- 1Pacific Northwest National Laboratory, Richland, WA, United States
- 2School of Biological Sciences, Washington State University, Richland, WA, United States
- 3Department of Environmental Systems Science, Institute of Integrative Biology, ETH Zürich, Zürich, Switzerland
- 4Institute of Arctic and Alpine Research, University of Colorado Boulder, Boulder, CO, United States
- 5School of Geography, Earth and Environmental Sciences, University of Birmingham, Birmingham, United Kingdom
- 6LEHNA- Laboratoire d’Ecologie des Hydrosystemes Naturels et Anthropises, University of Lyon, Lyon, France
- 7Department of Biology, East Carolina University, Greenville, NC, United States
- 8Department of Microbiology and Molecular Genetics, Michigan State University, East Lansing, MI, United States
- 9Texas A&M Agrilife, Texas A&M University, College Station, TX, United States
- 10Department of Ecology and Evolutionary Biology and Center for Research on Learning & Teaching, University of Michigan, Ann Arbor, MI, United States
- 11INRAE, UMR 1391 ISPA, Bordeaux Sciences Agro, Villenave d’Ornon, France
- 12Department of Biology, Wake Forest University, Winston-Salem, NC, United States
- 13Instituto de Investigaciones en Biodiversidad y Medioambiente – Consejo Nacional de Investigaciones Científicas y Técnicas, Buenos Aires, Argentina
- 14Institute for Systems Biology, Seattle, WA, United States
- 15Lawrence Berkeley National Laboratory, Berkeley, CA, United States
- 16Department of Biostatistics, Harvard School of Public Health, Boston, MA, United States
- 17Department of Geosciences and Geography, University of Helsinki, Helsinki, Finland
- 18Mote Marine Laboratory and Aquarium, Sarasota, FL, United States
- 19Desertification Research Centre, Spanish National Research Council (CIDE-CSIC), Valencia, Spain
- 20Department of Forest Resources, University of Minnesota, St. Paul, MN, United States
- 21Department of Biology, Duke University, Durham, NC, United States
- 22Oak Ridge National Laboratory, Oak Ridge, TN, United States
- 23Emory University, Atlanta, GA, United States
- 24Institut Agro, Univ Angers INRAE, IRHS, SFR QUASAV, Angers, France
- 25Institute of Geography and Spatial Organization, Polish Academy of Sciences, Warsaw, Poland
- 26Department of Geography, University of Wisconsin–Madison, Madison, WI, United States
- 27University of British Columbia, Vancouver, BC, Canada
- 28School of Biological Sciences, University of Canterbury, Christchurch, New Zealand
- 29Indiana University, Bloomington, IN, United States
- 30Kansas Geological Survey, University of Kansas, Lawrence, KS, United States
Disturbances fundamentally alter ecosystem functions, yet predicting their impacts remains a key scientific challenge. While the study of disturbances is ubiquitous across many ecological disciplines, there is no agreed-upon, cross-disciplinary foundation for discussing or quantifying the complexity of disturbances, and no consistent terminology or methodologies exist. This inconsistency presents an increasingly urgent challenge due to accelerating global change and the threat of interacting disturbances that can destabilize ecosystem responses. By harvesting the expertise of an interdisciplinary cohort of contributors spanning 42 institutions across 15 countries, we identified an essential limitation in disturbance ecology: the word ‘disturbance’ is used interchangeably to refer to both the events that cause, and the consequences of, ecological change, despite fundamental distinctions between the two meanings. In response, we developed a generalizable framework of ecosystem disturbances, providing a well-defined lexicon for understanding disturbances across perspectives and scales. The framework results from ideas that resonate across multiple scientific disciplines and provides a baseline standard to compare disturbances across fields. This framework can be supplemented by discipline-specific variables to provide maximum benefit to both inter- and intra-disciplinary research. To support future syntheses and meta-analyses of disturbance research, we also encourage researchers to be explicit in how they define disturbance drivers and impacts, and we recommend minimum reporting standards that are applicable regardless of scale. Finally, we discuss the primary factors we considered when developing a baseline framework and propose four future directions to advance our interdisciplinary understanding of disturbances and their social-ecological impacts: integrating across ecological scales, understanding disturbance interactions, establishing baselines and trajectories, and developing process-based models and ecological forecasting initiatives. Our experience through this process motivates us to encourage the wider scientific community to continue to explore new approaches for leveraging Open Science principles in generating creative and multidisciplinary ideas.
Introduction
Disturbances related to human activities, including both abrupt and long-term impacts of climate change, are predicted to continually intensify in the coming century (IPCC, 2019). For instance, wildfires have ravaged global landscapes over the last two decades, impacting human lives, crops, and biodiversity — highlighted by recent outbreaks in Australia, Brazil, California, and British Columbia (Cleetus and Mulik, 2014; Boer et al., 2020; Tedim et al., 2020). Twenty of the hottest years in history have occurred in the past 22 years (World Meteorological Organization, 2018), and extreme events like heat waves are projected to increase in frequency by more than an order of magnitude as climate change continues (IPCC, 2019). As well, long-term changes in temperature and moisture can lead to changes in ecosystem structure (e.g., species composition) and function (e.g., biogeochemical cycles). Such disturbances can radically alter trajectories of ecosystem dynamics, and importantly, they occur within a broader ecological context that can generate interactions among ecosystem processes and lead to unpredictable ecosystem responses (Paine et al., 1998; Calderón et al., 2018; Zscheischler et al., 2018; Knelman et al., 2019).
Despite increases in the frequency (e.g., return interval), duration (e.g., pulse vs. press events), and scale (e.g., severity, intensity, magnitude, extent, etc.) of disturbance events, predicting their onset, characteristics, and consequences remains difficult (Battisti et al., 2016). This is in part because of differences in conceptual models, scales of investigation, and language used across scientific disciplines (Salafsky et al., 2008; Battisti et al., 2016). Disturbances occur through space and time with different frequencies (number of occurrences per unit time), intensities (magnitude of the disturbance), and extents (spatiotemporal domain affected) (Sousa, 1979, 1984; Grimm and Wissel, 1997; Paine et al., 1998; Miller et al., 2011). Additionally, natural versus anthropogenic disturbances differ in their underlying causes and socio-ecological implications, yet are commonly discussed with the same terminology (Salafsky et al., 2008). Inconsistencies in disturbance frameworks have long been noted by the ecological community (Rykiel, 1985; Pickett et al., 1989; Poff, 1992; Peters et al., 2011; Gaiser et al., 2020), and the struggle to derive a common framework for understanding and predicting disturbances continues in modern literature (Smith, 2011b; Borics et al., 2013; Hobday et al., 2016; Jentsch and White, 2019). Disturbances are often inferred to be synonymous with pulse events, perturbations, threats, and/or stressors, and thus, the concept of disturbance encapsulates phenomena across a range of spatial and temporal conditions. However, these terms should not be used interchangeably, and have subtle and meaningful differences in specific fields of inquiry (Rykiel, 1985; Lake, 2000; Borics et al., 2013; Jentsch and White, 2019; Keeley and Pausas, 2019; Kemppinen et al., 2019). For instance, Slette et al. (2019) argued that the plethora of literature on drought is generally based on loose descriptions rather than explicit definitions or quantitative metrics of drought, while Hobday et al. (2018) noted that other disturbances lack even basic quantitative categorization or naming schemes. Because of these inconsistencies, attempts to compare disturbances across types and ecosystems have resulted in few outcomes that can be generalized across fields (Peters et al., 2011). Definitions of disturbance originally focused on ‘discrete events’ that alter an ecosystem or its function (Pickett and White, 2013), but recent definition frameworks have incorporated aspects of drivers of disturbance, disturbance regimes, and scale of disturbances in time and space (Turner, 2010; Peters et al., 2011; Gaiser et al., 2020). Collectively, these shortcomings point to the need for an interdisciplinary understanding of disturbances.
Differences in how disturbances are studied across scientific disciplines are driven in part by their spatial and temporal heterogeneity and in part by differences in typical scales of investigation. While some disturbance events have relatively discrete temporal and spatial boundaries (e.g., wildfires, hurricanes, earthquakes), others are diffuse or overlap in time and space (e.g., ocean acidification, overgrazing, nutrient loadings, droughts) (Godfrey and Peterson, 2017). This makes it difficult to identify which events depart from ‘normal’ ecosystem processes, especially within the broader context of ongoing environmental change (Duncan et al., 2010; Mishra and Singh, 2010; Slette et al., 2019). Finally, because the impacts of disturbances are contingent on historical events and local socio-economic conditions (Duncan et al., 2010; Seidl et al., 2016; Dietze et al., 2018; Słowiński et al., 2019), a single type of disturbance can be perceived in different ways depending on the environment and species of interest. Dynamic hydrology, for example, is fundamental to floodplain wetland systems, which are adapted and shaped by flooding events, but flooding events are typically considered disturbances in upland contexts.
To facilitate interdisciplinary investigation and understanding of disturbances, we need a generalizable framework with which to talk about such events. Ideally, this framework would be able to manage the heterogeneity inherent in disturbances while providing consistency in how disturbances are defined and studied. Such a foundation would consist of shared goals and be built upon commonly agreed-upon terms and metrics. We propose a generalizable disturbance framework that builds upon earlier frameworks (Grimm and Wissel, 1997; Peters et al., 2011; Newman et al., 2020) by emphasizing drivers (also called ‘driving forces’ or ‘indirect threats’) vs. impacts of disturbance, acknowledging multiple system responses to disturbance, and enabling cross-ecosystem comparisons. We expand on existing frameworks by recognizing multiple scales of interactions over space and time and acknowledging disturbance legacies that may alter the vulnerability of an ecosystem (e.g., risk of organismal, elemental, or other losses) to other drivers. The framework presented here provides a baseline of commonalities for interdisciplinary collaborations and communication; and it can be supplemented with discipline-specific variables for more in-depth investigation into particular aspects of disturbances.
To address this challenge, we used an open call on social media to assemble a cross-disciplinary team of 46 collaborators at different career stages across 38 institutions in 15 countries with a diverse suite of scientific specialties (Graham and Krause, 2020; Graham and Smith, 2020). We used our collective expertise to propose a pathway toward a new conceptual model of ecological disturbances that integrates contributions across disparate disciplines. The range of disciplines and scale of research represented by contributors include microbial or plant ecology at the gene, population, community, and ecosystem level; biogeochemistry across freshwater, marine, and terrestrial ecosystems; ecology focused at soil pore scale all the way to organisms at the landscape and watershed scales; environmental social science; and conservation biology. The project featured a flexible, collaborative, and iterative writing process (using Google docs), freely open authorship opportunities advertised via Twitter that recruited many early career scientists including graduate students and postdoctoral researchers. It was coordinated by a small international leadership team and helped break down barriers between researchers at various career stages, institutions, and disciplines. By proposing a generalizable framework for disturbances, we strive toward a common currency to compare ecological drivers and responses across conditions and systems.
We start by describing the framework, followed by an overview of factors that resonated across disciplines such as system stability theory and spatiotemporal considerations. The final sections propose minimum reporting standards for widely implementing this common framework and cross-disciplinary approaches for addressing areas of need. This emergent framework is intended to help synthesize ideas among historically disparate events and disciplines, more rigorous tracking of events across space and time, and new ways of understanding disturbance impacts between fields. In turn, resulting knowledge can influence the ways in which humans manage ecosystems and their responses to disturbances by aiding managers in identifying slow-developing disturbances as they occur, referencing disturbances against historical events by comparing quantitative characteristics, and being able to better predict ecological impacts to define conservation strategies.
