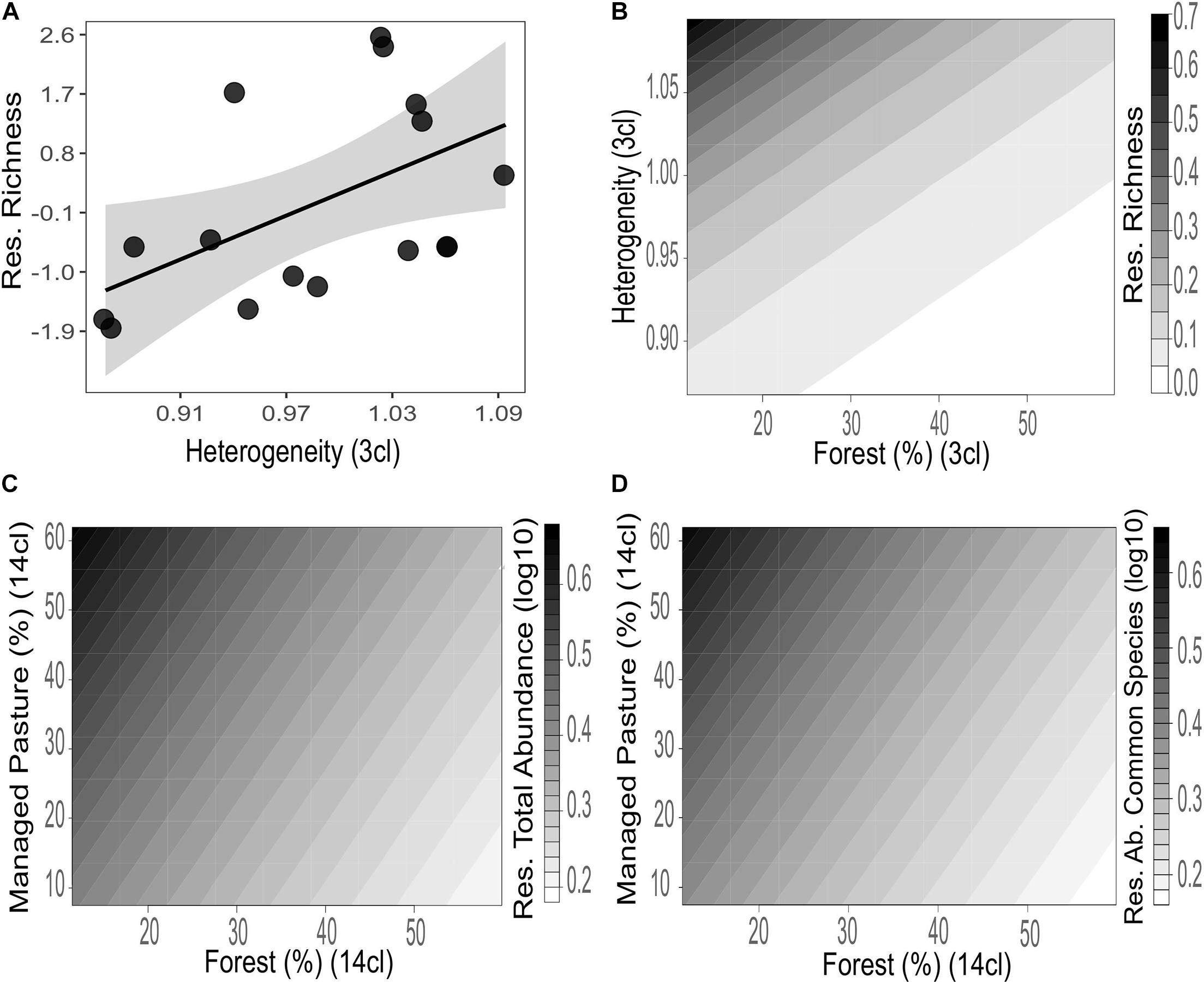
Figure 3. Influence of the landscape composition on the residues of richness (A,B), total abundance (C), and common species abundance (D) of Euglossini bees in different thematic resolutions. The black line represents the GLM model fitting and the gray shadow the 95% confidence interval.
