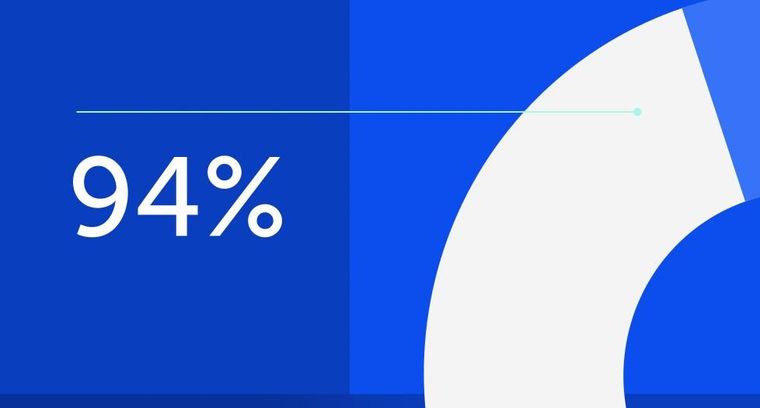
94% of researchers rate our articles as excellent or good
Learn more about the work of our research integrity team to safeguard the quality of each article we publish.
Find out more
ORIGINAL RESEARCH article
Front. Ecol. Evol., 17 May 2021
Sec. Conservation and Restoration Ecology
Volume 9 - 2021 | https://doi.org/10.3389/fevo.2021.612107
This article is part of the Research TopicEnvironmental DNA Innovations for ConservationView all 19 articles
Harmful algae can have profound economic, environmental, and social consequences. As the timing, frequency, and severity of harmful algal blooms (HABs) change alongside global climate, efficient tools to monitor and understand the current ecological context of these taxa are increasingly important. Here we employ environmental DNA metabarcoding to identify patterns in a wide variety of potentially harmful algae and associated ecological communities in the Hood Canal of Puget Sound in Washington State, USA. Tracking trends of occurrence in a series of water samples over a period of 19 months, we find algal sequences from genera with harmful members in a majority of samples, suggesting that these groups are routinely present in local waters. We report patterns in variants of the economically important genus Pseudo-nitzschia (of which some members produce domoic acid; family Bacillariaceae), as well as multiple potentially harmful algal taxa previously unknown or poorly documented in the region, including a cold-water variant from the genus Alexandrium (of which some members produce saxitoxin; family Gonyaulacaceae), two variants from the genus Karlodinium (of which some members produce karlotoxins; family Kareniaceae), and one variant from the parasitic genus Hematodinium (family Syndiniaceae). We then use data on environmental variables and the biological community surrounding each algal taxon to illustrate the ecological context in which they are commonly found. Environmental DNA metabarcoding thus simultaneously (1) alerts us to potential new or cryptic occurrences of algae from harmful genera, (2) expands our knowledge of the co-occurring conditions and species associated with the growth of these organisms in changing marine environments, and (3) suggests a pathway for multispecies monitoring and management moving forward.
Harmful algae and associated blooms create environmental, health, and economic challenges at a global scale, causing mass die-offs in ecosystems from de-oxygenation (Gobler, 2020; Griffith and Gobler, 2020), multiple types of poisoning in humans (Trainer et al., 2013), and significant losses of revenue for the aquaculture industry (Trainer and Yoshida, 2014; Diaz et al., 2019). For these reasons, local, national, and international governing bodies organize and fund monitoring programs to track HABs and identify the conditions that lead to their occurrence (Graneli and Lipiatou, 2002; Trainer, 2002; Lopez et al., 2008; Moestrup et al., 2020). In addition, changing marine environments appear to be causing increases in the duration, frequency, and severity of HABs globally in association with rising temperatures and declining pH (Gattuso et al., 2015a; Gobler et al., 2017; Gobler, 2020).
Hood Canal, a natural glacial fjord within the Puget Sound of Washington, USA, is a useful natural system in which to study the ecology of harmful algae and likely future changes to their patterns of occurrence. Surface temperatures of the region have risen 1.0°C since the 1950s, dissolved oxygen levels are below 5 mg/L in deeper sections of the sound, and pH has dropped by 0.05–0.15 units since pre-industrial era (~1750) (Feely et al., 2010; Busch et al., 2013; Mauger et al., 2015). Warmer temperatures and longer durations of warm conditions will create larger windows of growth for some HABs moving forward (Moore et al., 2011; Mauger et al., 2015; Brosnahan et al., 2020), with ocean acidification potentially exacerbating the impacts of these blooms by further increasing the toxicity and growth of some harmful algal species (Fu et al., 2012; Field et al., 2014; Raven et al., 2020).
Harmful algae fall into four primary categories: diatoms, dinoflagellates, haptophytes, and raphidophytes. Of particular concern locally are select diatoms from the genus Pseudo-nitzschia and dinoflagellates from the genera Alexandrium, Gonyaulax, and Protoceratium, each of which contain species that produce toxins capable of accumulating in shellfish grazers (Shimizu et al., 1975; Satake et al., 1998; Cembella et al., 2000; Trainer et al., 2009, 2016). When consumed by humans, the toxins then cause symptoms ranging from amnesia to paralysis, and can be deadly (Ferrante et al., 2013; Grattan et al., 2016). Additional harmful algae of concern are fish-killing species such as the diatom Chaetoceros concavicornis and the raphidophyte Heterosigma akashiwo (Yang and Albright, 1994; Khan et al., 1997). There is no current ensemble testing protocol for all of these local problematic algae, and both human-mediated transport and warming-related range shifts are likely to introduce additional taxa. For example, there is recent evidence that the toxic dinoflagellate Karenia mikimotoi (family Kareniaceae; described from Japan and also occurring in the North Atlantic) is now present along the west coast of North America, specifically off of Alaska and California (National Centers for Coastal Ocean Science., 2014).
Because the effects of harmful algae are wide-ranging and potentially devastating (Lewitus et al., 2012; Moore et al., 2019), monitoring these organisms and the environmental conditions with which they are associated has long been a public health priority in Puget Sound and in many locations around the world. Efforts to track blooms of harmful algae have historically relied on the work of skilled taxonomists using microscopic visual analysis of cells to identify species (e.g., Yang et al., 2000; Lapworth et al., 2001). More recently, satellite spectrographic data (Tomlinson et al., 2004; Ahn et al., 2006), molecular assays for toxin and cell detection (Pierce and Kirkpatrick, 2001; Groben and Medlin, 2005; Töbe et al., 2010; Murray et al., 2011), and flow cytometry coupled with machine learning (Campbell et al., 2010) have been employed to detect and track HAB taxa.
Adding to the list of technological advances for monitoring are two types of genetic techniques that rely on environmental DNA (eDNA) present in the water to classify and assess the abundance of harmful algae: quantitative Polymerase Chain Reaction (qPCR) and DNA metabarcoding (Al-Tebrineh et al., 2010; Erdner et al., 2010; Antonella and Luca, 2013; Grzebyk et al., 2017; Ruvindy et al., 2018; Jacobs-Palmer et al., 2020). The former method tracks known taxa individually and requires substantial sequence data to design species-specific primers and/or probes; the latter method involves PCR with less-specific primers to generate amplicons from a broad swath of taxa at a common locus. This second method, metabarcoding, allows detection and even quantification of many taxa simultaneously.
Because the specific target organisms need not be chosen a priori, metabarcoding may uncover taxa unexpected in the study region, and in addition, can reveal a cross-section of the biological community surrounding any particular group of interest (Deiner et al., 2017; Taberlet et al., 2018). Previous work has described the ecological context of harmful algae and their blooms using environmental covariates (e.g., Wells et al., 2015; Banerji et al., 2019), as well as assessments of bloom-associated taxa (typically other microorganisms or viruses) (e.g., Buskey, 2008; Loureiro et al., 2011), although the labor required for traditional survey methodology has limited the breadth of contextual taxa included in these studies.
Here, we couple environmental observations with eDNA metabarcoding to examine the ecological and biological context of taxa from dozens of harmful algae-containing genera across 19 months of sampling at 10 locations in Hood Canal. Specifically, we use a single primer set amplifying Cytochrome Oxidase I (COI) to detect focal algal taxa from within the economically and socially relevant genera Alexandrium (dinoflagellate family Gonyaulacaceae), Hematodinium (dinoflagellate family Syndiniaceae), Karlodinium (dinoflagellate family Kareniaceae), and Pseudo-nitzschia (diatom family Bacillariaceae), and to simultaneously survey hundreds of other eukaryotic taxa comprising their biological milieu. We note that our choice of locus and primers (Leray et al., 2013) suitable to amplify and identify a broad swath of eukarya to the level of genus allows us to cast a wide net in our search for organisms co-occurring with these algae but not to resolve individual species; alternative metabarcoding assays will yield different but equally valid views of the community, depending on survey goals. With these sequences in hand, we create exploratory models of the associations of individual algal lineages with key environmental variables, and subsequently improve the predictive value of these models by including co-occurring (both algal and non-algal) lineages as possible indicator taxa. Given the relatively small sample sizes in the current dataset, we see these models as demonstrating the power of community-based genetic monitoring to better understand harmful algal dynamics, rather than as an end-product immediately useful for broad application. In summary, while individual management teams must determine parameters such as the choice of metabarcoding primer set(s), depth of sampling, and model sets to test based on local needs and knowledge, we provide a basic framework for the use of eDNA metabarcoding to improve our understanding and management of harmful algae, both within the Puget Sound and globally.
We sampled seawater for eDNA from 10 sites within Hood Canal, a natural glacial fjord in Puget Sound, Washington, USA, and identified a broad range of potential harmful algal taxa and simultaneously survey the surrounding biological community. Five sampling sites were intertidal, and five were nearby nearshore locations at the approximate center of the fjord.