A Generalizable Framework
One essential limitation in our understanding and managing of disturbances is that the word ‘disturbance’ is used interchangeably to describe two distinct processes—events that cause ecological change and consequences of extreme events—that are both termed disturbances despite fundamental distinctions between the two types of processes. Some researchers define disturbances by properties that describe an event (e.g., type, duration, frequency, intensity) (Hobday et al., 2016, 2018), while others characterize disturbances by their impacts (e.g., ecological, or societal damages) (Smith, 2011a). Others try to integrate disturbance drivers and impacts by describing disturbances as a chain of events. For instance, the Driver-Pressure-State-Impact-Response (DPSIR), provides a structure in which a series of causal links from ‘driving forces’ (economic sectors, human activities) through ‘pressures’ (emissions, waste) to ‘states’ (physical, chemical and biological) and ‘impacts’ on ecosystems, human health and functions, leading to political ‘responses’ (prioritization, target setting, indicators) (Pirrone et al., 2005). Furthermore, many definitions of disturbances solely consider short-term events that represent rapid deviations from a biotic or abiotic background state without regard to historical processes (Jentsch and White, 2019). Finally, solely defining disturbances by their impact size directly conflicts with the idea of ecological resistance and the vast amount of theory developed for this phenomenon. If we were to define a disturbance based only on its impact, highly resistant ecosystems would never be disturbed regardless of the prevalence of extreme events.
Disturbance theory lacks a one-size-fits-all approach due to the spatial, temporal, and cross-disciplinary complexities in studying disturbances. A key challenge in the development of such an approach is that individual disturbances operate within a broader context of historical events that cumulatively alter disturbance magnitude and impact. For instance, Ryo et al. (2019) describe the temporal dependency of interacting disturbances in terms of ‘nestedness,’ wherein the complexity of interactions is dependent on the relative closeness of the events in question. Within this framework, a single event is a subset of multiple disturbances within a continuous trajectory. Importantly, there are carryover effects within trajectories in which disturbance impacts can accumulate and/or alter the internal mechanisms affecting responses through time, even for parts of an ecosystem not affected by earlier disturbances (Nowicki et al., 2019). Therefore, driver-response relationships are dependent on both short- and long-term histories. While Ryo’s framework only considers temporal aspects of disturbances (Ryo et al., 2019), it highlights the need for a fluid framework to provide a common foundation for studying disturbances across scales and lines of inquiry—one that can adjust for variation between systems and research goals.
We propose a robust and tangible framework of disturbance that is applicable regardless of the line of inquiry and/or spatiotemporal scale of investigation (Figure 1). Specifically, we define a disturbance event as the occurrence of a driver whereby a force, either biotic or abiotic, generates a deviation from the local, prevailing background conditions (i.e., a disturbance driver). In the proposed framework, a driver is characterized by its magnitude of deviation from an environmental baseline (low to high deviation describes weak to strong drivers). In contrast, a disturbance impact represents the social-ecological consequences of a driver relative to a scale-dependent baseline state. A key attribute of the framework is that drivers and impacts are both relative to a baseline state. Baselines are determined based on abiotic conditions that are relevant to the particular disturbance in question (e.g., moisture content and evapotranspiration, nutrient concentrations) as well as biotic factors such as population size, species composition, and life history dynamics. Using relationships between disturbance drivers and disturbance impacts, we generate four universal types of disturbances with variation within each type due to the strength of the driver and the size of the impact. Conceptualizing disturbance drivers, either abiotic or biotic, on an x-axis and the impact of disturbance impacts on a y-axis yields four quadrants: weak & positive, strong & positive, weak & negative, and strong & negative.
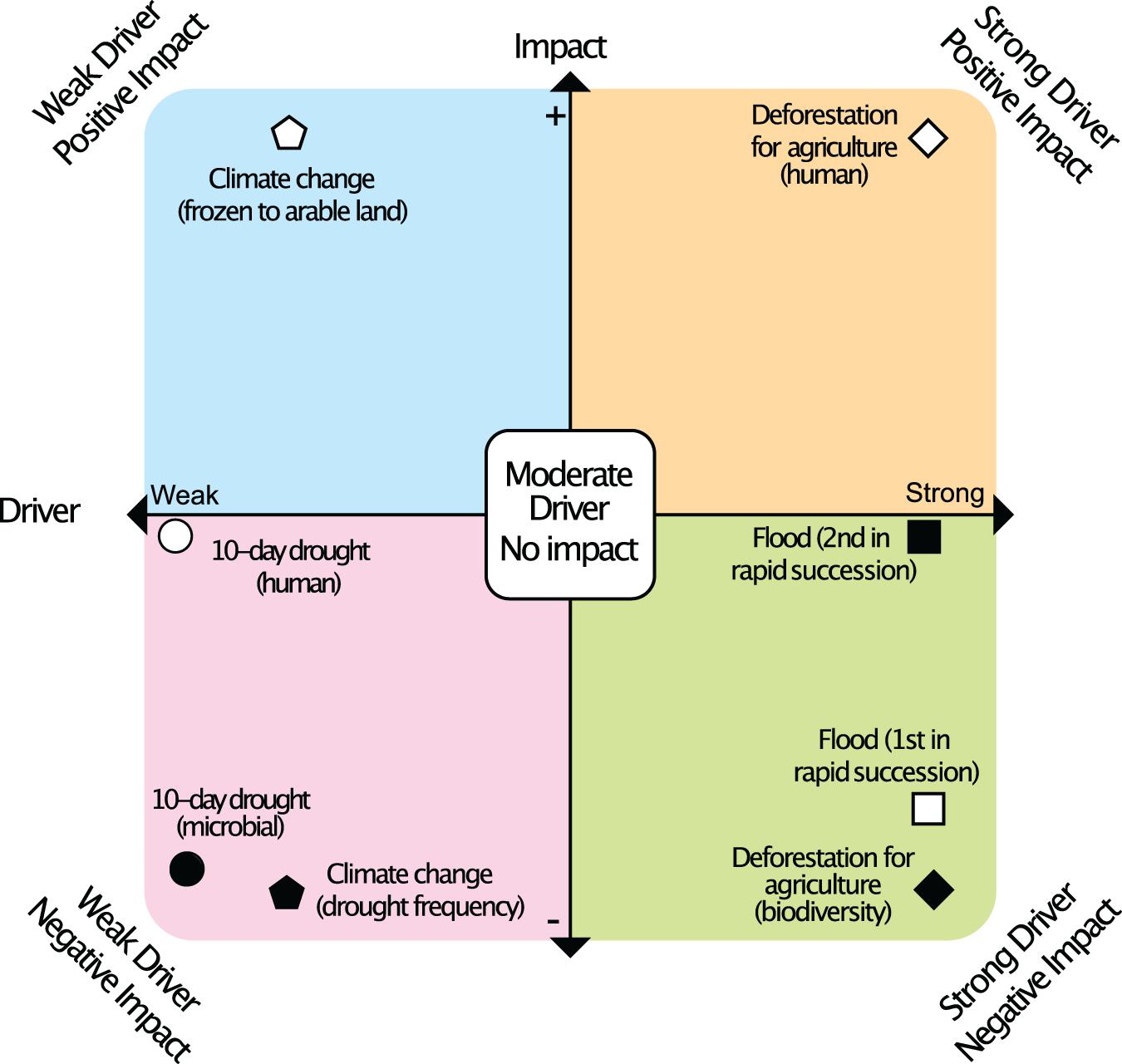
Figure 1. Current disturbance lexicon conflates two distinct processes—events that drive ecological change and impacts of extreme events—both interchangeably termed disturbances despite fundamental distinctions between the two types of processes. We disentangle these processes to derive four universal types of disturbances that are applicable regardless of the line of inquiry or its spatiotemporal scale. Drivers (x-axis) are defined as a force, either biotic or abiotic, that deviates from local, prevailing background conditions. A driver is characterized by its magnitude of deviation from an environmental baseline (low to high deviation denotes weak to strong driver). Impacts (y-axis) are defined as the impact of social-ecological consequences of a driver relative to a scale-dependent baseline state. Impacts can be positive or negative depending on the perspective of the study. Each quadrant is, therefore, a unique disturbance type defined in more detail in Table 1, and the position of drivers and impacts across and within the quadrants slides with the line of inquiry. Examples of disturbances across spatial and temporal scales are denoted within each quadrant.
The position of drivers and impacts across and within the quadrants varies with the line of inquiry (Figure 1). For example, a 10-day drought is a severe disturbance for a drought-sensitive microorganism, but probably inconsequential for humans in urban environments (Figure 1). Additionally, the disturbance impact for a single driver could be simultaneously positive and negative, dependent on scale. For example, deforestation for agriculture could be positive from an immediate human perspective (food production) but negative from an ecological perspective (habitat loss). This allows for interacting and compounding disturbances to be viewed within the same framework as single events and for events that cause tipping points to be represented as weak driver-high impact events.
Spatial and temporal scales are also implicitly represented in the proposed framework, as historical exposures have direct effects on the impact of a given driver and the scale of interest defines the magnitude of both the driver and impact. Likewise, the ecological state of a system (e.g., its stability, resistance, resilience, and successional stage) also influences the ‘impact’ axis of disturbances through escalating or mediating the impact. Further explanation, definitions and examples of each quadrant are presented in more detail in Table 1 and Figure 1.
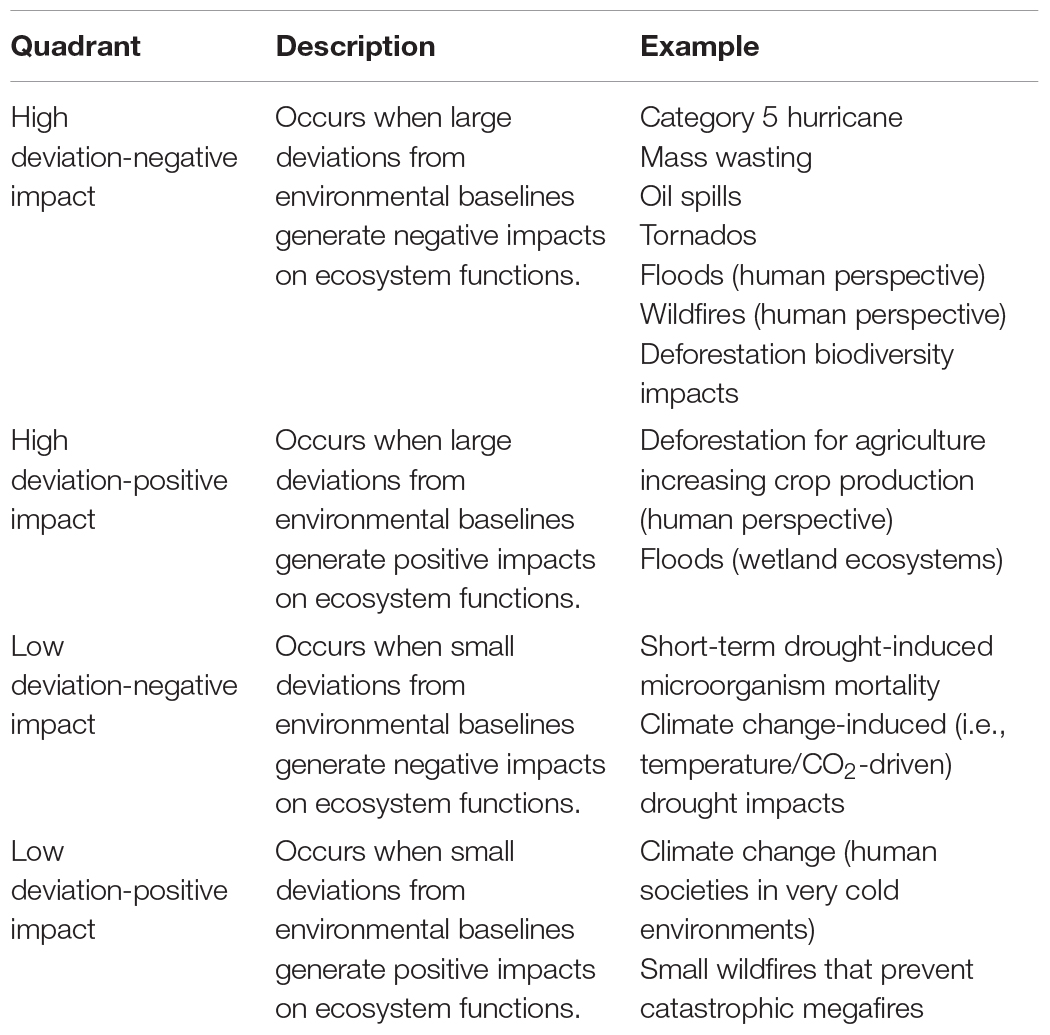
Table 1. Description and examples of the four universal disturbance types generated by the proposed framework.