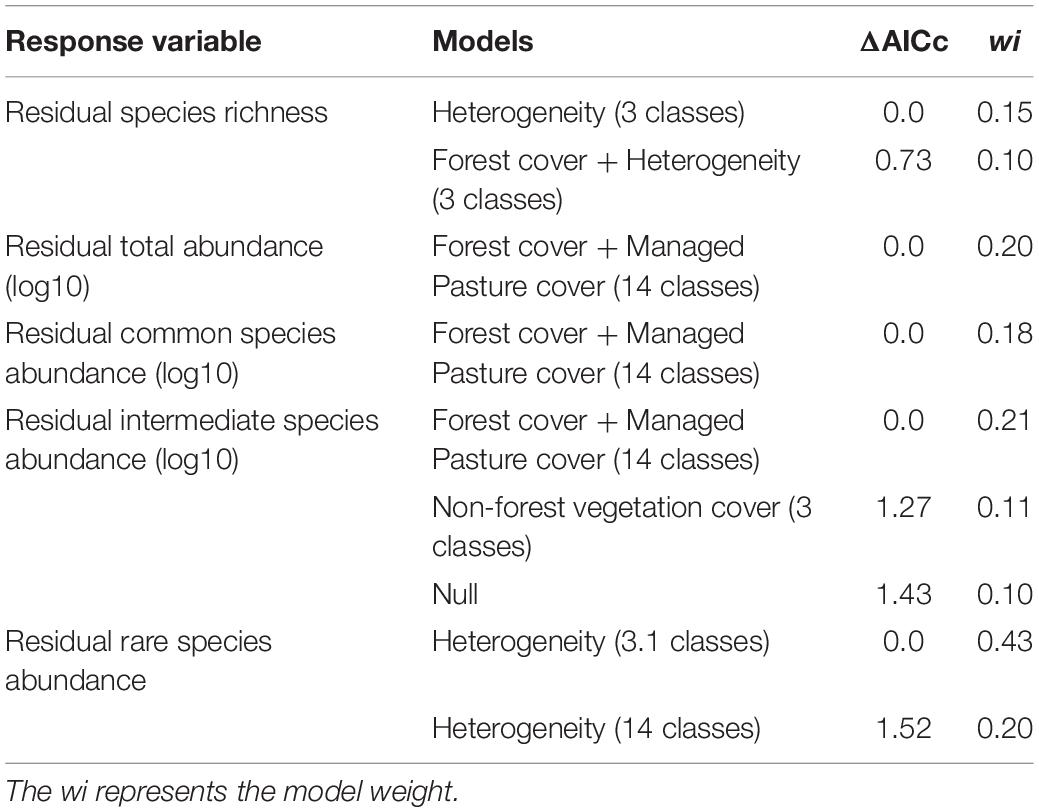
Table 3. Best models (ΔAICc < 2.0 and wi > 0.1) to explain the attributes of the Euglossini community from the landscape composition in different thematic resolutions.
In a high thematic resolution (14-class), the residual total abundance and residual common species abundance presented significant responses to landscape composition. The best models combined positive effects of managed pasture and negative effects of forest cover on both residual total abundance (R2 = 0.57) (Figure 3C) and residual common species abundance (R2 = 0.56) (Figure 3D and Table 3).
The landscape composition in both high and low thematic resolutions explained the residual abundance of intermediate and rare species. The 14-class resolution presented a positive effect of forest + managed pasture covers on the residual intermediate species abundance (R2 = 0.26) (Figure 4A and Table 3). These species abundance was also negatively related to the non-forest vegetation cover in the 3-class resolution (R2 = 0.19) (Figure 4B and Table 3). However, the null model was in the set of plausible models (ΔAICc < 2.0) (Table 3). Finally, the residual rare species abundance was best explained by a positive correlation with the landscape heterogeneity of the 3.1-class (R2 = 0.34) and 14-class resolution (R2 = 0.27) (Figures 4C,D and Table 3).
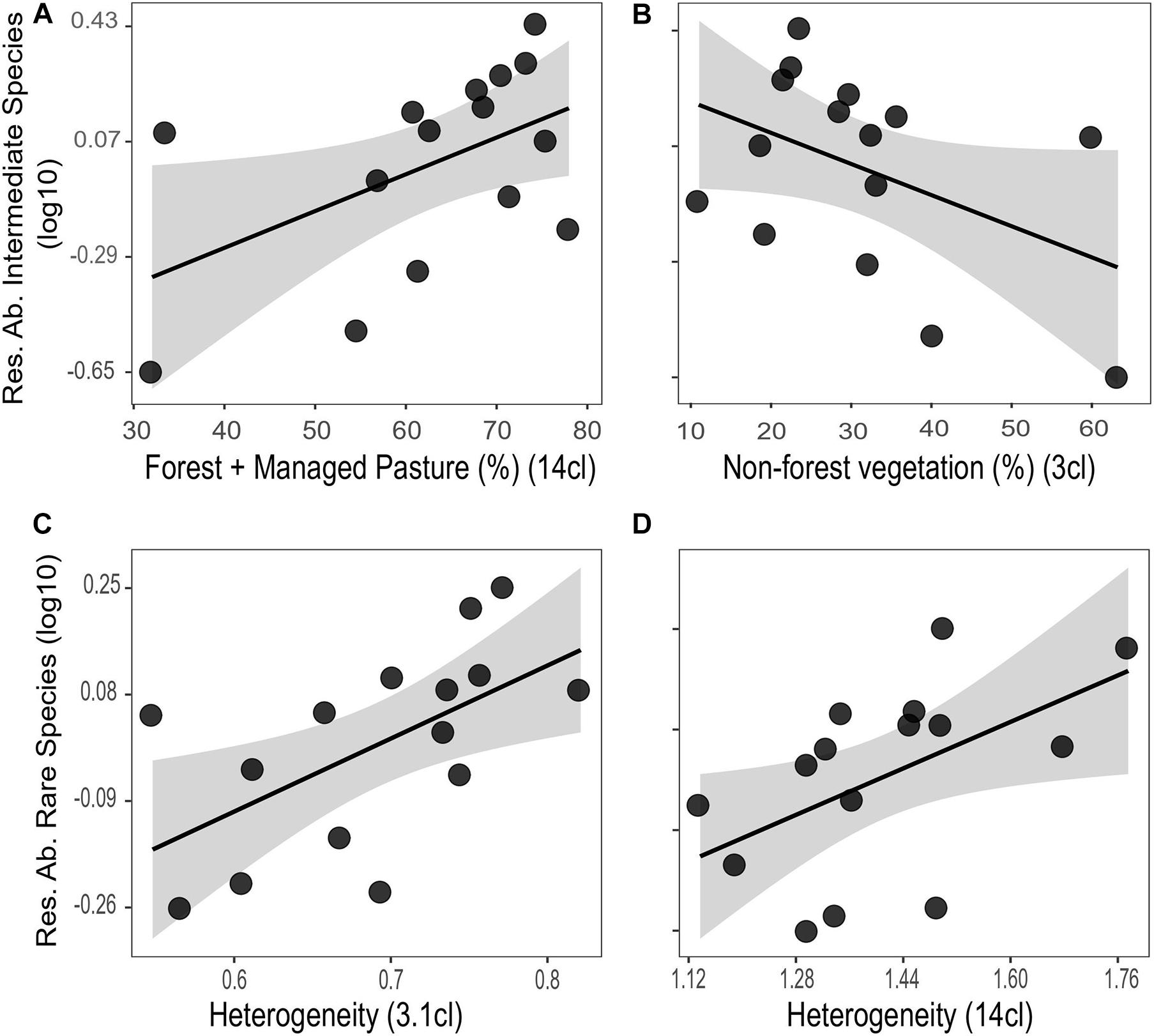
Figure 4. Influence of the landscape composition on the residues of intermediate species abundance (A,B), and rare species abundance (C,D) of Euglossini bees in different thematic resolutions. The black line represents the GLM model fitting and the gray shadow the 95% confidence interval.
