- 1Department of Biology, Bryn Mawr College, Bryn Mawr, PA, United States
- 2Department of Geography and Environmental Systems, University of Maryland, Baltimore County, Baltimore, MD, United States
- 3Department of Integrative Biology, Michigan State University, East Lansing, MI, United States
- 4Ecology, Evolution, and Behavior Program, Michigan State University, East Lansing, MI, United States
- 5Department of Biology, Indiana University, Bloomington, IN, United States
- 6Department of Integrative Biology, Oregon State University, Corvallis, OR, United States
- 7School of Biological Sciences, University of Canterbury, Christchurch, New Zealand
- 8Department of Biological Sciences, Southeast Environmental Research Center, Florida International University, Miami, FL, United States
- 9Marine Science Institute, University of California, Santa Barbara, Santa Barbara, CA, United States
- 10German Centre for Integrative Biodiversity Research (iDiv) Halle-Jena-Leipzig, Martin Luther University Halle-Wittenberg, Halle, Germany
- 11Department of Environmental Sciences, University of Virginia, Charlottesville, VA, United States
- 12School of Geographical Sciences and Urban Planning, Arizona State University, Tempe, AZ, United States
- 13National Ecological Observatory Network (NEON), Battelle, Boulder, CO, United States
- 14Institute of Arctic and Alpine Research (INSTAAR), University of Colorado Boulder, Boulder, CO, United States
Global loss of biodiversity and its associated ecosystem services is occurring at an alarming rate and is predicted to accelerate in the future. Metacommunity theory provides a framework to investigate multi-scale processes that drive change in biodiversity across space and time. Short-term ecological studies across space have progressed our understanding of biodiversity through a metacommunity lens, however, such snapshots in time have been limited in their ability to explain which processes, at which scales, generate observed spatial patterns. Temporal dynamics of metacommunities have been understudied, and large gaps in theory and empirical data have hindered progress in our understanding of underlying metacommunity processes that give rise to biodiversity patterns. Fortunately, we are at an important point in the history of ecology, where long-term studies with cross-scale spatial replication provide a means to gain a deeper understanding of the multiscale processes driving biodiversity patterns in time and space to inform metacommunity theory. The maturation of coordinated research and observation networks, such as the United States Long Term Ecological Research (LTER) program, provides an opportunity to advance explanation and prediction of biodiversity change with observational and experimental data at spatial and temporal scales greater than any single research group could accomplish. Synthesis of LTER network community datasets illustrates that long-term studies with spatial replication present an under-utilized resource for advancing spatio-temporal metacommunity research. We identify challenges towards synthesizing these data and present recommendations for addressing these challenges. We conclude with insights about how future monitoring efforts by coordinated research and observation networks could further the development of metacommunity theory and its applications aimed at improving conservation efforts.
Introduction
Biodiversity is a key asset for environmental sustainability via its role in maintaining ecosystem functions and services (Cardinale et al., 2012; Hooper et al., 2012; Díaz et al., 2019). However, over the past half-century, human activities have increased the rate of biodiversity loss more than at any other time in history (World Health Organization, 2005; United Nations, 2017). The term “biodiversity” has been used to describe variation at many different levels of biological organization, but here we focus on species diversity (i.e., data sets from which a researcher can calculate measures of species richness or evenness) (Cleland, 2011). In association with declining biodiversity, researchers have documented changes in species interactions (Tylianakis et al., 2008), distributions, and phenology across ecosystems (Parmesan and Yohe, 2003; Dudgeon et al., 2006; Parmesan, 2006; Martay et al., 2016). These changes, which are not ubiquitous across taxa and landscapes, are expected to accelerate in the future (Brook et al., 2008; Bellard et al., 2012; Urban, 2015), further affecting ecosystem structure and function (Seto et al., 2012; Grimm et al., 2013).
A key goal of biodiversity science in the Anthropocene is to anticipate threshold shifts in the distributions and abundances of organisms and the ecosystem services that they afford to society (United Nations, 2017). However, without explicit consideration of multiple spatial scales and the within- and among-species pool connections, conclusions about the processes driving patterns of biodiversity are incomplete. Community structure and processes are still very common research themes in ecology; however, the greatest increase in number of publications has been observed on themes such as scale, anthropogenic impacts, and climate change (McCallen et al., 2019). Metacommunity theory and its applications are essential to addressing scaling in terms of space (e.g., local vs. regional) and time (i.e., linked to organisms’ generation times), especially in today’s rapidly changing communities and ecosystems (Mouquet and Loreau, 2002; Fahrig, 2003; Fischer and Lindenmayer, 2007).
In the past two decades, short-term field studies have considerably advanced our understanding of metacommunities across space by demonstrating how dispersal traits and landscape heterogeneity can affect community assembly in predictable ways (Logue et al., 2011; Leibold and Chase, 2017; Wilcox et al., 2017). Such information can provide insight into the types of metacommunity dynamics that organize biodiversity in each ecosystem (e.g., Pulliam, 1988; De Bie et al., 2012). However, spatially explicit snapshot data are limited in their utility to identify the processes that underlie observed patterns (Leibold et al., 2004; Brown et al., 2017; Sokol et al., 2017). Because ecological studies are often limited to the short-term (Hughes et al., 2017), considerable variability often remains unexplained in examinations of observational data. Assemblage composition and habitat availability may vary through time because of disturbance, seasonality, multi-year climatic variation (e.g., El Niño Southern Oscillation), and shifts of propagules in and out of dormancy (Holyoak et al., 2020). Many investigators acknowledge the limitation of not having temporal data (Frishkoff et al., 2014), particularly in dynamic systems such as streams (Datry et al., 2016; Sarremejane et al., 2017; Tonkin et al., 2018). Not only can the environment change through time (e.g., habitat availability, environmental heterogeneity, and connectivity), but so too can the intrinsic processes regulating local biotic interactions (e.g., priority effects, intransitive competition) and dispersal from the relevant regional species pool (Chase, 2003; Fukami, 2015; Zarnetske et al., 2017). That is, temporal changes in biodiversity can occur regardless of environmental change.
With short-term spatially replicated metacommunity data, it can be difficult to distinguish between (1) exogenous environmental drivers of compositional changes and (2) endogenous community dynamics that may result from deterministic processes (e.g., multiple stable equilibria, endpoint assembly cycles, frequency-dependent coexistence in continuous space) on metacommunity composition. For instance, intransitive competition (i.e., “rock-paper-scissors” competition scenario) can result in endpoint assembly cycles (EACs) in which communities are decoupled from the influence of local environmental factors (Law and Morton, 1993, 1996; Steiner and Leibold, 2004). However, repeated observations in both space and time are necessary to demonstrate EAC dynamics. Specifically, the data would need to demonstrate that (1) all species involved in intransitive competitive dynamics persist regionally, (2) that the species involved cannot coexist locally, and (3) that low to moderate levels of dispersal among patches in the metacommunity allow for the EACs to play out. Therefore, both temporal and spatial replication are needed to test multiple hypotheses that explain how biodiversity is changing in such spatially complex landscapes (Leibold and Chase, 2017).
In the context of metacommunity assembly, given the problem of inferring spatio-temporal processes from spatial patterns alone, there have been multiple calls for long-term, spatial data collection to advance metacommunity research (Adler et al., 2005; Cottenie, 2005; Leibold and Chase, 2017; Holyoak et al., 2020). Financial and logistical constraints in field studies often hamper a comprehensive exploration of biodiversity trends across temporal and spatial scales. However, the various types of coordinated research networks [e.g., the United States Long Term Ecological Research (LTER) program, the International-LTER (ILTER), the global Nutrient Network, NutNet1, the Smithsonian ForestGEO Network], and observatory networks [e.g., the United States Global Lake Ecological Observatory Network (GLEON), the National Critical Zone Observatory (CZO), and the United States National Ecological Observatory Network (NEON; Bourgeron et al., 2018)], provide a growing resource of long-term data that can be leveraged in synthesis science. Most of these coordinated research and observation networks, hereafter referred to as observation networks, have been working for over 10 years (e.g., the United States LTER will be 40 years old in 2020, the ILTER is 27 years old, GLEON is 16 years old, CZO is 12 years old, and the Long Term Agricultural Research network is 9 years old), while NEON recently completed construction of all 81 field sites and is slated to collect data for the next 30 years (Table 1).

