- 1Wildlife Conservation Society, Bronx, NY, United States
- 2Independent Statistical Consultant/Researcher, Taunton, United Kingdom
- 3National Trust, Swindon, United Kingdom
- 4Department of Zoology, University of Oxford, Oxford, United Kingdom
International commercial trade in wildlife, whether legal or illegal, is one of the greatest threats to multiple species of wildlife today. Opinions on how to address it are deeply divided across the conservation community. Approaches fall into two broad categories: making the trade illegal to protect against any form of commercial trade or allowing some or all of the trade to be legal and seeking to manage it through sustainable trade. The conservation community is often deeply polarized on which is the better option. We posit that a way to choose between these options is by considering species-specific attributes of biological productivity, management context, and demand. We develop a conceptual framework to assess which option is more likely to result in successful conservation of a species. We show how to construct a Bayesian Belief Network (BBN) to model how these attributes (1) interact to affect the sustainability of the species’ population and (2) vary under different trade management regimes. This approach can support scientifically based decision-making, by predicting the likely sustainability outcome for a population of a species under different trade management regimes, given its particular characteristics and context. The BBN allows identification of key points at which conservation interventions could change the potential outcome. It also provides the opportunity to explore how different assumptions about how humans might respond to different trade regimes affects outcomes. We illustrate these ideas by using the BBN for a hypothetical terrestrial mammal species population and discuss how the BBN can be extended for species with different characteristics, for example, those that can be stockpiled or when there are multiple products. This approach has the potential to help the conservation community to assess the most appropriate regime for managing wildlife trade in a transparent, open, and scientifically based way.
Introduction
Setting the Scene
International commercial trade in wildlife, whether legal or illegal, is one of the greatest threats to wildlife today (Butchart et al., 2010; Nijman, 2010; Duckworth et al., 2012; Challender et al., 2015; Eaton et al., 2015). It affects multiple species, from timber and ornamental plants, to corals, to marine and terrestrial vertebrates. In spite of the threat that trade poses to the species, their ecosystems and the benefits that flow from them, opinions on how to address this threat are deeply divided across the conservation community. A key question is how to conserve a species when it is in international trade, but that trade is not sustainable.
We focus here on terrestrial mammals harvested from the wild for international commercial trade, although the general principles apply to other taxa. Terrestrial mammals are traded internationally for food and as pets, and their parts are traded for ornamental use (e.g., ivory, claws, teeth, musk), clothing (skins, furs), and traditional medicines (e.g., tiger bones, bear gall bladders, pangolin scales). Some 915 species of terrestrial mammals are listed on CITES Appendix I or II, so are in trade and deemed to require management; approximately 40% of these are on Appendix I, so considered threatened with extinction unless all international commercial trade is prohibited (data extracted from CITES, 2019).
We consider wildlife trade to be unsustainable if harvested populations, taken in aggregate across the species’ range, show a consistent decline in numbers, are reduced to densities where they are vulnerable to local extinction, and populations no longer fulfill their ecological and socioeconomic roles (adapted from Bennett and Robinson, 2000). Unsustainable trade, by definition, threatens the survival of the target species, and also the biodiversity of their habitats, since mammals hunted for trade are often keystone species which act as pollinators, dispersers, browsers, and ecosystem engineers (e.g., Waldram et al., 2008; Blake et al., 2009; Estes et al., 2011; Ripple et al., 2014). Increasingly, intact forests with their full faunal communities and ecological functions are also seen as crucial to tackling climate change (Bello et al., 2015; Peres et al., 2016; Berzaghi et al., 2019). Many traded species are important resources for local people, and their loss may threaten the livelihoods of some of the world’s poorest and most marginalized people (e.g., Cooney et al., 2015). For very high value species, illegal international trade may be linked to organized crime, and present security threats to local communities and regional governance (Wyler and Sheikh, 2013; Duffy and Humphries, 2016).
Approaches to Conserving Species in Demand From Trade
Approaches to conserving species in demand for commercial trade fall into two broad categories; making the trade illegal to protect against any form of commercial trade (domestic and/or international), and allowing some or all of the trade to be legal and seeking to manage through sustainable trade, either local, international or both. As a particular species becomes increasingly threatened by trade, the conservation community is often deeply polarized on which is the better option, especially for high profile, charismatic species (e.g., Walker and Stiles, 2010; Wasser et al., 2010; Roe et al., 2014; Biggs et al., 2017; Felbab-Brown, 2017; Moyle, 2017).
Proponents of a sustainable commercial trade argue that wildlife will only be conserved if it has a legitimate economic value, to mitigate against converting natural habitats to farmland (e.g., Stiles, 2004), to give incentives to local people to conserve wildlife (Bulte et al., 2003; Child, 2012; Biggs et al., 2013; Cooney et al., 2015), and so that the proceeds of high-value sales can support conservation efforts that benefit the species concerned, and other species, and offset the costs of enforcement (Child, 2012; Biggs et al., 2013; Di Minin et al., 2014). Further, sustainable trade to meet high levels of demand is proposed as a way to overcome negative cycles of increasing prices in underground markets that can occur with restricted legal trade, with resulting poaching and violence (‘t Sas-Rolfes, 2000; Challender and MacMillan, 2014).
In addition to ethical concerns (e.g., Pastor, 2010), opponents of trade argue that the presence of any markets creates demand that cannot be met sustainably (Maisels, 2012; Lusseau and Lee, 2016). If legal supplies are limited, this increased demand might spill over into an illegal market, especially if consumers cannot easily distinguish between legal and illegal products. Further, the presence of legal markets potentially makes smuggling and sale of illegal goods easier because illegal supplies can masquerade as legal ones. Corruption-enabled laundering of illegitimate items into legitimate markets also means that protection of the species across parts or all of its range is challenging (Gabriel et al., 2012; Bennett, 2015).
The pro-trade and anti-trade proponents are often deeply entrenched in their views, partly due to underlying differences in philosophy (Roe et al., 2014) and values (Biggs et al., 2017), making discussions surrounding CITES Conferences of Parties, for example, often extremely heated, with little room for compromise (e.g., McGrath, 2013; Kahumbu, 2015).
Assessment of the relative merits of each approach is hampered by the fact that, for terrestrial mammals, both are currently largely failing (Felbab-Brown, 2017). Indeed, it is rare to find an example of a species of terrestrial mammal with any level of commercial demand whose wild population has been stable or increasing over the past 20 years. Compared to many other plant and animal taxa, productivity of mammals is relatively low, especially of the larger species with low reproductive rates and long maturation times (Robinson and Redford, 1986; Read and Harvey, 1989). If such species face high levels of commercial demand for lethally harvested products, conservation is challenging, whichever strategy is employed. This is especially true for high-value species whose illegal trade often involves organized crime networks, facilitated by high levels of corruption (Wyler and Sheikh, 2013). Indeed, circumnavigating wildlife trade regulations is often characterized as high profit and low risk (Goncalves et al., 2012; Wyatt and Cao, 2015). Agencies responsible for wildlife management around the world are notoriously under-staffed and under-funded (Bennett, 2011), and the absence of strong institutional structures gives the opportunity for over-exploitation (Fischer, 2010).
Examples of trade bans leading to stable or increased populations of terrestrial mammals are scarce, and causation is often hard to attribute given that species generally face multiple, interacting threats. The fur trade was clearly the major threat to many species of big cats, and has declined greatly since they were listed on Appendix I of CITES in 1975 (IUCN, 2000), and hunting for trade is no longer their primary threat (e.g., Caso et al., 2008; Goodrich et al., 2015), with the possible exception of the snow leopard in parts of its range (McCarthy et al., 2017). Full legal protection and a CITES Appendix I listing of the giant otter (Pteronura brasiliensis) allowed the species gradually to recover in the Peruvian Amazon (Uscamaita and Bodmer, 2010). Trade bans are not always successful, however. The announcement of trade bans can stimulate trade prior to their coming fully into effect as people anticipate the ban (Rivalan et al., 2007), and can subsequently send trade underground rather than stopping it (Rosen and Smith, 2010). All international commercial trade in the Sunda pangolin (Manis javanica) and Chinese pangolin (Manis pentdactyla) has been banned since 2000 when CITES established zero export quotas for both species and subsequently listed both species on Appendix I; yet between 1996 and 2014, the status of both on the IUCN Red List of Threatened Species went from Least Concern to Critically Endangered, almost entirely due to illegal hunting feeding into international trade due to the combination of lax enforcement and corruption (Challender et al., 2019a, b).
Examples of sustainable international trade in terrestrial mammals leading to conservation of the target species are also scarce. International trade in fur-bearing animals from the US and Canada is well managed, and the current strict management program has contributed to the recovery of various species from historical unregulated trade (White et al., 2015). The best documented example of a single population of a species being conserved under a sustainable trade regime is skins from collared (Tayassu tajacu) and white-lipped (T. pecari) peccaries from the Peruvian Amazon, used in high-end gloves and shoes in Europe (Bodmer and Puertas, 2000; Bodmer and Fang, 2016); the impact of such trade on the species in other parts of their range is unclear but is probably not great since demand for skins in international markets is limited, and largely supplied by legal trade. Both of these examples involve intensive management and monitoring at multiple levels, with highly controlled hunting and trade, and rigorous monitoring programs along the trade chain including, in the latter case, a sophisticated chain of custody program. Other examples of long-term successful conservation of species in trade are scarce. One case previously deemed a success was the vicuña (Vicugna vicugna). Vicuña wool is in demand for high-end trade, and by 1994, over-hunting had reduced their numbers to about 5,000 animals. This resulted in all trade being banned under CITES. Programs of live-shearing by local communities for sale to international markets were then introduced, with local communities regarding the species as a valuable local resource, enabling them to benefit from trade. This led to successful conservation of some populations of the species, and their being downlisted to CITES Appendix II; by 2010, numbers had increased to more than 200,000 animals (McAllister et al., 2009; Lichtenstein, 2011). However, since then poaching has increased significantly because the open markets allowed the laundering of wool from illegally hunted animals since hunting is cheaper and easier than live-shearing, and hunters threatened local communities who anyway were only gaining a small percentage of the end-market price The situation was compounded by porous international borders and weak legislation (Nuwer, 2015). Hence, although the species’ status has been lowered to “Least Concern” by IUCN, conservation programs and tight control of the ongoing legal wool trade at local, national and international levels are deemed essential for the species’ continued viability (Acebes et al., 2018). Although this example is one in which animals do not need to be killed for their products to be traded, there are not many other situations in which live-harvesting of products to supply legal trade is possible. This is because the acquisition of most products (e.g., bones and tusks) leads to the death of the animal or, in the case of pets, removal from the wild. There are a few other instances, e.g., rhino horn, where live-harvest is possible; in these cases productivity over the life time of the animal can be much greater (‘t Sas-Rolfes and Fitzgerald, 2013).