Discussion
This study showed that our ability to explain attributes of the Euglossini community (richness, total abundance, abundance of common, intermediate, and rare species) from the landscape composition depends on the thematic resolution level. We refuted our hypothesis that the euglossine community are mainly influenced by forest cover since the landscape heterogeneity, and non-forest covers had a higher explanatory power on euglossine bees. We supported the hypothesis that high thematic resolution has a higher explanatory power through the relationship of the total abundance, abundance of common and intermediate species with the landscape composition in high thematic resolution (i.e., 14 classes). However, this hypothesis was refuted for the richness and rare species abundance explained by the landscape compositional heterogeneity in low thematic resolutions (3 and 3.1 classes).
Influence of Compositional Heterogeneity and Thematic Resolution
In fragmented landscapes, biodiversity is influenced by spatial heterogeneity (Fahrig et al., 2011). The positive relationship between the richness of different bee groups and landscape heterogeneity has been reported (Boscolo et al., 2017; Moreira et al., 2017; Coutinho et al., 2020; Opedal et al., 2020), indicating the importance of landscape diversity for the biological requirements of these pollinators. It is expected that the landscape structure in fine thematic resolutions better explains species richness (Lawler et al., 2004; Qiu et al., 2019). The association found between richness and heterogeneity at low resolution may indicate that several Euglossini bee species can perceive the landscape on a coarser scale, where different types of landscape cover have been aggregated into the same class. This may have occurred because, in addition to the forest, these bees use different types of cover for foraging (Aguiar et al., 2015; Brito et al., 2017), which may present a supply of resources, such as nectar and floral scents (Aguiar et al., 2015; Opedal et al., 2020). Despite the landscape heterogeneity in low thematic resolution reflects the aggregation of many landscape covers, the forest cover combined with heterogeneity in the model increased the explanatory power on species richness. This indicates the importance of this landscape composition attribute to explain the euglossine community (Brosi, 2009; Cândido et al., 2018). Although we expected a unimodal response of species richness to heterogeneity, we observed a linear relationship between these two attributes. These landscapes seem to support a level of compositional heterogeneity that results in a high euglossine richness. Unimodal relationships occur at high levels of spatial and environmental heterogeneity because it is when the negative effects of heterogeneity act on population dynamics (e.g., extinction, colonization) (Fahrig et al., 2011; Ben-Hur and Kadmon, 2020).
The highest total abundance and common species abundance were observed in landscapes with low forest cover and a high pasture cover. The association between total abundance and landscape cover was very similar to the association observed for common species abundance (Eulaema nigrita, Euglossa cordata, Euglossa fimbriata Rebêlo & Moure, and Euglossa securigera Dressler). If we consider the relative abundance of these four common species, they represented 94.3% of the sampled individuals. This calls attention to studies that consider only total abundance as a response variable to assess the effects of landscape changes on communities. These dominant species have higher phenotypic plasticity and tolerance to changes in the landscape (Silva and Marco, 2014; Aguiar et al., 2015; Carneiro et al., 2019). Therefore, this may underestimate the effects of these processes on groups of less abundant species in the community.
In addition to the forest, pasture cover (%) had a high influence on the abundance of most euglossine species. These bees have high flight capacity (Janzen, 1971), and several species have already been sampled on the forest edges or within the pasture matrix (Aguiar et al., 2015). Despite presenting different characteristics of forest environments (e.g., high temperatures, low humidity, high wind speed), this matrix may offer floral resources through pioneer plants for euglossine species with higher environmental plasticity (Aguiar et al., 2015). These relationships between total abundance, abundance of common and intermediate species with forest environments, and pasture matrices were best explained by mapping in high thematic resolution (i.e., 14 classes). In higher thematic resolutions, many areas that may be used as habitats are identified (Liang et al., 2013; Marshall et al., 2020). Thus, for these evaluated abundance variables, the 14-class thematic resolution can better indicate the “landscape complementation” (Dunning et al., 1992), as it would represent more realistically the environment mosaic that euglossine males use to obtain resources. Despite a lower explanatory power, the intermediate species abundance was negatively affected by the non-forest vegetation in the lowest thematic resolution. This landscape cover was mainly composed by crops (e.g., coffee, forestry). Some intermediate species (e.g., Euglossa clausii Nemésio & Engel, Euglossa truncata Rebêlo & Moure) have been known as restricted to forest (Ramalho et al., 2009; Aguiar et al., 2015). This may indicate that crop areas can negatively affect these species and the euglossine communities (Briggs et al., 2013; Aguiar et al., 2015).