Table 1. Summary of coordinated research networks with community data useful for metacommunity analyses.
With the maturation of long term data sets from such observation networks, the field of ecology is approaching an exciting point where there is the opportunity to empirically explore spatial and temporal representativeness of species within and among sites across ecosystems. Ultimately, a deeper understanding of the representativeness of species in space and time will lead to a better understanding of how scale influences metacommunity organization and biodiversity dynamics, which is key to making more general theoretical insights that transcend beyond the nuances of individual study systems. Here we provide evidence from a synthesis effort of LTER data showing that some LTER sites have the spatial replication within sites over time needed to capture asymptotic species-time-area-relationships (sensu Adler et al., 2005). The results of this synthesis suggest that these datasets could provide an untapped resource for metacommunity studies. When long-term studies include spatial contexts through multiple sites (within the regional species pool), they enable a more complete assessment of biodiversity change relative to long-term studies at a single site and allow for researchers to explore how sampling design might influence insights into metacommunities (Box 1). Spatio-temporal biodiversity patterns can take decades or longer to be described and explained (Magurran et al., 2010), partly owing to high temporal variability in community dynamics or lags in the processes, such as extinction debt, that structure biodiversity (Tilman et al., 1994; Kuussaari et al., 2009). More synthesis of data from long-term observation networks has the potential to uncover additional long-term, spatially replicated data that will aid in exploring the problem in identifying the spatio-temporal mechanisms underlying metacommunity assembly manifested in spatial biodiversity patterns.
Box 1. Uncovering the regional species pool.
One of the most challenging aspects of any metacommunity study is adequately characterizing the regional species pool of interest because it is context-dependent and the “region” is defined by the researcher. When samples are taken in multiple localities at a single point in time, we often assume that the species found across all localities capture the whole regional species pool. However, we know that not all species that could occur at study sites are observed in a single temporal snapshot because stochastic colonization/extinction events and/or transient dynamics might be occurring during the study (reviewed by Holyoak et al., 2020). For example, species experiencing diapause or having low detection probability might be missed or demographic stochasticity may eliminate one or more species in an isolated year (Figure 1). It takes time to sample the regional species pool, but spatially replicated sampling schemes can (1) increase the total number of species encountered and (2) decrease the time it takes to discover them. Additionally, succession and environmental change may add to the pool of species that can potentially occur in the study sites over time, as can the arrival of invasive species (Figure 2; Pickett, 1989; Li et al., 2020). Although the number of species observed at a site may be approximately the same across years, substantial compositional temporal turnover of species may take place (Tonkin et al., 2017).
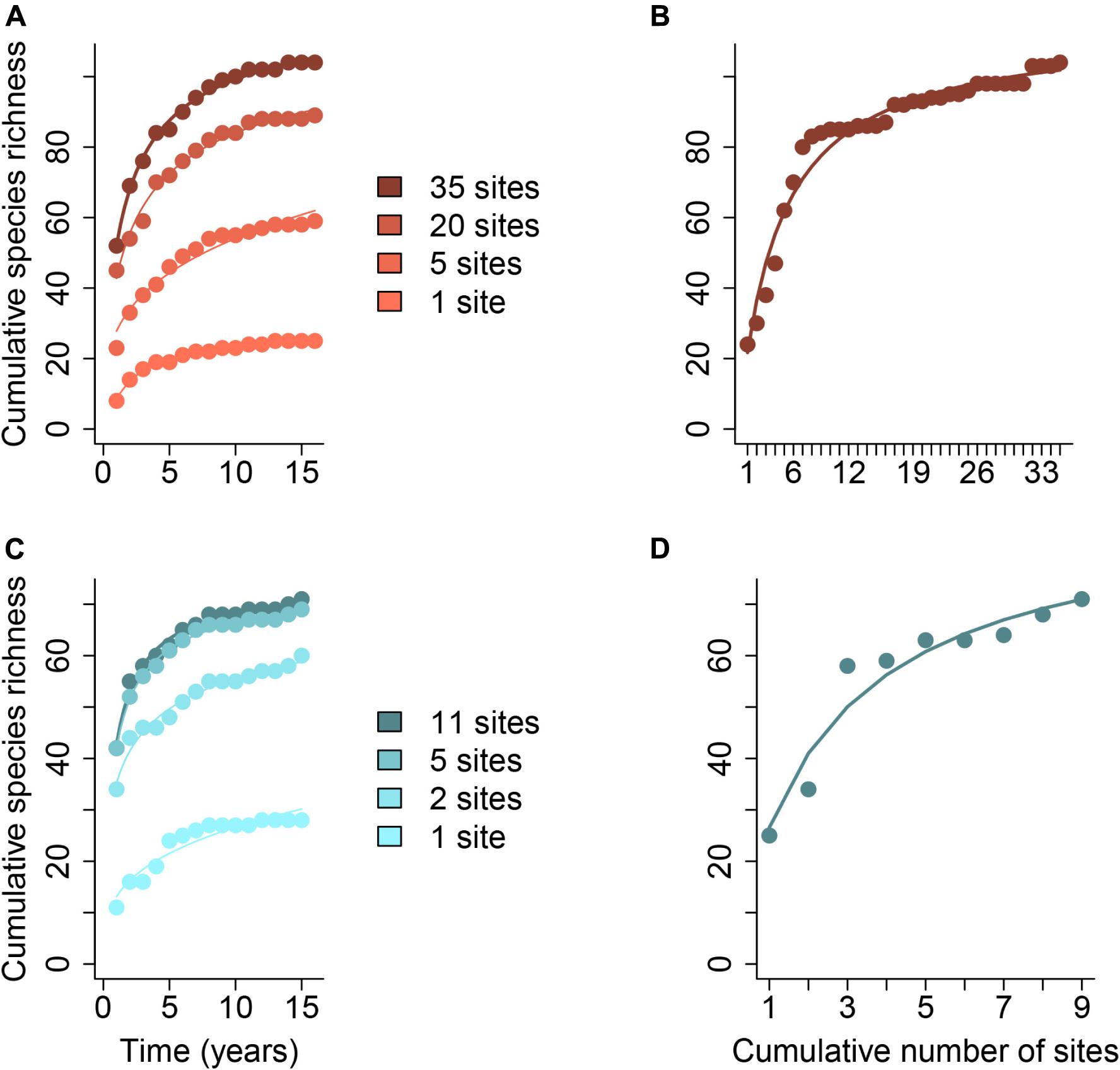
Figure 1. Species accumulation curves over time (years) and space with increasing numbers of study sites, indicated with different colors. Species accumulation curves through time (A,C) and space (B,D) are shown for birds from the Central Arizona – Phoenix United States LTER (2001–2016, Bateman et al., 2017) (A,B) and for sessile invertebrates from the Santa Barbara Channel United States LTER (2004–2016, Reed, 2018) (C,D). The total number of species increases with the number of study sites, and the steepness of the curve also tends to increase with the number of study sites. Curves were fit according to the Arrhenius, Lomolino, and Michaelis-Menten models described in Dengler (2009) using the R package vegan (Oksanen et al., 2017). The most parsimonious model (lowest AIC) is shown for each subset of sites. Data and R code for generating this figure can be found in the Supplementary Data Sheets 2–4.