The advantage of conceptualizing and classifying disturbances into this inclusive framework is to increase interoperability of disturbance research across scientific domains. While the current framework is qualitative in nature and based upon discipline-specific expert knowledge of driver and impact magnitudes, there are further opportunities to develop quantitative thresholds to separate quadrants for particular lines of investigation. For example, a disturbance driver and impact size fall within a range of historical variation that is specific to the event type. Quantitative thresholds for event types can then be developed to separate events along driver and impact axes based on the distribution of historical events along those axes.
Common Factors to Consider in a Generalizable Framework
Below, we describe a subset of factors related to disturbance that most strongly resonated across researchers from different disciplines when putting together our framework. Investigations of disturbances are vast and multifaceted, and we do not intend the sections below to be comprehensive reviews of the subjects mentioned. Rather, we present concepts that are most transferable and therefore able to underpin a common understanding. We point the reader to references within this section for more thorough reviews of the topics discussed.
System Stability
System stability has been a pillar of disturbance research across scientific domains as it can be used to describe a system’s response to environmental change (Ives and Carpenter, 2007; Duncan et al., 2010; Hodgson et al., 2015; Seidl et al., 2016; Todman et al., 2016). Here, we review core and emerging system stability theory to form a conceptual basis for an interdisciplinary approach to understanding disturbance discussed in later sections. There are several aspects of system stability theory that are common throughout social-ecological domains, including the concepts of resilience, resistance, and redundancy. While these concepts often underlie hypothesis-testing in disturbance ecology, the exact nature of their relationships to disturbance impacts and recovery trajectories remains unknown. Resilience is commonly defined as the ability of a system to recover from disturbance, while resistance is the ability of an ecosystem to remain unchanged when subjected to disturbance (Holling, 1973, 1996; Westman, 1978; McCann, 2000; Gunderson et al., 2002; Griffiths and Philippot, 2013; Seidl et al., 2016; Lamentowicz et al., 2019). Resistance and resilience are functions of biodiversity and species traits. They are quantified using various metrics, including the time, slope/rate, and angle of recovery relative to a baseline state (Connell and Sousa, 1983; Shade et al., 2012). Additionally, functional redundancy and similarity are also used in ecology to describe the capacity of a system to resist and recover from a disturbance, whereby the presence of functionally redundant phenotypes enhances ecosystem stability (Naeem and Li, 1997; Allison and Martiny, 2008). Similarly, response diversity, which relies on differential responses among species to environmental fluctuations and disturbance, is another factor in determining ecosystem stability (Yachi and Loreau, 1999; Elmqvist et al., 2003). Functional redundancy, functional similarity, and response diversity are often framed within the ‘insurance hypothesis,’ wherein higher biodiversity increases the likelihood that the community contains species with differential species functions or responses to the environment, providing “insurance” for aggregate properties of the community (Allison and Martiny, 2008; Mori et al., 2013). Indeed, biodiversity is an integral component of system stability and has been repeatedly linked to the capacity of ecosystems to be resistant to a diversity of disturbances, such as biological invasions and climate fluctuations (Cardinale and Palmer, 2002; Isbell et al., 2009; Cardinale et al., 2012; Kardol et al., 2018).
Another central paradigm in stability theory is that the intensity of the response to disturbances is often non-linearly related to the intensity of the disturbance itself. There is a growing understanding of the importance of tipping points that, when reached or exceeded, cause strongly non-linear system responses and sudden shifts in system behavior (Dai et al., 2012; Loecke et al., 2017). For instance, Scheffer et al. (2001) showed that ecosystems can deviate rapidly from their current state due to minor shifts in underlying biotic or abiotic drivers. Similarly, slow and often undetectable changes can reduce ecosystem resilience, leading to unpredictable system collapses (Walker et al., 2012). When pressures exceed ecosystem tipping points, regime shifts can occur, and ecosystems are pushed into a different (alternative) state that is maintained by self-reinforcing feedbacks (Pausas and Bond, 2020). Identifying which disturbance regimes are susceptible to regime shifts that result in a switch between stable states or to a new alternative state remains a key obstacle. While there is growing capacity to predict regime shifts (e.g., by rising variance in ecosystem properties or by slow recovery rates), several difficulties remain in their prediction, in part due to the challenge of measuring appropriate indicators for resilience (Van Nes and Scheffer, 2007; Scheffer et al., 2009; Scheffer, 2010; Dai et al., 2012, 2013). While system stability is also criticized as ambiguous or difficult to apply in practice (Grimm and Wissel, 1997), historical and emerging research on system stability pervades many disciplines and provides a common foundation for understanding disturbances across a broad suite of ecosystems and across lines of investigation with different underlying objectives.
Spatiotemporal Considerations
Spatial extent and temporal duration are integral components of disturbances, and quantifying these characteristics for individual disturbances is key to understanding the ecological impacts of those disturbances. Though the specific nature of disturbance extents and duration can vary greatly, all disturbances occur over space and time; any generalizable framework must, therefore, consider the spatiotemporal extent and variability of disturbances. This includes defining the baseline conditions relative to which a disturbance is assessed to build a set of domain-agnostic principles. These baselines may vary as a function of the spatiotemporal scale over which an analysis is being performed, and the deviation of a system from its baseline at a given scale can be used to assess a disturbance’s intensity and impact (E Silva et al., 2013). As ecosystems change in response to climate, land-use change, and other human activities, conditions that were once considered disturbed against a static baseline may now shift into a new normal range of variation (Figure 2). In this section, we review key spatial and temporal perspectives that influence disturbances and that should underlie an interdisciplinary understanding of disturbance.
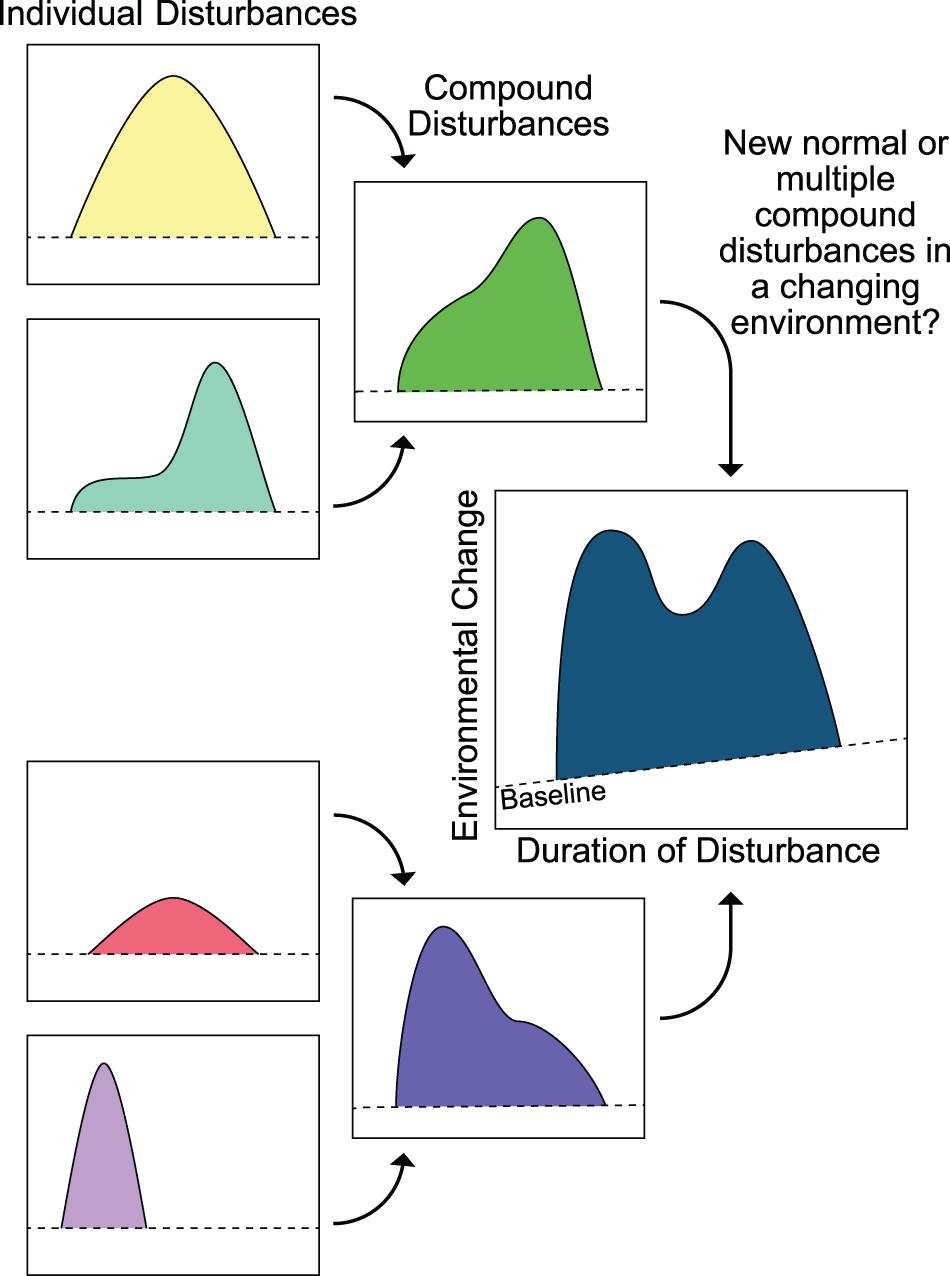
Figure 2. An obstacle to historical paradigms of disturbance theory is that changes in environmental conditions will not only alter the frequency of disturbances, but also the potential for multiple interacting disturbances. As multiple disturbances compound through time, a crucial question emerges: “When does a disturbed state become normal?” Compound disturbances can take many forms and result in both linear and non-linear ecosystem responses. As an example, this figure shows an additive trajectory of disturbances and resultant environmental change. The leftmost panels represent single disturbance events that have long been the targets of scientific research. As disturbances aggregate through time, a new class of ‘compound’ disturbances have been a rising topic (middle panels). With the continuing increases in the frequency and intensity of disturbances, a key challenge remains to disentangle multiple compounding disturbances from normal variability in ecosystem functions (rightmost panel). Another challenge is that environmental baselines (dashed line) shift through time, adding a chronic component to the study of short-term disturbance events.