The landscape heterogeneity was the most important variable to explain the rare species abundance. Among the response variables that we analyzed, the abundance of this group of species was the only one that showed a strong association with the thematic resolution of 3.1 classes. The responses to compositional heterogeneity for each group of species are distinct and driven by the relationship that organisms have with each landscape cover class (Lawler et al., 2004; Kendall et al., 2011; García-Álvarez et al., 2019). In the resolution of 3.1 classes, we considered ‘Unmanaged land cover’ as a heterogeneous environment, composed of classes with natural (i.e., forest) and semi-natural vegetation, which can provide resources and conditions for the euglossine species. However, the study area comprises an old forest cover loss, with most of the forest patches composed of secondary vegetation. Thus, many of the bee species categorized as rare may show adaptations to open environments. Some of these species (e.g., Eulaema atleticana Nemésio, Euglossa pleosticta Dressler) are medium and large size bees, which can forage in matrices neighboring the forest fragments (Aguiar et al., 2015; Rosa et al., 2015). Therefore, the conservation of these environments in the landscape is essential to keep the diversity of these species. The loss of compositional heterogeneity can negatively affect rare and specialist species, resulting in biological homogenization (Gámez-Virués et al., 2015; Martello et al., 2018).
Conclusion
This study showed that compositional heterogeneity influences the Euglossini bee community, both positively and negatively, and these responses depend on the thematic resolution used to characterize the landscape. The decision to aggregate class covers in low thematic resolutions showed that different types of patches in the landscape could be functionally similar for euglossine species. On the other hand, high thematic resolutions revealed that non-forest cover (i.e., managed pasture) has a high explanatory power on the most common species in the community. However, it is important to be cautious about the thematic resolution’s influence on explaining some euglossine community attributes. This because the abundance of rare and intermediate species were correlated with the landscape composition in both high and low thematic resolutions. Furthermore, we showed that species dominance could be an important proxy for understanding species sensitivity to landscape disturbances. Because in these areas of the Southeast of Brazil, forest cover is threatened by agricultural activities such as coffee crops, we call attention to conserving forest remnants. At the same time, these landscapes still have a high diversity of environments linked to the many types of land use that small farmers maintain on their properties (e.g., agroforestry, unmanaged pastures). These friendly landscapes favored, for example, the species richness and rare species abundance. Agricultural intensification is a growing phenomenon, especially in tropical regions. If these practices are associated with landscape homogenization, pollinators such as bees can be critically affected.
We also call attention to consider the influence of the thematic resolution on the association of biological parameters with landscape metrics in future studies. With the advent of new technologies and spatial data sets, including landscape mapping in large regions (e.g., MapBiomas in Brazil), the use of different thematic resolutions becomes more accessible. As we showed, the choice of thematic resolution is a critical step that influences our ability to explain biological parameters from the landscape structure.
Data Availability Statement
The original contributions presented in the study are included in the article/Supplementary Material, further inquiries can be directed to the corresponding author/s.
Author Contributions
LC and MG conceived the study design and wrote the manuscript. WA, MG, LC, and MR designed methodological approach. LC and WF-S collected the data. LC and MR calculated the landscape metrics. MR, WF-S, and LC analyzed the data. CP and LC mapped the landscapes. All authors revised the manuscript.
Funding
We thank the CNPq/MCTIC/IBAMA/A.B.E.L.H.A. for financial support (project 400614/2018-9). LC and WF-S thank CAPES for the scholarship (processes 88887.339454/2019-00; 88882.314552/2019-01); CP thanks FAPERJ for the scholarship. MG thanks CNPq (process 303894/2018-0) and FAPERJ (process 203.321/2017) for their financial support. MR thanks FAPESP (processes 2013/50421-2; 2020/01779-5), CNPq (processes 312045/2013-1; 312292/2016-3) and PROCAD/CAPES (project 88881.068425/2014-01) for their financial support.
Conflict of Interest
The authors declare that the research was conducted in the absence of any commercial or financial relationships that could be construed as a potential conflict of interest.
Acknowledgments
We thank the Instituto Brasileiro do Meio Ambiente e dos Recursos Naturais Renováveis-IBAMA for the authorization to collect biological material (No. 71013-3), the owners for authorization the study in their properties, Dr. Gabriel Augusto Rodrigues de Melo (UFPR) for taxonomic confirmation, and Vivian F. Manhães, Luiza F.S.L. Carvalho, Sônia G. Alves, Mariana S. Deprá, and Anna P. Hautequestt for field help.
Supplementary Material
The Supplementary Material for this article can be found online at: https://www.frontiersin.org/articles/10.3389/fevo.2021.628319/full#supplementary-material
References
Aguiar, W. M., and Gaglianone, M. C. (2008). Comunidade de abelhas Euglossina (Hymenoptera: Apidae) em remanescentes de mata estacional semidecidual sobre tabuleiro no estado do Rio de Janeiro. Neotrop. Entomol. 37, 118–125. doi: 10.1590/s1519-566x2008000200002
Aguiar, W. M., and Gaglianone, M. C. (2012). Euglossine bee communities in small forest fragments of the Atlantic Forest, Rio de Janeiro state, southeastern Brazil (Hymenoptera, Apidae). Rev. Bras. Entomol. 56, 210–219. doi: 10.1590/s0085-56262012005000018
Aguiar, W. M., Sofia, S. H., Melo, G. A., and Gaglianone, M. C. (2015). Changes in orchid bee communities across forest-agroecosystem boundaries in Brazilian Atlantic forest landscapes. Environ. Entomol. 44, 1465–1471. doi: 10.1093/ee/nvv130
Allen, T. F. H., and Hoekstra, T. W. (1991). “Role of heterogeneity in scaling of ecological systems under analysis,” in Ecological Heterogeneity, eds J. Kolasa and S. T. A. Pickett (New York, NY: Springer), 47–68.