One core reason why neither approach has been unambiguously successful in conserving species is that both trade bans and management of a regulated trade depend on high levels of management along the trade chain, especially if biological productivity is low and demand is high. Bans require the ability to prevent hunting, trafficking and illegal sales of wildlife along trade chains. Various localized examples show that strict site-based protection can result in successful conservation of species demanded in trade (e.g., Linkie et al., 2015; Global Initiative against Transnational Organized Crime, 2020), although such examples are rare. Sustainable trade requires transparent management and rigorous, long-term monitoring to ensure that offtakes are truly sustainable, and management capacity at all points that can easily distinguish legal, sustainably sourced items from unsustainably or illegally sourced ones. At all parts of the trade chain, the presence of organized crime presents a further management challenge, especially in the context of high levels of corruption. Such criminals are more likely to be involved in trades in items with high individual values, but those could be either species for which there is no legal trade (e.g., rhinos), or ones with potential parallel legal and illegal trades (e.g., musk deer).
In this paper, we posit that there is way to choose between the two options; by disaggregating the issue, we can develop a framework to assess which approach is more likely to result in successful conservation of any particular species. We first describe three categories of attributes of species and their management, and how these might be expected to affect the outcome of either legalizing or banning trade, as reflected in biological sustainability at the species level. We then introduce a modeling framework which would enable users to explore how these attributes interact to produce different outcomes for the two options. Recognizing that these attributes require joint consideration (Cooney et al., 2015), we use a Bayesian Belief Network (BBN) (McCann et al., 2006). This both recognizes the interactions between attributes, and allows identification of key points at which conservation interventions could change the potential outcome. We show how this framework could be used to support scientifically based decision making, by predicting the likely outcome in terms of the sustainability of a population of a hypothetical traded species under different trade management regimes.
Our approach does not model how human behavior will change in response to different management regimes within the BBN. Much of the controversy around the wildlife trade concerns how humans will behave in response to particular decisions and circumstances (Walker and Stiles, 2010; Roe et al., 2014; Biggs et al., 2017). Given that we are building a decision support tool, we did not want to hard-wire human behavior into the model, because this then builds in the controversies, rather than enabling users to step back from them. Instead, our approach makes it possible to explore how outcomes could differ depending on the assumptions being made about human behavior. For example, does a species population remain sustainable if we assume that consumer preferences change when a ban is implemented? What happens if consumer preferences do not change? The model allows the testing of such questions, and many more.
Over time, we hope that the model will be applied to different species with various types of trade structure, since solutions have to be customized to specific conditions (Felbab-Brown, 2017). As that happens, the model itself will inevitably evolve to incorporate other attributes, with different emphasis on those attributes of most importance to the species under consideration. Finally, we suggest next steps for operationalizing this model.
Key Species Attributes and Their Relevance to Management Options
The attributes relevant to management of a species in demand for commercial trade are complex, inter-related and influence each other. IUCN (IUCN, 2000) categorized attributes relevant to sustainable use into productivity, management control, and demand. We tweak those categories into: biological productivity, management context, and demand.
Different species in trade vary greatly according to these attributes (Figure 1). Hedgehogs are not in demand for trade, are subject to negligible management, and have a relatively high productivity. By contrast, peccary skins are traded, they also have relatively high productivity, management of the trade from the Peruvian Amazon is intense and of a high standard, and demand for the skins in international trade is relatively low (Bodmer and Fang, 2016). This combination of attributes means that trade is sustainable (Bodmer and Puertas, 2000; Bodmer and Fang, 2016). Productivity of vicuna wool is also relatively high since the animals do not have to be killed to acquire it, yet management in some areas is failing, and demand for the wool relatively high. Under this combination of attributes, trade overall is becoming unsustainable (Nuwer, 2015). Productivity of ivory from African elephants is extremely low (Maisels, 2012; Lusseau and Lee, 2016), management to prevent poaching and trafficking is also low across much of the species’ range, and demand for ivory over the past ten years has been extremely high (UNEP et al., 2013). That combination of attributes has led to unsustainable trade and major declines in elephants across large parts of their range, though not all Maisels et al., 2013; Thouless et al., 2016; CITES, 2017). North American bobcat and lynx are killed for their furs, but as cats they are relatively fecund, and management is sufficiently good that exploitation remains sustainable (White et al., 2015). Productivity of both Chinese and Sunda pangolins is low, management along the trade chain extremely poor, and demand for meat and scales very high; the result has been catastrophic declines of both species (Challender et al., 2019a, b).
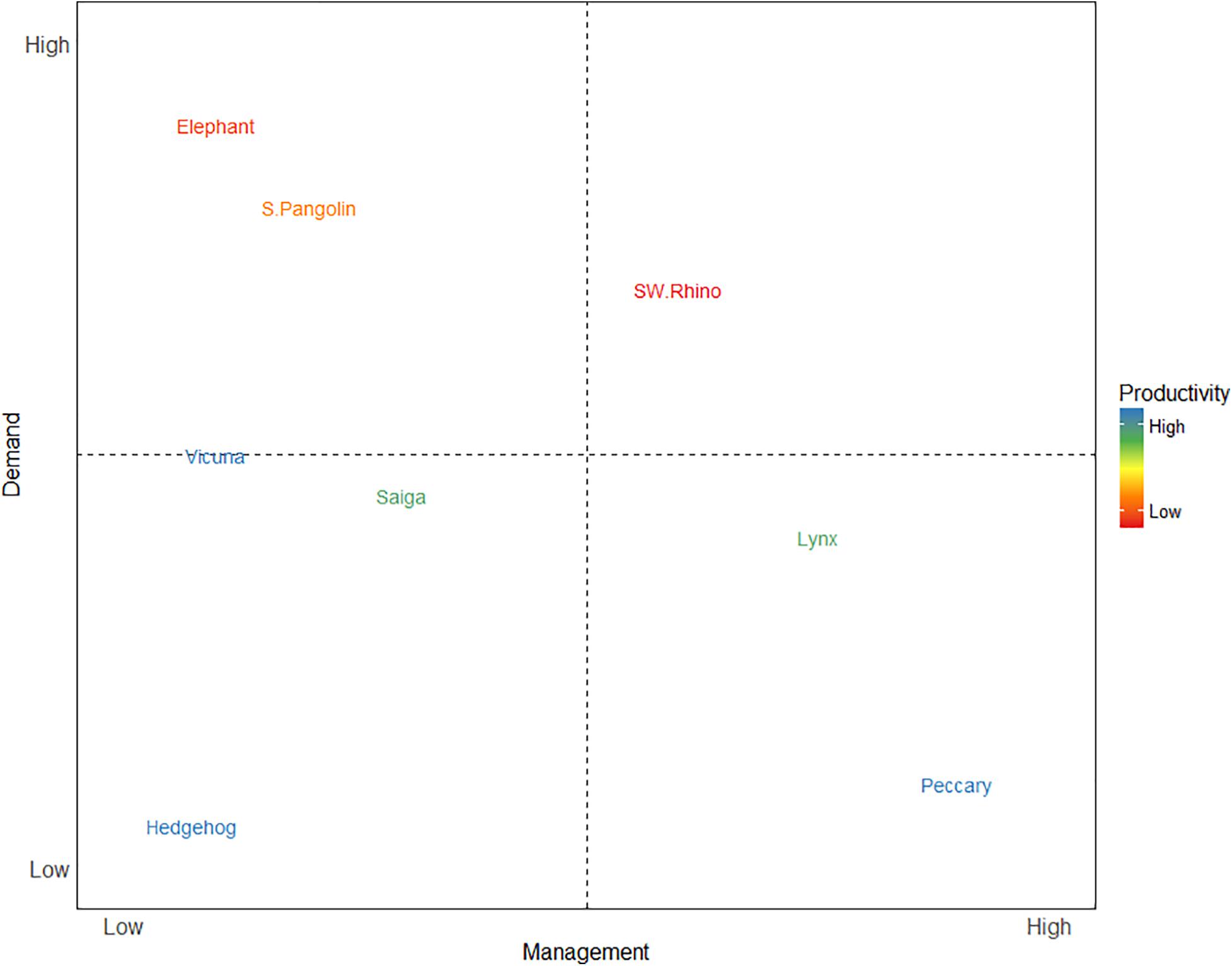
Figure 1. Productivity, demand, and management for different species (S. pangolin = Sunda pangolin; SW rhino = southern white rhino). Species are likely to have a more sustainable future if the demand is low and the management effectiveness is high, but the relative importance of these, hence which policies should be adopted, depend on productivity. This figure does not help in determining whether a trade ban or managed legal trade will be more effective at improving species sustainability. It does highlight which species are most likely to need help—those in the top left corner with low productivity. Please note, these results are illustrative and qualitative.
Any species could be situated within Figure 1 and its location will change as different policies affecting management or demand are enacted. For a species with low biological productivity and high demand, improving management should lead to a more sustainable future for a species. In comparison, if productivity of the traded item is high and demand is low, management might not need to be so intense for the species to be sustained. This characterization is useful in gaining a first insight into how different species might perform, and which species are most at risk, but it does not assist decision makers in determining whether a regulated trade or a ban is likely to be more effective at ensuring long-term conservation of the species. That requires a probabilistic comparison of the two approaches within a modeling framework, which allows for: (1) investigation of how changing any attribute feeds through the system to affect the potential outcome; and (2) understanding which of the attributes are most important for ensuring sustainable management of the species populations across their range.
Modeling Approach
We propose that construction of a modeling framework would allow for an objective discussion around management options. A challenge in doing this is that data for many species on some or all of the attributes are poor, and also people’s views on what are the most important determinants of outcomes differ, e.g., whether enforcement or local community involvement is more critical to effective site-based management. So a framework is needed which is flexible enough to include these different views and can incorporate uncertain knowledge. This framework should be applicable to a wide range of different species threatened by unsustainable trade. It should also be transparent and easy to visualize the results, allowing users who might have divergent views to explore different policy options and see their likely outcomes.
Here we demonstrate how a Bayesian Belief Network (BBN) may provide an appropriate modeling framework for describing the interactions between the different attributes of the wildlife trade, for comparing different management strategies for traded wildlife, and how these strategies might impact the sustainability of a given species’ population. We describe the key components of a BBN and how it could be applied to understanding the wildlife trade. The BBN we present is for illustrative purposes and does not capture all of the subtleties of actual scenarios. Neither does it include any real data because at this initial stage we wish to present a general concept and framework for consideration, rather than focusing on the potentially distracting specifics of any one species or set of species. Hence, we demonstrate its basic purpose and outline, then discuss how such a tool could be developed in practice and applied to specific cases.
Bayesian Belief Networks are simplified models that capture probabilistic relationships between variables (Cowell et al., 1999; Jensen, 2001; Aguilera et al., 2011). They have been applied to problems in natural resources management (Cain, 2001; McCann et al., 2006; Castelletti and Soncini-Sessa, 2007; Zorilla et al., 2009) and risk assessments of wildlife populations (Marcot et al., 2001; Pollino et al., 2007). Koen et al. (2017) used a BBN to understand rhino poaching in Kruger National Park. They have also been proposed as a strategy for understanding the illegal ivory trade (Burn et al., 2003; Martin et al., 2012). BBNs are particularly useful for contentious and data-poor situations, because they are relatively easily visualized and so are a good foundation for participatory modeling in which the effect of differing assumptions about attribute identities, values and interactions can be explored by knowledgeable researchers and practitioners (Düspohl et al., 2012; Saliou et al., 2017).