The drivers and impacts of disturbance are dependent on the spatial features of their broader landscapes and the spatial perspective of a given study’s objectives. For example, pre-existing ecosystem characteristics, such as habitat connectivity and topography, influence the spatial structure of disturbance impacts by dictating its ability to spread as well as the ecosystem’s ability to be recolonized by surviving organisms in neighboring spaces (Turner et al., 1994; Drever et al., 2006; Buma, 2015). Further, the spatial perspective taken when studying a disturbance can also heavily influence conclusions drawn about its effects. Some disturbances (e.g., fine-scale temperature shifts) may be apparent only at local scales while others influence regional and coarser scales (Aalto et al., 2017; Lembrechts et al., 2019). In general, disturbances that directly affect species interactions tend to be observable at local scales (Mod et al., 2014), while disturbances related to habitat alterations are detectable at coarser spatial resolution (Hamer and Hill, 2000; Dumbrell et al., 2008; Chase, 2014).
Both the impact of a disturbance as well as ecosystem responses to one ultimately depends on how the disturbances modify scale-specific factors that control ecosystem stability (Dobson et al., 1997; Dumbrell et al., 2008; Wei and Zhang, 2010; Cohen et al., 2016). For example, extant dispersal rates and disturbance scale can regulate the recovery of disturbed ecological communities and the spread of impacts across space to neighboring populations (Zelnik et al., 2019). Furthermore, the spatial extent and patterning of disturbances can influence disturbance impacts.
Additionally, impacts at multiple spatial scales can interact. Local disturbances can play an important role in maintaining regional biodiversity through patch dynamics mediated by intra- and inter- species dynamics such as competition and colonization trade-offs (Tilman, 1994; Grime, 2006; le Roux et al., 2013; He et al., 2019), which may reduce vulnerability to larger scale disturbances. Local disturbances can also exacerbate the impacts of more widespread regional disturbances, placing ecosystems under increased threat of collapse (Kendrick et al., 2019). If resilience is overcome because of multiple disturbances, then compound disturbances may cause a state change or ‘ecological surprise’ that is largely unpredictable (Paine et al., 1998).
For a common understanding of disturbances, we also need to acknowledge the central influence of time without explicitly defining a single general time scale of disturbances. Some disturbances impact ecosystem dynamics over short time scales (i.e., pulse events), whereas other disturbances operate over long time periods (i.e., ramp and press events) (Connell, 1997; Connell et al., 1997; Jentsch and White, 2019). Importantly, a single type of event may constitute a disturbance at one timescale, but not at another. While a forest fire may be a significant deviation from an environmental baseline when considered on an annual or decadal scale (and therefore, a disturbance at this timescale), it may fall within the historical range of environmental variation on a centennial timescale (and therefore, not a disturbance at this timescale) (Turner, 2010). Furthermore, the effects of slow increases in mean annual temperatures may be insignificant over the course of a few years when considering the background variation in mean annual temperatures (IPCC, 2019). However, at a centennial scale, the warming trend shifts the mean, as well as the frequency of extreme temperatures generating climates outside the range of historical variation. Similar arguments can be made for nitrogen deposition, chronic fertilization, pesticide applications, elevated CO2, and many other global disturbances (Jackson et al., 2001; Ferretti et al., 2010; Ripple et al., 2014).
This temporal perspective highlights that changing conditions through time (‘non-stationarity’, Milly et al., 2008; Vicente-Serrano and López-Moreno, 2008; Wolkovich et al., 2014) is also a central consideration for any conceptualization of disturbances to be applicable in the future. Baseline conditions and driver–response relationships are dynamically conditioned by the legacies of disturbance and ecological memory (Johnstone et al., 2016; Nowicki et al., 2019). Ecological succession is a classic example of ecosystem trajectories that interact with more discrete events to yield an aggregate disturbance impact. Disturbances can interrupt and potentially alter trajectories of succession through impacts on community dynamics that dramatically alter ecosystem functions (Ghoul and Mitri, 2016). For example, antibiotic administration and delivery mode can disrupt microbial community assembly and succession in the human infant gut microbiome that in turn can drive long-term impacts on host health (Koenig et al., 2011).
Rising Importance of Interacting Disturbances
An obstacle to historical paradigms of disturbance theory is that changes in environmental conditions will not only alter the frequency of disturbances, but also the potential for multiple interacting disturbances to impact system stability (Seidl et al., 2017). For example, drought may increase the vulnerability of wildfire in forests, or wildfires may enhance the probability of erosion and mudslides that affect ecosystems and communities downstream (Tiribelli et al., 2019). Multiple interacting disturbances can lead to novel ecosystem responses, sometimes impacting an ecosystem’s resilience to the second disturbance (Folt et al., 1999; Darling and Côté, 2008; Buma, 2015; Burton and Boulanger, 2018) and compromising our abilities to understand disturbances in unknown future environments (Hobbs et al., 2009, 2014; Pidgen and Mallik, 2013; Carlson et al., 2017; Mehran et al., 2017; Calderón et al., 2018; Zscheischler et al., 2018; Brando et al., 2019; Knelman et al., 2019; Ryo et al., 2019).
Given the variation that occurs both in disturbed systems and in the goals of disturbance studies and applications, we present a framework that describes a minimum foundation for best practices for creating and sharing knowledge about disturbed systems in a novel and changing world. Towards this end, it then becomes necessary to follow standardized reporting practices to characterize the historical range of variation of disturbances and to classify individual events within a scale-flexible framework.
Minimum Reporting Standards
Because scales of investigation vary tremendously between disciplines, it is necessary for researchers to present sufficient data in publications and community repositories that capture complexity for other researchers to evaluate placement of their investigated disturbances within this framework (Slette et al., 2019). When possible, standardized indices are suggested to explicitly describe disturbance driver (e.g., Palmer Drought Severity Index, Standardized Precipitation Evapotranspiration Index, Normalized Burn Ratio) and impacts (e.g., quantifying the response of species and communities to disturbance) (Palmer, 1965; van der Maarel, 1975; Eidenshink et al., 2007; Vicente-Serrano and López-Moreno, 2008; Veraverbeke et al., 2010; Battisti and Fanelli, 2015). In ecological research, indices are most well-described for plot-scale studies and anthropocentric framings of scale that relate to our own human experiences rather than ecological processes (e.g., monetary losses from hurricanes), while they are more nascent for cross-scale disturbance work. Therefore, in addition to indices, it is necessary to report variables that describe the magnitude, duration, and rate of change of drivers and response variables in a consistent manner that is applicable regardless of scale. For example, Salafsky et al. (2008) propose a hierarchical lexicon for biodiversity conservation that divides elements of investigation threats vs. actions and a suite of nested variables beneath these categories. While this lexicon encompasses some aspects of disturbances described here, it is focused on one aspect of disturbance impacts toward a specific end goal of determining priorities and resource allocations for conservation strategies.
We suggest three categories of variables for minimum reporting standards to facilitate a cohesive understanding of disturbances across scientific disciplines: (1) ecosystem properties, (2) driver descriptors, and (3) impact descriptors (Table 2). An integral distinction of these standards compared to previous efforts is the explicit recording of spatial and temporal scales needed for an interoperable understanding of disturbances (Peters et al., 2011). Ecosystem properties are foundational variables that provide context for disturbance interpretation (e.g., ecosystem type, successional state, and system stability). Driver and impact descriptors are each divided into three categories: reference, spatial, and temporal variables. These variables capture system stability and spatiotemporal dynamics that allow for multiscale comparisons including mild versus extreme intensity, acute versus chronic timescales, and abrupt versus gradual change (Ryo et al., 2019). Collectively, they allow for the placement of events on both the driver and impact axes of the proposed conceptual framework as well as providing context that describes the scale and scope of the investigation.
Promising Cross-Disciplinary Approaches to Address Areas of Need
While we address some challenges of disturbance research here, developments in technology, methodology, and cross-disciplinary approaches are necessary to close knowledge gaps. We highlight the need to integrate disturbance responses across scales of ecological organization from genes to ecosystems. We expect that future studies should consider multiple scales of sampling and analysis that comprehensively evaluate disturbances and their effects across spatial, temporal, and/or organismal scales. Ecological hierarchies underlie self-organized ecosystems and provide a structure for using information theory and other advanced statistical techniques to predict whole ecosystem impacts (Allen and Starr, 2017; Arora et al., 2019). Social-ecological applications of machine learning, graph theory, and information theory are exponentially increasing and can decipher complex relationships in multidimensional data streams as well as scale dynamics from pore-to-global scales (Peters et al., 2018; Weintraub et al., 2019). These approaches are used to collapse complex data types into tangible variables by deciphering classes of organisms and relationships among these classes through space or time. They reveal the organizational structure of a system through interaction networks that include both random and ordered processes (Ings et al., 2009). Remote sensing can also aid in evaluating the spatial extent and spatial patterning of disturbance, thereby defining the appropriate scale of sampling for these analyses (Shiklomanov et al., 2019). However, empirical tests on the potential for disturbance impacts to propagate through ecosystem hierarchies are lacking and is a major research need. One opportunity would be the use of paired experimental and modeling approaches to elucidate networked changes in ecological systems resulting from disturbance impacts. The use of experiments and clearly outlined hypotheses is increasingly argued as a core need for generating predictive understandings of ecosystem responses to disturbance (Spake et al., 2017; Currie, 2019).
Our second area of need also considers the broader issue of scale—understanding how disturbances interact with each other and potentially compound through space and time. Recent work has underscored interactions between extreme events occurring closely in space and time, for example by elucidating how the discrete effects of flooding on biogeochemistry are related to prior fire exposure (Knelman et al., 2019), and that the effects of a fire may depend on previous droughts or insect outbreaks (Burton and Boulanger, 2018). The long-term processes of environmental change also have multifaceted impacts on ecosystems but are most frequently studied independently (Rillig et al., 2019; Song et al., 2019). Such work accentuates questions into ecosystem trajectories—as disturbances increase through time, are there thresholds beyond which ecosystems are irreversibly altered? Thus, a multivariate perspective is necessary to accurately assess the impact of interacting disturbances (Zscheischler and Seneviratne, 2017).
The evolutionary consequences of living in an environment with recurrent disturbances are also poorly understood (Pausas and Keeley, 2014; Pausas et al., 2017). Some species, for example, have evolved specific life-history adaptations that enable them to not only survive and exploit disturbances but even to require them for their persistence (e.g., savannas, Mediterranean shrublands, alpine vegetation, riparian cottonwoods) (Mahoney and Rood, 1998; Lytle and Poff, 2004; Keeley et al., 2011; Dantas et al., 2013; le Roux et al., 2013). Similarly, disturbances have countervailing effects on population dynamics in that they can cause immediate mortality of species, but also create new habitat, thereby increasing growth rate or increasing population size post-disturbance (Pausas and Keeley, 2014; McMullen et al., 2017). For instance, if the consequences of climate change-related disturbances are studied separately, the results may be greatly biased as compared to when the consequences are considered simultaneously (Niittynen et al., 2018). Therefore, the interactions between disturbances that change eco-evolutionary dynamics provide a relatively unexplored area for future research.