Augusto, S. C., and Garófalo, C. A. (2007). Nidificação de Euglossa (Euglossa) melanotricha Moure (Hymenoptera: Apidae) no solo do cerrado. Neotrop. Entomol. 36, 153–156. doi: 10.1590/s1519-566x2007000100021
Bailey, D., Herzog, F., Augenstein, I., Aviron, S., Billeter, R., Szerencsits, E., et al. (2007). Thematic resolution matters: indicators of landscape pattern for European agro-ecosystems. Ecol. Indic. 7, 692–709. doi: 10.1016/j.ecolind.2006.08.001
Bender, D. J., and Fahrig, L. (2005). Matrix structure obscures the relationship between interpatch movement and patch size and isolation. Ecology 86, 1023–1033. doi: 10.1890/03-0769
Ben-Hur, E., and Kadmon, R. (2020). Heterogeneity–diversity relationships in sessile organisms: a unified framework. Ecol. Lett. 23, 193–207. doi: 10.1111/ele.13418
Boff, S., and Alves-dos-Santos, I. (2018). Cavities in bromeliad stolons used as nest sites by Euglossa cordata (Hymenoptera. Euglossini). J. Hymenopt. Res. 62, 33–44. doi: 10.3897/jhr.62.22834
Bolker, B., and R Development Core Team (2020). bbmle: Tools for General Maximum Likelihood Estimation. R Package Version 1.0.23.1. Available online at: https://CRAN.R-project.org/package=bbmle (accessed October 15, 2020).
Boscolo, D., and Metzger, J. P. (2009). Is bird incidence in Atlantic forest fragments influenced by landscape patterns at multiple scales? Landsc. Ecol. 24, 907–918. doi: 10.1007/s10980-009-9370-8
Boscolo, D., Tokumoto, P. M., Ferreira, P. A., Ribeiro, J. W., and Santos, J. S. (2017). Positive responses of flower visiting bees to landscape heterogeneity depend on functional connectivity levels. Perspect. Ecol. Conserv. 15, 18–24. doi: 10.1016/j.pecon.2017.03.002
Briggs, H. M., Perfecto, I., and Brosi, B. J. (2013). The role of the agricultural matrix: coffee management and euglossine bee (Hymenoptera: Apidae: Euglossini) communities in Southern Mexico. Environ. Entomol. 42, 1210–1217. doi: 10.1603/en13087
Brito, T. F., Phifer, C. C., Knowlton, J. L., Fiser, C. M., Becker, N. M., Barros, F. C., et al. (2017). Forest reserves and riparian corridors help maintain orchid bee (Hymenoptera: Euglossini) communities in oil palm plantations in Brazil. Apidologie 48, 575–587. doi: 10.1007/s13592-017-0500-z
Brosi, B. J. (2009). The effects of forest fragmentation on euglossine bee communities (Hymenoptera: Apidae: Euglossini). Biol. Conserv. 142, 414–423. doi: 10.1016/j.biocon.2008.11.003
Burnham, K. P., and Anderson, D. R. (2002). Model Selection and Multimodel Inference: A Practical Information-Theoretic Approach. New York, NY: Springer.
Buyantuyev, A., and Wu, J. (2007). Effects of thematic resolution on landscape pattern analysis. Landsc. Ecol. 22, 7–13. doi: 10.1007/s10980-006-9010-5
Cândido, M. E. M. B., Morato, E. F., Storck-Tonon, D., Miranda, P. N., and Vieira, L. J. S. (2018). Effects of fragments and landscape characteristics on the orchid bee richness (Apidae: Euglossini) in an urban matrix, southwestern Amazonia. J. Insect Conserv. 22, 475–486. doi: 10.1007/s10841-018-0075-7
Carneiro, L. S., Aguiar, C. M. L., Aguiar, W. M., Aniceto, E. S., Nunes, L. A., and Ferreira, V. S. (2019). Morphometric variability among populations of Euglossa cordata (Hymenoptera: Apidae: Euglossini) from different phytophysiognomies. Sociobiology 66, 575–581. doi: 10.13102/sociobiology.v66i4.4675
Castilla, G., Larkin, K., Linke, J., and Hay, G. J. (2009). The impact of thematic resolution on the patch-mosaic model of natural landscapes. Landsc. Ecol. 24, 15–23. doi: 10.1007/s10980-008-9310-z
Coutinho, J. G. E., Angel-Coca, C., Boscolo, D., and Viana, B. F. (2020). Heterogeneous agroecosystems support high diversity and abundance of trap-nesting bees and wasps among tropical crops. Biotropica 52, 991–1004. doi: 10.1111/btp.12809
Dodson, C. H., Dressler, R. L., Hills, H. G., Adams, R. M., and Williams, N. H. (1969). Biologically active compounds in orchid fragrances. Science 164, 1243–1249. doi: 10.1126/science.164.3885.1243
Dressler, R. L. (1982). Biology of the orchid bees (Euglossini). Annu. Rev. Ecol. Evol. Syst 13, 373–394. doi: 10.1146/annurev.es.13.110182.002105
Duarte, G. T., Santos, P. M., Cornelissen, T. G., Ribeiro, M. C., and Paglia, A. P. (2018). The effects of landscape patterns on ecosystem services: meta-analyses of landscape services. Landsc. Ecol. 33, 1247–1257. doi: 10.1007/s10980-018-0673-5
Dunning, J. B., Danielson, B. J., and Pulliam, H. R. (1992). Ecological processes that affect populations in complex landscapes. Oikos 65, 169–175. doi: 10.2307/3544901
Fahrig, L. (2003). Effects of habitat fragmentation on biodiversity. Annu. Rev. Ecol. Evol. Syst. 34, 487–515. doi: 10.1146/annurev.ecolsys.34.011802.132419
