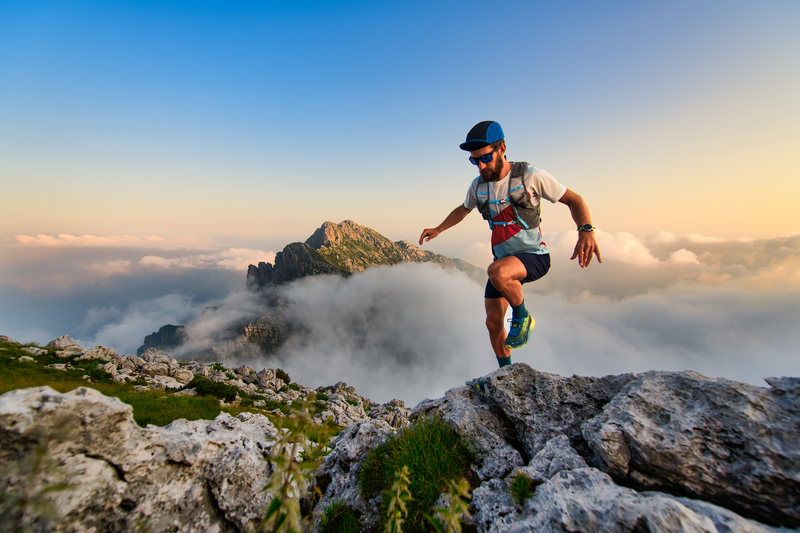
95% of researchers rate our articles as excellent or good
Learn more about the work of our research integrity team to safeguard the quality of each article we publish.
Find out more
ORIGINAL RESEARCH article
Front. Ecol. Evol. , 21 April 2021
Sec. Behavioral and Evolutionary Ecology
Volume 9 - 2021 | https://doi.org/10.3389/fevo.2021.619277
This article is part of the Research Topic Ecology, Evolution, and Behavior of Viviparous Fishes View all 19 articles
Predation risk and resource availability are two primary factors predicted by theory to drive the evolution of life histories. Yet, disentangling their roles in life-history evolution in the wild is challenging because (1) the two factors often co-vary across environments, and (2) environmental effects on phenotypes can mask patterns of genotypic evolution. Here, we use the model system of the post-Pleistocene radiation of Bahamas mosquitofish (Gambusia hubbsi) inhabiting blue holes to provide a strong test of the roles of predation and resources in life-history evolution, as the two factors do not co-vary in this system and we attempted to minimize environmental effects by raising eight populations under common laboratory conditions. We tested a priori predictions of predation- and resource-driven evolution in five life-history traits. We found that life-history evolution in Bahamas mosquitofish largely reflected complex interactions in the effects of predation and resource availability. High predation risk has driven the evolution of higher fecundity, smaller offspring size, more frequent reproduction, and slower growth rate—but this predation-driven divergence primarily occurred in environments with relatively high resource availability, and the effects of resources on life-history evolution was generally greater within environments having high predation risk. This implies that resource-driven selection on life histories overrides selection from predators when resources are particularly scarce. While several results matched a priori predictions, with the added nuance of interdependence among selective agents, some did not. For instance, only resource levels, not predation risk, explained evolutionary change in male age at maturity, with more rapid sexual maturation in higher-resource environments. We also found faster (not slower) juvenile growth rates within low-resource and low-predation environments, probably caused by selection in these high-competition scenarios favoring greater growth efficiency. Our approach, using common-garden experiments with a natural system of low- and high-predation populations that span a continuum of resource availability, provides a powerful way to deepen our understanding of life-history evolution. Overall, it appears that life-history evolution in this adaptive radiation has resulted from a complex interplay between predation and resources, underscoring the need for increased attention on more sophisticated interactions among selective agents in driving phenotypic diversification.
Growth rates and reproductive traits, such as age at sexual maturity and offspring size and number, can have strong effects on fitness and yet vary notably among and within species (Partridge and Harvey, 1988; Stearns, 1992; Roff, 2002). Life-history theory comprises a broad and diverse analytical framework that attempts to understand the causes and consequences of this variation. With their intimate relationship with fitness, combined with their importance in shaping the structure and dynamics of populations, communities, and ecosystems, life-history traits have attracted voluminous and long-standing research in evolutionary ecology (e.g., Lack, 1947; Reznick and Endler, 1982; Stearns, 1992; Roff, 2002; Bassar et al., 2010; Walsh et al., 2012). A fundamental goal of this work is to predict how life-history traits will evolve under particular environmental conditions (e.g., Stearns, 1976, 2000; Reznick and Endler, 1982; Reznick et al., 1997; Riesch et al., 2013; Moore et al., 2016).
A central axiom of life-history theory is that during an organism’s life, time and energy are finite, and thus organisms have evolved adaptive allocation of a limited energy budget across multiple competing tasks and functions (Stearns, 1992; Zera and Harshman, 2001; Roff, 2002; Flatt and Heyland, 2011). For instance, organisms must acquire necessary resources and allocate energy toward somatic growth, reproductive tissue, and other features. Trade-offs imposed by time and energy are proposed to drive phenotypic variation among different environmental conditions and across the lifespan of organisms (Van Noordwijk and de Jong, 1986; Stearns, 1989; Roff, 1992; Charnov, 1993; Roff, 2002). This trade-off framework forms a key foundation for the modern view of life-history evolution, yet we still have much to learn about how specific agents of selection directly and indirectly contribute to shape variation in key life-history traits in the wild (e.g., Riesch et al., 2020). For example, we have a wealth of research documenting statistical associations between important life-history traits and an array of potentially influential environmental variables (e.g., Ballinger, 1979; Reznick and Endler, 1982; Spitze, 1991; Martin, 1995; Riesch et al., 2014). Although informative, such associations are frequently difficult to interpret (due to covariation among ecological selective pressures) and thus, a contemporary challenge is to isolate and disentangle the role of specific ecological agents in the evolution of life histories.
Two of the most-studied ecological factors affecting time and energy allocation are predation risk and resource availability. These factors are generally considered the primary agents driving the evolution of life-history traits (e.g., Reznick and Endler, 1982; Martin, 1987; Lynch, 1989; Vanni and Lampert, 1992; Boggs and Ross, 1993; Martin, 1995; Reznick et al., 2001; Arendt and Reznick, 2005; Riesch et al., 2020). However, two key obstacles have so far hindered our ability to uncover their importance in shaping life histories in the wild. First, differences in resource availability often covary with predation regime: e.g., more productive and relatively nutrient-rich environments often support a larger number of interacting species (including predators), and predators often reduce prey population densities and indirectly elevate resource levels (Walsh and Reznick, 2008; Rudman et al., 2016). Thus, determining the relative roles of predation and resources in influencing life histories is statistically daunting, to say the least. Second, environmental effects on life-history phenotypes can obscure or even mask patterns of underlying genotypic life-history evolution—it may be naïve to assume that evolutionary responses to environmental factors mirror the phenotypic responses (e.g., Conover and Schultz, 1995). Hence, we need more than phenotype-environment associations observed in the field to understand genetic evolution of life histories (Berven, 1982; Reznick, 1982; Reznick and Bryga, 1996). One approach to address these obstacles is to measure evolutionary change subsequent to experimental manipulation of environmental factors (Reznick et al., 1990, 2019; Walsh and Reznick, 2011; Wathne et al., 2020). While certainly informative, this approach does not directly address how life histories actually evolve under natural conditions, and generally pertains to relatively short time scales. An alternative and powerful approach is to use model systems that offer “natural experiments,” where populations/species have evolved in distinct and well-characterized environments in the wild (Diamond, 1986). Ideally, we would like to investigate natural systems with (1) strong variation in, but little covariation among, predation risk and resource availability, (2) relatively few potentially confounding factors, and (3) the ability to raise the organism in a common environment to assess the genetic basis to trait divergence among populations.
Livebearing fishes (family Poeciliidae), such as guppies, mollies, swordtails, and mosquitofish, have been at the forefront of the development of life-history theory (e.g., Reznick and Endler, 1982; Reznick et al., 1990, 2002; Johnson, 2001; Johnson and Belk, 2001; Bronikowski et al., 2002; Jennions and Telford, 2002; Jennions et al., 2006; Riesch et al., 2014, 2015; Moore et al., 2016; Belk et al., 2020; Santi et al., 2020). One especially powerful model system of livebearing fish is the post-Pleistocene radiation of Bahamas mosquitofish (Gambusia hubbsi), as the system offers the opportunity to isolate the effects of predation risk and resource availability on life-history trait evolution. These small, livebearing fish inhabit blue holes that differ considerably in predation risk (presence/absence of predatory fish) and resource availability (continuous variation in density of zooplankton resources)—both factors are temporally consistent but do not covary with one another in this system (Heinen et al., 2013). Moreover, no known environmental factor should obscure the effects of these two agents on phenotypic evolution because previous research has investigated numerous environmental features of blue holes—e.g., temperature, salinity, turbidity, dissolved oxygen, pH, total phosphorus, total nitrogen, total organic carbon, surface area, water depth, and densities of chlorophyll a, phycocyanin, and phytoplankton—and documented a lack of covariation between these variables and either the presence of predatory fish or zooplankton density (e.g., Langerhans et al., 2007; Heinen et al., 2013; Björnerås et al., 2020). The fish are also highly amenable to laboratory rearing under common-garden conditions, enabling us to test for evolutionary divergence of life-histories in response to these ecological agents while minimizing direct environmental effects on the phenotypes (i.e., phenotypic plasticity).