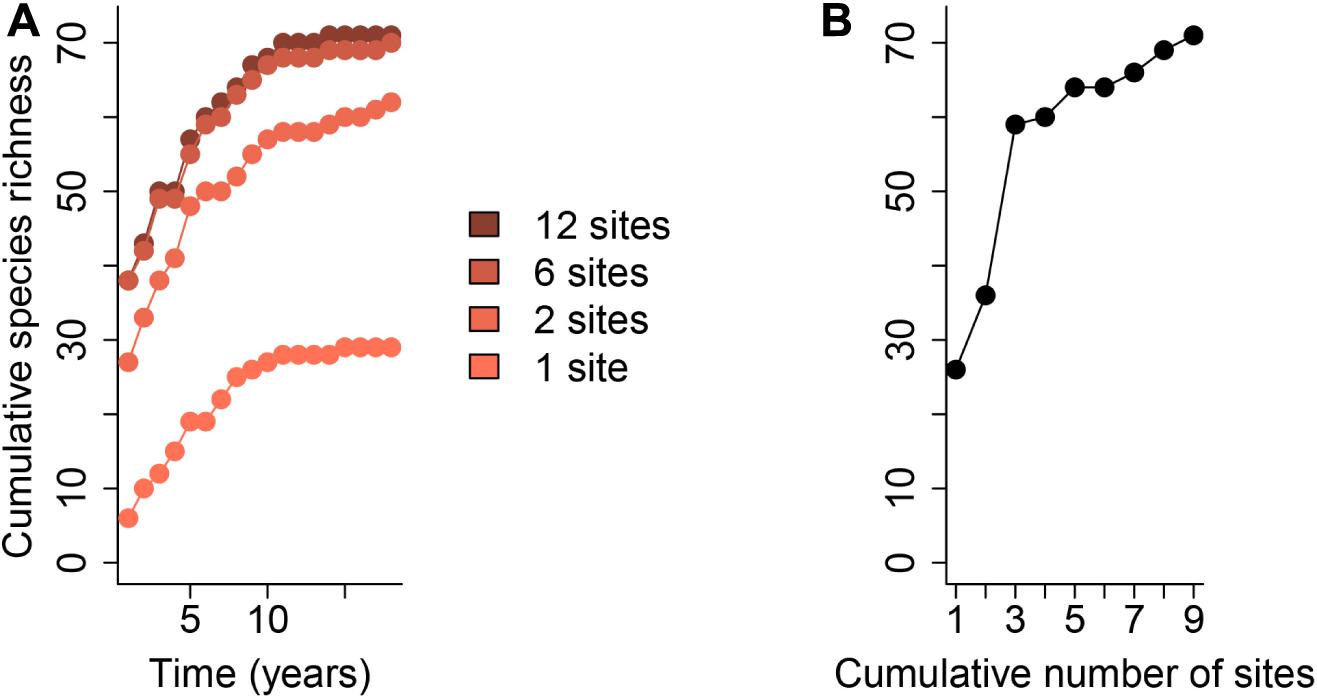
Figure 2. Changes in a regional species pool over time. The species accumulation curve for a collection of sites may not level off over time due to the successional nature of communities, species invasions, or environmental change. (A) shows the species accumulation curve over 21 years for plants growing in the pumice plain habitat on Mt. St. Helens, United States (1989–2009; del Moral, 2010), a community undergoing succession following volcanic eruption. (B) shows the species accumulation curve over space for the same sites. Data and R code for generating this figure can be found in the Supplementary Data Sheets 1, 4.
Long-term, spatially replicated data collection allows for the data to suggest the relevant spatial extent to study for characterizing the regional species pool for a given question. For example, researchers can see whether spatial or temporal samples saturate first and which sites continue to reveal more species diversity with greater sampling extent. Then, by looking at the temporal curves in a spatial context, researchers can better understand the most dynamic or undersampled regions of the landscape. This may help identify local sites that are on the periphery of the metacommunity that may be less influenced by spatio-temporal variation and/or dispersal (i.e., locally saturated sites whose composition is nested inside of the composition of other sites); and sites that are important integrators of spatio-temporal processes or important dispersal connections (i.e., sites with highly variable composition where repeated sampling continues to reveal new species and different composition). In a similar vein, Erõs and Schmera (2010) combined field survey data on fish in a temporally and spatially dynamic stream system with simulation experiments to explore how spatial and temporal scales and their interaction influence species accumulation, which can lead to different inferences about metacommunity organization (e.g., the role of spatial versus environmental effects; Sály and Erõs, 2016).
However, it is also important to note the limitations of species-time-area-relationships in evaluating sample representativeness over space and time within the context of understanding spatio-temporal metacommunity dynamics. For instance, species-time-area-relationship curves do not reveal insights about the temporal variability in changes in the abundance of organisms within and between sites, which is central to understanding mechanisms of metacommunity assembly [e.g., mass effects, species recovery from a historic disturbance (Scheffer, 2010)]. A new method for distinguishing changes in species richness across space due to separate effects of species abundance distribution, density, and the spatial configuration of individuals on a landscape presented by McGlinn et al. (2020) presents a promising path forward for more informed assessments of species-area-relationships, but incorporation of the temporal component into such new methods remains lacking. Regardless, we recommend that monitoring programs include the longitude and latitude of each sample plot if they want to be amenable to this new method, which requires plot locality information.
Another key aspect of some observation networks (e.g., LTER, LTAR) is the existence of manipulative experiments that may also aid in teasing apart pattern from process in metacommunities. Observational studies considering metacommunity dynamics across space and time can still result in limited inferences about the dynamics of a metacommunity in the future. Most ecological systems may exist in different states wherein species compositions and abundances vary considerably, for example, with gradual shifts between states during succession or abrupt transitions when tipping points are reached (Suding and Hobbs, 2009). Even with extensive time series, it may be difficult to know where organisms within communities and metacommunities lie on a given temporal trajectory relative to a previous disturbance or an impending state change (Bestelmeyer et al., 2011). By contrast, field experiments with manipulations that influence aspects of metacommunity dynamics present the opportunity to test theoretical metacommunity frameworks to learn where theory aligns with observations (Logue et al., 2011), thus allowing for more robust predictions.
Long-term field manipulations are a key component of most United States LTER Network and other long-term ecological research programs because they can experimentally test how biodiversity responds to ecological processes occurring over extended periods (Turner et al., 2003). For example, by simulating the loss of eastern hemlock (Tsuga canadensis) due to the invasive wooly adelgid (Adelges tsugae) and measuring ant biodiversity for 13 years, researchers at the Harvard Forest LTER found that this experimental disturbance reduced the importance of species sorting for community composition (Sackett et al., 2011; Record et al., 2018). Long-term manipulations can also reveal how biodiversity responds to repeated environmental fluctuations, yielding knowledge that cannot be obtained with short-term experiments. By simulating annual kelp forest loss from ocean storms for 9 years, researchers at the Santa Barbara Coastal LTER demonstrated strong shifts in marine biodiversity that were contrary to findings from an earlier 2-year study (Castorani et al., 2018).
The Cedar Creek Biodiversity Experiment is a long-term manipulation of soil nitrogen availability and plant diversity that critically demonstrated the importance of biodiversity for ecosystem functioning (Tilman et al., 2012). This experiment has also allowed United States LTER researchers to answer additional questions related to community assembly and metacommunity ecology. For example, to assess the extent to which plant communities were dispersal-limited, seed mixtures were added into plots of a native grassland (Tilman, 1997). Increased seed additions led to greater local species richness, providing evidence that some species are dispersal limited (Tilman, 1997). Seed addition experiments have also shown that local species interactions, such as resource competition, are important for structuring local plant communities (Fargione et al., 2003). These results, when placed in the broader context of metacommunity ecology, show how both local and regional processes can influence biodiversity.