Fahrig, L. (2013). Rethinking patch size and isolation effects: the habitat amount hypothesis. J. Biogeogr. 40, 1649–1663. doi: 10.1111/jbi.12130
Fahrig, L., Baudry, J., Brotons, L., Burel, F. G., Crist, T. O., Fuller, R. J., et al. (2011). Functional landscape heterogeneity and animal biodiversity in agricultural landscapes. Ecol. Lett. 14, 101–112. doi: 10.1111/j.1461-0248.2010.01559.x
Fischer, J., and Lindenmayer, D. B. (2007). Landscape modification and habitat fragmentation: a synthesis. Glob. Ecol. Biogeogr. 16, 265–280. doi: 10.1111/j.1466-8238.2007.00287.x
Gámez-Virués, S., Perović, D. J., Gossner, M. M., Börschig, C., Blüthgen, N., de Jong, H., et al. (2015). Landscape simplification filters species traits and drives biotic homogenization. Nat. Commun. 6:8568. doi: 10.1038/ncomms9568
Garbin, M. L., Saiter, F. Z., Carrijo, T. T., and Peixoto, A. L. (2017). Breve histórico e classificação da vegetação capixaba. Rodriguésia 68, 1883–1894. doi: 10.1590/2175-7860201768521
García-Álvarez, D., Lloyd, C. D., Van Delden, H., and Olmedo, M. T. C. (2019). Thematic resolution influence in spatial analysis. An application to land use cover change (LUCC) modelling calibration. Comput. Environ. Urban Syst. 78:101375. doi: 10.1016/j.compenvurbsys.2019.101375
Garibaldi, L. A., Steffan-Dewenter, I., Kremen, C., Morales, J. M., Bommarco, R., Cunningham, S. A., et al. (2011). Stability of pollination services decreases with isolation from natural areas despite honey bee visits. Ecol. Lett. 14, 1062–1072. doi: 10.1111/j.1461-0248.2011.01669.x
Gestich, C. C., Arroyo-Rodríguez, V., Ribeiro, M. C., Cunha, R. G. T., and Setz, E. Z. F. (2018). Unraveling the scales of effect of landscape structure on primate species richness and density of titi monkeys (Callicebus nigrifrons). Ecol. Res. 34, 150–159. doi: 10.1111/1440-1703.1009
Haddad, N. M., Brudvig, L. A., Clobert, J., Davies, K. F., Gonzalez, A., Holt, R. D., et al. (2015). Habitat fragmentation and its lasting impact on Earth’s ecosystems. Sci. Adv. 1:e1500052. doi: 10.1126/sciadv.1500052
Hanski, I. (2015). Habitat fragmentation and species richness. J. Biogeogr. 42, 989–993. doi: 10.1111/jbi.12478
Hesselbarth, M. H. K., Sciaini, M., With, K. A., Wiegand, K., and Nowosad, J. (2019). landscapemetrics: an open-source R tool to calculate landscape metrics. Ecography 42, 1648–1657. doi: 10.1111/ecog.04617
Hijmans, R. J. (2020). raster: Geographic Data Analysis and Modeling. R Package Version 3.1-5. Available online at: https://CRAN.R-project.org/package=raster (accessed September 27, 2020).
Janzen, D. H. (1971). Euglossine bees as long-distance pollinators of tropical plants. Science 171, 203–205. doi: 10.1126/science.171.3967.203
Kallioniemi, E., Åström, J., Rusch, G. M., Dahle, S., Åström, S., and Gjershaug, J. O. (2017). Local resources, linear elements and mass-flowering crops determine bumblebee occurrences in moderately intensified farmlands. Agric. Ecosyst. Environ. 239, 90–100. doi: 10.1016/j.agee.2016.12.039
Kendall, M. S., Miller, T. J., and Pittman, S. J. (2011). Patterns of scale-dependency and the influence of map resolution on the seascape ecology of reef fish. Mar. Ecol. Prog. Ser. 427, 259–274. doi: 10.3354/meps08945
Kruess, A., and Tscharntke, T. (2002). Contrasting responses of plant and insect diversity to variation in grazing intensity. Biol. Conserv. 106, 293–302. doi: 10.1016/s0006-3207(01)00255-5
Lawler, J. J., O’Connor, R. J., Hunsaker, C. T., Jones, K. B., Loveland, T. R., and White, D. (2004). The effects of habitat resolution on models of avian diversity and distributions: a comparison of two land-cover classifications. Landsc. Ecol. 19, 517–532. doi: 10.1023/b:land.0000036151.28327.01
Lechner, A. M., and Rhodes, J. R. (2016). Recent progress on spatial and thematic resolution in landscape ecology. Curr. Landsc. Ecol. Rep. 1, 98–105. doi: 10.1007/s40823-016-0011-z
Li, H., and Wu, J. (2004). Use and misuse of landscape indices. Landsc. Ecol. 19, 389–399. doi: 10.1023/b:land.0000030441.15628.d6
Liang, Y., He, H. S., Fraser, J. S., and Wu, Z. (2013). Thematic and spatial resolutions affect model-based predictions of tree species distribution. PLoS One 8:e67889. doi: 10.1371/journal.pone.0067889
López-Uribe, M. M., Oi, C. A., and Del Lama, M. A. (2008). Nectar-foraging behavior of Euglossine bees (Hymenoptera: Apidae) in urban areas. Apidologie 39, 410–418. doi: 10.1051/apido:2008023
Lumbreras, J. F., Carvalho Filho, A., Mota, P. E. F., Palmieri, F., Calderano, S. B., Baruqui, A. M., et al. (2004). Macropedoambientes da Região Noroeste Fluminense - Uma Contribuição ao Planejamento Ambiental. Rio de Janeiro: Embrapa Solos.