Here we examined Bahamas mosquitofish raised in a common laboratory environment derived from eight populations varying in predation risk and resource availability. Based on an extensive body of theoretical and empirical studies, we tested a priori predictions of adaptive life-history divergence in response to these two selective agents (e.g., Gadgil and Bossert, 1970; Stearns, 1976; Law, 1979; Michod, 1979; Reznick et al., 1990, 1996, 2001, 2019; Charlesworth, 1994; Abrams and Rowe, 1996; Downhower et al., 2000; Gasser et al., 2000; Johnson, 2001; Dmitriew, 2011; Riesch et al., 2013, 2020). While further mathematical development of underlying theory is desirable for making clear and unambiguous predictions, our predictions here derive from research that links mortality rates (across all age classes), density regulation (e.g., resource competition, juvenile survival), and food availability to the adaptive evolution of life histories. First, we predicted that elevated extrinsic mortality rates, combined with reduced population densities experienced in populations with high predation risk would select for (1) higher fecundity, (2) smaller offspring size, (3) more frequent reproduction, (4) earlier age at maturity, and (5) more rapid growth. These predictions derive from both the direct effects of predator-induced mortality and the potential indirect effects of elevated resource competition in the absence of predators where population densities are much higher. Second, we predicted that relatively high resource availability would select for (1) higher fecundity, (2) smaller offspring size, (3) more frequent reproduction, (4) earlier age at maturity, and (5) slower growth due to lower growth efficiency. We note that an alternative prediction for the effect of predation risk on growth rate exists and may apply in this system: lower mortality rates and high densities in low-predation environments could select for higher growth efficiency in these highly competitive environments, leading to the prediction of more rapid growth in populations with low predation risk (Arendt and Wilson, 1999; Robinson and Partridge, 2001; Lindgren and Laurila, 2005). While all environmental/transgenerational effects cannot be fully excluded from our study, we attempted to minimize these sources by raising multiple generations under common conditions. Thus, we interpret phenotype-environment correlations that emerge after common-garden rearing to reflect signals of local adaptation, and thus, largely of genetic origin.
Inland blue holes (water-filled, vertical caves) on Andros Island, The Bahamas were colonized by Bahamas mosquitofish during the past ∼15,000 years, harbor relatively simple fish and plankton communities (e.g., typically 1–3 fish species, 1–3 dominant zooplankton species), appear very stable through time, and are quite isolated from one another in most cases (Fairbanks, 1989; Langerhans and Gifford, 2009; Heinen et al., 2013; Heinen-Kay and Langerhans, 2013; Riesch et al., 2013; Björnerås et al., 2020; Sha et al., 2021). The post-Pleistocene radiation of Bahamas mosquitofish in these blue holes is largely characterized by predator-driven multi-trait adaptation (e.g., Langerhans et al., 2007; Heinen-Kay and Langerhans, 2013; Anderson and Langerhans, 2015; Heinen-Kay et al., 2015; Fowler et al., 2018; Langerhans, 2018), although resource availability appears to also play some role in phenotypic differentiation (e.g., Heinen et al., 2013; Martin et al., 2014; Riesch et al., 2020). In some blue holes, Bahamas mosquitofish coexist with a major predatory fish (bigmouth sleeper, Gobiomorus dormitor), resulting in a clear dichotomy between “high-predation” blue holes, where predators impose strong mortality and effectively reduce Bahamas mosquitofish population densities, and “low-predation” blue holes with no major fish predators and consequently relatively low mortality rates (e.g., Langerhans et al., 2007; Heinen et al., 2013; Martin et al., 2015; Riesch et al., 2020). While high-predation localities clearly have greater adult mortality rates, prior analyses of population densities and age structure suggest that all age classes of Bahamas mosquitofish suffer higher mortality in the presence of bigmouth sleepers, with population densities considerably lower than low-predation localities (Heinen et al., 2013; Riesch et al., 2020; see Supplementary Material).
Blue holes also range widely in primary productivity and resource availability, spanning over an order of magnitude in the density of their key prey, zooplankton (primarily copepods; Gluckman and Hartney, 2000; Heinen et al., 2013; Araujo et al., 2014; Sha et al., 2021). Following previous work, in this study we treated predation regime as a categorical variable based on the presence/absence of bigmouth sleeper, and measured resource availability as the density of zooplankton. Zooplankton densities were taken from a previous study (Heinen et al., 2013). Briefly, we estimated zooplankton densities using a 60-m tow of a zooplankton net (20-cm diameter, 153-μm mesh) at 0.5-m depth. Thus, zooplankton densities were estimated in habitats where Bahamas mosquitofish are abundant within all sites (Heinen et al., 2013). We counted all zooplankton within a 2.5-ml subsample of each plankton collection using a stereo microscope. Resource estimates in blue holes show high repeatability across seasons and years (intraclass correlation coefficients: 0.88–0.98; Heinen et al., 2013), and recent detailed investigations of zooplankton densities and depth distributions in a subset of six blue holes have confirmed the utility of these measurements in capturing consistent, among-population variation in resource levels (intraclass correlation coefficient of standardized zooplankton densities measured 7–8 years apart: 0.95; Sha et al., 2021). Zooplankton density does not covary with predation regime across blue holes (n = 19 blue holes, P = 0.71), nor with G. hubbsi density (n = 17 blue holes, P = 0.69); a pattern consistent within the subset of eight blue holes examined here (both P > 0.38). Zooplankton density appears to represent a strong and robust estimate of resource availability for Bahamas mosquitofish in blue holes (see Supplementary Material). However, owing to the large differences in G. hubbsi population densities between predation regimes caused by predator-induced mortality, some of the phenotypic differences between these predatory environments could reflect density-dependent selection. That is, the higher population densities within low-predation environments likely result in more intense resource competition compared to high-predation environments (see Supplementary Material). Therefore, when we refer to effects of predation risk in this study, those effects could arise from direct effects of mortality rates or indirect effects of altered population density (we assess the possible causes in the Discussion). Throughout, we use “predation regime” or “predation risk” to refer to the presence/absence of bigmouth sleepers in the population of origin, and “resource availability” or “resource level” to refer to the zooplankton density in the population of origin.
Wild G. hubbsi were collected under snorkel using hand-held dip nets from eight inland blue holes that differ in predation regime (4 low-predation, 4 high-predation; Supplementary Figure S1) during two occasions in 2016 (8–20 June, 30 September–3 October) and transported to experimental facilities at North Carolina State University. We selected these blue holes a priori as representative of the larger set of blue holes on Andros Island (e.g., Langerhans, 2018). The fish that formed the wild-caught parental generation (F0) were collected as newborns (in an attempt to minimize maternal and environmental effects) and subsequently reared to adulthood in single-sex groups (to keep fish virgin until breeding) in re-circulating systems providing biological, mechanical and UV filtration. Municipal source water was filtered using a 5-stage filtration system (Aqua FX Mako 5-stage RO/DI system, Aqua Engineering and Equipment, Inc., Winter Park, Florida), treated to reclaim electrolytes and general hardness (R/O Right, KENT Marine, Franklin, Wisconsin), buffered to a pH of approximately 8.3 (Marine Buffer, Seachem Laboratories, Madison, Georgia), and maintained at approximately 1.5 ppt salinity (∼2,850 μS, Instant Ocean, Blacksburg, Virginia). Digital networked controllers (ReefKeeper Lite, Digital Aquatics, Everett, Wisconsin; Remote Operator, Unitronics, Quincy, Massachusetts) maintained water temperature at approximately 25°C, photoperiod at 14-h light/10-h dark, and monitored salinity and pH. Fish were fed ad libitum daily with a mixture of TetraPro Tropical Crisps (Tetra, Blacksburg, Virginia) and freeze-dried daphnia, bloodworms and brine shrimp (Hikari, Hayward, California). F0 fish were raised in 115-L tanks with artificial vegetation (6 tanks per population, ∼10 fish per tank).
To measure fecundity, offspring size, and interbrood interval of lab-raised F0 fish (see below), and to acquire F1 fish, sexually mature F0 fish were uniquely mated: each female mated with a single male, with no fish used more than once. We isolated mated females within the re-circulating systems in 38-L chambers, and after approximately 4 weeks (or when visibly showing signs of late-stage pregnancy) we provided very dense artificial vegetation as safe refuge for newborn offspring (to minimize cannibalism from females). We visually screened for newborn offspring at least once per day. Mated females remained isolated after parturition of their first brood so we could potentially measure fecundity and offspring size across multiple broods per female.
