- 1Laboratoire de Biométrie et Biologie Evolutive, UMR 5558, Centre National de la Recherche Scientifique, Université Lyon 1, Université de Lyon, Villeurbanne Cedex, France
- 2CEFE, Univ. Montpellier, CNRS, EPHE, IRD, Univ. Paul Valéry Montpellier 3, Montpellier, France
- 3Mammal Research Institute, Department of Zoology and Entomology, Faculty of Natural and Agricultural Sciences, University of Pretoria, Pretoria, South Africa
- 4CNRS Hwange Environmental Research Development Program, Long-Term Socio-Ecological Research site France, Zone Atelier “Hwange”, Dete, Zimbabwe
- 5Wildlife Conservation Research Unit, Department of Zoology, Recanati-Kaplan Centre, Oxford University, Oxford, United Kingdom
Climate changes affect the distribution and abundance of organisms, often via changes in species interactions. Most animals experience predation, and a number of models have investigated how climate fluctuations can influence predator–prey dynamics by affecting prey abundance through changes in resource availability. However, field studies have shown that prey vulnerability is a key feature determining the outcome of predator–prey interactions, which also varies with climatic conditions, via changes in prey body condition or in habitat characteristics (e.g. vegetation cover). In this theoretical work, we explore, with large mammals of African savannas in mind, how the interplay between climate-induced changes in prey abundance and climate-induced changes in prey vulnerability affects the immediate and long-term responses of predator populations. We account for prey body condition and habitat effects on prey vulnerability to predation. We show that predictions on how predator abundance responds to climate fluctuations differ depending on how climate influences prey vulnerability (habitat characteristics vs. prey body condition). We discuss how species traits influence the relative importance of the different sources of vulnerability. For example, our results suggest that populations of cursorial predators (such as spotted hyaenas) are expected to fare better than populations of ambush predators (such as African lions) in African ecosystems that will be characterised by an aridification. This study highlights the importance of understanding, and accounting for, the vulnerability factors associated to a given predator–prey pair, and improves our comprehension of predator–prey relationships in a changing climate.
Introduction
The Earth’s climate is changing rapidly, largely because of human activities (IPCC, 2014b). Temperature is rising globally and will continue to do so, precipitation regimes are, or will be, locally altered, and extreme climatic events will become more common (IPCC, 2014b). Climate changes already have ecological impacts and are, or will soon be, a major driver of species dynamics and survival (Maclean and Wilson, 2011; Pacifici et al., 2017). So far, most studies on the impacts of climate changes have focussed on describing changes in population distribution and abundance (e.g. Parmesan et al., 1999; Martay et al., 2017), phenology and demography (e.g. Inouye et al., 2000; Moyes et al., 2011) or behaviour (Candolin and Wong, 2012). Even though these changes can sometimes emerge from the direct effect of climate on individuals, they often result from cascading effects occurring through biotic interactions, such as trophic interactions (e.g. Visser et al., 2006). Therefore, understanding how climate changes will affect species through their biotic interactions is fundamental to grasp the full picture of the impacts of climate changes.
Climate changes have the potential to modify predator–prey interactions, which are central in the functioning of populations, communities and ultimately ecosystems (Bastille-Rousseau et al., 2018). First, by altering primary production quality and quantity, climatic conditions influence the body condition of large mammalian herbivores (rainfall: Bourgarel et al., 2002; Owen-Smith, 2002; NAO: Mysterud et al., 2001; snow: Saether and Gravem, 1988). Herbivore body condition, in turn, influences demographic parameters, such as survival (Bender et al., 2007) or fecundity (Cook et al., 2004), which ultimately affect herbivore population abundance. As a result, climate indirectly influences prey abundance through density-dependent processes (Saether, 1997; Forchhammer et al., 1998). For example, several studies linked rainfall to herbivore abundance in savanna ecosystems (East, 1984; Ogutu et al., 2008), or showed the influence of climatic variations on the dynamics of several populations of Northern ungulates (Forchhammer et al., 1998; Post and Stenseth, 1999). Extreme climatic conditions also affect herbivore demographic parameters and ultimately population abundances (e.g. drought: Mduma et al., 1999; Foley et al., 2008). These changes in herbivore abundance originating from climate fluctuations will affect predator populations, as the maintenance of predator populations largely depends on prey abundance (East, 1984; Carbone and Gittleman, 2002). Prey body condition is thus a first pathway through which climate changes may alter predator–prey interactions and such climate-driven impact on prey demography has already been taken into account in pioneering predator–prey modelling works (e.g. Wilmers et al., 2007b; blue arrows in Figure 1).
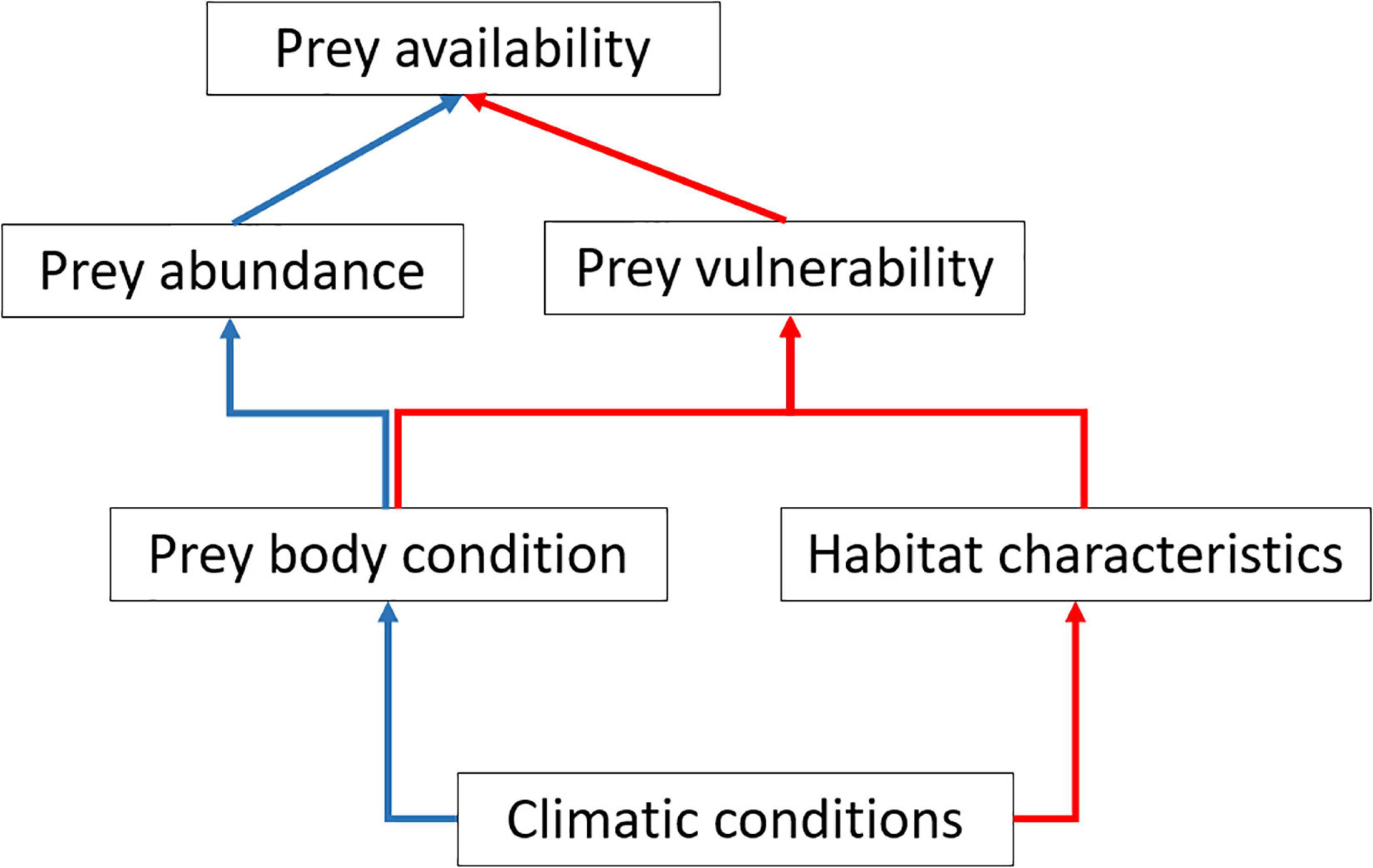
Figure 1. The potential pathways by which climatic conditions can affect prey availability for predators. The blue arrows represent the links classically modelled in existing models (scenario 1 in this study). The red arrows represent the links originally modelled in this work (through scenarios 2–4).