Structure of the BBN
The first step is to build the structure of the BBN. This requires identification of the different variables in the model, the values that they can take, and indicates which variables are related to each other (but not how). The different variables in a model (e.g., population density, illegal offtake, and demand) are represented in a BBN as nodes. The actual nodes can be modified as the BBN for any one species and type of trade is developed, so this is illustrative of the general approach. Each node can take several values—known as states. A node might have only two states; e.g., in Figure 2, the node Illegal Offtake might only take the values high and low, or it could be a continuous variable with an infinite number of states. Dependencies between the different nodes are represented by directed edges. For example, the arrow from the nodes Illegal Demand and Illegal Supply to Illegal Offtake indicates that these first two nodes have an influence on Illegal Offtake. Illegal offtake could be the point at which illegal supply and illegal demand curves intersect and the nodes describe these curves and their intersection. The node Illegal Offtake is then described as a child of its parents: Illegal Demand and Illegal Supply. Cyclical relationships are not allowed as they are logically impossible, although it is possible to represent feedbacks using a dynamic BBN where for example offtake in one timestep is a function of demand in the previous timestep. The nodes, their states and the directed edges represent the structure of the BBN. This structure is also known as a directed acyclic graph (DAG) or graphical model (Pearl, 1985).
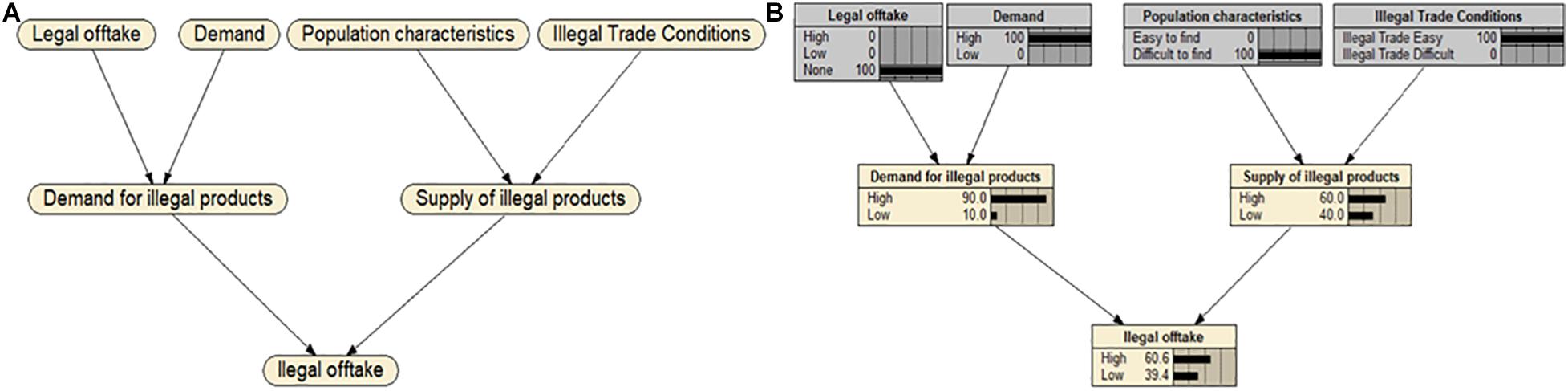
Figure 2. Example of a small BBN which could form part of the BBN for the illegal wildlife trade. (A) The relationship between different nodes. (B) The states of each node and the probability of being in each state. The gray boxes show findings—where the states of the nodes are set. Here, there is a 60.6% chance that Illegal offtake is high when there is no Legal Offtake, Demand is High, Population is Difficult to find, and Illegal trade conditions are Easy. These are illustrative probabilities only. The Basic Model section provides further reasoning for this BBN.
Quantifying the Relationships in the BBN
The relationship between nodes, as indicated by the directed edges, may be deterministic or, more usually, specified by a Conditional Probability Table (CPT). Each child node has a CPT that describes the conditional probability that the node is in a particular state, given the states of all its parent nodes. The CPT for the child node depends only on its parents and no other nodes in the BBN; not even its grandparents. For example, in Figure 2 if Illegal offtake, Illegal supply and Illegal demand each only have two states—high and low—then the probability that Illegal offtake is high is 0.20 if Illegal Supply is high and Illegal demand is low. The full set of conditional probabilities for this example is given in Table 1. The CPTs do not need to know about the state of grandparent nodes, for example Illegal Trade Conditions or the probability that overall Demand is high. This powerful property of BBNs, known as conditional independence (Jensen, 2001), makes it possible to construct complex BBNs out of many small sub-models, because only the direct relationships between children and their parents need to be quantified.
Building the BBN
Constructing a BBN is a trade-off between providing enough detail to capture the main features of the trade, and not becoming too detailed and unwieldy. Typically BBNs are constructed by thinking about outcomes of interest—in this case whether a population is sustainable—and then identifying the variables (parent nodes) that influence these outcomes. This becomes an iterative process as the parents of these variables are then identified. The BBN’s construction can be informed by a series of conceptual models built at different scales or different groups of experts and stakeholders Cain (2001).
Bayesian Belief Networks work best when nodes each have small numbers of parents, and, unless they are continuous, when nodes have few states; otherwise it can be difficult to define the CPTs, or the BBN may become intractable (as highlighted by Marcot et al., 2001).
Developing a working BBN would require engaging a group of experts to refine our provisional model (shown in Figure 3). Koen et al. (2017) describe a process by which BBNs could be developed and built by experts. For our BBN, experts would, for example, assist in: (1) clarifying the structure of the model, the main linkages and those about which there is debate; (2) identifying strategies for obtaining the CPTs to quantify the relationships between these different components; and (3) validating the BBN to ensure that it realistically reflects trade in the particular species concerned.
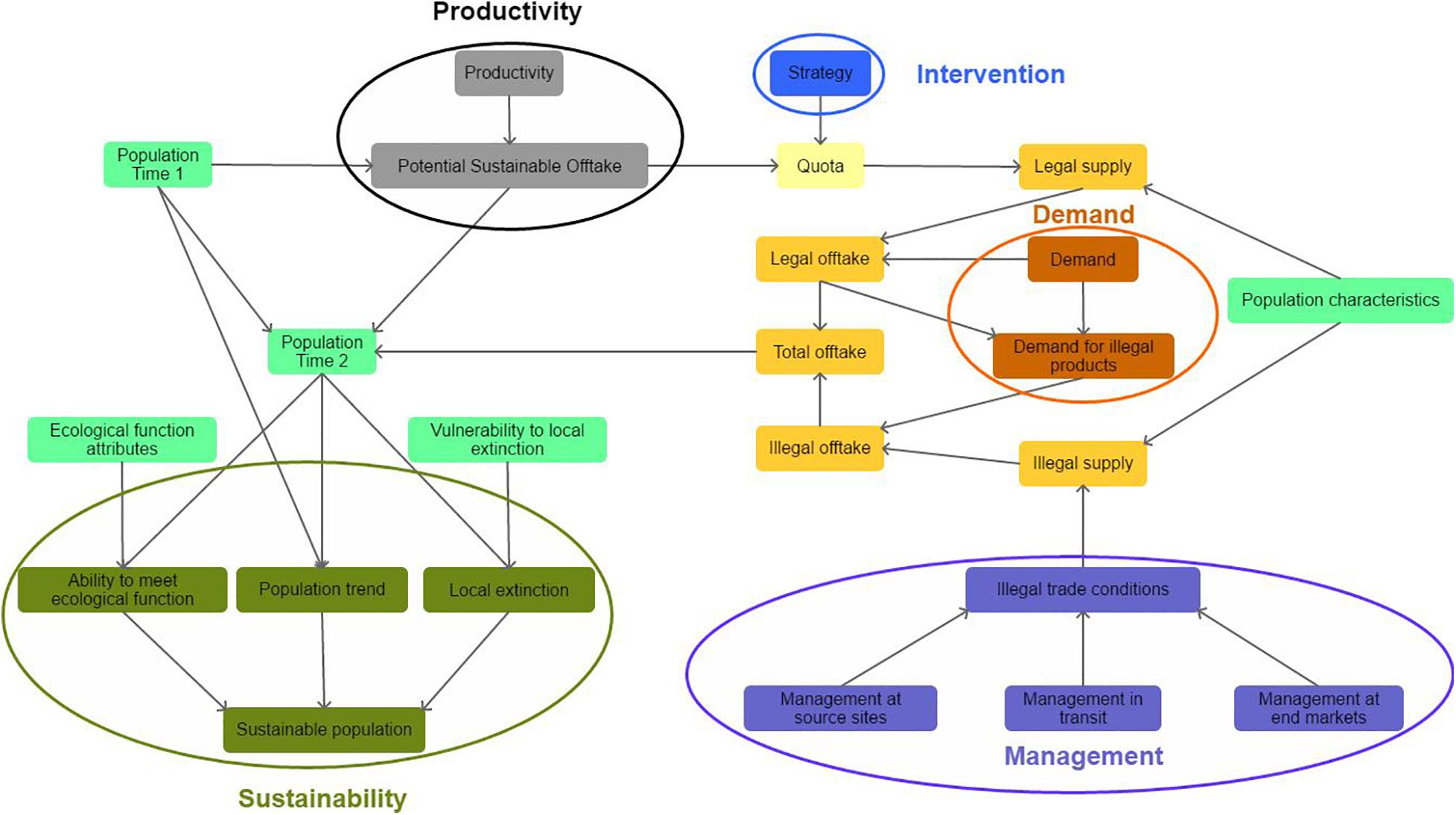
Figure 3. BBN representing the relationships between productivity, demand and management on the sustainability of the population. Nodes describing biological characteristics are colored bright green and nodes colored bright yellow describe supply and offtake.
A variety of strategies can be used to obtain the CPTs. Because of conditional independence, different parts of the model can be constructed using different data sources and types of information. Analysis of primary data might provide insights into some components of the model (Halls et al., 2002; Underwood et al., 2016), while the scientific literature may provide information on other links (Johnson et al., 2014). Where there are data and knowledge gaps, which might suggest future research priorities, consultations can elicit the CPTs from experts (Cain, 2001; Ticehurst et al., 2011). As an example, Koen et al. (2017) used a combination of expert knowledge, scientific literature and data sources to populate the CPTs of their BBN.
Once a model has been constructed and tested, the CPTs for a given species can be updated as new information becomes available, using BBN learning (e.g., Neapolitan, 2004). This might be particularly useful when there is limited current information for a particular species and more information arrives over time.
Using the BBN
Once the CPTs have been specified, probabilistic inference can be used to “interrogate” the BBN to answer different questions. That is, given the state of one or more nodes, known as findings, the probabilities of the states of all other nodes in the network can be calculated. There may be one or more nodes of particular interest, often called target nodes. Like findings, target nodes can fall anywhere in the BBN. Three different types of questions can be asked. The first is predictive reasoning—the likely outcomes of a child node given the states of parent nodes with no ancestors. In Figure 2B, an example of predictive reasoning is given, showing the probability of high and low illegal offtake for a species that is difficult to obtain and for which there is high demand, no legal trade and it is easy to trade the species illegally. Diagnostic reasoning is the converse - what is the most probable state of preceding nodes given the known state of a node with no children. For example, using the small subnetwork in Figure 2, what combination of legal offtake, demand, ease of obtaining the species and illegal trade conditions is best in order for illegal offtake to be low? A third type of question is sensitivity analysis—for example, whether the probability of high levels of illegal offtake changes more when the amount of legal trade changes or when the ease of illegal trade changes.