To measure fecundity and the size of offspring delivered by F1 fish, and to acquire F2 fish for our growth-rate and maturation experiment, we raised F1 fish in 115-L rearing tanks within the re-circulating systems and subsequently mated them after sexual maturity. We pooled outbred families of similar age within populations (152 total full-sibling F1 families, ∼9 115-L tanks per population) and separated sexes upon first sign of male maturation. We mated F1 females to multiple unrelated males from the same population (to maintain high genetic diversity), and held mated females in groups of ∼12 per 115-L tank with dense artificial vegetation. We screened tanks daily for newborn offspring.
Prior work examining numerous blue hole populations of Bahamas mosquitofish has shown higher fecundity and smaller offspring size in Bahamas mosquitofish inhabiting high-predation blue holes (Downhower et al., 2000; Riesch et al., 2013; Riesch et al., 2020). However, prior tests for genetically based divergence in these traits have been extremely limited (three populations), with no previous study measuring interbrood interval, age at maturity, or growth rate, or directly testing the roles of predation regime or resource levels on life histories of common-garden raised individuals. Here, we quantified fecundity (number of offspring per brood), offspring size (body size at birth), interbrood interval (number of days between reproductive events), age at maturity in males, and juvenile growth rate for fish derived from eight populations and raised under common laboratory conditions.
To measure fecundity and offspring size for F1 and F2 offspring broods, we examined a total of 471 mated lab-raised females derived from the eight populations (145 F0, 326 F1 females), comprising a total of 866 broods for fecundity (270 F1 broods, 596 F2 broods) and 747 broods for offspring size at birth (199 F1 broods, 548 F2 broods) (see Supplementary Table S1 for details). For F1 broods only, we measured the interbrood interval between 113 pairs of broods—we could not measure this in F2 broods because we did not individually track females across multiple broods in that case.
Each time newborn offspring were observed in a tank, we immediately removed all offspring, counted them, placed them in a 1-L beaker filled with <2-cm deep water, and photographed them from above using a tripod-mounted DSLR camera (Canon T3i; Canon Inc., Tokyo, Japan) equipped with a macro lens (Sigma 50 mm f/2.8 EX DG Macro; Sigma Corp., Ronkonkoma, New York). For F1 broods only, we additionally removed and photographed the female that had given birth following the same methodology. Using the digital photos, we used tpsDig2 (Rohlf, 2017) to measure the standard length (SL) of three offspring (or all offspring if brood size ≤3). The average offspring SL served as our estimate of offspring size at birth for a given brood. We additionally used the digital photos to measure the SL of each female after each parturition to include as a potential covariate for F1 broods, as larger females were expected to produce larger broods (e.g., Riesch et al., 2013). Although female body size was unknown for F2 broods (multiple females pooled together), female body size does not differ between predation regimes in the wild or in our common-garden experiment (see Supplementary Material), and we found that female size did not influence offspring size in F1 broods (see “Results”).
To examine age at maturity and juvenile growth rate, we conducted an experiment using 51 F2 broods (Supplementary Table S2). Each brood was photographed the day of birth as described above, raised in a separate 10-L tank within a recirculating system for approximately 61 days (61.18 ± 0.87, mean ± std. dev.), photographed again using the same methods, and then continued to be raised in the same conditions until all fish reached sexual maturity. Because external identification of sexual maturity in poeciliid females is not straightforward (sexual maturation largely occurs internally), but sexual maturity is readily identifiable for males, we only measured the age at maturity for males. Males were determined as sexually mature when their gonopodium had fully completed its entire morphological development (Turner, 1941). We removed males from the tanks when mature to minimize any possible effects of social interactions with adults on age at maturity in males (e.g., Borowsky, 1973; Sohn, 1977a,b; Hughes, 1985; Borowsky, 1987; Magellan and Magurran, 2009). To measure growth rate, we used the digital photos to measure SL of five fish per brood (or all fish if brood size ≤5) at each time point to measure the average body size at birth and after approximately 2 months of rearing. Growth rate was estimated as change in mm SL per day = SL2months – SLbirth/# of days. This 2-month duration captured the vast majority of juvenile growth. No fish initiated sexual differentiation or reached sexual maturity during this period, but males began external sexual differentiation within 2 weeks after this period.
Each day, we fed fish ad libitum as described above, checked for sexual maturity, and confirmed health and responsiveness to feeding for each fish to help ensure they all received adequate food daily. Water conditions followed that described above, with the addition that we recorded water temperature every 10 min throughout the experiment using a HOBO UA-002-64 temperature data logger for potential use as a covariate in statistical analysis (average 2-month water temperature across all broods: 24.90°C ± 0.12). A low level of mortality occurred early in the experiment in five populations (8 fish, 3.8% mortality), and thus effects of mortality or selection should be minimal. Because the experiment was run in two temporal blocks, with the second block starting immediately after the first block reached 2 months of age, we included a random Block term in our statistical analyses.
For fecundity, we first tested for effects of laboratory rearing on size-specific fecundity by comparing F1 brood sizes in our common-garden experiment to wild-caught females from the same eight populations (n = 100, data from Riesch et al., 2013). We conducted a generalized linear model using a Poisson distribution with a log link, with fecundity as the dependent variable, population, rearing environment (wild vs. lab), and their interaction as independent variables, and log10-transformed female SL as a covariate. We then tested whether predation regime or resource availability influenced among-population variation in fecundity of F1 and F2 broods. We conducted a generalized linear mixed-model with a Poisson distribution and log link using the number of offspring per brood as the dependent variable and predation regime, log10-transformed zooplankton density, generation (F1 vs. F2 broods), and their interactions as independent variables. Population was included as a random effect. Because female ID was unknown for F2 broods, we could not include female body size as a covariate. However, we performed an analogous statistical analysis for F1 brood sizes alone that did statistically adjust for any effects of female body size and brood number, and found qualitatively similar results (Supplementary Table S3). Because F1 females were smaller (younger) than F0 females (33.4 mm SL vs. 37.9 mm SL, on average), we expected smaller fecundity in F2 broods compared to F1 broods but were interested in the potential interactions between generation and predation regime or resource availability.
To test for effects of predation regime and resource availability on offspring size at birth, we conducted a general linear mixed-model with log10-transformed average offspring SL at birth as the dependent variable and predation regime, log10-transformed zooplankton density, generation (F1 vs. F2 broods), and their interactions as independent variables. Population was again included as a random effect. Once again, and for the same reason, we could not include female body size as a covariate but analysis of F1 offspring body size at birth that did statistically adjust for any effects of female body size and brood number found qualitatively similar results (and no effects of female body size or brood number; see Supplementary Table S3). Moreover, results were unchanged if we included fecundity as a covariate in the model.
To test for effects of predation regime and resource availability on the frequency with which females deliver broods, we conducted a generalized linear mixed-model with a Poisson distribution and log link using the interbrood interval (number of days between consecutive delivery of broods) as the dependent variable and predation regime, log10-transformed zooplankton density, and their interactions as independent variables. Log10-transformed female SL and brood number served as covariates, with population and female ID treated as random effects.
We tested for effects of predation regime and resource availability on male age at sexual maturity in lab-raised F2s using a generalized linear mixed-model with a Poisson distribution and log link. Age at maturity (# of days) served as the dependent variable, and predation regime, log10-transformed zooplankton density, and their interaction served as independent variables. We included population and brood ID as random effects. We initially included average water temperature as a covariate, but excluded it due lack of influence (P = 0.96).
To test for effects of predation regime and resource availability on juvenile growth rate in lab-raised F2s, we conducted a general linear mixed-model with average growth rate (mm per day) as the dependent variable, and predation regime, log10-transformed zooplankton density, and their interaction as independent variables. Population and block were included as random effects. We further included tank density and average water temperature as covariates, as these influenced average growth rate (see section “Results”).
To provide a multivariate overview of life-history variation among populations, we calculated population means for all 5 traits using values for F2 broods in all cases except interbrood interval (only F1 brood data available), and performed a principal component analysis (PCA) using the correlation matrix. We retained PC axes with eigenvalues greater than 1 for inspection. We plotted relevant PC scores for populations in each predation regime against resource availability to assess overall multivariate patterns of life-history divergence.
Analyses were performed using the R statistical program (R Development Core Team, 2009) with the lme4 and afex packages (for generalized linear mixed models) and the JMP statistical program (v. 14.2, 2018, SAS Institute Inc.; for general linear mixed models and generalized linear models). In all cases, to interpret interaction terms involving predation regime and resource availability, we provided two visual depictions: (1) average trait values for each predation regime at both low and high resource levels (values of 0.2 and 0.75 log10–transformed zooplankton density), and (2) plotted relevant population means within each predation regime against resource availability. To provide an intuitive metric of effect sizes, we present percent differences between groups.