Predator–prey relationships are also influenced by prey vulnerability, which may arise from intrinsic (inherent to the prey) or extrinsic (arising from the environment) sources. Prey body condition is typically an intrinsic factor that can determine prey vulnerability to predators. Indeed, several studies pointed out that predators tend to select the weakest individuals among prey and that prey in poorer body condition are more likely to be preyed upon (in savanna ecosystems: FitzGibbon and Fanshawe, 1989; Pole et al., 2004; in temperate ecosystems: Husseman et al., 2003; Atwood et al., 2007). On the other hand, habitat characteristics are extrinsic factors that can modulate prey vulnerability to their predators and that can be influenced by climatic conditions. Several studies stressed out the importance of vegetation structure and cover, which interact with the predator hunting technique and the prey escape technique to ultimately affect the hunting outcome. This was shown in savanna (Funston et al., 2001; Hopcraft et al., 2005) and temperate ecosystems (Husseman et al., 2003; Lone et al., 2014). Other habitat characteristics can interfere with the predator hunting success. For example, prey were either more vulnerable to predators with increasing amount of snow (Mech et al., 1971; Post et al., 1999) or less vulnerable to predators as snow hardness decreased (Stenseth et al., 2004).
Hence, the true prey availability to predators results from the interplay between prey abundance and prey vulnerability, which is characterised by different sources of vulnerability (red arrows in Figure 1). Little is known on the relative importance of climate-induced changes on the different components of prey availability to predators, and particularly on the different sources of prey vulnerability. Here, we integrate both prey abundance and vulnerability effects (Figure 1) in a theoretical study investigating the immediate response of predator populations to a year of a specific climatic condition, as well as the long-term response of predator populations to changes in average climatic conditions. This study aims at providing general insights on the interplay between climate-induced changes in prey abundance and prey vulnerability. The model is, on purpose, not designed for a specific predator–prey system, so that people studying predator–prey interactions worldwide can adapt the model to fit the system there are interested in. However, we designed our study with large mammalian herbivores and carnivores of African savannas in mind so that the parameters and the functional relationships used are easy to relate to a real world. Annual rainfall is expected to decrease in southern Africa and increase in eastern Africa and generally to become more variable (IPCC, 2014a). We therefore specifically focussed on the influence of rainfall conditions on predator–prey interactions in African savanna ecosystems. We modelled the population dynamics of a predator preying on a density-dependent age-structured prey population following the model described in Wilmers et al. (2007a, b), but we further and originally accounted for prey body condition and environmental effects on prey vulnerability to predation (Figure 1).
Materials and Methods
The Predator–Prey Model
The Prey Population Model Without Predation
We considered a female-only prey population (Caswell, 2001) which, in any year t, had a total population size of N(t) made of J(t) juveniles and A(t) adults. Fluctuations from year to year were driven by survival and reproduction of individuals, which are now described.
Body condition is a critical individual characteristic, as it links environmental conditions to demography. For simplicity, we assumed a linear relationship between body condition and survival, and thus only modelled the latter. We modelled the effect of body condition on reproduction using a relationship between the probability to reproduce and the probability of survival. Each year, individual survival probabilities pji(t) and pai(t), for juveniles and adults, respectively, were drawn from Beta distributions with shape parameters αj and βj and αa and βa for juvenile and adult prey, respectively, and differed from one individual to another, allowing to integrate individual heterogeneity. However, new probabilities were drawn each year and were not preserved through the individual’s life, and thus varied randomly within individuals from year to year. The method-of-moments gives:
for juvenile prey, and
for adult prey, where pj and pa are the mean survival rates and Sdpj and Sdpa are the standard deviations for juvenile and adult prey population, respectively. In any year t, mean survival rates pj and pa were:
where Pj and Pa are parameters defining the maximum survival rates for juveniles and adults, respectively (see Table 1 for all default values of model parameters), and gi and ga are scaling functions allowing to account for density- and resource-dependence in survival. These scaling functions (bounded between 0 and 1) are those used by Wilmers et al. (2007a) and follow:
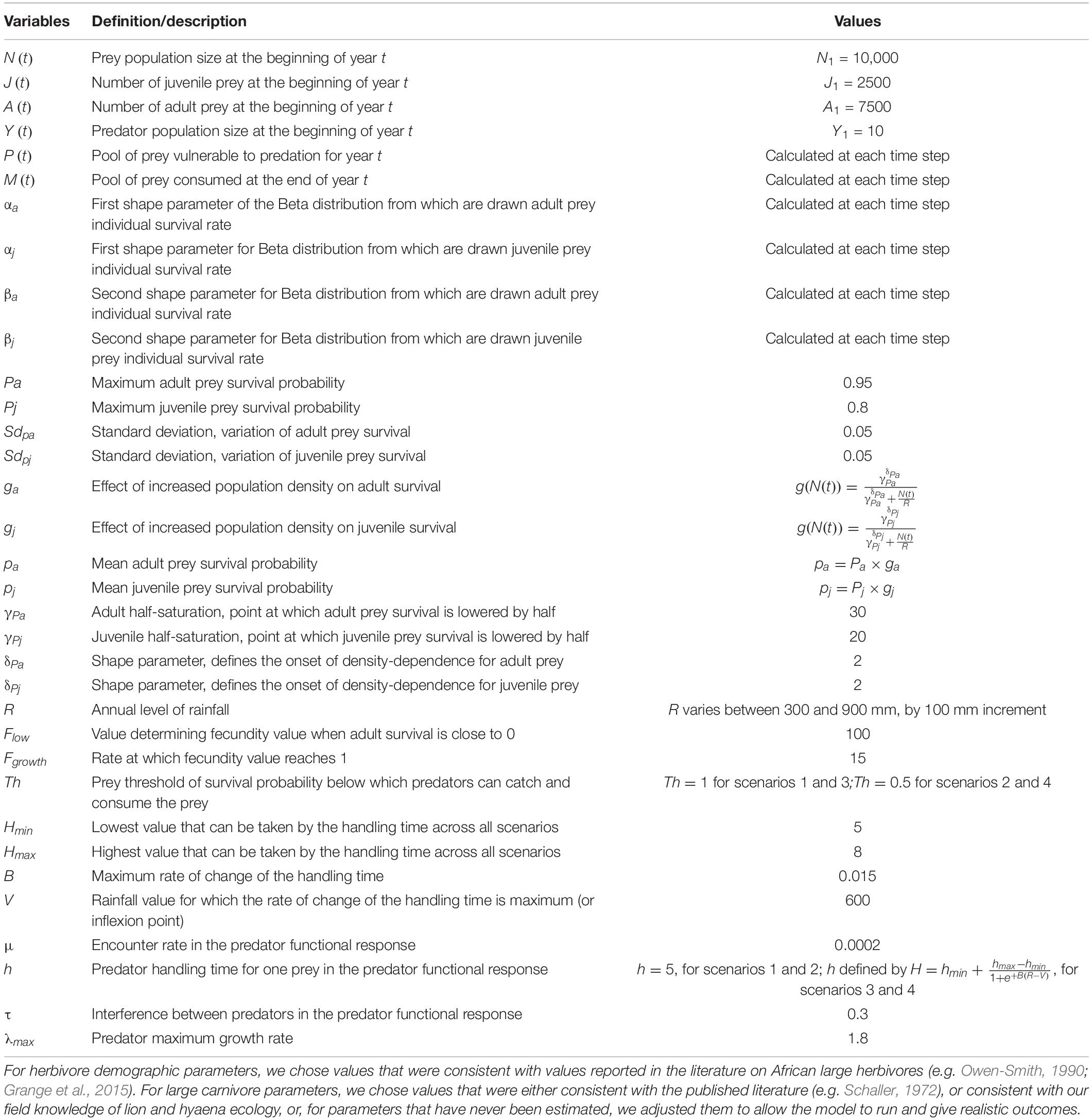
Table 1. Definitions and values of the state variables and parameters for prey and predators used in the model.
where R is the annual rainfall of the site. R varied from 300 to 900 mm by 100 mm increment, which are coherent values for annual rainfall in African savannas (Sankaran et al., 2005; Chamaillé-Jammes and Fritz, 2009). We assumed that rainfall determined directly and linearly prey resource availability (Rutherford, 1980). We thus considered rainfall as a proxy of the resource availability and the ratio N(t)/R as a number of individuals sharing the amount of available resource for year t. The half-saturation parameter γ determined the per capita resource availability at which the maximum survival rate was reduced by half. Finally, δ was a shape parameter setting the beginning and the strength of the density-dependence (as in Wilmers et al., 2007a).
Juvenile survival is expected to be the first demographic parameter affected by increasing density, followed by age at first reproduction, reproductive success and finally adult survival (Eberhardt, 1977). This hypothesis has found support in large ungulate studies (Bonenfant et al., 2009). As our model was conceptualised for large mammals (as for the one of Wilmers et al., 2007a), we assumed a greater sensitivity of juvenile survival to increasing density by assuming that γj was lower than γa.
In summary, both rainfall R and population size N determined g and ultimately the average survival rates pj and pa in the population (Figure 2A). Once mean survival probability had been computed, we determined the fate of each individual by randomly drawing a value from a uniform distribution on the [0–1] interval and comparing it to the individual’s survival probability. If the value was lower than the survival probability, the individual survived; if not, it died.