Model
Basic Model
Figure 3 is a representation of how our framework might look for a single species, traded for a single purpose, and for which there is no stockpiling of the traded products. The aim of this BBN is to model how an intervention (in this case, implementation of either a sustainable trade regime or a ban) might affect a population. In a simple model, this could be the total population of the species as a whole, but it might be necessary to model individual populations, especially for species with broad distributions if management varies greatly across their range. The BBN is dynamic because it considers the size of the population prior to the intervention (Population_T1) and then models the change in the population numbers due to the intervention to give the population in the next timestep (Population_T2). The effect of the intervention on the population is measured by our definition of sustainability—specifically what is happening to the population trend, whether the population is vulnerable to local extinction, and whether it fulfills its ecological functions. All three outcomes are affected by the size of the population after the intervention (Population_T2) and its other characteristics.
It is important to recognize that the timesteps in this BBN are not the same as those in many standard models that represent system dynamics (such as a population model or harvesting model, in which annual timesteps may be used to represent population change over time, and therefore infer the sustainability of harvesting). In our BBN, the nodes and links represent a cumulative understanding of the system’s dynamics (e.g., the trend in population size), in order to infer sustainability for decision-making purposes. The second timestep represents what happens to the sustainability of the system as a result of an intervention (such as a trade ban), enabling comparative analysis of policy scenarios. The BBN could be extended to include further time-steps, iterating this process, to investigate, for example, the impact of different sequences of interlinked policy interventions. These further timesteps therefore would refer to time-periods over which the sustainability outcomes of given intervention(s) are being considered. They could be short-term, such as a year, or long-term, such as several decades, depending on the speed of system change in response to interventions and the resolution of the relevant datasets.
The change in population size between the two time periods due to the intervention is captured by the relationship between Total Offtake and Potential Sustainable Offtake. The latter is a function of Productivity and Population Size, assuming that the population is otherwise stable and not affected by major habitat loss or environmental pressures such as drought. Total Offtake is a combination of both Legal Offtake, where relevant, and Illegal Offtake (assuming that other sources of human-induced mortality, such as problem animal control or habitat loss, are accounted for in productivity estimates, or modeled as separate components or nodes in a more complex model). Both legal and illegal offtake can be modeled as a function of the supply of and demand for goods.
One approach to legal supply is to set a quota determined by potential sustainable offtake which depends on productivity and the current population. Legal supply also depends on other population characteristics, which determine how easy it is to harvest individuals (the catchability). For example, if the quota is high but catchability is low (for example, because the species is cryptic), then supply will be lower. In our simple example, we assume that demand is first met from legal sources, then any unmet demand becomes a demand for illegal goods.
Beyond the biological productivity of the species, the supply of illegal goods depends mainly on illegal trade conditions. The occurrence and scale of illegal trade depends on management at source sites, transit routes and markets. For example, if demand is high but management along the trade chain is good, it is difficult for illegal goods to be obtained, transported and sold and illegal offtake will be low. Hence, management regulates the supply of illegal products.
Using predictive reasoning, the BBN could answer a question such as: for a species with a small population, low productivity, high demand, good management and a trade ban in place, what is the probability that the population can be maintained sustainably? Diagnostic reasoning could be used to answer a question such as: what is the best configuration of productivity, management structure, demand and trade regulation that would allow a population to be managed sustainably?
More generally, questions combining predictive and diagnostic reasoning can be asked, making it possible to investigate:
(1) the changes to the sustainability of the population under either a ban or regulated trade, given the current management structure, demand and biological productivity of the species;
(2) the best strategy for sustaining the population, given its biological productivity and current management structure and demand;
(3) the best configurations of productivity, current management and demand for maintaining sustainable populations under either a ban or regulated trade.
Components and Extensions of the Model
Although the model that we present here is tentative and preliminary, it captures our key proposition: that by considering the mechanisms by which productivity, demand and management interact, we can investigate how different conditions affect the sustainability of the population and the relative effectiveness of a ban or managed trade for a particular species. Many aspects of the model shown in Figure 3 would need further expansion for it to allow anything other than the most basic type of enquiry. For example, the BBN models a species rather than a product, since it is the conservation of the species that is the primary management goal. The model described above considers a single species which is traded for a single purpose. To model a species traded for multiple purposes, (e.g., pangolin meat and pangolin scales) and/or by different consumer groups, which might therefore also potentially follow different trade routes, the BBN would need separate nodes for each product and/or consumer group and/or transit route which then combine to a species-level node for offtake. More generally, the BBN can capture information about different source populations, varying degrees of management along different parts of a trade route and different consumption patterns in more or less detail, depending on the spatial and temporal resolution at which the model is to be used.
The model could also include other threats that act on the species, or be extended to include drivers of productivity, management and demand. This can be important because it is often these underlying threats and drivers, rather than the implementation of a ban or regulated trade, that conservation bodies can influence and that ultimately will influence the outcome. As such it helps to be able to understand the relative importance of different drivers. To illustrate this, we here describe some potential drivers of productivity, management and demand and how they would be represented as ancestors of these nodes in the BBN.
Total Productivity
The base level of biomass productivity [measured in kg of the traded product(s)] varies greatly with habitat; in comparison to tropical grassland and savannah habitats, for example, tropical forests have a very low potential productivity of mammal biomass/km2 (Robinson and Bennett, 2004). Within this, the productivity of individual species is a function of their life history, and of the history of exploitation in a given site. If a species occurs in multiple different locations, underlying productivity may vary between locations due to different ecologies or exploitation histories, potentially requiring a BBN that incorporates multiple locations in order to draw species-level conclusions.
Productivity also depends on whether the animal has to be killed to obtain a traded item. If the species can be live-harvested (e.g., for wool and horn), productivity (in terms of biomass per individual) may be higher. A white rhino can produce eight times as much horn in its lifetime if the horn is live-harvested periodically than if the animal is killed for a one-time harvest (‘t Sas-Rolfes and Fitzgerald, 2013).
Expanding the BBN to recognize drivers of productivity could include parent nodes for carrying capacity and the rate of population increase. The total standing population is a function of population density and geographic range and these could, potentially be included as parent nodes of the population size node. These parameters would relate only to that part of the population that can be traded.
Management Context
The management context is critical in determining the ability to manage legal supplies and control illegal ones. Weak management at any point in the trade chain potentially undermines both regimes of no trade or regulated trade. For example, management at sites where trade is permitted might be good, but if management at sites where trade is not permitted is poor, illegal trade can still occur, based on animals sourced from these other sites. As such, the BBN may need separate nodes for different source sites, transit routes and end markets.
Various attributes could be included in the model to represent the quality of management. These could include the capacity of the management agency (e.g., government or non-government agency, local community, and private landowner). Alternatively, the model could use a composite measure of capacity as captured in tools such as Protected Area Management Effectiveness (PAME) (Coad et al., 2015). Along the trade chain, whilst in transit, the primary management responsibility generally lies with customs agencies. At the market end of the trade chain, in many countries, the legal responsibility for enforcement often lies not with the wildlife authorities, but with transportation or urban authorities whose training and focus on wildlife management is negligible (Bennett, 2011).
In our example BBN, a first set of nodes could describe whether managers have:
(1) the legal mandate to manage—for example, whether local communities have clear legal ownership rights over the wildlife resources at sites;
(2) the capacity to manage – including the funding, technical skills, equipment, staffing levels and leadership needed to operate.
A second set of nodes could describe how effectively management can operate both legally and in general, given the mandate and resources. These factors describe the environment within which managers are operating and can apply to specific sites or to countries or regions. For example:
(1) Legal effectiveness. Without a legal framework such as the presence of appropriate legislation, a functioning judiciary and prosecution process, there is no ability to enforce a ban or manage a legal trade. Thus, two source sites from different countries might fall under the same legal mandate to manage the site, but if the legal framework is not in place, their mandate is not meaningful;
(2) Management effectiveness. Management effectiveness depends on the environment within which agencies are operating, especially the levels of governance and corruption. Corruption among government officials charged with implementing wildlife-related legislation plays a major part in facilitating illegal wildlife trade (Elliott, 2007; United Nations Office of Drugs and Crime [UNODC], 2010; Milliken and Shaw, 2012; Bennett, 2015; Smith et al., 2015; Wyatt and Cao, 2015; Felbab-Brown, 2017), both for species for which all commercial trade is illegal, and also those with a managed legal trade with the potential for illegally obtained items to be laundered into a legal market (Bennett, 2015). Thus, two source sites could have the same management capacity and the same legal mandate in countries with the same legal framework but could function very differently because of differing levels of corruption. Levels of corruption could be represented by the World Governance Indicators (World Bank, 2011). Other variables describing the socio-economic environment within which management is operating, such as GDP, inequality, and levels of poverty and alternative livelihood opportunities, may also be relevant here.
These nodes would share children with variables describing the capacity for management and the presence of a legal mandate to provide overall measures of management ability to control illegal trade.
A further set of attributes which affects the ability of management to control the illegal trade relates to properties of the product itself and how easy it is to identify. Specifically:
(1) Is it distinguishable from similar products from different species? If products look similar but have different legal status, it is difficult for managers to ensure that illegal products are not passing along the trade chain. This could be included in the BBN by including a node that represents the ease of identifying the species, or product. One parent of this Ease of identifying species node could be the legal status of other similar products in trade.
(2) Geographic range: For species with large geographic ranges spanning many countries, the challenge for management is greater if items from unsustainable or illegal sources are to be prevented from entering trade chains with sustainable, legal ones. Species with broad geographic ranges might have larger total populations hence more able to support a sustainable trade (Cooney et al., 2015), but ensuring that all of that trade is from legal and sustainable sources over a wide area is challenging. Thus geographic range might have an influence on the effectiveness of trade route management in addition to its effect on potential sustainable production.
Additional attributes might be specific to source, transit or end markets. For example, at source sites it might be important to include a node that describes whether local communities benefit in some way from the wildlife and wild lands, whether through some regime of sustainable offtake, or from non-consumptive uses such as tourism. Management of transit routes could potentially include a node that describes the number of potential trade routes – whether egress from a site is only through one mountain pass, or across multiple highly porous borders. Distance from a porous international land border could potentially be a node to describe ease of management at markets.
Demand
Many different factors affect the level of demand for a species. Three key factors are:
(1) Consumer preferences. These can be positive - an item is preferred because it is fashionable, prestigious, and/or fulfills cultural needs. They might also be negative, if the item is socially unacceptable. Rarity of a species can also affect consumer preferences; some species are in demand because of their extreme rarity, and can fetch high prices (Courchamp et al., 2006). Conversely, consumers might prefer to buy common species if they believe that they are not harming the population;
(2) Availability of acceptable substitutes. This determines elasticity of demand (Conrad, 2012). If alternatives are available, then once price goes above a certain level, demand for the species will drop as people seek alternatives. If acceptable substitutes are not available, for example, if buyers seek an item from the wild that has no substitutes because of its extreme rarity or due to the cultural belief in its unique medicinal efficacy, then demand might continue to increase even if prices are extremely high (Verheij et al., 2010; ‘t Sas-Rolfes and Fitzgerald, 2013);
(3) Wealth in the end-market. A key driver of demand is the degree of poverty or wealth in potential markets. For example, increased per capita spending in China is correlated with increased poaching of elephants in Africa (CITES, 2012).