For each site/date combination at intertidal locations (Salisbury Point County Park (SA), Triton Cove State Park (TR), Lilliwaup Tidelands State Park (LL), Potlatch State Park (PO), and Twanoh State Park (TW); see Figure 1; Supplementary Table 1 for location coordinates), we collected three 1 L samples of water (biological replicates) from immediately below the surface using a bleach-cleaned (10% bleach for 10 min) plastic bottle held at the end of a 1.7 m pole. We sampled intertidal locations every 1–2 months between March 2017 and July 2018 (see Supplementary Table 1 for sampling dates). At these same stations and simultaneous with eDNA sampling, we collected one 120 ml water sample from each site and poisoned it with 0.1 ml of saturated HgCl2 for carbonate chemistry analysis including pH (Dickson et al., 2007). We also collected in situ measurements of temperature and salinity using a handheld multiprobe (Hanna Instruments, USA) and a portable refractometer. We characterized sample carbonate chemistry by measuring Total Alkalinity (TA; open-cell automated titration based on a Dosimat plus (Metrohm AG) as part of a custom system assembled by Andrew Dickson (University of California San Diego) and used in the laboratory of Alex Gagnon at the University of Washington) and Dissolved Inorganic Carbon (DIC; Apollo Instruments, USA; CO2 extraction system with 10% (v/v) phosphoric acid). Both measurements were calibrated and validated with certified reference material from the Scripps Oceanographic Institute. Using DIC and TA, we calculated pH and the remaining carbonate system parameters with the R package “seacarb” (Gattuso et al., 2015b), removing a single outlier sample from the dataset used for environmental modeling (see below) due to an unreasonably low pH value (<7.5).
Figure 1. Intertidal and nearshore sampling locations in Hood Canal, Washington, USA. Site abbreviations are described in the text, and coordinates are given in Supplementary Table 1. Inset map shows the Pacific coast of the continental United States.
For nearshore locations, we sampled a selection of stations (P8, P14, P12, P11, P402) surveyed by the Washington Ocean Acidification Center during triannual cruises (see Figure 1; Supplementary Table 1 for location coordinates). The samples used here were collected in September 2017 (P12 and P402), April 2018 (P8, P12, and P402), and September 2018 (P14, P12, and P11); see Supplementary Table 1. At each station, a CTD was deployed with twelve Niskin bottles, and collected data on temperature, salinity, and pH (Alin et al., 2019a,b,c) in addition to water for a single eDNA sample from immediately below the surface.
For both intertidal and nearshore locations, we filtered 500 mL of each water sample for eDNA with a cellulose acetate filter (47 mm diameter, 0.45 μm pore size), and preserved this filter in Longmire buffer until DNA extraction (Renshaw et al., 2015). Many unmeasured variables influence planktonic communities (e.g., nutrients, sunlight, and wave energy); nevertheless the minimal set of parameters we analyzed here clearly distinguished communities and was adequate for the purposes of assessing temporal and spatial trends. Our purpose was to describe patterns of focal algal taxa over space and time, along with the environmental and ecological contexts in which they occurred, rather than to test any particular mechanism by which these algae might respond to different environmental parameters.
To extract DNA from sample filters, we used a phenol:chloroform:isoamyl alcohol protocol (modified from Renshaw et al., 2015). To maximize extraction efficiency and minimize co-extraction of inhibitors, we incubated filter membranes at 56°C for 30 min before adding 900 μL of phenol:chloroform:isoamyl alcohol and shaking vigorously for 60 s. We conducted two consecutive chloroform washes by centrifuging at 14,000 rpm for 5 min, transferring the aqueous layer to 700 μL chloroform, and shaking vigorously for 60 s. After a third centrifugation, we transferred 500 μL of the aqueous layer to tubes containing 20 μL 5 molar NaCl and 500 μL 100% isopropanol, and froze these at −20°C for approximately 15 h. Finally, we centrifuged samples at 14,000 rpm for 10 min, poured off or pipetted out any remaining liquid, and dried in a vacuum centrifuge at 45°C for 15 min. We resuspended the eluate in 200 μL water, and used 1 μL of diluted DNA extract (between 1:10 and 1:400) as template for PCR.
To identify a wide variety of metazoan taxa, including algal genera with harmful members and their surrounding biological communities using eDNA, we amplified a ~315 base pair segment of the Cytochrome Oxidase I (COI) using primers described in Leray et al. (2013) (although these primers were designed for metazoa, they consistently amplify a broad swath of eukaryotic phyla; (see, e.g., Gallego et al., 2020; Jacobs-Palmer et al., 2020). To distinguish technical from biological variance and to quantify each, we ran and sequenced in triplicate PCR reactions from each of the samples (i.e., individual bottles of water). For multiplex sequencing on an Illumina MiSeq, we followed a two-step PCR protocol (O'Donnell et al., 2016) with redundant 3' and 5' indexing. In the first step, we used a PCR reaction containing 1X HotStar Buffer, 2.5 mM MgCl2, 0.5 mM dNTP, 0.3 μM of each primer, and 0.5 units of HotStar Taq (Qiagen Corp., Valencia, CA, USA) per 20 μL reaction. The PCR protocol for this step consisted of 40 cycles, including an annealing touchdown from 62 to 46°C (−1°C per cycle), followed by 25 cycles at 46°C. In the second step, we added identical 6 base-pair nucleotide tags to both ends of our amplicons, with unique index sequences for each individual PCR reaction. We allowed for no sequencing error in these tags; only sequences with identical tags on both the forward and reverse read-directions survived quality control. This gave us high confidence in assigning amplicons back to individual field samples.
We generated amplicons from extracted tissue with the same replication scheme for positive control kangaroo (genus Macropus), selected because this genus is absent from the sampling sites and common molecular biology reagents, but amplifies well with the primer set (Leray et al., 2013) used in this study. We could therefore use positive control samples to identify possible cross-contamination: reads from other taxa that appear in these samples allow us to estimate and account for the proportion of sequences that are present in the incorrect PCR reaction (see section 2.3 below). We also amplified negative controls (molecular grade water) in triplicate alongside environmental samples and positive controls, and verified by gel electrophoresis and fluorometry that these PCR reactions contained no visible band of DNA (see Kelly et al., 2018 for a discussion of the merits of sequencing positive and not negative controls).
To prepare libraries of replicated, indexed samples and positive controls, we followed manufacturers' protocols (KAPA Biosystems, Wilmington, MA, USA; NEXTflex DNA barcodes; BIOO Scientific, Austin, TX, USA). We then performed sequencing on an Illumina MiSeq (250–300 bp, paired-end) platform in seven different sets of samples: six for the intertidal dataset and one for the nearshore dataset.
We followed updated versions of previously published procedures for bioinformatics, quality control, and decontamination (Kelly et al., 2018). This protocol uses a custom Unix-based script (available at https://github.com/ramongallego/demultiplexer_for_DADA2) calling third-party programs to perform initial quality control on sequence reads from all seven runs combined, demultiplexing sequences to their sample of origin and clustering unique variants into amplicon sequence variants (ASVs) (Martin, 2011; Callahan et al., 2016).
Specifically, to address possible cross-sample contamination (see Schnell et al., 2015), we subtracted the maximum proportional representation of each ASV across all positive control samples (see section 2.2 above) from the respective ASV in field samples. We estimated the probability of ASV occurrence by performing occupancy modeling (Royle and Link, 2006; Lahoz-Monfort et al., 2016). Following Lahoz-Monfort et al. (2016) and using the full Bayesian code for package rjags (Plummer et al., 2016) provided by those authors, we modeled the probability of occupancy (i.e., true presence) for each of the unique sequence variants in our dataset. We treated replicate PCR reactions of each water bottle as independent trials, estimating the true-positive rate of detection (P11), false-positive rate (P10), and occupancy probability (psi, ψ) in a binomial model. We then used these parameters to estimate the overall likelihood of occupancy (true presence) for each ASV; those with low likelihoods (<80%) were deemed unlikely to be truly present in the dataset, and therefore culled. We removed samples whose PCR replicates were highly dissimilar by calculating the Bray-Curtis dissimilarity amongst PCR replicates from the same bottle of water and discarding those with distance to the sample centroid outside a 95% confidence interval. The result was a dataset of 3.98 * 108 reads from 5,275 unique ASVs. Lastly, to collapse variants likely due to PCR error, we converted ASVs to operational taxonomic units (OTUs) by clustering with SWARM (Mahe et al., 2015) with a radius of 1. All bioinformatic and analytical code is included in a GitHub repository (https://github.com/ramongallego/Harmful.Algae.eDNA), including the details of parameter settings in the bioinformatics pipelines used. Sequence and annotation information are included as well, and the former are deposited and publicly available in GenBank Project ID: PRJNA699686; individual FASTQ files: SRR13659629-639 & SRR13685891-947.
We performed the taxonomic identification using a CRUX-generated database for the Leray fragment of the COI gene (see section 2.2 above), querying that database with a Bowtie2 algorithm (as described in Curd et al., 2019). The algorithm classifies the query sequence to the last common ancestor of ambiguously classified sequences. Only matches with a bootstrap support greater than 90% were kept. Here, we assigned taxonomy at the level of genus, rather than species, for two main reasons. First, for some taxa, variation may not be sufficient to distinguish species within a genus, and second, representation of local species in the databases used may not be complete, leading to the mis-assignment of sequences to their nearest represented neighbor. We denoted different lineages within genera using three-character abbreviation derived from the sequence variants themselves. Full sequences for each variant are provided in Supplementary Table 2, and within-genera DNA sequence alignments (Sievers and Higgins, 2014) are provided in Supplementary Table 3. To assess similarity of algal lineages from genera with harmful members, we translated nucleotide sequences with the ExPASy Bioinformatics Resource Portal Translate tool using the mold, protozoan, and coelenterate mitochondrial, mycoplasma/spiroplasma genetic code (Gasteiger et al., 2003), and aligned both nucleotide and amino acid sequences within genera using the Clustal Omega Multiple Sequence Alignment tool (Sievers and Higgins, 2014).