Long-term ecological research programs situated within a network of sites are critically important for understanding trends in biodiversity, especially when historical contexts are known and infrastructure enables long-term field experiments. Data sets published by observation networks often contain abundant and co-located biotic and abiotic data to investigate patterns of biodiversity through the lens of metacommunity theory. For example, spatio-temporal metacommunity dynamics can be investigated across numerous ecosystems and taxa by means of NEON’s frequent and coordinated biotic and abiotic sampling within plots or stream reaches nested within sites across 20 climatic domains (Keller et al., 2008). Such networks provide economies of scale for infrastructure for long-term and networked sites, dedicated human resources for data collection, and taxonomic expertise (Bourgeron et al., 2018). Based on insights we made while curating LTER data for metacommunity analyses, we identify challenges towards synthesizing these data and present recommendations for addressing these challenges. We also provide guidance for long-term monitoring programs based on our insights.
Challenges to Advancing Metacommunity Science and Paths Forward
Challenge One – Scale Mismatch Among Data Sets in Synthesis Efforts
To address broad questions about the generality of the metacommunity framework in ecology, it is necessary to broadly test the theory across ecosystems and organisms. This presents a challenge with respect to mismatches in temporal and spatial scaling across data sets (Lamy et al., 2018). Observatory networks can employ various scales of temporal resolution – days, weeks, seasons, years, or even decades and plot sizes (e.g., Keller et al., 2008). Cross-ecosystem syntheses of biodiversity often compare data that has been aggregated or standardized to a common temporal or spatial grain (e.g., annual observation frequencies; Collins et al., 2018). However, trends or shifts in biodiversity can be affected by species’ generation times (Kuussaari et al., 2009), phenological patterns, and the frequency and duration of dispersal events (e.g., Tilman, 1997) and environmental fluctuations (e.g., Free et al., 2013), which do not always align with common ways of standardizing space and time across data sets (e.g., annual sampling schedules).
In light of these challenges, research questions focused on understanding the underlying processes that structure metacommunity assembly (i.e., species interactions, environmental filtering, dispersal limitation) must be aware of heterogeneity in sampling effort and spatial grain (i.e., plot size) across studies, which biodiversity estimates and variability among samples are sensitive to [Chase and Knight (2013), Spake et al. (2020)]. Spake et al. (2020) suggest that in formal meta-analyses scale dependence in effect sizes may be assessed using meta-regressions exploring relationships between either spatial (i.e., plot size) or temporal (i.e., sampling interval) grain and effect sizes across studies. They also illustrate with simulated community data how effect sizes calculated with the log response ratio metric applied to biodiversity estimates (i.e., species richness) were more accurate than those calculated with the common Hedge’s g metric. In instances, when effect sizes applied to biodiversity estimates are highly scale dependent, the use of a scale-independent metric (e.g., Hurlbert’s Probability of Interspecific Encounter) is preferred (Chase and Knight, 2013). Given the challenges of synthesizing biodiversity data with varying grains of sampling in space and time, we have two recommendations for monitoring programs. First, ensure that raw data are published with ample metadata, so that synthesis researchers can extract relevant information on grains of sampling (Spake et al., 2020). Second, we recommend that programs coordinate efforts to agree upon standardized sampling protocols for particular taxa to promote synthesis (see more specifics on such coordination in the Challenge Three subsection below).
Challenge Two – Rare Species
In a non-stationary world, rare species will be crucial for predicting future states of novel ecosystems (Lyons et al., 2005; Jain et al., 2014). However, trends and patterns observed for rare taxa can be challenging to interpret because they arise from a combination of observation error and stochastic colonization dynamics (Hanski et al., 2004; McGill et al., 2007). Capturing rare species dynamics is an important step in quantifying the regional species pool, which is an essential component of studies that embrace spatial dimensions and/or dispersal. Only with long-term temporal data from multiple sites can one understand the presence or absence of rare taxa because transient or local dynamics can influence how community assembly proceeds (Brown et al., 1995; Pandit et al., 2009; Siqueira et al., 2012). Local and regional species composition patterns universally contain a few dominant species, while most taxa are rare and show stochastic local colonization and extinction dynamics (Hanski et al., 2004; McGill et al., 2007). Although dominant species can contribute disproportionately to ecosystem function (Degrassi et al., 2019), rare species can also contribute meaningfully to ecosystem functions and services through novel additions to functional diversity and functional redundancy in a community (Lyons et al., 2005; Jain et al., 2014; Leitao et al., 2016). Despite the low abundances of rare species, it is critical to better understand how they contribute to community trait diversity and resilience, as environmental change may favor their increase in abundance and influence future ecosystem functioning (Tilman and Downing, 1994; Lyons et al., 2005; MacDougall et al., 2013; Jain et al., 2014).
Based on insights from our LTER data synthesis, we suggest observation networks balance temporal and spatial replication to better characterize the regional species pool, including rare taxa (Box 1). For instance, a higher frequency of observations is necessary to capture seasonally distinct communities or seasonally rare taxa (Tonkin et al., 2017). Another approach would be to implement adaptive cluster sampling to capture rare species, where the study area is spatially partitioned into a grid and the intensity of survey effort is intensified around grid cells with higher counts of particular rare species (Brown et al., 2013). Also, working groups should develop strategies for monitoring and interpreting future trends of the rare taxa that might predict invasion or threshold responses in future climate scenarios.
Challenge Three – Economies of Scale
It can often be difficult to assess whether long-term data are collected at optimal temporal resolutions and spatial extents to capture all relevant community assembly dynamics (e.g., dispersal kernel shape, demographic rates) or structural characteristics (e.g., spatial heterogeneity of suitable habitat, species occurrence, biomass). Increasing the spatial extent of data collection around existing long-term study sites will enable researchers and managers to compile the information needed for understanding trends in biodiversity and will allow for better characterization of regional species pools informed by data. Resources are often not available in any individual research program to capture both the necessary spatial and temporal resolution. There is a growing need for both spatial and temporal replication in biodiversity data, which requires a plan for coordination among single-PI projects and multiple long-term observatories in a network of networks to provide economies of scale within the research community.
We recommend that scientific societies provide a hub for coordination among and between single-PI and large-scale observation networks to help identify opportunities where single-PI and observation network projects can fill complementary knowledge gaps. For instance, the National Science Foundation’s Macrosystems Biology program and NEON-Enabled Science solicitations provide an opportunity for short duration (3 years) studies that leverage NEON infrastructure. We also recommend that researchers use cross-project collaborative opportunities (e.g., Research Coordination Network working groups, United States LTER All Scientist Meeting working groups) to establish data collection priorities and standards for advancing metacommunity research.
Data collection priorities could inform monitoring frequency and spatial replication based on organismal life histories (e.g., time until reproduction, dispersal abilities) and current knowledge gaps (Wolfe et al., 1987). Adopting pre-existing, standardized sampling and data archiving protocols that are consistent among sites will also enable researchers and policy makers to scale-up local studies to global scale research initiatives (e.g., Group on Earth Observations – Biodiversity Observation Network’s essential biodiversity variables; Haase et al., 2018). Recent proposals for integrating measures of biodiversity and ecosystem integrity across observatory networks may advance synergy within and among these networks (Haase et al., 2018). In addition to observation networks, spatially replicated studies in long-term databases, such as BioTime (Dornelas et al., 2018), offer additional data sources.