Comparing wild-caught and lab-raised F0 females, we found that the eight populations examined showed strongly consistent size-specific fecundities, with no evidence of differences between the rearing environments in brood sizes (Supplementary Table S4). Thus, the laboratory rearing environment for F0 females did not result in altered size-specific fecundity for any population compared to that observed in the wild.
In our tests of the effects of predation regime and resource availability on fecundity in lab-raised Bahamas mosquitofish, we found clear evidence for a three-way interaction between predation regime, zooplankton density, and generation (Table 1). This finding reflected two main patterns. First, high-predation populations exhibited higher fecundity than low-predation populations within both F1 and F2 broods (Figure 1A; matching predictions). Second, the effects of resource availability depended on the predation regime and generation: a positive association between resource availability and fecundity was evident in most cases (albeit weakly in F1 broods of high-predation females) except for F2 broods of low-predation females (Figure 1B; largely matching predictions). Thus, we found evidence for a role of both predation risk and resource availability in the evolution of fecundity in Bahamas mosquitofish, however the influence of predation risk was stronger and more consistent than resource levels (Supplementary Table S5). On average, high-predation females had 46.2% higher fecundity in F1 broods than low-predation females, statistically controlling for body size, brood number, and resource availability. For F2 broods, high-predation females had a fecundity 37.7% higher on average than low-predation females, statistically controlling for effects of resource availability. Fecundity of F2 broods was smaller than F1 broods, but this likely reflected the smaller body size of females in the second generation.
Table 1. Statistical results examining variation in fecundity and offspring size of F1 and F2 broods of lab-raised Bahamas mosquitofish derived from eight blue holes varying in predation regime and resource availability.
Figure 1. Variation in life-history traits between predation regimes and across varying levels of native resource availability based on eight populations of lab-raised F1 and F2 broods of Bahamas mosquitofish reared under common conditions (estimated marginal means ± SE depicted). We illustrate variation between low-resource (0.2 log10 zooplankton density) and high-resource environments (0.75 log10 zooplankton density) (A,C,E), as well as across the continuous range of resource availability (B,D,F).
Across two generations of lab-raised fish, we found opposite effects of resource availability on offspring size in the different predation regimes (Table 1, “Pred × Res” term). In low-predation populations, offspring size tended to increase in sites with increasing levels of resources (opposite to a priori prediction), but offspring size in high-predation populations strongly decreased with higher resource availability in their native populations (matching a priori prediction) (Figure 1D and Supplementary Table S5). At low resource levels, we found no differences in offspring size between predation regimes, but at moderate to high resource levels, G. hubbsi showed larger offspring size at birth in the absence of predators (Figure 1C and Supplementary Table S5). The larger offspring size in F2 broods at least partially reflects the smaller fecundity in F2 broods.
For interbrood interval, we found evidence for an interaction between predation regime and resource availability, but no effects of female body size or brood number (Table 2). Reproductive frequency was more rapid in populations having higher resource availability (matching a priori prediction), but only within high-predation populations (Figure 1F and Supplementary Table S5). Within low-resource environments, predation risk has not led to any difference in interbrood interval, but at moderate-high resource levels, high-predation populations reproduce more frequently, as predicted (Figure 1E and Supplementary Table S5).
Table 2. Statistical results of a generalized linear mixed-model examining variation in interbrood interval of F1 broods in Bahamas mosquitofish derived from eight populations.
We found that predation regime had no influence on the age at maturity in lab-raised F2 males (contrary to a priori prediction), but resource availability had a strong effect matching our a priori prediction (Table 3). Male Bahamas mosquitofish have apparently evolved to mature more quickly in populations with higher resource levels, regardless of predation risk (Figures 2A,B). This resulted in a 13.9% later age at maturity, on average, in males derived from low-resource compared to high-resource environments (Supplementary Table S5).
Table 3. Statistical results of a generalized linear mixed-model examining variation in male age at maturity of F2 Bahamas mosquitofish derived from eight populations.
Figure 2. Variation in life-history traits between predation regimes and across varying levels of native resource availability based on eight populations of lab-raised F2 Bahamas mosquitofish reared under common conditions (estimated marginal means ± SE depicted). Following Figure 1, we illustrate variation between low-resource (0.2 log10 zooplankton density) and high-resource environments (0.75 log10 zooplankton density) (A,C), as well as across the continuous range of resource availability (B,D).
We observed independent effects of resource availability and predation regime on juvenile growth rate in lab-raised F2 Bahamas mosquitofish (Table 4). Matching our a priori prediction, populations that have evolved in blue holes with higher resource availability showed slower growth rates, regardless of whether they have evolved with or without predators (Figure 2D). At low resource levels, predation risk has not led to divergence in growth rates, but at moderate-high resource levels, populations without predatory fish have evolved higher growth rates than high-predation populations (Figure 2C and Supplementary Table S5). Our covariates revealed higher juvenile growth rates were associated with lower tank densities and higher water temperature.
Table 4. Statistical results of a generalized linear mixed-model examining variation in juvenile growth rate of F2 Bahamas mosquitofish derived from eight populations.
We retained two PC axes in our PCA of population mean life-history trait values. The first axis explained 60% of the among-population variance in life-history traits, capturing variation in four of the five traits, while the second axis explained 24% of the variance and captured variation only in male age at maturity (Supplementary Table S6). PC 1 illustrated that the major gradient in life-history divergence of Bahamas mosquitofish involves predation-regime dependent effects of resource availability (stronger in high-predation populations) that results in strong divergence between predation regimes only within environments having moderate to high resource availability (Figure 3). Positive PC 1 scores are associated with faster juvenile growth rates, larger offspring size, longer interbrood intervals, and lower fecundity (Supplementary Table S6). Because PC 2 largely described variation in male age at maturity (Supplementary Table S6), it essentially re-described variation already captured in Figure 2B.
Figure 3. Variation in PC1, illustrating that low-predation populations inhabiting environments with moderate to high resource availability have evolved a relatively faster juvenile growth rate, larger offspring size, a longer interbrood interval, and lower fecundity than high-predation counterparts.
In this study, we tested a set of a priori predictions of adaptive life-history divergence in response to predation and resource availability. To do so, we took advantage of a natural system where these primary agents of ecological selection vary considerably among populations, but do not covary with one another. Raising eight populations under common laboratory conditions, we uncovered important insights into the nature of evolutionary responses of life-history traits to predation and resource availability. Taken together, our findings strongly suggest that life-history evolution in this post-Pleistocene radiation has largely been shaped by interactions between these two factors. Not only did we reveal clear evidence that both predation and resource levels are important in driving life-history evolution, but the effect of predation regime mostly depended on resource availability, while the effects of resource availability often differed among predation regimes—results observed whether using either univariate, trait-by-trait analyses or multivariate, PCA analyses. While this radiation has garnered attention for the predictability of adaptive phenotypic evolution (Cain et al., 2014; Herron and Freeman, 2014; Reece et al., 2014; Langerhans, 2018), we instead found here that observed evolutionary patterns only met our simple predictions in 2 out of 10 cases (resource effects on male age at maturity and juvenile growth rate). Meanwhile, 7 of our a priori predictions were upheld only in a nuanced manner (e.g., smaller offspring size in high-resource environments, but only in high-predation localities; higher reproductive frequency in high-predation environments, but only in high-resource populations). These results suggest that variation in predation and resource availability can lead to relatively complex evolutionary patterns of life-history divergence, and that accurately predicting these trajectories may require the consideration of interactions among selective agents.