We plotted detection/non-detection for each focal algal taxon across all sampling events from both intertidal and nearshore eDNA collections in our time series between March 2017 and September 2018. We note that while detection almost certainly indicates true presence of a taxon, given our stringent standards for sequence quality and taxonomic assignment, non-detection does not absolutely confirm absence; however, given amplicon counts in the hundreds to tens of thousands for focal sequences, detection/non-detection should at the very least track biomass (see Kelly et al., 2019), and therefore be a useful metric for modeling. To explore the ways in which environmental variables were associated with the detection or non-detection of our focal harmful algal taxa, we compared logistic-regression models using taxon detection as outcome, and combinations of three environmental variables (temperature, pH, and salinity) as predictors. We also fit a variety of models in a Bayesian hierarchical framework, where the slopes of predictors and intercepts could vary by season; for purposes of the models, we designated April through September as being the “warm” season, and other months the “cool” season, based on the environmental data and the approximate congruence of these seasons' boundaries with the fall and spring equinoxes. Rather than mechanically testing all possible combinations of models, we proposed models that were reasonable given the observed patterns of occurrences; in total, this resulted in between five and 17 models per taxon. Given many possible predictor variables, developing a useful model without overfitting can be a challenge. To combat this, we compared models using the widely applicable information criterion (WAIC) (Watanabe, 2010), which makes no assumptions about the shape of the posterior probability distribution and – like information criteria in general – penalizes more complex models. Moreover, WAIC quickly approximates the results of leave-one-out cross-validation (McElreath, 2020) to estimate out-of-sample model performance. Following model selection using WAIC, we reported the in-sample model accuracy for reference.
We further note the difficulty of predicting relatively rare events with logistic regression; even using information criteria such as WAIC does not completely guard against overfitting, and where the number of candidate predictor variables approaches the number of observations of an event (say, the occurrence of an algal taxon), parameter estimates become unreliable (Peduzzi et al., 1996). For our multivariate models, we use a lasso prior to penalize these parameters and shrink them toward zero, making the estimate of their effect more conservative; this approach has shown good success in taming rare-events logistic models (Pavlou et al., 2016; van Smeden et al., 2019). Even so, recognizing the limited sample size with which we are working here, we regard these models as exploratory rather than as definitive descriptions of associations in nature. As more data of this kind becomes available and the number of observations of any given taxon grows, we can develop more robust models.
To determine the species most closely associated with our focal algal taxa, we performed canonical analysis of principal coordinates (CAP) for each focal variant by implementing the capscale function in vegan (Oksanen et al., 2013), which revealed the degree to which other taxa in the surrounding biological communities could be associated with detection of the focal algal taxon. Using this ordination technique avoids the problem of testing each co-occurring taxon for significant associations with our focal algae, thereby removing the need to statistically correct for multiple comparisons. We then used these putative indicator taxa as predictors in a second round of logistic regressions, adding only the single most-strongly associated taxon as a separate term to the best-fit environmental models for each of our focal lineages (above). Such contextual ecological information is useful to the extent that it helps to predict the occurrence of our focal algal taxa.
Environmental DNA metabarcoding of 63 samples from five intertidal and five nearshore locations in Hood Canal, Washington, United States revealed a total of 605 unique operational taxonomic units (OTUs) for which we were able to assign taxonomy to 262 distinct genera. Of these, exactly 100 OTUs were assigned to genera that are known to contain harmful algae (Horner and Postel, 1993; Horner et al., 1997; Trainer et al., 2016; Moestrup et al., 2020). These taxa are members of four main groups—diatoms, dinoflagellates, haptophytes, and raphidophytes—and represent seventeen genera (diatoms: Chaetoceros, Nitzschia, Pseudo-nitzschia; dinoflagellates: Alexandrium, Dinophysis, Gonyaulax, Gymnodinium (Akashiwo), Hematodinium, Heterocapsa, Karlodinium, Prorocentrum, Woloszynskia; haptophytes: Chrysocromulina, Phaeocystis; raphidophytes: Chattonella, Heterosigma, Pseudochattonella; See Supplementary Table 2 for a complete list of taxonomic assignments and COI sequences, as well as total read counts for each variant from an algal genus with harmful members).
Taxa that occur only in small numbers of samples lack sufficient observations to allow robust tests for association with environmental variables. Consequently, we focus hereafter on the OTUs detected in at least 10 percent of samples (minimum 7 occurrences out of 63 samples), an adequate sample size to compare with environmental variables and biological context. This subset of sequences included 191 total variants, 37 of which belong to genera containing harmful algal members (Table 1), and the rest to other organisms in the biological community. These potentially harmful algal variants belong to 12 genera containing differing degrees of sequence variation, with some such as Hematodinium represented by a single DNA and protein sequence, and others such as Nitzschia represented by a much larger number of DNA (10) and amino acid (5) variants. Members of each of the algal genera represented here exhibit varying degrees and types of toxicity or harm, ranging from physical irritation of fish gill tissue to production of toxins dangerous to human health (Table 1; Simonsen and Moestrup, 1997; Lindberg et al., 2005; Stentiford and Shields, 2005; Kotaki et al., 2006; Peperzak and Poelman, 2008; Skjelbred et al., 2011; Place et al., 2012; Trainer et al., 2016; Cho et al., 2017).
Table 1. Potential harmful algal taxa identified by eDNA in at least ten percent of samples from in Hood Canal, WA.
Amplicon sequences from environmental samples cannot be matched directly with phenotypes, by definition, and taxonomic annotations of those sequences depend upon adequate reference material. Acknowledging both the intra-specific variation that exists at the COI locus and the incompleteness of the GenBank reference database for many of these groups, we treat polymorphism within a putative genus as being ambiguous: these variants may be intra-specific, or they may represent distinct evolutionary lineages. For these reasons, we conservatively perform analyses on the sequence variants themselves (denoted with their genus names and a three-character code that abbreviates the hash of the unique nucleotide sequence) rather than making assumptions regarding their status as haplotypes vs. species.
The variants within a few genera containing harmful members are of particular interest, due to the nature of their toxicity (Alexandrium), to their unexpected presence in the study region (Hematodinium and Karlodinium), or to their potential economic impact (Pseudo-nitzschia). For these reasons, we chose to examine aspects of their taxonomy, distribution, and ecology in greater detail. We first examined COI sequences for these taxa from our original metabarcoding effort, noting that both Alexandrium and Karlodinium genera were each represented by two sequence variants, Pseudo-nitzschia by three sequence variants, and Hematodinium by a single sequence variant (Table 1). Amino acid translation revealed that the two Alexandrium OTUs differed by a single amino acid substitution, the two Karlodinium OTUs differed by five substitutions, and although two of the three Pseudo-nitzschia sequences (Pseudonitzschia_4e5 and Pseudonitzschia_d36) were identical in amino acid sequence, they differed from the third (Pseudonitzschia_d40) by two substitutions. The results below focus on these eight sequence variants, which we hereafter refer to as our “focal lineages.”
To identify the seasonal and spatial distributions of taxa from our eight focal lineages, we next visualized their patterns of detection and non-detection in time and space (Figure 2). The variants assigned to Alexandrium, Alexandrium_3fc and Alexandrium_2b2, had completely non-overlapping distributions in space and time, never appearing in the same sampling event. Alexandrium_3fc appeared solely in the warm (April-September) season (25 of 43 warm season samples vs. 0 of 20 cool season samples; p < 0.001) whereas Alexandrium_2b2 appeared primarily in the cool (October-March) season (1 of 43 warm season samples vs. 7 of 20 cool season samples; p < 0.001). In contrast, the single variant assigned to Hematodinium, Hematodinium_449, was not significantly seasonal (9 of 43 warm season samples and 3 of 20 cool season samples; p = 0.742); neither were the two variants assigned to Karlodinium, Karlodinium_8ed and Karlodinium_a27 (Karlodinium_8ed: 14 of 43 warm season samples and 7 of 20 cool season samples, p ~ 1; Karlodinium_a27: 6 of 43 warm season samples and 5 of 20 cool season samples, p = 0.322). One of the three variants assigned to Pseudo-nitzschia occurred significantly more frequently in the warm than in the cool season (Pseudonitzchia_d36: 10 of 43 warm season samples vs. 0 of 20 cool season samples, p = 0.023), while the others did not (Pseudonitzchia_4e5: 8 of 43 warm season samples and 1 of 20 cool season samples, p = 0.244; Pseudonitzchia_d40, 7 of 43 warm season samples and 4 of 20 in cool season samples; p = 0.737).
Figure 2. Spatial and temporal distribution of eight focal algal lineages across time and space. Black circles indicate detection; “x” symbol indicates non-detection at that site/date. Site abbreviations are as in Figure 1 and Supplementary Table 1.
All together, we detected at least one of the eight focal sequence variants in 51 out of 63 sampling events (81%), indicating that these algal taxa are present more often than not in local waters.
The above results suggest both Alexandrium lineages and at least one of the three Pseudo-nitzschia lineages are associated with environmental conditions that change seasonally, while the others are more stochastic in space and time. For each focal taxon, we fit a series of logistic-regression models (see section 2) describing taxon occurrence as a function of sea-surface temperature, pH, and salinity, both with and without a global intercept term (see Supplementary Table 4 for a complete list of models tested, by taxon). A subset of our models was also hierarchical, allowing slopes to vary according to season, and we used WAIC to identify the best-fit models of those tested (Table 2).
Three of these models involve multiple environmental parameters, making them difficult to adequately visualize in two dimensions. Nevertheless, plotting the probability of taxon detection as a function of the single most-influential environmental variable and capturing seasonal variation in slope when models are hierarchical illustrates the degree to which the models do (or do not) explain the observed variance in focal algal taxa (Figure 3).
Figure 3. Best-fit logistic models for eight focal algal lineages. Here, probability of detection is shown as a function of the single most influential environmental variable in the model, along with overall model means (lines) and 50 and 95% credibility intervals (shaded areas). Where two-panel figures are shown for a taxon, the best-fit model included a slope term that varied by season.