Data standards can also aid synthesis efforts. Furthermore, data to be used for multi-site analyses are best archived in harmonized datasets (with consistent structure and format). Examples include the GLEON DataONE Repository for synchronized hydrological sensor data2 and the ecocomDP standard data pattern for community data3 that members of our group have developed with the Environmental Data Initiative (EDI)4 for implementation in their data portal, which publishes data products from the United States LTER and NSF Macrosystems Biology programs. A key outcome of the LTER synthesis group that we are a part of has been to harmonize LTER community ecology data sets into the ecocomDP standard data pattern to promote future use of LTER data in metacommunity studies. A key first step in using LTER time series to address hypotheses in metacommunity ecology is the identification of appropriate data sets. The analysis ready data provided by ecocomDP provides additional metadata for improved discovery with information on taxonomic resolutions, and nesting of sampling designs over space and time – key pieces of information for identifying the suitability of a data set for a metacommunity study. Currently, seventy community ecology data sets generated by the LTER network have been formatted into the ecocomDP standard data pattern and there are ∼100 more data sets in the queue for processing. Completed data sets are discoverable by going to the EDI Data Repository5 and searching for the term “ecocomDP.” Ultimately, these types of harmonized data sets will allow for greater advances in metacommunity studies because efforts to clean and format data leading up to analyses do not have to be repeatedly performed by individual researchers and there is the additional benefit that results from studies can then also be more reproducible (Reichman et al., 2011).
Challenge 4 – Statistical Integration of Long-Term, Spatially Replicated Data With Theory
Although we are at a point where some observation networks have amassed long-term, spatially replicated community data sets with saturating species-time-area relationships, the statistical integration of these data with theoretical concepts remains a key challenge. There have long been calls for moving beyond the classic metacommunity conceptual archetypes (i.e., mass effects, patch dynamics, species sorting, neutral theory) to better account for temporal dynamics, but theoretical and statistical approaches remain incomplete (Leibold and Chase, 2017). For instance, ecosystem stability over large spatial scales can be addressed in a metacommunity framework with long-term, spatially replicated data (Wang and Loreau, 2014, 2016). However, such analyses often consider aggregate metrics of ecosystems (e.g., biomass) rather than species diversity and composition, which may be of greater interest to federal agencies or non-governmental organizations, as these often uphold policy based on species diversity rather than aggregate metrics (i.e., the Endangered Species Act).
Greater strides in metacommunity science will be made as long-term, spatially replicated observation network data enter a loop, wherein the data impart information into the development of models and theory, and models and theory inform future data collection (Dietze, 2017). A promising path forward involves incorporating novel approaches to quantify metacommunity dynamics [e.g., joint species distribution models (Ovaskainen et al., 2019), open-source simulation tools (Sokol et al., 2017), process-based models (Keyel et al., 2016; Thompson et al., 2020)] into such cyclical rapid data assimilation and model/theory refinement. For instance, recent work by Thompson et al. (2020) revisits the metacommunity concept with a process-based framework that integrates local and regional dynamics of ecological communities with three main underlying dimensions (i.e., density independent responses to abiotic conditions, density-dependent biotic interactions, and dispersal) that link to the classic metacommunity conceptual archetypes. Near-term forecasting of process-based metacommunity models based on this reconceived metacommunity framework could help to identify which species traits best capture variation within and between species that influence density dependence to inform future monitoring and data collection efforts.
Prospectus
Given the alarming rate at which biodiversity and associated ecosystem services are being lost (Ceballos et al., 2015; Johnson et al., 2017), understanding changes in biodiversity in both space and time is fundamental for science-informed conservation. While the metacommunity framework has the potential to uncover mechanisms explaining biodiversity patterns to inform conservation, the lack of spatio-temporal data has hindered researchers’ ability to disentangle environmental drivers from biotic niche-based processes generated within the community. Incorporating a long-term temporal dimension into field-based metacommunity research is key to understanding the mechanisms generating observed patterns in biodiversity. However, resources for field studies are limited and temporal replication often comes at the expense of spatial replication and taxonomic resolution (e.g., Keller et al., 2008). Large, collaborative observation networks provide the opportunity to inform metacommunity theory with empirical data at spatial and temporal scales greater than any single researcher could accomplish on their own. To better understand how metacommunity dynamics operate in reality, the infrastructure of manipulative field experiments at sites within these networks allows ecologists to test challenging questions posed by metacommunity theory at real-world scales. Looking forward, such large-scale efforts can be better leveraged (Bourgeron et al., 2018) to address issues of scale mismatches in data synthesis, rare species, economies of scale, and the integration of data with theory. By coordinating biodiversity research efforts, ecologists will better understand how and why species persist across space and time, and how biodiversity patterns emerge across a diverse range of ecosystems and over long temporal scales.
Data Availability Statement
The original contributions presented in the study are included in the article/Supplementary Material, further inquiries can be directed to the corresponding author.
Author Contributions
SR, NV, and ES led the writing and editing of the manuscript. ES, NW, and CS secured funding from the LTER to support the working group. All authors contributed to the conceptualization, review, data collection, and editing of the manuscript.
Funding
This work was conducted as a part of the LTER Metacommunity Dynamics and Community Responses to Disturbance Synthesis Group funded by the National Science Foundation under grant DEB#1545288, administered through the Long Term Ecological Research Network Communications Office (LNCO), National Center for Ecological Analysis and Synthesis, University of California, Santa Barbara. ecocommDP work by SR, NL, ES, and MC was funded by the National Science Foundation under grants DBI#1931143 to M. Servilla and DBI#1931174 to C. Gries. SR was additionally supported by National Science Foundation grant DEB#1926568.
Conflict of Interest
The authors declare that the research was conducted in the absence of any commercial or financial relationships that could be construed as a potential conflict of interest.
Supplementary Material
The Supplementary Material for this article can be found online at: https://www.frontiersin.org/articles/10.3389/fevo.2020.612794/full#supplementary-material
Supplementary Data Sheet 1 | The plant data from Mt. St. Helens in Figure 2 of Box 1.
Supplementary Data Sheet 2 | The bird data from the Central Arizona Phoenix LTER in Figure 1 of Box 1.
Supplementary Data Sheet 3 | The sessile invertebrate data from the Santa Barbara Channel LTER in Figure 1 of Box 1.
Supplementary Data Sheet 4 | The R code for generating the figures in Box 1.
Footnotes
- ^ http://www.nutnet.org/
- ^ http://gleon.org/data/repositories
- ^ https://github.com/EDIorg/ecocomDP
- ^ https://environmentaldatainitiative.org
- ^ https://portal.edirepository.org/nis/home.jsp
References
Adler, P. B., White, E. P., Lauenroth, W. K., Kaufman, D. M., Rassweiler, A., and Rusak, J. A. (2005). Evidence for a general species–time–area relationship. Ecology 86, 2032–2039. doi: 10.1890/05-0067
Bateman, H., Childers, D., Katti, M., Shochat, E., and Warren, P. (2017). Point-Count Bird Censusing: Long-Term Monitoring of Bird Abundance and Diversity in Central Arizona-Phoenix, Ongoing Since 2000. Environmental Data Initiative. Available online at: https://doi.org/10.6073/pasta/201add557165740926aab6e056db6988 (accessed December 15, 2020).