First, we found that high predation risk has generally resulted in the evolution of higher fecundity, smaller offspring size, and more frequent reproduction (shorter interbrood intervals), at least within populations having moderate-high resource levels. These trait changes concur with theoretical expectations and prior empirical evidence for how predator-induced extrinsic mortality, and its subsequent reduction in population density, should drive shifts in these life-history traits (e.g., Reznick and Endler, 1982; Reznick et al., 1990, 2002; Charlesworth, 1994; Gasser et al., 2000; Moore et al., 2016). In environments where predators cause elevated adult mortality rates in prey populations, natural selection should favor maternal investment to produce larger numbers of offspring at a relatively rapid pace, compared to environments with low mortality rates where populations are more limited by density-dependent mechanisms, such as resource competition. Further, larger offspring often have competitive advantages in low-predation environments, whereas smaller offspring can often better elude predators (Brockelman, 1975; Bashey, 2008; Jørgensen et al., 2011; Rollinson and Hutchings, 2013). Because of energy constraints, as well as space constraints in the case of livebearing organisms like G. hubbsi, offspring number and size often show a trade-off, where higher fecundity comes at the cost of smaller offspring size (Smith and Fretwell, 1974; Stearns, 1992; Qualls and Shine, 1995; Roff, 2002). Most prior work on these key life-history traits, including those on Bahamas mosquitofish (Riesch et al., 2013; Riesch et al., 2020) have primarily focused on wild-caught specimens, but only through common-garden studies can we establish evolutionary inferences of life-history divergence in the wild. The life-history shifts observed here may largely reflect genetic evolution across populations, as these life-history patterns occurred in animals raised under common conditions, and we found no effects of laboratory rearing on size-specific fecundity. These results contrast with prior arguments that life-history variation in G. hubbsi largely reflects phenotypic plasticity in response to food availability (Downhower et al., 2009). While comparatively few studies have so far examined how predation or resource levels may influence the evolution of reproductive frequency—which often requires captive rearing of reproductive females through multiple brood cycles—our findings do match those of previous studies which have found greater frequency of reproductive events in high-predation environments (Reznick and Endler, 1982). A larger number of prior studies have examined predation’s role in the evolution of offspring number and size, and our results generally match those previously reported for other livebearing fishes, including P. reticulata (Reznick and Endler, 1982), Brachyrhaphis episcopi (Jennions and Telford, 2002), Brachyrhaphis rhabdophora (Johnson and Belk, 2001), as well as a recent meta-analysis concluding that life-history divergence in response to predation has been consistent and predictable among multiple disparate species of livebearing fishes (Moore et al., 2016). This study adds to the growing evidence for predation’s direct and indirect roles in the evolution of these life-history traits and our findings also point to a previously underappreciated dependence of these effects on resource availability (see below).
The faster juvenile growth rates observed in low-predation populations of Bahamas mosquitofish compared to those evolving in the presence of predators contradict theoretical predictions that high extrinsic mortality rates and low density dependence should favor higher growth rates. Prior work has suggested that faster growth to adulthood should increase fitness in high-mortality environments, and some work in livebearing fishes has found evidence for more rapid growth in high-predation localities (Johnson, 2001; Johnson and Belk, 2001; Reznick et al., 2001; Arendt and Reznick, 2005). However, this research has sometimes observed no evolved differences in growth rates between predatory environments (Gale et al., 2013; Reznick et al., 2019), or found inconsistent patterns of divergence that appear to reflect the influence of resource availability (Arendt and Reznick, 2005), which covaried with predation risk in those systems. Here, we suggest that the more intense resource competition in low-predation populations (low mortality, high density) has placed a greater premium on juvenile growth efficiency, because quickly reaching a larger, more competitive body size is more important in the absence of predators than in their presence. A key difference between prior work and the current study is that we simultaneously considered both predation risk and resource availability—had we ignored the resource levels that populations have historically experienced, we would not have found any differences in juvenile growth rates between predation regimes.
In contrast with theoretical predictions, and with most prior work in similar taxa (e.g., Reznick and Bryga, 1987; Reznick et al., 1990; Reznick et al., 1997; Johnson, 2001), we found no evidence that predation risk has led to evolutionary changes in age at sexual maturity in male Bahamas mosquitofish. However, in previous work predation regime and resource availability are known to covary among populations, making it difficult to discern their relative impacts on changes in this life-history trait. Here we found that resource availability, not predation regime, has strongly influenced male age at maturity in a manner that could potentially explain prior findings of an earlier age at maturity in those high-predation environments that also had higher resource availability than their low-predation counterparts. With strong resource-driven selection, resource levels may constrain the evolution of male age at maturity so that any predator-driven selection has little influence on its evolution. Our findings concur with recent work in Trinidadian guppies where replicate populations introduced from a high-predation source to low-predation environments only evolved a later age at maturity after reaching high densities and reducing the resource levels of their streams (Reznick et al., 2019). Those results suggest that reduced resource levels, not altered mortality rates per se, drive the evolution of age at maturity in guppies, at least in the absence of major predatory fish. Results of our study suggest resource levels, not predation risk, drive the evolution of age at maturity across all study sites in Bahamas mosquitofish. In addition, fish in different predation regimes may experience similar selection for adult male body size owing to factors such as resource acquisition and female mating preferences (Reynolds and Gross, 1992; Rosenthal and Evans, 1998). If so, this could limit the fitness benefits of younger age at maturity in high-mortality environments because an earlier age at maturity typically comes at the cost of a smaller body size—male poeciliids virtually stop growth after sexual maturity (Turner, 1941; Schultz, 1961). Of course, small body size could have advantages in surviving predatory encounters (Langerhans, 2009), although prior work in this system has found no evidence of age/size-specific mortality or differences in adult body size between predation regimes (e.g., Heinen et al., 2013; Riesch et al., 2013, 2020; Langerhans, 2018). Further work on predation’s direct and indirect role in the evolution of age at maturity is required.
While predation risk has clearly influenced the evolutionary trajectories of a diverse set of life-history traits in this system, predation-driven divergence primarily occurred in environments with moderate to high resource availability. But why? Statistically speaking, a key reason that we observed greater divergence between predation regimes within environments having relatively higher resource availability is that the effects of resource levels on several life-history traits differed between predation regimes. Specifically, resource availability had highly consistent and predictable effects on all traits within high-predation environments, but had heterogenous effects within low-predation environments, ranging from strong and predictable (age at maturity, juvenile growth rate) to variable/weak (fecundity, interbrood interval) to opposite of predictions (offspring size). Thus, if we can understand the causes of the effects of resource levels on life histories within each predation regime, then we should uncover the underlying causes of why predator-driven divergence primarily occurred in sites with moderate to high resource availability. There are two patterns to be explained: effects of resource availability within (1) low-predation environments, and (2) high-predation environments.
In low-predation environments, we argue that the observed patterns of resource-driven variation in life histories are consistent with the notion that natural selection for the rapid attainment of a competitively superior large body size is generally stronger in these high-competition scenarios compared to high-predation environments. That is, low-predation populations may exhibit stronger resource limitation than high-predation populations at all levels of resources, with stronger density-dependent selection from resource competition in these predator-free, high-density environments (see Supplementary Material). In the absence of predators, Bahamas mosquitofish have evolved larger offspring in environments with higher resource levels, opposite to theoretical predictions (Pianka, 1970; Brockelman, 1975; Stearns, 1976, 1977). This could reflect the combination of (1) strong selection for larger juvenile body size due to its advantages in resource competition in low-predation environments, (2) the fact that higher resource availability, and thus the ability to rely on higher energy acquisition, allows the evolution of even larger offspring size, and (3) that optimal offspring size increases with decreasing growth rates, as observed in higher-resource environments (Jørgensen et al., 2011). With the evolution of larger offspring size in high-resource environments, this constrains the evolution of fecundity (due to space constraints in livebearing animals) and reproductive frequency (i.e., gestation period, due to developmental time constraints), potentially explaining why these low-predation populations have not evolved higher fecundity or more frequent reproductive events in localities with higher resource availability. Moreover, this selection for rapid attainment of an appropriate body size could additionally help explain why greater resource levels have resulted in earlier age at maturity, as individuals can more quickly reach a target body size under higher resource levels. Finally, this could explain the premium placed on rapid growth efficiency in low-predation populations, where fish showed more rapid juvenile growth than high-predation populations, and also showed elevated growth rates in low-resource environments where selection should strongly favor efficiency of converting energy from food to somatic growth.
In high-predation populations, the strong and predictable effects of resource availability on life-history traits might reflect two different causes. First, it could simply result from the theoretical effects of resource availability on life histories. For example, the earlier age at maturity, more frequent reproduction, and higher fecundity in populations with higher resource availability matches classic life-history predictions based on food levels. However, life-history theory does not necessarily predict smaller offspring size independent of fecundity in high-resource environments (which we observed) because theory focuses more on overall reproductive effort. Similarly, the finding of slower juvenile growth rates in populations with higher resource availability does not match predictions based on resource levels per se, but is consistent with selection on growth efficiency. Further, because optimal offspring size should increase with lower growth rates, these patterns are a bit perplexing. So, simple direct effects of resource availability may partially, but not fully, explain these findings. A second explanation is that higher resource levels somehow reflect stronger predator-driven selection. Under high resource levels, selection arising from extrinsic mortality rates can be exacerbated, where even stronger life-history shifts are favored with the reduction of energetic constraints. Moreover, even in this system where ecosystem productivity (e.g., chlorophyll a, phytoplankton density, zooplankton density) is decoupled from overall predation regime (presence/absence of predatory fish; e.g., Heinen et al., 2013), perhaps within high-predation environments resource availability still covaries with predation intensity. That is, where present, the density of the top predator in these systems (bigmouth sleepers) might increase with increasing levels of overall productivity, and thus exert greater predator-driven mortality or selection on Bahamas mosquitofish within those localities. Remarkably, bigmouth sleeper density does indeed correlate positively with zooplankton density across blue holes, although these variables are independent of G. hubbsi density (see Supplementary Material). This means that multiple factors may cause predator-driven selection to be stronger in environments with higher resource availability.