Although environmental covariates sea-surface temperature, pH, and salinity are associated with the detection of our eight focal lineages, accuracy of these models varies widely (Table 2). Notably, these covariates alone poorly predict occurrences for all taxa (Table 2, true positive rate). Consequently, we use eDNA metabarcoding data from the communities surrounding our focal lineages for potentially helpful information about the ecology of these algae.
To identify the biological community associated with our focal lineages, we searched for co-occurring taxa using a canonical analysis of principal coordinates (CAP) (Anderson and Willis, 2003). Constraining this multivariate analysis according to the detection of each focal algal variant revealed no striking patterns of association across taxa (Supplementary Tables 5–12), but rather helped to identify individual community members particularly likely or unlikely to co-occur with our focal lineages. These associated community members were those with the strongest deviations from 0 on the CAP1 axis (Table 3), and included lineages of Ditylum, a centric diatom, Prasinoderma, a non-harmful green algae, Saxidomus, a clam, Balanus, a barnacle, and Calanus, a copepod.
Table 3. Terms of the best-fit models combining environmental variables and most closely associated biological taxon for eight focal algal lineages.
For seven of our eight focal lineages, adding the most closely associated predictor taxon improved model fit even after accounting for the additional model complexity (Table 3). Thus, including information about co-occurring organisms alongside baseline environmental covariates substantially increased our ability to predict the detection of these algal taxa within the scope of our sampling. For example, Hematodinium_449 occurs somewhat stochastically in space and time (Figure 2) and is not strongly associated with environmental covariates (Table 2). However, the CAP analysis revealed that a haplotype from the clam genus Saxidomus (likely the species S. giganteus (butter clam), given the sampling location), was routinely found in samples in which Hematodinium also occurred (Table 3). Adding Saxidomus as a term in the previous best-fit model more than doubles the model's overall accuracy (overall accuracy 0.4 vs. 0.87; changes in true and false detection rates shown in Figure 4); adding this biological variable provides a better prediction than the measured environmental variables alone (ΔWAIC = −9.76).
Figure 4. In-sample predictive value of best-fit models for eight focal algal lineages, as measured by accuracy, true-negative rate, and true-positive rate. Red dots indicate values for models combining environmental information with a single associated predictor taxon; blue dots indicate values for models with environmental information alone. Where only a single dot is visible, models produced equivalent results.
Performing the same analysis for each of our focal lineages yields improvements across most taxa, although these are more modest (Figure 4), demonstrating an overall model accuracy above environmental covariates alone for most algal variants. Specifically, adding these candidate indicator taxa improved the true-positive rate of detection for six of the eight models (Karlodinium_8ed and Pseudonitzschia_d36 are the exceptions), which accounted for the increase in overall accuracy across models.
Here, we use genetic monitoring to highlight variants from harmful algae-containing genera within a larger survey of several hundred taxa in intertidal and nearshore marine habitats. Within these focal algal groups, we find several variants from lineages that are unexpected in the study area (Karlodinium, Hematodinium), in addition to an apparently cryptic lineage of Alexandrium, and multiple variants of economically important taxa (e.g., Pseudo-nitzschia). Our time-series sampling indicates different seasonal patterns and attendant associations of sea-surface temperature, pH, and salinity for some of these lineages, but on the whole, models using purely environmental covariates offer poor predictive value. We therefore use a constrained ordination to identify taxa (both algal and non-algal) that commonly occur in association with our focal lineages; adding only a single prospective biological indicator taxon improved most of the predictive models.
eDNA metabarcoding has a number of distinct advantages relative to common techniques currently used to identify harmful algae. First, this technique can reveal a diversity of potentially harmful algal variants present, rather than targeting specific species. In our survey of intertidal and nearshore communities using broad-spectrum mitochondrial COI primers (Leray et al., 2013), the taxa we identified were largely consistent with what we expected a priori, in that we found dozens of variants with excellent overlap from records of known local algal genera containing harmful members (Table 1; Horner and Postel, 1993; Horner et al., 1997; Trainer et al., 2016; Moestrup et al., 2020) such as Alexandrium, Chaetoceros, Heterocapsa, Nitzschia, Phaeocystis, and Pseudo-nitzschia (though it is important to note that confirming the harmful nature of individual variants requires identification to species level and a toxicity assay, which are beyond the scope of this study). While confirming expectations demonstrates the reliability of eDNA metabarcoding for identification, detecting unexpected taxa underscores the ability of this technique to reveal novel lineages, range-shifts, or nascent invasions with potentially profound ecological and economic consequences. For example, the genus Alexandrium, though known to cause paralytic shellfish poisoning in the region (Trainer et al., 2016), was not previously understood to have two distinct seasonal forms (see below). Additionally, members of Karlodinium produce karlotoxins responsible for fish kills in the United States and globally (e.g., Karlodinium veneficum, Place et al., 2012), yet this genus is not reported from Puget Sound in the peer-reviewed scientific literature, despite having been noted by a local cell-based monitoring program (Kolb et al., 2016). Similarly, member(s) of the genus Hematodinium, which have caused massive losses for the tanner crab (Chionoecetes bairdi) and snow crab (C. opilio) fisheries in the United States (Meyers et al., 1987, 1996; Wood et al., 2017) and among other species worldwide (Stentiford and Shields, 2005), have also not previously been reported within Puget Sound in the peer-reviewed scientific literature.
Additionally, eDNA metabarcoding can help to standardize data collection and analysis across studies. Here we employ samples collected with two distinct methods by two groups: intertidal water was gathered on foot and by hand, whereas nearshore water was gathered by boat on the Washington Ocean Acidification Cruise in a more routinized process. Nonetheless, taxa from harmful algae-containing genera identified in nearshore surface samples were all found within the larger intertidal dataset as well, suggesting excellent agreement despite differences in methods and personnel. Such congruence between studies also arises from how the data are analyzed: eDNA sequences from multiple studies can consistently identify cryptic taxa by sequence (e.g., Thomsen and Willerslev, 2015; Uchii et al., 2016), and can undergo identical taxonomic analyses that are not subject to differences in interpretation via morphology (Proschold and Leliaert, 2007). However, we note that the success of eDNA studies rests heavily on the shoulders of expert taxonomists: without their contributions to the identification of specimens with sequences in databases, it is impossible to link a fragment of DNA found in the water to an organism (Manoylov, 2014; Zimmermann et al., 2014). Furthermore, the presence-absence eDNA results we treat here do not reflect an absolute quantification of algal cells in a water sample, and do not reveal the toxicity of those cells (or lack thereof). We thus present our monitoring strategy not as a stand-alone method, but as an important complement to existing practices in the field (e.g., Yang et al., 2000; Groben and Medlin, 2005; Ahn et al., 2006; Campbell et al., 2010; Töbe et al., 2010; Murray et al., 2011).
Our spatial and temporal data indicate that variants from harmful algae-containing genera are constitutively present in the intertidal and nearshore environment, or at the very least are routinely detectable (Table 1; Figure 2). Although challenges in relating sequence counts to absolute organism abundances limit the utility of eDNA metabarcoding for precise measurement of bloom intensity, the ability of eDNA to reveal harmful algal taxa even when cell counts are much lower than bloom conditions can be advantageous. For example, we detect Alexandrium variants year-round in Hood Canal, including winters, when recorded Alexandrium blooms are rare, but when fisheries closures due to presence of paralytic shellfish toxin do exist (Trainer et al., 2003; Moore et al., 2011). When paired with more traditional methods, this tool therefore provides another layer of information regarding the behavior of potentially harmful algae, and at the very least can indicate a temporal and spatial starting place for more time-, labor-, and taxonomic expertise-intensive identification and counting strategies.
Sampling many taxa over time and space additionally facilitates important within- and cross-species comparisons (Figure 2). Here, such comparisons reveal two lineages of Alexandrium with different temporal patterns, and completely non-overlapping distributions. Although taxonomic revisions of the Alexandrium tamarense species complex – and competing classifications of local taxa (Lilly et al., 2007; John et al., 2014) – make it impossible to identify the variants present in our survey without additional information, amino acid differences in the COI sequence of the two lineages alongside temporal distribution information suggest that they may represent distinct species, rather than haplotypes. Regardless, recognizing two distinct Alexandrium lineages with opposite seasonal dynamics is likely to be important to local monitoring and research programs aiming to identify risk of saxitoxin poisoning (e.g., Kolb et al., 2016; Trainer et al., 2016). Previous work on Alexandrium within the Puget Sound found a lower limit for toxic bloom events at 13°C (Nishitani and Chew, 1984), with recent work identifying higher growth of cells above 17-18°C (Bill et al., 2016), and more frequent blooms accompanying warmer air and sea surface temperatures over multiple decades (Moore et al., 2009, 2011). Alexandrium_2b2, detected nearly exclusively in cool season samples, does not match the profile expected given these studies, suggesting either that it does not bloom frequently and/or that it is a recent introduction to the local algal community, whose role is not yet appreciated.
Nucleotide variants from the genus Pseudo-nitzschia identified here are not unexpected; multiple species from this genus have been described locally using both visual (e.g., Trainer et al., 2016) and molecular tools (e.g., Hubbard et al., 2014). In the Western Pacific, clades of a single Pseudo-nitzschia species (P. pungens) with distinct ecological niches and hybrid zones have been documented (Kim et al., 2018); it is interesting to note that here we similarly find two Pseudo-nitzschia variants with distinct nucleotide but identical amino acid sequences at the COI locus. Based on their overlap in time and space, it is likely these represent haplotype lineages that have begun to diverge but are not yet distinct species. Additionally, the specific local antecedents of toxicity in Pseudo-nitzschia are still under study (e.g., Zhu et al., 2017; Trick et al., 2018), with production of domoic acid historically limited to the outer coast of Washington (Trainer et al., 2017), but recently moving into Puget Sound (Trainer et al., 2007). Revealing the diversity and pattern of variants in this genus across time and space by eDNA metabarcoding might thus support efforts to better characterize the causes underlying dangerous and costly Pseudo-nitzschia bloom events.