In the BBN, demand could therefore be separated into three separate nodes describing consumer preferences, availability of alternatives and consumer wealth. The BBN aims to model a species rather than a product, since it is the conservation of the species that is the primary management goal. Thus, if a species is used for multiple purposes, for example pangolin meat and pangolin scales, and/or by different consumer groups, the BBN would need separate nodes for each product and/or consumer group, which then combine to a species-level node for offtake.
Extending the Model to Include Stockpiles
The BBN described in Figure 3 could be extended to be relevant to species whose trade involves stockpiles of the products. Non-perishable wildlife products can be stockpiled by globally distributed networks of buyers (Eriksson and Clarke, 2015). For mammals, such products include tusks, horns and, to some extent, furs and pelts. Stockpiles can act as a buffer between consumers and biological populations, leading to a time delay between changing demand and offtake. This can lead to a less clear signal between supply and demand. Thus the BBN could be extended to represent a three-time-step process so that the consequences of this buffering can be more easily measured.
Some stockpiles are owned and managed by governments, or other bodies which are registered or legally managed. In theory, at least, there is a clear mechanism by which products from stockpiles enter the trade chain. There is, however, evidence of leakages from government stockpiles entering the trade chain (CITES, 2016). Other stockpiles, sometimes legal sometimes not, are in the hands of private speculators, who keep products as investments in the expectation of future price increases; such increases are highly likely as a species becomes rarer and as it approaches or even reaches extinction (Mason et al., 2012). The BBN would therefore need to differentiate between these different types of stockpiles, the mechanisms by which products enter the trade, and the different ways that this buffers the link between supply and demand.
Modeling Controversies Around the Impacts of Different Interventions
Identifying the appropriate strategy for managing the trade in different species is controversial. A benefit of BBNs is that once one has been set up for a particular species, it is relatively quick to investigate the effect of different strategies on the population, and therefore to explore potential options with a group of stakeholders holding different views. The BBN does not test whether or not a particular hypothesis about the effect of trade or bans on species sustainability is correct. Rather, it provides a framework for examining what would happen under different management options, and evaluates how sensitive the species’ sustainability is to different scenarios. For example, what might happen to the populations of a specific species if it is down-listed from CITES Appendix I to CITES Appendix II, given different assumptions about consumer preferences and the resources available for management?
We demonstrate the power of a BBN to explore this and similar questions using a slightly expanded version of our model of a hypothetical traded species (Figure 4), including some of the drivers of management and demand. This model shows a species with high productivity, and high population prior to the intervention, where consumer preferences are negative, alternative products are available, management at transit sites is poor, and few resources are available for management at source sites. Given these findings (the gray boxes), probabilities of the states of other nodes are calculated (the yellow boxes). The probability that the population is sustainable under a trade ban is calculated to be 56%.
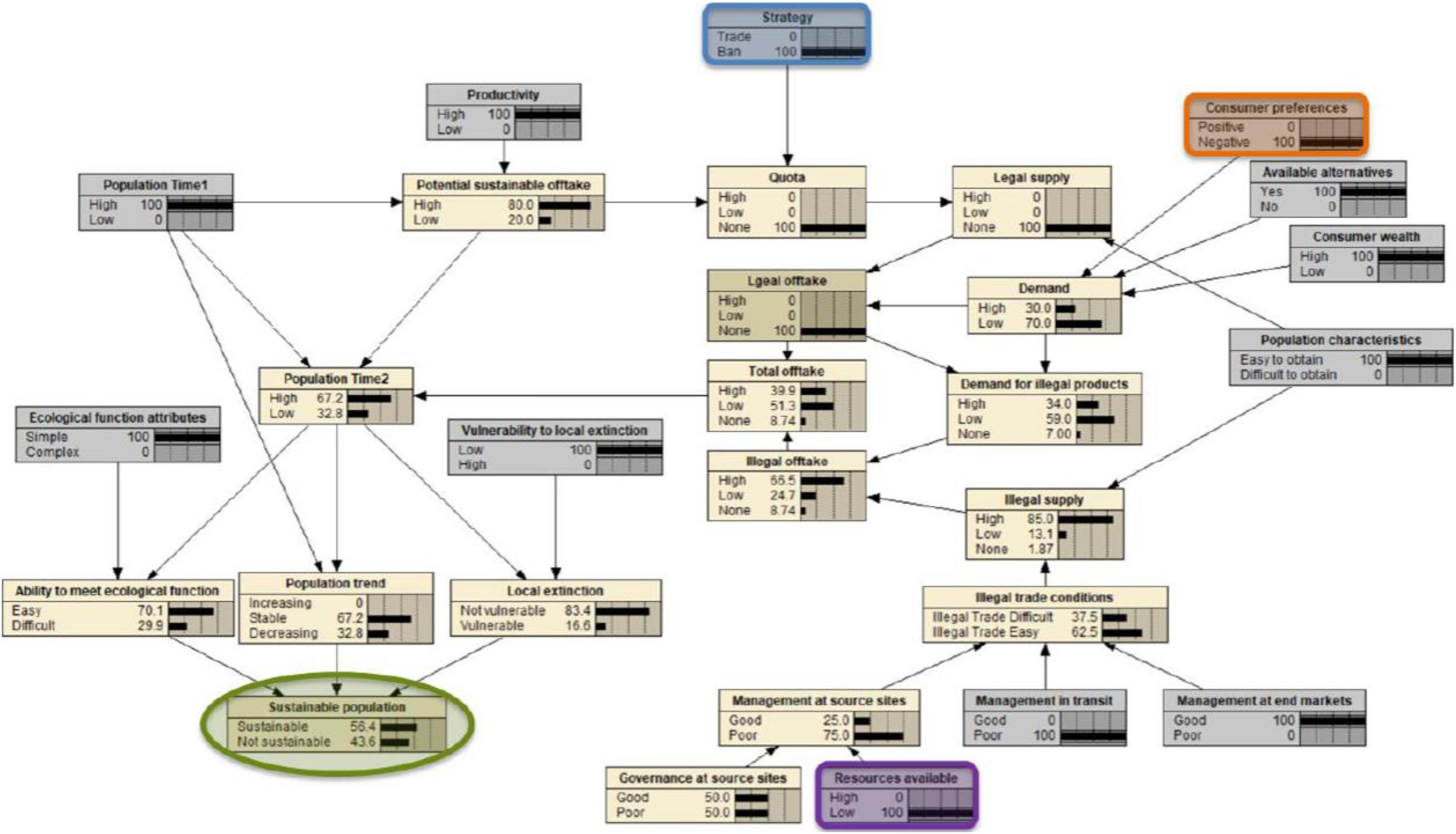
Figure 4. Illustrative expanded BBN from Figure 3 with additional nodes to represent how some of the drivers of demand and management could be represented in the model. The BBN shows the (hypothetical) probability of a sustainable population for a particular species with a particular set of findings (gray boxes) under a trade ban. Gray nodes are where the state is fixed and known. Yellow nodes show calculated probabilities for each state given the fixed states of the gray nodes. This BBN is a reduced and simplified model, all nodes only have two or three discrete states, only two time periods are included, and all relationships are simple. Note that all probabilities used to construct this BBN and shown in the figure are illustrative only.
Figure 5 shows the results from using the hypothetical BBN of Figure 4 to explore the probability that populations of two different species are sustainable under different trade strategies. The only two interventions considered are regulated trade or a trade ban. For both species, under a trade ban, the probability that the population is sustainable, (given negative consumer preferences and low resources) is 56% (point A in Figure 5 and as shown in Figure 4). If the Strategy changes to Trade, but all else remains the same (Figure 5 point B), for Species 1 the probability that the population is sustainable declines to 35%. For Species 2, however, the probability is the same at 56%. This would suggest that for Species 1, Ban is better than Trade but for Species 2 both strategies are equally good, assuming all else remains constant.
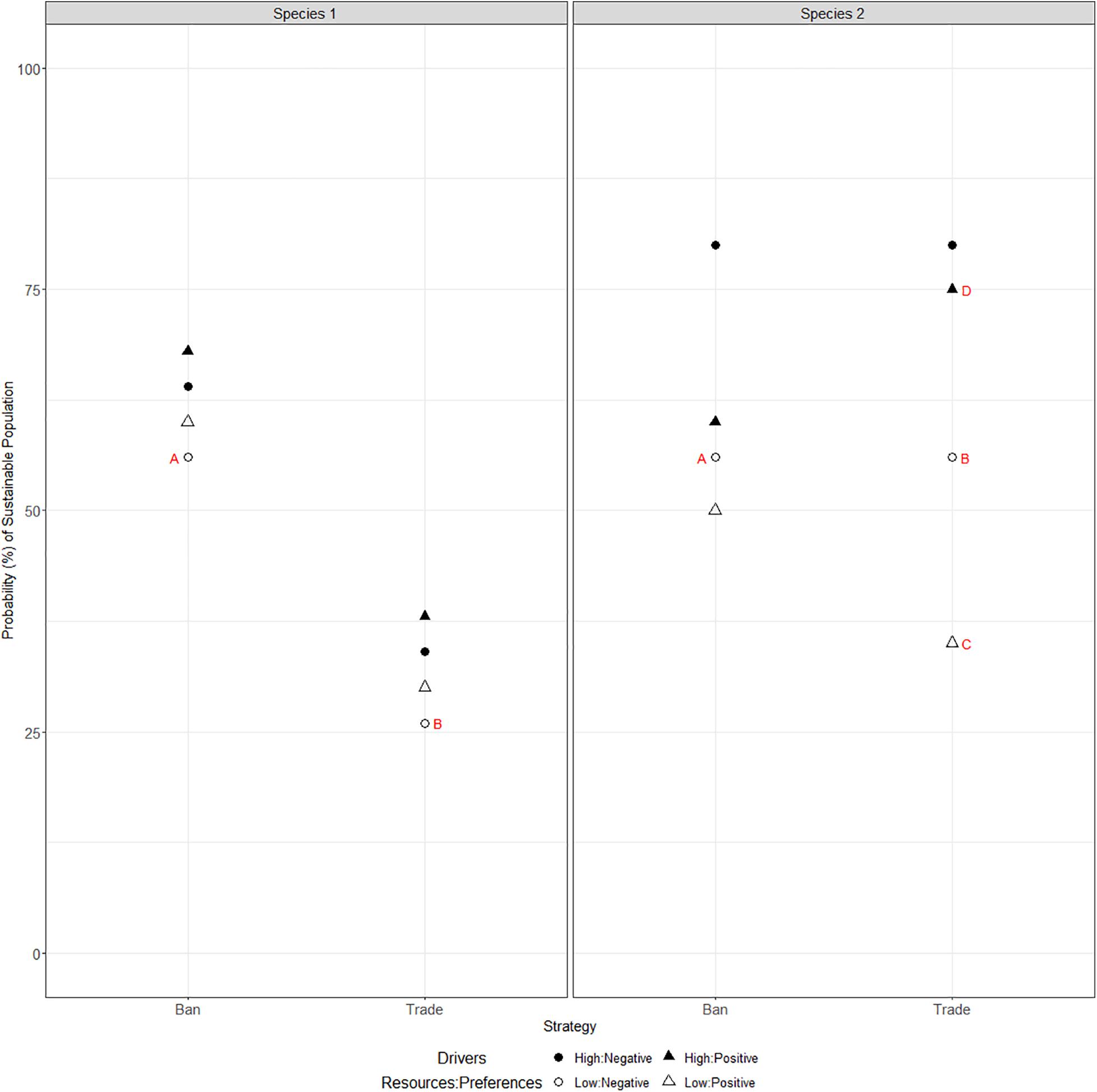
Figure 5. Hypothetical probabilities (as percentage) that two different species are sustainable under two different Strategies (Ban or Trade), given different combinations of the drivers: Resource Availability at source sites either High (filled symbol) or Low (empty symbol) and Consumer Preferences are either Negative (circle) or Positive (triangle). The probability that either species is sustainable when Resource Availability is Low and Consumer Preferences are Negative is point A when Strategy is Ban and point B when Strategy is Trade. For species 2, point C shows the probability under Trade when Consumer Preferences are Positive. Under point D Resource Availability is also High.