Bellard, C., Bertelsmeier, C., Leadley, P., Thuiller, W., and Courchamp, F. (2012). Impacts of climate change on the future of biodiversity. Ecol. Lett. 15, 365–377. doi: 10.1111/j.1461-0248.2011.01736.x
Bestelmeyer, B. T., Ellison, A. M., Fraser, W. R., Gorman, K. B., Holbrook, S. J., Laney, C. M., et al. (2011). Analysis of abrupt transitions in ecological systems. Ecosphere 2:art129. doi: 10.1890/ES11-00216.1
Bourgeron, P., Kliskey, A., Alessa, L., Loescher, H., Krauze, K., Virapongse, A., et al. (2018). Understanding large-scale, complex, human–environmental processes: a framework for social–ecological observatories. Front. Ecol. Environ. 16, S52–S66. doi: 10.1002/fee.1797
Brook, B. W., Sodhi, N. S., and Bradshaw, C. J. (2008). Synergies among extinction drivers under global change. Trends Ecol. Evol. 23, 453–460. doi: 10.1016/j.tree.2008.03.011
Brown, B. L., Sokol, E. R., Skelton, J., and Tornwall, B. (2017). Making sense of metacommunities: dispelling the mythology of a metacommunity typology. Oecologia 183, 643–652. doi: 10.1007/s00442-016-3792-1
Brown, J. A., Salehi, M., Moradi, M., Panahbehagh, B., and Smith, D. R. (2013). Adpative survey designs for sampling rare and clustered populations. Math. Comput. Simul. 93, 108–116. doi: 10.1016/j.matcom.2012.09.008
Brown, J. H., Mehlman, D. W., and Stevens, G. C. (1995). Spatial variation in abundance. Ecology 76, 2028–2043. doi: 10.2307/1941678
Cardinale, B. J., Duffy, J. E., Gonzalez, A., Hooper, D. U., Perrings, C., Venail, P., et al. (2012). Biodiversity loss and its impact on humanity. Nature 486, 59–67. doi: 10.1038/nature11148
Castorani, M. C., Reed, D. C., and Miller, R. J. (2018). Loss of foundation species: disturbance frequency outweighs severity in structuring kelp forest communities. Ecology 99, 2442–2454. doi: 10.1002/ecy.2485
Ceballos, G., Ehrlich, P. R., Barnosky, A. D., García, A., Pringle, R. M., and Palmer, T. M. (2015). Accelerated modern human–induced species losses: entering the sixth mass extinction. Sci. Adv. 1:e1400253. doi: 10.1126/sciadv.1400253
Chase, J. M. (2003). Community assembly: when should history matter? Oecologia 136, 489–498. doi: 10.1007/s00442-003-1311-7
Chase, J. M., and Knight, T. M. (2013). Scale-dependent effect sizes of ecological drivers on biodiversity: why standardised sampling is not enough. Ecol. Lett. 16:17–26. doi: 10.1111/ele.12112
Collins, S. L., Avolio, M. L., Gries, C., Hallett, L. M., Koerner, S. E., and Pierre, K J. La, et al. (2018). Temporal heterogeneity increases with spatial heterogeneity in ecological communities. Ecology 99, 858–865. doi: 10.1002/ecy.2154
Cottenie, K. (2005). Integrating environmental and spatial processes in ecological community dynamics. Ecol. Lett. 8, 1175–1182. doi: 10.1111/j.1461-0248.2005.00820.x
Datry, T., Melo, A. S., Moya, N., Zubieta, J., De la Barra, E., and Oberdorff, T. (2016). Metacommunity patterns across three Neotropical catchments with varying environmental harshness. Freshw. Biol. 61, 277–292. doi: 10.1111/fwb.12702
De Bie, T., De Meester, L., Brendonck, L., Martens, K., Goddeeris, B., Ercken, D., et al. (2012). Body size and dispersal mode as key traits determining metacommunity structure of aquatic organisms. Ecol. Lett. 15, 740–747. doi: 10.1111/j.1461-0248.2012.01794.x
Degrassi, A. L., Brantley, S., Levine, C. R., Mohan, J., Record, S., Tomback, D. F., et al. (2019). Loss of foundation species revisited: conceptual framework with lessons learned from eastern hemlock and whitebark pine. Ecosphere 10:e02917.
Dengler, J. (2009). Which function describes the species–area relationship best? A review and empirical evaluation. J. Biogeogr. 36, 728–744. doi: 10.1111/j.1365-2699.2008.02038.x
Díaz, S., Settele, J., Brondízio, E., Ngo, H., Guèze, M., Agard, J., et al. (2019). Summary for policymakers of the global assessment report on biodiversity and ecosystem services of the intergovernmental science-policy platform on biodiversity and ecosystem services. Popul. Dev. Rev. 45, 680–681. doi: 10.1111/padr.12283
Dornelas, M., Antão, L. H., Moyes, F., Bates, A. E., Magurran, A. E., Adam, D., et al. (2018). BioTIME: a database of biodiversity time series for the Anthropocene. Glob. Ecol. Biogeogr. 27, 760–786. doi: 10.1111/geb.12729
Dudgeon, D., Arthington, A. H., Gessner, M. O., Kawabata, Z.-I., Knowler, D. J., Lévêque, C., et al. (2006). Freshwater biodiversity: importance, threats, status and conservation challenges. Biol. Rev. 81, 163–182.
Erõs, T., and Schmera, D. (2010). Spatio-temporal scaling of bioidiversity and the species-time relationship in a stream fish assemblage. Freshw. Biol. 55:2391–2400. doi: 10.1111/j.1365-2427.2010.02438.x
Fahrig, L. (2003). Effects of habitat fragmentation on biodiversity. Annu. Rev. Ecol. Evol. Syst. 34, 487–515.
Fargione, J., Brown, C. S., and Tilman, D. (2003). Community assembly and invasion: an experimental test of neutral versus niche processes. Proc. Natl. Acad. Sci. U.S.A. 100, 8916–8920. doi: 10.1073/pnas.1033107100
Fischer, J., and Lindenmayer, D. B. (2007). Landscape modification and habitat fragmentation: a synthesis. Glob. Ecol. Biogeogr. 16, 265–280. doi: 10.1111/j.1466-8238.2007.00287.x
Free, C. L., Baxter, G. S., Dickman, C. R., and Leung, L. K. P. (2013). Resource pulses in desert river habitats: productivity-biodiversity hotspots, or mirages? PLoS One 8:e72690. doi: 10.1371/journal.pone.0072690
Frishkoff, L. O., Karp, D. S., M’Gonigle, L. K., Mendenhall, C. D., Zook, J., Kremen, C., et al. (2014). Loss of avian phylogenetic diversity in neotropical agricultural systems. Science 345, 1343–1346. doi: 10.1126/science.1254610
Fukami, T. (2015). Historical contingency in community assembly: integrating niches, species pools, and priority effects. Annu. Rev. Ecol. Evol. Syst. 46, 1–23. doi: 10.1146/annurev-ecolsys-110411-160340
Grimm, N. B., Chapin, F. S., Bierwagen, B., Gonzalez, P., Groffman, P. M., Luo, Y., et al. (2013). The impacts of climate change on ecosystem structure and function. Front. Ecol. Environ. 11, 474–482. doi: 10.1890/120282
Haase, P., Tonkin, J. D., Stoll, S., Burkhard, B., Frenzel, M. I, Geijzendorffer, R., et al. (2018). The next generation of site-based long-term ecological monitoring: linking essential biodiversity variables and ecosystem integrity. Sci. Total Environ. 613, 1376–1384. doi: 10.1016/j.scitotenv.2017.08.111
Hanski, I. A., Gaggiotti, O. E., and Gaggiotti, O. F. (eds) (2004). Ecology, Genetics and Evolution of Metapopulations. Cambridge, MA: Academic Press.