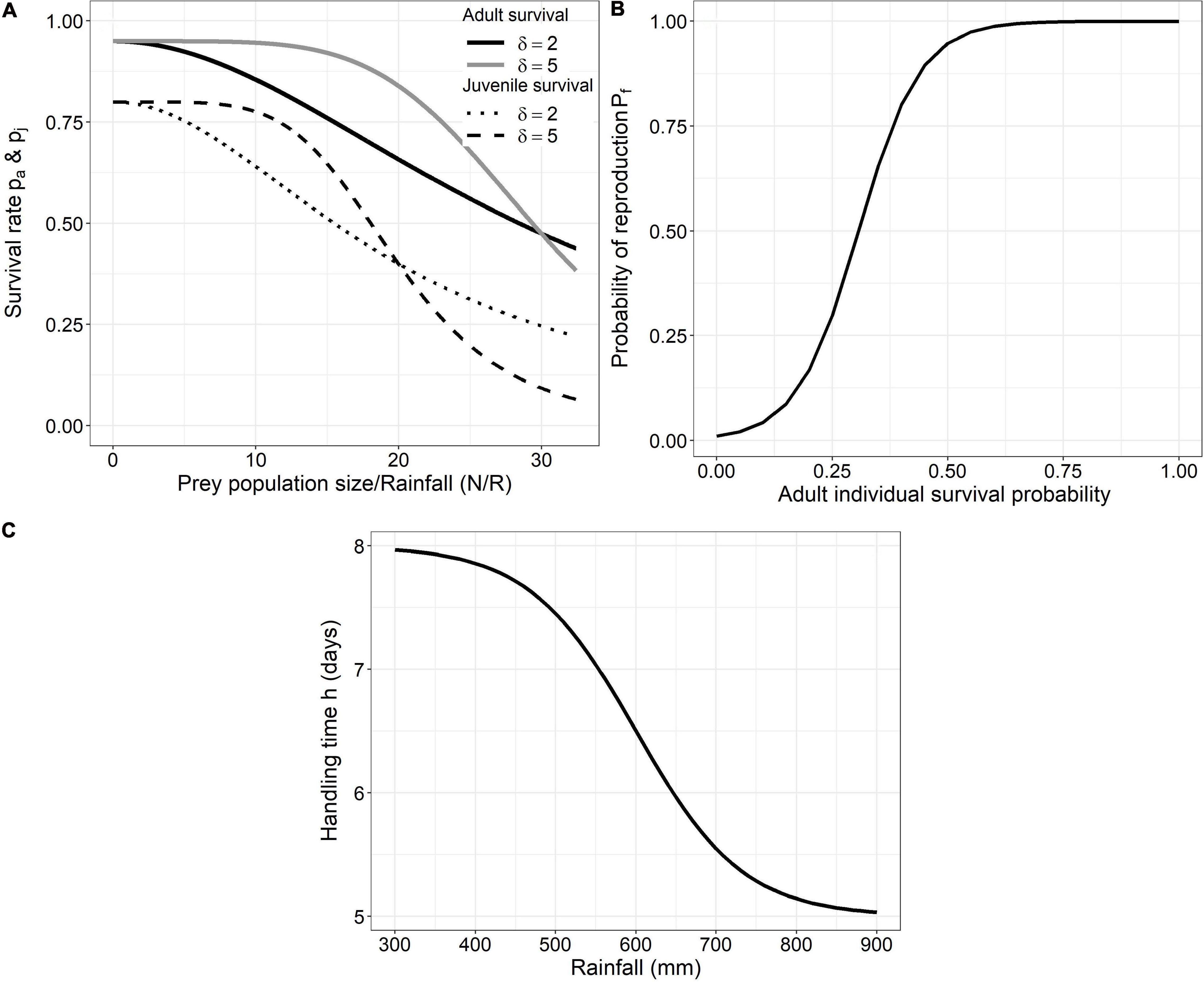
Figure 2. Summary of the functional relationships underlying the model. (A) Effect of increased number of individuals per resource unit (approximated by rainfall) on mean juvenile and adult prey survival rates adapted from Wilmers et al. (2007a). Mean adult and juvenile prey survival rates has been represented for two values of δ parameter, which controls the onset and the strength of the density dependence. Juvenile prey survival rate has been set to be the first impacted by increasing density. (B) Probability of reproduction as a function of survival probability pai(t). (C) Functional relationship between rainfall and predator handling time h as defined by Eq. 5 for parameters given in Table 1. The hunting success varies inversely with the handling time.
We assumed that juveniles became adults in 1 year (a realistic assumption for some herbivore species; Estes, 1991) and only adults were able to reproduce. Therefore, the number of juveniles at the beginning of a given year depended only on the fecundity of adults the year before. We also considered that, for each adult, the probability of reproducing (Pf) was a function of its survival probability pai(t), and the link between Pf and pai(t) was as follows:
where flow is a parameter determining the probability of reproduction Pf of individuals whose survival probability is close to 0 and fgrowth a parameter defining the speed at which Pf reaches the value of 1 with increasing pai (Figure 2B). We compared, for each individual, values drawn randomly from a uniform distribution on the [0–1] interval with their reproduction probability. If the value was lower than the reproduction probability, the individual produced one offspring; if not, it did not reproduce.
Integrating Predation Into the Prey Model
We modelled an unstructured predator population (similarly to Wilmers et al., 2007b), which, in any year t, had a total population size of Y(t). Predator population dynamics resulted from the combination of a functional response, which describes how the intake rate of a predator varies with prey abundance (Holling, 1959; Abrams and Ginzburg, 2000), and a numerical response, which relates predator intake to changes in its abundance (Bayliss and Choquenot, 2002).
We integrated the influence of prey body condition by accounting for the fact that only adult prey in poor condition, i.e. with a low survival probability, were vulnerable to predators, whereas all juvenile prey were vulnerable to predators, irrespectively of their body condition. To do so, we defined a threshold of survival probability Th. Any adult prey with an individual survival probability pai(t) below this threshold was integrated to the pool of adult prey vulnerable to predators Avuln. Rainfall and prey density both affected ga(t) and thus pa(t), the mean survival probability within the population. Consequently, they played a crucial role in determining the proportion of adult prey in poor body condition. As pa(t) decreased, there were more adult prey with low individual survival probabilities, and therefore more adult prey with a survival probability under the vulnerability threshold and exposed to predation.
We integrated the influence of habitat characteristics that could affect prey vulnerability through the manipulation of the handling time h, which is classically defined as the time needed by a predator to subdue and consume a prey and during which the predator cannot capture another prey (Abrams and Ginzburg, 2000). In our model, this time to subdue one prey corresponded to all predation attempts (with one predation attempt being a predation sequence: search, encounter and attack) leading to a successful prey capture. For African savannas, we assumed that an increase in annual rainfall leads to a greater vegetation cover (taller grass, more leaves in bushes and trees) and hence better concealment opportunities for predators to approach their prey undetected (Hopcraft et al., 2005). Hence, we considered that an increase in the climatic variable R would increase prey vulnerability and, consequently, decrease the number of attempts leading to a successful attack, i.e. lead to lower handling time h values (an alternate situation where handling time increases with rainfall is presented in Appendix A of the Supplementary Material). Therefore, h varied with rainfall according to the following equation:
with H(R) the value of handling time for a given year t and the rainfall value R, hmin is the lowest value of h, hmax is the maximum value of h, B is the maximum rate of change and V is the rainfall value at which the rate of change of h is maximum (or inflexion point). This equation allowed the handling time to vary non-linearly and negatively with rainfall (Figure 2C). One value of h was calculated for each rainfall value and was then incorporated into the functional response I(t).
For the functional response, we assumed that the number of prey killed and consumed by one predator during a given year t followed a type II Beddington–DeAngelis functional response. This equation allows the integration of interference between predators when searching for and capturing prey and is formulated as follows:
where P(t) is the number of vulnerable prey, Y(t) the number of predators, μ a parameter defining the encounter rate between predators and their prey, h the parameter defining the handling time and τ the interference between predators (Abrams and Ginzburg, 2000). Finally, this type of functional response allows both prey and predator densities to impact predators’ intake and therefore the regulation of predator populations.
Predation occurs all year around. Consequently, to account for the depletion of prey that occurred throughout the year and could affect the estimation of the number of prey actually captured, we recursively applied the functional response presented in Eq. 6 over daily time steps, adjusting prey number at each time step. We named M(t) the total number of prey killed by predators and calculated it as follows:
where P(t) is the number of vulnerable prey to predation, P(d) the pool of prey vulnerable to predation at the beginning of day d and Y(t) the number of predators at the beginning of year t.
The numbers of juvenile and adult prey killed by a given predator during a year are given by:
The Mj(t) juveniles killed in year t were randomly removed from the J(t) juveniles present at the beginning of the year t. Similarly, the Ma(t) adults killed in year t were randomly removed from the pool of vulnerable adults Avuln, i.e. individuals whose survival probabilities were below the threshold of survival probability Th. For simplification, we assumed that adult and juvenile prey were equally profitable to predators. Similar assumption can be found in other modelling studies (e.g. Wilmers et al., 2007b).