Altogether, the most likely explanation for the resource-dependent effects of predation regime on life-history divergence is the (1) strong selection for large juvenile body size and a reduction of energetic constraints with higher resource levels within low-predation populations, potentially combined with (2) relatively stronger selection from predator-induced mortality with higher resource availability within high-predation populations. That is, in low-resource environments resource-driven selection can be intense both in the absence and presence of predators, explaining the low levels of life-history divergence observed between those environments in this study. On the other hand, in environments with relatively abundant resources, predator-driven selection could overwhelm energy constraints in high-predation localities, while reduced energetic constraints can allow greater evolutionary responses to strong resource competition in the absence of predators, explaining the strong life-history divergence between predation regimes observed in those environments.
An important caveat regarding the findings here is that we only estimated patterns of evolutionary divergence in life histories under a single common environment of ad libitum food levels and the absence of predators. A more thorough understanding of life-history evolution requires assessments of phenotypes under multiple environmental conditions. Measuring multiple life-history traits across multiple rearing treatments for eight vertebrate populations presents strong logistical challenges—but such an approach can provide important insights into the evolution of phenotypic plasticity and possible population differences in plasticity (e.g., Arendt and Reznick, 2005; Gale et al., 2013). Future work should address this topic.
The extent to which the observed life-history shifts among populations reflect independent trait evolution or joint shifts of correlated traits remains largely unknown. Our multivariate assessment suggests that male age at maturity evolves independently of the rest of the life-history traits examined, but future work is needed to determine whether population differences in the other traits mostly reflect independent responses to direct selection or include substantial effects of indirect selection on correlated traits. Regardless, this study has uncovered intriguing patterns of life-history evolution across populations varying in predation risk and resource availability.
Because of the obvious connection between life-history traits and fitness, a large volume of comparative studies have investigated the roles of particular ecological variables, especially predation and resource availability, in driving population differences in these phenotypes. While informative, phenotype-environment associations derived from field studies can be difficult to interpret when strong covariation among ecological factors exists, and cannot directly address evolutionary variation in traits. Therefore, a contemporary challenge is to unravel the effects of individual selective agents on genetic divergence of key life-history traits (e.g., Reznick et al., 2019; Reznick and Travis, 2019). Our study adds new insights to this literature, suggesting that both predation risk and resource availability simultaneously and interactively influence the genetic evolution of life-history strategies in the wild. This knowledge deepens our understanding of adaptive life-history evolution, and explicitly points to the need of future work to address interactions among selective agents in the evolution of life histories.
The original contributions presented in the study are included in the article/Supplementary Material, and available from the Dryad Digital Repository: https://doi.org/10.5061/dryad.pc866t1nt (Langerhans and Hulthén, 2021).
The animal study was reviewed and approved by the Institutional Animal Care and Use Committee of North Carolina State University (protocols 16-193-O, 19-756-O).
RBL conceived and designed the study with major contributions from KH. RBL, KH, JH, and MJ collected the data. RBL analyzed the data and prepared the figures. RBL and KH coordinated the study and led the writing and revisions, to which all authors contributed. All authors read and approved the final manuscript.
Financial support was provided by the North Carolina State University, the Swedish Research Council (Grant No. 2016-03552), Helge Ax:son Johnsons Stiftelse, Magnus Bergvalls Stiftelse, and the Royal Physiographic Society of Lund.
The authors declare that the research was conducted in the absence of any commercial or financial relationships that could be construed as a potential conflict of interest.
We thank The Bahamas government for permission to conduct the work, Wilfred Johnson for invaluable logistical support in the field, and R. Gallagher, J. Lor, and M. Zimmerman for assistance with live fish care.
The Supplementary Material for this article can be found online at: https://www.frontiersin.org/articles/10.3389/fevo.2021.619277/full#supplementary-material
Abrams, P. A., and Rowe, L. (1996). The effects of predation on the age and size of maturity of prey. Evolution 50, 1052–1061. doi: 10.2307/2410646
Anderson, C. M., and Langerhans, R. B. (2015). Origins of female genital diversity: predation risk and lock-and-key explain rapid divergence during an adaptive radiation. Evolution 69, 2452–2467. doi: 10.1111/evo.12748
Araujo, M. S., Langerhans, R. B., Giery, S. T., and Layman, C. A. (2014). Ecosystem fragmentation drives increased diet variation in an endemic livebearing fish of the Bahamas. Ecol. Evol. 4, 3298–3308. doi: 10.1002/ece3.1140
Arendt, J. D., and Reznick, D. N. (2005). Evolution of juvenile growth rates in female guppies (Poecilia reticulata): predator regime or resource level? Proc. R. Soc. B. 272, 333–337. doi: 10.1098/rspb.2004.2899
Arendt, J. D., and Wilson, D. S. (1999). Countergradient selection for rapid growth in pumpkinseed sunfish: disentangling ecological and evolutionary effects. Ecology 80, 2793–2798.
Ballinger, R. E. (1979). Intraspecific variation in demography and life-history of the lizard, Sceloporus jarrovi, along an altitudinal gradient in southeastern Arizona. Ecology 60, 901–909. doi: 10.2307/1936858
Bashey, F. (2008). Competition as a selective mechanism for larger offspring size in guppies. Oikos 117, 104–113. doi: 10.1111/j.2007.0030-1299.16094.x
Bassar, R. D., Marshall, M. C., Lopez-Sepulcre, A., Zandona, E., Auer, S. K., Travis, J., et al. (2010). Local adaptation in Trinidadian guppies alters ecosystem processes. Proc. Nat. Acad. Sci. U.S.A. 107, 3616–3621. doi: 10.1073/pnas.0908023107
Belk, M. C., Ingley, S. J., and Johnson, J. B. (2020). Life history divergence in livebearing fishes in response to predation: is there a microevolution to macroevolution barrier? Diversity 12:179. doi: 10.3390/d12050179
Berven, K. A. (1982). The genetic-basis of altitudinal variation in the wood frog Rana sylvatica. 1. An experimental-analysis of life-history traits. Evolution 36, 962–983. doi: 10.2307/2408075
Björnerås, C., Skerlep, M., Gollnisch, R., Herzog, S. D., Ugge, G. E., Lee, M., et al. (2020). Inland blue holes of The Bahamas - chemistry and biology in a unique aquatic environment. Fundam. Appl. Limnol. 194, 95–106. doi: 10.1127/fal/2020/1330
Boggs, C. L., and Ross, C. L. (1993). The effect of adult food limitation on life-history traits in Speyeria mormonia (Lepidoptera, Nymphalidae). Ecology 74, 433–441. doi: 10.2307/1939305
Borowsky, R. L. (1973). Social control of adult size in males of Xiphophorus variatus. Nature 245, 332–335. doi: 10.1038/245332a0
Borowsky, R. L. (1987). Agonistic behavior and social inhibition of maturation in fishes of the genus Xiphophorus (Poeciliidae). Copeia 1987, 792–796. doi: 10.2307/1445679
Brockelman, W. Y. (1975). Competition, fitness of offspring, and optimal clutch size. Am. Nat. 109, 677–699. doi: 10.1086/283037
Bronikowski, A. M., Clark, M. E., Rodd, F. H., and Reznick, D. N. (2002). Population-dynamic consequences of predator-induced life history variation in the guppy (Poecilia reticulata). Ecology 83, 2194–2204.
Charlesworth, B. (1994). Evolution in Age-structured Populations. New York, NY: Cambridge University Press.
Conover, D. O., and Schultz, E. T. (1995). Phenotypic similarity and the evolutionary significance of countergradient variation. Trends Ecol. Evol. 10, 248–252. doi: 10.1016/s0169-5347(00)89081-3
Diamond, J. (1986). “Overview: laboratory experiments, field experiments, and natural experiments,” in Community Ecology, eds J. Diamond and T. J. Case (New York, NY: Harper and Row), 3–22.
Dmitriew, C. M. (2011). The evolution of growth trajectories: what limits growth rate? Biol. Rev. 86, 97–116. doi: 10.1111/j.1469-185X.2010.00136.x
Downhower, J. F., Brown, L. P., and Matsui, M. L. (2000). Life history variation in female Gambusia hubbsi. Environ. Biol. Fishes. 59, 415–428. doi: 10.1023/a:1026552527018
Downhower, J. F., Brown, L. P., and Matsui, M. L. (2009). Predation, food limitation, phenotypic plasticity and life-history variation in Gambusia hubbsi. Evolution 63, 557–560. doi: 10.1111/j.1558-5646.2008.00553.x
Fairbanks, R. G. (1989). A 17,000-year glacio-eustatic sea-level record - influence of glacial melting rates on the younger dryas event and deep-ocean circulation. Nature 342, 637–642. doi: 10.1038/342637a0
Flatt, T., and Heyland, A. (2011). Mechanisms of Life History Evolution: The Genetics and Physiology of Life History Traits and Trade-Offs. Oxford: Oxford University Press.