Holyoak, M., Caspi, T., and Redosh, L.W. (2020). Integrating disturbance, seasonality, multi-year temporal dynamics, and dormancy into the dynamics and conservation of metacommunities. Front. Ecol. Evol. 8:571130. doi: 10.3389/fevo.2020.571130
Hooper, D. U., Adair, E. C., Cardinale, B. J., Byrnes, J. E., Hungate, B. A., Matulich, K. L., et al. (2012). A global synthesis reveals biodiversity loss as a major driver of ecosystem change. Nature 486, 105. doi: 10.1038/nature11118
Hughes, B. B., Beas-Luna, R., Barner, A. K., Brewitt, K., Brumbaugh, D. R., Cerny-Chipman, E. B., et al. (2017). Long-term studies contribute disproportionately to ecology and policy. Bioscience 67, 271–281. doi: 10.1093/biosci/biw185
Jain, M., Flynn, D. F., Prager, C. M., Hart, G. M., DeVan, C. M., Ahrestani, F. S., et al. (2014). The importance of rare species: a trait-based assessment of rare species contributions to functional diversity and possible ecosystem function in tall-grass prairies. Ecol. Evol. 4, 104–112. doi: 10.1002/ece3.915
Johnson, C. N., Balmford, A., Brook, B. W., Buettel, J. C., Galetti, M., Guangchun, L., et al. (2017). Biodiversity losses and conservation responses in the Anthropocene. Science 356, 270–275. doi: 10.1126/science.aam9317
Keller, M., Schimel, D. S., Hargrove, W. W., and Hoffman, F. M. (2008). A continental strategy for the National Ecological Observatory Network. Front. Ecol. Environ. 6, 282–284. doi: 10.1890/1540-9295(2008)6[282:acsftn]2.0.co;2
Keyel, A. C., Gerstenlauer, J. L., and Wiegand, K. (2016). SpatialDemography: a spatially explicit, stage-structured, metacommunity model. Ecography 39, 1129–1137. doi: 10.1111/ecog.02295
Kuussaari, M., Bommarco, R., Heikkinen, R. K., Helm, A., Krauss, J., Lindborg, R., et al. (2009). Extinction debt: a challenge for biodiversity conservation. Trends Ecol. Evol. 24, 564–571.
Lamy, T., Reed, D. C., Rassweiler, A., Siegel, D. A., Kui, L., Bell, T. W., et al. (2018). Scale-specific drivers of kelp forest communities. Oecologia 186, 217–233. doi: 10.1007/s00442-017-3994-1
Law, R., and Morton, R. D. (1993). Alternative permanent states of ecological communities. Ecology 74, 1347–1361. doi: 10.2307/1940065
Law, R., and Morton, R. D. (1996). Permanence and the assembly of ecological communities. Ecology 77, 762–775. doi: 10.2307/2265500
Leibold, M. A., and Chase, J. M. (2017). Metacommunity Ecology. Princeton, NJ: Princeton University Press.
Leibold, M. A., Holyoak, M., Mouquet, N., Amarasekare, P., Chase, J. M., Hoopes, M. F., et al. (2004). The metacommunity concept: a framework for multi-scale community ecology. Ecol. Lett. 7, 601–613. doi: 10.1111/j.1461-0248.2004.00608.x
Leitao, R. P., Zuanon, J., Villéger, S., Williams, S. E., Baraloto, C., Fortunel, C., et al. (2016). Rare species contribute disproportionately to the functional structure of species assemblages. Proc. R. Soc. B 283:20160084. doi: 10.1098/rspb.2016.0084
Li, D., Olden, J. L., Lockwood, J. L., Record, S., McKinney, M. L., and Baiser, B. (2020). Changes in taxonomic and phylogenetic diversity in the Anthropocene. Proc. R. Soc. B Biol. Sci. 287:20200777. doi: 10.1098/rspb.2020.0777
Logue, J. B., Mouquet, N., Peter, H., and Hillebrand, H. Metacommunity Working Group (2011). Empirical approaches to metacommunities: a review and comparison with theory. Trends Ecol. Evol. 26, 482–491. doi: 10.1016/j.tree.2011.04.009
Lyons, K. G., Brigham, C. A., Traut, B. H., and Schwartz, M. W. (2005). Rare species and ecosystem functioning. Conserv. Biol. 19, 1019–1024. doi: 10.1111/j.1523-1739.2005.00106.x
MacDougall, A. S., McCann, K. S., Gellner, G., and Turkington, R. (2013). Diversity loss with persistent human disturbance increases vulnerability to ecosystem collapse. Nature 494, 86–89. doi: 10.1038/nature11869
Magurran, A. E., Baillie, S. R., Buckland, S. T., Dick, J. M., Elston, D. A., Scott, E. M., et al. (2010). Long-term datasets in biodiversity research and monitoring: assessing change in ecological communities through time. Trends Ecol. Evol. 25, 574–582. doi: 10.1016/j.tree.2010.06.016
Martay, B., Monteith, D. T., Brewer, M. J., Brereton, T., Shortall, C. R., and Pearce-Higgins, J. W. (2016). An indicator highlights seasonal variation in the response of Lepidoptera communities to warming. Ecol. Indic. 68, 126–133. doi: 10.1016/j.ecolind.2016.01.057
McCallen, E., Knott, J., Nunez−Mir, G., Taylor, B., Jo, I., and Fei, S. (2019). Trends in ecology: shifts in ecological research themes over the past four decades. Front. Ecol. Environ. 17, 109–116. doi: 10.1002/fee.1993
McGill, B. J., Etienne, R. S., Gray, J. S., Alonso, D., Anderson, M. J., Benecha, H. K., et al. (2007). Species abundance distributions: moving beyond single prediction theories to integration within an ecological framework. Ecol. Lett. 10, 995–1015. doi: 10.1111/j.1461-0248.2007.01094.x
McGlinn, D. J., Xiao, X., May, F., Gotelli, N. J., Engel, T., Blowes, S. A., et al. (2020). Measurement of Biodiversity (MoB): a method to separate the scale-dependent effects of species abundance distribution, density, and aggregation on diversity change. Methods Ecol. Evol. 10, 258–269. doi: 10.1111/2041-210x.13102
del Moral, R. (2010). Thirty years of permanent vegetation plots, Mount St. Helens, Washington, USA. Ecology 91, 2185–2185. doi: 10.1890/09-2357.1
Mouquet, N., and Loreau, M. (2002). Coexistence in metacommunities: the regional similarity hypothesis. Am. Nat. 159, 420–426. doi: 10.1086/338996
Oksanen, J., Blanchet, F. G., Friendly, M., Kindt, R., Legendre, P., McGlinn, D., et al. (2017). Vegan: Community Ecology Package. R Package Version 2.4-3. Vienna: R Foundation for Statistical Computing.
Ovaskainen, O., Rybicki, J., and Abrego, N. (2019). What can observational data reveal about metacommunity processes? Ecography 42, 1877–1886. doi: 10.1111/ecog.04444
Pandit, S. N., Kolasa, J., and Cottenie, K. (2009). Contrasts between habitat generalists and specialists: an empirical extension to the basic metacommunity framework. Ecology 90, 2253–2262. doi: 10.1890/08-0851.1
Parmesan, C. (2006). Ecological and evolutionary responses to recent climate change. Annu. Rev. Ecol. Evol. Syst. 37, 637–669. doi: 10.1146/annurev.ecolsys.37.091305.110100
Parmesan, C., and Yohe, G. (2003). A globally coherent fingerprint of climate change impacts across natural systems. Nature 421, 37–42. doi: 10.1038/nature01286
Pickett, S. T. (1989). “Space-for-time substitution as an alternative to long-term studies,” in Long-Term Studies in Ecology, ed. G. E. Likens (New York, NY: Springer), 10–135.
Pulliam, H. R. (1988). Sources, sinks, and population regulation. Am. Nat. 132, 652–661. doi: 10.1086/284880
Record, S., McCabe, T., Baiser, B., and Ellison, A. M. (2018). Identifying foundation species in North American forests using long-term data on ant assemblage structure. Ecosphere 9, 02139.
Reed, D. C. (2018). SBC LTER: Reef: Annual Time Series of Biomass for Kelp Forest Species, Ongoing Since 2000. Environmental Data Initiative. Available online at: https://doi.org/10.6073/pasta/23965abf42954f345cfd6642fe3c4810 (accessed December 15, 2020).