Marshall, L., Beckers, V., Vray, S., Rasmont, P., Vereecken, N. J., and Dendoncker, N. (2020). High thematic resolution land use change models refine biodiversity scenarios: a case study with Belgian bumblebees. J. Biogeogr. 48, 345–358. doi: 10.1111/jbi.14000
Martello, F., Andriolli, F., de Souza, T. B., Dodonov, P., and Ribeiro, M. C. (2016). Edge and land use effects on dung beetles (Coleoptera: Scarabaeidae: Scarabaeinae) in Brazilian cerrado vegetation. J. Insect Conserv. 20, 957–970. doi: 10.1007/s10841-016-9928-0
Martello, F., Bello, F., Morini, M. S. C., Silva, R. R., Souza-Campana, D. R., Ribeiro, M. C., et al. (2018). Homogenization and impoverishment of taxonomic and functional diversity of ants in Eucalyptus plantations. Sci. Rep. 8:3266. doi: 10.1038/s41598-018-20823-1F
Moreira, E. F., Boscolo, D., and Viana, B. F. (2015). Spatial heterogeneity regulates plant-pollinator networks across multiple landscape scales. PLoS One 10:e0123628. doi: 10.1371/journal.pone.0123628
Moreira, E. F., Boscolo, D., and Viana, B. F. (2018). Beyond good and evil: context-dependent effects of agriculture on pollinattors’ communities and its interactions. Oecol Aust. 22, 489–502. doi: 10.4257/oeco.2018.2204.11
Moreira, E. F., Santos, R. L. D. S., Silveira, M. S., Boscolo, D., Neves, E. L. D., and Viana, B. F. (2017). Influence of landscape structure on Euglossini composition in open vegetation environments. Biota Neotrop. 17:e20160294. doi: 10.1590/1676-0611-bn-2016-0294
Moure, J. S., Melo, G. A. R., and Faria, L. R. R. Jr. (2012). “Euglossini Latreille, 1802,” in Catalogue of bees (Hymenoptera, Apoidea) in the Neotropical Region-Online Version, eds J. S. Moure, D. Urban, and G. A. R. Melo (Curitiba: Sociedade Brasileira de Entomologia).
Neel, M. C., McGarigal, K., and Cushman, S. A. (2004). Behavior of class-level landscape metrics across gradients of class aggregation and area. Landsc. Ecol. 19, 435–455. doi: 10.1023/b:land.0000030521.19856.cb
Nemésio, A. (2009). Orchid bees (Hymenoptera: Apidae) of the Brazilian Atlantic Forest. Zootaxa 2041, 1–242. doi: 10.11646/zootaxa.2041.1.1
Nemésio, A., and Silveira, F. A. (2010). Forest fragments with larger core areas better sustain diverse orchid bee faunas (Hymenoptera: Apidae: Euglossina). Neotrop. Entomol. 39, 555–561. doi: 10.15i">10.1590/s1519-566x2010000400014
PubMed Abstract | 10.1590/s1519-566x2010000400014" target="_blank">CrossRef Full Text | Google Scholar
Nery, L. S., Takata, J. T., Camargo, B. B., Chaves, A. M., Ferreira, P. A., and Boscolo, D. (2018). Bee diversity responses to forest and open areas in heterogeneous Atlantic Forest. Sociobiology 65, 686–695. doi: 10.13102/sociobiology.v65i4.3472
Opedal, ØH., Albertsen, E., Armbruster, W. S., Pérez-Barrales, R., Falahati-Anbaran, M., and Pélabon, C. (2016). Evolutionary consequences of ecological factors: pollinator reliability predicts mating-system traits of a perennial plant. Ecol. Lett. 19, 1486–1495. doi: 10.1111/ele.12701
Opedal, ØH., Martins, A. A., and Marjakangas, E. L. (2020). A database and synthesis of euglossine bee assemblages collected at fragrance baits. Apidologie 51, 519–530. doi: 10.1007/s13592-020-00739-4
Palma, S. G. (1975). Contribuitión al studio de los Sifonoforos encontrados frente a la costa de Valparaiso. Aspectos ecológicos. Memorias del Segundo Simposio Latinoamericano de Oceanografia Biològica, University de Oriente Cumaná, Venezuela 2, 119–133.