Finally, the predator numerical response was given by:
where Y(t) is the number of predators present in year t, λmax is the maximum growth rate of the predator population and Prel(t) was calculated as follows:
with hmin the smallest value possible for the handling time. Prel was therefore bounded between 0 and 1 and quantified predators hunting efficiency over a year by expressing the amount of prey actually eaten in year t as a proportion of the maximum amount of prey that predators could have eaten.
Simulations
To study how climatic conditions may impact predator populations through their influence on prey body condition or habitat characteristics, we designed four scenarios:
Scenario 1: Climatic conditions influence prey availability through their effect on prey body condition and ultimately on prey abundance. Prey vulnerability to predators did not vary with prey body condition (all prey were vulnerable to predators) nor with habitat characteristics. Climatic conditions therefore influenced predator–prey interactions only through their impacts on prey survival and fecundity, i.e. through prey abundance only (blue arrows in Figure 1). This demographic effect of climate is present in all scenarios.
Scenario 2: Prey vulnerability was affected by climatic conditions through their influence on prey body condition (red arrows in Figure 1). The poorer a prey body condition, the more vulnerable to predators the prey was. Therefore, climatic conditions influence prey availability through their effect on prey body condition affecting both abundance and prey vulnerability to predation.
Scenario 3: Prey vulnerability was affected by climatic conditions through climate-driven changes in habitat characteristics (e.g. vegetation) (red arrows in Figure 1). Therefore, climatic conditions influence prey availability through their effect on prey body condition affecting prey abundance and on habitat characteristics affecting prey vulnerability to predation.
Scenario 4: Prey vulnerability was affected by both prey body condition and climate-driven changes in habitat characteristics. In this scenario combining scenarios 2 and 3, climatic conditions influence prey availability through their effect on prey body condition and habitat characteristic and, ultimately, prey abundance and vulnerability to predation.
While in scenarios 1 and 3, predators could capture any prey individual, in scenarios 2 and 4, predators could capture any juvenile, but could only subdue adult prey that were in poor body condition. These differences between scenarios were modelled by setting the value of the threshold of survival probability Th at 1 in scenarios 1 and 3, and at 0.5 in scenarios 2 and 4 (Table 1). As vulnerability to predators was independent from habitat characteristics in scenarios 1 and 2, the handling time was set constant and equal to h = 5 in these scenarios. In scenarios 3 and 4, prey vulnerability to predators was dependent on habitat characteristics and handling time h varied with climatic conditions following Eq. 5 (for a summary of the model functioning, see Figure 3).
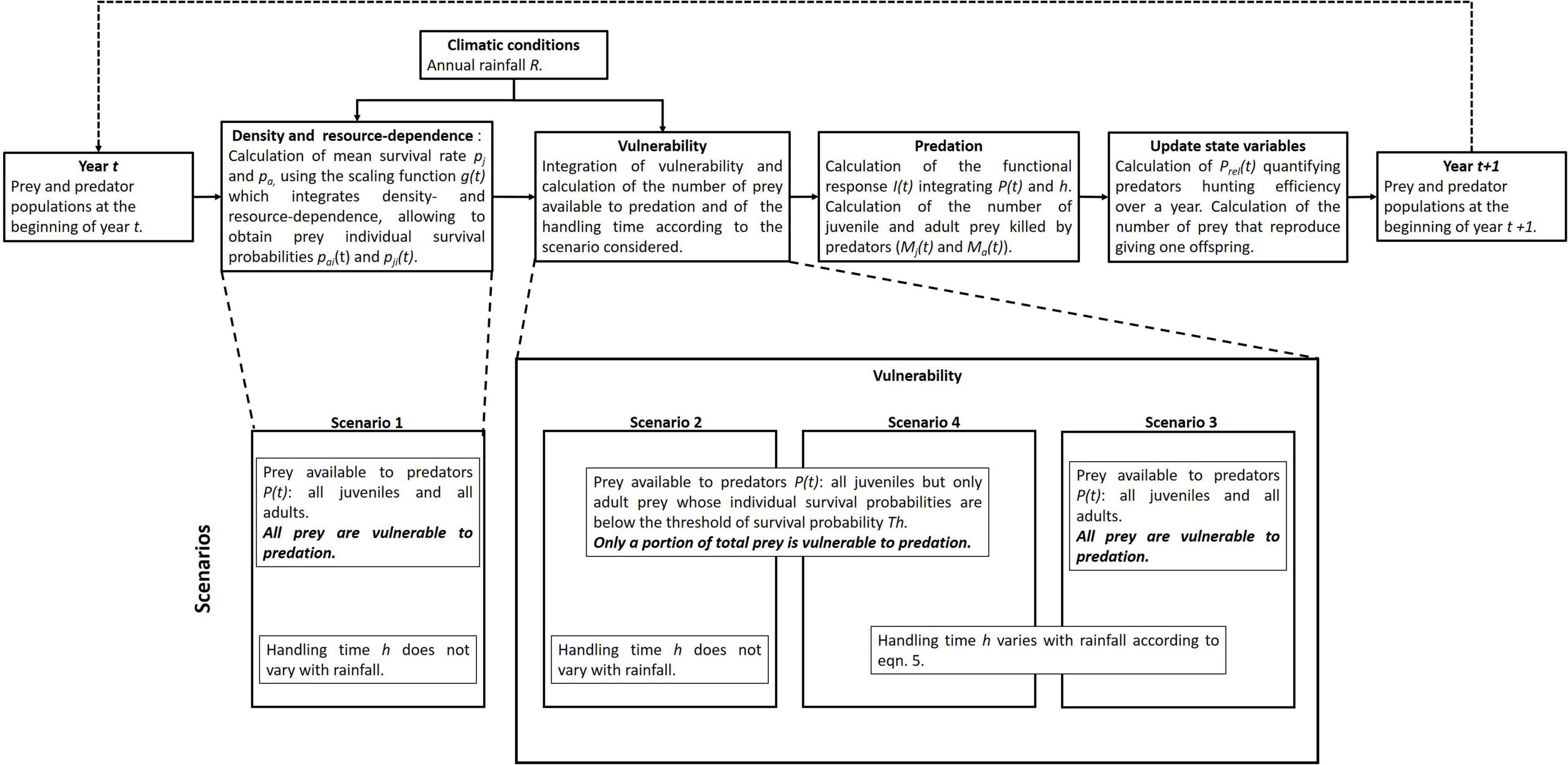
Figure 3. Model summary with the key model steps. The dashed line arrow symbolises the next time step. As scenario 1 is independent from vulnerability sources, it is presented aside from the other scenarios. Conversely, details of the processes defining vulnerability related to either body condition or habitat characteristics are presented in scenarios 2–4.
We focussed on these four scenarios only, as the goal of this study was to assess how the addition of prey vulnerability to traditional predator–prey models that considered prey abundance only would change the expectations in terms of responses of predator populations to climatic changes.
For simulations, parameters were given default values considered realistic for African large mammals. These default values and initial values for state variables are presented in Table 1. The sensitivity of the main results to some of these parameter values was investigated by sensitivity analyses (see section below). As we were mostly interested in how the link between climatic conditions and prey vulnerability could affect predator dynamics, predators were characterised by identical parameter values in the different scenarios, but for the threshold Th and handling time h values. Therefore, in our work, all differences in predator dynamics between scenarios emerged from differences in these two parameters.
Immediate Response of Predator Populations to Specific Rainfall Conditions
We assessed, for each scenario, the immediate response of the predator population to specific rainfall conditions. We simulated over 198 years the dynamics of the predator population under the long-term annual rainfall 600 mm, then imposed a predefined rainfall in year 199 (different values were tested: from 300 to 900 mm, by 100 mm increments) and calculated the predator population growth rate over that year (calculated as λ = Yt + 1/Yt). To obtain robust estimates of predator population growth rates and account for within-simulation variability in prey demographic rates, each simulation, characterised by a long-term annual rainfall value of 600 mm and one predefined rainfall for the last year, was replicated 1000 times.
Long-Term Response of Predator Populations to Changes in Mean Annual Rainfall
We assessed, for each scenario, how a change in mean annual rainfall may affect predator populations on the long run. We compared the average predator population size (over the last 21 years of a 200-year simulation) across simulations run under different long-term rainfall averages. The mean annual rainfall values varied from 300 to 900 mm, by 100 mm increments. For each value, simulations were replicated 1000 times, allowing again to account for within simulation variability in prey demographic rates.
Sensitivity Analyses
Because model outcomes can be sensitive to the choice of parameter values, we conducted sensitivity analyses for some model parameters. We first investigated the effect of changes in parameter hmin and Th, which are of importance because differences between scenarios originate from these two parameters. In addition, we also tested for the influence of changes in λmax values, which is a central parameter of the predator numerical response. Finally, we carried out a sensitivity analysis for the δ parameter, which describes the shape of density dependence and determines the magnitude of the prey population response, the number of individuals per resource units and ultimately prey dynamics. We always varied this parameter simultaneously for adult and juvenile, ensuring that juvenile survival never exceeded adult survival (see Figure 2A). Sensitivity analyses were conducted by increasing or decreasing each of these parameters, one at a time, by 20% around its default value. For each parameter value, simulations were replicated 500 times. Results of the sensitivity analyses are presented in Appendix B of the Supplementary Material.