Reichman, O. J., Jones, M. B., and Schildhauer, M. P. (2011). Challenges and opportunities of open data in ecology. Science 331, 703–705. doi: 10.1126/science.1197962
Sackett, T. E., Record, S., Bewick, S., Baiser, B. H., Sanders, N. J., and Ellison, A. M. (2011). Response of macroarthropod assemblages to the loss of hemlock (Tsuga canadensis), a foundation species. Ecosphere 2:art74. doi: 10.1890/es11-00155.1
Sály, P., and Erõs, T. (2016). Effect of field sampling design on variation partitioning in a dendritic stream network. Ecol. Complex. 28, 187–199. doi: 10.1016/j.ecocom.2016.05.002
Sarremejane, R., Cañedo-Argüelles, M., Prat, N., Mykrä, H., Muotka, T., and Bonada, N. (2017). Do metacommunities vary through time? Intermittent rivers as model systems. J. Biogeogr. 44, 2752–2763. doi: 10.1111/jbi.13077
Spake, R., Mori, A. S., Beckmann, M., Martin, P. A., Duguid, M. C., and Doncaster, C. P. (2020). Implications of scale dependence for cross-study syntheses of biodiversity differences. Ecol. Lett. (in press). doi: 10.1111/ele.13641
Scheffer, M. (2010). Complex systems: foreseeing tipping points. Nature 467, 411. doi: 10.1038/467411a
Seto, K. C., Güneralp, B., and Hutyra, L. R. (2012). Global forecasts of urban expansion to 2030 and direct impacts on biodiversity and carbon pools. Proc. Natl. Acad. Sci. U.S.A. 109, 16083–16088. doi: 10.1073/pnas.1211658109
Siqueira, T., Bini, L. M., Roque, F. O., Marques Couceiro, S. R., Trivinho-Strixino, S., and Cottenie, K. (2012). Common and rare species respond to similar niche processes in macroinvertebrate metacommunities. Ecography 35, 183–192. doi: 10.1111/j.1600-0587.2011.06875.x
Sokol, E. R., Brown, B. L., and Barrett, J. E. (2017). A simulation-based approach to understand how metacommunity characteristics influence emergent biodiversity patterns. Oikos 126, 723–737. doi: 10.1111/oik.03690
Steiner, C. F., and Leibold, M. A. (2004). Cyclic assembly trajectories and scale-dependent productivity–diversity relationships. Ecology 85, 107–113. doi: 10.1890/03-3010
Suding, K. N., and Hobbs, R. J. (2009). Threshold models in restoration and conservation: a developing framework. Trends Ecol. Evol. 24, 271–279. doi: 10.1016/j.tree.2008.11.012
Thompson, P. L., Guzman, L. M., De Meester, L., Horváth, Z., Ptacnik, R., Vanschoenwinkel, B., et al. (2020). A process-based metacommunity framework linking local and regional scale community ecology. Ecol. Lett. 23, 1314–1329. doi: 10.1111/ele.13568
Tilman, D. (1997). Community invasibility, recruitment limitation, and grassland biodiversity. Ecology 78, 81–92. doi: 10.1890/0012-9658(1997)078[0081:cirlag]2.0.co;2
Tilman, D., and Downing, J. A. (1994). Biodiversity and stability in grasslands. Nature 367, 363. doi: 10.1038/367363a0
Tilman, D., May, R. M., Lehman, C. L., and Nowak, M. A. (1994). Habitat destruction and the extinction debt. Nature 371, 65–66. doi: 10.1038/371065a0
Tilman, D., Reich, P. B., and Isbell, F. (2012). Biodiversity impacts ecosystem productivity as much as resources, disturbance, or herbivory. Proc. Natl. Acad. Sci. U.S.A. 109, 10394–10397. doi: 10.1073/pnas.1208240109
Tonkin, J. D., Bogan, M. T., Bonada, N., Rios-Touma, B., and Lytle, D. A. (2017). Seasonality and predictability shape temporal species diversity. Ecology 98, 1201–1216. doi: 10.1002/ecy.1761
Tonkin, J. D., Death, R. G., Muotka, T., Astorga, A., and Lytle, D. A. (2018). Do latitudinal gradients exist in New Zealand stream invertebrate metacommunities? PeerJ. 6:e4898. doi: 10.7717/peerj.4898
Turner, M. G., Collins, S. L., Lugo, A. L., Magnuson, J. J., Rupp, T. S., and Swanson, F. J. (2003). Disturbance dynamics and ecological response: the contribution of long-term ecological research. AIBS Bull. 53, 46–56. doi: 10.1641/0006-3568(2003)053[0046:ddaert]2.0.co;2
Tylianakis, J. M., Didham, R. K., Bascompte, J., and Wardle, D. A. (2008). Global change and species interactions in terrestrial ecosystems. Ecol. Lett. 11, 1351–1363. doi: 10.1111/j.1461-0248.2008.01250.x
Urban, M. C. (2015). Accelerating extinction risk from climate change. Science 348, 571–573. doi: 10.1126/science.aaa4984
Wang, S., and Loreau, M. (2014). Ecosystem stability in space: α, β and γ variability. Ecol. Lett. 17, 891–901. doi: 10.1111/ele.12292
Wang, S., and Loreau, M. (2016). Biodiversity and ecosystem stability across scales in metacommunities. Ecol. Lett. 19, 510–518. doi: 10.1111/ele.12582
Wilcox, K. R., Tredennick, A. T., Koerner, S. E., Grman, E., Hallett, L. M., Avolio, M. L., et al. (2017). Asynchrony among local communities stabilises ecosystem function of metacommunities. Ecol. Lett. 20, 1534–1545. doi: 10.1111/ele.12861
Wolfe, D. A., Champ, M. A., Flemer, D. A., and Mearns, A. J. (1987). Long-term biological data sets: their role in research, monitoring, and management of estuarine and coastal marine systems. Estuaries 10:181. doi: 10.2307/1351847
World Health Organization (2005). Millennium Ecosystem Assessment: Ecosystems and Human Well-Being. Geneva: World Health Organization.
Keywords: LTER, NCO, synthesis, metacommunity, biodiversity, spatio-temporal, long-term
Citation: Record S, Voelker NM, Zarnetske PL, Wisnoski NI, Tonkin JD, Swan C, Marazzi L, Lany N, Lamy T, Compagnoni A, Castorani MCN, Andrade R and Sokol ER (2021) Novel Insights to Be Gained From Applying Metacommunity Theory to Long-Term, Spatially Replicated Biodiversity Data. Front. Ecol. Evol. 8:612794. doi: 10.3389/fevo.2020.612794
Received: 30 September 2020; Accepted: 03 December 2020;
Published: 14 January 2021.
Edited by:
Pedro Giovâni Da Silva, Federal University of Minas Gerais, BrazilReviewed by:
Tibor Eros, Centre for Ecological Research, Hungarian Academy of Sciences, HungaryAndreu Castillo-Escrivà, University of Valencia, Spain
Joseph Mihaljevic, Northern Arizona University, United States
Copyright © 2021 Record, Voelker, Zarnetske, Wisnoski, Tonkin, Swan, Marazzi, Lany, Lamy, Compagnoni, Castorani, Andrade and Sokol. This is an open-access article distributed under the terms of the Creative Commons Attribution License (CC BY). The use, distribution or reproduction in other forums is permitted, provided the original author(s) and the copyright owner(s) are credited and that the original publication in this journal is cited, in accordance with accepted academic practice. No use, distribution or reproduction is permitted which does not comply with these terms.
*Correspondence: Sydne Record, c3JlY29yZEBicnlubWF3ci5lZHU=