However, disagreements about which is the best strategy are often due to different assumptions, or hypotheses about which drivers are also expected to change when a particular strategy is implemented, e.g., whether allowing legal trade will lead to changes in consumer preferences or availability of resources for management at source sites. For Species 1, Figure 5 shows that the probability that the population is sustainable is always higher under a Ban than under Trade, irrespective of Consumer Preferences and Resource Availability. However, for Species 2, to determine how other drivers are expected to change as a result of a change in trading strategy, discussions are needed to identify the best outcome for the species. For example, one hypothesis might be that switching to Trade also leads to positive Consumer Preferences. Then the probability that the population is sustainable drops to 35% (Figure 5 point C). If a further hypothesis is that the effect of switching to Trade also leads to the Resources Available for management of source sites increasing from low to high, the probability the population is sustainable increases to 75% (Figure 5 point D).
Hence, even if different stakeholders disagree about what other changes will occur under a different trade strategy, the BBN could help them agree that it will not affect the overall outcome for the species (as for Species 1). Alternatively, the BBN might indicate that changing the trade regime has no effect on the sustainability of the population but other drivers, reflected in another part of the BBN (e.g., management at sites), are more critical. In other cases, the choice between a ban or legal trade could lead to very different probabilities that the species population is sustainable, depending on beliefs about what else will change as a result of a change in trade regime (as for Species 2). This could suggest that further work, and potentially a more refined modeling approach, are needed to understand which hypotheses are most likely.
Modeling Uncertainty
There are three main sources of uncertainty in a BBN. The first (1) is that relationships between variables are probabilistic—i.e., given the states of a particular set of nodes, outcomes are not predicted with certainty. This is modeled inherently by the BBN. The other two depend on the model components, specifically (2) structural uncertainty—our understanding of which variables and drivers to include in the BBN and how they affect each other (the linkages between nodes) and (3) parameter uncertainty—our knowledge about the values of the CPT that describe the relationships between nodes.
In some cases, controversy might arise around these model components. For example, views on the importance (reflected in the CPT values) of each of the parents of the Demand node (Figure 4) might differ. In this case, one would examine how changing the CPTs affects the outcomes of interest. This might suggest that further research is needed to obtain better evidence about what the CPTs should be. Alternatively, it might indicate that changing the CPTs in this part of the BBN network does not materially affect the outcome, so is not a research priority.
One constraint of a BBN is that the CPTs do not have estimates of uncertainty attached to them. Thus although BBNs can be used to better understand and model complex problems, identify knowledge gaps, prioritize future research and evaluate management options (Johnson et al., 2014), they would need to be complemented by other approaches if the uncertainties in the probabilities need to be captured for decision making. Strategies for estimating these uncertainties have been proposed (Van Allen et al., 2008; Donald and Mengersen, 2014), but they are not currently available in standard BBN software. This current limitation on BBNs does not, however, undercut the value of the general approach of constructing a BBN and exploring it to investigate the relationships between species productivity, different aspects of management and demand, in order to assess impacts of different policy decisions on the sustainability of species’ populations.
Discussion
Discussions around whether or not a particular species should, or should not, be subject to a sustainable trade are often divisive, and the contrast between the approach and even the language of the pro- and anti-trade proponents in the literature is great. Both are concerned about the implications of unsustainable trade for conservation, but the pro-trade literature focuses on empowering and incentivizing local communities and benefiting source countries (e.g., Cooney et al., 2015), and the anti-trade literature on increasing enforcement and supporting criminal justice systems (e.g., Wyatt and Cao, 2015).
Models can help to move us beyond such entrenched value-based assumptions to a scientific discussion, giving another way to look at an issue (Addison et al., 2013; Biggs et al., 2017). Models do not provide definitive answers, but provide a framework that can help resolve difficult issues by identifying assumptions, and estimating probabilities of success for alternative actions based on the best available information (Starfield and Beloch, 1991; VanderWerf et al., 2006). BBNs are a potentially powerful tool for presenting and investigating problems and communicating solutions, because they make it possible to explore the consequences of different decisions (Uusitalo, 2007; Korb and Nicholson, 2010). Most importantly, they provide a transparent basis for a logical discussion and dialog between proponents of different viewpoints (Bromley et al., 2005). All interested parties can examine the values of the different attributes for a particular species, the structure of the BBN and the CPTs, and jointly see the potential outcomes of a particular course of action. For example Henriksen et al. (2012) demonstrated how BBNs could be used as a way of communicating and engaging with different stakeholders about groundwater management in Spain.
We have described a broad framework to capture how the outcome for a species population of a particular strategy (ban or sustainable trade) is determined by the interaction of three components: biological productivity, management context and demand. We have shown how this framework can be turned into a BBN to allow us to examine the likely outcome for the population of a species in demand in trade if we implement a program of sustainable use, or a partial or total trade ban, given the current attributes of the species, management context, and demand attributes. The BBN can be used to assess which strategy is more likely to succeed in conserving a species, given the particular characteristics of that species and the context. It can also help us to examine what needs to be changed to make a particular trade regime succeed in conserving the species, or whether other drivers which might respond to a change in trade regime could impact the outcome for a species. If the BBN shows that under current conditions, populations are unlikely to be sustainable under either trade bans or a sustainable trade regime (as is currently the case for many species), the model can help to identify what needs to change to result in a successful outcome for each approach. Furthermore, the BBN can be used to assess whether particular assumptions about how human behavior responds to particular trade regimes impact on the outcome.
This is the first attempt to construct a BBN to assess the impact of different options for managing wildlife trade on species populations. The BBN presented here is simple, to convey the basic principles involved. It could potentially be used for any species in a relatively quick-and-dirty way, by setting appropriate values for the nodes (e.g., productivity low, management low, and demand high). A more complex BBN could also be built to reflect the biological and trade characteristics of an individual species. The former might be useful for general discussions about the trade, while the latter might be useful for those trying to manage a particular species. An operational BBN for use on a real species would need to be constructed through expert debate and a consultative process.
The current model was developed for terrestrial mammals, but the basic principles can be applied to any other taxonomic group. Hopefully, this approach could help the conservation community to move beyond the rancor which can typify debates, and instead allow us to assess the most appropriate regime for managing trade in species of both conservation and commercial value in a transparent, open and scientifically based way.
Data Availability Statement
The original contributions presented in the study are included in the article/supplementary material, further inquiries can be directed to the corresponding author/s.
Author Contributions
EB was primarily responsible for the wildlife trade literature review and providing species-specific examples and information. FU was primarily responsible for the model literature review and developing the BBN. EM-G brought the group together and provided critical thinking to improve the manuscript throughout. All authors contributed to the article and approved the submitted version.
Funding
EB was funded by the Wildlife Conservation Society and a Biodiversity Fellowship at the Interdisciplinary Centre for Conservation Science, University of Oxford. EM-G was funded by the Oxford Martin Programme on the IllegalWildlife Trade.
Conflict of Interest
The authors declare that the research was conducted in the absence of any commercial or financial relationships that could be construed as a potential conflict of interest.
Acknowledgments
We thank Bob Burn and John Robinson for discussions and comments on drafts of the paper, and to the two reviewers for their comments.
References
Acebes, P., Wheeler, J., Baldo, J., Tuppia, P., Lichtenstein, G., Hoces, D., et al. (2018). Vicugna vicugna (errata version published in 2019). IUCN Red List Threat. Spec. 2018:eT22956A145360542. doi: 10.2305/IUCN.UK.2018-2.RLTS.T22956A145360542.en
Addison, P. F. E., Rumpff, L., Bau, S. S., Carey, J. M., Chee, Y. E., Jarrad, F. C., et al. (2013). Practical solutions for making models indispensable in conservation decision making. Divers. Distrib. 19, 490–502. doi: 10.1111/ddi.12054
Aguilera, P. A., Fernández, A., Fernández, R., Rumí, R., and Salmerón, A. (2011). Bayesian networks in environmental modelling. Environ. Model. Softw. 26, 1376–1388. doi: 10.1016/j.envsoft.2011.06.004
Bello, C., Galetti, M., Pizo, M. A., Magnago, L. F. S., Rocha, M. F., Lima, R. A. F., et al. (2015). Defaunation affects carbon storage in tropical forests. Sci. Adv. 1:11. doi: 10.1126/sciadv.1501105
Bennett, E. L. (2011). Another inconvenient truth: the failure of enforcement systems to save charismatic species. Oryx 45, 476–479. doi: 10.1017/s003060531000178x
Bennett, E. L. (2015). Legal ivory trade in a corrupt world: a recipe for extinction. Conserv. Biol. 29, 54–60. doi: 10.1111/cobi.12377
Bennett, E. L., and Robinson, J. G. (2000). Hunting of Wildlife in Tropical Forests: Implications for Biodiversity and Forest Peoples. Washington DC: The World Bank.
Berzaghi, F., Longo, M., Ciais, P., Blake, S., Bretagnolle, F., Vieira, S., et al. (2019). Carbon stocks in Central African forests enhanced by elephant disturbance. Nat. Geosci. 12, 725–729. doi: 10.1038/s41561-019-0395-6
Biggs, D., Courchamp, F., Martin, R., and Possingham, H. P. (2013). Legal trade of Africa’s rhino horns. Science 339, 1038–1039. doi: 10.1126/science.1229998
Biggs, D., Holden, M. H., Braczkowski, A., Cook, C. N., Milner-Gulland, E. J., Phelps, J., et al. (2017). Breaking the deadlock on ivory: an iterative process that recognizes different value systems may help to protect elephants. Science 358, 1378–1381.
Blake, S., Deem, S. L., Mossimbo, E., Maisels, F., and Walsh, P. (2009). Forest elephants: tree planters of the Congo. Biotropica 41, 459–468. doi: 10.1111/j.1744-7429.2009.00512.x
Bodmer, R. E., and Fang, T. G. (2016). Evaluación De Pecaríes En La Amazonia Peruana De Loreto Para Las Cuotas Máximas Sostenibles. Loreto: Fundamazonia.
Bodmer, R. E., and Puertas, P. (2000). “Community-based comanagement of wildlife in the Peruvian Amazon,” in Hunting for Sustainability in Tropical Forests, eds J. G. Robinson and E. L. Bennett (New York: Columbia University Press), 395–409.