An example of the behaviour of the model for one run showing fluctuations of rainfall, prey and predator population size over 200 years is presented in Supplementary Figure S7 (Appendix C of the Supplementary Material), and a summary of the key steps of the modelling process and of the different scenarios can be found in Figure 3. All simulations were conducted in R 4.0.2 (R Core Team, 2020).
Results
Immediate Response of Predator Populations to Specific Rainfall Conditions
In scenario 1, i.e. when climatic conditions influenced prey abundance only (through survival and fecundity) and not prey vulnerability, the population growth rate of predators was not influenced by the specific climatic condition of the year (Figure 4A). This result was not surprising as according to the model design, the demographic consequences of climate are expected to be visible in prey population the following year (t + 1) and, therefore, would only impact predator growth rate calculated over the year t + 1 and t + 2. In contrast, in the three other scenarios, i.e. when climatic conditions influenced prey vulnerability, either through changes in prey body condition (scenario 2) or through changes in habitat characteristics (scenario 3) or both (scenario 4), the population growth rate of predators 1 year was influenced by the specific climatic condition of the year. Population growth rates in scenarios 2 and 3 showed opposite patterns (Figure 4A). Predators from scenario 2 benefited from below-average rainfall (600 mm) and their populations increased, as shown by the growth rate being greater than 1, but there was a negative effect of above-average rainfall (Figure 4A). In scenario 3, there was a negative effect of below-average rainfall and a positive effect of above-average rainfall on predator growth rate (Figure 4A). Because scenario 4 is a combination of scenarios 2 and 3, the population growth rate of predators from scenario 4 was intermediate between those of scenarios 2 and 3 (Figure 4A).
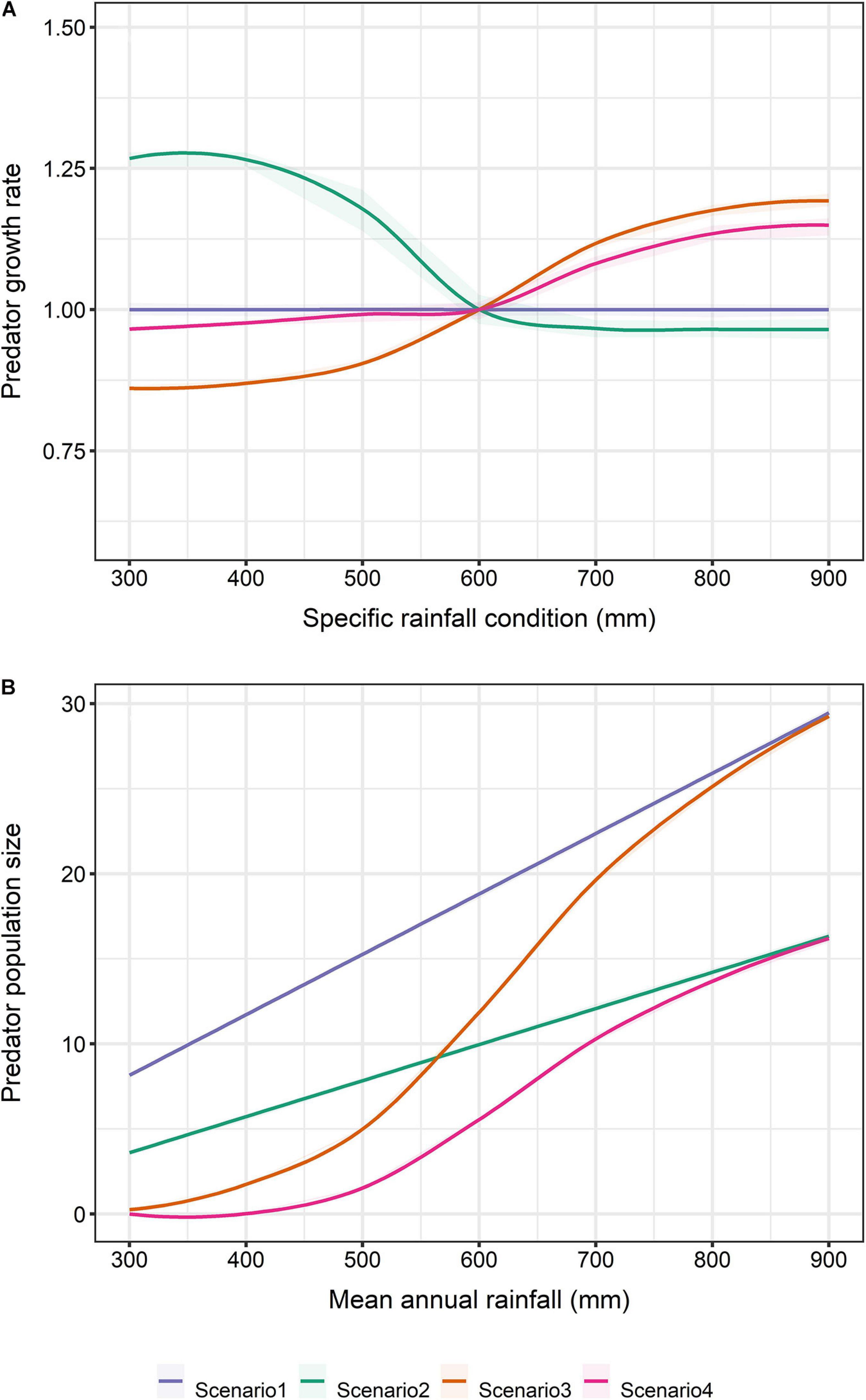
Figure 4. (A) Immediate response of predator populations to a specific rainfall condition. Relationship between the growth rate of predator populations and a specific annual rainfall in a system characterised by a long-term mean annual rainfall of 600 mm. (B) Long-term response of predator populations to changes in mean annual rainfall. Relationship between the size of predator populations and long-term mean annual rainfall. While in scenario 1, prey vulnerability to predators did not vary with climatic conditions, in scenarios 2–4, climatic conditions affected prey vulnerability through, respectively, changes in prey body condition, in habitat characteristics or both. Light-coloured envelops include minimum and maximum values of predator growth rates (A) or mean predator population sizes (B).
Long-Term Response of Predator Populations to Changes in Mean Annual Rainfall
Our results showed an effect of mean annual rainfall on the size of predator populations for all scenarios, but the shape of the relationships varied. In scenario 1 (when climatic conditions influenced prey abundance only), the size of predator populations increased near-linearly with rainfall (Figure 4B). As expected because all individuals were vulnerable to predation, the size of predator populations in this scenario was always greater than the one observed in other scenarios. The size of predator populations in scenario 2 (when climatic conditions influenced prey vulnerability through changes in prey body condition only) also increased near-linearly with mean annual rainfall. It was consistently lower than the size of the predator population in scenario 1 because only a fraction of the prey population is vulnerable to predation in scenario 2. However, the effect of climatic conditions on predator population sizes was weaker in scenario 2 than in scenario 1: as the amount of rainfall increased, the difference between scenarios 1 and 2 in the size of predator populations increased (Figure 4B). Conversely, the size of predator populations varied non-linearly with increasing rainfall in scenario 3, when climatic conditions influenced prey vulnerability through changes in habitat characteristics only, and in scenario 4, when climatic conditions influenced prey vulnerability through both changes in prey body condition and habitat characteristics. More specifically, the size of predator populations in scenario 3 was smaller than those from scenario 2 up until mean annual rainfall reached values around 600 mm, but was greater for values above (Figure 4B). In addition, for a mean annual rainfall of 900 mm, the sizes of predator populations in scenarios 1 and 3 were almost equal. In scenario 4, changes in the size of predator populations with increasing mean annual rainfall generally followed a similar pattern than those observed from scenario 3. However, the difference in the size of predator populations between scenarios 4 and 3 increased with increasing rainfall (Figure 4B). Conversely, the size of predator populations was virtually identical between scenarios 4 and 2 at the wettest end of the rainfall gradient (Figure 4B). In all cases, the size of predator populations in scenario 4 was always equal or lower than in the other scenarios (Figure 4B).
Discussion
Our work shows how predator populations could respond differently to changing climatic conditions depending on how climate influences prey vulnerability (habitat characteristics vs. body condition). For example, in a context of aridification, a predator–prey system where prey vulnerability depends mainly on prey body condition (scenario 2) would be characterised by larger predator populations than a system where prey vulnerability is mainly affected by the habitat (scenario 3) (see section “How Species Traits Influence the Relative Importance of the Different Sources of Vulnerability” for the role of species traits). It thus highlights the importance of having a good understanding of the processes determining vulnerability at play within the predator–prey pair studied, as integrating climate influence on prey vulnerability, in addition to resource-mediated climate effects on prey abundance, leads to different responses of predator populations (on the short- and the long-term) to changes in climatic conditions.