Quantitative models of each focal lineage with respect to environmental variables (Table 2), motivated by taxon-specific patterns in space and time (Figure 2), yield a synoptic view of the occurrence of many algal taxa in the region – a perspective that is otherwise not easily achievable, though many detailed quantitative models have been built for individual harmful algal species (e.g., Hubbard et al., 2014; Moore et al., 2015). Across variants, we note that values expected with global climate change (higher sea surface temperature) and ocean acidification (lower pH) are typically associated with increases in the occurrence of our focal lineages, such as Alexandrium_2b2, Alexandrium_3fc, Hematodinium_449, and Karlodinium_a27. These results in sum align with other studies of local harmful algal taxa, suggesting future scenarios will involve greater seasonal windows of opportunity for toxic bloom events (e.g., Moore et al., 2011; Trainer et al., 2020).
Overall, however, the quantitative models we have built with environmental variables alone have low accuracy, and universally fail to predict a majority of occurrences for our focal lineages (Table 2). The difficulty of predicting the presence of harmful algal taxa and their blooms based on a limited number of environmental covariates is not atypical; building accurate models of these species' ecology has long been a challenge for the field (Flynn and McGillicuddy, 2018), even when many more environmental covariates are considered. In this study, Hematodinium_449 and Pseudonitzschia_4e5 are extreme representatives of this challenge; our environmental models do not predict their detection correctly even once (Table 2), though they appear in 12 and 9 of 63 samples gathered, respectively. These models are consequently worse than uniformative; they can be actively misleading by inaccurately predicting the absence of harmful species.
Fortunately, eDNA metabarcoding provides an additional layer of information regarding the context of algal variants: the surrounding biological community members (both algal and non-algal). Specifically, the choice of primers amplifying a common molecular marker (mitochondrial COI; Leray et al., 2013) allows us to identify over 600 taxa in total, from more than 250 genera. These data enable us to associate the detection of individual focal variants with a wide range of eukaryotes by CAP (Table 3; Supplementary Tables 5–12). Studies such as this one that examine individual taxa from harmful algae-containing genera within the breadth of their biological communities are rare, but provide an opportunity to improve prediction (e.g., Banerji et al., 2019). Here, we see that adding only the single best predictor taxon to our quantitative models of focal lineages generally improves their utility (Table 3), justifying added model complexity. Although it may appear circular to identify co-occurring species in the dataset and subsequently add them into the model of the same dataset, use of WAIC allows us to assess the value of additional information for future out-of-sample data, generating testable hypotheses for indicator species. As an example, adding the detection of an easily surveyed taxon, a Saxidomus clam, improves the prediction of Hematodinium_449 dramatically, including the true-positive measure essential to management (Figure 4). Likewise, the majority of focal lineages examined here show an improvement in model accuracy with addition of biological information. These improvements are driven by increases in the true positive rate, which are particularly notable for taxa in which the environmental variables surveyed had low predictive power.
In this study, we employ COI primers (Leray et al., 2013) to simultaneously identify dozens of algal variants from genera with harmful members, as well as hundreds of other local taxa comprising the biological context for these algae. The broad nature of eDNA metabarcoding surveys allows us to track both expected and unexpected taxa, and the distribution in time and space of eight focal variants from the genera Alexandrium, Hematodinium, Karlodinium, and Pseudo-nitzschia suggests the constitutive presence of taxa from these groups in the study region, as well the possibility of nascent range shifts, invasions, and/or ongoing evolutionary divergence. Building individual quantitative models for each of eight focal lineages, we find that many variants are likely to become more common under conditions of higher sea surface temperature and ocean acidification, but note that models using environmental covariates alone have low explanatory power. Adding even a single associated member of the biological community, however, improves most models, and in particular boosts the true positive rates useful for prediction of these algal taxa in the field. eDNA metabarcoding is hence an opportunity to reveal potentially harmful algae outside of bloom events and expected ranges, to map phylogenetic complexity underlying HAB dynamics, to interrogate the relevant environmental context in an era of global change, and to improve models of algal prediction with inclusion of the biological milieu.
The fastq files have been deposited in NCBI - The Project ID is PRJNA699686, and individual FASTQ files are SRR13659629 - 639 & SRR13685891-947.
EJ-P, RG, KC, and RK collected the samples and performed the computational analysis. EJ-P, RG, KC, and AK performed the laboratory analysis. EJ-P and RK wrote the manuscript. EJ-P, RG, KC, AK, and RK edited the manuscript. All authors contributed to the article and approved the submitted version.
The Washington Ocean Acidification Center supported this work by purchasing a computer for data analysis. The Washington State Department of Natural Resources supported the salaries of EJP and RPK through Interagency Agreement 93-099546.
The authors declare that the research was conducted in the absence of any commercial or financial relationships that could be construed as a potential conflict of interest.
We thank the Washington Ocean Acidification Center (WOAC) National Oceanic and Atmospheric Administration Harmful Algal Blooms (NOAA HABs) program cruise, and in particular Simone Alin for her provision of samples and environmental data, as well as Terrie Klinger and Jan Newton for their expert comments on the manuscript. We thank our colleagues at the NOAA Northwest Fisheries Science Center, including the Conservation Biology Molecular Genetics Laboratory for their use of the Illumina MiSeq, as well as Linda Rhodes, Stephanie Moore, Vera Trainer, and Nic Adams for their direction of the analysis in its early stages. We thank Micah Horwith and the Washington State Department of Natural Resources for field assistance. Finally, we thank Alex Gagnon, in whose lab we performed carbonate chemistry analysis for the intertidal dataset, and the lab groups in the University of Washington's Center for Environmental Genomics, with whom we share a work space.
The Supplementary Material for this article can be found online at: https://www.frontiersin.org/articles/10.3389/fevo.2021.612107/full#supplementary-material
Supplementary Table 1. Sample location, date, and read count information.
Supplementary Table 2. Taxonomic assignments and COI sequences with total read counts for each OTU within genera containing harmful algal species.
Supplementary Table 3. Within-genera COI sequence alignments for each genus containing harmful algal species (produced with Clustal Omega).
Supplementary Table 4. Complete list of logistic-regression models tested describing focal algal taxon occurrence as a function of sea-surface temperature, pH, and salinity.
Supplementary Table 5. Top 10 taxa associated with Alexandrium_2b2 by CAP, with association strength and direction.
Supplementary Table 6. Top 10 taxa associated with Alexandrium_3fc by CAP, with association strength and direction.
Supplementary Table 7. Top 10 taxa associated with Hematodinium_449 by CAP, with association strength and direction.
Supplementary Table 8. Top 10 taxa associated with Karlodinium_8ed by CAP, with association strength and direction.
Supplementary Table 9. Top 10 taxa associated with Karlodinium_a27 by CAP, with association strength and direction.
Supplementary Table 10. Top 10 taxa associated with Pseudonitzschia_4e5 by CAP, with association strength and direction.
Supplementary Table 11. Top 10 taxa associated with Pseudonitzschia_d36 by CAP, with association strength and direction.
Supplementary Table 12. Top 10 taxa associated with Pseudonitzschia_d40 by CAP, with association strength and direction.
Ahn, Y-H., Shanmugam, P., Ryu, J-H., and Jeong, J-C. (2006). Satellite detection of harmful algal bloom occurrences in Korean waters. Harmful Algae 5, 213–231. doi: 10.1016/j.hal.2005.07.007
Alin, S. R., Newton, J., Greeley, D., Curry, B., Herndon, J., Ostendorf, M. L., et al. (2019a). Dissolved Inorganic Carbon (DIC), Total Alkalinity (TA), Temperature, Salinity, Oxygen, Nutrient, and CTD Data Collected From Discrete Profile Measurements During Puget Sound Cruise CAB1079 (EXPOCODE 33CB20170911) on R/V Clifford A. Barnes from 2017-09-11 to 2017-09-15 (NCEI Accession 0206674). NOAA National Centers for Environmental Information.
Alin, S. R., Newton, J., Greeley, D., Curry, B., Herndon, J., Ostendorf, M. L., et al. (2019b). Dissolved Inorganic Carbon (DIC), Total Alkalinity (TA), Temperature, Salinity, Oxygen, Nutrient, and CTD Data Collected from Discrete Profile Measurements During Puget Sound Cruise RC001 (EXPOCODE 33IY20180407) on R/V Rachel Carson from 2018-04-07 to 2018-04-11 (NCEI Accession 0206802). NOAA National Centers for Environmental Information.
Alin, S. R., Newton, J., Greeley, D., Curry, B., Herndon, J., Ostendorf, M. L., et al. (2019c). Dissolved Inorganic Carbon (DIC), Total Alkalinity (TA), Temperature, Salinity, Oxygen, Nutrient, and CTD Data Collected from Discrete Profile Measurements During Puget Sound Cruise RC007 (EXPOCODE 33IY20180911) on R/V Rachel Carson from 2018-09-11 to 2018-09-15 (NCEI Accession 0206804). NOAA National Centers for Environmental Information.