Fowler, A. E., Lor, D. J., Farrell, C. E., Bauman, M. A., Peterson, M. N., and Langerhans, R. B. (2018). Predator loss leads to reduced antipredator behaviours in Bahamas mosquitofish. Evol. Ecol. Res. 19, 387–405.
Gadgil, M., and Bossert, W. H. (1970). Life historical consequences of natural selection. Am. Nat. 104, 1–24. doi: 10.1086/282637
Gale, B. H., Johnson, J. B., Schaalje, G. B., and Belk, M. C. (2013). Effects of predation environment and food availability on somatic growth in the Livebearing Fish Brachyrhaphis rhabdophora (Pisces: Poeciliidae). Ecol. Evol. 3, 326–333. doi: 10.1002/ece3.459
Gasser, M., Kaiser, M., Berrigan, D., and Stearns, S. C. (2000). Life-history correlates of evolution under high and low adult mortality. Evolution 54, 1260–1272. doi: 10.1111/j.0014-3820.2000.tb00559.x
Gluckman, T. L., and Hartney, K. B. (2000). A trophic analysis of mosquitofish, Gambusia hubbsi Breder, inhabiting blue holes on Andros Island, Bahamas. Caribb. J. Sci. 36, 104–111.
Heinen, J. L., Coco, M. W., Marcuard, M. S., White, D. N., Peterson, M. N., Martin, R. A., et al. (2013). Environmental drivers of demographics, habitat use, and behavior during a post-Pleistocene radiation of Bahamas mosquitofish (Gambusia hubbsi). Evol. Ecol. 27, 971–991. doi: 10.1007/s10682-012-9627-6
Heinen-Kay, J. L., and Langerhans, R. B. (2013). Predation-associated divergence of male genital morphology in a livebearing fish. J. Evol. Biol. 26, 2135–2145. doi: 10.1111/jeb.12229
Heinen-Kay, J. L., Morris, K. E., Ryan, N. A., Byerley, S. L., Venezia, R. E., Peterson, M. N., et al. (2015). A trade-off between natural and sexual selection underlies diversification of a sexual signal. Behav. Ecol. 26, 533–542. doi: 10.1093/beheco/aru228
Hughes, A. L. (1985). Seasonal trends in body size of adult male mosquitofish, Gambusia affinis affinis, with evidence for their social control. Environ. Biol. Fishes 14, 251–258. doi: 10.1007/bf00002628
Jennions, M. D., and Telford, S. R. (2002). Life-history phenotypes in populations of Brachyrhaphis episcopi (Poeciliidae) with different predator communities. Oecologia 132, 44–50. doi: 10.1007/s00442-002-0942-4
Jennions, M. D., Wong, B. B. M., Cowling, A., and Donnelly, C. (2006). Life-history phenotypes in a live-bearing fish Brachyrhaphis episcopi living under different predator regimes: seasonal effects? Environ. Biol. Fishes. 76, 211–219. doi: 10.1007/s10641-006-9022-7
Johnson, J. B. (2001). Adaptive life-history evolution in the livebearing fish Brachyrhaphis rhabdophora: genetic basis for parallel divergence in age and size at maturity and a test of predator-induced plasticity. Evolution 55, 1486–1491.
Johnson, J. B., and Belk, M. C. (2001). Predation environment predicts divergent life-history phenotypes among populations of the livebearing fish Brachyrhaphis rhabdophora. Oecologia 126, 142–149. doi: 10.1007/s004420000504
Jørgensen, C., Auer, S. K., and Reznick, D. N. (2011). A model for optimal offspring size in fish, including live-bearing and parental effects. Am. Nat. 177, E119–E135. doi: 10.1086/659622
Lack, D. (1947). The significance of clutch-size. Ibis 89, 302–352. doi: 10.1111/j.1474-919X.1947.tb04155.x
Langerhans, R. B. (2009). Morphology, performance, fitness: functional insight into a post-Pleistocene radiation of mosquitofish. Biol. Lett. 5, 488–491. doi: 10.1098/rsbl.2009.0179
Langerhans, R. B. (2018). Predictability and parallelism of multitrait adaptation. J. Hered. 109, 59–70. doi: 10.1093/jhered/esx043
Langerhans, R. B., and Gifford, M. E. (2009). Divergent selection, not life-history plasticity via food limitation, drives morphological divergence between predator regimes in Gambusia hubbsi. Evolution 63, 561–567. doi: 10.1111/j.1558-5646.2008.00556.x
Langerhans, R. B., Gifford, M. E., and Joseph, E. O. (2007). Ecological speciation in Gambusia fishes. Evolution 61, 2056–2074. doi: 10.1111/j.1558-5646.2007.00171.x
Law, R. (1979). Optimal life histories under age-specific predation. Am. Nat. 114, 399–417. doi: 10.1086/283488
Lindgren, B., and Laurila, A. (2005). Proximate causes of adaptive growth rates: growth efficiency variation among latitudinal populations of Rana temporaria. J. Evol. Biol. 18, 820–828. doi: 10.1111/j.1420-9101.2004.00875.x
Lynch, M. (1989). The life-history consequences of resource depression in Daphnia pulex. Ecology 70, 246–256. doi: 10.2307/1938430
Magellan, K., and Magurran, A. E. (2009). The effect of social environment during ontogeny on life history expression in the guppy Poecilia reticulata. J. Fish Biol. 74, 2329–2337. doi: 10.1111/j.1095-8649.2009.02245.x
Martin, R. A., McGee, M. D., and Langerhans, R. B. (2015). Predicting ecological and phenotypic differentiation in the wild: a case of piscivorous fish in a fishless environment. Biol. J. Linn. Soc. 114, 588–607. doi: 10.1111/bij.12449
Martin, R. A., Riesch, R., Heinen-Kay, J. L., and Langerhans, R. B. (2014). Evolution of male coloration during a post-pleistocene radiation of bahamas mosquitofish (Gambusia hubbsi). Evolution 68, 397–411. doi: 10.1111/evo.12277
Martin, T. E. (1987). Food as a limit on breeding birds: a life-history perspective. Annu. Rev. Ecol. Syst. 18, 453–487. doi: 10.1146/annurev.ecolsys.18.1.453
Martin, T. E. (1995). Avian life-history evolution in relation to nest sites, nest predation, and food. Ecol. Monogr. 65, 101–127. doi: 10.2307/2937160
Michod, R. E. (1979). Evolution of life histories in response to age-specific mortality factors. Am. Nat. 113, 531–550. doi: 10.1086/283411
Moore, M. P., Riesch, R., and Martin, R. A. (2016). The predictability and magnitude of life-history divergence to ecological agents of selection: a meta-analysis in livebearing fishes. Ecol. Lett. 19, 435–442. doi: 10.1111/ele.12576
Partridge, L., and Harvey, P. H. (1988). The ecological context of life-history evolution. Science 241, 1449–1455. doi: 10.1126/science.241.4872.1449
Qualls, C. P., and Shine, R. (1995). Maternal body-volume as a constraint on reproductive output in lizards: evidence from the evolution of viviparity. Oecologia 103, 73–78. doi: 10.1007/bf00328427
R Development Core Team (2009). R: A Language and Environment for Statistical Computing. Vienna: R Foundation for Statistical Computing.
Reece, J. B., Urry, L. A., Cain, M. L., Wasserman, S. A., Minorsky, P. V., Jackson, R. B., et al. (2014). Campbell Biology. San Francisco, CA: Benjamin Cummings.
Reynolds, J. D., and Gross, M. R. (1992). Female mate preference enhances offspring growth and reproduction in a fish. Poecilia reticulata. Proc. Royal Soc. B 250, 57–62. doi: 10.1098/rspb.1992.0130
Reznick, D. (1982). The impact of predation on life-history evolution in Trinidadian guppies: genetic-basis of observed life-history patterns. Evolution 36, 1236–1250. doi: 10.2307/2408156
Reznick, D., Bryant, M. J., and Bashley, F. (2002). r- and K-selection revisited: the role of population regulation in life-history evolution. Ecology 83, 1509–1520.
Reznick, D., and Endler, J. A. (1982). The impact of predation on life-history evolution in Trinidadian guppies (Poecilia reticulata). Evolution 36, 160–177. doi: 10.2307/2407978
Reznick, D. A., Bryga, H., and Endler, J. A. (1990). Experimentally induced life-history evolution in a natural-population. Nature 346, 357–359. doi: 10.1038/346357a0
Reznick, D. N., Bassar, R. D., Handelsman, C. A., Ghalambor, C. K., Arendt, J., Coulson, T., et al. (2019). Eco-evolutionary feedbacks predict the time course of rapid life-history evolution. Am. Nat. 194, 671–692. doi: 10.1086/705380
Reznick, D. N., and Bryga, H. (1987). Life-history evolution in guppies (Poecilia reticulata). I. Phenotypic and genetic changes in an introduction experiment. Evolution 41, 1370–1385. doi: 10.2307/2409101
Reznick, D. N., and Bryga, H. A. (1996). Life-history evolution in guppies (Poecilia reticulata: Poeciliidae). V. Genetic basis of parallelism in life histories. Am. Nat. 147, 339–359. doi: 10.1086/285855
Reznick, D. N., Butler, M. J., and Rodd, H. (2001). Life-history evolution in guppies. VII. The comparative ecology of high- and low-predation environments. Am. Nat. 157, 126–140.