How Species Traits Influence the Relative Importance of the Different Sources of Vulnerability
Ultimately, whether the predator–prey pair studied will respond according to one scenario or another will depend upon the traits of the species involved. Here, we will illustrate the importance of the predator hunting mode and the prey escape tactics, as well as the importance of the relative size of the predator and the prey. Predator hunting mode (cursorial or ambush) and prey escape tactics (fleeing, fighting or freezing) are key behavioural traits involved in predator–prey interactions that can affect how climatic conditions contribute, indirectly via forage resources or vegetation cover, to determine the vulnerability of prey to predation. For example, cursorial predators, such as spotted hyaenas Crocuta crocuta (Cooper, 1990) and wild dogs Lycaon pictus (Pole et al., 2004) in African savannas, or wolves in temperate ecosystems (Mech et al., 2015), are active predators, i.e. they can chase down their prey over long distances and are more likely to kill individuals in poor body condition. They therefore exemplify predators from scenario 2 (climatic conditions influence prey vulnerability through changes in prey body condition only), as prey in poorer condition because of a shortage of food will be particularly more vulnerable to predation from these predators (Mills, 1995; Pole et al., 2004; Atwood et al., 2007). Conversely, ambush predators, such as African lions Panthera leo and leopards Panthera pardus in African savannas, or cougars Puma concolor and lynx Lynx lynx in temperate ecosystems, stalk their prey before attacking them by surprise when they come within short distance (Elliott et al., 1977; Husseman et al., 2003). As such, they rely less on prey body condition. However, to approach their prey undetected, ambush predators need concealment. Therefore, their hunting success is prone to be influenced by vegetation (Funston et al., 2001; Lone et al., 2014), and negatively affected by an increased aridity and a subsequent decrease in vegetation density (scenario 3, climatic conditions influence prey vulnerability through changes in habitat characteristics only). Whereas prey escape tactics might not be that crucial for the outcome of an encounter with an ambush predator, a climate-driven decrease in prey body condition will negatively influence prey fleeing capacity leading to an overall disadvantage against cursorial predators (scenario 2).
Predator body size influences the prey body size targetted by the predator (Radloff and Du Toit, 2004; Owen-Smith and Mills, 2008a) and climate-driven changes in prey body condition can interfere with the chance of successfully subduing an adult prey depending on the relative size of the predator and the prey considered (Mech et al., 2001; Metz et al., 2012). For example, for lion–impala interactions, it is very likely that climatic conditions will not affect the likelihood of lions to capture an adult impala Aepyceros melampus, while in the case of lion–buffalo Syncerus caffer interactions, lions are expected to successfully hunt adult buffaloes, which are dangerous prey for them, in dry conditions only (scenario 2, Owen-Smith, 2008). This is also true at the interspecific level: prey species that are usually inaccessible because they are too difficult to be captured under normal climatic conditions may become vulnerable due to unfavourable conditions, allowing predators to kill young individuals of larger species (e.g. with elephants: Salnicki et al., 2001; Loveridge et al., 2006).
Importance of Integrating Climate-Driven Changes in Prey Vulnerability
Our results show how predator population responses to changes in climatic conditions differ when we take into account climate-driven changes in prey vulnerability in addition to climate-driven changes in prey abundance (existing work takes into account climate-driven changes in prey abundance only – Figure 1). For example, while the overall trend depicted in all four scenarios on the long term is that there are more predators when rainfall increases, because of an overall increase in prey population abundance, the originality of our study is to illustrate how prey vulnerability and its drivers influence the link between predator populations and climate.
When changes in climatic conditions influence prey vulnerability through changes in prey body condition (scenario 2), a below average rainfall event leads to poorer herbivore body condition and hence results in an increase in the pool of adult prey vulnerable to predators (Supplementary Figure S8A). Unsurprisingly, this, in turn, favours the immediate growth rate of the predator population. This result is in accordance with field observations, which reported a positive effect of drought conditions over the short term on large carnivore populations (Mills, 1995). These dry-condition increases in prey vulnerability can be assimilated to “resource pulses” for the predators and to be beneficial to them (Holt, 2008; Yang et al., 2008). Contrarily, above average rainfall events negatively affect the population growth rate of predators, as very few prey adults are vulnerable in years characterised by high levels of resources (Supplementary Figure S8A). Over the long term, predator populations benefit from the overall increase in prey populations associated with increasing rainfall (Supplementary Figure S9). However, predators in scenario 2 can only subdue a portion of adult prey and have a smaller pool of available prey than predators in scenario 1. They are therefore maintained at smaller population size. In addition, the proportion of vulnerable adult prey decreases with increasing rainfall (Supplementary Figure S8B), which leads to the observed increase in the difference between the size of predator populations in scenarios 1 and 2.
When changes in climatic conditions influence prey vulnerability through changes in habitat characteristics and habitat-driven hunting success increases with rainfall (scenario 3), predator growth rates are negatively impacted by below average rainfall and positively influenced by above average rainfall. This is typically the case in African savannas where grass height is related to rainfall (Rutherford, 1980) and lions are hunters that are more successful in tall grass (Funston et al., 2001). Over the long term, predator populations benefit from the combined linear increase of prey abundance (Supplementary Figure S9) and non-linear increase of hunting success (i.e. decrease of handling time, Figure 2C), leading to an overall non-linear increase as rainfall increases.
Finally, when changes in climatic conditions influence prey vulnerability through changes in both prey body condition and habitat characteristics (scenario 4), the growth rate of predator populations combines the effects of both sources of vulnerability and predators are affected by opposite effects of different sources of vulnerability. For below average rainfall, predators benefit from the positive effect of lower prey body conditions, which buffers the negative effect of a lower hunting success due to habitat characteristics, and inversely for above rainfall level, leading to the observed intermediate pattern of predator growth rate (Figures 2C, 4A and Supplementary Figure S8A). Over the long term, predator populations of scenario 4 follow the same patterns as the populations in scenario 3 with the exception that they can only subdue a proportion of adult prey. They subsequently remain at a smaller population size.
Contrasting Predator Responses to Short- and Long-Term Climate Fluctuations
Our study highlights the importance of the temporal scale considered as the patterns observed differed whether we considered the short- or long-term temporal scale. While a sudden arid event can be beneficial to predator population, increasing aridity over time leads to an overall decrease in predator population size. The explanation of such differences lies in the fact that a predator response over the long term integrates the predator responses over the short term, along with the long-term response of prey, which declines with increasing aridity (Supplementary Figure S9). Therefore, our results illustrate that the long-term response of predator populations to changes in mean annual rainfall cannot be predicted only from the immediate response of predator populations to specific rainfall conditions.
Considerations on the Model
Modelling studies are difficult exercises where one walk on a thin line between complexity/realism and simplification that is required for conceptualisation, i.e. building some theoretical understanding. We have tried to do this successfully but we want to underline here a few simplifications made in this study (and already made in classical models of predator–prey interactions – e.g. Wilmers et al., 2007a,b). It is noteworthy that the code of the model is available1 so that one can parameterise it for a specific system and add the complexity that would be considered critical. First, in our model, juvenile prey contribute as much energy as adult prey. Second, the predator population is not age-structured. Third, the predator–prey system modelled here involves one prey and one predator only, yet multi-species assemblages often characterise both prey and predator communities. Predators often prey on several prey species, with primary and secondary prey, and display prey switching depending on prey availability and catchability (Owen-Smith and Mills, 2008b; Elbroch et al., 2013). The presence of an alternative prey could influence model outcomes for scenarios 2–4: when the vulnerability of the primary prey decreases, if the predator can switch to an alternate prey species available and more vulnerable, the decrease in predator population growth rate or over the long term may be weaker or not observed. In contrast, the presence of competitors preying on the same prey could intensify the decrease in the number of vulnerable prey available to each predator owing to additive effects (Melis et al., 2009) and negatively influence predator population size.
Conclusion
Despite its general simplicity, this theoretical study highlights the importance of understanding, and accounting for, the vulnerability factors in predator–prey relationships, and sheds light on the complexity of predicting predator–prey relationships in a changing climate. We believe it is a prospective study that suggests interesting future research directions. Based on our work, we advise that future theoretical and empirical works should integrate climate-driven changes in prey vulnerability if we want to grasp the full picture of the impacts of climate changes on predator–prey interactions.
Data Availability Statement
The code used for the model and the simulations is available from a GitHub repository: https://github.com/aissamorin/climate-prey-vulnerability-and-predators.
Author Contributions
MV and SC-J conceived the ideas. AM and SC-J designed the model. All authors analysed the results, wrote the manuscript, and gave approval for publication. All authors contributed to the article and approved the submitted version.
Funding
This work was partly funded by the Agence Nationale de la Recherche (project Landthirst ANR-16-CE02-0001-01 and FUTURE-PRED ANR-18-CE02-0005-01) and was further supported by a grant from the “Ministère français de l’Enseignement supérieur, de la Recherche et de l’Innovation” through the “Ecole Doctorale E2M2” of “Université Claude Bernard Lyon 1”.