Al-Tebrineh, J., Mihali, T. K., Pomati, F., and Neilan, B. A. (2010). Detection of saxitoxin-producing Cyanobacteria and Anabaena circinalis in environmental water blooms by quantitative PCR. Appl. Environ. Microbiol. 76, 7836–7842. doi: 10.1128/AEM.00174-10
Anderson, M. J., and Willis, T. J. (2003). Canonical analysis of principal coordinates: a useful method of constrained ordination for ecology. Ecology 84, 511–525. doi: 10.1890/0012-9658(2003)084[0511:CAOPCA]2.0.CO;2
Antonella, P., and Luca, G. (2013). The quantitative real-time PCR applications in the monitoring of marine harmful algal bloom (HAB) species. Environ. Sci. Pollut. Res. 20, 6851–6862. doi: 10.1007/s11356-012-1377-z
Banerji, A., Bagley, M. J., Shoemaker, J. A., Tettenhorst, D. R., Nietch, C. T., Allen, H. J., et al. (2019). Evaluating putative ecological drivers of microcystin spatiotemporal dynamics using metabarcoding and environmental data. Harmful Algae 86, 84–95. doi: 10.1016/j.hal.2019.05.004
Bill, B. D., Moore, S. K., Hay, L. R., Anderson, D. M., and Trainer, V. L. (2016). Effects of temperature and salinity on the growth of Alexandrium (Dinophyceae) isolates from the Salish Sea. J. Phycol. 52, 230–238. doi: 10.1111/jpy.12386
Brosnahan, M. L., Fischer, A. D., Lopez, C. B., Moore, S. K., and Anderson, D. M. (2020). Cyst-forming dinoflagellates in a warming climate. Harmful Algae 91:101728. doi: 10.1016/j.hal.2019.101728
Busch, D. S., Harvey, C. J., and McElhany, P. (2013). Potential impacts of ocean acidification on the Puget Sound food web. ICES J. Mar. Sci. 70, 823–833. doi: 10.2307/4451315
Buskey, E. J. (2008). How does eutrophication affect the role of grazers in harmful algal bloom dynamics? Harmful Algae 8, 152–157. doi: 10.1016/j.hal.2008.08.009
Callahan, B. J., McMurdie, P. J., Rosen, M. J., Han, A. W., Johnson, A. J. A., and Holmes, S. P. (2016). DADA2: high-resolution sample inference from Illumina amplicon data. Nat. Methods 13:581. doi: 10.1038/nmeth.3869
Campbell, L., Olson, R. J., Sosik, H. M., Abraham, A., Henrichs, D. W., Hyatt, C. J., et al. (2010). First harmful Dinophysis (Dinophyceae, Dinophysales) bloom in the U.S. is revealed by automated imaging flow cytometry. J. Phycol. 46, 66–75. doi: 10.1111/j.1529-8817.2009.00791.x
Cembella, A. D., Lewis, N. I., and Quilliam, M. A. (2000). The marine dinoflagellate Alexandrium ostenfeldii (Dinophyceae) as the causative organism of spirolide shellfish toxins. Phycologia 39, 67–74. doi: 10.2216/i0031-8884-39-1-67.1
Cho, K., Kasaoka, T., Ueno, M., Basti, L., Yamasaki, Y., Kim, D., et al. (2017). Haemolytic activity and reactive oxygen species production of four harmful algal bloom species. Eur. J. Phycol. 52, 311–319. doi: 10.1080/09670262.2017.1286525
Curd, E. E., Gold, Z., Kandlikar, G. S., Gomer, J., Ogden, M., O'Connell, T., et al. (2019). Anacapa Toolkit: an environmental DNA toolkit for processing multilocus metabarcode datasets. Methods Ecol. Evol. 10, 1469–1475. doi: 10.1111/2041-210X.13214
Deiner, K., Bik, H. M., Mochler, E., Seymour, M., Lacoursiere-Roussel, A., Altermatt, F., et al. (2017). Environmental DNA metabarcoding: transforming how we survey animal and plant communities. Mol. Ecol. 26, 5872–5895. doi: 10.1111/mec.14350
Diaz, P. A., Alvarez, A., Varela, D., Perez-Santos, I., Diaz, M., Molinet, C., et al. (2019). Impacts of harmful algal blooms on the aquaculture industry: Chile as a case study. Perspect. Phycol. 6, 39–50. doi: 10.1127/pip/2019/0081
Dickson, A. G., Sabine, C. L., and Christian, J. R. (2007). Guide to Best Practices for Ocean Co2 Measurements. North Pacific Marine Science Organization.
Erdner, D. L., Percy, L., Keafer, B., Lewis, J., and Anderson, D. M. (2010). A quantitative real-time PCR assay for the identification and enumeration of Alexandrium cysts in marine sediments. Deep Sea Res. Top. Stud. Oceanogr. 57, 279–287. doi: 10.1016/j.dsr2.2009.09.006
Feely, R. A., Alin, S. R., Newton, J., Sabine, C. L., Warner, M., Devol, A., et al. (2010). The combined effects of ocean acidification, mixing, and respiration on pH and carbonate saturation in an urbanized estuary. Estuar. Coast. Shelf Sci. 88, 442–449. doi: 10.1016/j.ecss.2010.05.004
Ferrante, M., Conti, G. O., Fiore, M., Rapisarda, V., and Ledda, C. (2013). Harmful algal blooms in the Mediterranean sea: effects on human health. EuroMediterranean Biomed. J. 8, 25–34. doi: 10.3269/1970-5492.2013.8.6
Field, C. B., Barros, V. R., Mastrandrea, M. D., Mach, K. J., Abdrabo, M. A.-K., Adger, W. N., et al. (2014). Summary for Policy Makers: Working Group 11 Contribution to the Fifth Assessment Report of the Intergovernmental Panel on Climate Change. Intergovernmental panel on Climate Change.
Flynn, K. J., and McGillicuddy, D. J. (2018). Modeling marine harmful algal blooms: Current status and future prospects, in Harmful Algal Blooms: A Compendium Desk Reference, eds S. E. Shumway, J. M. Burkholder, and S. L. Morton (New York, NY: Wiley Science Publishers), 115–134.
Fu, F. X., Tatters, A. O., and Hutchins, D. A. (2012). Global change and the future of harmful algal blooms in the ocean. Mar. Ecol. Prog. Ser. 470, 207–233. doi: 10.3354/meps10047
Gallego, R., Jacobs-Palmer, E., Cribari, K., and Kelly, R. P. (2020). Environmental DNA metabarcoding reveals winners and losers of global change in coastal waters. Proc. R. Soc. B 287:20202424. doi: 10.1098/rspb.2020.2424
Gasteiger, E., Gattiker, A., Hoogland, C., Ivanyi, I., Appel, R. D., and Bairoch, A. (2003). ExPASy: The proteomics server for in-depth protein knowledge and analysis. Nucleic Acids Res. 31, 3784–3788. doi: 10.1093/nar/gkg563
Gattuso, J.-P., Epitalon, J.-M., Lavigne, H., Orr, J., Gentili, B., Hofmann, A., et al. (2015b). seacarb: Seawater Carbonate Chemistry. R package version.
Gattuso, J.-P., Magnan, A., Bille, R., Cheung, W. W. L., Howes, E. L., Joos, F., et al. (2015a). Contrasting futures for ocean and society from different anthropogenic CO2 emissions scenarios. Science 349:aac4722. doi: 10.1126/science.aac4722
Gobler, C. J. (2020). Climate change and harmful algal blooms: insights and perspective. Harmful Algae 91:101731. doi: 10.1016/j.hal.2019.101731
Gobler, C. J., Doherty, O. M., Hattenrath-Lehmann, T. K., Griffith, A. W., Kang, Y., and Litaker, R. W. (2017). Ocean warming since 1982 has expanded the niche of toxic algal blooms in the North Atlantic and North Pacific oceans. Proc. Natl. Acad. Sci. U.S.A. 114, 4975–4980. doi: 10.1073/pnas.1619575114
Graneli, E., and Lipiatou, E. (2002). EUROHAB, Part B, Research and Infrastructural Needs, National European and International Programmes. Luxembourg: Office for Official Publications of the European Communities.
Grattan, L. M., Holobaugh, S., and Morris, J. G. Jr (2016). Harmful algal blooms and public health. Harmful Algae 57, 2–8. doi: 10.1016/j.hal.2016.05.003
Griffith, A. W., and Gobler, C. J. (2020). Harmful algal blooms: a climate change co-stressor in marine and freshwater ecosystems. Harmful Algae 91:101590. doi: 10.1016/j.hal.2019.03.008
Groben, R., and Medlin, L. (2005). In situ hybridization of phytoplankton using fluorescently labeled rRNA probes, in Methods in Enzymology, eds E. A. Zimmer and E. H. Roalson (Elsevier), 299–310.
Grzebyk, D., Audic, S., Lasserre, B., Abadie, E., Vargas, C., and Bec, B. (2017). Insights into the harmful algal flora in northwestern Mediterranean coastal lagoons revealed by pyrosequencing metabarcodes of the 28S rRNA gene. Harmful Algae 68, 1–16. doi: 10.1016/j.hal.2017.06.003
Horner, R. A., Garrison, D. L., and Plumley, F. G. (1997). Harmful algal blooms and red tide problems on the US west coast. Limnol. Oceanogr. 42, 1076–1088. doi: 10.4319/lo.1997.42.5_part_2.1076
Horner, R. A., and Postel, J. R. (1993). Toxic diatoms in western Washington waters (US west coast), in Twelfth International Diatom Symposium (Renesse: Springer), 197–205.
Hubbard, K. A., Olson, C. E., and Armbrust, E. V. (2014). Molecular characterization of Pseudo-nitzschia community structure and species ecology in a hydrographically complex estuarine system (Puget Sound, Washington, USA). Mar. Ecol. Prog. Ser. 507, 39–55. doi: 10.3354/meps10820
Jacobs-Palmer, E., Gallego, R., Ramon-Laca, A., Kunselman, E., Cribari, K., Horwith, M., et al. (2020). A halo of reduced dinoflagellate abundances in and around eelgrass beds. PeerJ 8:e8869. doi: 10.7717/peerj.8869
John, U., Litaker, R. W., Montresor, M., Murray, S., Brosnahan, M. L., and Anderson, D. M. (2014). Formal revision of the Alexandrium tamarense species complex (Dinophyceae) taxonomy: the introduction of five species with emphasis on molecular-based (rDNA) classification. Protist 165, 779–804. doi: 10.1016/j.protis.2014.10.001
Kelly, R. P., Gallego, R., and Jacobs-Palmer, E. (2018). The effect of tides on nearshore environmental DNA. PeerJ 2018. doi: 10.7717/peerj.4521
Kelly, R. P., Shelton, A. O., and Gallego, R. (2019). Understanding PCR processes to draw meaningful conclusions from environmental DNA studies. Sci. Rep. 9:12133. doi: 10.1038/s41598-019-48546-x
Khan, S., Arakawa, O., and Onoue, Y. (1997). Neurotoxins in a toxic red tide of Heterosigma akashiwo (Raphidophyceae) in Kagoshima Bay, Japan. Aquaculture Res. 28, 9–14. doi: 10.1046/j.1365-2109.1997.t01-1-00823.x
Kim, J. H., Wang, P., Park, B. S., Kim, J.-H., Patidar, S. K., and Han, M.-S. (2018). Revealing the distinct habitat ranges and hybrid zone of genetic sub-populations within Pseudo-nitzschia pungens (Bacillariophyceae) in the West Pacific area. Harmful algae 73, 72–83. doi: 10.1016/j.hal.2018.01.007
Kolb, A., Hannach, G., and Swanson, L. (2016). Marine Phytoplankton Monitoring Program Sampling and Analysis Plan. Seattle, WA: King County Department o fNatural Resources; Parks.