Reznick, D. N., Rodd, F. H., and Cardenas, M. (1996). Life-history evolution in guppies (Poecilia reticulata). 6. Differential mortality as a mechanism for natural selection. Evolution 50, 1651–1660. doi: 10.1111/j.1558-5646.1996.tb03937.x
Reznick, D. N., Shaw, F. H., Rodd, F. H., and Shaw, R. G. (1997). Evaluation of the rate of evolution in natural populations of guppies (Poecilia reticulata). Science 275, 1934–1937. doi: 10.1126/science.275.5308.1934
Reznick, D. N., and Travis, J. (2019). Experimental studies of evolution and eco-evo dynamics in guppies (Poecilia reticulata). Annu. Rev. Ecol. Evol. Syst. 50, 335–354. doi: 10.1146/annurev-ecolsys-110218-024926
Riesch, R., Easter, T., Layman, C. A., and Langerhans, R. B. (2015). Rapid human-induced divergence of life-history strategies in Bahamian livebearing fishes (Family Poeciliidae). J. Anim. Ecol. 84, 1732–1743. doi: 10.1111/1365-2656.12425
Riesch, R., Martin, R. A., and Langerhans, R. B. (2013). Predation’s role in life-history evolution of a livebearing fish and a test of the Trexler-DeAngelis model of maternal provisioning. Am. Nat. 181, 78–93. doi: 10.1086/668597
Riesch, R., Martin, R. A., and Langerhans, R. B. (2020). Multiple traits and multifarious environments: integrated divergence of morphology and life history. Oikos 129, 480–492. doi: 10.1111/oik.06344
Riesch, R., Plath, M., Schlupp, I., Tobler, M., and Langerhans, R. B. (2014). Colonisation of toxic environments drives predictable life-history evolution in livebearing fishes (Poeciliidae). Ecol. Lett. 17, 65–71. doi: 10.1111/ele.12209
Robinson, S. J. W., and Partridge, L. (2001). Temperature and clinal variation in larval growth efficiency in Drosophila melanogaster. J. Evol. Biol. 14, 14–21. doi: 10.1046/j.1420-9101.2001.00259.x
Roff, D. (1992). The Evolution of Life Histories: Theory and Analysis. New York, NY: Chapman and Hall.
Rohlf, F. J. (2017). TpsDig2. Stony Brook, NY: Department of Ecology and Evolution, State University of New York.
Rollinson, N., and Hutchings, J. A. (2013). The relationship between offspring size and fitness: integrating theory and empiricism. Ecology 94, 315–324. doi: 10.1890/2-0552.1
Rosenthal, G. G., and Evans, C. S. (1998). Female preference for swords in Xiphophorus helleri reflects a bias for large apparent size. Proc. Natl. Acad. Sci. U.S.A. 95, 4431–4436. doi: 10.1073/pnas.95.8.4431
Rudman, S. M., Heavyside, J., Rennison, D. J., and Schluter, D. (2016). Piscivore addition causes a trophic cascade within and across ecosystem boundaries. Oikos 125, 1782–1789. doi: 10.1111/oik.03204
Santi, F., Petry, A. C., Plath, M., and Riesch, R. (2020). Phenotypic differentiation in a heterogeneous environment: morphological and life-history responses to ecological gradients in a livebearing fish. J. Zool. 310, 10–23. doi: 10.1111/jzo.12720
Schultz, R. J. (1961). Reproductive mechanism of unisexual and bisexual strains of the viviparous fish Poeciliopsis. Evolution 15, 302–325. doi: 10.2307/2406230
Sha, Y. C., Zhang, H., Lee, M., Björnerås, C., Skerlep, M., Gollnisch, R., et al. (2021). Diel vertical migration of copepods and its environmental drivers in subtropical Bahamian blue holes. Aquat. Ecol. (in press). doi: 10.1007/s10452-020-09807-4
Smith, C. C., and Fretwell, S. D. (1974). Optimal balance between size and number of offspring. Am. Nat. 108, 499–506. doi: 10.1086/282929
Sohn, J. J. (1977a). Consequences of predation and competition upon demography of Gambusia manni (Pisces: Poeciliidae). Copeia 1977, 224–227.
Sohn, J. J. (1977b). Socially induced inhibition of genetically determined maturation in platyfish. Xiphophorus maculatus. Science 195, 199–201. doi: 10.1126/science.831271
Spitze, K. (1991). Chaoborus predation and life-history evolution in Daphnia pulex: temporal pattern of population diversity, fitness, and mean-life history. Evolution 45, 82–92. doi: 10.2307/2409484
Stearns, S. C. (1976). Life-history tactics: a review of the ideas. Q. Rev. Biol. 51, 3–47. doi: 10.1086/409052
Stearns, S. C. (1977). Evolution of life-history traits: critique of theory and a review of data. Annu. Rev. Ecol. Syst. 8, 145–171. doi: 10.1146/annurev.es.08.110177.001045
Stearns, S. C. (1989). Trade-offs in life-history evolution. Funct. Ecol. 3, 259–268. doi: 10.2307/2389364
Stearns, S. C. (2000). Life history evolution: successes, limitations, and prospects. Naturwissenschaften 87, 476–486. doi: 10.1007/s001140050763
Turner, C. L. (1941). Morphogenesis of the gonopodium in Gambusia affinis affinis. J. Morphol. 69, 161–185. doi: 10.1002/jmor.1050690107
Van Noordwijk, A. J., and de Jong, G. (1986). Acquisition and allocation of resources: their influence on variation in life-history tactics. Am. Nat. 128, 137–142. doi: 10.1086/284547
Vanni, M. J., and Lampert, W. (1992). Food quality effects on life-history traits and fitness in the generalist herbivore Daphnia. Oecologia 92, 48–57. doi: 10.1007/bf00317261
Walsh, M. R., DeLong, J. P., Hanley, T. C., and Post, D. M. (2012). A cascade of evolutionary change alters consumer-resource dynamics and ecosystem function. Proc. R. Soc. B. 279, 3184–3192. doi: 10.1098/rspb.2012.0496
Walsh, M. R., and Reznick, D. N. (2008). Interactions between the direct and indirect effects of predators determine life history evolution in a killifish. Proc. Natl. Acad. Sci. U.S.A. 105, 594–599. doi: 10.1073/pnas.0710051105
Walsh, M. R., and Reznick, D. N. (2011). Experimentally induced life-history evolution in a killifish in response to the introduction of guppies. Evolution 65, 1021–1036. doi: 10.1111/j.1558-5646.2010.01188.x
Wathne, I., Enberg, K., Jensen, K. H., and Heino, M. (2020). Rapid life-history evolution in a wild Daphnia pulex population in response to novel size-dependent predation. Evol. Ecol. 34, 257–271. doi: 10.1007/s10682-020-10031-7
Keywords: common-garden experiment, growth rate, life histories, mortality rates, predator-prey, resource availability, divergent natural selection, Poeciliidae
Citation: Hulthén K, Hill JS, Jenkins MR and Langerhans RB (2021) Predation and Resource Availability Interact to Drive Life-History Evolution in an Adaptive Radiation of Livebearing Fish. Front. Ecol. Evol. 9:619277. doi: 10.3389/fevo.2021.619277
Received: 19 October 2020; Accepted: 30 March 2021;
Published: 21 April 2021.
Edited by:
Jerald B. Johnson, Brigham Young University, United StatesReviewed by:
Mark C. Belk, Brigham Young University, United StatesCopyright © 2021 Hulthén, Hill, Jenkins and Langerhans. This is an open-access article distributed under the terms of the Creative Commons Attribution License (CC BY). The use, distribution or reproduction in other forums is permitted, provided the original author(s) and the copyright owner(s) are credited and that the original publication in this journal is cited, in accordance with accepted academic practice. No use, distribution or reproduction is permitted which does not comply with these terms.
*Correspondence: Randall Brian Langerhans, bGFuZ2VyaGFuc0BuY3N1LmVkdQ==
†Present address: Kaj Hulthén, Department of Biology, Aquatic Ecology Unit, Lund University, Lund, Sweden
Disclaimer: All claims expressed in this article are solely those of the authors and do not necessarily represent those of their affiliated organizations, or those of the publisher, the editors and the reviewers. Any product that may be evaluated in this article or claim that may be made by its manufacturer is not guaranteed or endorsed by the publisher.
Research integrity at Frontiers
Learn more about the work of our research integrity team to safeguard the quality of each article we publish.