Conflict of Interest
The authors declare that the research was conducted in the absence of any commercial or financial relationships that could be construed as a potential conflict of interest.
Acknowledgments
We thank C. Wilmers for providing the code of the published model that serves as the basis of ours. In addition, this work benefited from the computing cluster platform of the Centre d’Ecologie Fonctionnelle et Evolutive and from Biosphere, the IFB cloud for life sciences. We thank Bruno Spataro and Stéphane Delmotte from the LBBE computing services for their help in using computing facilities. Finally, we thank two reviewers for their fruitful comments on a previous draft of this manuscript.
Supplementary Material
The Supplementary Material for this article can be found online at: https://www.frontiersin.org/articles/10.3389/fevo.2020.601202/full#supplementary-material
Footnotes
References
Abrams, P. A., and Ginzburg, L. R. (2000). The nature of predation: prey dependent, ratio dependent or neither? Trends Ecol. Evol. 15, 337–341. doi: 10.1016/S0169-5347(00)01908-X
Atwood, T. C., Gese, E. M., and Kunkel, K. E. (2007). Comparative patterns of predation by cougars and recolonizing wolves in Montana’s madison range. J. Wildl. Manage. 71, 1098–1106. doi: 10.2193/2006-102
Bastille-Rousseau, G., Schaefer, J. A., Peers, M. J. L., Ellington, E. H., Mumma, M. A., Rayl, N. D., et al. (2018). Climate change can alter predator-prey dynamics and population viability of prey. Oecologia 186, 141–150. doi: 10.1007/s00442-017-4017-y
Bayliss, P., and Choquenot, D. (2002). The numerical response: rate of increase and food limitation in herbivores and predators. Philos. Trans. R. Soc. B Biol. Sci. 357, 1233–1248. doi: 10.1098/rstb.2002.1124
Bender, L. C., Lomas, L. A., and Browning, J. (2007). Condition, survival, and cause-specific mortality of adult female mule deer in north-central New Mexico. J. Wildl. Manage. 71, 1118–1124. doi: 10.2193/2006-226
Bonenfant, C., Gaillard, J. M., Coulson, T., Festa-Bianchet, M., Loison, A., Garel, M., et al. (2009). Empirical evidence of density-dependence in populations of large herbivores. Adv. Ecol. Res. 41, 313–357. doi: 10.1016/S0065-2504(09)00405-X
Bourgarel, M., Fritz, H., Gaillard, J. M., De Garine-Wichatitsky, M., and Maudet, F. (2002). Effects of annual rainfall and habitat types on the body mass of impala (Aepyceros melampus) in the Zambezi Valley, Zimbabwe. Afr. J. Ecol. 40, 186–193. doi: 10.1046/j.1365-2028.2002.00377.x
Candolin, U., and Wong, B. B. M. (2012). Behavioural Responses to a Changing World. Oxford: Oxford University Press.
Carbone, C., and Gittleman, J. L. (2002). A common rule for the scaling of carnivore density. Science 295, 2273–2276. doi: 10.1126/science.1067994
Caswell, H. (2001). Matrix Population Models: Construction, Analysis, and Interpretation, 2nd Edn. Massachusetts, MA: Sinauer Associates.
Chamaillé-Jammes, S., and Fritz, H. (2009). Precipitation-NDVI relationships in eastern and southern African savannas vary along a precipitation gradient. Int. J. Remote Sens. 30, 3409–3422. doi: 10.1080/01431160802562206
Cook, J. G., Johnson, B. K., Cook, R. C., Riggs, R. A., Delcurto, T., Bryant, L. D., et al. (2004). Effects of summer-autumn nutrition and parturition date on reproduction and survival of Elk. Wildl. Monogr. 155, 1–61.
Cooper, S. M. (1990). The hunting behaviour of spotted hyaenas (Crocuta crocuta) in a region containing both sedentary and migratory populations of herbivores. Afr. J. Ecol. 28, 131–141. doi: 10.1111/j.1365-2028.1990.tb01145.x
East, R. (1984). Rainfall, soil nutrient status and biomass of large African savanna mammals. Afr. J. Ecol. 22, 245–270. doi: 10.1111/j.1365-2028.1984.tb00700.x
Eberhardt, L. L. (1977). Optimal policies for conservation of large mammals, with special reference to marine ecosystems. Environ. Conserv. 4, 205–212. doi: 10.1017/S0376892900025819
Elbroch, L. M., Lendrum, P. E., Newby, J., Quigley, H., and Craighead, D. (2013). Seasonal foraging ecology of non-migratory cougars in a system with migrating prey. PLoS One 8, 1–14. doi: 10.1371/journal.pone.0083375
Elliott, J. P., Cowan, I. M., and Holling, C. S. (1977). Prey capture by the African lion. Can. J. Zool. 55, 1811–1828. doi: 10.1139/z77-235
Estes, R. (1991). The Behavior Guide to African Mammals: Including Hoofed Mammals, Carnivores, Primates. California, CA: University of California Press.
FitzGibbon, C. D., and Fanshawe, J. H. (1989). The condition and age of Thomson’s gazelles killed by cheetas and wild dogs. J. Zool. 218, 99–107. doi: 10.1111/j.1469-7998.1989.tb02528.x
Foley, C., Pettorelli, N., and Foley, L. (2008). Severe drought and calf survival in elephants. Biol. Lett. 4, 541–544. doi: 10.1098/rsbl.2008.0370
Forchhammer, M. C., Stenseth, N. C., Post, E., and Langvatn, R. (1998). Population dynamics of Norwegian red deer: density-dependence and climatic variation. Proc. R. Soc. B Biol. Sci. 265, 341–350. doi: 10.1098/rspb.1998.0301
Funston, P. J., Mills, M. G. L., and Biggs, H. C. (2001). Factors affecting the hunting success of male and female lions in the Kruger National Park. J. Zool. 253, 419–431. doi: 10.1017/S0952836901000395
Grange, S., Barnier, F., Duncan, P., Gaillard, J. M., Valeix, M., Ncube, H., et al. (2015). Demography of plains zebras (Equus quagga) under heavy predation. Popul. Ecol. 57, 201–214. doi: 10.1007/s10144-014-0469-7
Holling, C. S. (1959). The components of predation as revealed by a study of small-mammal predation of the european pine sawfly. Can. Entomol. 91, 293–320. doi: 10.4039/Ent91293-5
Holt, R. D. (2008). Theoretical perspectives on resource pulses. Ecology 89, 671–681. doi: 10.1890/07-0348.1
Hopcraft, G. J. C., Sinclair, A. R. E., and Packer, C. (2005). Planning for success: serengeti lions seek prey accessibility rather than abundance. J. Anim. Ecol. 74, 559–566. doi: 10.1111/j.1365-2656.2005.00955.x
Husseman, J. S., Murray, D. L., Power, G., Mack, C., Wenger, C. R., and Quigley, H. (2003). Assessing differential prey selection patterns between two sympatric large carnivores. Oikos 101, 591–601. doi: 10.1034/j.1600-0706.2003.12230.x
Inouye, D. W., Barr, B., Armitage, K. B., and Inouye, B. D. (2000). Climate change is affecting altitudinal migrants and hibernating species. Proc. Natl. Acad. Sci. U.S.A. 97, 1630–1633. doi: 10.1073/pnas.97.4.1630
IPCC, (2014a). Climate Change 2014: Impacts, Adaptation, and Vulnerability. Part B: Regional Aspects. Contribution of Working Group II to the Fifth Assessment Report of the Intergovernmental Panel on Climate Change. Cambridge: Cambridge University Press, 688.
Lone, K., Loe, L. E., Gobakken, T., Linnell, J. D. C., Odden, J., Remmen, J., et al. (2014). Living and dying in a multi-predator landscape of fear: roe deer are squeezed by contrasting pattern of predation risk imposed by lynx and humans. Oikos 123, 641–651. doi: 10.1111/j.1600-0706.2013.00938.x
Loveridge, A. J., Hunt, J. E., Murindagomo, F., and Macdonald, D. W. (2006). Influence of drought on predation of elephant (Loxodonta africana) calves by lions (Panthera leo) in an African wooded savannah. J. Zool. 270, 523–530. doi: 10.1111/j.1469-7998.2006.00181.x
Maclean, I. M. D., and Wilson, R. J. (2011). Recent ecological responses to climate change support predictions of high extinction risk. Proc. Natl. Acad. Sci. U.S.A. 108, 12337–12342. doi: 10.1073/pnas.1017352108
Martay, B., Brewer, M. J., Elston, D. A., Bell, J. R., Harrington, R., Brereton, T. M., et al. (2017). Impacts of climate change on national biodiversity population trends. Ecography 40, 1139–1151. doi: 10.1111/ecog.02411
Mduma, S. A. R., Sinclair, A. R. E., and Hilborn, R. (1999). Food regulates the Serengeti wildebeest: a 40-year record. J. Anim. Ecol. 68, 1101–1122. doi: 10.1046/j.1365-2656.1999.00352.x
Mech, D. L., Smith, D. W., Murphy, K. M., and MacNulty, D. R. (2001). Winter severity and wolf predation on a formerly wolf- free elk herd. J. Wildl. Manage. 65, 998–1003. doi: 10.2307/3803048
Mech, L. D., Frenzel, D. J., and Karns, P. D. (1971). “The effect of snow conditions on the vulnerability of white-tailed deer to wolf predation,” in Ecological Studies of the Timber Wolf in Northeastern Minnesota, eds L. D. Mech and D. J. Frenzel, 51–59. (Minnesota: North Central Forest Experimental Station) USDA Forest Service Research Paper NC-52.