Pinto, A. R., Silveira, G. D. C., Gaglianone, M. C., and Freitas, L. (2019). Abrupt decrease in the diversity of Euglossini bees (Hymenoptera: Apidae) in a montane rainforest. J. Apic. Res. 58, 682–693. doi: 10.1080/00218839.2019.1637223
Powell, A. H., and Powell, G. V. N. (1987). Population dynamics of male euglossine bees in amazonian forest fragments. Biotropica 19, 176–179. doi: 10.2307/2388742
Prevedello, J. A., and Vieira, M. V. (2010). Does the type of matrix matter? A quantitative review of the evidence. Biodivers. Conserv. 19, 1205–1223. doi: 10.1007/s10531-009-9750-z
Qiu, Y., Teng, S. N., Zhang, Y., Santana, J., Svenning, J. C., Reino, L., et al. (2019). The resolution-dependent role of landscape attributes in shaping macro-scale biodiversity patterns. Glob. Ecol. Biogeogr. 28, 767–778. doi: 10.1111/geb.12889
Ramalho, A. V., Gaglianone, M. C., and Oliveira, M. L. (2009). Comunidades de abelhas Euglossina (Hymenoptera, Apidae) em fragmentos de Mata Atlântica no Sudeste do Brasil. Rev. Bras. Entomol 53, 95–101. doi: 10.1590/s0085-56262009000100022
Rocha-Filho, L. C., Krug, C., Silva, C. I., and Garófalo, C. A. (2012). Floral resources used by Euglossini bees (Hymenoptera: Apidae) in coastal ecosystems of the Atlantic Forest. Psyche 2012, 1–13. doi: 10.1155/2012/934951
Rocha-Filho, L. C., Montagnana, P. C., Boscolo, D., and Garofalo, C. A. (2020). Species turnover and low stability in a community of euglossine bees (Hymenoptera: Apidae) sampled within 28 years in an urban forest fragment. Apidologie 51, 921–934. doi: 10.1007/s13592-020-00772-3
Rosa, J. F., Ramalho, M., Monteiro, D., and Silva, M. D. (2015). Permeability of matrices of agricultural crops to Euglossina bees (Hymenoptera, Apidae) in the Atlantic Rain Forest. Apidologie 46, 691–702. doi: 10.1007/s13592-015-0359-9
Roubik, D. W., and Hanson, P. E. (2004). Orchids Bees of Tropical America: Biology and Field Guide. Heredia: INBio Press.
Silva, D. P., and Marco, P. Jr. (2014). No evidence of habitat loss affecting the orchid bees Eulaema nigrita Lepeletier and Eufriesea auriceps Friese (Apidae: Euglossini) in the Brazilian Cerrado Savanna. Neotrop. Entomol. 43, 509–518. doi: 10.1007/s13744-014-0244-7
Stein, A., Gerstner, K., and Kreft, H. (2014). Environmental heterogeneity as a universal driver of species richness across taxa, biomes and spatial scales. Ecol. Lett. 17, 866–880. doi: 10.1111/ele.12277
Tonhasca, A. J., Albuquerque, G. S., and Blackmer, J. L. (2003). Dispersal of euglossine bees between fragments of the Brazilian Atlantic Forest. J. Trop. Ecol. 19, 99–102. doi: 10.1017/s0266467403003122
Tonhasca, A. J., Blackmer, J. L., and Albuquerque, G. S. (2002). Abundance and Diversity of Euglossine Bees in the Fragmented Landscape of the Brazilian Atlantic Forest1. Biotropica 34, 416–422.
Turner, M. G., and Gardner, R. H. (2015). Landscape Ecology in Theory and Practice. New York, NY: Springer.
Venables, W. N., and Ripley, B. D. (2002). Modern Applied Statistics With S. Statistics and Computing, New York, NY: Springer, doi: 10.1007/978-0-387-21706-2
Viana, B. F., Boscolo, D., Mariano Neto, E., Lopes, L. E., Lopes, A. V., Ferreira, P. A., et al. (2012). How well do we understand landscape effects on pollinators and pollination services? J. Pollinat. Ecol. 7, 31–40. doi: 10.26786/1920-760320122
Zhang, D. (2020). rsq: R-Squared and Related Measures. R Package Version 2.1. Available online at: https://CRAN.R-project.org/package=rsq (accessed February 10, 2021).
Keywords: Atlantic Forest, Brazil, orchid bees, landscape structure, landscape diversity, mapping, pollinators
Citation: Carneiro LS, Aguiar WM, Priante CF, Ribeiro MC, Frantine-Silva W and Gaglianone MC (2021) The Interplay Between Thematic Resolution, Forest Cover, and Heterogeneity for Explaining Euglossini Bees Community in an Agricultural Landscape. Front. Ecol. Evol. 9:628319. doi: 10.3389/fevo.2021.628319
Received: 11 November 2020; Accepted: 13 April 2021;
Published: 07 May 2021.
Edited by:
Juliana Hipólito, National Institute of Amazonian Research (INPA), BrazilReviewed by:
Eduardo Freitas Moreira, Federal University of Bahia, BrazilØystein Opedal, Lund University, Sweden
Copyright © 2021 Carneiro, Aguiar, Priante, Ribeiro, Frantine-Silva and Gaglianone. This is an open-access article distributed under the terms of the Creative Commons Attribution License (CC BY). The use, distribution or reproduction in other forums is permitted, provided the original author(s) and the copyright owner(s) are credited and that the original publication in this journal is cited, in accordance with accepted academic practice. No use, distribution or reproduction is permitted which does not comply with these terms.
*Correspondence: Lázaro da Silva Carneiro, bGF6YXJvY2FybmVpcm8xNkBnbWFpbC5jb20=