Bromley, J., Jackson, N. A., Clymer, O. J., Giacomello, A. M., and Jensen, F. V. (2005). The use of Hugin® to develop Bayesian networks as an aid to integrated water resource planning. Environ. Model. Softw. 20, 231–242. doi: 10.1016/j.envsoft.2003.12.021
Bulte, E. H., van Kooten, G. C., and Swanson, T. (2003). “Economic incentives and wildlife conservation,” in Proceedings of the CITES Workshop on Economic Incentives and Trade Policy (Geneva: CITES), 1–3.
Burn, R. W., Underwood, F. M., and Hunter, N. D. (2003). MIKE Data Analysis Strategy. Geneva: MIKE, CITES, UNEP.
Butchart, S. H. M., Walpole, M., Collen, B., van Strien, A., Scharlemann, J. P. W., Almond, R. A. E., et al. (2010). Global biodiversity: indicators of recent declines. Science 328, 1164–1168.
Cain, J. (2001). Planning Improvements in Natural Resources Management: Guidelines for Using Bayesian Networks to Support the Planning and Management of Development Programmes in the Water Sector and Beyond. Wallingford, UK: Centre for Ecology and Hydrology.
Caso, A., Lopez-Gonzalez, C., Payan, E., Eizirik, E., de Oliveira, T., Leite-Pitman, R., et al. (2008). Panthera onca. IUCN Red List Threat. Spec. 2008:eT15953A5327466. doi: 10.2305/IUCN.UK.2008.RLTS.T15953A5327466.en
Castelletti, A., and Soncini-Sessa, R. (2007). Bayesian Networks and participatory modelling in water resource management. Environ. Model. Softw. 22, 1075–1088.
Challender, D., Willcox, D. H. A., Panjang, E., Lim, N., Nash, H., Heinrich, S., et al. (2019a). Manis javanica. IUCN Red List Threat. Spec. 2019:eT12763A123584856.
Challender, D., Wu, S., Kaspal, P., Khatiwada, A., Ghose, A., Ching-Min Sun, N., et al. (2019b). Manis pentadactyla (errata version published in 2020). IUCN Red List Threat. Spec. 2019:eT12764A168392151. doi: 10.2305/IUCN.UK.2019-3.RLTS.T12764A168392151.en
Challender, D. W. S., Harrop, S. R., and MacMillan, D. C. (2015). Towards informed and multi-faceted trade interventions. Glob. Ecol. Conserv. 3, 129–148. doi: 10.1016/j.gecco.2014.11.010
Challender, D. W. S., and MacMillan, D. C. (2014). Poaching is more than an enforcement problem. Conserv. Lett. 7, 484–494. doi: 10.1111/conl.12082
Child, B. (2012). The sustainable use approach could save South Africa’s rhinos. S. Afr. J. Sci. 108, 21–25. doi: 10.4102/sajs.v108i7/8.1338
CITES (2012). Interpretation and Implementation of the Convention. Species Trade and Conservation: Elephants. Elephant Conservation, Illegal Killing and Ivory Trade. SC62 Doc 46.1. Geneva: Sixty-Second Meeting of the Standing Committee.
CITES (2016). Ivory stockpiles: proposed revision of Resolution Conf. 10.10 (Rev. CoP16) on Trade in Elephant Specimens. CoP17 Doc. 57.3. Geneva: CITES.
CITES (2017). Status of Elephant Populations, Levels of Illegal Killing and the Trade in Ivory: A Report to the CITES Standing Committee. Geneva: CITES.
Coad, L., Leverington, F., Knights, K., Geldmann, J., Eassom, A., Kapos, V., et al. (2015). Measuring impact of protected area management interventions: current and future use of the global database of protected area management effectiveness. Philos. Trans. R. Soc. B 370:20140281. doi: 10.1098/rstb.2014.0281
Conrad, K. (2012). Trade bans: a perfect storm for poaching? Trop. Conserv. Sci. 5, 245–254. doi: 10.1177/194008291200500302
Cooney, R., Kasterine, A., MacMillan, D., Milledge, S., Nossal, K., Roe, D., et al. (2015). The Trade in Wildlife: A Framework to Improve Biodiversity and Livelihood Outcomes. Geneva: International Trade Centre.
Courchamp, F., Angulo, E., Rivalan, P., Hall, R. J., Signoret, L., Bull, L., et al. (2006). Rarity value and species extinction: the anthropogenic Allee effect. PLoS Biol. 4:e0040415. doi: 10.1371/journal.pbio.0040415
Cowell, R. G., Dawid, P., Lauritzen, S. L., and Spiegelhalter, D. J. (1999). Probabilistic Networks and Expert Systems. New York: Springer.
Di Minin, E., Laitilla, J., Montesino-Pouzols, F., Leader-Williams, N., Slotow, R., Goodman, P. S., et al. (2014). Identification of policies for a sustainable legal trade in rhinoceros horn based on population projection and socioeconomic models. Conserv. Biol. 29, 545–555. doi: 10.1111/cobi.12412
Donald, M. R., and Mengersen, K. L. (2014). Methods for constructing uncertainty intervals for queries of bayesian nets. Austral. N. Zeal. J. Stat. 56, 407–427. doi: 10.1111/anzs.12095
Duckworth, J. W., Batters, G., Belant, J. L., Bennett, E. L., Brunner, J., Burton, J., et al. (2012). Why South-East Asia should be the world’s priority for averting imminent species extinctions, and a call to join a developing cross-institutional programme to tackle this urgent issue. SAPIENS 5, 77–95.
Duffy, R., and Humphries, J. (2016). Poaching, trafficking and human security. Whitehall Papers 86, 22–37. doi: 10.1080/02681307.2016.1252122
Düspohl, M., Frank, S., and Döll, P. (2012). A review of bayesian networks as a participatory modeling approach in support of sustainable environmental management. J. Sustain. Dev. 5, 1–18. doi: 10.5539/jsd.v5n12p1
Eaton, J. A., Shepherd, C. R., Rheindt, F. E., Harris, J. B. C., van Balen, S. B., Wilcove, D. S., et al. (2015). Trade-driven extinctions and near-extinctions of avian taxa in Sundaic Indonesia. Forktail 31, 1–12.
Elliott, L. (2007). Transnational environmental crime in the Asia-Pacific: an “un(der)-securitized” security problem? Pac. Rev. 20, 499–522. doi: 10.1080/09512740701671995
Eriksson, H., and Clarke, S. (2015). Chinese market responses to over-exploitation of sharks and sea cucumbers. Biol. Conserv. 184, 163–173. doi: 10.1016/j.biocon.2015.01.018
Estes, J. A., Terborgh, J., Brashares, J. S., Power, M. E., Jerger, J., Bond, W. J., et al. (2011). Trophic downgrading of planet earth. Science 333, 301–306.
Felbab-Brown, V. (2017). The Extinction Market; Wildlife Trafficking and How to Counter It. New York, NY: Oxford University Press.
Fischer, C. (2010). Does trade help or hinder the conservation of natural resources? Rev. Environ. Econ. Policy 4, 103–121. doi: 10.1093/reep/rep023
Gabriel, G. G., Hua, N., and Wang, J. (2012). Making a Killing: a 2011 Survey of Ivory Markets in China. Yarmouth Port: IFAW.
Global Initiative against Transnational Organized Crime (2020). Why Poaching has Decreased Dramatically in Mozambique’s Niassa Reserve. Geneva: Global Initiative against Transnational Organized Crime.
Goncalves, M. P., Panjer, M., Greenberg, T. S., and Magrath, W. B. (2012). Justice for Forests: Improving Criminal Justice Efforts to Combat Illegal Logging. Washington, DC: World Bank.
Goodrich, J., Lynam, A., Miquelle, D., Wibisono, H., Kawanishi, K., Pattanavibool, A., et al. (2015). Panthera tigris. IUCN Red List Threat. Spec. 2015:eT15955A50659951. doi: 10.2305/IUCN.UK.2015-2.RLTS.T15955A50659951.en
Halls, A. S., Burn, R. W., and Abeyasekera (2002). Interdisciplinary Multivariate Analysis for Adaptive Co-Management. Project R7834 Final Technical Report to the Department for International Development DFID. London: DFID.
Henriksen, H. J., Zorrilla-Miras, P., De la Hera, A., and Brugnach, M. (2012). Use of Bayesian belief networks for dealing with ambiguity in integrated groundwater management. Integr. Environ. Assess. Manag. 8, 430–444. doi: 10.1002/ieam.195
IUCN (2000). Sustainable Use: IUCN Policy Statement. IUCN Species Survival Commission Sustainable Use Specialiist Group. Gland: IUCN.
Johnson, S., Abal, E., Ahern, K., and Hamilton, G. (2014). From science to management: using Bayesian Network to learn about Lyngbya. Stat. Sci. 29, 36–41. doi: 10.1214/13-sts424
Kahumbu, P. (2015). “Things R Elephant”: Heated Debate in Kenya Gets to the Heart of What It Will Take to Save the Species. Available online at: http://voices.nationalgeographic.com/2015/04/27/things-r-elephant-heated-debate-in-kenya-gets-to-the-heart-of-what-it-will-take-to-save-the-species/ (accessed May 19, 2016).
Koen, H., de Villiers, J. P., Roodt, H., and de Waal, A. (2017). An expert-driven causal model of the rhino poaching problem. Ecol. Model. 347, 29–39. doi: 10.1016/j.ecolmodel.2016.12.007
Korb, K. B., and Nicholson, A. E. (2010). Bayesian Artificial Intelligence Second Edition. Boca Raton, London. New York, NY: CRC press.
Lichtenstein, G. (2011). “Use of vincuñas (Vicugna vicugna) and guanacos (Lama guanicoe) in Andean countries: linking community-based conservation initiatives with international markets,” in CITES and CBNRM: Proceedings of an International Symposium on ‘The Relevance of CBNRM to the Conservation and Sustainable Use of CITES-Listed Species in Exporting Countries’, eds M. Abensperg-Traun, D. Roe, and C. O’Croidain (Gland: IUCN), 103–108.
Linkie, M., Martyr, D. J., Harihar, A., Risdianto, D., Nugraha, R. T., Maryati, M., et al. (2015). Safeguarding Sumatran tigers: evaluating effectiveness of law enforcement patrols and local enforcement netrworks. J. Appl. Ecol. 52, 851–860. doi: 10.1111/1365-2664.12461
Lusseau, D., and Lee, P. C. (2016). Can we sustainably harvest ivory? Curr. Biol. 26, 2951–2956. doi: 10.1016/j.cub.2016.08.060
Maisels, F. (2012). Comments on the final report by Martin et al. “Decision-Making Mechanisms and Necessary Conditions for a Future Trade in Elephant Ivory”. IUCN African Elephant Specialist Group report to the CITES Secretariat; 2012. Available online at: https://cmsdata.iucn.org/downloads/afesg_comments_finalreport_30august2012.pdf (accessed May 26, 2016).