A third research need, establishing appropriate baselines and trajectories for different ecosystem, disturbance, and organism types is essential for evaluating disturbances that alter ecosystem structure and function. Paleoecological data can provide historical reference baselines, help evaluate sensitivity to disturbances across different windows of space and time, and unveil past state changes that provide a foundation for understanding how ecological hierarchies will respond to future environmental changes (Lamentowicz et al., 2019). Time-series methods are also well-equipped to separate disturbances from long-term trends and evaluate changes in disturbance regimes through time (e.g., wavelet analysis) (Keitt, 2008; Tonkin et al., 2017). For instance, Sabo and Post (2008) developed tools based on Fourier analysis to disentangle the periodic (seasonal), stochastic (interannual), and catastrophic components of river flow regimes. Space-for-time approaches, in which distances from an event are used as a proxy for the time-since-event, can reveal long-term impacts without necessitating decades of monitoring (Pickett et al., 1989; Walker et al., 2010). Although space-for-time investigations require a correlation between the age of an ecosystem attribute and spatial structuring that may not be applicable to highly disturbed landscapes, chronosequences can be used to investigate plant and soil successional processes at decadal to millennial timescales (Walker et al., 2010; Laliberté et al., 2013; Sutherland et al., 2016; Fanin et al., 2018).
Finally, we underline the need for enhancing predictive capabilities through process-based models and ecological forecasting initiatives that represent the impacts of disturbance drivers on ecosystem attributes, going beyond historical correlations that fail to represent causal relationships (Dietze et al., 2018; Tonkin et al., 2019). Generating a model robust to disturbance type, ecosystem, and scale that allows managers to detect disturbance drivers and predict disturbance impact sizes is one of the ultimate goals of disturbance ecology. Mechanistic models can further advance progress toward this goal by representing interactions among species through time (Tonkin et al., 2018). Process-based and forecasting models can be tailored to highly specific conditions and can provide managers with both a predicted outcome and a range of uncertainty based on the underlying driver (Tonkin et al., 2019). They are commonly used to guide management practices in fisheries and conservation efforts (Tonkin et al., 2019). Collectively, process-based and forecasting models are potential tools developing mitigation strategies and informing how humans might intervene at individual, local, regional, and global scales to minimize social-ecological damages caused by disturbances (Dale et al., 1998; Berkes et al., 2000; Folke et al., 2005; D’Amato et al., 2011; Goldstein et al., 2020).
Conclusion
Our work synthesizes knowledge globally across institutions using crowdsourced open science and demonstrates that novel approaches can generate emergent ideas greater than the sum of their independent disciplinary parts. The integration of interdisciplinary contributions of 46 individuals, from 38 institutions — from academic, governmental, and non-governmental organizations — in 15 countries, into the novel conceptual framework presented here demonstrates the currently untapped potential for supporting collaborative co-creation of research, facilitated by social media and collaborative writing platforms. For a detailed description of the writing process and contributor demographics, see Graham and Smith (2020). Our experiences through this process motivate us to encourage the wider scientific community to continue to explore the suitability of similar approaches for facilitating collaborative research that benefits from a large interdisciplinary knowledge base and allows us to fully embrace Open Science principles in collaborative interdisciplinary research.
Using a completely open and crowdsourced scientific approach, we integrate insights from numerous scientific perspectives to present a generalizable framework for cross-disciplinary disturbance investigations. We discuss and use ideas that are common across multiple disciplines to underlie the framework as a foundation for investigations into the causes and consequences of disturbances. Discipline-specific variables can supplement this framework to generate deeper insight into specific research questions. We highlight how the current lexicon used to discuss disturbances generates confusion by conflating events that drive ecological change with the impacts of extreme events. To overcome this challenge, we propose parsing disturbance theory between disturbance drivers and disturbance impacts and encourage researchers to be explicit about how they define their studied disturbance within this context.
Using drivers and impacts as axes of variation, the framework generates four universal disturbance types that are applicable regardless of the line of inquiry or its spatiotemporal scale (Figure 1). To provide consistency in comparing disturbances within this framework, we suggest three categories of variables for minimum reporting standards: (i) ecosystem properties that provide context and (ii) disturbance driver and (iii) disturbance impact descriptors that capture system stability and spatiotemporal dynamics.
We also highlight promising lines of research to generate a more universal understanding of disturbance events and their impacts, including integrating scales of ecological research, understanding how disturbances interact with each other, establishing appropriate baselines and trajectories, and developing process-based models and ecological forecasting initiatives that will enable robust prediction capabilities and mitigation strategies. As global change accelerates the threats of disturbances, the framework presented here serves as a foundation for cross-disciplinary discussion of the complexities of understanding the causes and consequences of disturbances across studies with different scientific and management goals. We encourage researchers to be explicit in how they define disturbance drivers and impacts and to continue to work toward interoperable terminology and knowledge of disturbances.
Contributor Consortium
The following authors, who are listed in alphabetical order, contributed to the work of Contributor Consortium:
Arie Staal, Utrecht University, a.staal@uu.nl; Bhavna Arora, Lawrence Berkeley National Laboratory, barora@lbl.gov; Callie Oldfield, University of Georgia, callieoldfield@uga.edu; Dipankar Dwivedi, Lawrence Berkeley National Laboratory, DDwivedi@lbl.gov; Erin Larson, Alaska Pacific University, elarson@alaskapacific.edu; Ezequiel Santillan, Singapore Centre for Environmental Life Sciences Engineering; Nanyang Technological University, Singapore, esantillan@ntu.edu.sg; J. Aaron Hogan, Department of Biological Sciences, Florida International University, Oak Ridge National Lab, jamesaaronhogan@gmail.com; Jeff Atkins, Virginia Commonwealth University, jwatkins6@vcu.edu; Jianqiu Zheng, Pacific Northwest National Laboratory, jianqiu.zheng@pnnl.gov; Jonas Lembrechts, University of Antwerp, jonas.lembrechts@uantwerpen.be; Kaizad Patel, Pacific Northwest National Laboratory, kaizad.patel@pnnl.gov; Kelsey Copes-Gerbitz, University of British Columbia, kelsey.copes-gerbitz@ubc.ca; Kevin Winker, University of Alaska Fairbanks, kevin.winker@alaska.edu; Laura Mudge, University of North Carolina at Chapel Hill, lmudge@live.unc.edu; Mark Wong, University of Oxford, markwong.research@outlook.com; Martin Nuñez, Universidad del Comahue/CONICET, nunezm@gmail.com; Miska Luoto, Department of Geosciences and Geography, University of Helsinki, Finland, miska.luoto@helsinki.fi; Rebecca Barnes, Colorado College, rbarnes@coloradocollege.edu.
Author Contributions
The leadership team was responsible for conceiving the project, contributing content, and editing the manuscript. All other authors listed in the author list were significant contributors to content and/or revisions. All authors contributed to the article and approved the submitted version. Other contributing members are mentioned in “Contributor Consortium” section.
Funding
This research was supported by the U.S. Department of Energy (DOE), Office of Biological and Environmental Research (BER), as part of Subsurface Biogeochemical Research Program’s Scientific Focus Area (SFA) at the Pacific Northwest National Laboratory (PNNL). PNNL is operated for DOE by Battelle under contract DE-AC06-76RLO 1830.
Conflict of Interest
The authors declare that the research was conducted in the absence of any commercial or financial relationships that could be construed as a potential conflict of interest.
Acknowledgments
We thank many members of the scientific community for helping us spread the word of this project via social media and for valuable feedback during this project. We are also grateful for the suggestions of the reviewers.
References
Aalto, J., Riihimäki, H., Meineri, E., Hylander, K., and Luoto, M. (2017). Revealing topoclimatic heterogeneity using meteorological station data. Int. J. Climatol. 37, 544–556. doi: 10.1002/joc.5020
Allen, T. F., and Starr, T. B. (2017). Hierarchy: Perspectives for Ecological Complexity. Chicago, IL: University of Chicago Press.
Allison, S. D., and Martiny, J. B. (2008). Resistance, resilience, and redundancy in microbial communities. Proc. Natl. Acad. Sci. U.S.A. 105, 11512–11519. doi: 10.1073/pnas.0801925105
Arora, B., Wainwright, H. M., Dwivedi, D., Vaughn, L. J., Curtis, J. B., Torn, M. S., et al. (2019). Evaluating temporal controls on greenhouse gas (GHG) fluxes in an Arctic tundra environment: an entropy-based approach. Sci. Total Environ. 649, 284–299. doi: 10.1016/j.scitotenv.2018.08.251
Battisti, C., and Fanelli, G. (2015). Don’t think local! Scale in conservation, parochialism, dogmatic bureaucracy and the implementing of the European Directives. J. Nat. Conserv. 24, 24–30. doi: 10.1016/j.jnc.2015.01.005
Battisti, C., Poeta, G., and Fanelli, G. (2016). An Introduction to Disturbance Ecology. (Cham: Springer), 13–29.
Berkes, F., Colding, J., and Folke, C. (2000). Rediscovery of traditional ecological knowledge as adaptive management. Ecol. Appl. 10, 1251–1262. doi: 10.1890/1051-0761(2000)010[1251:roteka]2.0.co;2
Boer, M. M., De Dios, V. R., and Bradstock, R. A. (2020). Unprecedented burn area of Australian mega forest fires. Nat. Clim. Change 10, 171–172. doi: 10.1038/s41558-020-0716-1
Borics, G., Várbíró, G., and Padisák, J. (2013). Disturbance and stress: different meanings in ecological dynamics? Hydrobiologia 711, 1–7. doi: 10.1007/s10750-013-1478-9
Brando, P. M., Silvério, D., Maracahipes-Santos, L., Oliveira-Santos, C., Levick, S. R., Coe, M. T., et al. (2019). Prolonged tropical forest degradation due to compounding disturbances: implications for CO2 and H2O fluxes. Glob. Change Biol. 25, 2855–2868. doi: 10.1111/gcb.14659
Buma, B. (2015). Disturbance interactions: characterization, prediction, and the potential for cascading effects. Ecosphere 6:70.
Burton, P. J., and Boulanger, Y. (2018). Characterizing combined fire and insect outbreak disturbance regimes in British Columbia, Canada. Landsc. Ecol. 33, 1997–2011. doi: 10.1007/s10980-018-0710-4
Calderón, K., Philippot, L., Bizouard, F., Breuil, M.-C., Bru, D., and Spor, A. (2018). Compounded disturbance chronology modulates the resilience of soil microbial communities and N-cycle related functions. Front. Microbiol. 9:2721. doi: 10.3389/fmicb.2018.02721
Cardinale, B. J., Duffy, J. E., Gonzalez, A., Hooper, D. U., Perrings, C., Venail, P., et al. (2012). Biodiversity loss and its impact on humanity. Nature 486, 59–67. doi: 10.1038/nature11148
Cardinale, B. J., and Palmer, M. A. (2002). Disturbance moderates biodiversity–ecosystem function relationships: experimental evidence from caddisflies in stream mesocosms. Ecology 83, 1915–1927. doi: 10.2307/3071774
Carlson, A. R., Sibold, J. S., Assal, T. J., and Negron, J. F. (2017). Evidence of compounded disturbance effects on vegetation recovery following high-severity wildfire and spruce beetle outbreak. PLoS One 12:e0181778. doi: 10.1371/journal.pone.0181778
Chase, J. M. (2014). Spatial scale resolves the niche versus neutral theory debate. J. Veg. Sci. 25, 319–322. doi: 10.1111/jvs.12159
Cleetus, R., and Mulik, K. (2014). Playing With Fire: How Climate Change and Development Patterns are Contributing to the Soaring Costs of Western Wildfires. Available online at: http://www.ucsusa.org/assets/documents/global_warming/playing-with-fire-report.pdf (accessed September 9, 2014).