Kotaki, Y., Furio, E. F., Bajarias, F. A., Satake, M., Lundholm, N., Koike, K, et al. (2006). New stage of the study on domoic acid-producing diatoms–a finding of Nitzschia navis-varingica that produces domoic acid derivatives as major toxin components. Coast. Mar. Sci. 30, 116–120.
Lahoz-Monfort, J. J., Guillera-Arroita, G., and Tingley, R. (2016). Statistical approaches to account for false-positive errors in environmental DNA samples. Mol. Ecol. Resour. 16, 673–685. doi: 10.1111/1755-0998.12486
Lapworth, C., Hallegraeff, G. M. J., and Ajani, P. A. (2001). Identification of domoic-acid-producing Pseudo-nitzschia species in Australian waters in Harmful Algal Blooms 2000 Proceedings of the Ninth International Conference on Harmful Algal Blooms (Paris), 38–41.
Leray, M., Yang, J. Y., Meyer, C. P., Mills, S. C., Agudelo, N., Ranwez, V., et al. (2013). A new versatile primer set targeting a short fragment of the mitochondrial COI region for metabarcoding metazoan diversity: application for characterizing coral reef fish gut contents. Front. Zool. 10:34. doi: 10.1186/1742-9994-10-34
Lewitus, A. J., Horner, R. A., Caron, D. A., Garcia-Mendoza, E., Hickey, B. M., Hunter, M., et al. (2012). Harmful algal blooms along the North American west coast region: History, trends, causes, and impacts. Harmful Algae 19, 133–159. doi: 10.1016/j.hal.2012.06.009
Lilly, E. L., Halanych, K. M., and Anderson, D. M. (2007). Species boundaries and global biogeography of the Alexandrium tamarense complex (Dinophyceae) 1. J. Phycol. 43, 1329–1338. doi: 10.1111/j.1529-8817.2007.00420.x
Lindberg, K., Moestrup, E., and Daugbjerg, N. (2005). Studies on woloszynskioid dinoflagellates i: Woloszynskia coronata re-examined using light and electron microscopy and partial lsu rDNA sequences, with description of tovellia gen. nov. And jadwigia gen. nov. (Tovelliaceae fam. Nov.). Phycologia 44, 416–440.
Lopez, C. B., Jewett, E. B., Dortch, Q., Walton, B. T., and Hudnell, H. K. (2008). Scientific Assessment of Freshwater Harmful Algal Blooms. Interagency Working Group on Harmful Algal Blooms, Hypoxia, and Human Health.
Loureiro, S., Rene, A., Garces, E., Camp, J., and Vaque, D. (2011). Harmful algal blooms (HABs), dissolved organic matter (DOM), and planktonic microbial community dynamics at a near-shore and a harbour station influenced by upwelling (SW Iberian Peninsula). J. Sea Res. 65, 401–413. doi: 10.1016/j.seares.2011.03.0040
Mahe, F., Rognes, T., Quince, C., Vargas, C., and Dunthorn, M. (2015). Swarm v2: highly-scalable and high-resolution amplicon clustering. PeerJ 3:e1420. doi: 10.7717/peerj.1420
Manoylov, K. M. (2014). Taxonomic identification of algae (morphological and molecular): species concepts, methodologies, and their implications for ecological bioassessment. J. Phycol. 50, 409–424. doi: 10.1111/jpy.12183
Martin, M. (2011). Cutadapt removes adapter sequences from high-throughput sequencing reads. EMBnet J. 17:10. doi: 10.14806/ej.17.1.200
Mauger, G., Casola, J., Morgan, H., Strauch, R., Jones, B., Curry, B., et al. (2015). State of Knowledge: Climate Change in Puget Sound. NOAA and the University of Washington Climate Impacts Group.
McElreath, R. (2020). Statistical Rethinking: A Bayesian Course With Examples in R and Stan. Boca Raton, FL: CRC Press.
Meyers, T., Morado, J., Sparks, A., Bishop, G., Pearson, T., Urban, D., et al. (1996). Distribution of bitter crab syndrome in Tanner crabs (Chionoecetes bairdi, C. opilio) from the Gulf of Alaska and the Bering Sea. Dis. Aquat. Organ. 26, 221–227.
Meyers, T. R., Koeneman, T. M., Botelho, C., and Short, S. (1987). Bitter crab disease: a fatal dinoflagellate infection and marketing problem for Alaskan Tanner crabs Chionoecetes bairdi. Dis. Aquat. Organ. 3, 195–216.
Moestrup, E., Akselmann-Cardella, R., Churro, C., Fraga, S., Hoppenrath, M., Iwataki, M., et al. (2020). IOC-UNESCO taxonomic reference list of harmful micro algae. doi: 10.14284/362
Moore, S. K., Cline, M. R., Blair, K., Klinger, T., Varney, A., and Norman, K. (2019). An index of fisheries closures due to harmful algal blooms and a framework for identifying vulnerable fishing communities on the US West Coast. Marine Policy 110:103543. doi: 10.1016/j.marpol.2019.103543
Moore, S. K., Johnstone, J. A., Banas, N. S., and Salathe, E. P. Jr. (2015). Present-day and future climate pathways affecting Alexandrium blooms in Puget Sound, WA, USA. Harmful Algae 48, 1–11. doi: 10.1016/j.hal.2015.06.008
Moore, S. K., Mantua, N. J., Hickey, B. M., and Trainer, V. L. (2009). Recent trends in paralytic shellfish toxins in Puget Sound, relationships to climate, and capacity for prediction of toxic events. Harmful Algae 8, 463–477. doi: 10.1016/j.hal.2008.10.003
Moore, S. K., Mantua, N. J., and Salathe, E. P. Jr (2011). Past trends and future scenarios for environmental conditions favoring the accumulation of paralytic shellfish toxins in puget sound shellfish. Harmful Algae 10, 521–529. doi: 10.1016/j.hal.2011.04.004
Murray, S. A., Wiese, M., Stuken, A., Brett, S., Kellmann, R., Hallegraeff, G., et al. (2011). sxtA-based quantitative molecular assay to identify saxitoxin-producing harmful algal blooms in marine waters. Appl. Environ. Microbiol. 77, 7050–7057. doi: 10.1128/AEM.05308-11
National Centers for Coastal Ocean Science. (2014). Working With State to Document First Occurrence of Harmful Karenia mikimotoi Algae in Alaskan Waters.
Nishitani, L., and Chew, K. K. (1984). Recent developments in paralytic shellfish poisoning research. Aquaculture 39, 317–329.
O'Donnell, J. L., Kelly, R. P., Lowell, N. C., and Port, J. A. (2016). Indexed PCR primers induce template-specific bias in large-scale DNA sequencing studies. PLoS ONE 11:e0148698. doi: 10.1371/journal.pone.0148698
Oksanen, J., Blanchet, F. G., Kindt, R., Legendre, P., Minchin, P. R., O'hara, R. B., et al. (2013). Package “vegan”. Community Ecology Package, version 2.
Pavlou, M., Ambler, G., Seaman, S., De Iorio, M., and Omar, R. Z. (2016). Review and evaluation of penalised regression methods for risk prediction in low-dimensional data with few events. Stat. Med. 35, 1159–1177. doi: 10.1002/sim.6782
Peduzzi, P., Concato, J., Kemper, E., Holford, TR., and Feinstein, A. R. (1996). A simulation study of the number of events per variable in logistic regression analysis. J. Clin. Epidemiol. 49, 1373–1379.
Peperzak, L., and Poelman, M. (2008). Mass mussel mortality in the Netherlands after a bloom of Phaeocystis globosa (prymnesiophyceae). J. Sea Res. 60, 220–222. doi: 10.1016/j.seares.2008.06.001
Pierce, R. H., and Kirkpatrick, G. J. (2001). Innovative techniques for harmful algal toxin analysis. Environ. Toxicol. Chem. Int. J. 20, 107–114. doi: 10.1897/1551-5028(2001)020<0107:itfhat>2.0.co;2
Place, A. R., Bowers, H. A., Bachvaroff, T. R., Adolf, J. E., Deeds, J. R., and Sheng, J. (2012). Karlodinium veneficum—The little dinoflagellate with a big bite. Harmful Algae 14, 179–195. doi: 10.1016/J.HAL.2011.10.021
Proschold, T., and Leliaert, F. (2007). Systematics of the green algae: Conflict of classic and modern approaches. Syst. Assoc. Spcl. Vol. 75:123. doi: 10.1201/9780849379901.ch7
Raven, J. A., Gobler, C. J., and Hansen, P. J. (2020). Dynamic Co2 and pH levels in coastal, estuarine, and inland waters: theoretical and observed effects on harmful algal blooms. Harmful Algae 91:101594. doi: 10.1016/j.hal.2019.03.012
Renshaw, M. A., Olds, B. P., Jerde, C. L., McVeigh, M. M., and Lodge, D. M. (2015). The room temperature preservation of filtered environmental DNA samples and assimilation into a phenol–chloroform–isoamyl alcohol DNA extraction. Mol. Ecol. Resour. 15, 168–176. doi: 10.1111/1755-0998.12281
Royle, J. A., and Link, W. A. (2006). Generalized site occupancy models allowing for false positive and false negative errors. Ecology 87, 835–841. doi: 10.1890/0012-9658(2006)87[835:gsomaf]2.0.co;2
Ruvindy, R., Bolch, C. J., MacKenzie, L., Smith, K. F., and Murray, S. A. (2018). qPCR assays for the detection and quantification of multiple paralytic shellfish toxin-producing species of Alexandrium. Front. Microbiol. 9:3153. doi: 10.3389/fmicb.2018.03153
Satake, M., MacKenzie, L., and Yasumoto, T. (1998). Identification of Protoceratium reticulatum as the biogenetic origin of yessotoxin. Nat. Toxins 5, 164–167.