Mech, L. D., Smith, D. W. and MacNulty, D. R. (eds.) (2015). “Introduction the wolf as a killing machine,” in Wolves on the Hunt the Behavior of Wolves Hunting Wild Prey (Chicago: The University of Chicago Press), 1–9.
Melis, C., Jȩdrzejewska, B., Apollonio, M., Bartoñ, K. A., Jȩdrzejewski, W., Linnell, J. D. C., et al. (2009). Predation has a greater impact in less productive environments: variation in roe deer, Capreolus capreolus, population density across Europe. Glob. Ecol. Biogeogr. 18, 724–734. doi: 10.1111/j.1466-8238.2009.00480.x
Metz, M. C., Smith, D. W., Vucetich, J. A., Stahler, D. R., and Peterson, R. O. (2012). Seasonal patterns of predation for gray wolves in the multi-prey system of Yellowstone National Park. J. Anim. Ecol. 81, 553–563. doi: 10.1111/j.1365-2656.2011.01945.x
Mills, M. G. L. (1995). Notes on wild dog Lycaon pictus and lion Panthera leo population trends during a drought in the Kruger National Park. Koedoe 38, 95–99. doi: 10.4102/koedoe.v38i1.309
Moyes, K., Nussey, D. H., Clements, M. N., Guinness, F. E., Morris, A., Morris, S., et al. (2011). Advancing breeding phenology in response to environmental change in a wild red deer population. Glob. Chang. Biol. 17, 2455–2469. doi: 10.1111/j.1365-2486.2010.02382.x
Mysterud, A., Stenseth, N. C., Yoccoz, N. G., Langvatn, R., and Steinheim, G. (2001). Nonlinear effects of large-scale climatic variability on wild and domestic herbivores. Nature 410, 1096–1099. doi: 10.1038/35074099
Ogutu, J. O., Piepho, H.-P., Dublin, H. T., Bhola, N., and Reid, R. S. (2008). Rainfall influences on ungulate population abundance in the Mara-Serengeti ecosystem. J. Anim. Ecol. 77, 814–829. doi: 10.1111/j.1365-2656.2008.01392.x
Owen-Smith, N. (1990). Demography of a large herbivore, the greater kudu Tragelaphus strepsiceros, in relation to rainfall. J. Anim. Ecol. 59, 893–913. doi: 10.2307/5021
Owen-Smith, N. (2008). Changing vulnerability to predation related to season and sex in an African ungulate assemblage. Oikos 117, 602–610. doi: 10.1111/j.0030-1299.2008.16309.x
Owen-Smith, N., and Mills, M. G. L. (2008a). Predator-prey size relationships in an African large-mammal food web. J. Anim. Ecol. 77, 173–183. doi: 10.1111/j.1365-2656.2007.01314.x
Owen-Smith, N., and Mills, M. G. L. (2008b). Shifting prey selection generates contrasting herbivore dynamics within a large-mammal predator-prey web. Ecology 89, 1120–1133. doi: 10.1890/07-0970.1
Owen-Smith, N. (2002). Adaptive Herbivore Ecology from Resources to Populations in Variable Environments. Cambridge: Cambridge University Press, doi: 10.1017/CBO9780511525605
Pacifici, M., Visconti, P., Butchart, S. H. M., Watson, J. E. M., Cassola, F. M., and Rondinini, C. (2017). Species’ traits influenced their response to recent climate change. Nat. Clim. Chang. 7, 205–208. doi: 10.1038/nclimate3223
Parmesan, C., Ryrholm, N., Stefanescu, C., Hill, J. K., Thomas, C. D., Descimon, H., et al. (1999). Poleward shifts in geographical ranges of butterfly species associated with regional warming. Nature 399, 579–583. doi: 10.1038/21181
Pole, A., Gordon, I. J., Gorman, M. L., and MacAskill, M. (2004). Prey selection by African wild dogs (Lycaon pictus) in southern Zimbabwe. J. Zool. 262, 207–215. doi: 10.1017/S0952836903004576
Post, E., Peterson, R. O., Stenseth, N. C., and McLaren, B. E. (1999). Ecosystem consequences of wolf behavioural response to climate. Nature 401, 905–907. doi: 10.1038/44814
Post, E., and Stenseth, N. C. (1999). Climatic variability, plant phenology, and northern ungulates. Ecology 80, 1322–1339. doi: 10.1890/0012-9658(1999)080[1322:CVPPAN]2.0.CO;2
Radloff, F. G. T., and Du Toit, J. T. (2004). Large predators and their prey in a southern African savanna: a predator’s size determines its prey size range. J. Anim. Ecol. 73, 410–423. doi: 10.1111/j.0021-8790.2004.00817.x
R Core Team (2020). R: A Language and Environment for Statistical Computing. Vienna: R Foundation for Statistical Computing.
Rutherford, M. C. (1980). Annual plant production precipitation relations in arid and semi-arid regions. S. Afr. J. Sci. 76, 53–57.
Saether, B.-E. (1997). Environmental stochasitcity and population dynamics of large herbivores: a search for mechanisms. Trends Ecol. Evol. 12, 143–149. doi: 10.1016/S0169-5347(96)10068-9
Saether, B.-E., and Gravem, A. J. (1988). Annual variation in winter body condition of Norwegian moose calves. J. Wildl. Manage. 52, 333–336. doi: 10.2307/3801245
Salnicki, J., Teichmann, M., and Wilson, V. J. and Murindagomo, F. (2001). Spotted hyaenas Crocuta crocuta prey on new-born elephant calves in Hwange National Park, Zimbabwe. Koedoe 44, 79–83. doi: 10.4102/koedoe.v44i2.177
Sankaran, M., Hanan, N. P., Scholes, R. J., Ratnam, J., Augustine, D. J., Cade, B. S., et al. (2005). Determinants of woody cover in African savannas. Nature 438, 846–849. doi: 10.1038/nature04070
Schaller, G. B. (1972). The Serengeti Lion A study of Predator-Prey Relations. Chicago: The University of Chicago Press.
Stenseth, N. C., Shabbar, A., Chan, K., Boutin, S., Rueness, E. K., Ehrich, D., et al. (2004). Snow conditions may create an invisible barrier for lynx. Proc. Natl. Acad. Sci. U.S.A. 101, 10632–10634. doi: 10.1073/pnas.0308674101
Visser, M. E., Holleman, L. J. M., and Gienapp, P. (2006). Shifts in caterpillar biomass phenology due to climate change and its impact on the breeding biology of an insectivorous bird. Oecologia 147, 164–172. doi: 10.1007/s00442-005-0299-6
Wilmers, C. C., Post, E., and Hastings, A. (2007a). A perfect storm: the combined effects on population fluctuations of autocorrelated environmental noise, age structure, and density dependence. Am. Nat. 169, 673–683. doi: 10.1086/513484
Wilmers, C. C., Post, E., and Hastings, A. (2007b). The anatomy of predator-prey dynamics in a changing climate. J. Anim. Ecol. 76, 1037–1044. doi: 10.1111/j.1365-2656.2007.01289.x
Keywords: population dynamics, traits, vulnerability, climate changes, predator–prey interactions
Citation: Morin A, Chamaillé-Jammes S and Valeix M (2021) Climate Effects on Prey Vulnerability Modify Expectations of Predator Responses to Short- and Long-Term Climate Fluctuations. Front. Ecol. Evol. 8:601202. doi: 10.3389/fevo.2020.601202
Received: 31 August 2020; Accepted: 31 December 2020;
Published: 22 January 2021.
Edited by:
Johan T. du Toit, Utah State University, United StatesReviewed by:
David Iles, Canadian Wildlife Service, Environment and Climate Change Canada, CanadaRyan Wilson, U.S. Fish and Wildlife Service, United States
Copyright © 2021 Morin, Chamaillé-Jammes and Valeix. This is an open-access article distributed under the terms of the Creative Commons Attribution License (CC BY). The use, distribution or reproduction in other forums is permitted, provided the original author(s) and the copyright owner(s) are credited and that the original publication in this journal is cited, in accordance with accepted academic practice. No use, distribution or reproduction is permitted which does not comply with these terms.
*Correspondence: Aïssa Morin, morinaissa@gmail.com