Cohen, J. M., Civitello, D. J., Brace, A. J., Feichtinger, E. M., Ortega, C. N., Richardson, J. C., et al. (2016). Spatial scale modulates the strength of ecological processes driving disease distributions. Proc. Natl. Acad. Sci. U.S.A. 113, E3359–E3364.
Connell, J. H., Hughes, T. P., and Wallace, C. C. (1997). A 30-year study of coral abundance, recruitment, and disturbance at several scales in space and time. Ecol. Monogr. 67, 461–488. doi: 10.1890/0012-9615(1997)067[0461:aysoca]2.0.co;2
Connell, J. H., and Sousa, W. P. (1983). On the evidence needed to judge ecological stability or persistence. Am. Nat. 121, 789–824. doi: 10.1086/284105
Currie, D. J. (2019). Where Newton might have taken ecology. Glob. Ecol. Biogeogr. 28, 18–27. doi: 10.1111/geb.12842
Dai, L., Korolev, K. S., and Gore, J. (2013). Slower recovery in space before collapse of connected populations. Nature 496, 355–358. doi: 10.1038/nature12071
Dai, L., Vorselen, D., Korolev, K. S., and Gore, J. (2012). Generic indicators for loss of resilience before a tipping point leading to population collapse. Science 336, 1175–1177. doi: 10.1126/science.1219805
Dale, V. H., Lugo, A. E., Macmahon, J. A., and Pickett, S. T. (1998). Ecosystem management in the context of large, infrequent disturbances. Ecosystems 1, 546–557. doi: 10.1007/s100219900050
D’Amato, A. W., Bradford, J. B., Fraver, S., and Palik, B. J. (2011). Forest management for mitigation and adaptation to climate change: insights from long-term silviculture experiments. For. Ecol. Manage. 262, 803–816. doi: 10.1016/j.foreco.2011.05.014
Dantas, V. D. L., Batalha, M. A., and Pausas, J. G. (2013). Fire drives functional thresholds on the savanna–forest transition. Ecology 94, 2454–2463. doi: 10.1890/12-1629.1
Darling, E. S., and Côté, I. M. (2008). Quantifying the evidence for ecological synergies. Ecol. Lett. 11, 1278–1286. doi: 10.1111/j.1461-0248.2008.01243.x
Dietze, M. C., Fox, A., Beck-Johnson, L. M., Betancourt, J. L., Hooten, M. B., Jarnevich, C. S., et al. (2018). Iterative near-term ecological forecasting: Needs, opportunities, and challenges. Proc. Natl. Acad. Sci. U.S.A. 115, 1424–1432. doi: 10.1073/pnas.1710231115
Dobson, A. P., Rodriguez, J. P., Roberts, W. M., and Wilcove, D. S. (1997). Geographic distribution of endangered species in the United States. Science 275, 550–553. doi: 10.1126/science.275.5299.550
Drever, C. R., Peterson, G., Messier, C., Bergeron, Y., and Flannigan, M. (2006). Can forest management based on natural disturbances maintain ecological resilience? Can. J. For. Res. 36, 2285–2299. doi: 10.1139/x06-132
Dumbrell, A. J., Clark, E. J., Frost, G. A., Randell, T. E., Pitchford, J. W., and Hill, J. K. (2008). Changes in species diversity following habitat disturbance are dependent on spatial scale: theoretical and empirical evidence. J. Appl. Ecol. 45, 1531–1539. doi: 10.1111/j.1365-2664.2008.01533.x
Duncan, S. L., Mccomb, B. C., and Johnson, K. N. (2010). Integrating ecological and social ranges of variability in conservation of biodiversity: past, present, and future. Ecol. Soc. 15:5.
E Silva, M. C. P., Semenov, A. V., Schmitt, H., Van Elsas, J. D., and Salles, J. F. (2013). Microbe-mediated processes as indicators to establish the normal operating range of soil functioning. Soil Biol. Biochem. 57, 995–1002. doi: 10.1016/j.soilbio.2012.10.002
Eidenshink, J., Schwind, B., Brewer, K., Zhu, Z.-L., Quayle, B., and Howard, S. (2007). A project for monitoring trends in burn severity. Fire Ecol. 3, 3–21. doi: 10.4996/fireecology.0301003
Elmqvist, T., Folke, C., Nyström, M., Peterson, G., Bengtsson, J., Walker, B., et al. (2003). Response diversity, ecosystem change, and resilience. Front. Ecol. Environ. 1, 488–494. doi: 10.1890/1540-9295(2003)001[0488:rdecar]2.0.co;2
Fanin, N., Gundale, M. J., Farrell, M., Ciobanu, M., Baldock, J. A., Nilsson, M.-C., et al. (2018). Consistent effects of biodiversity loss on multifunctionality across contrasting ecosystems. Nat. Ecol. Evol. 2, 269–278. doi: 10.1038/s41559-017-0415-0
Ferretti, F., Worm, B., Britten, G. L., Heithaus, M. R., and Lotze, H. K. (2010). Patterns and ecosystem consequences of shark declines in the ocean. Ecol. Lett. 13, 1055–1071.
Folke, C., Hahn, T., Olsson, P., and Norberg, J. (2005). Adaptive governance of social-ecological systems. Annu. Rev. Environ. Resour. 30, 441–473.
Folt, C., Chen, C., Moore, M., and Burnaford, J. (1999). Synergism and antagonism among multiple stressors. Limnol. Oceanogr. 44, 864–877. doi: 10.4319/lo.1999.44.3_part_2.0864
Gaiser, E. E., Bell, D. M., Castorani, M. C., Childers, D. L., Groffman, P. M., Jackson, C. R., et al. (2020). Long-term ecological research and evolving frameworks of disturbance ecology. Bioscience 70, 141–156. doi: 10.1093/biosci/biz162
Ghoul, M., and Mitri, S. (2016). The ecology and evolution of microbial competition. Trends Microbiol. 24, 833–845. doi: 10.1016/j.tim.2016.06.011
Godfrey, C. M., and Peterson, C. J. (2017). Estimating enhanced Fujita scale levels based on forest damage severity. Weather Forecast. 32, 243–252. doi: 10.1175/waf-d-16-0104.1
Goldstein, A., Turner, W. R., Spawn, S. A., Anderson-Teixeira, K. J., Cook-Patton, S., Fargione, J., et al. (2020). Protecting irrecoverable carbon in Earth’s ecosystems. Nat. Clim. Change 10, 287–295. doi: 10.1038/s41558-020-0738-8
Graham, E., and Krause, S. (2020). Social media sows consensus in disturbance ecology. Nature 577:170. doi: 10.1038/d41586-020-00006-7
Graham, E. B., and Smith, A. P. (2020). Crowdsourcing global perspectives in ecology using social media. EcoEvoRxiv [Preprint]. doi: 10.32942/osf.io/pv3w
Griffiths, B. S., and Philippot, L. (2013). Insights into the resistance and resilience of the soil microbial community. FEMS Microbiol. Rev. 37, 112–129. doi: 10.1111/j.1574-6976.2012.00343.x
Grime, J. P. (2006). Plant Strategies, Vegetation Processes, and Ecosystem Properties. Chichester: John Wiley & Sons.
Grimm, V., and Wissel, C. (1997). Babel, or the ecological stability discussions: an inventory and analysis of terminology and a guide for avoiding confusion. Oecologia 109, 323–334. doi: 10.1007/s004420050090
Gunderson, L. H., Holling, C. S., Pritchard, L., and Peterson, G. D. (2002). “Resilience of large-scale resource systems,” in Resilience and Behaviour of Large-scale Systems, Vol. 60, eds L. H. Gunderson and L. Pritchard Jr. (Washington, DC: Island Press), 3–20.
Hamer, K., and Hill, J. (2000). Scale-dependent consequences of habitat modification for species diversity in tropical forests. Conserv. Biol. 14, 1435–1440. doi: 10.1046/j.1523-1739.2000.99417.x
He, T., Lamont, B. B., and Pausas, J. G. (2019). Fire as a key driver of Earth’s biodiversity. Biol. Rev. 94, 1983–2010. doi: 10.1111/brv.12544
Hillebrand, H., Langenheder, S., Lebret, K., Lindström, E., Östman, Ö., and Striebel, M. (2018). Decomposing multiple dimensions of stability in global change experiments. Ecol. Lett. 21, 21–30.
Hobbs, R. J., Higgs, E., Hall, C. M., Bridgewater, P., Chapin Iii, F. S., Ellis, E. C., et al. (2014). Managing the whole landscape: historical, hybrid, and novel ecosystems. Front. Ecol. Environ. 12, 557–564.
Hobbs, R. J., Higgs, E., and Harris, J. A. (2009). Novel ecosystems: implications for conservation and restoration. Trends Ecol. Evol. 24, 599–605. doi: 10.1016/j.tree.2009.05.012
Hobday, A. J., Alexander, L. V., Perkins, S. E., Smale, D. A., Straub, S. C., Oliver, E. C., et al. (2016). A hierarchical approach to defining marine heatwaves. Prog. Oceanogr. 141, 227–238. doi: 10.1016/j.pocean.2015.12.014
Hobday, A. J., Oliver, E. C., Gupta, A. S., Benthuysen, J. A., Burrows, M. T., Donat, M. G., et al. (2018). Categorizing and naming marine heatwaves. Oceanography 31, 162–173.
Hodgson, D., Mcdonald, J. L., and Hosken, D. J. (2015). What do you mean,‘resilient’? Trends Ecol. Evol. 30, 503–506.
Holling, C. S. (1973). Resilience and stability of ecological systems. Annu. Rev. Ecol. Syst. 4, 1–23. doi: 10.1007/978-3-030-54560-4_1
Holling, C. S. (1996). Engineering resilience versus ecological resilience. Eng. Ecol. Constraints 31:32.
Ings, T. C., Montoya, J. M., Bascompte, J., Blüthgen, N., Brown, L., Dormann, C. F., et al. (2009). Ecological networks–beyond food webs. J. Anim. Ecol. 78, 253–269.
IPCC (2019). IPCC Special Report on the Ocean and Cryosphere in a Changing Climate, eds H.-O. Pörtner, D. C. Roberts, V. Masson-Delmotte, P. Zhai, M. Tignor, and E. Poloczanska et al. (Geneva: IPCC).
Isbell, F. I., Polley, H. W., and Wilsey, B. J. (2009). Biodiversity, productivity and the temporal stability of productivity: patterns and processes. Ecol. Lett. 12, 443–451. doi: 10.1111/j.1461-0248.2009.01299.x
Ives, A. R., and Carpenter, S. R. (2007). Stability and diversity of ecosystems. Science 317, 58–62.