Maisels, F., Strindberg, S., Blake, S., Wittemeyer, G., Hart, J., Williamson, E. A., et al. (2013). Devastating decline of forest elephants in Central Africa. PLoS One 8:e0059469. doi: 10.1371/journal.pone.0059469
Marcot, B. G., Holthausen, R. S., Raphael, M. G., Rowland, M. M., and Wisdom, M. J. (2001). Using Bayesian belief networks to evaluate fish and wildlife population viability under land management alternatives from an environmental impact statement. For. Ecol. Manag. 153, 29–42. doi: 10.1016/s0378-1127(01)00452-2
Martin, R. B., Cumming, D. H. M., Craig, G. C., Gibson, D., and Peake, D. A. (2012). Decision-Making Mechanisms and Necessary Conditions for a Future Trade in African Elephant Ivory. Available online at: https://www.cites.org/sites/default/files/eng/com/sc/62/E62-46-04-A.pdf (accessed May 26, 2016).
Mason, C. F., Bulte, E., and Horan, R. D. (2012). Banking on extinction: endangered species and speculation. Oxf. Rev. Econ. Policy 28, 180–192. doi: 10.1093/oxrep/grs006
McAllister, R. R. J., McNeill, D., and Gordon, I. J. (2009). Legalizing markets and consequences for poaching of wildlife species: the vincuña as a case study. J. Environ. Manage. 90, 120–130.
McCann, R. K., Marcott, B. G., and Ellis, R. (2006). Bayesian belief networks: application in ecology and natural resource management. Can. J. For. Res. 36, 3053–3062. doi: 10.1139/x06-238
McCarthy, T., Mallon, D., Jackson, R., Zahler, P., and McCarthy, K. (2017). Panthera uncia. IUCN Red List Threat. Spec. 2017:eT22732A50664030. doi: 10.2305/IUCN.UK.2017-2.RLTS.T22732A50664030.en
McGrath, M. (2013). S Africa Fears 2013 Rhino Slaughter will Break Records. Africa News and Analysis. 8th March. Available online at:https://africajournalismtheworld.com/tag/cites-and-rhino-appoaching/ (accessed June 20, 2016).
Milliken, T., and Shaw, J. (2012). The South Africa – Viet Nam Rhino Horn Trade Nexus: A Deadly Combination of Institutional Lapses, Corrupt Wildlife Industry Professionals and Asian Crime Syndicates. Kuala Lumpur: TRAFFIC Southeast Asia.
Moyle, B. (2017). Wildlife markets in the presence of laundering. Biodivers. Conserv. 26, 2979–2985. doi: 10.1007/s10531-017-1396-7
Neapolitan, R. E. (2004). Learning Bayesian Networks. Upper Saddle River, NJ: Pearson Prentice Hall.
Nijman, V. (2010). An overview of international wildlife trade from Southeast Asia. Biodivers. Conserv. 19, 1101–1114. doi: 10.1007/s10531-009-9758-4
Nuwer, R. (2015). Poaching upsurge threatens South America’s Iconic Vincuña. London: Scientific American.
Pastor, M. A. (2010). Legal, moral and biological implications of poaching and illegal animal trafficking on an international scale. Pell Schol. Senior Theses 47:661.
Pearl, J. (1985). Probabilistic Reasoning in Intelligent Systems: Networks of Plausible Inference. Burlington, MA: Morgan Kaufmann.
Peres, C. A., Emilio, T., Schietti, J., Desmouliere, S. J. M., and Levi, T. (2016). Dispersal limitation induces long-term biomass collapse in overhunted Amazonian forests. Proc. Natl. Acad. Sci. U.S.A. 113, 892–897. doi: 10.1073/pnas.1516525113
Pollino, C. A., Woodberry, O., Nicholson, A., Korb, K., and Hart, B. T. (2007). Parameterisation and evaluation of a Bayesian network for use in an ecological risk assessment. Environ. Model. Softw. 22, 1140–1152. doi: 10.1016/j.envsoft.2006.03.006
Read, A. F., and Harvey, P. H. (1989). Life history differences among the eutherian radiations. J. Zool. 219, 329–353. doi: 10.1111/j.1469-7998.1989.tb02584.x
Ripple, W. J., Estes, J. A., Beschta, R. L., Wilmers, C. C., Ritchie, E. G., Hebblewhite, M., et al. (2014). Status and ecological effects of the world’s largest carnivores. Science 343:1241484. doi: 10.1126/science.1241484
Rivalan, P., Delmas, V., Angulo, E., Bull, L. S., Hall, R. J., and Courchamp, F. (2007). Can bans stimulate wildlife trade? Science 447, 529–530. doi: 10.1038/447529a
Robinson, J. G., and Bennett, E. L. (2004). Having your wildlife and eating it too: an analysis of hunting sustainability across tropical ecosystems. Anim. Conserv. 7, 397–408. doi: 10.1017/s1367943004001532
Robinson, J. G., and Redford, K. H. (1986). Body size, diet, and population density of Neotropical forest mammals. Am. Nat. 128, 665–680.
Roe, D., Millege, S., Cooney, R., ‘t Sas-Rolfes, M., Biggs, D., Murphree, M., et al. (2014). The Elephant in the Room: Sustainable Use in the Illegal Wildlife Trade Debate. London: International Institute for Environment and Development.
Rosen, G. E., and Smith, K. F. (2010). Summarizing the evidence on the international trade in illegal wildlife. EcoHealth 7, 24–32. doi: 10.1007/s10393-010-0317-y
Saliou, N., Bernaud, C., Vialatte, A., and Monteil, C. (2017). A participatory Bayesian Belief Network approach to explore ambiguity among stakeholders about socio-ecological systems. Environ. Model. Softw. 96, 199–209. doi: 10.1016/j.envsoft.2017.06.050
Smith, R. J., Biggs, D., St. John, F. A. V., ‘t Sas-Rolfes, M., and Barrington, R. (2015). Elephant conservation and corruption beyond the ivory trade. Conserv. Biol. 29, 953–956. doi: 10.1111/cobi.12488
Starfield, A. M., and Beloch, A. L. (1991). Building Models for Conservation and Wildlife Management. Edina: Interaction Book Company.
Stiles, D. (2004). The ivory trade and elephant conservation. Environ. Conserv. 31, 309–321. doi: 10.1017/s0376892904001614
Thouless, C. R., Dublin, H. T., Blanc, J. J., Skinner, D. P., Daniel, T. E., Taylor, R. D., et al. (2016). African elephant status report 2016: an update from the African Elephant Database. Paper Presented at the Occasional Paper Series of the IUCN Species Survival Commission, No. 60, IUCN African Elephant Specialist Group, (Gland: IUCN).
Ticehurst, J., Curtis, A., and Merritt, W. (2011). Using Bayesian Networks to complement conventional analyses to explore landholder management of native vegetation. Environ. Model. Softw. 26, 52–65. doi: 10.1016/j.envsoft.2010.03.032
Underwood, F. M., Parkes, G. J., and Swasey, J. H. (2016). Building Bayesian Belief Networks to investigate how fishery performance responds to management interventions. Fish. Res. 182:28. doi: 10.1016/j.fishres.2015.12.005
UNEP, CITES, IUCN, and TRAFFIC (2013). Elephants in the Dust - The African Elephant Crisis. A Rapid Respose Assessment. Arendal: United Nations Environment Programme.
United Nations Office of Drugs and Crime [UNODC] (2010). The Globalization of Crime: A Transational Organized Crime Threat Assessment. Vienna: UNODC Publishing.
Uscamaita, M. R., and Bodmer, R. (2010). Recovery of the Endangered giant otter Pteronura brasiliensis on the Yavarí-Mirín and Yavarí Rivers: a success story for CITES. Oryx 44, 83–88. doi: 10.1017/s0030605309990196
Uusitalo, L. (2007). Advantages and challenges of Bayesian networks in environmental modelling. Ecol. Model. 3-4, 312–318. doi: 10.1016/j.ecolmodel.2006.11.033
Van Allen, T., Singh, A., Greiner, R., and Hooper, P. (2008). Quantifying the uncertainty of a belief net response: bayesian error-bars for belief net inference. Artif. Intellig. 172, 483–513. doi: 10.1016/j.artint.2007.09.004
VanderWerf, E. A., Groombridge, J. J., Fretz, J. S., and Swinnerton, K. J. (2006). Decision analysis to guide recovery of the po’ouli, a critically endangered Hawaiian honeycreeper. Biol. Conserv. 129, 383–392. doi: 10.1016/j.biocon.2005.11.005
Verheij, P. M., Foley, K. E., and Engel, K. (2010). Reduced to Skin and Bones. An Analysis of Tiger Seizures from 11 Tiger Range Countries (2000-2010). Cambridge, MA: TRAFFIC.
Waldram, M. S., Bond, W. J., and Stock, W. D. (2008). Ecological engineering by a mega-grazer: white rhino impacts on a South African savanna. Ecosystems 11, 101–112. doi: 10.1007/s10021-007-9109-9
Walker, J. F., and Stiles, D. (2010). Consequences of legal ivory trade. Science 328, 1633–1634. doi: 10.1126/science.328.5986.1633-c
Wasser, S., Nowak, K., Poole, J., Hart, J., Beyers, R., Lee, P., et al. (2010). Response. Science 328, 1634–1635.
White, H. B., Decker, T., O’Brien, M. J., Organ, J. F., and Roberts, N. M. (2015). Trapping and furbearer management in North American wildlife conservation. Int. J. Environ. Stud. 72, 756–769. doi: 10.1080/00207233.2015.1019297
Wyatt, T., and Cao, A. N. (2015). Corruption and Wildlife Trafficking. U4 Issue Number 11. Washington, DC: U4 Anti-Corruption Resource Center.
Wyler, L. S., and Sheikh, P. (2013). “International illegal trade in wildlife: threats and U.S. policy,” in Illicit Trade in Wildlife and the Economics of Agricultural and Wildlife Smuggling, ed. R. Gagnier (New York, NY: Nova Science Publishers), 1–55.
Zorilla, P., Carmona, G., De la Hera, Á, Varela-Ortega, C., Martínez-Santos, P., Bromley, J., et al. (2009). Evaluation of Bayesian Networks in participatory water resources management, Upper Guadiana Basin, Spain. Ecol. Soc. 15:12.
‘t Sas-Rolfes, M. (2000). “Assessing CITES: four case studies,” in Endangered Species, Treatened Convention: The Past, Present and Future of CITES, eds J. Hutton and B. Dickson (London UK: Earthscan).
Keywords: Bayesian Belief Networks, commercial wildlife trade, resolving controversies, decision support tools, terrestrial mammals
Citation: Bennett EL, Underwood FM and Milner-Gulland EJ (2021) To Trade or Not to Trade? Using Bayesian Belief Networks to Assess How to Manage Commercial Wildlife Trade in a Complex World. Front. Ecol. Evol. 9:587896. doi: 10.3389/fevo.2021.587896
Received: 27 July 2020; Accepted: 15 February 2021;
Published: 03 March 2021.
Edited by:
Krithi K. Karanth, Centre for Wildlife Studies, IndiaReviewed by:
Nicolas Salliou, ETH Zürich, SwitzerlandDivya Vasudev, Wildlife Conservation Society, India
Copyright © 2021 Bennett, Underwood and Milner-Gulland. This is an open-access article distributed under the terms of the Creative Commons Attribution License (CC BY). The use, distribution or reproduction in other forums is permitted, provided the original author(s) and the copyright owner(s) are credited and that the original publication in this journal is cited, in accordance with accepted academic practice. No use, distribution or reproduction is permitted which does not comply with these terms.
*Correspondence: Elizabeth L. Bennett, lizbennettwcs@gmail.com
†These authors have contributed equally to this work