Schnell, I. B., Bohmann, K., and Gilbert, M. T. P. (2015). Tag jumps illuminated–reducing sequence-to-sample misidentifications in metabarcoding studies. Mol. Ecol. Resour. 15, 1289–1303. doi: 10.1111/1755-0998.12402
Shimizu, Y., Alam, M., Oshima, Y., and Fallon, W. E. (1975). Presence of four toxins in red tide infested clams and cultured Gonyaulax tamarensis cells. Biochem. Biophys. Res. Commun. 66, 731–737. doi: 10.1016/0006-291X(75)90571-9
Sievers, F., and Higgins, D. G. (2014). Clustal Omega, accurate alignment of very large numbers of sequences, in: Multiple Sequence Alignment Methods, ed D. J. Russell (New York, NY: Springer), (New York, NY: Springer), 105–116.
Simonsen, S., and Moestrup, E. (1997). Toxicity tests in eight species of Chrysochromulina (Haptophyta). Can. J. Bot. 75, 129–136.
Skjelbred, B., Horsberg, T. E., Tollefsen, K. E., Andersen, T., and Edvardsen, B. (2011). Toxicity of the ichthyotoxic marine flagellate Pseudochattonella (Dictyochophyceae, Heterokonta) assessed by six bioassays. Harmful Algae 10, 144–154. doi: 10.1016/j.hal.2010.08.007
Stentiford, G. D., and Shields, J. D. (2005). A review of the parasitic dinoflagellates Hematodinium species and Hematodinium-like infections in marine crustaceans. Dis. Aquat. Organ. 66, 47–70. doi: 10.3354/dao066047
Taberlet, P., Bonin, A., Coissac, E., and Zinger, L. (2018). Environmental DNA: For Biodiversity Research and Monitoring. Oxford University Press.
Thomsen, P. F., and Willerslev, E. (2015). Environmental DNA–An emerging tool in conservation for monitoring past and present biodiversity. Biol. Conserv. 183, 4–18. doi: 10.1016/j.biocon.2014.11.019
Töbe, K., Kulis, D., Anderson, D. M., Gladstone, M., and Medlin, L. K. (2010). Detecting intact algal cells with whole cell hybridisation assays. Microsc. Mol. Methods Quant. Phytoplankton Anal.55.
Tomlinson, M. C., Stumpf, R. P., Ransibrahmanakul, V., Truby, E. W., Kirkpatrick, G. J., Pederson, B. A., et al. (2004). Evaluation of the use of SeaWiFS imagery for detecting Karenia brevis harmful algal blooms in the eastern Gulf of Mexico. Remote Sens. Environ. 91, 293–303. doi: 10.1016/j.rse.2004.02.014
Trainer, V., King, T., Bill, B., and Runyan, J. (2016). SoundToxins Manual: Puget Sound Harmful Algal Bloom Monitoring Program. NOAA/NMFS/NWFSC Marine Biotoxins Program for Washington Sea Grant.
Trainer, V., Moore, L., Bill, B., Adams, N., Harrington, N., Borchert, J., da Silva, D., et al. (2013). Diarrhetic shellfish toxins and other lipophilic toxins of human health concern in Washington State. Mar. Drugs 11, 1815–1835. doi: 10.3390/md11061815
Trainer, V. L. (2002). Harmful algal blooms on the US west coast, in Harmful Algal Blooms in the PICES Region of the North Pacific, eds F. J. R Taylor and V. L. Trainer (Sidney, BC), 89–118.
Trainer, V. L., Adams, N. G., Bill, B. D., Ayres, D. L., Forster, Z. R., Odell, A., et al. (2017). Pseudo-nitzschia blooms in the northeastern Pacific Ocean. PICES Sci. Rep 53, 37–48.
Trainer, V. L., Cochlan, W. P., Erickson, A., Bill, B. D., Cox, F. H., Borchert, J. A., et al. (2007). Recent domoic acid closures of shellfish harvest areas in Washington state inland waterways. Harmful Algae 6, 449–459. doi: 10.1016/j.hal.2006.12.001
Trainer, V. L., Eberhart, B.-T. L., Wekell, J. C., Adams, N. G., Hanson, L., Cox, F., et al. (2003). Paralytic shellfish toxins in Puget Sound, Washington state. J. Shellfish Res. 22, 213–223.
Trainer, V. L., Moore, S. K., Hallegraeff, G., Kudela, R. M., Clement, A., Mardones, J. I., and Cochlan, W. P. (2020). Pelagic harmful algal blooms and climate change: lessons from nature's experiments with extremes. Harmful Algae 91:101591. doi: 10.1016/j.hal.2019.03.009
Trainer, V. L., Wells, M. L., Cochlan, W. P., Trick, C. G., Bill, B. D., Batgh, K. A., et al. (2009). An ecological study of a massive bloom of toxigenic Pseudo-nitzschia cuspidata off the Washington State coast. Limnol. Oceanogr. 54, 1461–1474. doi: 10.4319/lo.2009.54.5.1461
Trainer, V. L., and Yoshida, T. (2014). Proceedings of the Workshop on Economic Impacts of Harmful Algal Blooms on Fisheries and Aquaculture. North Pacific Marine Science Organization (PICES).
Trick, C. G., Trainer, V. L., Cochlan, W. P., Wells, M. L., and Beall, B. (2018). The successional formation and release of domoic acid in a Pseudo-nitzschia bloom in the Juan de Fuca Eddy: a drifter study. Harmful Algae 79, 105–114. doi: 10.1016/j.hal.2018.08.007
Uchii, K., Doi, H., and Minamoto, T. (2016). A novel environmental dna approach to quantify the cryptic invasion of non-native genotypes. Mol. Ecol. Resour. 16, 415–422. doi: 10.1111/1755-0998.12460
van Smeden, M., Moons, KG., Groot, J. A., Collins, G. S., Altman, D. G., Eijkemans, M. J., et al. (2019). Sample size for binary logistic prediction models: beyond events per variable criteria. Stat. Methods Med. Res. 28, 2455–2474. doi: 10.1177/0962280218784726
Watanabe, S. (2010). Asymptotic equivalence of Bayes cross validation and widely applicable information criterion in singular learning theory. J. Mach. Learn. Res. 11, 3571–3594.
Wells, M. L., Trainer, V. L., Smayda, T. J., Karlson, B. S. O., Trick, C. G., Kudela, R. M., et al. (2015). Harmful algal blooms and climate change: learning from the past and present to forecast the future. Harmful Algae 49, 68–93. doi: 10.1016/j.hal.2015.07.009
Wood, K., Stratman, J. P., Messmer, A., and Palof, K. J. (2017). Annual Management Report for the 2016/2017 Southeast Alaska and Yakutat Tanner Crab Fisheries. Alaska Department of Fish; Game, Divisions of Sport Fish; Commercial Fisheries.
Yang, C. Z., and Albright, L. J. (1994). The harmful phytoplankter Chaetoceros concavicornis causes high mortalities and leucopenia in chinook salmon (Oncorhynchus tshawytscha) and coho salmon (O. kisutch). Can. J. Fish. Aquat. Sci. 51, 2493–2500.
Yang, Z. B., Takayama, H., Matsuoka, K., and Hodgkiss, I. J. (2000). Karenia digitata sp. nov. (Gymnodiniales, Dinophyceae), a new harmful algal bloom species from the coastal waters of west Japan and Hong Kong. Phycologia 39, 463–470. doi: 10.2216/i0031-8884-39-6-463.1
Zhu, Z., Qu, P., Fu, F., Tennenbaum, N., Tatters, A. O., and Hutchins, D. A. (2017). Understanding the blob bloom: Warming increases toxicity and abundance of the harmful bloom diatom Pseudo-nitzschia in California coastal waters. Harmful Algae 67, 36–43. doi: 10.1016/j.hal.2017.06.004
Keywords: harmful algal bloom, environmental DNA, ecological model, metabarcoding, Alexandrium, Pseudo-nitzchia, Hematodinium, Karlodinium
Citation: Jacobs-Palmer E, Gallego R, Cribari K, Keller AG and Kelly RP (2021) Environmental DNA Metabarcoding for Simultaneous Monitoring and Ecological Assessment of Many Harmful Algae. Front. Ecol. Evol. 9:612107. doi: 10.3389/fevo.2021.612107
Received: 30 October 2020; Accepted: 12 April 2021;
Published: 17 May 2021.
Edited by:
Hiroki Yamanaka, Ryukoku University, JapanReviewed by:
Nansheng Chen, Institute of Oceanology, Chinese Academy of Sciences (CAS), ChinaCopyright © 2021 Jacobs-Palmer, Gallego, Cribari, Keller and Kelly. This is an open-access article distributed under the terms of the Creative Commons Attribution License (CC BY). The use, distribution or reproduction in other forums is permitted, provided the original author(s) and the copyright owner(s) are credited and that the original publication in this journal is cited, in accordance with accepted academic practice. No use, distribution or reproduction is permitted which does not comply with these terms.
*Correspondence: Ryan P. Kelly, cnBrZWxseUB1dy5lZHU=
Disclaimer: All claims expressed in this article are solely those of the authors and do not necessarily represent those of their affiliated organizations, or those of the publisher, the editors and the reviewers. Any product that may be evaluated in this article or claim that may be made by its manufacturer is not guaranteed or endorsed by the publisher.
Research integrity at Frontiers
Learn more about the work of our research integrity team to safeguard the quality of each article we publish.