Jackson, J. B., Kirby, M. X., Berger, W. H., Bjorndal, K. A., Botsford, L. W., Bourque, B. J., et al. (2001). Historical overfishing and the recent collapse of coastal ecosystems. Science 293, 629–637. doi: 10.1126/science.1059199
Jentsch, A., and White, P. (2019). A theory of pulse dynamics and disturbance in ecology. Ecology 100:e02734.
Johnstone, J. F., Allen, C. D., Franklin, J. F., Frelich, L. E., Harvey, B. J., Higuera, P. E., et al. (2016). Changing disturbance regimes, ecological memory, and forest resilience. Front. Ecol. Environ. 14, 369–378. doi: 10.1002/fee.1311
Kardol, P., Fanin, N., and Wardle, D. A. (2018). Long-term effects of species loss on community properties across contrasting ecosystems. Nature 557, 710–713. doi: 10.1038/s41586-018-0138-7
Keeley, J. E., and Pausas, J. G. (2019). Distinguishing disturbance from perturbations in fire-prone ecosystems. Int. J. Wildl. Fire 28, 282–287. doi: 10.1071/wf18203
Keeley, J. E., Pausas, J. G., Rundel, P. W., Bond, W. J., and Bradstock, R. A. (2011). Fire as an evolutionary pressure shaping plant traits. Trends Plant Sci. 16, 406–411. doi: 10.1016/j.tplants.2011.04.002
Keitt, T. H. (2008). Coherent ecological dynamics induced by large-scale disturbance. Nature 454, 331–334. doi: 10.1038/nature06935
Kemppinen, J., Niittynen, P., Aalto, J., Le Roux, P. C., and Luoto, M. (2019). Water as a resource, stress and disturbance shaping tundra vegetation. Oikos 128, 811–822. doi: 10.1111/oik.05764
Kendrick, G. A., Nowicki, R. J., Olsen, Y. S., Strydom, S., Fraser, M. W., Sinclair, E. A., et al. (2019). A systematic review of how multiple stressors from an extreme event drove ecosystem-wide loss of resilience in an iconic seagrass community. Front. Mar. Sci. 6:455. doi: 10.3389/fmars.2019.00455
Knelman, J. E., Schmidt, S. K., Garayburu-Caruso, V., Kumar, S., and Graham, E. B. (2019). Multiple, compounding disturbances in a forest ecosystem: fire increases susceptibility of soil edaphic properties, bacterial community structure, and function to change with extreme precipitation event. Soil Syst. 3:40. doi: 10.3390/soilsystems3020040
Koenig, J. E., Spor, A., Scalfone, N., Fricker, A. D., Stombaugh, J., Knight, R., et al. (2011). Succession of microbial consortia in the developing infant gut microbiome. Proc. Natl. Acad. Sci. U.S.A. 108, 4578–4585. doi: 10.1073/pnas.1000081107
Lake, P. S. (2000). Disturbance, patchiness, and diversity in streams. J. N. Am. Benthol. Soc. 19, 573–592. doi: 10.2307/1468118
Laliberté, E., Grace, J. B., Huston, M. A., Lambers, H., Teste, F. P., Turner, B. L., et al. (2013). How does pedogenesis drive plant diversity? Trends Ecol. Evol. 28, 331–340. doi: 10.1016/j.tree.2013.02.008
Lamentowicz, M., Gałka, M., Marcisz, K., Słowiński, M., Kajukało-Drygalska, K., Dayras, M. D., et al. (2019). Unveiling tipping points in long-term ecological records from Sphagnum-dominated peatlands. Biol. Lett. 15:20190043. doi: 10.1098/rsbl.2019.0043
le Roux, P. C., Virtanen, R., and Luoto, M. (2013). Geomorphological disturbance is necessary for predicting fine-scale species distributions. Ecography 36, 800–808. doi: 10.1111/j.1600-0587.2012.07922.x
Lembrechts, J. J., Nijs, I., and Lenoir, J. (2019). Incorporating microclimate into species distribution models. Ecography 42, 1267–1279. doi: 10.1111/ecog.03947
Loecke, T. D., Burgin, A. J., Riveros-Iregui, D. A., Ward, A. S., Thomas, S. A., Davis, C. A., et al. (2017). Weather whiplash in agricultural regions drives deterioration of water quality. Biogeochemistry 133, 7–15. doi: 10.1007/s10533-017-0315-z
Lytle, D. A., and Poff, N. L. (2004). Adaptation to natural flow regimes. Trends Ecol. Evol. 19, 94–100. doi: 10.1016/j.tree.2003.10.002
Mahoney, J. M., and Rood, S. B. (1998). Streamflow requirements for cottonwood seedling recruitment—an integrative model. Wetlands 18, 634–645. doi: 10.1007/bf03161678
McMullen, L. E., De Leenheer, P., Tonkin, J. D., and Lytle, D. A. (2017). High mortality and enhanced recovery: modelling the countervailing effects of disturbance on population dynamics. Ecol. Lett. 20, 1566–1575. doi: 10.1111/ele.12866
Mehran, A., Aghakouchak, A., Nakhjiri, N., Stewardson, M. J., Peel, M. C., Phillips, T. J., et al. (2017). Compounding impacts of human-induced water stress and climate change on water availability. Sci. Rep. 7:6282.
Miller, A. D., Roxburgh, S. H., and Shea, K. (2011). How frequency and intensity shape diversity–disturbance relationships. Proc. Natl. Acad. Sci. U.S.A. 108, 5643–5648. doi: 10.1073/pnas.1018594108
Milly, P., Betancourt, J., Falkenmark, M., Hirsch, R. M., Kundzewicz, Z. W., Lettenmaier, D. P., et al. (2008). Stationarity is dead: Whither water management? Earth 4:20.
Mishra, A. K., and Singh, V. P. (2010). A review of drought concepts. J. Hydrol. 391, 202–216. doi: 10.1016/j.jhydrol.2010.07.012
Mod, H. K., Le Roux, P. C., and Luoto, M. (2014). Outcomes of biotic interactions are dependent on multiple environmental variables. J. Veg. Sci. 25, 1024–1032. doi: 10.1111/jvs.12148
Mori, A. S., Furukawa, T., and Sasaki, T. (2013). Response diversity determines the resilience of ecosystems to environmental change. Biol. Rev. 88, 349–364. doi: 10.1111/brv.12004
Naeem, S., and Li, S. (1997). Biodiversity enhances ecosystem reliability. Nature 390, 507–509. doi: 10.1038/37348
Newman, E. A., Wilber, M. Q., Kopper, K. E., Moritz, M. A., Falk, D. A., Mckenzie, D., et al. (2020). Disturbance macroecology: a comparative study of community structure metrics in a high-severity disturbance regime. Ecosphere 11:e03022.
Niittynen, P., Heikkinen, R. K., and Luoto, M. (2018). Snow cover is a neglected driver of Arctic biodiversity loss. Nat. Clim. Change 8, 997–1001. doi: 10.1038/s41558-018-0311-x
Nowicki, R., Heithaus, M., Thomson, J., Burkholder, D., Gastrich, K., and Wirsing, A. (2019). Indirect legacy effects of an extreme climatic event on a marine megafaunal community. Ecol. Monogr. 89:e01365.
Paine, R. T., Tegner, M. J., and Johnson, E. A. (1998). Compounded perturbations yield ecological surprises. Ecosystems 1, 535–545. doi: 10.1007/s100219900049
Palmer, W. C. (1965). Meteorological Drought. Research Paper No. 45. Washington, DC: US Department of Commerce.
Pausas, J. G., and Bond, W. J. (2020). Alternative biome states in terrestrial ecosystems. Trends Plant Sci. 25, 250–263.
Pausas, J. G., and Keeley, J. E. (2014). Evolutionary ecology of resprouting and seeding in fire-prone ecosystems. New Phytol. 204, 55–65. doi: 10.1111/nph.12921
Pausas, J. G., Keeley, J. E., and Schwilk, D. W. (2017). Flammability as an ecological and evolutionary driver. J. Ecol. 105, 289–297. doi: 10.1111/1365-2745.12691
Peters, D. P., Burruss, N. D., Rodriguez, L. L., Mcvey, D. S., Elias, E. H., Pelzel-Mccluskey, A. M., et al. (2018). An integrated view of complex landscapes: a big data-model integration approach to transdisciplinary science. Bioscience 68, 653–669. doi: 10.1093/biosci/biy069
Peters, D. P., Lugo, A. E., Chapin Iii, F. S., Pickett, S. T., Duniway, M., Rocha, A. V., et al. (2011). Cross-system comparisons elucidate disturbance complexities and generalities. Ecosphere 2, 1–26.
Pickett, S., Kolasa, J., Armesto, J., and Collins, S. (1989). The ecological concept of disturbance and its expression at various hierarchical levels. Oikos 54, 129–136. doi: 10.2307/3565258
Pickett, S. T., and White, P. S. (eds) (2013). The Ecology of Natural Disturbance and Patch Dynamics. London: Academic Press.
Pidgen, K., and Mallik, A. U. (2013). Ecology of compounding disturbances: the effects of prescribed burning after clearcutting. Ecosystems 16, 170–181. doi: 10.1007/s10021-012-9607-2
Pirrone, N., Trombino, G., Cinnirella, S., Algieri, A., Bendoricchio, G., and Palmeri, L. (2005). The Driver-Pressure-State-Impact-Response (DPSIR) approach for integrated catchment-coastal zone management: preliminary application to the Po catchment-Adriatic Sea coastal zone system. Reg. Environ. Change 5, 111–137. doi: 10.1007/s10113-004-0092-9
Poff, N. L. (1992). Why disturbances can be predictable: a perspective on the definition of disturbance in streams. J. N. Am. Benthol. Soc. 11, 86–92. doi: 10.2307/1467885
Rillig, M. C., Ryo, M., Lehmann, A., Aguilar-Trigueros, C. A., Buchert, S., Wulf, A., et al. (2019). The role of multiple global change factors in driving soil functions and microbial biodiversity. Science 366, 886–890. doi: 10.1126/science.aay2832
Ripple, W. J., Estes, J. A., Beschta, R. L., Wilmers, C. C., Ritchie, E. G., Hebblewhite, M., et al. (2014). Status and ecological effects of the world’s largest carnivores. Science 343, 1241484. doi: 10.1126/science.1241484
Rykiel, E. J. Jr. (1985). Towards a definition of ecological disturbance. Aust. J. Ecol. 10, 361–365. doi: 10.1111/j.1442-9993.1985.tb00897.x
Ryo, M., Aguilar-Trigueros, C. A., Pinek, L., Muller, L. A., and Rillig, M. C. (2019). Basic principles of temporal dynamics. Trends Ecol. Evol. 34, 723–733. doi: 10.1016/j.tree.2019.03.007
Sabo, J. L., and Post, D. M. (2008). Quantifying periodic, stochastic, and catastrophic environmental variation. Ecol. Monogr. 78, 19–40. doi: 10.1890/06-1340.1
Salafsky, N., Salzer, D., Stattersfield, A. J., Hilton-Taylor, C., Neugarten, R., Butchart, S. H., et al. (2008). A standard lexicon for biodiversity conservation: unified classifications of threats and actions. Conserv. Biol. 22, 897–911. doi: 10.1111/j.1523-1739.2008.00937.x
Scheffer, M., Bascompte, J., Brock, W. A., Brovkin, V., Carpenter, S. R., Dakos, V., et al. (2009). Early-warning signals for critical transitions. Nature 461, 53–59.
Scheffer, M., Carpenter, S., Foley, J. A., Folke, C., and Walker, B. (2001). Catastrophic shifts in ecosystems. Nature 413, 591–596. doi: 10.1038/35098000
Seidl, R., Spies, T. A., Peterson, D. L., Stephens, S. L., and Hicke, J. A. (2016). Searching for resilience: addressing the impacts of changing disturbance regimes on forest ecosystem services. J. Appl. Ecol. 53, 120–129. doi: 10.1111/1365-2664.12511
Seidl, R., Thom, D., Kautz, M., Martin-Benito, D., Peltoniemi, M., Vacchiano, G., et al. (2017). Forest disturbances under climate change. Nat. Clim. Change 7, 395–402. doi: 10.1038/nclimate3303
Shade, A., Peter, H., Allison, S. D., Baho, D., Berga, M., Bürgmann, H., et al. (2012). Fundamentals of microbial community resistance and resilience. Front. Microbiol. 3:417. doi: 10.3389/fmicb.2012.00417
Shiklomanov, A. N., Bradley, B. A., Dahlin, K. M., Fox, A. M., Gough, C. M., Hoffman, F. M., et al. (2019). Enhancing global change experiments through integration of remote-sensing techniques. Front. Ecol. Environ. 17, 215–224. doi: 10.1002/fee.2031
Slette, I. J., Post, A. K., Awad, M., Even, T., Punzalan, A., Williams, S., et al. (2019). How ecologists define drought, and why we should do better. Glob. Change Biol. 25, 3193–3200. doi: 10.1111/gcb.14747
Słowiński, M., Lamentowicz, M., Łuców, D., Barabach, J., Brykała, D., Tyszkowski, S., et al. (2019). Paleoecological and historical data as an important tool in ecosystem management. J. Environ. Manage. 236, 755–768. doi: 10.1016/j.jenvman.2019.02.002
Smith, M. D. (2011a). An ecological perspective on extreme climatic events: a synthetic definition and framework to guide future research. J. Ecol. 99, 656–663. doi: 10.1111/j.1365-2745.2011.01798.x
Smith, M. D. (2011b). The ecological role of climate extremes: current understanding and future prospects. J. Ecol. 99, 651–655. doi: 10.1111/j.1365-2745.2011.01833.x
Song, J., Wan, S., Piao, S., Knapp, A. K., Classen, A. T., Vicca, S., et al. (2019). A meta-analysis of 1,119 manipulative experiments on terrestrial carbon-cycling responses to global change. Nat. Ecol. Evol. 3, 1309–1320.
Sousa, W. P. (1979). Disturbance in marine intertidal boulder fields: the nonequilibrium maintenance of species diversity. Ecology 60, 1225–1239. doi: 10.2307/1936969
Sousa, W. P. (1984). The role of disturbance in natural communities. Annu. Rev. Ecol. Syst. 15, 353–391. doi: 10.1146/annurev.es.15.110184.002033
Spake, R., Lasseur, R., Crouzat, E., Bullock, J. M., Lavorel, S., Parks, K. E., et al. (2017). Unpacking ecosystem service bundles: towards predictive mapping of synergies and trade-offs between ecosystem services. Glob. Environ. Change 47, 37–50. doi: 10.1016/j.gloenvcha.2017.08.004
Sutherland, I. J., Bennett, E. M., and Gergel, S. E. (2016). Recovery trends for multiple ecosystem services reveal non-linear responses and long-term tradeoffs from temperate forest harvesting. For. Ecol. Manage. 374, 61–70. doi: 10.1016/j.foreco.2016.04.037
Tedim, F., Leone, V., Coughlan, M., Bouillon, C., Xanthopoulos, G., Royé, D., et al. (2020). “Extreme wildfire events: the definition,” in Extreme Wildfire Events and Disasters, eds F. Tedim, V. Leone, and T. K. McGee (Amsterdam: Elsevier), 3–29. doi: 10.1016/b978-0-12-815721-3.00001-1
Tilman, D. (1994). Competition and biodiversity in spatially structured habitats. Ecology 75, 2–16. doi: 10.2307/1939377
Tiribelli, F., Morales, J. M., Gowda, J. H., Mermoz, M., and Kitzberger, T. (2019). Non-additive effects of alternative stable states on landscape flammability in NW Patagonia: fire history and simulation modelling evidence. Int. J. Wildl. Fire 28, 149–159. doi: 10.1071/wf18073
Todman, L., Fraser, F., Corstanje, R., Deeks, L., Harris, J. A., Pawlett, M., et al. (2016). Defining and quantifying the resilience of responses to disturbance: a conceptual and modelling approach from soil science. Sci. Rep. 6:28426.
Tonkin, J. D., Bogan, M. T., Bonada, N., Rios-Touma, B., and Lytle, D. A. (2017). Seasonality and predictability shape temporal species diversity. Ecology 98, 1201–1216. doi: 10.1002/ecy.1761
Tonkin, J. D., Merritt, D. M., Olden, J. D., Reynolds, L. V., and Lytle, D. A. (2018). Flow regime alteration degrades ecological networks in riparian ecosystems. Nat. Ecol. Evol. 2, 86–93. doi: 10.1038/s41559-017-0379-0
Tonkin, J. D., Poff, N. L., Bond, N. R., Horne, A., Merritt, D. M., Reynolds, L. V., et al. (2019). Prepare river ecosystems for an uncertain future. Nature 570, 301–303. doi: 10.1038/d41586-019-01877-1
Turner, M. G. (2010). Disturbance and landscape dynamics in a changing world. Ecology 91, 2833–2849. doi: 10.1890/10-0097.1
Turner, M. G., Romme, W. H., and Gardner, R. H. (1994). Landscape disturbance models and the long-term dynamics of natural areas. Nat. Areas J. 14, 3–11. doi: 10.1016/b978-0-08-050495-7.50006-5
van der Maarel, E. (1975). “Man-made natural ecosystems in environmental management and planning,” in Unifying Concepts in Ecology, eds W. H. van Dobben and R. H. Lowe-McConnell (Dordrecht: Springer), 263–274. doi: 10.1007/978-94-010-1954-5_22
Van Nes, E. H., and Scheffer, M. (2007). Slow recovery from perturbations as a generic indicator of a nearby catastrophic shift. Am. Nat. 169, 738–747. doi: 10.2307/4136993
Veraverbeke, S., Lhermitte, S., Verstraeten, W. W., and Goossens, R. (2010). The temporal dimension of differenced Normalized Burn Ratio (dNBR) fire/burn severity studies: the case of the large 2007 Peloponnese wildfires in Greece. Remote Sens. Environ. 114, 2548–2563. doi: 10.1016/j.rse.2010.05.029
Vicente-Serrano, S. M., and López-Moreno, J. I. (2008). Differences in the non-stationary influence of the North Atlantic Oscillation on European precipitation under different scenarios of greenhouse gas concentrations. Geophys. Res. Lett. 35:L18710.
Walker, B. H., Carpenter, S. R., Rockstrom, J., Crépin, A.-S., and Peterson, G. D. (2012). Drivers,“slow” variables,“fast” variables, shocks, and resilience. Ecol. Soc. 17:30.
Walker, L. R., Wardle, D. A., Bardgett, R. D., and Clarkson, B. D. (2010). The use of chronosequences in studies of ecological succession and soil development. J. Ecol. 98, 725–736. doi: 10.1111/j.1365-2745.2010.01664.x
Wei, X., and Zhang, M. (2010). Quantifying streamflow change caused by forest disturbance at a large spatial scale: a single watershed study. Water Resour. Res. 46:W12525.
Weintraub, S. R., Flores, A. N., Wieder, W. R., Sihi, D., Cagnarini, C., Gonçalves, D. R. P., et al. (2019). Leveraging environmental research and observation networks to advance soil carbon science. J. Geophys. Res. Biogeosci. 124, 1047–1055. doi: 10.1029/2018jg004956
Westman, W. E. (1978). Measuring the inertia and resilience of ecosystems. Bioscience 28, 705–710. doi: 10.2307/1307321
Wolkovich, E., Cook, B., Mclauchlan, K., and Davies, T. (2014). Temporal ecology in the Anthropocene. Ecol. Lett. 17, 1365–1379. doi: 10.1111/ele.12353
World Meteorological Organization (2018). WMO Climate Statement [Online]. Available online at: https://public.wmo.int/en/media/press-release/wmo-climate-statement-past-4-years-warmest-record (accessed March 1, 2020).
Yachi, S., and Loreau, M. (1999). Biodiversity and ecosystem productivity in a fluctuating environment: the insurance hypothesis. Proc. Natl. Acad. Sci. U.S.A. 96, 1463–1468. doi: 10.1073/pnas.96.4.1463
Zelnik, Y. R., Arnoldi, J. F., and Loreau, M. (2019). The three regimes of spatial recovery. Ecology 100:e02586. doi: 10.1002/ecy.2586
Zscheischler, J., and Seneviratne, S. I. (2017). Dependence of drivers affects risks associated with compound events. Sci. Adv. 3:e1700263. doi: 10.1126/sciadv.1700263
Keywords: perturbation, resistance, resilience, ecosystem stability, interacting disturbances, compounding disturbances, spatial, temporal
Citation: Graham EB, Averill C, Bond-Lamberty B, Knelman JE, Krause S, Peralta AL, Shade A, Smith AP, Cheng SJ, Fanin N, Freund C, Garcia PE, Gibbons SM, Van Goethem MW, Guebila MB, Kemppinen J, Nowicki RJ, Pausas JG, Reed SP, Rocca J, Sengupta A, Sihi D, Simonin M, Słowiński M, Spawn SA, Sutherland I, Tonkin JD, Wisnoski NI, Zipper SC and Contributor Consortium (2021) Toward a Generalizable Framework of Disturbance Ecology Through Crowdsourced Science. Front. Ecol. Evol. 9:588940. doi: 10.3389/fevo.2021.588940
Received: 29 July 2020; Accepted: 29 January 2021;
Published: 03 March 2021.
Edited by:
Enrique Martínez-Meyer, National Autonomous University of Mexico, MexicoReviewed by:
Giuliano Fanelli, Sapienza University of Rome, ItalyCorrado Battisti, Roma Tre University, Italy
Copyright © 2021 Graham, Averill, Bond-Lamberty, Knelman, Krause, Peralta, Shade, Smith, Cheng, Fanin, Freund, Garcia, Gibbons, Van Goethem, Guebila, Kemppinen, Nowicki, Pausas, Reed, Rocca, Sengupta, Sihi, Simonin, Słowiński, Spawn, Sutherland, Tonkin, Wisnoski, Zipper and Contributor Consortium. This is an open-access article distributed under the terms of the Creative Commons Attribution License (CC BY). The use, distribution or reproduction in other forums is permitted, provided the original author(s) and the copyright owner(s) are credited and that the original publication in this journal is cited, in accordance with accepted academic practice. No use, distribution or reproduction is permitted which does not comply with these terms.
*Correspondence: Emily B. Graham, emily.graham@pnnl.gov
†Denotes leadership team member